- 1CARRTEL (UMR 042), Université Savoie Mont Blanc, Le Bourget du Lac, France
- 2Laboratoire de Mathématiques de Besançon – UMR 6623 Centre National de la Recherche Scientifique, Université de Bourgogne – Franche-Comté, Besançon, France
- 3Laboratoire Chrono-Environnement – UMR 6249 Centre National de la Recherche Scientifique, Université de Bourgogne – Franche-Comté, Besançon, France
- 4Ecological Systems Laboratory, Ecole Polytechnique Fédérale de Lausanne, Lausanne, Switzerland
A number of methods have recently been developed to identify early warning signals (EWSs) within time-series structure typically characteristic of the rise of critical transitions. Inherent technical constraints often limit the possibility to obtain from sediment both regular and high-resolution time series rather most palaeoecological time series obtained from sediment records represent time-aggregated ecological signals. In this study, the robustness of EWS detection to temporal aggregation was addressed using simulated time series mimicking ecological dynamics. Using a stochastic differential equation based on a deterministic model exhibiting a critical transition between two stable equilibria, two different scenarios were simulated using different combinations of forcing and noise intensities (critical slowing-down and driver-mediated flickering scenarios). The temporal resolution of each simulated time series was progressively decreased by averaging the data from Δt = 1 up to Δt = 10 time-unit intervals. EWSs [standard deviation, autocorrelation at lag-1 (AR(1)), skewness and kurtosis] were applied to all time series. Robustness of EWSs to data aggregation was assessed through a block-based approach using Kendall rank correlation Tau. Standard deviation appeared to be robust to data aggregation up to Δt = 10 for the slowing-down scenario and up to Δt = 5 for the driver-mediated flickering scenario while autocorrelation remained robust up to Δt = 2 for the slowing-down scenario and did not support data aggregation for the driver-mediated scenario. Skewness and kurtosis performed poorly for the two scenarios and were not considered as robust EWSs even for the original simulated time series using the block-based approach. Our results suggest that high-resolution palaeoecological time series could be in a large extent suitable to support EWS analyses.
Introduction
Since the Industrial Revolution, the enhancement of anthropogenic pressures worldwide led to drastic changes in ecosystem functioning (Estes et al., 2011) and species dynamics (Anderson et al., 2008). Ecosystems are complex adaptive systems and species exhibit a large number of biotic and abiotic interactions, their responses to forcings can be linear and progressive but also nonlinear, exhibiting rapid, and abrupt changes (Scheffer et al., 2001, 2012; Dakos et al., 2015). Such catastrophic shifts are related to the existence of multiple attractors and fold bifurcations in response to slow variations in forcing variables (May, 1977; Seekell et al., 2013). In many cases, the human-induced regime shifts of ecosystems or species trigger important alterations of ecosystem services (Folke et al., 2004; Millennium Ecosystem Assessment, 2005). As a consequence, over the last decade, a growing body of research (Scheffer et al., 2001, 2009, 2012; Dakos et al., 2008, 2015; Carpenter et al., 2011) has been developed to define indicators of the rising of a critical transition. Various indicators or early warning signals (EWSs) have been proposed to anticipate such regime shifts (reviewed in Scheffer et al., 2012). The basic assumption is that the structure of the time series of a state variable differs whether the system is close to or far away from an attractor within the attraction basin. EWS methods have been successfully used to identify changes in time series prior of regime shift in various contexts such as climate change (Dakos et al., 2008; Lenton et al., 2012), lakes (Carpenter et al., 2011; Wang et al., 2012), plankton (Veraart et al., 2012) and zooplankton (Drake and Griffen, 2010). Nonetheless, time series required to seek for EWS should be long and recorded at high-resolution. For instance, Dakos et al. (2012a) argued that the frequency of the observations should be higher than the characteristic rate of change of the state variable in the considered dynamic system. This often limits the possibility of using EWS methods to ecological time series and especially palaeoecological time series, which usually have lower temporal resolution than the genuine ecological dynamics it is supposed to describe.
Sediment records have commonly been used to obtain long-term time series of ecosystem's state variables (Dakos et al., 2008) or species (Caswell and Frid, 2013). Spanning specific periods of interest (e.g., late Glacial transition, Little Ice Age, Industrial Revolution), the use of EWSs on reconstructed dynamics would greatly improve our ability to better assess resilience and stability of species or ecosystems. Unfortunately, finest time resolution (i.e., 1–2 years) is seldom to be achieved due to limited or changing sedimentation rate as well as bioturbation that can induce errors in the relative age estimates between successive samples. Interpolation has been regarded has a mean to deal with unevenly spaced empirical time series (e.g., Dakos et al., 2008). Nonetheless, interpolation can spuriously alter the statistical properties of time series (Carstensen et al., 2013). As an alternative, the robustness of EWSs to temporal upscaling or data aggregation may be questioned. What is the lower temporal resolution of data needed for a consistent EWS analysis? Lenton et al. (2012) addressed the effect of data aggregation on their GENIE-2 simulations over different time scales and identify significant effects on the results (specifically temporal autocorrelation) whereas Carstensen et al. (2013) questioned a possible influence of the temporal resolution on EWS detection.
This study aims at assessing the effect of temporal resolution on EWS detection using simulated time series. Specific questions that motivated this study were: (i) To what extent EWS patterns remain consistent after data aggregation? (ii) Is the influence of data aggregation similar when dealing with different kinds of regime shift scenarios?
Four hundred time series were simulated from a stochastic version of the model of Ludwig et al. (1978) using two different parameterizations (details below). This model, widely used in ecological modeling (e.g., May, 1977; Dakos et al., 2012a), exhibits alternative stable states and finely matches abrupt shifts in ecosystem states or in species abundances. Species dynamics greatly differed among species especially due to differences among body size (Yodzis and Innes, 1992). Therefore, to cope with these intrinsic differences, two intensities of Gaussian white noise (i.e., high and low) were used (i) to enable the EWS detection, (ii) to match with patterns found in real data. The different combinations of noise and forcing rate of change aimed at reproducing patterns mostly reported for ecological dynamics prior to a critical transition, namely slowing-down or flickering. Slowing-down is characterized by a slower recovery of the system as long as the forcing variable increases in a context of low noise level (Dakos et al., 2008; Lenton et al., 2012). The flickering phenomenon is characterized by a high noise level which triggers switch of the system state between two basins of attraction due to relatively large disturbances (Guttal and Jayaprakash, 2007; Dakos et al., 2013). In this case, a critical transition can be due to both deterministic and stochastic processes.
Methods
Alternative Stable State Model
The model used to simulate time series is a stochastic differential Equation (1) derived from the deterministic model of Ludwig et al. (1978). The first part of the model provides the classical logistic growth of a population X with maximum per-capita growth rate r and carrying capacity K. For all simulations, K = 10 and r = 1. The second part of the model represents a harvest function following a type-III S-shaped functional response (Holling, 1959) mimicking nonlinear relationship between a forcing and a response variable (often between a consumer and its resource). The harvest function was retained as it corresponds to a large number of interactions among aquatic species (Morozov, 2010). Ecologically, speaking, c expresses the extent of the forcing intensity or saturation level of the predator for which no more prey can be consumed and h represents a switching value that indicates the scale at which predators switch on new preys under learning process. Tuning this model for K = 10 allows producing alternative stable state scenarios intersected by a critical transition (May, 1977). For all analyses, c (hereafter forcing intensity) linearly increased (Table 1), and h was set constant at 1. Noise consisted in Gaussian white (Wiener) noise dW centered on 0 with a constant standard deviation (σ, hereafter noise intensity) and was introduced in the model to take into account random variations in forcing pressure. The stochastic equations were simulated using Euler discretization and solved with Ito stochastic calculus (Horsthemke and Lefever, 1984).
Adjusting values for both forcing and noise intensities (c and σ, respectively) led to simulate two different scenarios of time series that mimic different possible scenarios matching empirical time series (Table 1). The “slowing-down scenario” was obtained considering a slow linear increase for the forcing variable c and a low and constant amount of noise σ. According to Dakos et al. (2013), the flickering behavior is characterized by a high amount of noise and is thought to express highly stochastic dynamics compared to slowing-down scenario. Therefore, in the “driver-mediated flickering” scenario the forcing variable c increases also linearly with time but a higher constant level of noise is applied. For the slowing-down scenario, the low noise level was adjusted to trigger state shift very close to the actual fold bifurcation (Dakos et al., 2013). By contrast, in the flickering scenario the higher amount of noise was set so as the system could flip from one state to another with forcing facilitation (driver-mediated) (Dakos et al., 2013). For each scenario, 200 time series were simulated.
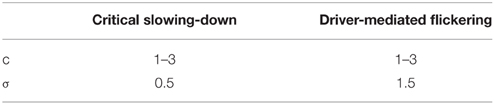
Table 1. Characteristics of forcing (c) and noise intensities with σ representing standard deviation used in the two different scenarios.
Time Series Aggregation
The process of aggregation of time series considered all full-length time series simulated. The process produced surrogate time series for which temporal resolution ranges from 1 to 10 time units. Explicitly, for the aggregated time series at Δt = 2 time units, each two consecutive values were averaged. Similarly, for Δt = 3 time units, each three consecutive values were averaged and so on until Δt = 10 time units. The range of temporal aggregation, from 1 to 10 time units, was retained to match the usual time intervals usually reached in high-resolution palaeolimnological studies (e.g., Frossard et al., 2013; Millet et al., 2014).
Early Warning Signals
A large array of EWS methods has been proposed over the last years to detect change in time series structure prior to a critical transition. Four indicators were used in the analyses. (i) The standard deviation (SD) of the state variable X is expected to increase due to wider fluctuations in the system when approaching a tipping point as a consequence of lower recovery rate following disturbances, entraining the system far from the center of the basin of attraction. (ii) The temporal autocorrelation was considered through the autoregressive coefficient of an autoregressive model of order 1 fitted using conditional least-squares method (AR(1)). AR(1) is also expected to increase as the system approaches a critical transition because fluctuations become larger, implying that the successive data become progressively more similar and therefore autocorrelated. (iii) Skewness and (iv) kurtosis, respectively the standardized third and fourth moments around the mean of a distribution of a X were also considered. These two indicators highlight how, as the system reaches a critical transition, it exhibits slower recovery and higher proportion of extreme values. Different patterns can be produced by these two indicators whether the next alternative stable state is lower or higher than the previous one.
All time series were split in two parts using constrained hierarchical clustering based on Euclidean distance between successive time steps to obtain the portions of the simulated time series prior to critical transition on which the analyses were conducted. These portions were then adjusted to the shortest time series in order to compare EWS patterns and detrended using Gaussian kernels (Dakos et al., 2012a).
The use of a moving sliding window can introduce statistical dependence among EWSs that biases statistical estimates of trends and may limit our ability to detect true alarms (Boettiger and Hastings, 2012). To overcome this issue we used a block-based approach similarly to Wouters et al. (2015) to compute independent EWSs. Time series from the critical slowing-down scenario were restricted to 300 time steps (i.e., the shortest length for driver-mediated scenario) so that each block for the two scenarios had equal number of observations. Time series were split in 10 blocks among which Gaussian detrending was applied followed by EWS computation within each block. The length of the time series (300 time steps) could not support aggregation for higher numbers of blocks and restricted EWS computation for aggregation level above Δt = 10. In the context of this study, it can be considered among the longer ecological time series that can be obtained. Kendall rank correlation Tau then was used to assess the value and the significance of EWS pattern using the block-based approach.
Simulations from the stochastic differential equation were produced with Scilab 5.5.0 (Scilab Enterprises S.A.S). Data aggregation and statistical analyses were performed using R 3.2.0 (R Core Team Development, 2015) as well as the packages “earlywarnings” (Dakos et al., 2012a), “vegan” (Oksanen et al., 2015) and “rioja” (Juggins, 2014).
Results
Two specific scenario patterns were obtained using different combinations of c and σ (Table 1, Figure 1). Simulated time series were split at the onset of the tipping points using constrained cluster analysis and sections of the time series prior the tipping points were retained to produce consistent EWS comparison for the slowing-down and driver-mediated flickering scenarios. Patterns for the different EWSs were then assessed using Kendall correlations.
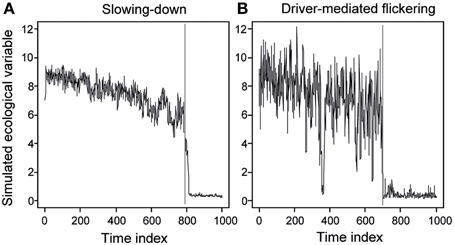
Figure 1. Examples of single time series simulated from Equation (1) producing different patterns according to variations in c and σ. (A) represents a slowing-down scenario for which c increases over time with a low level of noise (small σ). (B) refers to a driver-mediated flickering scenario for which c increases over time with a high level of noise (high σ). The gray vertical lines indicate the time at which an observed bifurcation occurs toward a lower alternative stable state using the constrained hierarchical clustering based on Euclidian distance.
EWS Patterns
For each of the two scenarios, the 200 simulated time series allowed accounting for particular behavior of time series due to the stochastic part in Equation (1). Visual inspection indicated that patterns differed among EWSs and that the effect of data aggregation varied both among EWS and scenarios (Figures 2, 3).
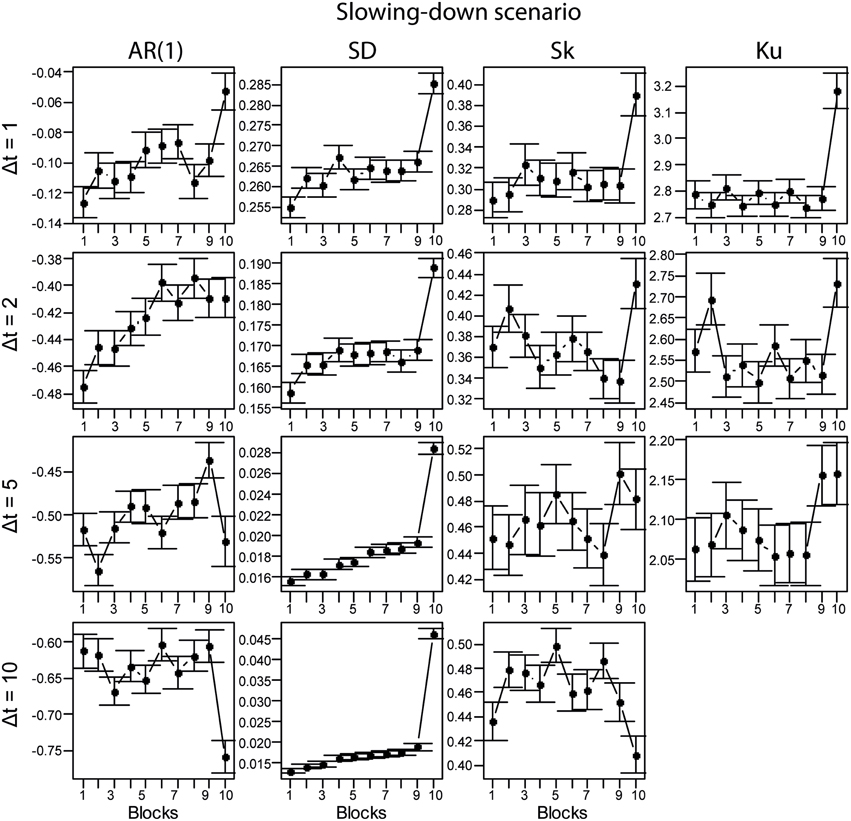
Figure 2. Patterns of four EWSs for the slowing-down scenario from 200 simulated time series using the block-based approach. Time aggregation ranged from Δt = 1 to Δt = 10. Black circles associated with whiskers represent mean values and standard errors of EWSs. As time scale is reversed, the transition occurs at the end of the 10th block. AR(1), autocorrelation; SD, standard deviation; Sk, skewness; Ku, kurtosis. Kurtosis could not be computed for aggregation Δ = 10 because of the lack of data for each block (n = 3) for this aggregation level.
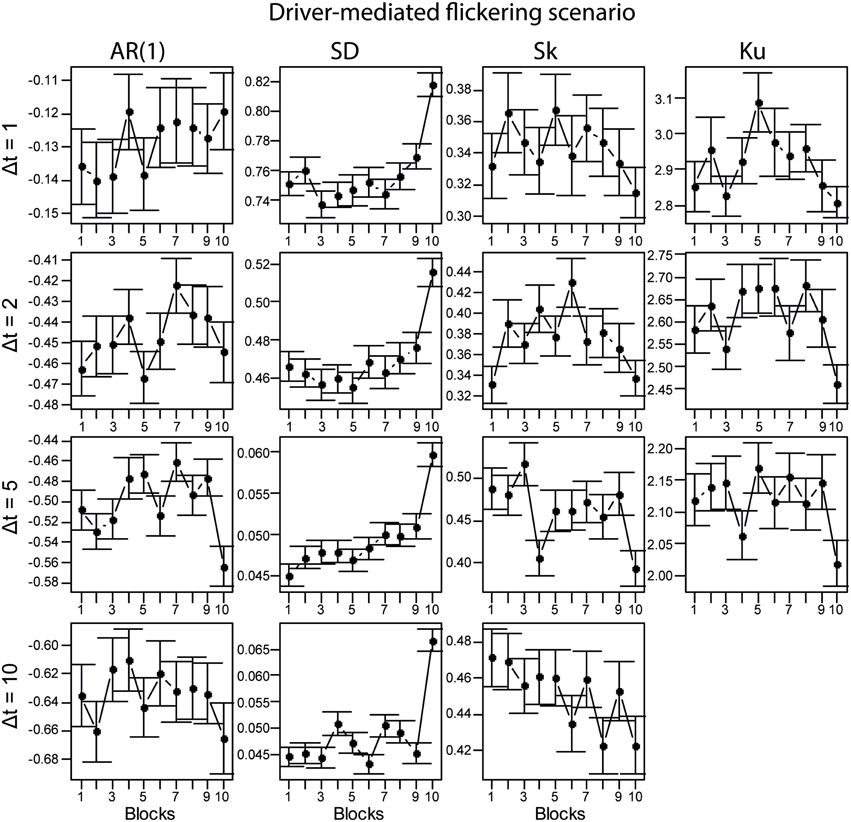
Figure 3. Patterns of four EWSs for the driver-mediated flickering scenario from 200 simulated time series using the block-based approach. Time aggregation ranged from Δt = 1 to Δt = 10. Black circles associated with whiskers represent mean values and standard errors of EWSs. As time scale is reversed, the transition occurs at the end of the 10th block. AR(1), autocorrelation; SD, standard deviation; Sk, skewness; Ku, kurtosis. Kurtosis could not be computed for aggregation Δ = 10 because of the lack of data for each block (n = 3) for this aggregation level.
For the slowing-down scenario (Figure 2), increasing patterns of autocorrelation can be identified at aggregation level of Δt = 1 and Δt = 2. At higher aggregation levels, patterns are flattened and even exhibit a decrease in autocorrelation in the last block, especially at Δt = 10. Differently, standard deviation exhibited unambiguous increasing patterns over the range of aggregation. Its variability among simulations within each block decreased as aggregation increases, which is likely related to our data averaging process. Standard deviation drastically increases in the 10th block, the last sequence before the transition. The time series we used did not integrate data after the transition. Therefore, although an increase in standard deviation can be informative between successive blocks, the last sequence prior to the transition seems to clearly indicate the imminence of the transition. Skewness and kurtosis were mostly characterized by rather flat or decreasing trends at least up to the 8th block. As for standard deviation, these two EWS increased drastically in the last 10th block up to the data aggregation of Δt = 2. Although, it seems to effectively indicate the rise of the transition, the lack of previous trends limit greatly their ability of anticipate the critical transition in this scenario.
For the driver-mediated flickering scenario, the highest noise intensity compared to the slowing-down scenario produced EWS patterns that were differently conserved over the aggregation range considered (Figure 3). For autocorrelation, the within-block variability was rather high as indicated by the large standard error bars. Increasing patterns were not pronounced, except for Δt = 1. A decrease in autocorrelation within the last two blocks occurs up to Δt = 2. Differently for standard deviation, increasing trends associated with a marked increase in the last block were conserved up to Δt = 5. At Δt = 10, although the 10th block exhibited clearly a high standard deviation, trend prior the 10th block was absent. Neither, skewness nor kurtosis exhibited increasing trends over any level of aggregation for the driver-mediated scenario. In a majority of cases, these two EWS tented to decrease over the last three blocks at aggregation level up to Δt = 2.
Robustness of EWS to Aggregation
Important differences were found regarding EWS robustness to data aggregation. For standard deviation, Kendall Tau was significantly positive for the slowing-down scenario over the range of aggregation. It increased from ~0.5 to ~1 from Δt = 1 to Δt = 10, indicating strong increasing pattern when approaching the critical transition (Figure 4). For the driver-mediated scenario, the Kendall Tau of the standard deviation was still positive but close to significance at Δt = 1 and significant at Δt = 2 and Δt = 5 (Figure 4). Kendall correlations for temporal autocorrelation tended to decrease over the range of data aggregation but were high for the low aggregation level at ~0.5. They remain significant up to Δt = 2 for the slowing-down scenario and were close to significance for the driver-mediated scenario only at Δt = 1 (Figure 4). Skewness and kurtosis did not exhibited significant trends even among the original simulated time series (Figure 4). The Kendall Tau tended to decrease with date aggregation as expected from the patterns shown in Figures 2, 3. This decreasing pattern becoming more pronounced as data aggregation increases, it can explain the concomitant decrease of the p-value of Kendall Tau, especially for skewness in the driver-mediated scenario. For skewness and kurtosis trends tended to decrease with data aggregation. This counterintuitive result could be due to the smaller sample size in blocks over aggregation steps. Nonetheless, Kendall Tau was negative, contrary to our primary expectations. Based on our primary expectations of a positive and significant Kendall Tau, these two EWSs did not meet the required criteria at any level of aggregation to inform about the rising of a critical transition. The robustness of EWSs, assessed using significance of Kendall Tau from the block-based approach, is summarized in Table 2.
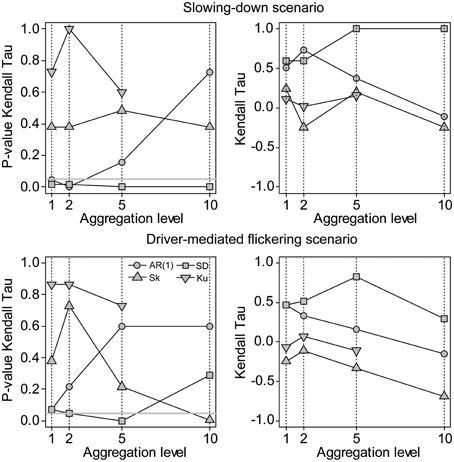
Figure 4. Effect of data aggregation on the detection of four EWS patterns for the two scenarios using the block-based approach. Top: slowing-down scenario. Bottom: driver-mediated flickering scenario. For both scenarios, left panels show p-values and right panels show the estimates of Kendall rank correlation for mean EWS patterns obtained from 200 simulated time series. The gray lines indicate the threshold of P = 0.05 under which correlations are considered significant. AR(1), autocorrelation; SD, standard deviation; Sk, skewness; Ku, kurtosis. Kurtosis could not be computed for aggregation Δ = 10 because of the lack of data for each block (n = 3) for this aggregation level.
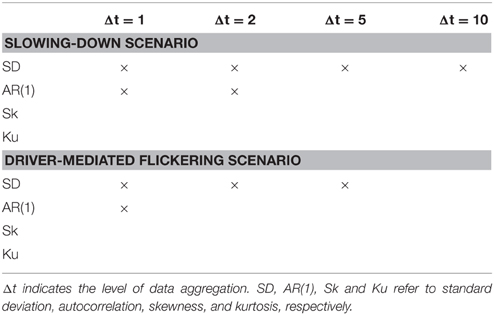
Table 2. Summary of the robustness of EWSs to data aggregation for the two scenarios according to significance of Kendall Tau using the block-based approach.
Discussion
The expanding use of time series obtained from sediment records to address current ecological threats and long-term ecosystem dynamics progressively reconcile palaeoecology and neoecology. A shared time continuum recently described by Rull (2014) identifies time scales that can be retrieved from lacustrine archives to reconstruct high-resolution, ecologically relevant dynamics. The unprecedented increase of anthropogenic forcings ongoing since the beginning of the twentieth century (Anthropocene era; Steffen et al., 2011) deeply altered most of natural ecosystems and their associated species fitness leading to possible massive ecosystem or species collapses. In this context, seeking for EWSs that could inform for the rise of critical regime shifts among times series obtained from lake sediments is likely to be a future important demand of conservationists and practitioners to palaeoecologists in a changing environment. Nevertheless, detectability and reliability of EWSs using palaeoecological data were remaining to be tested to develop with confidence their application to a wide array of ecological variables that can be reached from sediment archives. Justifications of model characteristics will be first presented, before comparing EWS advantages in different time series scenarios. The robustness of the EWSs is then discussed prior to provide ecological considerations for ecosystem and species dynamics that could be subjected to EWS analyses.
Model Choice and Parameterization
Various model formulations exhibiting alternative stable states associated with catastrophic regime shifts have been proposed (e.g., May, 1977; Carpenter et al., 1999). The current model was considered because of its long use in theoretical ecology and especially in recent EWS studies. Moreover, its simplicity allows obtaining highly different scenarios only by varying two parameters (c and σ) related to strong ecological assumptions (forcing intensity, noise on the forcing). Directionality of shift can influence the EWS patterns (Dakos et al., 2012b). For instance, critical slowing-down can be especially detected when the system shifts to basins of attraction from high to low values and more hardly from low to high values. Hence, the model retained was expected to be especially suitable to identify EWS patterns for the different simulated scenarios. In the model formulation, the stochastic component was set to be multiplicative. Although multiplicative noise has been shown to possibly produce spurious patterns leading to difficulties to identify critical transition (Dakos et al., 2012b), this kind of noise (white Gaussian noise applied on c) prevents the occurrence of unrealistic negative results during the simulations especially in a context of important noise.
Early Warning Patterns
The patterns of EWS prior to bifurcation differed among the two studied scenarios. Standard deviation, autocorrelation increased over time prior to the observed bifurcation point for both scenarios. Our results for the driver-mediated flickering scenario differed from those of Dakos et al. (2013) who used a different model (Carpenter et al., 1999) for which standard deviation and autocorrelation decreased prior to the theoretical critical transition. These authors suggested that a decrease in system's sensitivity to forcings or a reduction of the system's ability to follow high-frequency variations in the environment could explain this pattern. The difference with these results could also be attributable to the fact that we considered only portions of the simulated time series prior the observed critical transition whereas Dakos et al. (2013) accounted the ones that encompass the critical transitions. Nonetheless, by considering time series up to the bifurcation point, we could show that, at least standard deviation and autocorrelation showed an increasing pattern prior a critical transition therefore supporting consistent patterns for the two scenarios. Opposite alternative attractors between the models of Carpenter et al. (1999) and May (1977) as well as the use of portions of time series prior the critical transition are likely to explain the failure to detect clear patterns for skewness whereas Dakos et al. (2013) reported decreasing patterns. Clear patterns for kurtosis were also almost absent prior to observed bifurcation point for the two scenarios suggesting that these two latter EWSs should be considered with caution to identify the rise of a fold bifurcation.
Early Warning Robustness
The robustness of EWSs to data aggregation was addressed using a block-based approach as recently developed by Wouters et al. (2015) in order to produce independent EWS estimates and prevent overestimation of the ability of EWS signal to identify effective rise of a critical transition (Boettiger and Hastings, 2012). Our analyses revealed clear influence of data aggregation on EWSs, in line with Lenton et al. (2012) who identified a significant influence of data aggregation on temporal autocorrelation for the GENIE-2 simulations. Dakos et al. (2012b) addressed the robustness of the standard deviation and AR(1) on a similar model than ours but in a different perspective. They showed that disturbances had different effects when acting on different parameters of the model. Important differences were found among the robustness of EWS to data aggregation (Table 2). Standard deviation was more robust to data aggregation than autocorrelation for the two scenarios considered although is decreased from Δt = 10 for the slowing-down to Δt = 5 for the driver-mediated flickering. Autocorrelation was less robust to data aggregation by conserving increasing trends for the slowing-down scenario up to Δt = 2 and only at Δt = 1 for the driver-mediated flickering. The higher robustness of standard deviation could originate from the different structure of the aggregated time series compared to the original one. It was likely to be due to the aggregation that merged successive high (or low) data (autocorrelation at lag-1 altered) whereas it can conserve the relative variability structure of the time series. This finding reinforces the interest of standard deviation as generic EWS for different scenarios thriving to a critical transition. Neither, skewness nor kurtosis met the criteria to be robust EWS at any level of data aggregation even for the original time series. Therefore, these two EWSs should be used with caution when applied to ecological time series because different patterns could be expected depending on the structure of the data. As a consequence, the use of a combination of EWSs is suggested to be needed to estimate confidently an approaching regime shift as well as to help at distinguishing between the different possible structures of palaeoecological time series (slowing-down vs. flickering) especially if directionality and extent of environmental fluctuations are unknown.
Ecological Opportunities
To date, reaching suitable ecological time series to perform EWS analyses still remain challenging. Few field data have highlighted changes in the statistical structure of time series prior to sudden shifts exhibited at different time scales. Carpenter et al. (2011) measured chlorophyll at a 5 min time intervals during 3 years in their whole-lake experiment and showed EWS from chlorophyll (change in spectral power) following largemouth bass addition one year prior the food web transition. Hewitt and Thrush (2010) reported an increasing temporal variability (flickering) in the composition of intertidal communities before a trophic and functional change to an alternative community type using bimonthly monitoring data over 20 years. At higher time scale, Beaugrand et al. (2008) identified an increase in the local variance of biological variables (e.g., phytoplankton, zooplancton) prior abrupt climate-driven ecosystem shifts in the North Atlantic using data at an annual scale over around 50 years.
Our results indicated that samples integrating up to 5 years could be suitable for applying EWS among the two scenarios considered (especially for standard deviation; Table 2). At higher time scales, only standard deviation for the critical slowing-down scenario appeared to be suitable, up to 10 year time sample, to identify changes in the statistical structure of a time series prior to a critical transition. The implicit assumption that one time unit obtained from the model was equivalent to 1 year could be a bit restrictive in different cases, for instance for plurivoltine species. Apart technical difficulties to reach higher resolution than annual resolution from sediment cores, many lacustrine species are nonetheless univoltine (annual recruitment) and or typically uni-seasonal such as diatoms, meaning that they are also recorded annually (Giguet-Covex et al., 2010). For populations and communities, EWSs can provide relevant information especially for abundant aquatic species whose past abundance can be tracked in sediment archives through biological remains such as chironomids (Frossard et al., 2013) or newly developed molecular analyses (ancient DNA, Savishcheva et al., 2011; Belle et al., 2014).
Species can be expected to intrinsically possess different indicative potential toward EWS analyses because of their different functional responses to environmental changes. For instance, Brodersen et al. (2004, 2008), who studied respiration of midges (Diptera; Chironomidae) in West Greenland and British Columbian lakes, found that species sustained their respiration along an oxygen gradient (forcing equivalent to c) in different ways following linear, break-point and cubic-like patterns. Oxygen being a primary environmental forcing for these species their different physiological respiration is likely to induce different patterns in EWS toward a population critical transition. Additionally, because a critical transition is especially expected in highly connected foodwebs (Scheffer et al., 2012), the position and interaction linking a species to the others could influence the indicative value of EWS for a specific species to the sustainability of the whole foodweb. As a consequence, apical species should be especially suitable to inform of a possible regime shift as they theoretically embed information of basal species (Sugihara et al., 2012). Luckily, they also usually exhibit longer (pluriannual) generation times allowing a fine match using palaeoecological records of their genuine dynamics.
The increasing anthropogenic forcings make the flickering scenarios plausible in the present and future times, especially driver-mediated flickering due to the increase of the number and the strength of forcings (Steffen et al., 2011). Our analyses showed that in this context, EWSs, especially standard deviation, were robust to consistent time aggregation (Δt = 5). Under highly noisy dynamics (noise-mediated) for which flickering can be induced by different causes (e.g., intrinsic and/or environmental stochasticity), EWS detection could be especially difficult (Contamin and Ellison, 2009; Perretti and Munch, 2012). Additionally, no linear dynamics could be triggered by changes in forcing combinations leading to regime shifts not preceded by EWSs before regime shift (Hastings and Wysham, 2010). Therefore, for the analyses of time series, EWSs should be coupled to nonlinear analyses (Hsieh et al., 2008; Ives and Dakos, 2012) to strengthen the robustness of palaeoecological interpretations.
Conclusions
The detectability of the EWS tested was shown to be robust to data aggregation usually found among high-resolution palaeoecological time series. Nonetheless, the use of a combination of EWSs associated with unbiased methods to estimate statistics of EWS trends such as block-based Kendall Tau should be encouraged to strengthen the robustness of identified patterns. Considered as resilience metrics, EWSs also offer promising decision tools to management ecologists to assess ecological vulnerability under external and internal forcings. They have also been shown to respond to other types of threshold dynamics (e.g., transcritical transitions; Kéfi et al., 2012; Hansen et al., 2013). Hence, their use is expected to be relevant in a large context of ecological dynamics.
Conflict of Interest Statement
The authors declare that the research was conducted in the absence of any commercial or financial relationships that could be construed as a potential conflict of interest.
Acknowledgments
We are grateful to Richard James Telford and Peter Guy Langdon that provided relevant suggestions that greatly improved early drafts of the manuscript.
References
Anderson, C. N. K., Hsieh, C.-H., Sandin, S. A., Hewitt, R., Hollowed, A., Beddington, J., et al. (2008). Why fishing magnifies fluctuations in fish abundance. Nature 452, 835–839. doi: 10.1038/nature06851
Beaugrand, G., Edwards, M., Brander, K., Luczak, C., and Ibanez, C. (2008). Causes and projections of abrupt climate-driven ecosystem shifts in the North Atlantic. Ecol. Lett. 11, 1157–1168. doi: 10.1111/j.1461-0248.2008.01218.x
Belle, S., Parent, C., Frossard, V., Verneaux, V., Millet, L., Chronopoulou, P.-M., et al. (2014). Temporal changes in the contribution of methane-oxidizing bacteria to the biomass of chironomid larvae determined using stable carbon isotopes and ancient DNA. J. Paleolimnol. 52, 215–228. doi: 10.1007/s10933-014-9789-z
Boettiger, C., and Hastings, A. (2012). Quantifying limits to detection of early warning for critical transitions. Interface 9, 2527–2539. doi: 10.1098/rsif.2012.0125
Brodersen, K., Pedersen, O., Walker, I., and Jensen, M. (2008). Respiration of midges (Diptera; Chironomidae) in British Columbian lakes: oxy-regulation, temperature and their role as palaeo-indicators. Freshwater Biol. 53, 593–602. doi: 10.1111/j.1365-2427.2007.01922.x
Brodersen, K. P., Pedersen, O., Lindegaard, C., and Hamburger, K. (2004). Chironomids (Diptera) and oxy-regulatory capacity: An experimental approach to paleolimnological interpretation. Limnol. Oceanogr. 49, 1549–1559. doi: 10.4319/lo.2004.49.5.1549
Carpenter, S. R., Cole, J. J., Pace, M. L., Batt, R., Brock, W. A., Cline, T., et al. (2011). Early warnings of regime shifts: a whole-ecosystem experiment. Science 332, 1079–1082. doi: 10.1126/science.1203672
Carpenter, S. R., Ludwig, D., Brock, W. A., and Columbia, B. (1999). Management of eutrophication for lakes subject to potentially irreversible change. Ecol. Appl. 9, 751–771. doi: 10.1890/1051-0761(1999)009[0751:MOEFLS]2.0.CO;2
Carstensen, J., Telford, R. J., and Birks, H. J. B. (2013). Diatom flickering prior to regime shift. Nature 498, E11–E12. doi: 10.1038/nature12272
Caswell, B. A., and Frid, C. L. J. (2013). Learning from the past: functional ecology of marine benthos during eight million years of aperiodic hypoxia, lessons from the Late Jurassic. Oikos 122, 1687–1699. doi: 10.1111/j.1600-0706.2013.00380.x
Contamin, R., and Ellison, A. M. (2009). Indicators of regime shifts in ecological systems: what do we need to know and when do we need to know it. Ecol. Appl. 19, 799–816. doi: 10.1890/08-0109.1
Dakos, V., Carpenter, S. R., Brock, W. A., Ellison, A. M., Guttal, V., Ives, A. R., et al. (2012a). Methods for detecting early warnings of critical transitions in time series illustrated using simulated ecological data. PLoS ONE 7:e41010. doi: 10.1371/journal.pone.0041010
Dakos, V., Carpenter, S. R., van Nes, E. H., and Scheffer, M. (2015). Resilience indicators: prospects and limitations for early warnings of regime shifts. Phil. Trans. R. Soc. B. 370:20130263. doi: 10.1098/rstb.2013.0263
Dakos, V., Scheffer, M., van Nes, E. H., Brovkin, V., Petoukhov, V., and Held, H. (2008). Slowing down as an early warning signal for abrupt climate change. Proc. Natl. Acad. Sci. U.S.A. 105, 14308–14312. doi: 10.1073/pnas.0802430105
Dakos, V., van Nes, E., D'Odorico, P., and Scheffer, M. (2012b). Robustness of variance and autocorrelation as indicators of critical slowing down. Ecology. 93, 264–271. doi: 10.1890/11-0889.1
Dakos, V., van Nes, E., and Scheffer, M. (2013). Flickering as an early warning signal. Theoret. Ecol. 6, 309–317. doi: 10.1007/s12080-013-0186-4
Drake, J. M., and Griffen, B. D. (2010). Early warning signals of extinction in deteriorating environments. Nature 467, 456–459. doi: 10.1038/nature09389
Estes, J. A., Terborgh, J., Brashares, J. S., Power, M. E., Berger, J., Bond, W. J., et al. (2011). Trophic downgrading of Planet Earth. Science 333, 301–306. doi: 10.1126/science.1205106
Folke, C., Carpenter, S., Walker, B., Scheffer, M., Elmqvist, T., Gunderson, L., et al. (2004). Regime shifts, resilience, and biodiversity in ecosystemmanagement. Annu. Rev. Ecol. Evol. Syst. 35, 557–581. doi: 10.1146/annurev.ecolsys.35.021103.105711
Frossard, V., Millet, L., Verneaux, V., Jenny, J.-P., Arnaud, F., Magny, M., et al. (2013). Chironomid assemblages in cores from multiple water depths reflect oxygen-driven changes in a deep French lake over the last 150 years. J. Paleolimnol. 50, 257–273. doi: 10.1007/s10933-013-9722-x
Giguet-Covex, C., Arnaud, F., Poulenard, J., Enters, D., Reyss, J. L., Millet, L., et al. (2010). Sedimentological and geochemical records of past trophic state and hypolimnetic anoxia in large, hard-water Lake Bourget, French Alps. J. Paleolimnol. 43, 171–190. doi: 10.1007/s10933-009-9324-9
Guttal, V., and Jayaprakash, C. (2007). Impact of noise on bistable ecological systems. Ecol. Model. 201, 420–428. doi: 10.1016/j.ecolmodel.2006.10.005
Hansen, G. J. A., Ives, A. R., Vander Zanden, M. J., and Carpenter, S. R. (2013). Are rapid transitions between invasive and native species caused by alternative stable states, and does it matter? Ecology 94, 2207–2219. doi: 10.1890/13-0093.1
Hastings, A., and Wysham, D. B. (2010). Regime shifts in ecological systems can occur with no warning. Ecol. Lett. 13, 464–472. doi: 10.1111/j.1461-0248.2010.01439.x
Hewitt, J., and Thrush, S. (2010). Empirical evidence of an approaching alternate state produced by intrinsic community dynamics, climatic variability and management actions. Mar. Ecol. Progr. Series 413, 67–276. doi: 10.3354/meps08626
Holling, C. S. (1959). The components of predation as revealed by a study of small-mammal predation of the European Pine Sawfly. Can. Entomol. 91, 293–320. doi: 10.4039/Ent91293-5
Horsthemke, W., and Lefever, R. (1984). Noise-induced transitions: theory and applications in Physics, Chemistry, and Biology Series. Springer Series Synergetics 15, 322.
Hsieh, C.-H., Anderson, C., and Sugihara, G. (2008). Extending nonlinear analysis to short ecological time series. Am. Nat. 171, 71–80. doi: 10.1086/524202
Ives, A., and Dakos, V. (2012). Detecting dynamical changes in nonlinear time series using locally linear state-space models. Ecosphere 3, 1–15. doi: 10.1890/ES11-00347.1
Juggins, S. (2014). Rioja: Analysis of Quaternary Science Data. R Package Version 0.9–3. Available online at: http://www.staff.ncl.ac.uk/stephen.juggins/
Kéfi, S., Dakos, V., Scheffer, M., van Nes, E., and Rietkerk, M. (2012). Early warning signals also precede non-catastrophic transitions. Oikos 122, 641–648. doi: 10.1111/j.1600-0706.2012.20838.x
Lenton, T. M., Livina, V. N., Dakos, V., van Nes, E. H., and Scheffer, M. (2012). Early warning of climate tipping points from critical slowing down: comparing methods to improve robustness. Philo. T R. Soc. A. 370, 1185–1204. doi: 10.1098/rsta.2011.0304
Ludwig, D., Jones, D. D., and Holling, C. S. (1978). Qualitative analysis of insect outbreak systems: the spruce budworm and forest. J. Anim. Ecol. 47, 315–332. doi: 10.2307/3939
May, R. M. (1977). Thresholds and breakpoints in ecosystems with a multiplicity of stable states. Nature 269, 471–477. doi: 10.1038/269471a0
Millennium Ecosystem Assessment. (2005). Ecosystems and Human Well-being: Synthesis. Washington, DC: Island Press.
Millet, L., Massa, C., Bichet, V., Frossard, V., Belle, S., and Gauthier, E. (2014). Anthropogenic versus climatic control in a high-resolution 1500-year chironomid stratigraphy from a southwestern Greenland lake. Quat. Res. 81, 193–202. doi: 10.1016/j.yqres.2014.01.004
Morozov, A. (2010). Emergence of Holling type III zooplankton functional response: bringing together field evidence and mathematical modelling. J. Theor. Biol. 265, 45–54. doi: 10.1016/j.jtbi.2010.04.016
Oksanen, J., Blanchet, G., Kindt, R., Legendre, P., Minchin, P., O'Hara, R., et al. (2015). vegan: Community Ecology Package. R Package Version 2.2–1. Available online at: http://CRAN.R-project.org/package=vegan
Perretti, C. T., and Munch, S. B. (2012). Regime shift indicators fail under noise levels commonly observed in ecological systems. Ecol. Appl. 22, 1772–1779. doi: 10.1890/11-0161.1
R Core Team Development (2015). R: A Language and Environment for Statistical Computing. Vienna: R Foundation for Statistical Computing.
Rull, V. (2014). Time continuum and true long-term ecology: from theory to practice. Front. Ecol. Evol. 2, 1–7. doi: 10.3389/fevo.2014.00075
Savishcheva, O., Debroas, D., Kurmayer, R., Villar, C., Jenny, J. P., Arnaud, F., et al. (2011). Quantitative PCR enumeration of total/toxic Plankthotrix rubescens and total Cyanobacteria in preserved DNA isolated from lake sediments. Appl. Environ. Microbiol. 77, 8744–8752. doi: 10.1128/AEM.06106-11
Scheffer, M., Bascompte, J., Brock, W. A., Brovkin, V., Carpenter, S. R., Dakos, V., et al. (2009). Early-warning signals for critical transitions. Nature 461, 53–59. doi: 10.1038/nature08227
Scheffer, M., Carpenter, S., Foley, J. A., Folke, C., and Walker, B. (2001). Catastrophic shifts in ecosystems. Nature 413, 591–596. doi: 10.1038/35098000
Scheffer, M., Carpenter, S. R., Lenton, T. M., Bascompte, J., Brock, W., Dakos, V., et al. (2012). Anticipating Critical Transitions. Science 338, 344–348. doi: 10.1126/science.1225244
Seekell, D., Cline, T., Carpenter, S., and Pace, M. (2013). Evidence of alternate attractors from a whole-ecosystem regime shift experiment. Theor. Ecol. 6, 385–394. doi: 10.1007/s12080-013-0183-7
Steffen, W., Grinevald, J., Crutzen, P., and McNeill, J. (2011). The Anthropocene: conceptual and historical perspectives. Philo. T. Roy. Soc. A. 369, 842–867. doi: 10.1098/rsta.2010.0327
Sugihara, G., May, R., Ye, H., Hsieh, C.-H., Deyle, E., Fogarty, M., et al. (2012). Detecting Causality in Complex Ecosystems. Science 338, 496–500. doi: 10.1126/science.1227079
Veraart, A. J., Faassen, E. J., Dakos, V., Van Nes, E. H., Lürling, M., and Scheffer, M. (2012). Recovery rates reflect distance to a tipping point in a living system. Nature 481, 357–359. doi: 10.1038/nature11029
Wang, R., Dearing, J. A., Langdon, P. J., Zhang, E., Yang, X., Dakos, V., et al. (2012). Flickering gives early warning signals of a critical transition to a eutrophic lake state. Nature 492, 419–422. doi: 10.1038/nature11655
Wouters, N., Dakos, V., Edwards, M., Serafim, M. P., Valayer, P. J., and Cabral, H. N. (2015). Evidencing a regime shift in the North Sea using early-warning signals as indicators of critical transitions. Estuar. Coast. Shelf S. 152, 65–72. doi: 10.1016/j.ecss.2014.10.017
Keywords: early warning signal, time series, temporal aggregation, resilience, stability, lakes
Citation: Frossard V, Saussereau B, Perasso A and Gillet F (2015) What is the robustness of early warning signals to temporal aggregation? Front. Ecol. Evol. 3:112. doi: 10.3389/fevo.2015.00112
Received: 24 January 2015; Accepted: 14 September 2015;
Published: 29 September 2015.
Edited by:
Isabelle Larocque-Tobler, The L.A.K.E.S. Institute, SwitzerlandReviewed by:
Richard James Telford, University of Bergen, NorwayPeter Guy Langdon, University of Southampton, UK
Copyright © 2015 Frossard, Saussereau, Perasso and Gillet. This is an open-access article distributed under the terms of the Creative Commons Attribution License (CC BY). The use, distribution or reproduction in other forums is permitted, provided the original author(s) or licensor are credited and that the original publication in this journal is cited, in accordance with accepted academic practice. No use, distribution or reproduction is permitted which does not comply with these terms.
*Correspondence: Victor Frossard, CARRTEL (UMR 042), Université Savoie Mont Blanc, Domaine Universitaire, Belledonne, 212, 73376 Le Bourget-du-Lac, France,dmljdG9yLmZyb3NzYXJkQHVuaXYtc2F2b2llLmZy