- 1School of Biological and Chemical Sciences, Queen Mary University of London, London, United Kingdom
- 2Department of Marine Sciences, University of the Aegean, Mytilene, Greece
- 3Department of Environment, University of the Aegean, Mytilene, Greece
- 4School of Pure and Applied Sciences, Open University of Cyprus, Nicosia, Cyprus
Invasive alien species cause major changes to ecosystem functioning and patterns of biodiversity, and the main factors involved in invasion success remain contested. Using the Mediterranean island of Crete, Greece as a case study, we suggest a framework for analyzing spatial data of alien species distributions, based on environmental predictors, aiming to gain an understanding of their spatial patterns and spread. Mediterranean islands are under strong ecological pressure from invading species due to their restricted size and increased human impact. Four hypotheses of invasibility, the “propagule pressure hypothesis” (H1), “biotic resistance hypothesis vs. acceptance hypothesis” (H2), “disturbance-mediated hypothesis” (H3), and “environmental heterogeneity hypothesis” (H4) were tested. Using data from alien, native, and endemic vascular plant species, the propagule pressure, biotic resistance vs. acceptance, disturbance-mediated, and environmental heterogeneity hypotheses were tested with Generalized Additive Modeling (GAM) of 39 models. Based on model selection, the optimal model includes the positive covariates of native species richness, the negative covariates of endemic species richness, and land area. Variance partitioning between the four hypotheses indicated that the biotic resistance vs. acceptance hypothesis explained the vast majority of the total variance. These results show that areas of high species richness have greater invasibility and support the acceptance hypothesis and “rich-get-richer” distribution of alien species. The negative correlation between alien and endemic species appears to be predominantly driven by altitude, with fewer alien and more endemic species at greater altitudes, and habitat richness. The negative relationship between alien and endemic species richness provides potential for understanding patterns of endemic and alien species on islands, contributing to more effective conservation strategies.
Introduction
Alien species are non-indigenous taxa introduced to areas beyond their natural distribution and biogeographical barriers by human activity. They can become invasive and have major impacts on native biodiversity by causing local or global extinctions, modifying community composition, and impeding or altering ecosystem functioning (Kolar and Lodge, 2001; Richardson and Pyšek, 2006; Reaser et al., 2007; Blackburn et al., 2014; Kumschick et al., 2015). Invasion can be viewed as a function of the relationship between abiotic characteristics of an invaded ecosystem, the traits of alien species and recipient community, in addition to propagule pressure that includes the number of non-native individuals in an introduced group and the number of introduction events (Lockwood et al., 2005; Catford et al., 2009). Each of these factors contribute to the success of an invasion, although their influence is unlikely to be equal, and in many cases, there are multiple, largely interactive factors underlying invasibility (Alpert et al., 2000; Catford et al., 2009, 2011).
Several hypotheses have been proposed for explaining successful invasions (Hierro et al., 2005; Jeschke, 2014). These hypotheses may actually be overlapping and they are not necessarily mutually exclusive. According to the “propagule pressure hypothesis” (H1), high supply and frequency of plant propagule introductions (including adult plants, seeds or reproductive vegetative fragments) should raise the probability of successful invasion through increased genetic diversity, seed swamping and ongoing addition of new individuals, greater probability of introduction into a favorable environment, and increasing the chances of overcoming environmental and demographic stochasticity (Lockwood et al., 2005, 2013; Catford et al., 2009; Simberloff, 2009). In the British Isles, the H1 may explain, to a degree, why many plant invasions occur close to human sites and settlements (Crawley et al., 1996).
The “biotic resistance hypothesis” predicts ecosystems will have greater resistance to invasion with high native species richness compared to ecosystems with low richness (Elton, 1958; Levine and D'Antonio, 1999; Lonsdale, 1999; Jeschke, 2014). It is a special formulation of the “diversity-stability hypothesis,” a more general ecological hypothesis, according to which ecosystems with high biodiversity are more stable than ecosystems with low biodiversity. This is closely related to the “empty niche” hypothesis, which posits that when an ecosystem is unsaturated from low indigenous species diversity, invading groups will occupy underutilized niches and use available resources (MacArthur, 1970; Hierro et al., 2005). This hypothesis predicts a negative correlation between native and alien species richness. However, a number of studies reported the opposite pattern, i.e., a positive correlation between native and alien species richness (McKinney, 2002), leading to the formulation of the “acceptance hypothesis” and to a “rich-get-richer” pattern (Stohlgren et al., 2003, 2006). As “biotic resistance hypothesis vs. acceptance hypothesis” (H2) are essentially two sides of the same coin we have therefore treated them as one testable hypothesis, i.e., the chances of successful invasion depend (positively or negatively) on the native species richness.
The “disturbance-mediated hypothesis” (H3) states that disturbance can reset succession and improve availability of resources, providing alien species with an equal or improved chance of success in colonization and establishment (Catford et al., 2009). A strong global relationship has been proposed between human-induced disturbance and invasibility (Zhang et al., 2006), explained both by human activities creating pathways for the introduction of alien species and the ongoing generation of new (artificial or agricultural) habitat types due to human-mediated habitat destruction (of natural habitat types) and biodiversity loss.
According to the “environmental heterogeneity hypothesis” (H4), ecosystems can host a diversity of species when they contain a variety of niches and high environmental variability (Melbourne et al., 2007; Catford et al., 2009). Heterogeneity in key environmental factors, such as temperature, water, nutrients, sunlight and physical disturbances plays a definite role in community-level resistance to new invasions, as well as in their outcome and the severity of their impacts (Melbourne et al., 2007).
Islands are geographically isolated and often have a distinct combination of species compared to those found on continents, and are under particularly strong ecological threat from invasive alien species (Reaser et al., 2007). The Mediterranean islands are considered more vulnerable to invasive alien species introductions than their comparable closest mainland areas due to their proportionally lower native diversity and the presence of unsaturated local communities (Hulme et al., 2008), and evidence suggests there have been major increases in the number of invasive alien species recently (Celesti-Grapow et al., 2016). Moreover, the historical and modern importance of ports, trade and anthropogenic disturbance, with changes to land use and climate are likely facilitating further the introduction and establishment of alien species (Ross et al., 2008). Crete, the fifth largest Mediterranean island, and surrounding islets provide an interesting case study to test hypotheses on plant invasions, as the islands are a biodiversity hotspot containing a unique flora (Medail and Quezel, 1997). The islands have been populated by humans for over 9,000 years, but there are recent severe anthropogenic pressures and land use changes (Papanastasis and Kazaklis, 1998; Sarris et al., 2005). We sought to quantify the patterns of alien vascular plant species richness by testing four invasibility hypotheses.
Methods
Study Area
The island of Crete and 49 surrounding islets (i.e., the Cretan area Turland et al., 1993) were examined, covering an area of 8,374 km2 and including 1,647 vascular plant species (Turland et al., 1993). The Crete area exhibits rich plant diversity and is a red alert biodiversity hotspot within the Mediterranean (Médail and Diadema, 2009), and around 10% of its total native number of species comprises endemic vascular flora (Turland et al., 1993). Apart from the endemic species, the flora comprises significant Eastern Mediterranean and Anatolian elements, (e.g., Datisca sp), Tertiary relictual Aegean endemics (e.g., Lomelosia sp), and North African species (Erodium sp). There are three mountain massifs in Crete, with Psiloritis the highest (at 2,456 m) and Lefka Ori the most extensive. The latter, situated at the western part on the island include 15 peaks above 2,200 m (Vogiatzakis et al., 2003). The distribution of elevations on the island changes steeply, particularly on its south-western part (Vogiatzakis et al., 2003). Coastal areas are highly modified mainly for tourism infrastructure, and the human population of the island mainly resides on the north side where most cities are located (and thus most human-created and modified habitats are present). There is a significant number of cultivated land areas (agricultural habitats) predominantly with olive trees and grapes.
Linking Hypothesis with Covariates
Statistical models were designed to explicitly test the four hypotheses. The covariates used to test H1 were the percentage cover of artificial and agricultural habitats, with each providing a measure of human presence, activity and intensity of the main pathways for alien plants, i.e., ornamental planting, trade of contaminated commodities, and cultivation, (see European Alien Species Information Network; Katsanevakis et al., 2015), and used herein as a surrogate of propagule pressure. For H2, native and endemic species richness provides a measure of species diversity. For H3, artificial and agricultural habitat richness were used measuring the variety of anthropogenic habitats and thus available empty habitats deriving from human-mediated disturbance. Artificial plus agricultural percentage of cover was partly correlated with total habitat richness (linear regression, R2 = 23.1%) and thus to that end there is some co-linearity between the covariates for H1 and H3 (see Figure S1). For H4, environmental traits measuring heterogeneity were used; these included altitude range, habitat richness, soil richness, temperature range, and precipitation range. In the plant atlas by Turland et al. (1993) coastal cells have unequal surface area with inland cells. In order to account for this effect the total surface area of each cell was normalized by the surface area of inland cells (8.25 × 8.25 = 68.0625 km2). All hypotheses included the effects of cell land area as a fixed covariate in order to account for the effect of unequal land surface area of cells neighboring with the sea. Therefore, apart from cell area, all four hypotheses included different sets of covariates. A full list with all the statistical models explored and their link with the four hypotheses is provided in Table 1.
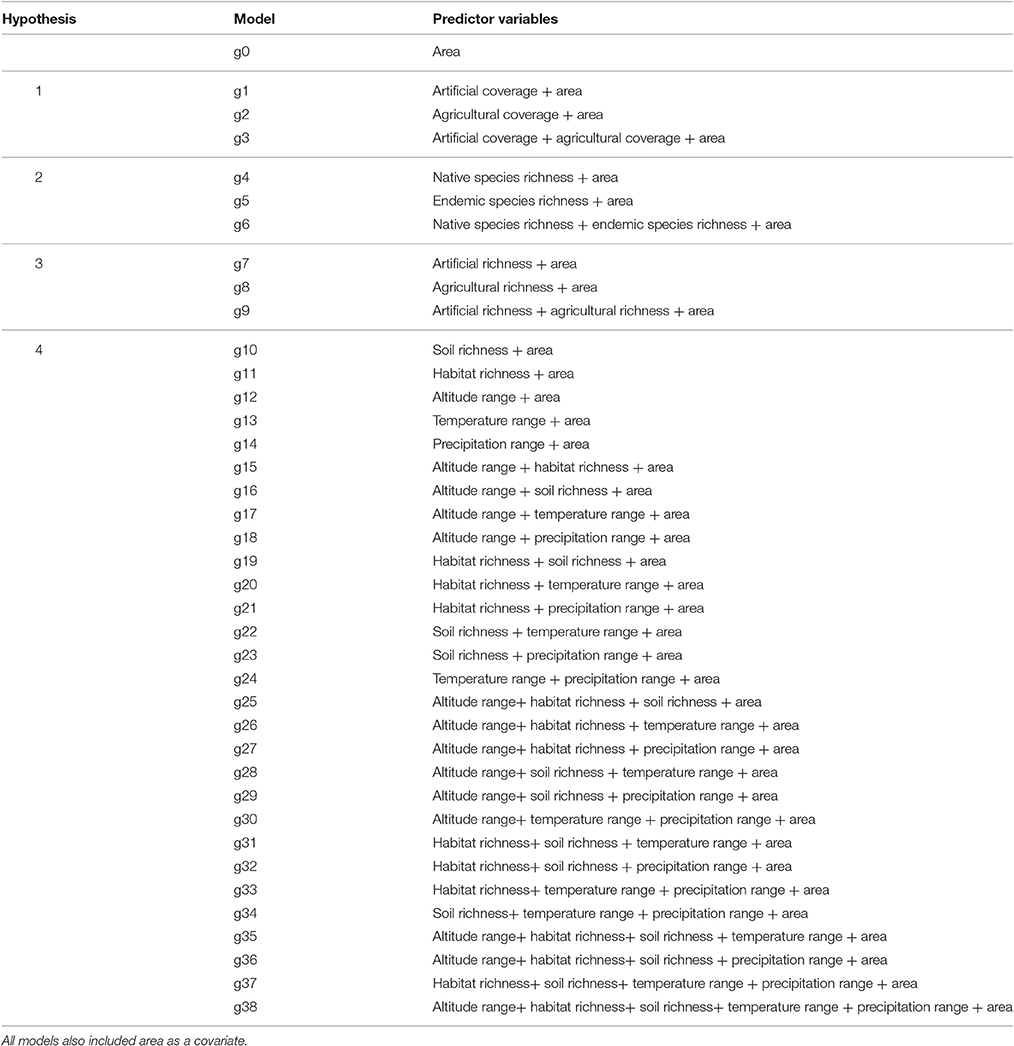
Table 1. List of all models and predictor covariates of alien species richness related to each of the four hypotheses.
Plant Data
Maps of presence-absence vascular plant species distributions in the Cretan area were digitized from Turland et al. (1993) and its latest supplement (Chilton and Turland, 2008). The main island of Crete and the surrounding islands were divided into 162 grid cells of 8.25 × 8.25 km following the grid cell size of Turland et al. (1993). For each cell, the native, endemic, and alien species richness was calculated. We used (Turland et al., 1993; Chilton and Turland, 2008) and references therein to define native (nnat = 1,395) and endemic (nend = 174) species, and the vascular plants from D'Agata et al. (2009) that are listed in Turland et al. (1993) and Chilton and Turland (2008) were used to define alien (nalien = 78) species.
Land Cover Data
Habitat classification relied on the most detailed resolution available of the CORINE Landcover (level 3, spatial resolution 100 m; EEA, 2010), to calculate the richness and percentage of every land cover class within every grid cell, using Patch Analyst 5.1 within ArcGIS. In order to avoid potential temporal deviance between habitat classifications and species presences in cells, the last updated available supplement for the flora of Crete published in 2008 (Chilton and Turland, 2008) and the closest available time snapshot of the CORINE landcover for Crete in 2010 were used. The classification process resulted in 29 habitat types, of which 9 were agricultural, 7 were artificial, and 13 were natural. We recorded habitat richness per cell as the number of different land cover types present on each cell as well as their percentage of cover.
Climatic, Soil, and Altitude Data
Climatic variables were derived from WorldClim (Hijmans et al., 2005) for Crete and surrounding islets. The original resolution of the climatic data was 1 km. In order to re-scale them to 8.25 km and match them with the grid of the plant data the mean values of the 1 km data within the 8.25 km cells were calculated and used. The climatic variables used here were: annual mean temperature (Tempmean), annual mean temperature of warmest quarter (Tempwarm), annual mean temperature of coldest quarter (Tempcold) all in °C, annual mean precipitation (Precipmean), precipitation of wettest quarter (Precipwet), precipitation of driest quarter (Precipdry) all in mm year−1. Soil data were derived from SoilGrid (Hengl et al., 2014) and were rescaled from 1 to 8.25 km as done for the climatic data. The soil variable used was soil richness in the cell (Soildiv) derived as the number of different soil types occurring within each cell. The indices of elevation recorded were the mean of all elevation scores within the cell (Alt) and the range of elevation within the cell (Alt range) both in meters.
Statistical Analysis
The inherently non-linear relationship between alien species richness and potential explanatory covariates were modeled with Generalized Additive Models (GAMs); (Hastie and Tibshirani, 1990). GAMs are a generalization of the multiple regression model maintaining the additive nature of the model replacing the terms of the linear equation with non-parametric smooth functions s(Xi) of each independent variable (Xi). In GAMs the distribution of the dependent variable can be explicitly quantified and therefore does not need to be normal nor does it have to be continuous. Having specified the underlying distribution of the dependent variable, values are predicted from a linear combination of independent variables, via a link function which specifies the distribution of the dependent variable:
where Y is the dependent variable, E[Y] is the expected value of Y and g(E[Y]) is the link function, that links the dependent variable to the predictor variables Xi.
Specifically, the expected value of alien species richness in each 8.25 × 8.25 km cell was related to the explanatory covariates, according to the general formulation:
where g is the link function, sm(·) is the one-dimensional smooth function for covariate m, and Xmi is the value of covariate Xm for the ith cell. All the smooth functions sm(·) were implemented using penalized cubic regression splines (with the default basis dimension q = 10, estimated by penalized iterative least squares; Wood, 2006). Optimum smoothing was determined using generalized cross validation (GCV), increasing by a factor of γ = 1.4 in the GCV score the amount that the effective degree of freedom of each model counts. As occasionally the GCV method may result in over-fitting a value of γ ≈ 1.4 was used in order to account for this without compromising model fit (Kim and Gu, 2004). The model fitting was conducted using the mgcv package (Wood, 2006) in R v.3.1.2 (R Development Core Team, 2016).
To identify the best underlying probability distribution, five different error distributions were examined including Gaussian, Lognormal, Gamma, Poisson, and Negative binomial; these distributions were compared based on a full model with all of the predictor variables included using the Akaike Information Criterion (Akaike, 1973; Burnham and Anderson, 2002). After identifying the best probability distribution, AIC was used for model selection among the set of candidate models. Thirty-nine alternative GAMs gi, i = 0–38 were fitted, where a reference model go was used with area as the only predictor variable. The subsequent 39 models all involved one or more predictor variables related with the four hypotheses (see Table 1 for the full list). The equations of the maximal model for H1, H2, H3, and H4, respectively are:
The model with the lowest AIC was selected as the best model. An empirical semivariogram of the residuals of the optimal model showed no evidence of spatial autocorrelation (Legendre and Fortin, 1989).
Variance partitioning was employed in order to provide a further measure of how much independent variation is accounted for by each of the four hypotheses (Borcard and Legendre, 1994). We added together all the relevant covariates to each hypothesis and quantified the independent contribution of each hypothesis to the total pattern of alien species richness (H1 included variables as described in the model g3, H2 as in g6, H3 as in g9, and H4 as in g38). Randomizations (n = 99) were also performed in order to asses confidence intervals (Mac Nally, 2002).
Results
An initial data mining exploration between all dependent and independent variables with multi-scatter plots is provided in Figure 1 and a cluster analysis is provided in the Supplementary Material (Figure S1). From a simple initial linear regression or smoothing perspective, alien species richness have a positive relationship with native species richness, and no relationship (regression slope close to zero) or weakly negative based on smoothing trend lines with endemic species richness (Figure 1A). Endemic species richness was positively correlated with native species richness (Figure 1A). Overall the relationship between richness and cover is not straightforward as either regression slopes are relatively low or there is a deviance between regressions and smoothing (Figure 1B). Altitude is positively correlated with altitude range and mean precipitation with precipitation range but temperature mean has no clear relationship with temperature range (Figure 1C). As expected altitude is negatively correlated with mean temperature, but not with temperature range (Figure 1C). Altitude is also negatively correlated with soil richness (Figure 1C).
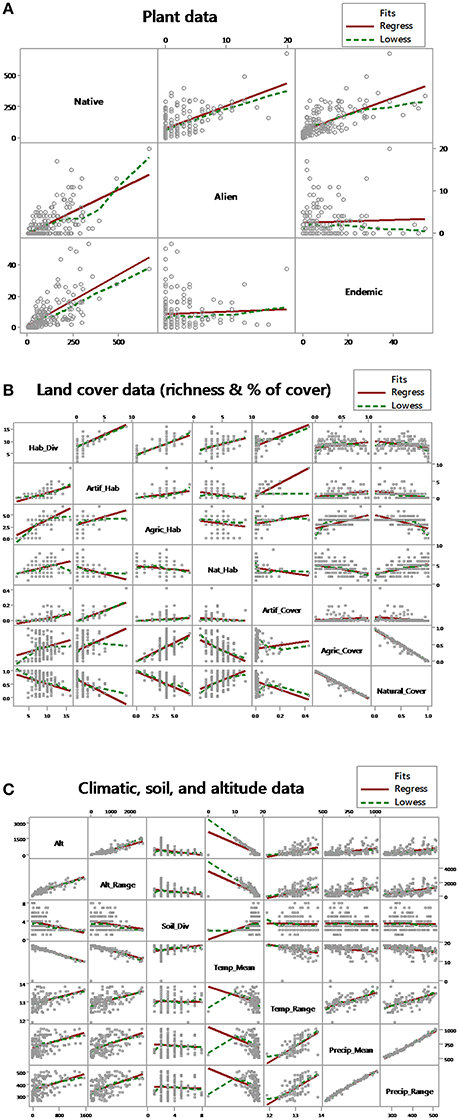
Figure 1. Initial data mining: (A) exploring the relationship between species richness data (described in the main text, methods “Plant data”) (B) land cover indices (described in the main text, methods “Land cover data”), and (C) environmental and climatic data (described in the main text, methods “Climatic, soil, and altitude data”) with multi-scatter plots. Grey circles indicate data points, solid red lines linear regression, and dashed green lines a lowess smoother between each two data indices.
The Poisson probability distribution had the lowest AIC score (Table S1), and thus all models were run using a Poisson error distribution and log as the natural link function. From the full analyses of all 39 models (Table S2), the model g6 related with the H2 was the best-supported model with the lowest AIC (550.7793) and explained 67.8% of deviance. Model g6 included native species richness, endemic species richness and area as predictor variables. Alien species richness was positively correlated with native species richness, negatively correlated with endemic species richness, and, as expected, increased with area across the main range of cell land areas (the slightly declining richness with area at high values of area was non-significant and was based on a very low number of data points); (Figure 2).
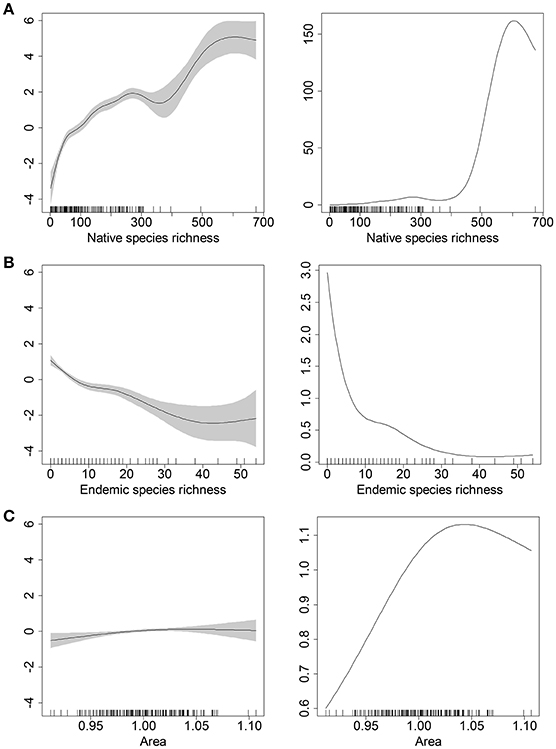
Figure 2. Estimated smooth terms for the best model of alien species richness. The top panels (A) show the positive correlation between alien species richness and native species richness, (B) the middle panel shows the negative correlation with endemic species richness, and (C) bottom panel the relationship with area. For panels on the left, smooth terms (black lines) are given in the linear predictor scale and the corresponding 95% confidence intervals are indicated with gery intervals. For panels on the right, smooth terms are given in the response scale of the raw data (i.e., smooth terms were exp-transformed). The numbers after the commas in the y-axis titles are the estimated degrees of freedom of the smooth terms. Rug on the horizontal axis represents density of datapoints.
Variance partitioning of the habitat and environmental covariates included in each hypothesis showed the variables involved in H2 explained close to 60% of variation in alien richness, whereas H1 explained just over 10%, H3 close to 30%, and H4 under 10% of variation (Figure 3).
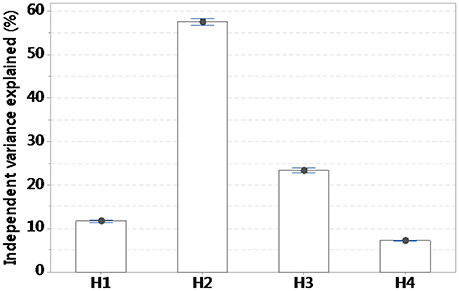
Figure 3. Variance partitioning of environmental and habitat covariates included in each of four hypotheses. H1, propagule pressure hypothesis; H2, biotic resistance vs. acceptance hypotheses; H3, disturbance-mediated hypothesis; H4, environmental heterogeneity hypothesis.
Discussion
Patterns of alien species in the Cretan area, based on testable hypotheses, provided the greatest support for the “biotic resistance vs. acceptance hypotheses” (H2) both in terms of model selection out of 39 models as well as from variance partitioning—nearly 60% of the total variance was explained by H2. This does not mean there is no relationship between alien species richness and human activity and environmental disturbance. Indeed, other models than the optimal had strong explanatory power and all hypotheses showed patterns as expected with alien species richness been positively correlated with propagule pressure (Wallace and Prather, 2016), human activity (Malavasi et al., 2016), and environmental disturbance (Peltzer et al., 2016) as well as synergistic interactions (Barney et al., 2016; Berg et al., 2016). These results are only partly shown here, as the deviance explained by each model and variance partitioning show the magnitude of the result but not the sign of the relationship, and interactions of covariates between different hypotheses were not explored.
Our results indicate that rather than a “black and white” or a “single variable” attempt to explain patterns of alien species, the reality seems to lie in between—all hypotheses contributed to explaining the pattern. The concept of a single “true” predictive model seems to be of little utility in ecology (Evans et al., 2014) with more data resulting in more complex models (Evans and Moustakas, 2016). Nevertheless, in this study the goal was not to develop the best possible predictive model (i.e., explaining as much of the variance as possible) but rather to investigate the differential support of the main hypotheses in invasion biology. Note that several more testable hypotheses exist—(see e.g., Hierro et al., 2005; Catford et al., 2009; Jeschke, 2014)—regarding invasibility that we did not explore because some of them are overlapping and would require using the same covariates to explore different hypotheses.
According to the results derived here there is a strong implication for the acceptance hypothesis and a “rich-get-richer” distribution of invasive vascular plant species in the Cretan area, whereby regions with high native species richness are associated with high alien species richness (Stohlgren et al., 2003). The positive correlation between alien and native species richness is consistent with multiple previous large-scale observational studies (Knops et al., 1995; Robinson et al., 1995; Planty-Tabacchi et al., 1996; Levine and D'Antonio, 1999; Lonsdale, 1999; Levine, 2000; McKinney, 2002; Stohlgren et al., 2003, 2006; Souza et al., 2011; Blackburn et al., 2016). It is difficult to ascertain the full causal relationship between alien and native species distributions, as their apparent co-variation may be due to similar, independent reactions to the same factors, specifically favorable environmental conditions, adequate resources and fertile land, meaning the conditions good for native species are also good for alien species (Levine and D'Antonio, 1999; Stohlgren et al., 1999; Richardson and Pyšek, 2006).
There are several studies reporting an inverse relationship between alien and native species, in terms e.g., of environmental energy and human impact (Marini et al., 2009), responses to soil fertility and in general of trait or performance differences among plant functional groups (Peltzer et al., 2016). However, the existence of different patterns between alien and native species is not consistent worldwide (Ordonez and Olff, 2013), and the result of inverse patterns between alien and endemic species reported here appears to be fairly novel.
We performed a post-hoc analysis examining separately the relationship of alien and endemic species richness with altitude and habitat richness, and the correlation between endemic and native species richness (Figure 4). There is a positive correlation between endemic species richness and altitude, the opposite pattern found for alien species (Figures 4A,B), indicating the observed relationship between alien and endemic species richness is possibly linked with altitude. Indeed, almost half (43%) of Cretan endemics are found solely at elevations higher than 1,000 m (Legakis and Kypriotakis, 1994). Endemic species richness is known to increase with altitude (Cañadas et al., 2014), and typically peaks at higher altitudes compared to total species richness (Vetaas and Grytnes, 2002). Alien and endemic species distributions have been shown to differ along a longitudinal gradient in rivers in another Mediterranean island (Sardinia), with endemic species more common in natural upper parts and alien species more common in lower and middle parts, the latter linked to human impact and agriculture (Angiolini et al., 2013). In Tenerife (Canary islands) altitude was the most important factor determining (inverse) patterns in endemic and native species along roadsides (Bacaro et al., 2015). Altitude itself is likely to be masking several long-term acting processes regarding endemism: the increased proportion of Cretan endemics with altitude is regarded as being due to diversification linked to the Cretan mountains (especially mid-altitude areas), driven by altitudinal-driven ecological isolation (Trigas et al., 2013). Climate filtering reflected in varying patterns along altitudinal gradients is an important determinant of the richness of alien species (which are not adapted to high altitudes), while anthropogenic pressures may explain the richness of alien species at low elevation (Bacaro et al., 2015). Higher elevations have an interaction effect between area and habitat diversity: area since in small islands land surface area extends with elevation, and components of habitat diversity, such as more heterogeneous topography, a broader selection of micro-habitats, and a reduced effect of the sea are related with elevation (Panitsa et al., 2006). It is therefore possible that the effect of environmental heterogeneity is hidden behind elevation.
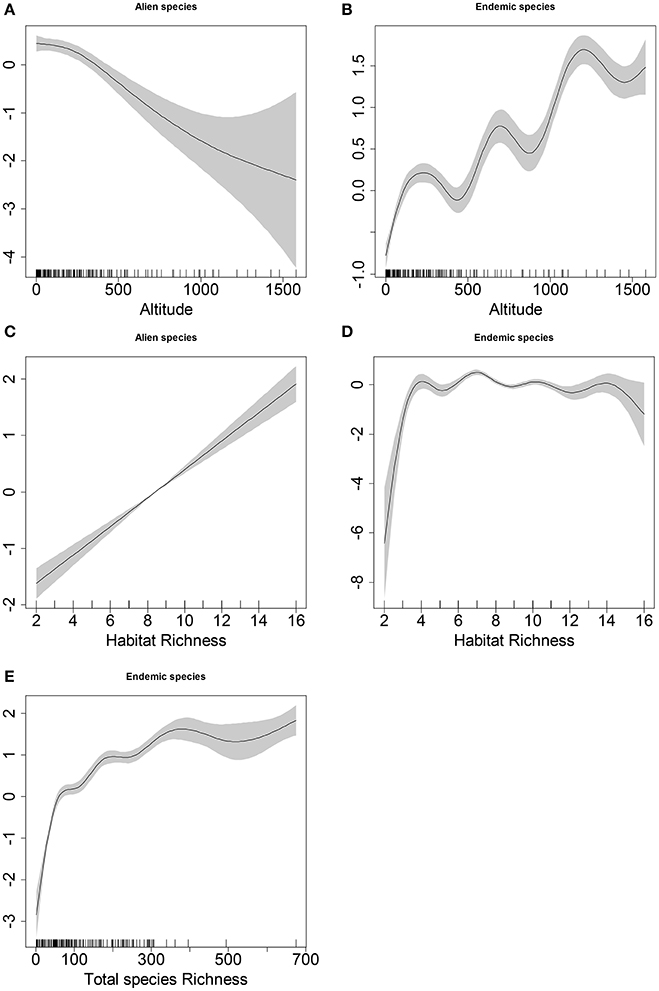
Figure 4. Estimated smooth terms for (A) alien species richness and altitude, (B) endemic species richness and altitude, (C) alien species richness and habitat richness, (D) endemic species richness and habitat richness, and (E) endemic species richness and native species richness. Smooth terms (black lines) are given in the linear predictor scale and the corresponding 95% confidence intervals are indicated with gray intervals. The numbers after the commas in the y-axis titles are the estimated degrees of freedom of the smooth terms. Rug on the horizontal axis represents density of datapoints.
Our post-hoc analysis indicated a further variable of interest, habitat richness, which showed a positive correlation with alien species richness (Figure 4C), supporting the H4 but no consistent effect on endemic species (Figure 4D). This shows the two groups have different responses to habitat richness, but the inconclusive results for endemic species seem to rule out habitat richness as a primary driver of the relationship between alien and endemic species richness. In this case this implies that habitat richness is explored differently by alien and endemics potentially because alien species are habitat generalists while endemics are specialists (Harrison, 1999; Evangelista et al., 2008). More often than not, high habitat richness locations contain agricultural or artificial habitats (i.e., high habitat richness is associated with non-natural, modified habitats). This is particularly important as due to climatic change, disproportionately more high altitude habitats are likely to be modified (Louca et al., 2015), thereby increasing habitat richness in high altitute locations (Dirnböck et al., 2011). Additional post-hoc analysis regarding alien species richness exhibited no consistent trends against indices of temperature (mean, warmest, and coldest) or precipitation (mean, wettest, and driest); (Figure S2).
Understanding the mechanisms by which alien plants impact native (Vilà et al., 2011) or endemic species (Thomson, 2005) is a key question for understanding alien species dynamics. The interaction between alien and native species may also be positive through mutualism or facilitation (Mooney and Cleland, 2001; Rodriguez, 2006). A key question that arises here is whether patterns of endemic species can provide long-term resistance indicators to invasions, or if the inverse pattern between alien and endemics is simply a result of isolation and inaccessibility due to high altitude. Eitherway this has strong implications for conservation: high altitude locations would need to remain unmodified as their habitat modification could result in increased habitat richness that in turn can be explored by alien species (Marvier et al., 2004). While speciation in endemic species could be linked to their biogeographical and genetic isolation from closely related species (Cañadas et al., 2014), the positive correlation with native species richness shows more endemic species are present in areas with higher richness (Figure 4E), and the dynamics of the relationship between endemic and alien species richness are not simply proxies for relationships with total species richness. In addition, endemic and alien components of the flora are not random assemblages of species: there are more alien than endemic families, and the pool of alien species is less of a random selection of species than it is for endemics (Vilà and López-Darias, 2006).
Conclusions
Using the Mediterranean island of Crete as a case study, we suggest a framework for analyzing spatial data of alien species distributions, based on environmental predictors, aiming to gain an understanding of their spatial patterns and spread. The analysis involved a three-way coupling of data [(i) spatial plant species distributions, (ii) land-use data, and (iii) physical geography-related data, such as climatic, altitudinal, and soil data]. Technological developments in smart sensors, social networks, and digital maps, spatio-temporal data are more available than ever before (Reis et al., 2015; Miyazaki et al., 2016; Niphadkar and Nagendra, 2016) and ecology in the big data era needs to integrate novel methods for their analysis (Moustakas, 2017). The availability of large datasets poses great challenges in data analytics (Moustakas and Evans, 2017) but also increased availability of computing power facilitates the use of computationally-intensive methods for the analysis of such data in ecology (Moustakas and Evans, 2015).
Despite the relatively large volume of data, the analysis conducted was on a hypothesis-based model selection rather than statistical-only model selection. Recent debates in data analytics have argued that the availability of a large volume of data will make the scientific method obsolete (Anderson, 2008); hypothesis-driven, or equation-driven research will become irrelevant and hypothesis-free data mining will be used instead (Anderson, 2008). This analysis showcases that if one simply relies on data-driven science several components of scientific methods could be made poorer (Moustakas, 2017). This study has identified an inverse relation between alien and endemic species richness, to our knowledge a novel result. The result was derived via a correlative analysis which does not show causality nor provide a mechanism. However, it has identified the potential for learning patterns, traits, and proccesses from endemic species as counter preferences or potentially competitive exclusion between alien and endemic species. This could be achieved via (a) field experiments and mechanistic studies (b) biogeographic analysis of alien and endemics and (c) exploring additional variables than the ones explored here at a macroecological scale as potential traits.
Author Contributions
AB performed the majority of the statistical analysis, and wrote a significant part of the manuscript. SK formulated the hypotheses, contributed to the statistical analysis and to manuscript writing. IV performed the land use, soil, climatic, and physical geography remote sensing analysis resulting into the environmental dataset, and commented on the manuscript. AG provided the total, endemic and alien species data and commented on the manuscript. AM supervised and coordinated the research, performed part of the statistical analysis, and wrote a significant part of the manuscript.
Conflict of Interest Statement
The authors declare that the research was conducted in the absence of any commercial or financial relationships that could be construed as a potential conflict of interest.
Supplementary Material
The Supplementary Material for this article can be found online at: http://journal.frontiersin.org/article/10.3389/fevo.2017.00091/full#supplementary-material
References
Akaike, H. (1973). Maximum likelihood identification of Gaussian autoregressive moving average models. Biometrika 60, 255–265. doi: 10.1093/biomet/60.2.255
Alpert, P., Bone, E., and Holzapfel, C. (2000). Invasiveness, invasibility and the role of environmental stress in the spread of non-native plants. Perspect. Plant Ecol. Evol. Syst. 3, 52–66. doi: 10.1078/1433-8319-00004
Angiolini, C., Nucci, A., Landi, M., and Bacchetta, G. (2013). Distribution of endemic and alien plants along Mediterranean rivers: a useful tool to identify areas in need of protection? C. R. Biol. 336, 416–423. doi: 10.1016/j.crvi.2013.07.001
Bacaro, G., Maccherini, S., Chiarucci, A., Jentsch, D., Rocchini, D., Torri, M., et al. (2015). Distributional patterns of endemic, native and alien species along a roadside elevation gradient in Tenerife, Canary Islands. Commun. Ecol. 16, 223–234. doi: 10.1556/168.2015.16.2.10
Barney, J. N., Ho, M. W., and Atwater, D. Z. (2016). Propagule pressure cannot always overcome biotic resistance: the role of density-dependent establishment in four invasive species. Weed Res. 56, 208–218. doi: 10.1111/wre.12204
Berg, J. A., Meyer, G. A., and Young, E. B. (2016). Propagule pressure and environmental conditions interact to determine establishment success of an invasive plant species, glossy buckthorn (Frangula alnus), across five different wetland habitat types. Biol. Invasions 18, 1363–1373. doi: 10.1007/s10530-016-1073-x
Blackburn, T. M., Delean, S., Pyšek, P., and Cassey, P. (2016). On the island biogeography of aliens: a global analysis of the richness of plant and bird species on oceanic islands. Global Ecol. Biogeogr. 25, 859–868. doi: 10.1111/geb.12339
Blackburn, T. M., Essl, F., Evans, T., Hulme, P. E., Jeschke, J. M., Kühn, I., et al. (2014). A unified classification of alien species based on the magnitude of their environmental impacts. PLoS Biol. 12:e1001850. doi: 10.1371/journal.pbio.1001850
Borcard, D., and Legendre, P. (1994). Environmental control and spatial structure in ecological communities: an example using oribatid mites (Acari, Oribatei). Environ. Ecol. Stat. 1, 37–61. doi: 10.1007/BF00714196
Burnham, K. P., and Anderson, D. R. (2002). Model Selection and Multimodel Inference. New York, NY: Springer Verlag.
Cañadas, E. M., Fenu, G., Peñas, J., Lorite, J., Mattana, E., and Bacchetta, G. (2014). Hotspots within hotspots: endemic plant richness, environmental drivers, and implications for conservation. Biol. Conserv. 170, 282–291. doi: 10.1016/j.biocon.2013.12.007
Catford, J. A., Jansson, R., and Nilsson, C. (2009). Reducing redundancy in invasion ecology by integrating hypotheses into a single theoretical framework. Divers. Distrib. 15, 22–40. doi: 10.1111/j.1472-4642.2008.00521.x
Catford, J. A., Vesk, P. A., White, M. D., and Wintle, B. A. (2011). Hotspots of plant invasion predicted by propagule pressure and ecosystem characteristics. Divers. Distrib. 17, 1099–1110. doi: 10.1111/j.1472-4642.2011.00794.x
Celesti-Grapow, L., Bassi, L., Brundu, G., and D'Auria, U. (2016). Plant invasions on small Mediterranean islands: an overview. Plant Biosys. Int. J. Dealing Aspects Plant Biol. 150, 1119–1133. doi: 10.1080/11263504.2016.1218974
Chilton, L., and Turland, N. J. (2008). Flora of Crete a Supplement II, Additions 1997-2008. Available online at: http://www.marengowalkscom/fcshtml
Crawley, M. J., Harvey, P. H., and Purvis, A. (1996). Comparative ecology of the native and Alien Floras of the British Isles. Philos. Trans. 351, 1251–1259. doi: 10.1098/rstb.1996.0108
D'Agata, C. D. C., Skoula, M., and Brundu, G. (2009). A preliminary inventory of the alien flora of Crete (Greece). Bocconea 23, 301–315. Available online at: http://www.herbmedit.org/bocconea/23.pdf#page=301
Dirnböck, T., Essl, F., and Rabitsch, W. (2011). Disproportional risk for habitat loss of high-altitude endemic species under climate change. Glob. Change Biol. 17, 990–996. doi: 10.1111/j.1365-2486.2010.02266.x
Evangelista, P. H., Kumar, S., Stohlgren, T. J., Jarnevich, C. S., Crall, A. W., Norman, J. B. III., et al. (2008). Modelling invasion for a habitat generalist and a specialist plant species. Divers. Distrib. 14, 808–817. doi: 10.1111/j.1472-4642.2008.00486.x
Evans, M. R., Benton, T. G., Grimm, V., Lessells, C. M., O'Malley, M. A., Moustakas, A., et al. (2014). Data availability and model complexity, generality, and utility: a reply to Lonergan. Trends Ecol. Evol. 29, 302–303. doi: 10.1016/j.tree.2014.03.004
Evans, M. R., and Moustakas, A. (2016). A comparison between data requirements and availability for calibrating predictive ecological models for lowland UK woodlands: learning new tricks from old trees. Ecol. Evol. 6, 4812–4822. doi: 10.1002/ece3.2217
Harrison, S. (1999). Local and regional diversity in a patchy landscape: native, alien, and endemic herbs on serpentine. Ecology 80, 70–80. doi: 10.1890/0012-9658(1999)080[0070:LARDIA]2.0.CO;2
Hengl, T., de Jesus, J. M., MacMillan, R. A., Batjes, N. H., Heuvelink, G. B. M., Ribeiro, E., et al. (2014). SoilGrids1km—global soil information based on automated mapping. PLoS ONE 9:e105992. doi: 10.1371/journal.pone.0105992
Hierro, J. L., Maron, J. L., and Callaway, R. M. (2005). A biogeographical approach to plant invasions: the importance of studying exotics in their introduced and native range. J. Ecol. 93, 5–15. doi: 10.1111/j.0022-0477.2004.00953.x
Hijmans, R. J., Cameron, S. E., Parra, J. L., Jones, P. G., and Jarvis, A. (2005). Very high resolution interpolated climate surfaces for global land areas. Int. J. Climatol. 25, 1965–1978. doi: 10.1002/joc.1276
Hulme, P. E., Brundu, G., Camarda, I., Dalias, P., Lambdon, P., Lloret, F., et al. (2008). “Assessing the risks to Mediterranean islands ecosystems from alien plant introductions,” in Plant Invasions: Human Perception, Ecological Impacts and Management, eds B. Tokarska-Guzik, J. H. Brock, G. Brundu, L. Child, C. C. Daehler, P. Pyšek (Leiden: Backhuys Publishers), 39–56.
Jeschke, J. M. (2014). General hypotheses in invasion ecology. Divers. Distrib. 20, 1229–1234. doi: 10.1111/ddi.12258
Katsanevakis, S., Deriu, I., D'Amico, F., Nunes, A. L., Sanchez, S. P., Crocetta, F., et al. (2015). European alien species information network (EASIN): supporting European policies and scientific research. Manag. Biol. Invasions 6, 147–157. doi: 10.3391/mbi.2015.6.2.05
Kim, Y., and Gu, C. (2004). Smoothing spline Gaussian regression: more scalable computation via efficient approximation. J. R. Stat. Soc. B 66, 337–356. doi: 10.1046/j.1369-7412.2003.05316.x
Knops, J. M. H., Griffin, J. R., and Royalty, A. C. (1995). Introduced and native plants of the Hastings Reservation, central coastal California: a comparison. Biol. Conserv. 71, 115–123. doi: 10.1016/0006-3207(94)00008-E
Kolar, C. S., and Lodge, D. M. (2001). Progress in invasion biology: predicting invaders. Trends Ecol. Evol. 16, 199–204. doi: 10.1016/S0169-5347(01)02101-2
Kumschick, S., Gaertner, M., Vilà, M., Essl, F., Jeschke, J. M., Pyšek, P., et al. (2015). Ecological impacts of alien species: quantification, scope, caveats, and recommendations. Bioscience 65, 55–63. doi: 10.1093/biosci/biu193
Legakis, A., and Kypriotakis, Z. (1994). A biogeographical analysis of the Island of Crete, Greece. J. Biogeogr. 21, 441–445. doi: 10.2307/2845761
Legendre, P., and Fortin, M. J. (1989). Spatial pattern and ecological analysis. Vegetatio 80, 107–138. doi: 10.1007/BF00048036
Levine, J. M. (2000). Species diversity and biological invasions: relating local process to community pattern. Science 288, 852–854. doi: 10.1126/science.288.5467.852
Levine, J. M., and D'Antonio, C. M. (1999). Elton revisited: a review of evidence linking diversity and invasibility. Oikos 87, 15–26. doi: 10.2307/3546992
Lockwood, J. L., Cassey, P., and Blackburn, T. (2005). The role of propagule pressure in explaining species invasions. Trends Ecol. Evol. 20, 223–228. doi: 10.1016/j.tree.2005.02.004
Lockwood, J. L., Hoopes, M. F., and Marchetti, M. P. (2013). Invasion Ecology, 2nd Edn. Malden, MA: Wiley-Blackwell.
Lonsdale, W. M. (1999). Global patterns of plant invasions and the concept of invasibility. Ecology 80, 1522–1536. doi: 10.1890/0012-9658(1999)080[1522:GPOPIA]2.0.CO;2
Louca, M., Vogiatzakis, I. N., and Moustakas, A. (2015). Modelling the combined effects of land use and climatic changes: coupling bioclimatic modelling with Markov-chain Cellular Automata in a case study in Cyprus. Ecol. Inform. 30, 241–249. doi: 10.1016/j.ecoinf.2015.05.008
MacArthur, R. (1970). Species packing and competitive equilibrium for many species. Theor. Popul. Biol. 1, 1–11. doi: 10.1016/0040-5809(70)90039-0
Mac Nally, R. (2002). Multiple regression and inference in ecology and conservation biology: further comments on identifying important predictor variables. Biodivers. Conserv. 11, 1397–1401. doi: 10.1023/A:1016250716679
Malavasi, M., Santoro, R., Cutini, M., Acosta, A. T. R., and Laura Carranza, M. (2016). The impact of human pressure on landscape patterns and plant species richness in Mediterranean coastal dunes. Plant Biosyst. 150, 73–82. doi: 10.1080/11263504.2014.913730
Marini, L., Gaston, K. J., Prosser, F., and Hulme, P. E. (2009). Contrasting response of native and alien plant species richness to environmental energy and human impact along alpine elevation gradients. Global Ecol. Biogeogr. 18, 652–661. doi: 10.1111/j.1466-8238.2009.00484.x
Marvier, M., Kareiva, P., and Neubert, M. G. (2004). Habitat destruction, fragmentation, and disturbance promote invasion by habitat generalists in a multispecies metapopulation. Risk Anal. 24, 869–878. doi: 10.1111/j.0272-4332.2004.00485.x
McKinney, M. L. (2002). Influence of settlement time, human population, park shape and age, visitation and roads on the number of alien plant species in protected areas in the USA. Divers. Distribut. 8, 311–318. doi: 10.1046/j.1472-4642.2002.00153.x
Médail, F., and Diadema, K. (2009). Glacial refugia influence plant diversity patterns in the Mediterranean Basin. J. Biogeogr. 36, 1333–1345. doi: 10.1111/j.1365-2699.2008.02051.x
Medail, F., and Quezel, P. (1997). Hot-spots analysis for conservation of plant biodiversity in the Mediterranean Basin. Ann. Missouri Bot. Gard. 84, 112–127. doi: 10.2307/2399957
Melbourne, B. A., Cornell, H. V., Davies, K. F., Dugaw, C. J., Elmendorf, S., Freestone, A. L., et al. (2007). Invasion in a heterogeneous world: resistance, coexistence or hostile takeover? Ecol. Lett. 10, 77–94. doi: 10.1111/j.1461-0248.2006.00987.x
Miyazaki, Y., Teramura, A., and Senou, H. (2016). Biodiversity data mining from Argus-eyed citizens: the first illegal introduction record of Lepomis macrochirus macrochirus Rafinesque, 1819 in Japan based on Twitter information. ZooKeys 569, 123–133. doi: 10.3897/zookeys.569.7577
Mooney, H. A., and Cleland, E. E. (2001). The evolutionary impact of invasive species. Proc. Natl. Acad. Sci. U.S.A. 98, 5446–5451. doi: 10.1073/pnas.091093398
Moustakas, A. (2017). Spatio-temporal data mining in ecological and veterinary epidemiology. Stoch. Environ. Res. Risk Assess. 31, 829–834. doi: 10.1007/s00477-016-1374-8
Moustakas, A., and Evans, M. (2015). Coupling models of cattle and farms with models of badgers for predicting the dynamics of bovine tuberculosis (TB). Stoch. Environ. Res. Risk Assess. 29, 623–635. doi: 10.1007/s00477-014-1016-y
Moustakas, A., and Evans, M. R. (2017). A big-data spatial, temporal and network analysis of bovine tuberculosis between wildlife (badgers) and cattle. Stoch. Environ. Res. Risk Assess. 31, 315–328. doi: 10.1007/s00477-016-1311-x
Niphadkar, M., and Nagendra, H. (2016). Remote sensing of invasive plants: incorporating functional traits into the picture. Int. J. Remote Sens. 37, 3074–3085. doi: 10.1080/01431161.2016.1193795
Ordonez, A., and Olff, H. (2013). Do alien plant species profit more from high resource supply than natives? A trait-based analysis. Global Ecol. Biogeogr. 22, 648–658. doi: 10.1111/geb.12019
Panitsa, M., Tzanoudakis, D., Triantis, K. A., and Sfenthourakis, S. (2006). Patterns of species richness on very small islands: the plants of the Aegean archipelago. J. Biogeogr. 33, 1223–1234. doi: 10.1111/j.1365-2699.2006.01481.x
Papanastasis, V. P., and Kazaklis, A. (1998). “Land use changes and conflicts in the mediterranean-type ecosystems of western Crete,” in Landscape Disturbance and Biodiversity in Mediterranean-Type Ecosystems, eds P. Rundel, G. Montenegro and F. Jaksic (Berlin; Heidelberg: Springer), 141–154.
Peltzer, D. A., Kurokawa, H., and Wardle, D. A. (2016). Soil fertility and disturbance interact to drive contrasting responses of co-occurring native and nonnative species. Ecology 97, 515–529. doi: 10.1890/15-0298.1
Planty-Tabacchi, A.-M., Tabacchi, E., Naiman, R. J., Deferrari, C. and Décamps, H. (1996). Invasibility of species-rich communities in riparian zones. Conserv. Biol. 10, 598–607. doi: 10.1046/j.1523-1739.1996.10020598.x
R Development Core Team (2016). R: A Language and Environment for Statistical Computing. Vienna: R Foundation for Statistical Computing. ISBN 3-900051-07-0.
Reaser, J. K., Meyerson, L. A., Cronk, Q., and De Poorter, M. (2007). Ecological and socioeconomic impacts of invasive alien species in island ecosystems. Environ. Conserv. 34, 98–111. doi: 10.1017/S0376892907003815
Reis, S., Seto, E., Northcross, A., Quinn, N. W., Convertino, M., Jones, R. L., et al. (2015). Integrating modelling and smart sensors for environmental and human health. Environ. Model. Softw. 74, 238–246. doi: 10.1016/j.envsoft.2015.06.003
Richardson, D. M., and Pyšek, P. (2006). Plant invasions: merging the concepts of species invasiveness and community invasibility. Prog. Phys. Geogr. 30, 409–431. doi: 10.1191/0309133306pp490pr
Robinson, G. R., Quinn, J. F., and Stanton, M. L. (1995). Invasibility of Experimental Habitat Islands in a California Winter Annual Grassland. Ecology 76, 786–794. doi: 10.2307/1939344
Rodriguez, L. F. (2006). Can invasive species facilitate native species? evidence of how, when, and why these impacts occur. Biol. Invasions 8, 927–939. doi: 10.1007/s10530-005-5103-3
Ross, L. C., Lambdon, P. W., and Hulme, P. E. (2008). Disentangling the roles of climate, propagule pressure and land use on the current and potential elevational distribution of the invasive weed Oxalis pes-caprae L. on Crete. Perspect. Plant Ecol. Evol. Syst. 10, 251–258. doi: 10.1016/j.ppees.2008.06.001
Sarris, A., Maniadakis, M., Lazaridou, O., and Pirintsos, S. A. (2005). Studying land use patterns in Crete Island, Greece, through a time sequence of landsat images and mapping vegetation patterns. WSEAS Trans. Envir. Dev. 1, 272–279. Available online at: http://emeric.ims.forth.gr/Landuse%20patterns-Vegetation%20mapping%20of%20Crete.pdf
Simberloff, D. (2009). The role of propagule pressure in biological invasions. Annu. Rev. Ecol. Evol. Syst. 40, 81–102. doi: 10.1146/annurev.ecolsys.110308.120304
Souza, L., Bunn, W. A., Simberloff, D., Lawton, R. M., and Sanders, N. J. (2011). Biotic and abiotic influences on native and exotic richness relationship across spatial scales: favourable environments for native species are highly invasible. Funct. Ecol. 25, 1106–1112. doi: 10.1111/j.1365-2435.2011.01857.x
Stohlgren, T. J., Barnett, D. T., and Kartesz, J. T. (2003). The rich get richer: patterns of plant invasions in the United States. Front. Ecol. Environ. 1, 11–14. doi: 10.1890/1540-9295(2003)001[0011:TRGRPO]2.0.CO;2
Stohlgren, T. J., Binkley, D., Chong, G. W., Kalkhan, M. A., Schell, L. D., Bull, K. A., et al. (1999). Exotic plant species invade hot spots of native plant diversity. Ecol. Monogr. 69, 25–46. doi: 10.1890/0012-9615(1999)069[0025:EPSIHS]2.0.CO;2
Stohlgren, T. J., Jarnevich, C., Chong, G. W., and Evangelista, P. H. (2006). Scale and plant invasions: a theory of biotic acceptance. Preslia 78, 405–426. Available online at: http://www.preslia.cz/P064CSto.pdf
Thomson, D. (2005). Measuring the effects of invasive species on the demography of a rare endemic plant. Biol. Invasions 7, 615–624. doi: 10.1007/s10530-004-5853-3
Trigas, P., Panitsa, M., and Tsiftsis, S. (2013). Elevational gradient of vascular plant species richness and endemism in crete–the effect of post-isolation mountain uplift on a continental island system. PLoS ONE 8:e59425. doi: 10.1371/journal.pone.0059425
Turland, N. J., Chilton, L., and Press, J. R. (1993). Flora of the Cretan Area: Annotated Checklist and Atlas. London: The Natural History Museum, HMSO.
Vetaas, O. R., and Grytnes, J.-A. (2002). Distribution of vascular plant species richness and endemic richness along the Himalayan elevation gradient in Nepal. Global Ecol. Biogeogr. 11, 291–301. doi: 10.1046/j.1466-822X.2002.00297.x
Vilà, M., Espinar, J. L., Hejda, M., Hulme, P. E., Jarošík, V., Maron, J. L., et al. (2011). Ecological impacts of invasive alien plants: a meta-analysis of their effects on species, communities and ecosystems. Ecol. Lett. 14, 702–708. doi: 10.1111/j.1461-0248.2011.01628.x
Vilà, M., and López-Darias, M. (2006). Contrasting biogeography of endemic and alien terrestrial species in the Canary Islands. Orsis 21, 91–101. Available online at: https://ddd.uab.cat/pub/orsis/02134039v21/02134039v21p91.pdf
Vogiatzakis, I. N., Griffiths, G. H., and Mannion, A. M. (2003). Environmental factors and vegetation composition, Lefka Ori massif, Crete, S. Aegean. Global Ecol. Biogeogr. 12, 131–146. doi: 10.1046/j.1466-822X.2003.00021.x
Wallace, J. M., and Prather, T. S. (2016). Invasive spread dynamics of Anthriscus caucalis at an ecosystem scale: propagule pressure, grazing disturbance and plant community susceptibility in canyon grasslands. Biol. Invasions 18, 145–157. doi: 10.1007/s10530-015-0997-x
Wood, S. (2006). Generalized Additive Models: An Introduction with R. Boca Raton, FL: Chapman & Hall/CRC.
Keywords: biological invasions, alien species, endemic species, statistical hypothesis testing, data mining, statistical interpretation, island ecosystems, Crete
Citation: Bjarnason A, Katsanevakis S, Galanidis A, Vogiatzakis IN and Moustakas A (2017) Evaluating Hypotheses of Plant Species Invasions on Mediterranean Islands: Inverse Patterns between Alien and Endemic Species. Front. Ecol. Evol. 5:91. doi: 10.3389/fevo.2017.00091
Received: 25 April 2017; Accepted: 21 July 2017;
Published: 07 August 2017.
Edited by:
Juergen Pilz, Alpen-Adria-Universität Klagenfurt, AustriaReviewed by:
Ijaz Hussain, Quaid-i-Azam University, PakistanLuis Gomez, University of Las Palmas de Gran Canaria, Spain
Copyright © 2017 Bjarnason, Katsanevakis, Galanidis, Vogiatzakis and Moustakas. This is an open-access article distributed under the terms of the Creative Commons Attribution License (CC BY). The use, distribution or reproduction in other forums is permitted, provided the original author(s) or licensor are credited and that the original publication in this journal is cited, in accordance with accepted academic practice. No use, distribution or reproduction is permitted which does not comply with these terms.
*Correspondence: Alexander Bjarnason, YnQwMzE3NUBxbXVsLmFjLnVr
Aristides Moustakas, YXJpc21vdXN0YWthc0BnbWFpbC5jb20=