- 1Cornell Lab of Ornithology, Cornell University, Ithaca, NY, United States
- 2Department of Ecology and Evolutionary Biology, Cornell University, Ithaca, NY, United States
- 3Technology for Animal Biology and Environmental Research (TABER), Department of Ecology and Evolutionary Biology, Cornell University, Ithaca, NY, United States
Swallows and martins (Aves: Hirundinidae) are well-studied with respect to their breeding biology, but major aspects of their individual aerial movement behavior and ecology are poorly understood. Atmospheric conditions can strongly influence both the availability and distribution of flying insects that aerial insectivores rely upon. Because aerial insects are often found in distinct clusters within the aerosphere, we wanted to explore whether aerial insectivore flight altitudes were species-specific and if they were associated with atmospheric conditions. We examined these questions with novel tag technology, an altitude datalogger, on breeding populations of Purple Martin (Progne subis), Tree Swallow (Tachycineta bicolor), and Barn Swallow (Hirundo rustica) in upstate New York during the summer of 2016, providing individual-level flight data on a per minute basis. Using mixed models, we investigated differences in flight altitudes between individuals, species, and varying atmospheric conditions. The major findings were that individuals of each species spent significantly different proportions of their time throughout the day in different aerial strata. In addition, higher flying species such as Purple Martins and Tree Swallows responded positively to greater thermal uplift whereas this predictor had no effect on Barn Swallow flight altitudes. Finally, the differing relationships for all species between their flight altitudes and weather variables suggest that each species may use different atmospheric cues for tracking their environment and/or prey. More research spanning greater time scales and a wider range of atmospheric conditions is needed to determine these relationships in finer detail. We encourage broader use of this or similar methodologies to better understand the behavior and ecology of aerial insectivores globally.
Introduction
The aerial ecosystem is a dynamic sub-layer of the troposphere closest to Earth's surface. Despite its global presence, the aerial ecosystem, or aerosphere, has received relatively little attention from ecologists until recent decades. In terms of biomass, the aerosphere is primarily comprised of aerial arthropods, often referred to as “aerial plankton,” since many of its inhabitants have a limited capacity for powered flight and many use prevailing atmospheric conditions as a mechanism for dispersal (Drake and Farrow, 1989; Russell and Wilson, 1996, 1997; Chapman et al., 2010). The major consumers of this abundant yet ephemeral and patchily distributed resource are flying vertebrates such as bats and birds, which spend much of their lives in the aerosphere capturing these prey. Although the breeding biology of some avian aerial insectivores is well-known to the point that they are considered model organisms [e.g., Tree Swallows (Tachycineta bicolor), (Jones, 2003)], documentation of how they interact with the aerial environment and the drivers of their movement behavior is much more scarce. More information on how these taxa interact with the aerosphere is necessary if we are to have a more comprehensive understanding of how environmental variation and ecological dynamics affect their survival and reproduction.
The emergent discipline of aeroecology seeks to understand the physical characteristics of the aerosphere, the organisms that exist and interact within the aerosphere, and the abiotic and biotic selective pressures exerted by the aerosphere on its inhabitants (Kunz et al., 2008; Chilson et al., 2012). While aeroecology is truly interdisciplinary and has drawn upon a wide range of subjects and technologies to grow and firmly establish itself as a discipline in recent decades, most studies that have been conducted thus far have focused primarily on using coarse-scale radar systems to characterize behavior, usually movements or migrations at the population scale (Kunz et al., 2008; Westbrook, 2008; Kelly et al., 2012; Farnsworth et al., 2016; Horton et al., 2016; Shipley et al., 2017a). Furthermore, technological limitations have constrained organismal-level studies of flight behavior in aerial insectivores to the perspective of human observers from the ground (e.g., Collins, 2000; Manchi and Sankaran, 2010; Shipley et al., 2017a). Thus, connecting an organismal-level framework to large scale phenomena is critical to improve the scientific understanding of the aerial ecosystem and the ecology of its inhabitants.
For instance, although weather conditions crucially impact aerial insectivore ecology, the existing framework of knowledge remains surprisingly simple. Theory dictates that temperature mediates daily abundance of insects in the aerosphere, since warmer temperatures drive their phenology and development (Ratte, 1984) and prompt larger emergences of insects (Taylor, 1963). Most flying insects are found within a few meters off the ground, and their abundance drops off exponentially with altitude (Johnson, 1957; Elkins, 2010). Above these first few meters, factors such as wind speed, direction, and convection can scatter or concentrate aerial plankton, creating ephemeral clumps of insects (Pedgley et al., 1990; Elkins, 2010). By contrast, days characterized by colder temperatures, heavy precipitation, and strong winds can greatly suppress the availability of flying insects and have negative consequences for both adult survival and reproductive success in swallows (Winkler et al., 2013), sometimes resulting in mass mortality events for young nestling swallows (Winkler et al., 2013) and even adults (Elkins, 2010). These conditions can also produce a “lake effect” where aerial insectivores must forage low over the surface of large bodies of water to find prey (Newton, 2007; Elkins, 2010). Beyond this paradigm, however, scientific knowledge of how weather affects the flight behavior of flying vertebrates has remained limited in precision and detail.
Our need for a better understanding of aerial insectivore ecology is even more pressing because aerial insectivores as a guild have experienced significant, broad-scale declines in North America and Europe over the past several decades (Sanderson et al., 2006; Nebel et al., 2010; Oresman et al., 2013; Sauer et al., 2014). Decreasing insect populations and changing insect phenology have been hypothesized to be one of several possible drivers of these declines in insectivorous birds (Hallmann et al., 2017). Thus, beginning to understand how insectivorous birds are moving and foraging in the aerosphere is not only imperative to understanding the ecology of this “hidden ecosystem” and the species within it, but it could also begin to provide insight into some of the possible drivers of these precipitous declines.
Given this need, we set out to gain a comparative, organismal level understanding of the daily flight patterns of several avian aerial insectivores. In upstate New York during the summer of 2016, we deployed data loggers on breeding Tree Swallows (Tachycineta bicolor, TRES), Barn Swallows (Hirundo rustica, BARS), and Purple Martins (Progne subis, PUMA), three species in the same family (Hirundinidae) with well-known breeding biologies, which are also declining in eastern North America. Here we use mixed effect models on flight altitude data to investigate two primary questions: (1) whether basic differences exist between individuals as they move and forage in the aerosphere and whether these differences persist at the level of species and (2) how atmospheric conditions such as temperature, turbulence, dew point, boundary layer, and precipitation rate affect the flight altitude of individual swallows. Because the availability of insects can be concentrated by atmospheric conditions, we predicted that variation in flight altitudes will be greater between species than within species, and that atmospheric conditions influence the flight altitudes of individual of all swallows species similarly.
Materials and Methods
We conducted fieldwork from May 22nd to July 20th, 2016, in upstate New York at four locations that support breeding populations of the focal taxa. We sampled Tree Swallows at Unit 1 of the Cornell Experimental Research Ponds in Ithaca (42.505° N, −76.466°W, 335 m) and the Homer C. Thompson Vegetable Research Farm in Freeville (42.518815 N, −76.332651 W, 320 m). We collected data on Purple Martins and Barn Swallows at private residences near Watkins Glen (42.392°N,−76.881°W, 130 m) and Danby (42.288°N, −76.454°W, 395 m), respectively. We collected data on Purple Martins and Barn Swallows at private residences near Watkins Glen (42.392°N,−76.881°W, 130 m) and Danby (42.288°N, −76.454°W, 395 m), respectively. We used specific, known breeding sites for each species to increase the probability of datalogger recapture, but due to the specialized nesting biology of swallows, there was only one study species breeding at a given site. However, all study species were present at every site, with the exception of Purple Martins, which were only found at their breeding site. Moreover, behavioral observations at each site confirmed that all species, especially Purple Martins, frequently ventured outside of the immediate airspace of the sampling localities, so any site-specific effects were limited and likely non-significant. The maximum distance between sites was ~33 km. Our methods consisted of capturing individual birds and attaching to them data loggers (hereafter referred to as “barologgers”) which recorded air temperature and pressure at a frequency of one sample per minute using an onboard low power microprocessor, allowing for near-continuous measurements of flight altitude to the nearest several meters (Shipley et al., 2017b). The barologgers weighed ~450 mg with epoxy weather-proofing and were attached to the birds using Rappole leg loop harnesses (Rappole and Tipton, 1991) made of 0.5 mm Stretch Magic polymer twine. We recaptured birds between 8 and 13 days after tagging (mean = 8.47 ± 1.73 days). Upon recapture, we removed the barologgers and downloaded their data, deriving flight altitudes using the formula below. We corrected for pressure changes by interpolating to the nearest minute from hourly pressure level readings from the nearest airport weather station (KITH) in Ithaca, NY (42.4908°N, −76.4583°W, 335 m).
Where A = barologger altitude (in meters), P0 = sea level pressure (in pascals), P = barologger pressure (in pascals), and T = barologger temperature (in Kelvin).
For meteorological variables, we acquired data on temperature and dewpoint from KITH, data on turbulence kinetic energy (TKE) from the North American Regional Reanalysis (NARR, Mesinger et al., 2006), and data on boundary layer height and precipitation rate from the Env-DATA Track Annotation Service on Movebank (Dodge et al., 2013). The weather data was interpolated to the minute level to conform with the altitudinal data using bilinear interpolation.
Analysis of Individual Altitude Data
Altitudinal Partitioning
To test for differences in the aerial behavior between the three study species, we partitioned the median altitude per flight bout into six different bins relative to time of day. Starting at 5 a.m. local time, data was divided into dawn, morning, midday, afternoon, evening, and night at 180 min increments. Night was not used for analysis. We analyzed the data using a linear model with log(altitude) as the response variable, and time bin and species as predictors. We also performed pairwise comparisons and independent contrasts using Tukey honest significant differences in a post-hoc test.
Atmospheric Effects on Flight Bout Altitude
We also wanted to determine the relationships between flight altitude throughout the day and atmospheric conditions. Because telemetry data tend to demonstrate high degrees of spatial and temporal autocorrelation, we initially checked for autocorrelation calculating the autocorrelation functions for each individual (acf, base R, R Core Team, 2016). Although the characteristics of the autocorrelation relationship can be informative in regard to behavior and movement traits Boyce et al. (2010), we accounted for it in our analyses using two different approaches.
First, we accounted for the temporal autocorrelation using two generalized additive mixed effects models (GAMM) modeled with and without the correlation structure (corAR) fit to the process errors. For each model, the ordinary least squares residuals were used to test for autocorrelation and partial correlation. We compared the naïve model with the corAR model using an information theoretic approach (AICc).
In the second approach, we accounted for temporal autocorrelation by subsetting the data, calculating the median altitude for each flight bout (Figure 1). We then analyzed the relationship between median flight bout altitude and the predictor variables using a generalized additive mixed effects model (GAMM) model. We calculated the autocorrelation function for the median flight bout dataset to estimate the remaining temporal autocorrelation.
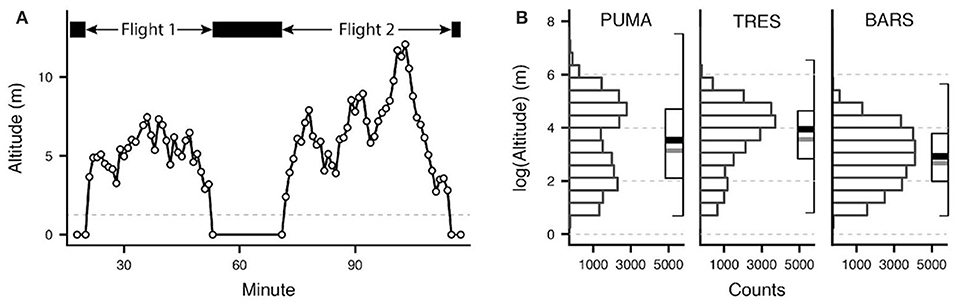
Figure 1. Determination of individual flight bouts and distribution of flight altitudes. In subfigure (A), we considered multiple consecutive data points above an altitude threshold of 2 m (gray dashed line) as an individual flight bout. In the second set of subfigures (B), altitude data from all recorded flight bouts is presented as a histogram and a combined boxplot with the median (thick black line) and the mean (thick gray line). The outer edges of the box represent the IQR.
All generalized additive mixed effects models (GAMMs) were used to compare differences in (log(flight altitudes)) in response to environmental predictor variables. The day of the year, individual identity, and species were included as random effects. Continuous predictor variables were tested for collinearity and those with a Pearson's correlation coefficient (PCC) > 0.5 were excluded from the same model. This resulted in a final predictor set of temperature, thermal uplift, and atmospheric pressure. We analyzed the data with the statistical program R, version 3.3.1 R Core Team (2016).
Animal Ethics and Institutional Approval
All work involving wild animals for this research was approved under protocol 2001-0051 to DW Winkler by the Institutional Animal Care and Use Committee at Cornell University.
Results
Of the 20 barologgers deployed on Tree Swallows, 12 on Barn Swallows, and 7 on Purple Martins, we successfully retrieved 8 from Tree Swallows, 7 from Barn Swallows, and 4 from Purple Martins. On 3 Tree Swallows and 1 Barn Swallow, the barologgers malfunctioned, leaving 5 Tree Swallows and 6 Barn Swallows with usable data. Summary statistics related to logged time recorded, flight altitude, and flight bout durations are summarized in Table 1.
Altitudinal Partitioning
In the linear model, both time bin and species were significant as predictors at a p < 0.05 level. The results of pairwise comparisons and independent contrasts post-hoc test suggested that the greatest differences between species were morning and midday. The complete results are presented in Figure 2, Table 2.
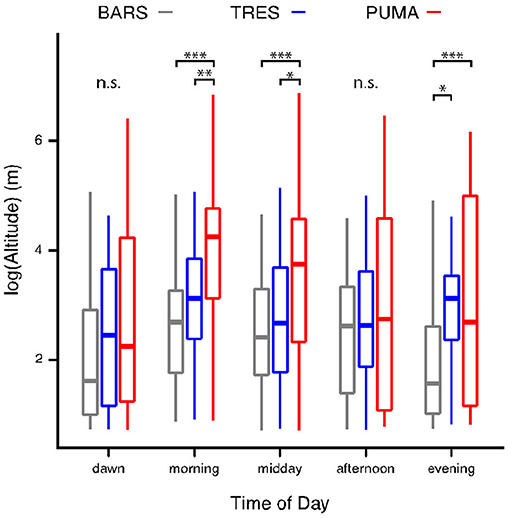
Figure 2. Differences in altitude at different times of day for 3 study species—We compared the median log flight altitude of each flight bout between species using a linear model with pairwise comparison of contrasts using a Tukey HSD test. In 3 out of 5 time bins, there were significant differences between Purple Martins and Barn Swallows. The greatest differences between species occur in morning and midday in this study period. ***p < 0.001, **p < 0.01, *p < 0.05, n.s., non-significant.
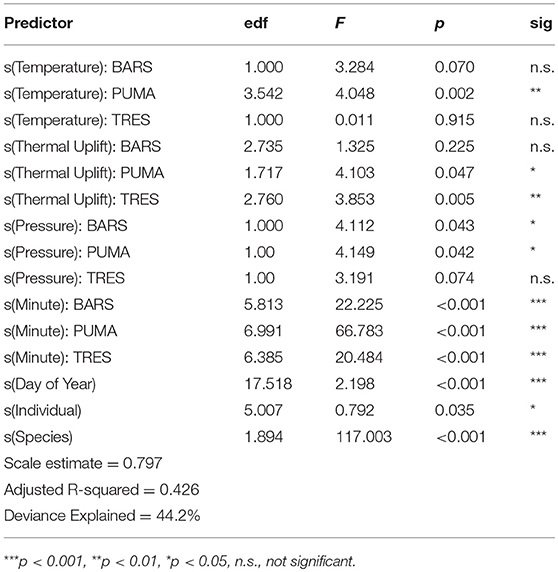
Table 2. Results of the Generalized Additive Mixed Model (GAMM) analyzing flight altitude and atmospheric condition predictors.
Atmospheric Effects on Flight Bout Altitude
In the first set of models on entire flight bout data, the naïve, and corAR process model explained 46.5 and 44.1% of the variation in the data. However, visual inspection of the naïve and corAR model coupled with the plot of acf suggested the persistence of temporal autocorrelation. Thus, we decided to only retain the subsampled dataset with the median altitude for each flight bout for analysis. Results of the mixed effects model suggested that all species flight altitudes were affected by time of day, and higher-flying species (PUMA and TRES) were affected by atmospheric conditions such as thermal uplift (Table 3, Figure 3). In addition, the random effects of both species and day of year were significant as was individual ID. The best model explained 42.6% of the variation in the data and the complete GAMM results are presented in Table 3.
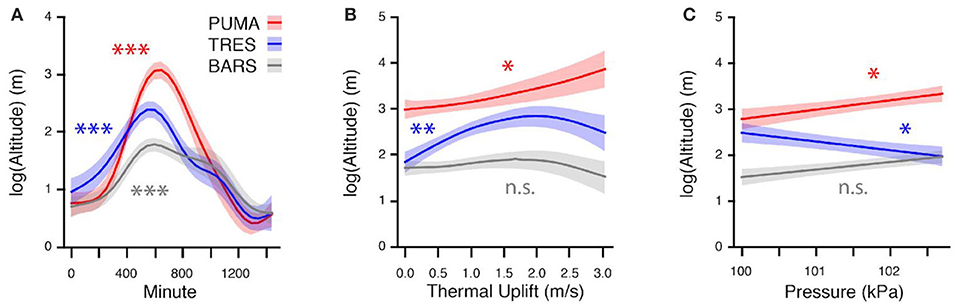
Figure 3. Relationship between predictor variables and flight altitude for three study species. The shaded region represents the standard error for each smooth function estimate. In the first subfigure (A), the relationship between time of day (Minute) and altitude is significant for all three species, with highest altitudes occurring near mid-day. In subfigure (B), Altitude significantly increases with thermal uplift for Purple Martins (PUMA) and Tree Swallows (TRES), but not for Barn Swallows (BARS). In the final subfigure (C), there is no clear relationship between atmospheric pressure and flight bout altitudes, with PUMA and TRES having significant but opposite effects. ***p < 0.001, **p < 0.01, *p < 0.05, n.s., non-significant.
Discussion
Our results demonstrate that individual Barn Swallows, Tree Swallows, and Purple Martins use significantly different altitude strata in their daily aerial movement and foraging, despite considerable variation and plasticity in their aerial behavior (Table 2, Figure 2). Independent contrasts suggested that this was most pronounced during late morning and midday, where Purple Martins were consistently the highest species in our study, followed by Tree Swallows and Barn Swallows, respectively. However, these differences were non-existent both at dawn and in the later afternoon. This suggests that there may be a degree of temporally-driven ecological structure in the aerosphere. Since swallows capitalize on ephemeral clusters of insects in the air Elkins (2010), it follows that differences between species should be minimal unless they are consuming different insects that are found at different altitudinal bands. Aerial insectivores feed opportunistically on a wide variety of insect taxa, but previous research has revealed broad differences in diet between Purple Martins, Tree Swallows, and Barn Swallows (Beal, 1918; Brown and Brown, 1999; Winkler et al., 2011; Brown and Tarof, 2013); moreover, Helms et al. (2016) used tag technology similar to this study on Purple Martins to demonstrate from their diets that insect taxa do in fact have different altitudinal distributions within the aerial environment. Given this, it is intuitive that since aerial insectivores have differentiated diets and since the aerial “plankton” they feed on have distinct altitudinal distributions, then aerial insectivores may partition their foraging into altitudinal bands where they presumably feed on their preferred prey. While more sampling is needed to confirm that these differences truly persist at the species level, this finding represents a novel insight into the ecology of aerial insectivores, and future research should examine dietary differences as a possible explanation for this pattern.
These results also provide novel insight on how prevailing weather conditions can affect the movement behavior of individual avian aerial insectivores. Thermal uplift had a significant, positive effect on median flight bout altitude for the two higher-flying species, Tree Swallows and Purple Martins, but not for Barn Swallows (Table 3, Figure 3). Foraging strategy may be a explanation for this pattern, since Barn Swallows are smaller and more slender, suited for “coursing” by foraging low over grassy fields and meadows to pick off prey just above the ground; they have even been noted to prefer single, large insects over swarms (Brown and Brown, 1999). Therefore, thermal uplift seems less likely to influence their movement and foraging behavior compared with Tree Swallows and Purple Martins. Surprisingly, temperature itself only had a significant, positive effect on the median flight bout altitude of Purple Martins and no other species (Table 3, Figure 3). This is interesting, since it is thought that temperature mediates daily insect emergence and abundance (Taylor, 1963; Ratte, 1984). However, the relationship between aerial insect abundance and temperature has been shown to be non-linear, where lower temperatures suppress aerial insect activity completely (Winkler et al., 2013). In this study, our samples are restricted to the breeding season, which experiences a considerably smaller range of temperatures than (Winkler et al., 2013). Thus, the relationship between temperature and aerial activity of swallows may be weaker than if we had data across a wider range of temperatures. Finally, the time of day did have a significant effect on median flight altitude for all three species, and the effect on median flight bout altitude was most positive at midday when daily temperatures are highest and insects are likely to be at their greatest height in the aerosphere.
Regardless of any specific predictors, the fact that the relationships between median flight bout altitude and weather variables changed significantly depending on time of day and the date demonstrates that individual swallows are likely to use changing environmental conditions to inform their decisions on when and where to fly and forage. Moreover, the differential response of individuals within each species to the same environmental variables suggests that the study taxa may possess different strategies for foraging in response to weather patterns, although more research is needed to confirm whether these are consistent, species-specific differences.
The varying relationships between flight altitudes and weather variables may be further explained by the ever-changing context of cues and strategies influencing the behavior of individual organisms (Winkler et al., 2014). Future research should address endogenous factors such as age, sex, and body condition that are likely to affect movement behavior. More precise weather data may also be necessary to elucidate the relationships between flight altitude and meteorological variables, as the scale of our weather data was both spatially and temporally coarse. Overall, more research is needed to examine these relationships across more individuals with longer sampling periods and a wider breadth of weather conditions. Additionally, a three-dimensional analysis could greatly help to better understand the relationships between meteorological variables and flight behavior in aerial insectivores.
In using newly developed tools to characterize the aerial behavior of three avian aerial insectivores, we have established a precise, broadly applicable method to better understand the ecology of aerial insectivores globally. This method can contribute further to aeroecology by providing high resolution data on the individual lives of large, flying vertebrates that inhabit the aerosphere. We therefore encourage others interested in the behavioral ecology of aerial insectivores to deploy this or similar technologies on a wider breadth of species across a greater geographic and temporal extent and to conduct careful experiments to better understand the effects of life history, geography, and weather on aerial insectivore behavioral ecology.
Data Availability Statement
The data generated and analyzed to support the conclusions of this study is available upon reasonable request.
Author Contributions
All authors conceived and designed the project jointly. JS and DW developed the methodology. RD and JS collected the data. RD and JS formatted and analyzed the data. RD drafted the manuscript. All authors revised the manuscript and approved the version submitted.
Funding
This research was in part funded by a NSF research grant to DW (NSF IBDR 1556138) and a research grant to JS from the Cornell Atkinson Center for a Sustainable Future.
Conflict of Interest Statement
The authors declare that the research was conducted in the absence of any commercial or financial relationships that could be construed as a potential conflict of interest.
Acknowledgments
We thank the 2016 Ithaca field crew of the Golondrinas de las Americas project, in particular friend and field site manager Teresa Pegan, for their assistance deploying the barologgers. We thank homeowners Dave Crans and Janice Beale for allowing us access to their Purple Martins and Barn Swallows, respectively. Dave Crans is due extra thanks for his assistance in capturing Purple Martins and deploying the barologgers. RD thanks Reid Rumelt for his assistance writing R code and Lynn Johnson at Cornell Statistical Consulting Unit for her advice.
References
Beal, F. E. L. (1918). Food Habits of the Swallows: A Family of Valuable Native Birds (No. 619). Washington, DC: US Department of Agriculture.
Boyce, M. S., Pitt, J., Northrup, J. M., Morehouse, A. T., Knopff, K. H., Cristescu, B., et al. (2010). Temporal autocorrelation functions for movement rates from global positioning system radiotelemetry data. Philos. Trans. R. Soc. Lond. B Biol. Sci. 365, 2213–2219. doi: 10.1098/rstb.2010.0080
Brown, C. R., and Brown, M. B. (1999). “Barn Swallow (Hirundo rustica),” in The Birds of North America, ed P. G. Rodewald (Ithaca, NY: Cornell Lab of Ornithology). Available online at: https://birdsna.org/Species-Account/bna/species/barswa
Brown, C. R., and Tarof, S. (2013). “Purple Martin (Progne subis),” in The Birds of North America, ed P. G. Rodewald (Ithaca, NY: Cornell Lab of Ornithology). Available online at: https://birdsna.org/Species-Account/bna/species/purmar
Chapman, J. W., Nesbit, R. L., Burgin, L. E., Reynolds, D. R., Smith, A. D., Middleton, D. R., et al. (2010). Flight orientation behaviors promote optimal migration trajectories in high-flying insects. Science 327, 682–685. doi: 10.1126/science.1182990
Chilson, P. B., Frick, W. F., Kelly, J. F., Howard, K. W., Larkin, R. P., Diehl, R. H., et al. (2012). Partly cloudy with a chance of migration: weather, radars, and aeroecology. Bull. Am. Meteorol. Soc. 93, 669–686. doi: 10.1175/BAMS-D-11-00099.1
Collins, C. T. (2000). Foraging of glossy and pygmy swiftlets in Palawan, Philippine Islands. Forktail 16, 53–55.
Dodge, S., Bohrer, G., Weinzierl, R., Davidson, S. C., Kays, R., Douglas, D., et al. (2013). The environmental-data automated track annotation (Env-DATA) system: linking animal tracks with environmental data. Mov. Ecol. 1:3. doi: 10.1186/2051-3933-1-3
Drake, V. A., and Farrow, R. A. (1989). The ‘aerial plankton’ and atmospheric convergence. Trends Ecol. Evol. 4, 381–385.
Farnsworth, A., Van Doren, B. M., Hochachka, W. M., Sheldon, D., Winner, K., Irvine, J., et al. (2016). A characterization of autumn nocturnal migration detected by weather surveillance radars in the northeastern USA. Ecol. Appl. 26, 752–770. doi: 10.1890/15-0023
Hallmann, C. A., Sorg, M., Jongejans, E., Siepel, H., Hofland, N., Schwan, H., et al. (2017). More than 75 percent decline over 27 years in total flying insect biomass in protected areas. PLoS ONE 12:e0185809. doi: 10.1371/journal.pone.0185809
Helms, J. A., Godfrey, A. P., Ames, T., and Bridge, E. S. (2016). Predator foraging altitudes reveal the structure of aerial insect communities. Sci. Rep. 6:28670. doi: 10.1038/srep28670
Horton, K. G., Van Doren, B. M., Stepanian, P. M., Farnsworth, A., and Kelly, J. F. (2016). Seasonal differences in landbird migration strategies. The Auk 133, 761–769. doi: 10.1642/AUK-16-105.1
Johnson, C. G. (1957). The distribution of insects in the air and the empirical relation of density to height. J. Anim. Ecol. 26, 479–494. doi: 10.2307/1760
Jones, J. (2003). Tree Swallows (Tachycineta bicolor): a new model organism? Auk 120, 591–599. doi: 10.1642/0004-8038(2003)120[0591:TSTBAN]2.0.CO;2
Kelly, J. F., Shipley, J. R., Chilson, P. B., Howard, K. W., Frick, W. F., and Kunz, T. H. (2012). Quantifying animal phenology in the aerosphere at a continental scale using NEXRAD weather radars. Ecosphere 3, 1–9. doi: 10.1890/ES11-00257.1
Kunz, T. H., Gauthreaux, S. A., Hristov, N. I., Horn, J. W., Jones, G., Kalko, E. K., et al. (2008). Aeroecology: probing and modeling the aerosphere. Integr. Comp. Biol. 48, 1–11. doi: 10.1093/icb/icn037
Manchi, S. S., and Sankaran, R. (2010). Foraging habits and habitat use by Edible-nest and Glossy swiftlets in the Andaman Islands, India. Wilson J. Ornithol. 122, 259–272. doi: 10.1676/09-144.1
Mesinger, F., Di Mego, G., Kalnay, E., and Mitchell, K. (2006). North American regional reanalysis. Bull. Am. Meteorol. Soc. 87:343. doi: 10.1175/BAMS-87-3-343
Nebel, S., Mills, A., McCracken, J., and Taylor, P. (2010). Declines of aerial insectivores in North America follow a geographic gradient. Avian Conserv. Ecol. 5:1. doi: 10.5751/ACE-00391-050201
Newton, I. (2007). Weather related mass mortality events in migrants. Ibis 149, 453–467. doi: 10.1111/j.1474-919X.2007.00704.x
Oresman, S., McCracken, J., Bull, M., Winkler, D., Rubega, M., Kearney-McGee, S., et al. (2013). The Seventh Habitat and the Decline of Our Aerial Insectivores. Fairfield, CT: Connecticut State of the Birds.
Pedgley, D. E., Scorer, R. S., Purdom, J. F. W., Simpson, J. E., Wickham, P. G., Dickison, R. B. B., et al. (1990). Concentration of flying insects by the wind [and discussion]. Philos. Trans. R. Soc. Lond. B Biol. Sci. 328, 631–653. doi: 10.1098/rstb.1990.0133
R Core Team (2016). R: A Language and Environment for Statistical Computing. Vienna: R Foundation for Statistical Computing. Available online at: https://www.R-project.org/
Rappole, J. H., and Tipton, A. R. (1991). New harness design for attachment of radio transmitters to small passerines (Nuevo Diseño de Arnés para Atar Transmisores a Passeriformes Pequeños). J. Field Ornithol. 62, 335–337.
Ratte, H. T. (1984). “Temperature and insect development,” in Environmental Physiology and Biochemistry of Insects, ed K. H. Hoffman (Berlin; Heidelberg: Springer), 33–66.
Russell, R. W., and Wilson, J. W. (1997). Radar-observed “fine Lines” in the optically clear boundary layer: reflectivity contributions from aerial plankton and its predators. Boundary Layer Meteorol. 82, 235–262.
Sanderson, F. J., Donald, P. F., Pain, D. J., Burfield, I. J., and Van Bommel, F. P. (2006). Long-term population declines in Afro-Palearctic migrant birds. Biol. Conserv. 131, 93–105. doi: 10.1016/j.biocon.2006.02.008
Sauer, J. R., Hines, J. E., Fallon, J. E., Pardieck, K. L., Ziolkowski, D. J. Jr., and Link, W. A. (2014). The North American Breeding Bird Survey, Results and Analysis 1966 - 2013. Version 01.30.2015 (Laurel, MD: USGS Patuxent Wildlife Research Center).
Shipley, J., Ryan, K., Dreelin, J., Andrew, R., and Winkler, D. W. (2017a). An open-source sensor-logger for recording vertical movement in free-living organisms. Meth. Ecol. Evol. 9, 465–471. doi: 10.1111/2041-210X.12893
Shipley, J. R., Kelly, J. F., and Frick, W. F. (2017b). Toward integrating citizen science and radar data for migrant bird conservation. Remote Sens. Ecol. Conserv. 4, 127–136. doi: 10.1002/rse2.62
Taylor, L. R. (1963). Analysis of the effect of temperature on insects in flight. J. Anim. Ecol. 32, 99–117.
Westbrook, J. K. (2008). Noctuid migration in Texas within the nocturnal aeroecological boundary layer. Integr. Comp. Biol. 48, 99–106. doi: 10.1093/icb/icn040
Winkler, D. W., Hallinger, K. K., Ardia, D. R., Robertson, R. J., Stutchbury, B. J., and Cohen, R. R. (2011). “Tree Swallow (Tachycineta bicolor),” in The Birds of North America, ed P. G. Rodewald (Ithaca, NY: Cornell Lab of Ornithology). Available online at: https://birdsna.org/Species-Account/bna/species/treswa
Winkler, D. W., Jørgensen, C., Both, C., Houston, A. I., McNamara, J. M., Levey, D. J., et al. (2014). Cues, strategies, and outcomes: how migrating vertebrates track environmental change. Mov. Ecol. 2:10. doi: 10.1186/2051-3933-2-10
Keywords: aerial insectivore, aeroecology, flight, behavior, ecology, swallow, Hirundinidae, datalogger
Citation: Dreelin RA, Shipley JR and Winkler DW (2018) Flight Behavior of Individual Aerial Insectivores Revealed by Novel Altitudinal Dataloggers. Front. Ecol. Evol. 6:182. doi: 10.3389/fevo.2018.00182
Received: 31 July 2018; Accepted: 23 October 2018;
Published: 15 November 2018.
Edited by:
Thomas Wassmer, Siena Heights University, United StatesReviewed by:
Adrian C. Gleiss, Murdoch University, AustraliaKathryn Battle, Conservation International, United States
Copyright © 2018 Dreelin, Shipley and Winkler. This is an open-access article distributed under the terms of the Creative Commons Attribution License (CC BY). The use, distribution or reproduction in other forums is permitted, provided the original author(s) and the copyright owner(s) are credited and that the original publication in this journal is cited, in accordance with accepted academic practice. No use, distribution or reproduction is permitted which does not comply with these terms.
*Correspondence: R. Andrew Dreelin, cmFkMzM3QGNvcm5lbGwuZWR1