- 1Faculty of Biology, Altay State University, Barnaul, Russia
- 2Department of Geography and Planning, St. Cloud State University, St. Cloud, MN, United States
- 3Archaeometry Center, Kazan Federal University, Kazan, Russia
Background and Aims: Reconstruction of past ecosystems requires a robust understanding of modern deposition patterns and taphonomy for the proxies utilized. Recent advances in phytolith analysis have contributed to improved understanding of these processes, but many gaps remain. This study aims to test a few specific hypotheses that have been proposed by research outside the tropics in the Northern Hemisphere. Our study area focuses on the Northern Altay, a culturally important region, entirely within Russia, north of China, and Mongolia. We collected 60 phytolith assemblages from modern soils at 300 to 2,300 m a.s.l. elevations, sampled from 20 plots in triple replicates within 13 different plant communities. Detailed releves of these plant communities, including forests, meadows, steppe, and alpine tundra, were obtained during the summer of 2017. We used a locally derived scheme of V. P. Sedelnikov to assign studied communities to ecological categories based on moisture and temperature availability.
Methods: Standard oxidation and heavy liquid flotation methods of extraction were used. Morphotypes were counted under 400–1,000x magnification on an optical microscope. We used a two-tier approach to phytolith morphotypes classification: a detailed one with over 40 morphotypes included and a shorter one with only sums of selected morphotypes. The former approach can produce some interesting results, such as using various types of rondels (e.g., pyramidal vs. keeled) or large vs. small lanceolate (trichomes). Using sums may be more widely applicable, because the researchers can replicate these results better and less training is needed. However, there are fewer diagnostic options with the sums approach.
Key Results: Our results, using discriminant analysis, canonical correspondence analysis and other multivariate statistical methods, confirm earlier studies, both in the region and elsewhere that despite redundancy in phytolith distributions in soils, there are some selected morphotypes that can reliably distinguish communities at various positions along elevational, moisture, and temperature gradients. We developed a regionally diagnostic key that allows researchers to quickly identify various plant communities based on their phytolith assemblages in soils.
Conclusions: Seven of 13 regionally important communities at medium elevations in the Altay Mountains can be distinguished by using aggregated and more detailed phytolith morphotypes.
Introduction
Despite much recent progress in describing modern phytolith assemblages from temperate soils around the world (Blinnikov et al., 2013; McCune and Pellatt, 2013; Traoré et al., 2015; Gavrilov and Loyko, 2016; Lada, 2016; Feng et al., 2017; Gao et al., 2018), including the Russian Altay (Speranskaja et al., 2018), a number of issues persist that hamper phytolith use in the identification of past communities. First, many morphotypes are highly redundant and are found across many communities of various composition at similar concentrations (Blinnikov, 1994, 2005; Fredlund and Tieszen, 1994). Second, almost all studies done in temperate regions demonstrate much lower phytolith accumulation under forests as compared to grasslands (Beavers and Stephen, 1958; Verma and Rust, 1969; Volkova et al., 1995; Hyland et al., 2013) and this has to be accounted for in the interpretation of paleoassemblages. Third, outside of grasses, sedges, conifers, ferns, and sunflower families, few truly diagnostic forms exist that allow unequivocal identification of taxa in the temperate regions, although “trees and shrubs” as a rule can be detected (Blinnikov, 1994, 2005; Yost et al., 2013; McCune et al., 2015). Fourth, conifers produce phytoliths that may be easily confused with grasses (Klein and Geis, 1978; An, 2016) and this may lead to misinterpretation of some assemblages. Fifth, certain morphotypes demonstrate higher solubility in sediments and may be therefore underrepresented in the paleoassemblages (Cabanes et al., 2011).
Our earlier paper (Speranskaja et al., 2018) demonstrated utility of phytoliths in differentiating forests, meadows, and steppes in the Altaysky Kray region of Russia, in the lowlands. This study uses a new dataset collected from the neighboring and considerably more mountainous Republic of Gorny Altay, a culturally important region of the world (Reich et al., 2010). We use bi-level classification of phytoliths across the main elevational gradient (and associated temperature and moisture gradients) to complement the previous study from the foothills and the plains. The following fresearch questions were investigated:
1) Are phytolith assemblages within the same community statistically more similar to each other than to any other communities (including communities of the same type elsewhere)?
2) Can bi-level morphotype classification improve our ability to detect specific plant communities (e.g., can we use specific types of rondels or lanceolate forms to distinguish communities)?
3) Which morphotypes or sums of morphotypes are able to best distinguish communities across the gradient spanning 2,000 m of elevation difference? Conversely, which morphotypes or sums of morphotypes are redundant? Which communities have the most distinct phytolith record in modern soils and which have the least?
4) What is the assemblage sensitivity to temperature and moisture? Do common phytolith indices such as D/P or Ic developed for the tropics work in the temperate region? Can we modify them to allow detection of important climatic or vegetational thresholds of change despite having few C4 grasses and no tropical vegetation in the region?
Materials and Methods
Area of Study
Sixty samples of modern topsoil were collected from 20 different sites (three replicates per site, same vegetation) in the northern Republic of Altay, Russia in the summer of 2017 (Figure 1). Samples were collected from four mountain ranges comprising Northern Altay within the Republic of Gorny Altay in Russia: Anuy (highest point 1,815 m a.s.l.), Iolgo (highest point 2,618 m a.s.l.), Seminsky (highest point 2,507 a.s.l.), and Cherginsky (highest point 2,014 m a.s.l.) (Table 1).
The mean January temperature ranges from −28 to −16°C and the mean July temperature ranges from +8 to +20°C. The mean annual precipitation ranges from 500 mm at lower elevations to 800 mm at higher elevations, especially on the slopes with western exposure. The elevations range from 340 to 2,400 m a.s.l. Elevation and locational data were obtained in the field using a hand-held Garmin GPS unit to ~5 m horizontal accuracy and corrected using topographic maps, as necessary. Temperature of the warmest month (July mean 1970–2017) and mean precipitation values (1970–2017) were estimated using a proprietary gridded GIS dataset of the Altay State University physical geography and GIS department derived from both published and unpublished sources, and estimates of local orographic variation. Additionally, we relied on a locally derived scheme of Sedelnikov (1988), who classified all habitats in our region into a few distinct classes of long-term temperature and moisture regimes ranging from warm to cool to cold and from wet to semi-wet to semi-dry to dry. To assign site to a class, we performed vegetation community identification based on plant composition, visual appearance, and slope position. The advantage of using this classification is that it reflects a long-term climatic signal of each site (e.g., hydric vs. xeric).
Twenty geobotanical relevees and herbarium sampling of all graminoids and many forbs were obtained, one for each site. Plant cover was estimated to about 5% accuracy, and below that value only presence was noted. When plant identification in the field was not sufficient, additional identification was made using botanical keys at the herbarium of Altay State University. Thirteen different plant communities were sampled: pine, spruce, larch, birch-larch, and Siberian cedar pine forests; dry meadow and steppe meadows; true steppe and meadow-steppe; subalpine meadow, alpine meadow, alpine birch-heath, and alpine heath. The data on phytolith production in plants from the region are available from the research team, but were published elsewhere (Speranskaja et al., 2018).
At each site, called Bigplot in the Results section, all above ground plant diversity was described by percent cover in mid-July of 2017, the peak flowering season (Table 1). Aggregated random pinch samples of the upper 1 cm of topsoil, cleared of all litter, were collected within each site on three 10 × 10 m plots randomly chosen within the larger site. Approximately 10 g of dry soil was collected. Care was taken not to include any large plant pieces. Soil was dried and sieved in the lab at a coarse sieve to remove smaller fragments of plant matter before being subject to chemical treatment.
Lab Procedures
Lab processing followed procedure of Golyeva (2007) as modified in Speranskaja et al. (2018) ~40 g of soil was boiled in 15% hydrochloric acid for 1 h to destroy carbonates and most organics. After that, the residue was cooled to 20°C and sand was removed by rapid sieving through a 250 micron sieve and settling for 30 s to the 15 cm depth in a graduated cylinder. The residue below the 15 cm mark in the cylinder and on the sieve was mainly sand fraction and was discarded. The remaining suspension of clay and silt fractions was then subjected to a few cycles of gravity sedimentation and decantation to remove suspended clays near the top (after 3 h, repeated 3–7 times) and the pH was neutralized. Phytoliths were floated in a heavy liquid solution of CdI2 and KI at 2.3 g/cm3. The samples were mixed thoroughly with a glass rod and centrifuged for 10 min at a slow speed (~1,000 rpm). The floated phytoliths were collected by a Pasteur pipette from the top 5 mm of the solution, transferred to clean test tubes, sunk by adding distilled water in proportion of 3:1, and dried. The phytolith-rich residue was stored dry in glass vials.
Phytolith abundance was estimated as percent of the original dry weight of the soil sample. Phytoliths were counted in immersion oil under an Olympus optical microscope (x400-x1000) to examine 3D shapes under rotation. Between 500 and 600 phytoliths were counted per sample. Phytolith morphotypes were documented by light microphotographs and permanent reference slides.
All identifiable phytoliths larger than 10 μm were counted, not only short cells (rondels, bilobates, polylobates, and saddles), but also long cells and other grains of identifiable shape. We followed the classification system of Blinnikov (2005), originally modified from Mulholland (1989), and Fredlund and Tieszen (1994), in describing grass morphotypes; and Bozarth (1992) and Piperno (2006) in describing non-grass morphotypes (Figure 2). We also provide descriptions following the Glossary for the International Code for Phytolith Nomenclature 1.0 (Madella et al., 2005) for each morphotype.
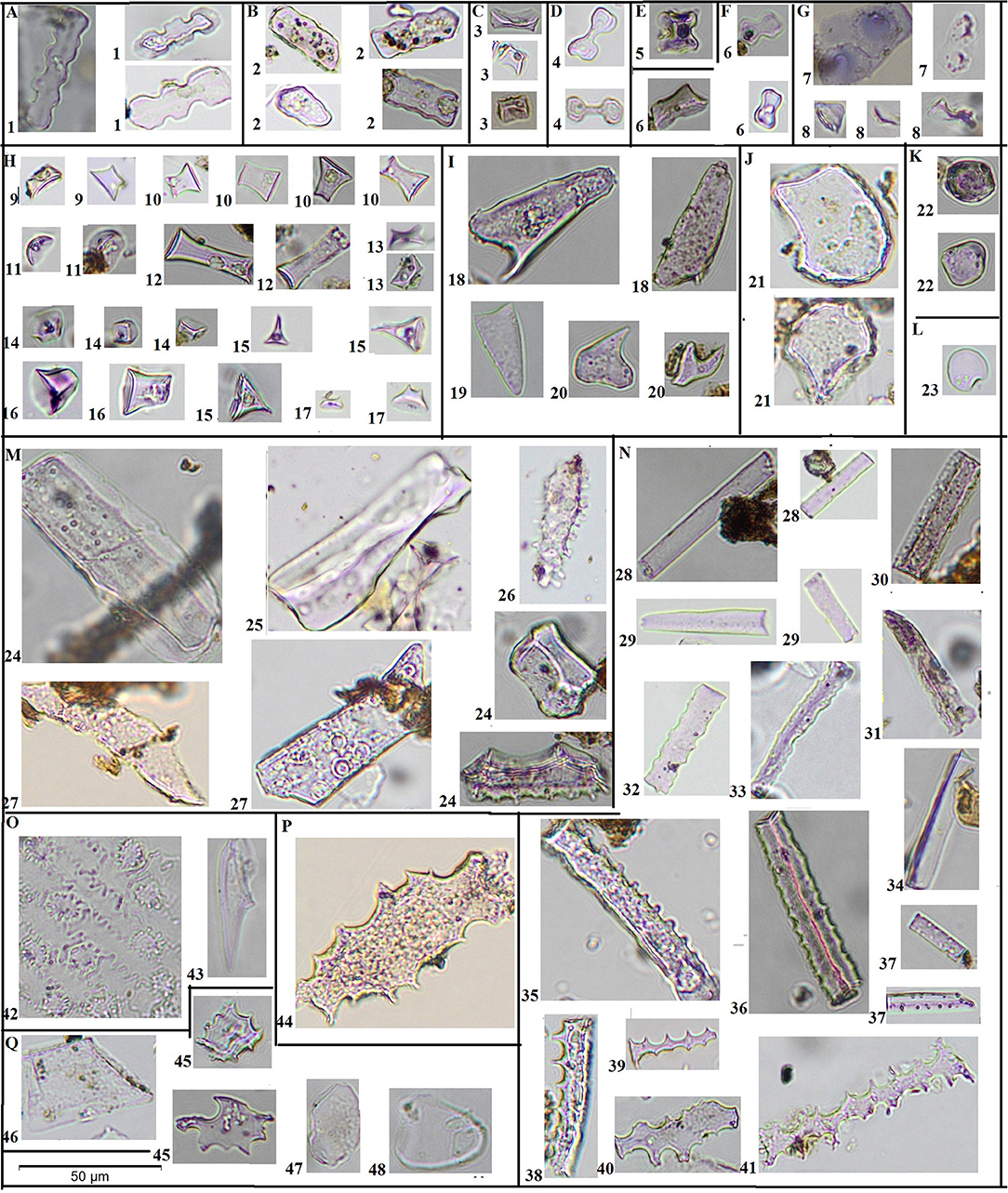
Figure 2. Microphotographs of morphotypes. Microphotographs of all phytolith morphotypes used in this study. Scale bar = 50 μm and applies to all photographs. A1—polylobate trapeziforms; B2—wavy plates; C3—saddles; D4—True bilobates (Panicoid); E5—cross (symmetrical quadrilobate); F6—trapeziform bilobate (“Stipa-type”); G7 conical of Carex, G8—conical with wavy bottom, H—rondels, including H9—low conical rondel, H10—tall conical rondel, H11—spherical bottom rondel, H12—elongated rondel; H13—saddle-top rondel; H14—low trapezoid (pyramidal) rondel; H15—single-keeled rondel; H16—tall trapezoid (pyramidal) rondel; H17—multiple keeled rondel; I—lanceolates (trichomes), including I18—with large base and short awn; I19—triangular, I20—with small base and long awn; J21—bulliform cells; K22—globular irregular; L23 spherical; M—conifer, including M24—multiangled ribbed; M25—blocky with pores (cf. conifer); M26—club-shaped with protrusions (cf. Pinus); M27—conifer tracheids; N—long cells of grasses (possibly some belong to sedges), including N28—psilate symmetrical; N29—psilate asymetrical; N30—psilate ribbed; N31 psilate ribbed asymmetrical; N32—psilate wavy symmetical; N33—psilate wavy asymmetrical; N34—three-angled; N35—papillate; N36—slightly indented; N37—perforated; N38—strongly indented symmetrical; N39—strongly indented asymmetrical; N40—dendritic asymmetrical; N41—dendritic symmetrical; O42—cuticle casts (not used in analysis); O43—microhairs; P44—irregular dentate; Q45—jigsaw dicot epidermis; Q46—angled dicot epidermis; Q47—irregular plates; Q48—oval plates.
Statistical Analyses
Assemblages were assessed at two different levels: most detailed (55 morphotypes; Figure 2) and less detailed (22 morphotypes, including sums of rondels, long cells, and lanceolates; Figure 3). The former classification required more careful identification by the analyst under the microscope. The latter classification was easier for other analysts to replicate (for example, instead of 8 different rondel types only the sum of all rondels was used). Whenever possible, we followed ICPN 1.0 (Madella et al., 2005) in describing morphotypes. In some samples, a limited number of large fragments of silicified epidermis were encountered. We did not include them in the analysis.
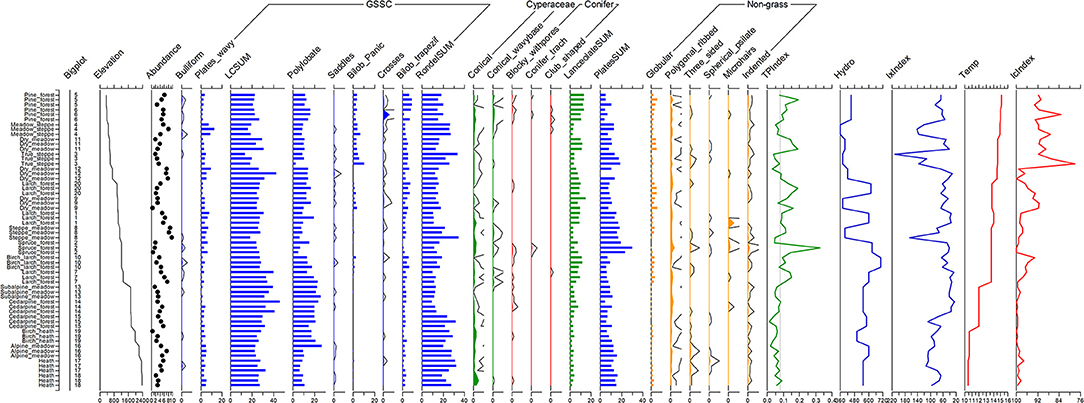
Figure 3. Diagram of phytoliths at the aggregated level of classification (sums of rondels, long cells, lanceolates used). Bigplot numbers correspond to sampling locations in Figure 1. Elevation is in m a.s.l. Abundance of phytoliths is expressed as % of phytolith extract relative to the original dry weight of each sample. All morphotype values are in % of the total sum of all counted phytoliths per sample (between 500 and 600 were counted). GSSC—phytoliths of grass silica short cells. LCSum is the sum of all long cells. TP, Ic, and Ix indices are based on phytolith data and are explained in text. Temp and hydro regime values are based on the scheme of Sedelnikov (1988) and are explained in text.
Canonical correspondence analysis (CCA) of multivariate aggregated morphotype data (ter Braak, 1986) was carried out with the help of paleontological statistics software (PAST 3.20; Hammer et al., 2001). CCA is a direct gradient analysis method, with three parametric environmental variables (elevation, temperature of the warmest month, and mean annual precipitation) used in our study to simultaneously ordinate both morphotypes and samples in one hyperspace. CCA assumes unimodal distribution of species (morphotypes, in our study) along an environmental gradient, which is true for almost all morphotypes in our study (Figure 3). CCA advantage over Principal Components Analysis is that the former allows direct assessment of each environmental factor contribution to the morphotype and site distribution, and also detrends values along each axis, thus removing arching of resulting scores, a problem with PCA.
Cluster analysis, discriminant analysis, and mixed-effects model using percentages of phytoliths was performed using MINITAB version 18 (MINITAB version 18., 2018). C2 data analysis software (Juggins, 2003) was used to plot the pseudo-stratigraphic diagram of phytolith frequency data. Discriminant analysis (Manly, 2004, ch. 8) classifies samples into groups, when you have a sample with known groups. In our case, groups were communities, and we used 22 aggregated morphotypes as predictors. The squared distance (the Mahalanobis distance) of sample x to the center (mean) of group t for linear discriminant is given by the following general form:
where x is a sample, mt is column vector of length p containing the means of the predictors calculated from the data in group t, and Sp is pooled covariance matrix for linear discriminant analysis.
Results
Common Morphotypes and Phytolith Abundance
We primarily relied on the 22 aggregated phytolith morphotypes in reporting the results (Figure 3). Some inferences are made from the more detailed set of 55 morphotypes later in the paper. Of the aggregated morphotypes, 10 were explicitly grass morphotypes, while the rest were conifer, sedge, fern, or dicot tree, and shrub morphotypes. Lanceolate forms (trichomes) and some long cells can be produced by both grasses and sedges. All phytolith counts are reported as percentages of the total. The estimates of phytolith abundance are shown on Figures 3, 4. Percentage of phytoliths extracted relative to dry weight of the original samples were the highest for steppe meadow (mean = 9.0%, n = 3), 2nd highest for meadow steppe (6%), 3rd highest for alpine meadow (5.7%), and the lowest for spruce swamp (1.4%). Most communities had values between 2 and 5%. The difference in phytolith extract means between communities is significant using one-way ANOVA test (F = 4.84, p < 0.001).
The most common aggregated morphotypes were long cells (mean value = 27.3%), rondels (mean value = 19.2%), trapeziform polylobates (13.0%), all plates except wavy (11.7%), lanceolates (6.7%), wavy plates (4%), trapeziform bilobates (3.75%), globular blocky (2.6%), true “Panicoid” bilobates (1.5%), and conical of Carex (1%). All other morphotypes, including some taxonomically important, such as conifer tracheids, Panicoid crosses, or bulliform cells of grasses, had mean values <1%. As expected, non-grass morphotypes were always a small minority of the total assemblage, even in wet spruce forest with sedges and very few grasses, or in pine forest with a heavy presence of ferns.
Are Phytoliths on Local Plots More Similar to Each Other, Than to Other Communities?
We ran a MANOVA test using Bigplot as the model factor and 22 morphotypes as responses. The results were highly significant with Wilks' lambda F = 3.033 (p < 0.001) and Lawley-Hotteling F = 4.883 (p < 0.001). Mean values for the three local plots within each community (20 “big plots”) were more similar to each other than to all other means. Not all morphotypes were statistically significant contributors to this effect. Wavy plates, polylobate trapezoids, bilobate Panicoid, bilobate trapeziform, rondels, conical, lanceolate, long cells, plates, and globular morphotypes were highly significant at p < 0.001. Conifer phytoliths, spherical psilate, and three-sided forms were less significant at p < 0.05. Rarer forms were significant, probably because they were only found in a few plots. For example, bulliform cells and saddles, both of which can be very important indicators of ecological conditions in other regions (Fredlund and Tieszen, 1994; Brémond et al., 2005b), were very scarce in our study.
Discriminant Analysis
Discriminant analysis (DA) indicated that all 13 communities in our study could be distinguished based on their soil phytolith assemblages (Table 2). Of the 60 samples, 57 (or 95%) were correctly classified when using the aggregated classification with 22 morphotypes. Of the three misclassified samples, one was a dry meadow misclassified as larch forest, and two were larch forest samples classified as a dry meadow. These two communities have the shortest squared Mahalanobis distance of 9.516 in the set compared to the longest distance of 214.558 between pine forest and alpine meadow. Based on DA, the most similar assemblages are produced by: (a) the group of high-elevation communities including alpine meadow, alpine heath, and subalpine birch; (b) Siberian cedar pine forest (typically found near the upper treeline) and subalpine meadow (intermediate elevations); and finally (c) true steppe and meadow steppe of low elevations. Spruce forest and pine forest appear individually as distinct assemblages with longer distances to other, because they have unique conifer and moss morphotypes.
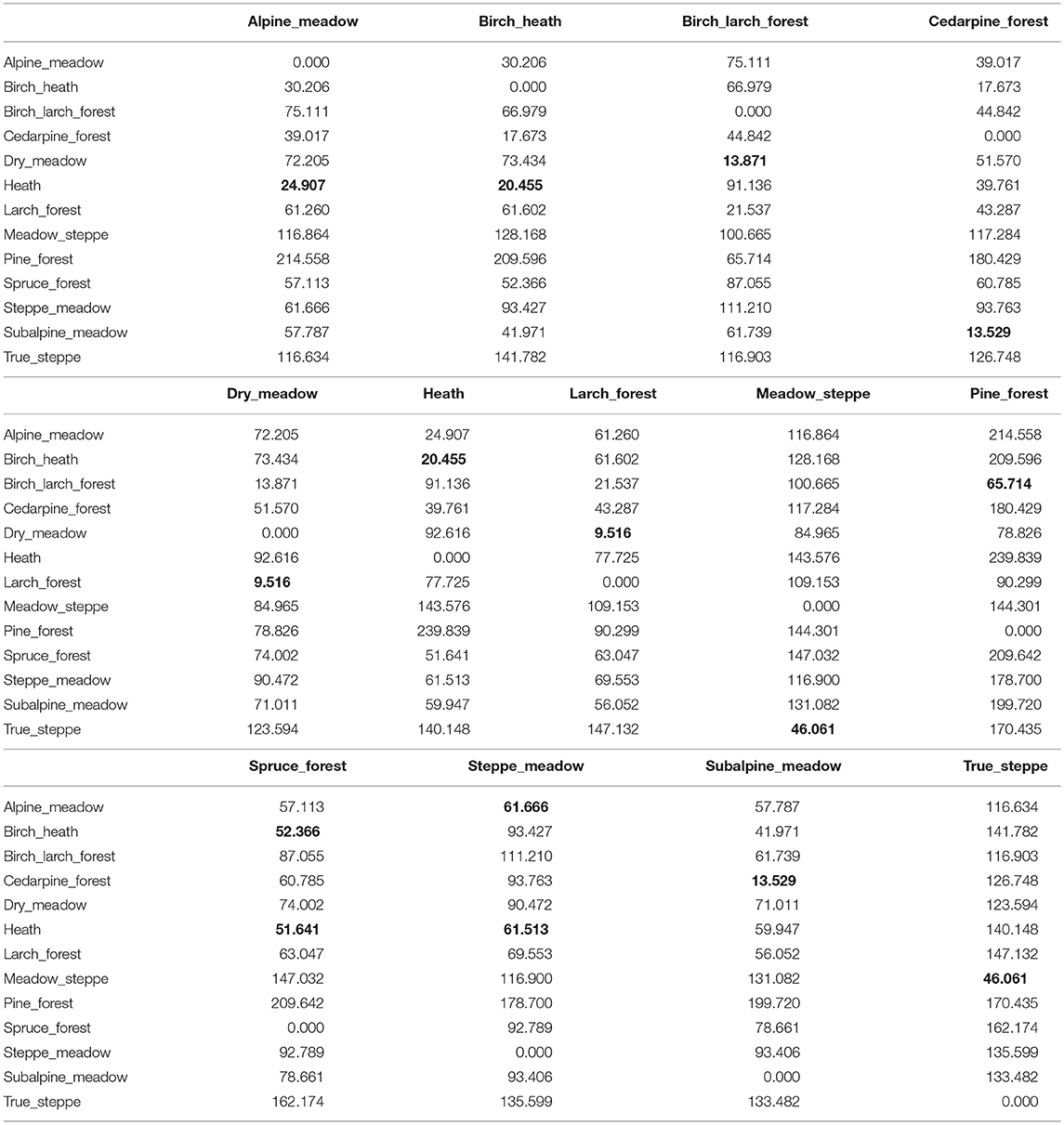
Table 2. Results of discriminant analysis performed on aggregated phytolith morphotype percentages using squared Mahalanobis distance between groups (shortest distance in bold shows most similar assemblages).
The linear discriminant function (Table 3) suggested that the following morphotypes were important for distinguishing plant community types: three-sided forms—alpine meadows, conical—birch heaths and heaths, spherical psilate—heaths, lanceolate sum of grasses and sedges—birch-larch forest, conifer tracheids—dry meadow (see Discussion for possible explanation), globular and polygonal ribbed—larch forest, indented irregular of dicots—meadow steppe, a few different forms including conifer with pores and club-shaped—pine forest, conical, and coniferous tracheids—spruce forest, saddles, and conical with wavy base—steppe meadow, and trapeziform and true bilobates, as well as crosses and sum of all plates—true steppe. Subalpine meadows and cedar pine forests did not have one predominately discriminant form. However, these two assemblages were similar to each other. It is important to note that the Siberian cedar pine community sampled near the treeline in our study produces few phytoliths, and its forest understory is frequently similar to that of the surrounding subalpine meadows.
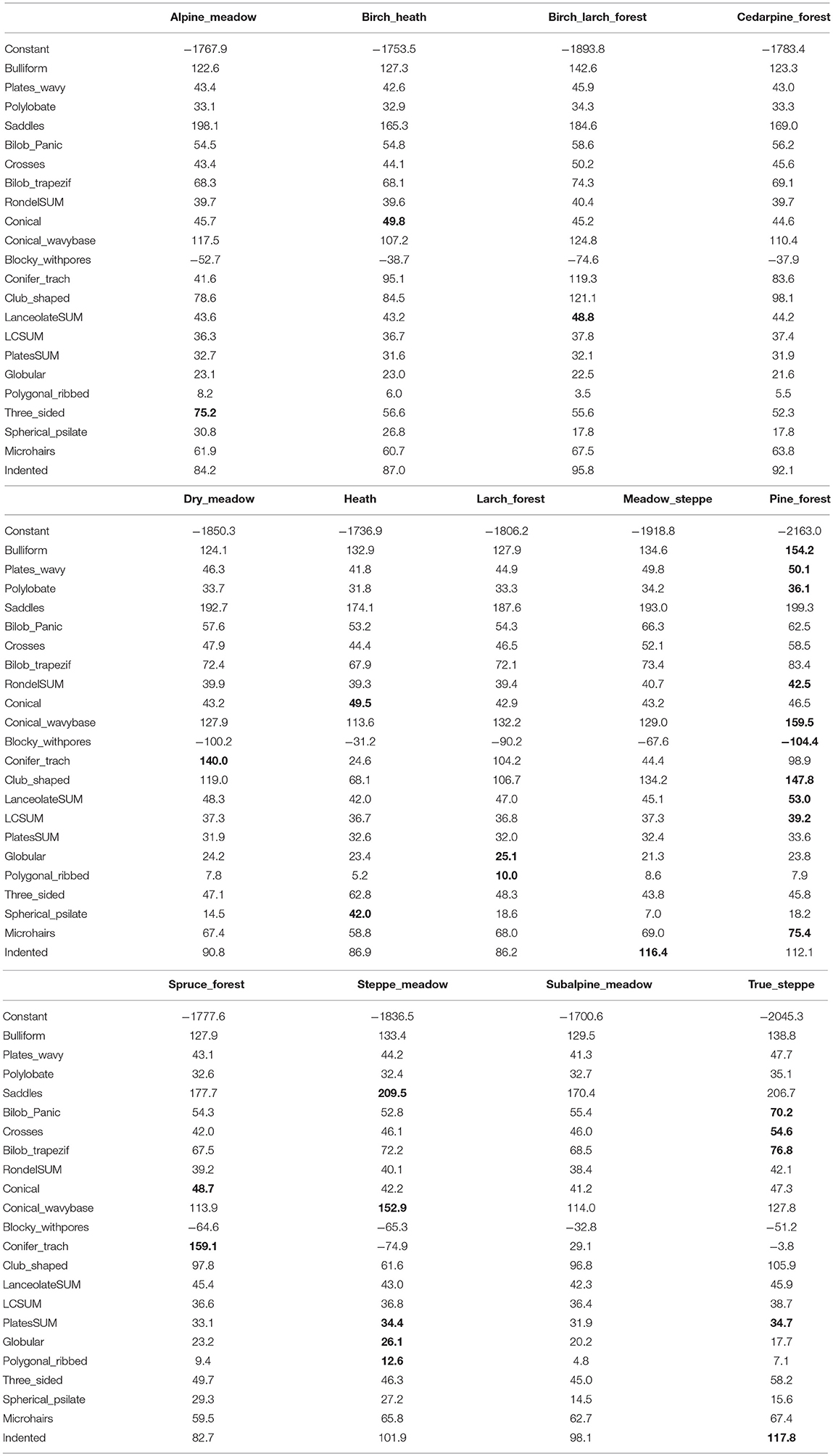
Table 3. Results of discriminant analysis showing linear discriminant function for groups (highest score in each community for each morphotype is shown in bold).
What Factors Determine Phytolith Assemblage Composition on Plots?
Results of Mixed-Effects Model
A mixed effects model with 60 plots as random and other factors as fixed (20 big plots, communities, temperature regime, hydro regime, and elevation) was run on the aggregate morphotype dataset. Testing the significance of contribution of each factor to the overall assemblage composition, elevation was a significant factor (p < 0.05) for seven morphotypes (wavy plate, Panicoid and trapeziform bilobates, rondel, conical, conical with wavy base, and long cell sum), while hydro regime was an important, but not statistically significant, component of the variance for the two kinds of bilobates (Panicoid and trapeziform), for long cells, plates, and lanceolate forms. Temperature was important (but not statistically significant) for the conical morphotype. All other phytoliths were not numerous enough to produce significant results with this method.
Canonical Correspondence Analysis
The main gradient in our study is the elevation, which is inversely correlated with temperature (higher is colder, ~6°C MAT decrease per 1,000 m) and mostly correlated with moisture (higher is wetter, ~150 mm increase per 1,000 m), although the spruce forest sample is the wettest habitat in the middle of the gradient due to local soil conditions. The first axis (eigenvalue 0.0367) accounts for 73% of all variability in the data and represents elevational-temperature gradient (on the left are high elevation, cold communities, on the right are low elevation, warm communities). The second axis (eigenvalue 0.0153) accounts for most of the remaining variability in the data (29%) and is mostly related to the moisture signal. The axes were significantly related to the environmental data as tested with permutation technique at 999 permutations, p-values were <0.001. The upper left corner of the CCA diagram (Figure 5) is occupied by high elevation alpine heath and meadow communities characterized by rondels, polylobates, three-sided, and spherical psilate morphotypes. The lower left is occupied by mid-elevation moist spruce forest characterized by polylobates, conical, coniferous tracheids, microhairs, and plates. The upper right is occupied by meadow steppe and true steppe characterized by wavy plates, true and trapeziform bilobates, crosses, and saddles. These communities have the assemblages most dominated by grass morphotypes (>90%). The lower right are larch and birch-larch communities with some conifer phytolith presence.
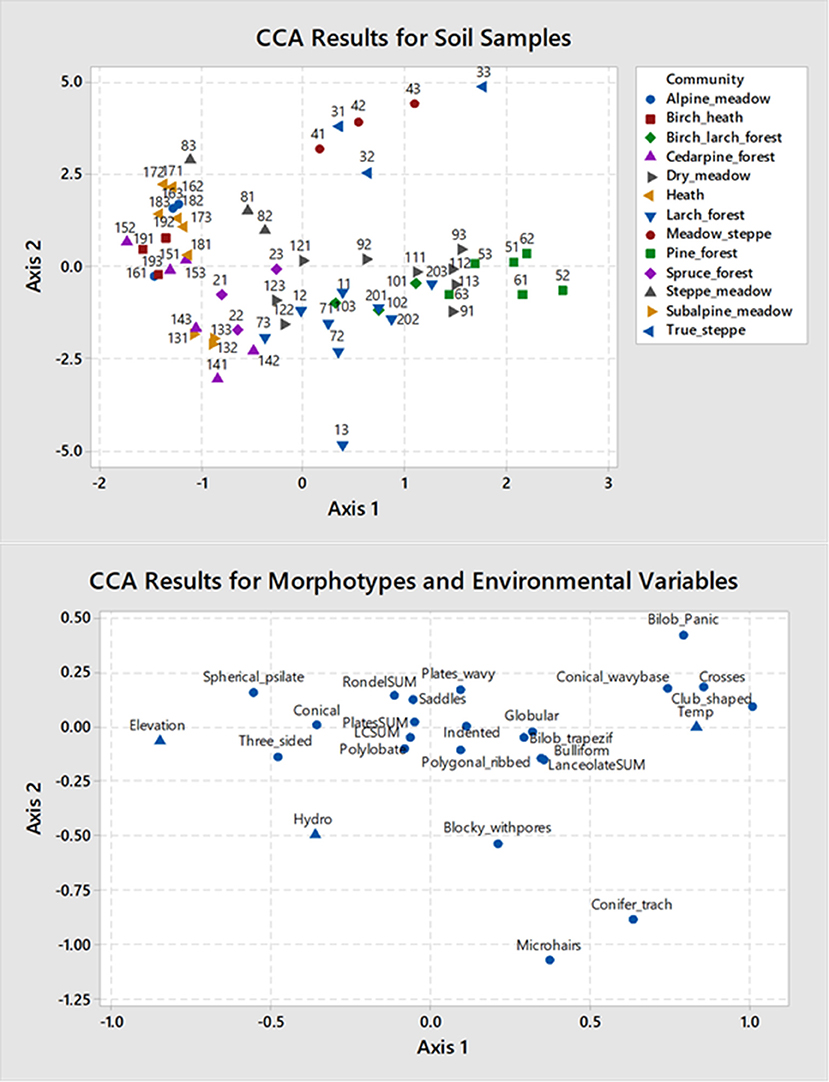
Figure 5. Triplot showing ordination results by Canonical Correspondence Analysis. First two axes are shown.
It should be noted that birch-larch and larch forests look similar to some dry meadows, and rather different from steppe communities. These are characterized by the presence of conifer phytoliths, conical with wavy bases, lanceolate, globular, polygonal ribbed, and indented irregular forms. Most of the phytolith morphotypes found in the soils under these communities are from non-grasses even though ~80% of the total phytoliths in the soil assemblage are derived from grasses in other communities.
Cluster Analysis
Cluster analysis (Figure 6) suggests two major groups of assemblages: those from true steppe, meadow steppe, steppe meadow, alpine heath, alpine meadow, birch subalpine heath and cedar pine forests near the treeline (right side of the dendrogram) and those from pine, spruce, larch, and birch-larch forests, subalpine meadow, and dry meadows at lower elevations (left side of the dendrogram). The first group lacks conifer phytoliths, has low proportion of lanceolate forms (<5%) and has high values for rondels and some other grass silica short cells (GSSC). The second group has some conifer phytoliths in most samples, higher values for lanceolate forms (>8%), as well as lower values for GSSC. Subclusters are well defined for pine forests, birch and birch-larch forests, meadows steppes, true steppes, and heaths. In contrast, dry meadows, spruce forests, subalpine meadows, and alpine meadows do not fit into tight clusters, probably reflecting the divergent composition of these communities.
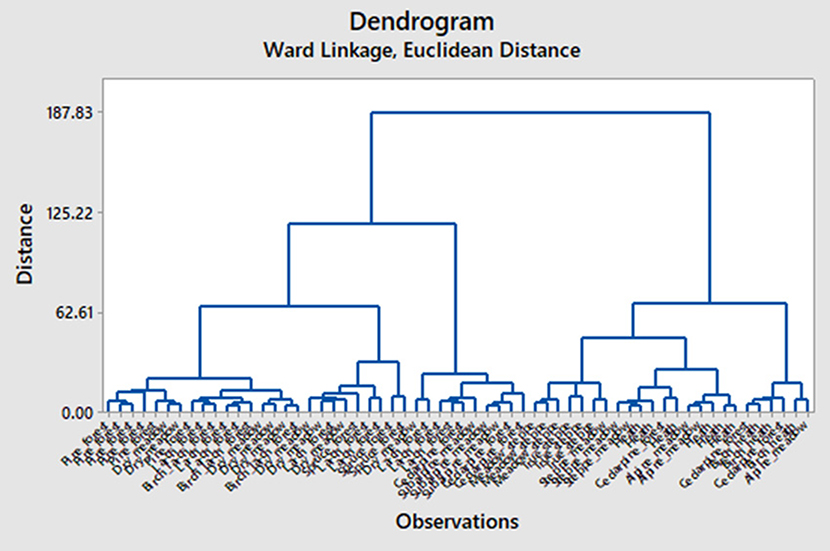
Figure 6. Cluster Analysis Dendrogram (Ward's method, Euclidean distance) linking assemblages by Community Type.
Can We Use Phytolith Indices (T/P, Ic, Ix) to Distinguish Specific Communities?
We propose a new index Tree/Poaceae as a modified version of the well-known D/P° index (Brémond et al., 2005a). In our region there are few phytoliths produced by dicotyledonous trees originally used for D in D/P° index in Africa, but there are several produced by conifer trees. Our T/P index is the sum of all tree and shrub phytoliths divided by grass phytoliths from short cells:
T/P ranges from 0.01 to 0.33 with the median value 0.078 and the mean value 0.091 (Figure 7). The value 0.08 distinguishes all forests at lower elevations from all subalpine and alpine communities. Cedar pine forests near the treeline cannot be distinguished from non-forested communities of the alpine and subalpine zone using this index. Likewise, dry meadows of lower elevations cannot be distinguished from the forests.
Ic (%) is the ratio of phytoliths mainly produced by Pooideae to the sum of phytoliths mainly produced by Pooideae, Chloridoideae, and Panicoideae and is typically used to detect a temperature signal, with high values corresponding to lower temperatures:
In our study area there are few Panicoid and no Chloridoid grasses. Nevertheless, saddles, bilobates, and crosses (quadrilobates) are in fact produced by either Stipa or wild Panicum related species that are more common at lower sites with higher temperatures. The index can be used to distinguish true steppes, meadow steppes, and pine forests of lower elevations (Ic < 90%, warmer conditions) from high elevation communities (Ic > 96%, cold conditions; Figure 8). However, there is a large group of communities at intermediate elevations that include larch and birch-larch forests, that have Ic (%) values similar to the alpine and subalpine communities and making it of limited use to detect the intermediate temperature signal in our area.
Iph index was not very useful in our study, because there were few saddles in most samples (the index uses the ratio of saddles to bilobates to distinguish dry from wet conditions). A more useful index for our area to detect xeric signal is:
This index is able to easily distinguish the two most xeric communities (group 1) in our study: true steppes and meadow steppes, with values exceeding 120 (Figure 9). However, its utility for separating communities at a higher level of moisture is low. Both mesoxeric (2) and xeromesic (3) communities have values between 30 and slightly over 100. The three wettest samples from spruce forest (4) have slightly higher values (45–50) than some of the samples in categories 2 and 3. We found that aggregating morphotype sums in different ways to define this index did not improve its performance. Thus, given the paucity of chloridoid and panicoid phytoliths, the xeric signal is harder to detect than the temperature signal in our study area.
Can Rondels and Lanceolate Subtypes Be Used to Distinguish Specific Communities?
Table 4 shows the results of the discriminant analysis performed using only nine different rondel morphotypes:
Pyramidal low rondel looks like a truncated pyramid, more or less square in top view, and with height < length in side view (Figure 2). Sometimes, the same morphotype is called short cell square trapeziform. It is moderately common in many communities and is most common in alpine meadow, alpine birch heath, and alpine heath (values between 6 and 9% of all phytoliths), which is confirmed by the high linear discriminant scores for this morphotype in these three communities and suggesting it as a characteristic morphotype of alpine habitats.
Pyramidal tall rondel has height > length in side view, and is a rare form, most common in alpine meadow (0.9%) and for which it has the highest discriminant value, although it is also found at even higher values in some steppe meadow samples.
Conical low rondel looks like a truncated cone, more or less round in top view, and with height < length in side view (Figure 2). It is the most common rondel type, ranging from 5 to 17.5% in most communities. Its discriminant value is highest in meadow steppe and true steppe, but because it is so common, its overall diagnostic utility is low.
Keeled type 1 rondel has a keel, instead of flat bottom, and its distribution is similar to pyramidal low rondel because it is most common in alpine meadows and alpine birch heaths (about 2%) and has high discriminant values for those community types. However, it is also slightly common (1%) in larch and pine forests and in meadow steppe.
A ratio of pyramidal low and keeled type 1 rondel relative to keeled low rondel may help distinguish alpine communities (high values) from steppes (medium values) from forests (low values; Figure 10).
Keeled type 2 rondel is most common in birch heath and true steppe (about 2% in each). Based on DA, its absence may be characteristic of alpine meadows and help distinguish that community from birch heath.
Conical high rondel (round in top view, height > length in side view) has a high occurrence (2–12%) and a high discriminant value in steppe meadows. These communities are more mesic than meadow steppes and are generally found at higher elevations (>1,200 m).
Oblong rondel (elongated in top view with usually flat or slightly keeled bottom) is the rarest rondel and is only found in true steppe and meadow steppe (0.4–0.5%) as also confirmed by DA scores.
Saddletop rondel has wavy, saddle-like top, but is trapeziform in side view. It is most common in alpine heath, birch heath and slightly less common in alpine meadows and cedar pine forests near the treeline (1.5%). The morphotype is also found in true steppe (1%), at much lower elevations. Its discriminant value is highest for the two types of heath.
Round bottom rondel appears round in top view, but are not trapeziform or conical in side view, rather, their bottom half is a hemisphere (Figure 2). It is common only in alpine heaths (2%), but its absence is particularly indicative of the true steppe.
We distinguished three kinds of lanceolate cells (trichomes) (Figure 2). They are common in both grasses and sedges. Large base lanceolate is most common in birch, birch-larch and pine forests, and dry meadows (3%), while steppe and alpine communities have <1% of this morphotype (Figure 11A). Lanceolate form with long awn is most common in pine forests (8%), but also in larch, birch-larch, and dry meadow communities (5%) (Figure 11B). Finally, triangular lanceolate form is most common in spruce forests (2–5%). The lanceolates as a group are good indicators of many different forests, but not meadows or steppe, except in the forest zone (dry meadows in our study).
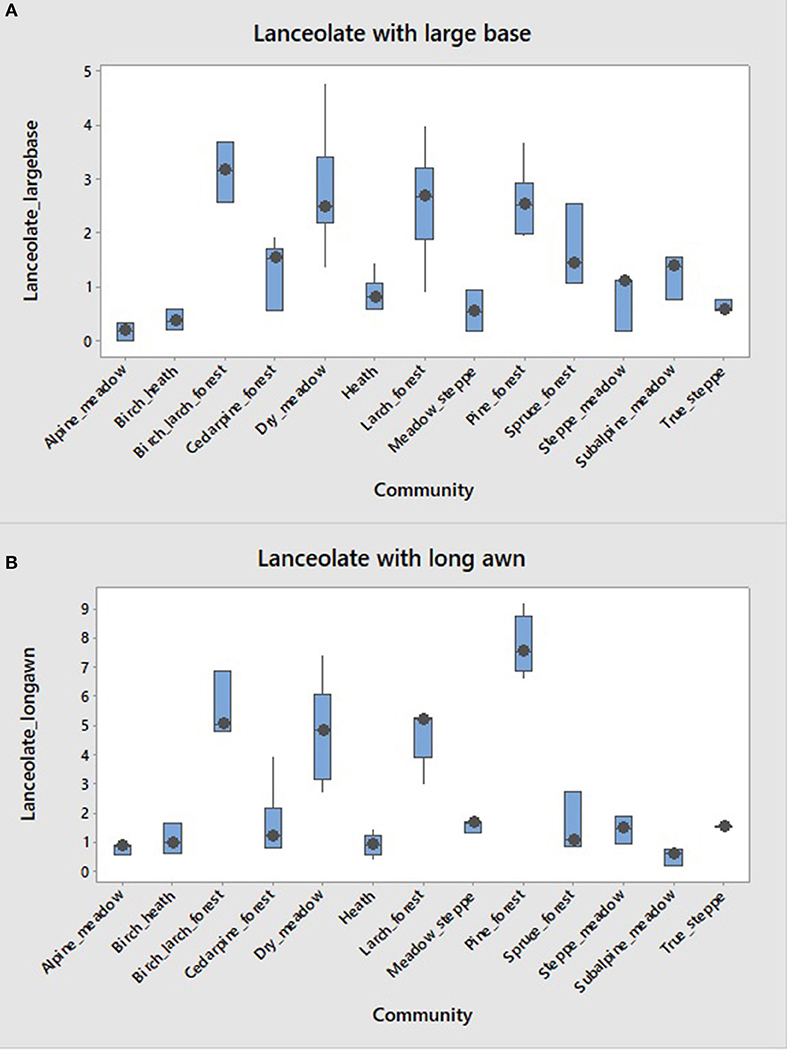
Figure 11. Boxplots showing distribution of frequencies of two kinds of lanceolate forms. (A) large base short awn/”forest” and (B) small base long awn/”meadow.”
We also distinguished 15 morphotypes of long cells (LC), which mostly come from grasses, but some are found in sedges and conifers (Figure 2). One LC with three ribs apparently comes from ferns. Due to the ubiquity of long cells and their higher degree of silicification with increased moisture, we did not expect their high utility in detecting specific communities.
The following observations can be made: long cells with smooth parallel walls (LC psilate) were most common in spruce forests (12%), and in cedar pine forests and nearby subalpine meadows (~10%). They were also common in larch and birch-larch forests and alpine heaths (6%). Most other types were rare in all communities (<1%), for example, dendritic cells of Triticeae tribe were found only in alpine meadows and heaths, dry meadows, and true steppe.
Short plates with parallel walls were by far the most common in the spruce forest (25%) as well as polygonal ribbed phytoliths (2–4%). Some rare types in this community may be contributed by mosses.
Diagnostic Key for Plant Communities (All Percent Values Are From the Total of All Morphotypes)
Based on the results, we developed a simple diagnostic key to quickly identify each of the 13 communities in our study area.
1. Conifer phytoliths present (blocky with pores, conifer tracheids, and/or club-shaped) ……… 3
2. Conifer phytoliths absent …………… 5
3. Polylobate trapezoids <10%, plates are 30%, conical phytoliths 1–3%, club shaped absent, triangular lanceolate is 2–5%………………….Spruce forest
a. Coniferous tracheids may help detect spruce forest
4. Polylobate trapezoids>10%, plates <10%, conical phytoliths rare (<1%), club shaped usually present, triangular lanceolate is <2%………………….Pine forest
a. Conifer with pores, club shaped and lanceolate with long awn may be characteristic of this community
5. True bilobates and crosses (quadrilobates) present, polylobate trapezoids <10%………….7
6. True bilobates and crosses (quadrilobates) absent, polylobate trapezoids>10%……………………….…………… 11
7. True bilobates, crosses and trapeziform bilobates together >10%, rondels >30%…… True steppe
8. True bilobates, crosses and trapeziform bilobates together <7%…………… 9
9. True bilobates, crosses and trapeziform bilobates <7%, rondels ~27%…….Meadow steppe
a. Indented irregular plates of dicots may help detect this community
10. True bilobates, crosses and trapeziform bilobates <4%, rondels <22% (including conical high rondel 2–12%)…….Steppe meadow
a. Saddles and conical with wavy base may help detect this community
11. Lanceolate >10%…………Dry meadow
12. Lanceolate <10%…………………… 13
13. Rondels >30% (including pyramidal low rondel 6–9%, saddle top and round bottom rondels may be present in small numbers)………………….…Alpine meadow, alpine heath, and alpine birch-heath
a. Three-sided forms can help detect alpine meadow
b. Spherical psilate and conical can help detect heath
c. Conical can also help detect birch-heath
14. Rondels <30% (including pyramidal low rondel <6%)…………………………….15
15. Polylobate trapezoid 20–25%, lanceolate phytoliths 3–6%……….Subalpine meadow and cedar pine forest
16. Polylobate trapezoid <20%, lanceolate phytoliths 6–10%……….Larch and birch-larch forest
a. Both of these communities may also be detected by presence of polygonal ribbed and globular forms
b. Lanceolate may help distinguish birch-larch forests
Discussion
Our study contributes to the growing body of knowledge regarding the ability of phytoliths in subrecent assemblages in modern soils in temperate regions of the world to detect vegetation zones and specific plant communities (Kiseleva, 1982; Blinnikov, 1994, 2005; Fredlund and Tieszen, 1994; Volkova et al., 1995; Kerns, 2001; Blinnikov et al., 2013; McCune and Pellatt, 2013; Traoré et al., 2015; Gavrilov and Loyko, 2016; Lada, 2016; Feng et al., 2017; Gao et al., 2018). In the Republic of Gorny Altay of Russia this is the first attempt of its kind and complements our study of the lowlands in the Altaysky Kray (Speranskaja et al., 2018). Overall, MANOVA results from our study confirm that neighboring samples have more similar phytolith assemblages compared to those further apart, even from similar communities.
Our study also confirms the earlier findings that some morphotypes (e.g., rectangular plates) or their sums (long cells) are highly redundant across important environmental gradients, such as elevation, temperature or moisture (Blinnikov, 2005; Speranskaja et al., 2018); yet, combinations of phytoliths can detect relatively specific community types, such as true steppes (more grasses) vs. meadow steppes (more forbs), pine vs. spruce forests, subalpine meadows vs. alpine meadows, etc. The diagnostic key we developed for this study should be more widely tested across similar communities in Central Eurasia, including for example Altay extensions in Mongolia and Kazakhstan, the Sayan mountains of Russia and possibly even Central Asian mountains in Kazakhstan, Kyrgyzstan and Tajikistan.
Some of our findings pertaining to specific mountain communities corroborate earlier work in the Caucasus (Kiseleva, 1992; Blinnikov, 1994; Volkova et al., 1995), NE China (Traoré et al., 2015; Gao et al., 2018), the western European mountains (Carnelli et al., 2001; Delhon et al., 2003), and temperate North America (Kerns, 2001; Blinnikov, 2005; McCune et al., 2015). For example, the high incidence of rondels in grassland communities and high incidence of lanceolate forms in forests reported in many of these studies is also supported by our study. The Volkova et al. (1995) study of subalpine and alpine communities of Teberda Nature Reserve in the northwestern Caucasus demonstrated distinct assemblages in eight communities, of which five are broadly analogous to this study: alpine heath, alpine meadow with forbs, alpine tussock grass community, subalpine meadow, and mid-elevation pine forest. Many species of plants in the Altay and the Caucasus are related vicariant species (Körner, 2003). Therefore, we expect to see some broad similarities in their phytolith production. In the Caucasus study, the community with the highest proportion of rondels (“hats” in Volkova et al., 1995) was the tussock grassland (57%) and it is the “true steppe” with tussock grasses in this study (25%). The alpine forb-rich meadow had high proportion of wavy forms in the Caucasus (mean value = 11%), similar to our findings (mean value = 12%). The alpine heath community had the highest proportion of conical phytoliths of Carex in the Caucasus study (6%); in our study, this community has more of this morphotype than any other community, varying from 2 to 5%. The pine forest assemblage from the Caucasus had 7% Pinus club-shaped phytoliths. In our study, pine forests have only 0.5%, but this is the highest percentage of the three communities in which this morphotype is found.
Traoré et al. (2015) found that in NE China over 50% of phytoliths in broadleaf forests may be of tree origin, and about 20% in pine forests at higher elevations. We did not observe values that high in any of our forests, only in single percentage points; the production of tree phytoliths in the humid subtropical trees is evidently much greater than in the cold continental species.
Gao et al. (2018) studied modern phytolith assemblages in soils at 108 sites from Changbai and Lesser Kingan Mountains and Songnen grassland in NE China. They listed 5 communities, including a mixed pine forest with Pinus koraiensis, but also with oaks; larch-birch forest with Larix olgensis and Betula platyfilla; broadleaf forest with Quercus and Juglans; low elevation steppe, and sparse mixed parkland. Of the five, pine and larch-birch forests in their study are very similar to ours, albeit with different species (but the same tree genera), and their parkland is similar to our open Siberian cedar pine forests and subalpine meadows mix. Similar to our study, the proportion of woody phytoliths in their assemblages rarely exceeds 10%, while grasses account for 80–90%. Their grassland assemblage is dominated by rondels and bilobates, as is the steppe in our study. Based on discriminant analysis, larch and broadleaf forest have distinct assemblages in their study (like larch forest in ours) due to presence of some tree phytoliths, but their pine forest assemblage can be confused with grassland or parkland because of low production of distinct morphotypes in Korean pine. Interestingly, this is similar to our cedar pine forests in this study, but is different for regular pine forests of Pinus sylvestris, which has more distinct phytolith assemblages, including pine club-shaped phytoliths (also see Kerns, 2001; McCune et al., 2015 for the description of similar phytoliths in Pinus ponderosa).
Delhon et al. (2003) looked at various Mediterranean communities in the lower Rhone valley in France, including a pine forest, a reed patch, a Pooid grassland, an oak forest and a riparian forest. Their results generally correspond to ours for pine forest and for grassland: pine phytoliths do occur in the forest, but not in grassland, and rondels may represent up to 50% of all phytoliths in the grassland.
The novel aspect of our work is the utilization of specific rondel and lanceolate types that can be specific to particular communities. While usually found in small quantities, some of these morphotypes proved very useful in detecting subtle community differences, such as alpine meadow vs. alpine heath or birch-larch vs. larch forest. Some physiognomically different communities located at comparable elevations yield similar phytolith records in this study (e.g., dry meadows vs. pine forests and subalpine meadows vs. cedar pine forests). Two explanations come to mind: first, and most obvious, is that these communities have similar sets of phytolith producers, their main dominants do not produce phytoliths and are therefore “silent” or “quiet” taxa. For example, Scotch pine produces only very small amounts of its diagnostic club shaped form, making it a “quiet taxon” (Volkova et al., 1995; Delhon et al., 2003), but grasses of the pine forest have copious production and are the same species as those in the surrounding dry meadows. Cedar pine produces almost no phytoliths (“silent taxon”), but its understory grasses are very similar to those of the surrounding subalpine meadows. Therefore, grass signal masks pine presence and is almost the same in forest and non-forest in both cases as was also noted by Gao et al. (2018) for NE China forests. A second explanation is that a high level of spatial heterogeneity creates a temporally shifting mosaic commonly observed at treelines (Körner, 2003; Onipchenko, 2004). In this case, subrecent phytolith signal reflects prior inheritance and a mixture of both communities (for example, cedar pine vs. subalpine meadow in this study), demonstrating that there may be limits to the usefulness of phytolith analysis.
Our results confirm previous research that certain narrowly-defined morphotypes, such as various kinds of rondels (Kiseleva, 1992), and to a lesser extent lanceolate forms (Golyeva, 2007), can be used to distinguish plant communities. Rondel subtypes may be particularly useful in the temperate zone of the world dominated by the Pooideae subfamily of grasses and the consequent lack of Panicoid or Chloridoid forms. Low conical rondels found in steppes can be produced, for example, by Helictotrichon pubescens (Huds.) Pilg., Stipa capillata L., and Phleum phleoides L. In cedar pine forest the same morphotype is likely from Poa sibirica Roshev. In alpine lichen heath it may be from Festuca ovina. In contrast, low pyramidal rondel in the alpine and subalpine communities may be contributed by other Festuca species, and in lowland communities by Elymus-Leymus group of species (Speranskaya et al., 2018).
Another important morphotype that could prove useful is trapezoidal bilobate (“Stipa-type” of Mulholland, 1989; Fredlund and Tieszen, 1994). In North America it was originally defined from what is now considered a separate genus Achnatherum (Barkworth, 1981), but is ironically relatively rare in true Stipa in Eurasia, which produces more saddles or tall rondels (Speranskaya et al., 2018). However, in this study, many trapezoidal bilobates were found in communities dominated by Brachipodium pinnatum (L.) Beauv., an important dominant grass of pine forests of Eurasia. This is a novel result, because this species has not been evaluated for phytolith production. This morphotype is found in small quantities in many grasses with trapezoidal polylobate forms (e.g., Agrostis and Calamagrostis).
Lanceolate forms earlier described as “forest trichomes” (those with a large base and a short awn) or “meadow trichomes” (those with a small base and a long awn), have been used by Golyeva (2007) to distinguish certain communities in European Russia. We found that large base lanceolate forms are indeed more common in birch-larch, pine, and larch forests, but not in spruce or cedar pine forests (Figure 11A). They are also common in dry meadows, where they may be either inherited from a prior forest or produced locally by species similar to those growing in pine forests nearby (e.g., Calamagrostis langsdorffii (Link) Trin., C. arundinacea (L.) Roth, Brachypodium pinnatum (L.) Beauv.). Many of these forms likely come from upland sedges (e.g., Carex muricata L.), not from grasses. Long-awn form is also common in the same four communities, as can be seen on the boxplots. It is not common in any meadows, except dry meadow at lower elevations near pine or larch forest, where its presence can be again explained in the same fashion as their long-base cousins.
A third, triangular lanceolate type, was found primarily in spruce forest (which is actually a swamp in this study, not an upland spruce community), which were reported also in soils under spruce forests in western Siberia (Gavrilov and Loyko, 2016); it may be contributed by sedges found in this specific habitat. We found stronger linkage between hydro regime and lanceolate abundance than with specific community type in our study. Mesophytic communities have more large base, short awn (“forest type” of Golyeva) than small base, long awn type (“meadow type” of Golyeva). More studies of lanceolate production as it related to moisture regime is needed. In a recent study of common reed from China, Liu et al. (2016) found that lanceolate forms tend to be larger in plants growing in a higher evapotranspiration regime, an effect also earlier reported from West Africa (Brémond et al., 2005b). Attempts to distinguish taxa based on identification of many different types of long cells, except dendritic forms of Triticeae tribe, were not successful.
We found utility in three phytolith indices: T/P (this study, Delhon et al., 2003) to detect forests at values >0.08, Ic (Brémond et al., 2005a) to detect temperature signal (Ic>96% are cold alpine communities), and Ix (this study, to replace Iph, which is not applicable in our region) to detect aridity (values>120 indicate xeric communities). Given the coarse resolution of available climate data and the relatively short climatic gradient of our study, we cannot recommend relying on these for derivation of reliable climate transfer functions for the Altay, although this may be possible when research is performed along longer gradients and in different settings.
Conclusions
Sixty total samples from 20 sites (triple replicates at each site) reliably differentiated 7 of 13 studied communities, including similar communities such as true steppe vs. meadow steppe or alpine meadow vs. alpine heath. Replicate samples from each site were more similar to each other than to other samples even from similar communities on different sites.
Our study failed to distinguish dry meadow from nearby pine and larch forest, two kinds of larch forest from each other, and cedar pine forest near the treeline from subalpine meadow, even when a very detailed classification scheme of rondels and lanceolate forms was followed. However, the phytolith approach was successful distinguishing other communities. Using only rondel, lanceolate and long cell sums is a more easily replicable, practical approach for most researchers and that can still detect fairly small changes in community composition. In our study area true bilobate and saddle forms were very rare but were occasionally found among the weedy species. Bilobate trapezoids could be contributed by Stipa, as well as by Brachypodium of pine forest (a novel finding). Lanceolate forms were contributed by both grasses and sedges. The relationship between their size and abundance with moisture regime should be subject of further study using morphometric approach.
Data Availability Statement
Datasets are available on request. The raw data supporting the conclusions of this manuscript will be made available by the authors, without undue reservation, to any qualified researcher.
Author Contributions
All authors contributed equally to this research. MMS and NS designed the study and organized field work and supervised students. MYS carried out microscopy analysis. MB analyzed statistics and designed most figures, except 1 and 2 which were designed by MYS. All authors helped to write and proofread the manuscript.
Conflict of Interest Statement
The authors declare that the research was conducted in the absence of any commercial or financial relationships that could be construed as a potential conflict of interest.
Acknowledgments
We acknowledge financial support from the Russian Foundation for Basic Research (RFBR) 17-04-00437 Influence of ecological and climatic factors on the composition of phytolith assemblages in modern soils under main types of plant communities of the Northern Altai to MMS and Saigo Fund and the Foundation of St. Cloud State University to MB. Climate data were provided by N. F. Kharlamova of the Physical Geography and GIS department of Altay State University. Figure 1 was made by M. A. Borisenko of the same department.
References
An, X.-H. (2016). Morphological characteristics of phytoliths from representative conifers in China. Palaeoworld 25, 116–127. doi: 10.1016/j.palwor.2016.01.002
Barkworth, M. E. (1981). Foliar epidermis and taxonomy of North American Stipeae (Gramineae). Syst. Bot. 6, 136–152. doi: 10.2307/2418544
Beavers, A. H., and Stephen, I. (1958). Some features of the distribution of plant opal in Illinois soils. Soil Sci. 86, 1–5.
Blinnikov, M. (1994). “Phytolith analysis and the Holocene dynamics of alpine vegetation,” in Experimental Investigation of Alpine Plant Communities in the Northwestern Caucasus, eds V. Onipchenko and M. Blinnikov (Zürich: Veröffentlichungen des Geobotanischen Institutes der ETH, Stiftung Rübel, H), 23–40.
Blinnikov, M. S. (2005). Phytoliths in plants and soils of the interior Pacific Northwest, USA. 2005. Rev. Palaeobot. Palynol. 135, 71–98. doi: 10.1016/j.revpalbo.2005.02.006
Blinnikov, M. S., Bagent, C. M., and Reyerson, P. E. (2013). Phytolith assemblages and opal concentrations from modern soils differentiate temperate grasslands of controlled composition on experimental plots at Cedar Creek, Minnesota. Quat. Int. 287, 101–113. doi: 10.1016/j.quaint.2011.12.023
Bozarth, S. R. (1992). “Classification of opal phytoliths formed in selected dicotyledons native to the Great Plains,” in Phytolith Systematics: Emerging Issues, eds G. Rapp Jr and S.C. Mulholland (New York, NY: Plenum Press), 193–214. doi: 10.1007/978-1-4899-1155-1_10
Brémond, L., Alexandre, A., Hély, C., and Guiot, J. (2005a). A phytolith index as a proxy of tree cover density in tropical areas: calibration with Leaf Area Index along a forest–savanna transect in southeastern Cameroon. Global Planet. Change 45, 277–293. doi: 10.1016/j.gloplacha.2004.09.002
Brémond, L., Alexandre, A., Peyron, O., and Guiot, J. (2005b). Grass water stress estimated from phytoliths in West Africa. J. Biogeog. 32, 311–327. doi: 10.1111/j.1365-2699.2004.01162.x
Cabanes, D., Weiner, S., and Shahack-Gross, R. (2011). Stability of phytoliths in the archaeological record: a dissolution study of modern and fossil phytoliths. J. Archaeol. Sci. 38, 2480–2490. doi: 10.1016/j.jas.2011.05.020
Carnelli, A. L., Madella, M., and Theurillats, J.-P. (2001). Biogenic silica production in selected alpine plant species and plant communities. Ann. Bot. 87, 425–434. doi: 10.1006/anbo.2000.1355
Delhon, C., Alexandre, A., Berger, J., Thiébault, S., Brochier, J., and Meunier, J. (2003). Phytolith assemblages as a promising tool for reconstructing Mediterranean Holocene vegetation. Quat. Res. 59, 48–60. doi: 10.1016/S0033-5894(02)00013-3
Feng, Y., Jie, D., Guo, M., Dong, S. h., Chen, X., et al. (2017). Phytolith loss and enrichment in soil phytolith assemblages revealed by comparisons of phytoliths in vegetation and surface soils of altitudinal belts in the Changbai Mountains, Northeast China. Flora 236, 84–93. doi: 10.1016/j.flora.2017.08.005
Fredlund, G., and Tieszen, L. (1994). Modern phytolith assemblages from the North American Great Plains. J. Biogeog. 21, 321–335. doi: 10.2307/2845533
Gao, G., Jie, D., Li, D., Li, N., Liu, L., et al. (2018). Reliability of phytoliths for reconstructing vegetation dynamics in northern temperate forest regions: a case study in northeast China. Quat. Sci. Rev. 201, 1–12. doi: 10.1016/j.quascirev2018.10.020
Gavrilov, D. A., and Loyko, S. V. (2016). Fitolity pochvtemnokhvojnyh gemiborealnykh lesov yugo-vostoka Zapadnoj Sibiri (In Russian). Dinamika Okr. Sredy i Glob. Izm. Klim. 1, 41–53. doi: 10.17816/edgcc7141-53
Golyeva, A. (2007). “Various phytolith types as bearers of different kinds of ecological information,” in Plants, People and Places: Recent Studies in Phytolith Analysis, eds M. Madella and Z. Zurro (Oxford: Oxbow Books), 196–200.
Hammer, Ø., Harper, D. A. T., and Ryan, P. D. (2001). PAST: paleontological statistics software package for education and data analysis. Palaeontol. Electron. 4:9.
Hyland, E., Smith, S. Y., and Sheldon, N. D. (2013). Representational bias in phytoliths from modern soils of central North America: implications for paleovegetation reconstructions. Palaeogeog. Palaeoclim. Palaeoecol. 374, 338–348. doi: 10.1016/j.palaeo.2013.01.026
Juggins, S. (2003). C2 User guide. Software for Ecological and Palaeoecological Analysis and Visualisation. Newcastle upon Tyne: University of Newcastle.
Kerns, B. (2001). Diagnostic phytoliths for a ponderosa pine-bunchgrass community near Flagstaff, Arizona. Southwest. Naturalist 46, 282–294. doi: 10.2307/3672424
Kiseleva, N. K. (1982). Izuchenie fitolitov v pochvah dlya vyjasnenija istorii rastitel'nosti stepej vostochnoj Mongolii (in Russian). Izvestiya AN SSSR, Ser. Geog. 2, 95–106.
Kiseleva, N. K. (1992). “Botanicheskij I fitolitnyj analiz golotsenovyh otlozhenij of mammal dung in North Ossetiya (in Russian),” in Istoricheskaya ekologiya dikih i domashnih kopytnyh. Istorya pastbischnyh ekosistem. Moscow, 24–83.
Klein, R. L., and Geis, J. W. (1978). Biogenetic opal in the Pinaceae. Soil Sci. 126, 145–156. doi: 10.1097/00010694-197809000-00003
Körner, C. (2003). Alpine Plant Life: Functional Plant Ecology of High Mountain Ecosystems 2nd edn. Berlin: Springer. doi: 10.1007/978-3-642-18970-8
Lada, N. Y. (2016). Uslovija formirovanija mikrobiomorfnykh spektrov stepnykh priozernykh landshaftov severnoj Kulundy (In Russian). Dinamika Okr. Sredy i Glob. Izm. Klim. 1, 85–92. doi: 10.17816/edgcc7185-92
Liu, L., Jie, D., Liu, H., Gao, G., Gao, Z., et al. (2016). Assessing the importance of environmental factors to phytoliths of Phragmites Communis in North-Eastern China. Ecol. Indicators 69, 500–507. doi: 10.1016/j.ecolind.2016.05.009
Madella, M., Alexandre, A., and Ball, T. (2005). International Code for Phytolith Nomenclature 1.0. Ann. Bot. 96, 253–260. doi: 10.1093/aob/mci172
McCune, J. L., and Pellatt, M. G. (2013). Phytoliths of Southeastern Vancouver Island, Canada, and their potential use to reconstruct shifting boundaries between Douglas-fir forest and oak savannah. Palaeogeog. Palaeoclim. Palaeoecol 383–384, 59–71. doi: 10.1016/j.palaeo.2013.05.003
McCune, J. L., Vellend, M., and Pellatt, M. G. (2015). Combining phytolith analysis with historical ecology to reveal the long-term, local-scale dynamics within a savannah-forest landscape mosaic. Biodiv. Conserv. 24, 609–626. doi: 10.1007/s10531-014-0840-1
Mulholland, S. C. (1989). Phytolith shape frequencies in North Dakota grasses: a comparison to general patterns. J. Archaeol. Sci. 16, 489–511. doi: 10.1016/0305-4403(89)90070-8
Onipchenko, V. G. (2004). Alpine Ecosystems in the Northwest Caucasus. Geobotany: 29. Dordrecht: Kluwer Academic. doi: 10.1007/978-1-4020-2383-5
Piperno, D. R. (2006). Phytoliths: A Comprehensive Guide for Archaeologists and Paleoecologists. Lanham, MD: Altamira Press.
Reich, D., Green, R. E., Kircher, M., Krause, J., Patterson, N., Durand, E. Y., et al. (2010). Genetic history of an archaic hominin group from Denisova Cave in Siberia. Nature 468, 1053–1060. doi: 10.1038/nature09710
Sedelnikov, V. P. (1988). Vysokogornaya Rastitelnost Altae-Syanaskoy Gornoy Oblasti. (In Russian.) Novosibirsk: Nauka.
Speranskaja, M., Solomonova, M., Speranskaja, N., and Blinnikov, M. S. (2018). Phytoliths of temperate forest-steppe: a case study from the Altay, Russia. Rev. Palaeobot. Palynol. 250, 1–15. doi: 10.1016/j.revpalbo.2017.12.002
Speranskaya, N., Yu Solomonova, M., Yu Silantyeva, M. M., Genrih, Y. u.V., et al. (2018). Cereal phytoliths of Northern Altay. Ukr. J. Ecol. 8, 762–771. doi: 10.15421/2018_278
ter Braak, C. J. F. (1986). Canonical correspondence analysis: a new eigenvector technique for multivariate direct gradient analysis. Ecology 67, 1167–1179. doi: 10.2307/1938672
Traoré, D. D., Gu, Y., Liu, H., Shemsanga, C., and Ge, J. (2015). Vegetation types and climate conditions reflected by the modern phytolith assemblages in the subalpine Dalaoling Forest Reserve, Central China. Front. Earth Sci. 9, 268–275. doi: 10.1007/s11707-014-0475-2
Verma, S. D., and Rust, R. H. (1969). Observation on opal phytoliths in a soil biosequence in southeastern Minnesota. Soil Sci. Soc. Am. Proc. 33, 749–751. doi: 10.2136/sssaj1969.03615995003300050035x
Volkova, E. V., Onipchenko, V. G., and Blinnikov, M. S. (1995). Subrecent phytolith assemblages of alpine communities in the Teberda Nature Reserve, the north-western Caucasus, Russia. Oec. Mont. 4, 1–8.
Keywords: elevational gradient, modern soils, phytoliths, plant communities, the Altay
Citation: Solomonova MY, Blinnikov MS, Silantyeva MM and Speranskaja NY (2019) Influence of Moisture and Temperature Regimes on the Phytolith Assemblage Composition of Mountain Ecosystems of the Mid Latitudes: A Case Study From the Altay Mountains. Front. Ecol. Evol. 7:2. doi: 10.3389/fevo.2019.00002
Received: 06 November 2018; Accepted: 04 January 2019;
Published: 29 January 2019.
Edited by:
Terry B. Ball, Brigham Young University, United StatesReviewed by:
Rand Evett, University of California, Berkeley, United StatesDongmei Jie, Northeast Normal University, China
Copyright © 2019 Solomonova, Blinnikov, Silantyeva and Speranskaja. This is an open-access article distributed under the terms of the Creative Commons Attribution License (CC BY). The use, distribution or reproduction in other forums is permitted, provided the original author(s) and the copyright owner(s) are credited and that the original publication in this journal is cited, in accordance with accepted academic practice. No use, distribution or reproduction is permitted which does not comply with these terms.
*Correspondence: Mikhail S. Blinnikov, bXNibGlubmlrb3ZAc3RjbG91ZHN0YXRlLmVkdQ==