- 1Hawkesbury Institute for the Environment, Western Sydney University, Sydney, NSW, Australia
- 2Metabolomics Australia, The University of Melbourne, Melbourne, VIC, Australia
- 3US Department of Energy Joint Genome Institute, Walnut Creek, CA, United States
- 4INRA, Interactions Arbres/Microorganismes, Laboratory of Excellence ARBRE, INRA-Nancy, Champenoux, France
- 5School of BioSciences, The University of Melbourne, Melbourne, VIC, Australia
Plant roots co-inhabit the soil with a diverse consortium of microbes of which a number attempt to enter symbiosis with the plant. These microbes may be pathogenic, mutualistic, or commensal. Hence, the health and survival of plants is heavily reliant on their ability to perceive different microbial lifestyles and respond appropriately. Emerging research suggests that there is a pivotal role for plant root secondary metabolites in responding to microbial colonization. However, it is largely unknown if plants are able to differentiate between microbes of different lifestyles and respond differently during the earliest stages of pre-symbiosis (i.e., prior to physical contact). In studying plant responses to a range of microbial isolates, we questioned: (1) if individual microbes of different lifestyles and species caused alterations to the plant root metabolome during pre-symbiosis, and (2) if these early metabolite responses correlate with the outcome of the symbiotic interaction in later phases of colonization.
We compared the changes of the root tip metabolite profile of the model tree Eucalyptus grandis during pre-symbiosis with two isolates of a pathogenic fungus (Armillaria luteobubalina), one isolate of a pathogenic oomycete (Phytophthora cinnamomi), two isolates of an incompatible mutualistic fungus (Suillus granulatus), and six isolates of a compatible mutualistic fungus (Pisolithus microcarpus). Untargeted metabolite profiling revealed predominantly positive root metabolite responses at the pre-symbiosis stage, prior to any observable phenotypical changes of the root tips. Metabolite responses in the host tissue that were specific to each microbial species were identified. A deeper analysis of the root metabolomic profiles during pre-symbiotic contact with six strains of P. microcarpus showed a connection between these early metabolite responses in the root with later colonization success. Further investigation using isotopic tracing revealed a portion of metabolites found in root tips originated from the fungus. RNA-sequencing also showed that the plant roots undergo complementary transcriptomic reprogramming in response to the fungal stimuli. Taken together, our results demonstrate that the early metabolite responses of plant roots are partially selective toward the lifestyle of the interacting microbe, and that these responses can be crucial in determining the outcome of the interaction.
Introduction
The rhizosphere is a narrow zone of soil adjacent to plant roots where soil microbes interact with, and influence, plant physiology (Hartmann et al., 2008, 2009). Similarly, interactions occurring at this interface significantly impact the soil microbial community structure (Baudoin et al., 2003; Broeckling et al., 2008). This root-microbe interaction is mainly mediated by the exchange of chemical signals as demonstrated by identification of metabolites in root exudates that can attract or defend against certain microbes (Steinkellner et al., 2007; Badri and Vivanco, 2009; Baetz and Martinoia, 2014). Therefore, theoretically, adjusting the chemical composition of root exudates should enable plants to selectively recruit beneficial microbes while deterring pathogens (Hartmann et al., 2009). Further, within a microbial genus or guild, the regulation of some plant secondary metabolites could also foster colonization of certain microbial individuals over others based on their relative benefit to plant health and fitness.
The early stage of interaction between plants and microbes prior to coming into physical contact, known as the pre-symbiotic stage, is crucial in priming plants to respond according to the nature of the microbes. Previous studies have suggested that the alteration of plant metabolic responses during this stage correspond to the lifestyles of the microbe attempting to infect the plant tissues. For example, root exudate composition was found to vary with the type of endophytes colonizing tall fescue (Guo et al., 2015). Sugar and amino acid content in tomato root exudates have been shown to be differentially impacted by the application of biocontrol Fusarium sp. in comparison to pathogenic Pseudomonas bacteria (Kamilova et al., 2006). Distinctive transcriptomic responses to symbionts, as opposed to pathogens, have also been demonstrated at this early pre-symbiotic stage (Giovannetti et al., 2015). Plant responses may also vary between different isolates of the same microbial species whereby discrete modifications in plant responses to intra-specific microbial variation could correlate with improved compatibility in certain interactive pairs over others. A recent comparative study has demonstrated significant differences in the responsiveness of roots interacting with the rhizobia of different species, impacting compatibility at an early stage of interaction (i.e., 1–3 days; Kelly et al., 2018). Further, a plant hosts' metabolic regulation of amino acid or nitrogen homeostasis is suggested to determine the colonization levels of endophytes and pathogens (López-Berges et al., 2010; Stuttmann et al., 2011; Lahrmann et al., 2013). The above examples highlight the significant impact of early root responses during microbial interaction. However, we are currently lacking a comprehensive study of how the global metabolic profile of plant roots changes in response to different microbial lifestyles and to different microbial isolates within one species.
A great deal of effort has also gone into determining what signals from a microbe might initiate specific plant-based responses. The majority of these studies have focused on how plants distinguish different microbes via receptor-mediated recognition of chemical signals (Stracke et al., 2002; Radutoiu et al., 2003). In this model, specific chemical signals secreted by different microbes should activate distinct signaling pathways in the plant which effectively determine the fate of the interaction—either triggering mutualistic or defense responses. Evidence of distinct microbial signals include Nod-factors in Rhizobium-legume symbioses, Myc-factors in arbuscular mycorrhizal fungi (AM fungi)-plant symbioses or microbe-associated molecular patterns (MAMPs) in a variety of pathosystems (Felix et al., 1999; Zipfel et al., 2004; Raudaskoski and Kothe, 2014; Hacquard et al., 2017). Yet, emerging research has also pointed out that a single plant receptor can be triggered by microbial signals released by both mutualistic and pathogenic microbes (Rey et al., 2013; Miyata et al., 2014; Zhang et al., 2015). Further, instead of triggering the symbiotic pathway, mutualists can gain entry to host roots by releasing pathogen-like virulence factors that block the plant's innate immunity (Tellström et al., 2007; Thomma et al., 2011; Okazaki et al., 2013). These examples imply that plant-microbe recognition is not solely dependent on individual pairings of microbial chemical signals with corresponding plant receptors, but rather that the combination of diverse signals generated by a given microbe is required for full plant recognition. In this regard, a comprehensive account of microbial signals perceived by roots during the pre-symbiotic stage should be considered when trying to understand how plants are able to differentiate between different microbes.
In this study, we aim to examine the influence for microbes of (i) different species and lifestyles, (ii) different geographic origin, and (iii) different colonization potential, on root metabolite profiles during the stage of pre-symbiotic interaction. Experiments were conducted with Eucalyptus grandis seedlings interacting with multiple strains of a pathogenic fungus (Armillaria luteobubalina), a pathogenic oomycete (Phytophthora cinnamomi), a commensal fungus (Suillus granulatus), and a mutualistic fungus (Pisolithus microcarpus) under the same abiotic conditions. These microbes are selected for this study as they are frequently found in the soil of Australian forests and plantations.
A. luteobubalina is a common pathogen that is endemic to Australia and causes root rot of eucalypts, particularly in disturbed native forest and plantation sites (Kile, 2000). In a natural ecosystem, Armillaria infections are typically characterized by the development of white mycelial sheets, rhizomorphs and honey-colored basidiocarps at the root or lower stem (Kile, 2000). Ph. cinnamomi, on the other hand, is an introduced, hemi-biotrophic pathogen that causes root disease in over 150 native eucalypt species in Australia due to a lack of resistance toward the exotic species (Shearer and Smith, 2000). Infected trees show symptoms such as root and crown rots, chlorosis and crown thinning, and in tree mortality (Facelli et al., 2018; Sena et al., 2018). Both P. microcarpus and S. granulatus are mutualistic ectomycorrhizal (ECM) fungi but only the former can form mutualistic interactions with E. grandis. S. granulatus is an exotic fungus believed to be introduced into Australia along with the plantation of pines (Chapela et al., 2001). On the contrary, P. microcarpus is an ECM fungi that is native to Australia. Like other ECM fungi, the extra-radical mycelium of ECM fungi enhances tree nutrient acquisition effectively by acting as an extension of the root system and increasing the interface with adjacent soil. ECM fungi are capable of mobilizing organic soil N, thus providing plant host access to soil N resources that would otherwise be unavailable to uninfected plant roots (Burgess et al., 1994). However, it is worth mentioning that the benefits and colonization efficiency of ECM associations to E. grandis vary in an intraspecific manner (Plett et al., 2015).
Focusing on one lifestyle, the mutualist, we have also tracked the origin of metabolites in root tips using stable isotope tracing, as well as the transcriptomic responses of eucalypt roots during this pre-symbiotic interaction. Overall, we demonstrate that a small, but significant, number of molecular features in E. grandis are uniquely responsive to different lifestyles of microbes. Further, we identify plant metabolite responses that correlate with colonization success of the ECM fungus P. microcarpus. Our isotope tracing and transcriptomic analysis also indicated that E. grandis roots undergo specific transcriptomic changes in response to exposure to a range of fungal signaling metabolites during pre-symbiotic interaction with P. microcarpus.
Materials and Methods
Plant Materials And Microbial Isolates
Eucalyptus grandis seeds were obtained from the Commonwealth Scientific and Industrial Research Organization (CSIRO, Clayton, VIC Australia) tree seed center (Seedlot 20974). Two isolates of Armillaria luteobubalina, six isolates of P. microcarpus and two isolates Suillus granulatus, and an isolate of oomycete Phytophthora cinnamomi, were collected from several locations within New South Wales, Australia (Table 1).
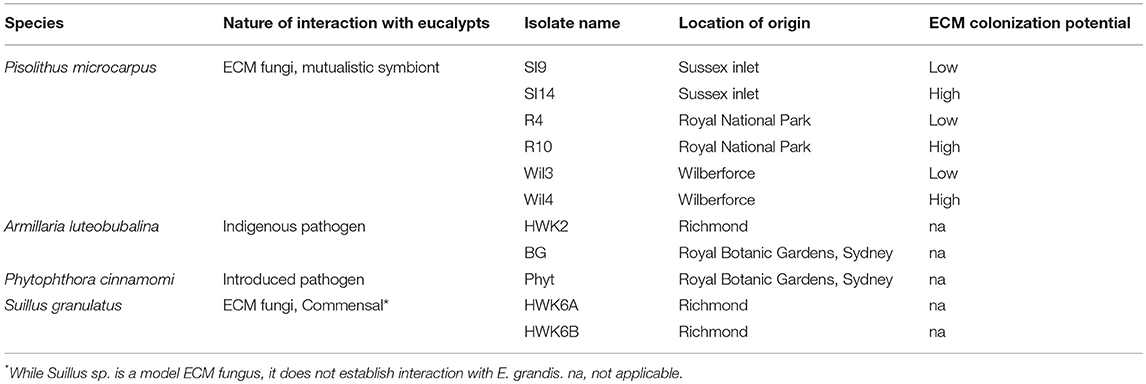
Table 1. Table summarized the species and location of origin within NSW, Australia of the fungi/oomycete isolates used in this study, and the colonization potential of the ECM fungal strains.
Plant Growth Conditions and Fungal/Oomycete Culture Condition
Seeds were sterilized in 30% H2O2 (v/v) for 10 min and then washed with sterile deionized water for five times prior to germination on 1% (w/v) water agar. After 1 month, seedlings were transferred into half-strength modified Melin-Norkrans (MMN) media (0.25 g/L (NH4)2HPO4, 0.15 g/L KH2PO4, 0.07 g/L 7H2O, 1 g/L glucose, 0.5 mL/L of CaCl2 5% stock solution, 0.5 mL/L of NaCl 2.5% stock solution, 0.5 mL/L of ZnSO4 0.3% stock solution, 67.5 μL/L of thiamine 0.1% stock solution, 0.5 mL/L of citric acid + Fe EDTA 1.25% stock solution and 13 g/L agar in de-ionized water, pH 5.5 ± 0.2) and were grown in a controlled environment growth cabinet (22–30°C night/day temperature; 16 h light cycle) for 1 month. P. microcarpus and S. granulatus isolates were cultured on the half-strength MMN media, while Ph. cinnamomi and A. luteobubalina were cultured on V8 agar media (30mM CaCO3, 15 g/L agar, 20% (v/v) V8 juice in de-ionized water, pH 7.2 ± 0.2) and potato dextrose agar (PDA) media (39 g/L PDA powder (Sigma-Aldrich) in de-ionized water), respectively, for 14 days prior to setting up the pre-symbiosis interaction.
Untargeted Root Metabolite Profiling Experimental Set-Up and Sample Collection
Three days prior to the pre-symbiotic interaction experimental set-up, all microbial cultures and eucalypt seedlings were transferred to half-strength MMN media without glucose. To allow pre-symbiotic interaction, E. grandis seedlings (2 months old) were placed into the same Petri dish as the fungal/oomycete mycelium separated by a cellophane membrane (Kleerview Covers by Fowlers Vacola Manufacturing Co Ltd.) (Figure 1). Use of a cellophane membrane allows the exchange of diffusible chemicals but prevents direct contact between seedlings and mycelium. Un-inoculated controls were also set up with the seedlings in contact with a cellophane membrane. After 24 h of pre-symbiotic interaction, root tips (~1 cm in length measured from each root tip; 10–25 mg per sample; 4 biological replicates in total) were harvested, immediately frozen in liquid nitrogen, and stored in a −80°C freezer until extraction.
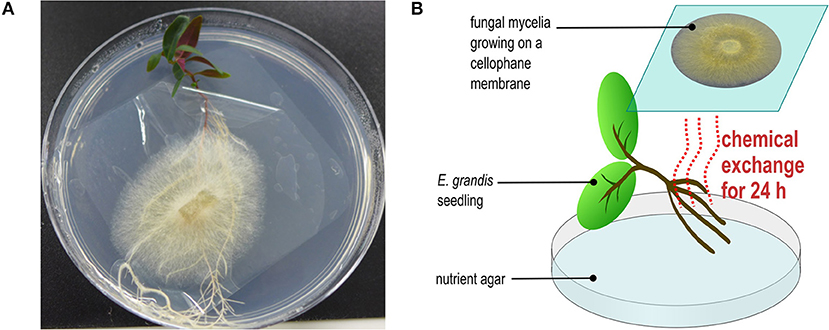
Figure 1. Experimental microcosm used for this study (A) the picture demonstrated the experimental set-up for pre-symbiotic interaction between E. grandis and microbes. (B) The conceptual diagram explained the pre-symbiosis set-up.
13C- Isotopic Labeling and Tracing Experimental Set-Up
In this experiment, the fungal mycelia of P. microcarpus was cultured in 13C-labeled media prior to pre-symbiotic interaction with E. grandis root tips. Therefore, theoretically, all fungal metabolites will have a higher 13C-labeled isotope signal than plant metabolites. Cultures of P. microcarpus isolate SI14 were grown in half-strength MMN where the normal sugar source was replaced with 99% labeled 13C6-glucose (Cambridge Isotope Laboratories, Inc.) for one month. The fungal cultures were then transferred to a half-strength MMN medium (without glucose) for 3 d, and subsequently subjected to pre-symbiotic interaction with E. grandis seedlings for 24 h as described above. The root tip samples and fungal mycelia samples were harvested and frozen in liquid nitrogen and stored in a −80°C freezer until extraction as described below.
Sample Preparation and LC-MS Analysis
Samples were extracted and analyzed by Metabolomics Australia (School of BioSciences, University of Melbourne). Aliquots (10–25 mg) of homogenized, fresh root tips were transferred to Cryomill tubes and their weights recorded. Methanol (MeOH, 250 μL per 10 mg of sample) containing the following internal standards, 13C6-Sorbitol (0.02 mg/mL) and 13C5-15N-Valine (0.02 mg/mL), was added to the sample tubes. The samples were homogenized using a Cryomill (Bertin Technologies; Program #2 (6100–3 × 45 × 45) at −10°C) and incubated in a Thermomixer (Eppendorf) at 30°C with a mixing speed of 1,400 rpm for 15 min, followed by 15 min of centrifugation at 13,000 rpm (15,900 × g). The MeOH supernatant was transferred into a 1.5 mL Eppendorf tube and set aside. De-ionized water (250 μL per 10 mg of sample) was added to the remaining sample pellet and vortexed before being centrifuged for 15 min at 13,000 rpm (15,900 × g). The supernatant was removed and combined with the MeOH supernatant. Metabolite profiling was then performed with the MeOH extract on a liquid chromatogram coupled-mass spectrometry (LC-MS) platform. Instrument and LC-MS set-up were as follows: Agilent 6520 QTOF MS system (Agilent Technologies, Santa Clara, CA, USA) with a dual sprayer ESI source and attached to an Agilent 1200 series HPLC system comprised of a vacuum degasser, binary pump, thermostated auto-sampler, and column oven. Ten microliters of sample extract was used in the injection. The MS was operated in positive or negative mode using the following conditions (ESI+/−, respectively): nebulizer pressure 30/45 psi, gas flow-rate 10 L/min, gas temperature 300°C, capillary voltage 4,000/−3,500 V, fragmentor 150, and skimmer 65 V. The instrument was operated in the extended dynamic range mode with data collected in m/z range 70–1,700. Chromatography was carried out using an Agilent Zorbax Eclipse XDB-C18, 2.1 × 100 mm, 1.8 μm column maintained at 40°C (± 1°C) at a flow rate of 400 μL min−1 with a 20 min run time. A gradient LC method was used with mobile phases comprised of (A) 0.1% formic acid in deionized water and (B) 0.1% formic acid in acetonitrile: 5 min linear gradient from 5 to 30% mobile phase B, followed by a 5 min gradient to 100% mobile phase B and then a 5 min hold, followed by a 5 min re-equilibration at 5% mobile phase B.
Metabolomics Data Preprocessing and Statistical Analysis
For data generated from untargeted root metabolite profiling, Molecular Feature Extraction (MFE) was conducted using Agilent MassHunter Qualitative analysis (version B.07.00) and MassHunter Profinder (version B.08.00). Binning and alignment tolerances were set to: retention time = ± 0.1%+0.1 min; mass window = ± 20 ppm + 5 mDa. Allowed ion species: +H, +Na, +K, +NH4, and neutral losses: H2O, H3PO4, CO2, C6H12O6. MFE was restricted to the 2,500 largest features and 1–2 charge states. The acquired data matrices were exported and further analyzed with MetaboAnalystR package (version 0.0.0.9000) (Chong et al., 2018) in R environment (version 3.4.2). All data were normalized with internal standard and sample weight, log-transformed, and auto-scaled. Univariate statistical tests (t-test and ANOVA) and multivariate statistical tests (PCA and PLS-DA) were both carried out with MetaboAnalystR. PERMANOVA analysis was done with the vegan package (version 2.5.2). A level-5 putative identification of metabolite identities was conducted by searching the exact mass and composite spectrum of molecular features against public chemical databases including METLIN (Smith et al., 2005), HMDB (Wishart et al., 2018), and Kegg (Kanehisa et al., 2006) with the use of CEU Mass Mediator (Schymanski et al., 2014; Gil de la Fuente et al., 2018). Search results matching to synthetic metabolites, drugs or animal-derived metabolites were filtered. The putative metabolite classes were described based on the common chemical structure in cases of multiple matching hits.
For the isotopic labeling experiment, peak detection, alignment and data pre-processing were performed by using the XCMS package (version 3.0.2; Smith et al., 2006) in R environment. The xcmsSet output was then introduced to X13CMS package (version 1.4; Huang et al., 2014) to detect isotopically labeled compounds. Briefly, isotopically labeled compounds were detected by selecting for detection peaks which exhibit a positive shift of mz-value around the same retention time as the original unlabeled counterpart (Huang et al., 2014). The relative abundance of each 13C-labeled compound was calculated by dividing the 13C-labeled compound intensity detected in labeled condition by that in unlabeled control condition.
Transcriptomic Profiling of P. microcarpus Interacting E. grandis
E. grandis seedlings generated as described above were set up to interact with P. microcarpus isolate SI14 in two contrasting conditions—pre-symbiotic contact and direct physical contact for 24 h. The former was prepared as mentioned above. To set up the latter, E. grandis seedling roots were placed directly onto the mycelia of P. microcarpus growing on half-strength MMN under the same growth conditions. An untreated E. grandis control was prepared in parallel. After 24 h, the same type of root tissues of E. grandis seedlings, as described for metabolomic profiling, were sampled and immediately frozen with liquid N2. In total, four biological replicates for each condition were taken and extracted. The RNA from the root samples were then extracted with ISOLATE II Plant miRNA kit (Bioline) as per the manufacturer's instructions. RNA for four biological replicates each of the conditions were sequenced at the Joint Genomes Institute (JGI). Plate-based RNA sample prep was performed on the PerkinElmer Sciclone NGS robotic liquid handling system using Illumina's TruSeq Stranded mRNA HT sample prep kit utilizing poly-A selection of mRNA following the protocol outlined by Illumina in their user guide: https://support.illumina.com/sequencing/sequencing_kits/truseq-stranded-mrn a-workflow.html, and with the following conditions: total RNA starting material was 100 ng per sample and 10 cycles of PCR was used for library amplification. The prepared libraries were quantified using KAPA Biosystem's next-generation sequencing library qPCR kit and run on a Roche LightCycler 480 real-time PCR instrument. The quantified libraries were then multiplexed with other libraries, and the pool of libraries was then prepared for sequencing on the Illumina HiSeq sequencing platform utilizing a TruSeq paired-end cluster kit, v4, and Illumina's cBot instrument to generate a clustered flow cell for sequencing. Sequencing of the flow cell was performed on the Illumina HiSeq2500 sequencer using HiSeq TruSeq SBS sequencing kits, v4, following a 2 × 150 indexed run recipe. Raw RNA-Seq reads were filtered and trimmed using the JGI QC pipeline. Using BBDuk (https://sourceforge.net/projects/bbmap/), raw reads were evaluated for artifact sequence by kmer matching (kmer = 25), allowing 1 mismatch and detected artifact was trimmed from the 3' end of the reads. RNA spike-in reads, PhiX reads, and reads containing any Ns were removed. Quality trimming was performed using the phred trimming method set at Q6. Finally, following trimming, reads under the length threshold were removed (minimum length 25 bases or 1/3 of the original read length—whichever was longer).
Filtered reads from each library were aligned with the E. grandis genome (Myburg et al., 2014). Only primary hits assigned to the reverse strand were included in the raw gene counts (-s 2 -p −−primary options). Raw gene counts were used to evaluate the level of correlation between biological replicates using Pearson's correlation and to determine which replicates would be used in the Differential Gene Expression analysis. DESeq2 (version 1.18.1; Love et al., 2014) was subsequently used to determine which genes were differentially expressed between pairs of conditions. Features assigned to the forward strand were also tabulated (-s 1 -p −−primary options). The strandedness of each library was estimated by calculating the percentage of reverse-assigned fragments to the total assigned fragments (reverse plus forward hits).
Arabidopsis thaliana homologs of upregulated genes (log(fold change) > 5) in E. grandis were used to perform gene ontology (GO) enrichment with g:Profiler (Reimand et al., 2007). A GO enrichment network was then generated with Enrichment Map plugin (version 3.1.0; Merico et al., 2010) on the Cytoscape platform (version 3.6.1; Shannon et al., 2003). Gene pathway analyses were performed by using Pathview Web (Luo et al., 2017). Gene expression values for Arabidopsis thaliana homologs in E. grandis were mapped onto metabolite biosynthesis pathways on the basis of KEGG pathway database.
Results
Metabolite Responses Triggered by Microbes With Different Lifestyles
Using LC-MS-based untargeted metabolite profiling, a total of 1,868 and 2,226 molecular features were identified in 48 E. grandis root tip samples across all treatments in the ESI+ and ESI− mode, respectively (Supplementary Tables 1, 2). A principle component analysis (PCA) of the E. grandis root tip metabolite profiles failed to show any clear separation based on either the species or trophic lifestyle of the interacting microbes (Figure 2A; Supplementary Figure 1). However, a permutational multivariate analysis of variance analysis (PERMANOVA) confirmed a significant variation among E. grandis root tip metabolite profiles in relation to different species of microbes (p = 0.018, R2 = 0.1291 at 1,000 permutations for ESI+ mode; p = 0.012, R2 = 0.1261 at 1,000 permutations for ESI− mode).
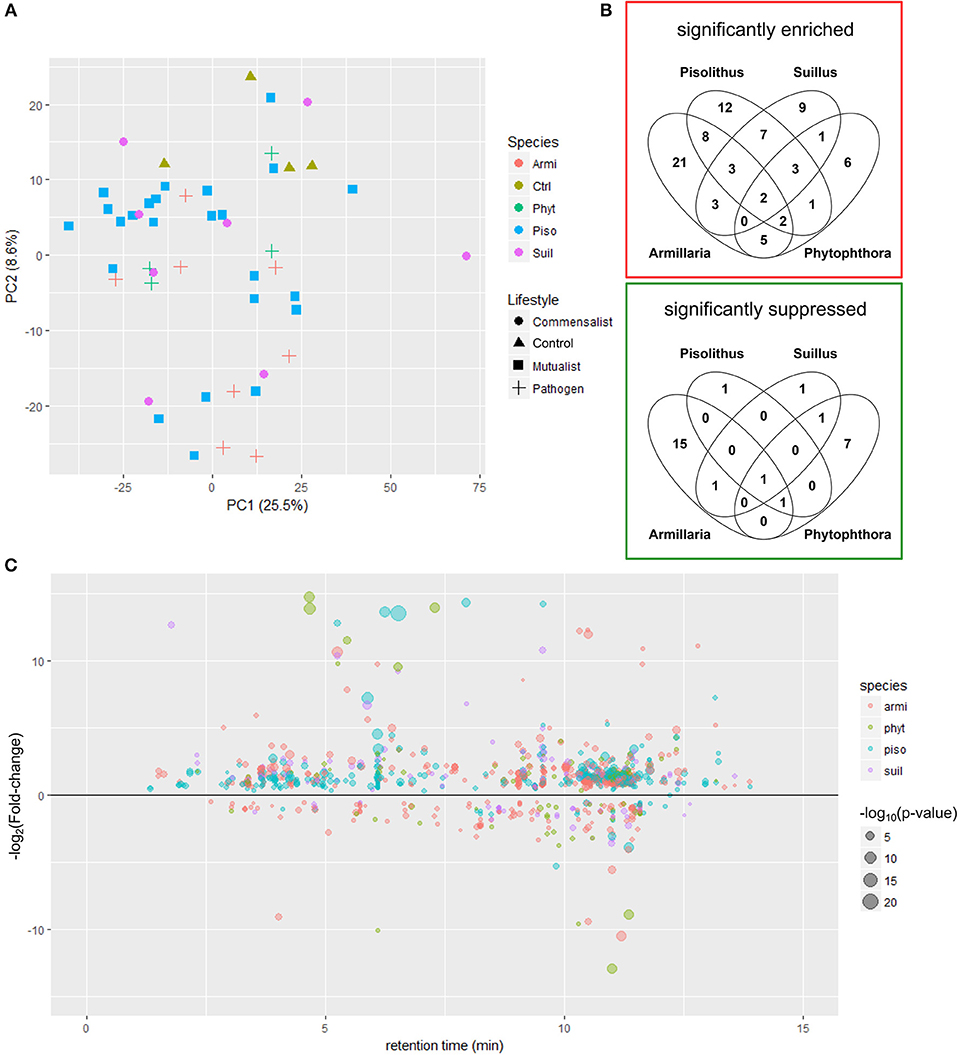
Figure 2. Metabolite responses triggered by different lifestyles of interacting microbe (A) PCA score plot showing the variation among metabolite profiles (LC-MS ESI+ mode) of E. grandis root tips after 24 h pre-symbiosis with different microbes. The color and shape of symbols represent the species and the lifestyles of the interacting microbes. Armi, Armillaria luteobubalina; piso, Pisolithus microcarpus; suil, Suillus granulatus; phyt, Phytophthora cinnamomi; ctrl, uninfected control (B) venn diagrams showing the significantly enriched and suppressed molecular features amongst different microbe-treated roots in comparison to the untreated control. Student t-test was performed for each pair of comparison between the control and the microbes-treated root tips. Significantly regulated molecular features profiled with ESI+ mode that exhibit more than 5-fold of intensity change and with p < 0.05 are shown in the Venn diagram. (C) The scatter plot showing distribution of the significantly regulated metabolites (p < 0.05) across the span of retention time of metabolite profiling in root tips after pre-symbiotic interaction with microbes of different species that are captured in LC-MS (ES+ mode).
We sought to identify the key metabolite responses that separated one microbial species from another. A Venn diagram analysis of the ESI+ mode showed that several significantly induced and suppressed molecular features are specifically regulated depending upon which microbe is interacting with E. grandis (Figure 2B; Supplementary Table 3; ESI− mode in Supplementary Figure 2). Generally, there were more molecular features significantly induced than suppressed by interaction with any of the tested microbes (Figure 2C; ESI− mode in Supplementary Figure 3). In ESI+ mode, 38, 44, 28, and 20 molecular features were identified to be significantly regulated (p < 0.05; fold change > 5) after 24 h pre-symbiosis with P. microcarpus, A. luteobubalina, S. granulatus, and Ph. cinnamomi, respectively (Figure 2B; Supplementary Table 3). A number of these molecular features induced by different microbial species (as shown in different color in Figure 2C) were eluted at a different retention time across the chromatograph, suggesting that they are potential specific metabolite responses toward different microbial species. For instance, 12 molecular features were specifically upregulated in root tips interacting with P. microcarpus. Most of the suppressed molecular features correspond to responses expected toward pathogens (i.e., A. luteobubalina and/or Ph. cinnamomi) rather than mutualists (i.e., P. microcarpus) and commensalists (i.e., S. granulatus). For instance, there were 15 significant molecular features exclusively suppressed by A. luteobubalina as opposed to only one molecular feature significantly suppressed by P. microcarpus.
By matching the neutral mass of the molecular features to public databases, we have putatively identified some metabolites or their functional class (Table 2). Most significantly, the regulated molecular features belongs to common plant root metabolite classes such as terpenoids, flavonoids and fatty acids. However, some molecular features identified in root tips also appear to be of microbial origin. For example, Cp1793, a molecular feature that is exclusively induced by P. microcarpus, is putatively identified as hypaphorine (Table 2). Hypaphorine is a known auxin inhibitor secreted by Pisolithus which modulates plant root development during the early phase of ECM establishment (Ditengou and Lapeyrie, 2000). Several A. luteobubalina-induced molecular features also matched to known sesquiterpenes derived from Armillaria species (i.e., armillarilin and armillarinin; Table 2; Kobori et al., 2015).
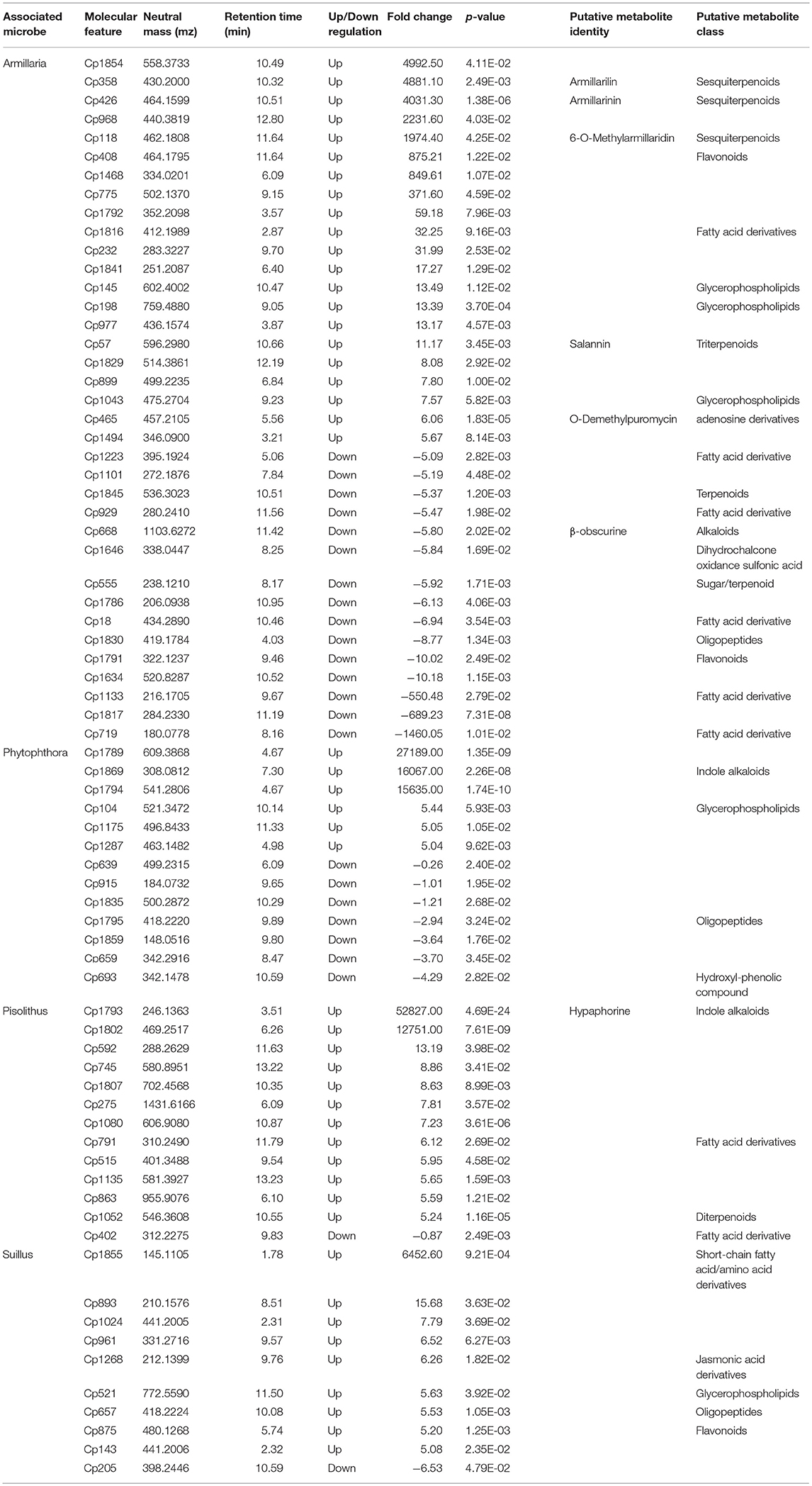
Table 2. Table describing the specifically-regulated molecular features and their putative identities in root tips by different associated microbes (ESI+ mode).
The Relationship Between Root Metabolite Responses and Colonization Potential of Pisolithus Isolates
We wished to gain a more in-depth understanding of the diversity of host metabolic responses toward different isolates within one species of fungus. Therefore, we ran further tests on the early metabolite responses of E. grandis roots with six different isolates of P. microcarpus isolated from different geographic locations and which exhibited differing host colonization potential (Plett et al., 2015). By performing a univariate t-test, the metabolite profiles of root tips after pre-symbiotic interaction with P. microcarpus (n = 24) was compared with the untreated control root tip samples (n = 4). Overall, there were more molecular features induced in the E. grandis root tips than suppressed by the pre-symbiotic interaction with P. microcarpus in either the ESI+ mode (Figure 3) or ESI− mode (Supplementary Figure 4).
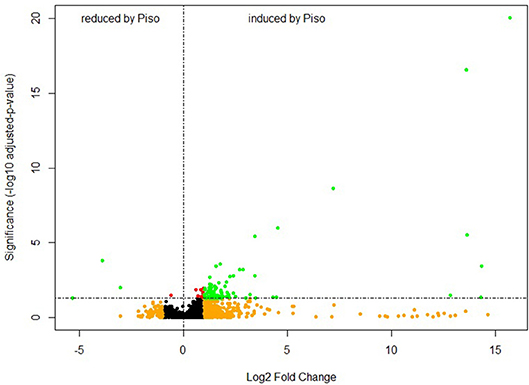
Figure 3. Pre-symbiotic interaction induces a large number of metabolites in E. grandis roots. Volcano plot showing the varied molecular features in E. grandis root tips after pre-symbiosis with P. microcarpus (n = 24) for 24 h in comparison to control root tips (n = 4). The metabolite profiles are derived from LC-MS (ESI+ mode). The y-axis and x-axis correspond to the mean fold change of Pisolithus-interacting root tips in comparison to control, and the significance value in terms of p-value (adjusted by Bonferroni-method). Red = p < 0.05; Orange = fold change > 2; Green = p < 0.05 and Intensity fold change >2.
The PCA plot of the metabolite profiles in ESI+ mode (Figure 4A), showed a separation between control root tips and those interacting with Pisolithus isolates. Further, the metabolite profiles for root tips interacting with Pisolithus isolates clustered according to the colonization potential (p = 0.001, R2 = 0.2506 at 1,000 permutations), suggesting a possible correlation between early metabolic responses of the host root during pre-symbiosis and later colonization success. In ESI− mode, there was no similar metabolic separation based on either mycorrhization rate or geographic origin (Supplementary Figure 5). Despite this, PERMANOVA indicated that the colonization rate was a significant predictor of the root tip metabolite profile dispersion in ESI− mode, as is in the ESI+ mode (p = 0.001, R2 = 0.1878 at 1,000 permutations).
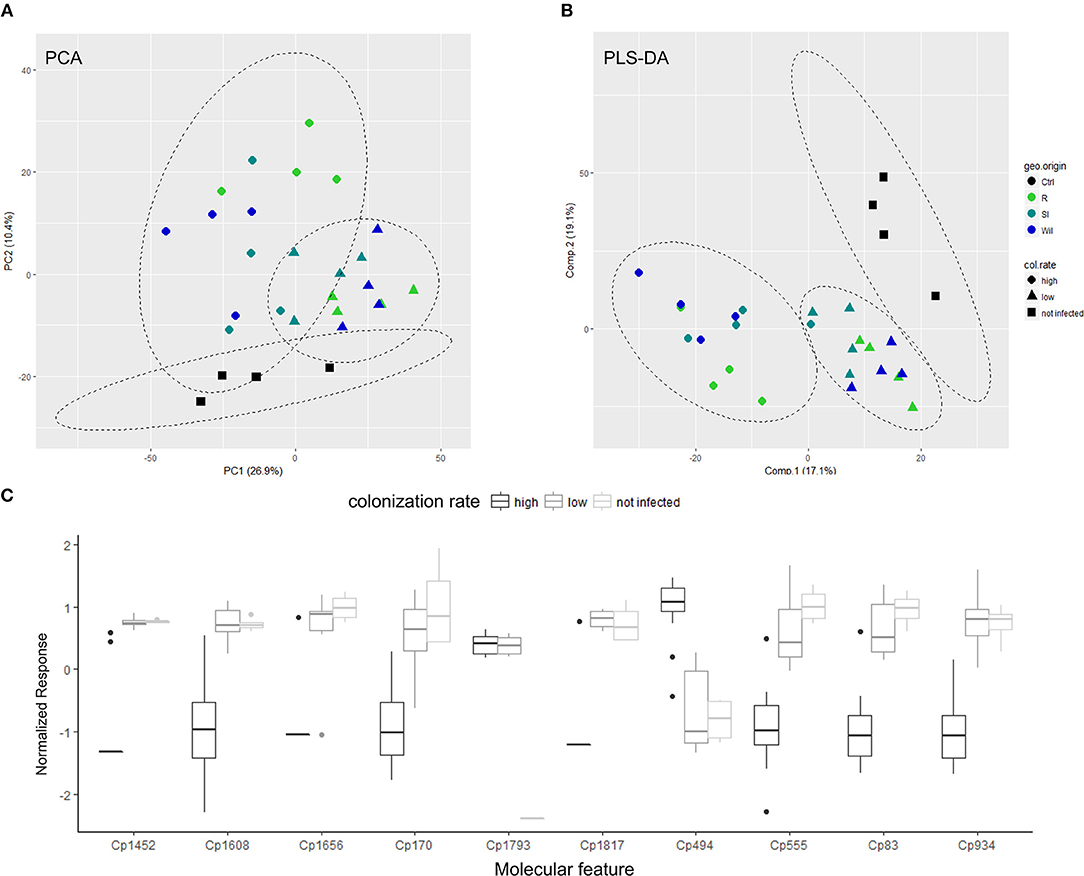
Figure 4. Specific metabolites exhibit correlation to root colonization potential of interacting-Pisolithus isolate. (A) PCA score plot showing the multivariate-variation among Pisolithus-interacting root tips samples in terms of metabolite profiles derived from LC-MS (ESI+ mode). (B) PLS-DA score plot showing the first two components of the supervised classification PLS model that separates metabolite profiles of Pisolithus-interacting root tips samples in terms of colonization rate. The color of the symbols represents the geographical origin (geo. origin) of the Pisolithus strains, while the shape of the symbols represents the colonization rates (col. rate). Ctrl, un-infected control roots; R, Royal National Park; SI, Sussex Inlet; Wil, Wilberforce. (C) The boxplot depicting the response levels of the top-10 important features with highest VIP scores that are derived from the first component of the PLS model. Responses = intensity of compound peak/intensity of internal standard / fresh weight (g). Normalization of metabolite responses is done with generalized logarithm followed by autoscaling.
In an effort to identify the important early metabolite responses that correlate with later colonization success by P. microcarpus isolates, we performed a supervised-multivariate analysis—partial least square-discriminant analysis (PLS-DA) on the metabolite profiles of Pisolithus-treated root tips. The performance and fitting of the PLS model was evaluated by an internal cross-validation and permutation test to have significant predictive power and to be non-random (R2 = 0.9958, Q2 = 0.6851, Accuracy = 0.8929 at 4 components with leave-one-out cross-validation, p = 0.008 at 1,000 permutations). As shown in Figure 4B, the PLS model clearly separates root metabolite profiles corresponding to colonization potential (ESI− mode in Supplementary Figure 6). According to the first component of the model, the top 25 important features with the highest discriminative capacities in terms of Variable Importance in Projection (VIP) scores were selected for further characterization based on the mass-to-charge ratio (mz) value and retention time (Table 3). VIP score is an index measuring the importance for the selected features in explaining the predefined classification, which in this case are the uninfected control, low colonization, and high colonization groups (Cho et al., 2008). The higher the VIP score is, the more important the feature is. VIP scores > 1.0 (Weljie et al., 2011; Davis et al., 2012) are generally used as a cut-off for selecting important features, and all of the top 25 important features selected in Table 3 have achieved a VIP score > 2. Important features such as Cp 170, a fatty acid derivative, and Cp494, a diterpenoid, demonstrated distinctive responses in root tips that were interacting with Pisolithus isolates of high colonization potential vs. those of low colonization potential (Figure 4C; Table 3). Intriguingly, besides Cp 494, most of the important molecular features that co-vary with colonization potential appear to have a negative relationship with colonization potential (Figure 4C). Cp1793, putatively fungal-derived hypaphorine as mentioned above, were also selected by the PLS model as one of the important features that were induced by the presence of Pisolithus (Figure 4C).
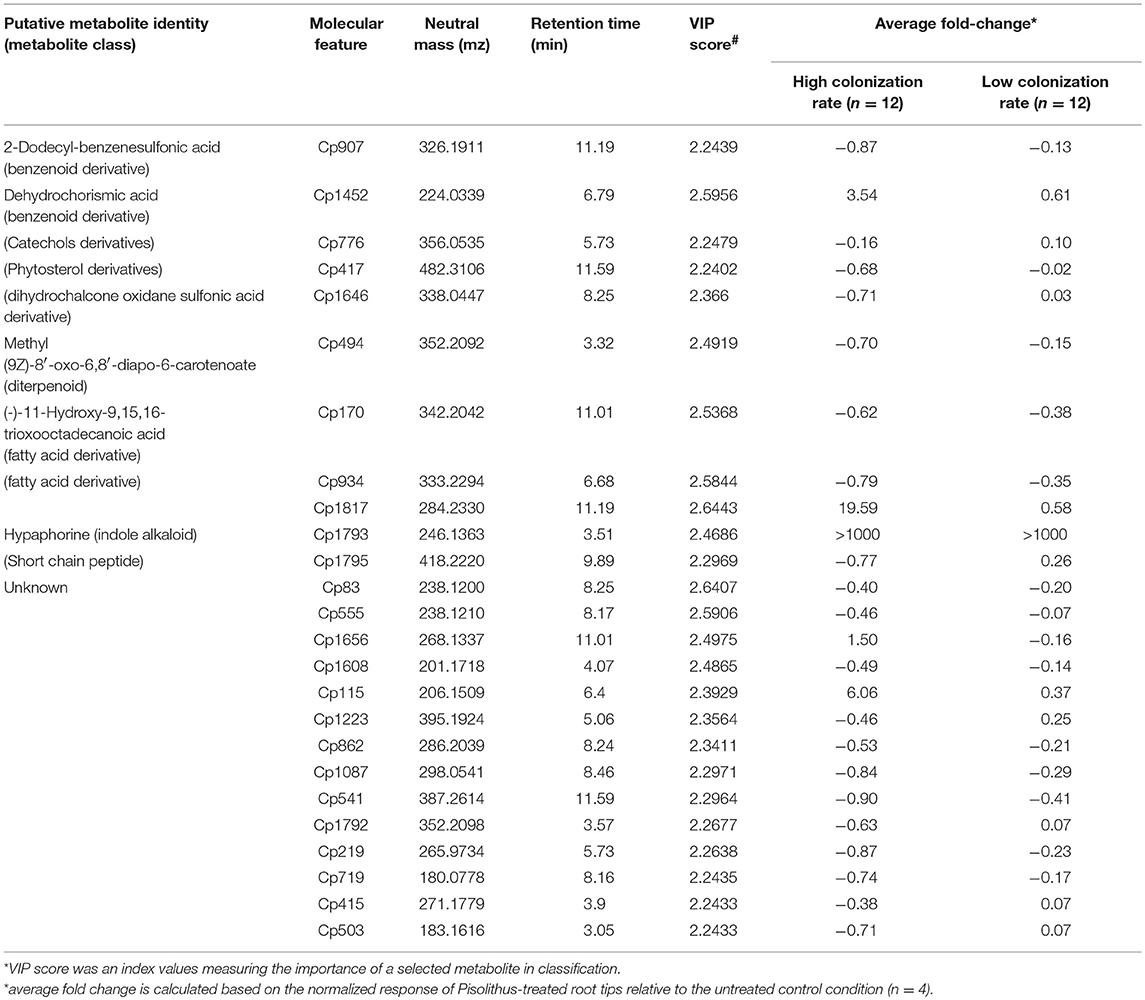
Table 3. Putative identities and response levels in Pisolithus-treated root tips of top-25 important molecular features identified in PLS-DA (LCMS-ESI+ mode).
Fungal Signaling Metabolites Are Detected in Roots During Pre-symbiotic Interaction
The discovery of a hypaphorine-like metabolite in the pre-symbiotic roots of E. grandis led us to investigate what proportion of the metabolites detected in the plant tissues came from the fungus via secretion. We conducted a stable isotope labeling and tracing experiment in conjunction with metabolite profiling to investigate the origin of the detected metabolites in root tips interacting with Pisolithus. Our experimental setup successfully incorporated 13C onto most of the Pisolithus fungal metabolites. The labeling efficiency of fungal mycelia was evaluated by computing the average percentage of 13C-isotope-labeled metabolite intensity corresponding to each of the 1084 detected metabolite species (ESI+ mode in Figure 5A; ESI− mode in Supplementary Figure 7). Overall, there is a significantly higher abundance of the 13C-isotope-labeled metabolite in labeled fungal mycelia (mean = 88.62%) in comparison with the natural 13C-isotope abundance in unlabeled control (mean = 14.40%). After pre-symbiotic interaction with 13C-labeled P. microcarpus mycelia, the root tips samples exhibited two major groups of metabolites; one with a significantly higher abundance of 13C-labeled metabolites (>10 log (relative abundance)) and another with natural 13C-labeled abundance (Figure 5B). The 13C-labeled metabolites (n = 201) are likely to have originated from the isotopically-labeled P. microcarpus, while the latter set of metabolites (n = 317) originate from plant processes. Generally, 13C-labeled fungal metabolites were detected in low absolute intensity as compared to plant derived metabolites. This observation suggests that this isotopic labeling and tracing approach can detect a range of Pisolithus fungal signaling metabolites in E. grandis roots during pre-symbiotic interaction, but the abundance of these metabolites remains low as compared to plant metabolites.
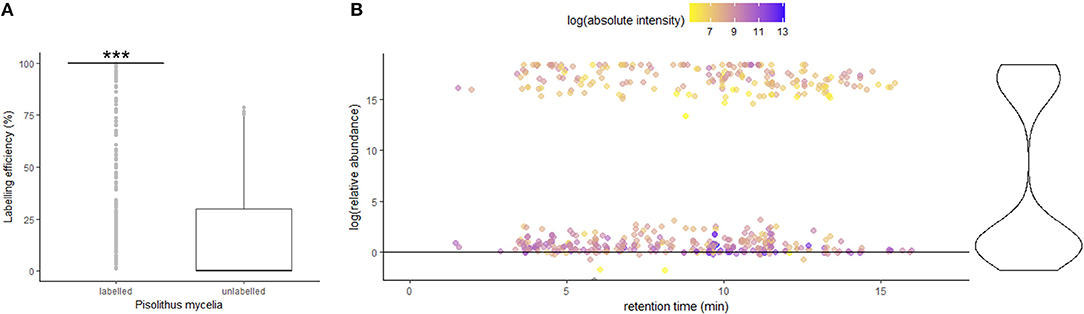
Figure 5. Fungal signaling metabolites are detected in roots during pre-symbiotic interaction (A) Box plot depicting the 13C-labeling efficiency of each compound detected in Pisolithus mycelia samples (n = 2 per condition) in labeled setup compared with unlabeled setup (negative control). Labeling efficiency = intensity of 13C isotope-labeled compound/(intensity of 13C -labeled compound + unlabeled compound) × 100. The asterisks (***) indicate a highly significant difference in terms of labeling efficiency in labeled condition in comparison to unlabeled control (p < 2.2e−16 with paired t-test). (B, left) Scatter plot showing the abundance of 13C-labeled compounds in Pisolithus-interacting root tips in labeled setup in relation to unlabeled setup, across the retention time span of LC-MS ESI+ mode metabolite profiling. The color of the points showing the absolute intensity of the compound peak detected by the LC-MS. An unlabeled or mis-detected labeled metabolite would have a log (relative abundance) ≤ 0. (B, right) The violin plot demonstrates the distribution of 13C-labeled compounds with different relative abundance.
Pre-symbiotic Signals From P. microcarpus Induce Distinct Gene Signaling Networks in E. grandis Roots When Compared to Direct Physical Contact
As the 13C labeling and tracing experiment recovered a portion of fungal metabolites in P. microcarpus-interacting root tips during the pre-symbiotic stage, we carried out transcriptomic profiling to test if there were plant host genes that could be transcriptionally responsive solely to these putative fungal signaling compounds. We achieved this by comparing the root transcriptomic changes during pre-symbiotic interaction with that of the roots in direct physical contact with the P. microcarpus. A comparable number of genes were differentially expressed in both interaction conditions. We identified 654 highly-induced genes (log(fold-change) > 5) and 565 repressed genes (log(fold change) <−5) in roots after pre-symbiotic interaction, while there were 554 induced genes and 662 repressed genes in roots that are in direct physical contact. Amongst genes that are differentially regulated in the pre-symbiotic interaction, disease resistance gene families such as Leucine-rich repeat protein kinase and Cysteine-rich receptor kinases are over-represented (Supplementary Table 4). Furthermore, the differential responses amongst genes related to disease resistance showed that there is a selective activation/repression of pathogen-related receptor responses toward P. microcarpus. Gene ontology (GO) enrichment analysis suggests gene-sets up-regulated in E. grandis roots during pre-symbiotic interaction are related to metabolism of organic substances and other metabolites, interaction with organisms (e.g., response to other organism, multi-organism process, response to external biotic stimulus) and transporter activity (Supplementary Figure 8). These enriched GO terms are aligned with the metabolic induction we observed in the abovementioned metabolomic analysis. The enriched gene-sets are different during pre-symbiotic interaction in comparison with direct physical contact (Figure 6; Supplementary Table 5). Gene-sets corresponding to the metabolic compound process and transporter activity are exclusively upregulated in pre-symbiotic interaction. On the other hand, there are overlapping gene-sets with functions corresponding to response to other organisms and response to chemical stimuli are enriched in both interaction conditions. As our metabolomics analysis (Table 2) suggested that terpenoids, flavonoids and fatty acids are the main metabolite classes induced by pre-symbiotic interaction with P. microcarpus, we examined the expression patterns of the gene-sets associated to their biosynthesis pathways (Supplementary Figures 9–12). Congruent with our metabolic analysis, the expression of key genes involved in the biosynthesis of these chemical groups were significantly induced by pre-symbiotic contact. For example, the downstream reactions for fatty acid, diterpenoid and flavonoid biosynthesis pathways were induced by Pisolithus pre-symbiotic interaction (Supplementary Figures 10–12). However, the gene expression patterns for these enzymes in pre-symbiotic contact condition were different from those in the direct physical contact condition.
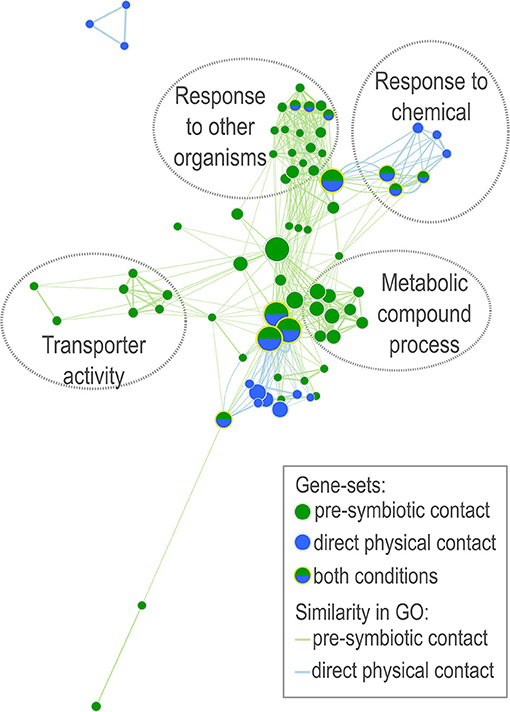
Figure 6. Network analysis of early transcriptomic responses of E. grandis to P. microcarpus. The network illustrates the gene ontology (GO) enrichment resulted with transcriptome data derived from E. grandis roots after two different kind of contact interaction with mycelia of P. microcarpus —pre-symbiotic contact (green) and direct physical contact (blue). Only significantly up-regulated genes in comparison to untreated control roots with log(fold change) > 5 are included. Circled with dotted-lines are clusters of enriched gene-sets with related GO functions. Node size represents the number of genes within the enriched gene-set of certain GO (q < 0.1). Edges connect gene-sets with overlapping genes (similarity coefficient > 0.5). Edge thickness and length are correlated with the similarity coefficient between gene-sets.
Discussion
To prime symbiotic interactions, it is well-established that plants and their interacting microbes alter in physiological parameters and growth just prior to both parties coming into physical contact (Fries et al., 1987; van Brussel et al., 1992; St-Arnaud et al., 1996; Beguiristain and Lapeyrie, 1997; Felten et al., 2010). Such changes during the pre-symbiotic stage are mediated by an exchange of various metabolic signals that define the outcome of these interactions (Steinkellner et al., 2007). In the present study, we used E. grandis roots as a model to assess the effect of microbial pre-symbiotic interaction on the root metabolome. With untargeted LC-MS metabolite profiling, we observed that microbial interaction led predominantly to an accumulation of metabolites in E. grandis. Our analyses suggest a significant specificity of the root metabolite responses toward the microbial species, as well as a correlation with subsequent colonization success. Therefore, metabolic regulation must be considered when attempting to understand plant-microbe interactions.
In terms of tree-ECM fungal interaction models, a study by Zmasek et al. (2016) has predicted the metabolic reprogramming in Populus-Laccaria interaction at early pre-symbiotic phase through in silico modeling based on transcriptomic datasets. Yet, as far as we know, our present study is one of the few assessing the global metabolite responses in tree roots during pre-symbiotic interaction with ECM fungi. Furthermore, few studies have simultaneously compared root responses to multiple different microbes prior to infection using the same experimental system. Our current study demonstrates that plant metabolite changes occur with partial specificity toward different microbial species attempting to enter symbiosis with the plant. This is in agreement with past research in this field (Kamilova et al., 2006; Giovannetti et al., 2015; Kelly et al., 2018). While we were unable to determine the exact identities of the specific metabolites in our tissues, they fell into several common plant metabolite classes such as terpenoids, flavonoids, and fatty acids. Terpenoids and flavonoids are generally known to be secreted by roots and mediate microbial interactions (Steinkellner et al., 2007). Strigolactones, which are terpenoids, are phytohormones that induce AM fungi—plant interactions (Akiyama et al., 2005). Similarly, flavonoids are also known to attract rhizobia into nodulation with legume plants (Steinkellner et al., 2007). Given our finding that some of these metabolites are specifically induced by only one fungal species, these metabolites have the potential to be a part of plant-encoded microbe-specific signaling pathways that enable tailored responses on the part of the plant to microbes of different lifestyles. In addition to species-specific metabolite responses, our results indicate that there are also general, overlapping, root metabolite responses triggered by pathogens, commensalists and/or mutualistic microbes. This suggests that, in addition to highly specific metabolites, there are also generic metabolites regulated in E. grandis roots in response to microbial presence. This is in agreement with previous studies that pointed out the overlapping perception mechanisms toward both pathogenic and mutualistic microbes in rice and legumes (Rey et al., 2013; Miyata et al., 2014; Zhang et al., 2015).
In our previous study, we showed that different isolates of P. microcarpus exhibit a variation in colonization rates with E. grandis (Plett et al., 2015). Fungal secretory metabolites such as sesquiterpenes and hypaphorine, as well as plant metabolites including rutin and abietic acid have been previously characterized as signaling molecules activating symbiotic plant-ECM interactions (Fries et al., 1987; Ditengou and Lapeyrie, 2000; Lagrange et al., 2001; Akiyama et al., 2005). Upon exchange of metabolite signals, lateral root growth and ECM fungal mycelia growth toward the plant roots were facilitated, leading to the initiation of colonization (Martin et al., 2001). Despite the fact that the aforementioned pre-symbiotic metabolite signaling is considered to be crucial for ECM colonization, the contribution of these metabolite responses in the early phase of interaction to the observed intra-specific variation in ECM colonization success was unexplored. By making use of the same P. microcarpus isolates, we have demonstrated that root metabolite responses at the early pre-symbiotic interaction (24 h after inoculation) are correlated with the ultimate colonization success (measured at 1 month after inoculation). The important metabolite responses that differentiated between P. microcarpus isolates of high and low colonization rates were mainly suppressed by P. microcarpus isolates with high colonization potential while they stayed at similar levels to untreated control roots when P. microcarpus isolates with low colonization potential were present. In other words, repression of these metabolites appears to be necessary during the pre-symbiotic interaction for the fungus to achieve high levels of root colonization. Given that these metabolites were repressed in Pisolithus colonized roots, they are likely to be plant metabolites rather than fungal. This result suggests that the general suppression of plant metabolic responses during early pre-symbiotic ECM interaction may be crucial for roots to establish successful ECM colonization in later stages. A possible interpretation is that the Pisolithus isolates of the high colonization group are more successful in inactivating the plant immune responses. This result is in agreement with the transcriptomic study by Giovannetti et al. (2015), which demonstrated suppression of defense-related responses in Lotus roots after 48 h of pre-symbiotic interaction with mutualistic mycorrhizal fungal exudates.
There is currently insufficient published information on tree root and fungal metabolites, making it challenging to determine the origins of detected molecular features without any a priori knowledge of their identities. In this study, we leveraged the use of stable 13C-isotopic tracing of fungal metabolites in conjunction with untargeted metabolite profiling to differentiate plant metabolites from fungal metabolites. Isotopic labeling with 13C and 15N are common practice in rhizosphere studies to trace the fate and partitioning of carbon and nitrogen flow between plants and soil microbes (Haichar et al., 2016). However, most of these studies focused on the transfer of total carbon in established plant-soil microbial interactions rather than the exchange of specific metabolite signals prior to that (e.g., Simard et al., 1997; Deslippe et al., 2016). Also the approach used by these studies can only identify the overall isotopic composition of the overall metabolite pool (e.g., Simard et al., 1997), or target metabolite in companies with appropriate internal standards (e.g., Deslippe et al., 2016). On the contrary, the approach we adopted (i.e., incorporation of isotopic labeling with untargeted metabolomics) predicted the labeling pattern of each individual metabolite species detected, giving a more comprehensive ability to track each metabolite's fate (Huang et al., 2014). Similar approaches have been used to track metabolites produced by Pseudomonas syringae during interaction with Arabidopsis epidermal cells on the leaves (Pang et al., 2018). Our result suggested that a significant number of detected molecular features (202 out of 518 in total) in Pisolithus-treated roots are highly 13C-enriched, implying that these 13C-labeled metabolites are putatively originated from the ECM fungus even after only 24 h of interaction, despite the low detection intensity. It is uncertain whether these detected ECM fungal metabolites were translocated into the root tissues or remains on the root surface. However, the mass-to-charge ratio of most of the detected metabolites by an untargeted metabolomics approach here is small (mz range 70–1,700). Given that known fungal signaling molecules with a comparable mz range such as lipochitooligosaccharides and chitin oligomers for AM fungal symbiosis are known to diffuse into root tissues (Maillet et al., 2011; Genre et al., 2013), it is plausible that the ECM fungal metabolites detected here in the present study were diffusible as well. A further comparative transcriptomic analysis on E. grandis roots confirmed that plant genes of functions related to plant-microbial interaction are activated during pre-symbiotic interaction. Besides the metabolism for synthesis and processing of metabolites, the induction of genes related to transporter activity and interaction with other organisms also suggested that the roots perceived fungal metabolite signals. The activation of these genes is also interaction-context dependent—in pre-symbiotic interaction as opposed to direct physical contact. A selection of genes corresponding to biosynthesis pathways for flavonoid, terpenoid and fatty acid were also upregulated in E. grandis in response to the Pisolithus pre-symbiotic interaction. Altogether, these findings imply that there are many more microbial metabolite signals perceived by plants in additional to what has previously been reported. Furthermore, sole perception of these microbial metabolite signals without actual physical contact occurring in pre-symbiotic interaction, is likely responsible for an activation of distinctive gene-sets in the host that affect the later stage of interaction.
In conclusion, we have provided a comprehensive account on the metabolite responses in roots of E. grandis seedlings during the pre-symbiotic interaction with a range of microbes. We revealed distinctive root metabolite response patterns in relation to microbes of different lifestyles, as well as the colonization potential of isolates within the same microbial species. Like many other untargeted metabolomics studies, our present study provided limited putative identification for the detected molecular features with a low level of confidence due to the technical difficulties in metabolite identification (Dunn et al., 2013). Despite this drawback, we have harnessed the advantageous high sensitivity provided by untargeted metabolomics and coupled it with the use of stable isotope labeling to uncover a significant number of microbial signaling metabolites perceived by plant roots. While specific pairings of “key” microbial chemical signals with plant receptors are generally accepted to serve as a means by which plants distinguish different microbes (Felix et al., 1999; Zipfel et al., 2004; Raudaskoski and Kothe, 2014; Hacquard et al., 2017), our results would argue that, instead of relying on individual microbial signal, plant recognition of different microbes depends on a collection of metabolite cues released by the microbe. Further work is needed to understand the role of this compendium of microbial signaling metabolites in plant-microbial interaction.
Data Availability Statement
The raw data generated from the metabolomics analysis can be accessed through the following link: http://hie-pub.westernsydney.edu.au/f9e58de4-1e8b-11e9-b26c-525400daae48/. The sequencing data can be found in the Short Read Archive on National Center for Biotechnology Information (NCBI) with the following accession number (SRX3905006, SRX3905005, SRX3905007, SRX5064673, SRX5064680, SRX5064681, SRX5064682, SRX5064676, and SRX5064687).
Author Contributions
JW and JP designed and conducted the experiments and sample collections. AL, SN, and UR conducted the metabolite profiling. MW, VN, and IG generated the transcriptomic analysis data. JW performed the data analysis with input from JP, AL, and SN. JW wrote the first draft of the manuscript under the supervision of JP and IA. All authors contributed substantially in revision.
Conflict of Interest Statement
The authors declare that the research was conducted in the absence of any commercial or financial relationships that could be construed as a potential conflict of interest.
Acknowledgments
JW would like to thank Western Sydney University for a PhD research scholarship and JP would like to acknowledge the Australian Research Council for research funding (DE150100408). We would also like to thank J. Rigg, E. Liew, and M. Laurence from the Botanic Gardens & Centennial Parklands for providing isolates of Armillaria luteobubalina and Phytophthora cinnamomi and S. Hortal for providing the Richmond isolates of A. luteobubalina and Suillus. The metabolite analysis was conducted at Metabolomics Australia (School of BioSciences, The University of Melbourne, Australia), a NCRIS initiative under Bioplatforms Australia Pty Ltd. We would like to thank K. Plett for aid in metabolic compound identification. FM's research group is funded by the Laboratory of Excellence ARBRE (ANR-11-LABX-0002-01) and the Region Lorraine Research Council. The work conducted by the U.S. Department of Energy Joint Genome Institute, a DOE Office of Science User Facility, is supported by the Office of Science of the U.S. Department of Energy under Contract No. DE-AC02-05CH11231. We would also like to acknowledge the New South Wales National Parks and Wildlife Services for approving the collection of the Pisolithus fruiting bodies (scientific license number S13146).
Supplementary Material
The Supplementary Material for this article can be found online at: https://www.frontiersin.org/articles/10.3389/fevo.2019.00010/full#supplementary-material
Supplementary Figure 1. PCA score plot showing the variation among metabolite profiles (LC-MS ESI− mode) of E. grandis root tips after 24 h pre-symbiosis with different microbes. The color and shape of symbols represent the species and the lifestyles of the interacting microbes. armi, Armillaria luteobubalina; piso, Pisolithus microcarpus; suil, Suillus granulatus; phyt, Phytophthora cinnamomi.
Supplementary Figure 2. Venn diagrams showing the significantly enriched and suppressed molecular features amongst different microbe-treated roots in comparison to the untreated control. Student t-test was performed for each pair of comparison between the control and the microbes-treated root tips. Significantly regulated molecular features profiled with ESI− mode that exhibit more than 5-fold of intensity change and with p < 0.05 are shown in the Venn diagram.
Supplementary Figure 3. The scatter plot showing distribution of the significantly regulated metabolites (p < 0.05) across the span of retention time of metabolite profiling in root tips after pre-symbiotic interaction with microbes of different species that are captured in LC-MS (ESI−). armi, Armillaria luteobubalina; piso, Pisolithus microcarpus; suil, Suillus granulatus; phyt, Phytophthora cinnamomi.
Supplementary Figure 4. Volcano plot showing the varied molecular features in E. grandis root tips after pre-symbiosis with P. microcarpus (n = 24) for 24 h in comparison to control root tips (n = 4). The metabolite profiles are derived from LC-MS (ESI− mode). The y-axis and x-axis correspond to the mean fold change of Pisolithus-interacting root tips in comparison to control, and the significance value in terms of p-value (adjusted by Bonferroni-method). Red = p < 0.05; Orange = Fold Change > 2; Green = p < 0.05 and Intensity fold change >2.
Supplementary Figure 5. PCA score plot showing the multivariate-variation among Pisolithus-interacting root tips samples in terms of metabolite profiles derived from the ESI− mode, LC-MS. The color of the symbols represents the geographical origin (geo. origin) of the Pisolithus strains, while the shape of the symbols represents the colonization rates (col. rate). Ctrl, un-infected control roots; R, Royal National Park; SI, Sussex Inlet; Wil, Wilberforce.
Supplementary Figure 6. PLS-DA score plot showing the first two components of the supervised classification model that separates metabolite profiles of Pisolithus-interacting root tips samples (LC-MS ESI− mode) in terms of colonization rate. The color of the symbols represents the geographical origin (geo. origin) of the Pisolithus isolates, while the shape of the symbols represents the colonization rates (col. rate). Ctrl, un-infected control roots; R, Royal National Park; SI, Sussex Inlet; Wil, Wilberforce.
Supplementary Figure 7. (Left) Scatter plot showing the abundance of 13C-labeled compounds in Pisolithus-treated root tips in labeled setup in relation to unlabeled setup, across the retention time span of LC-MS ESI− mode metabolite profiling. The color of the points showing the absolute intensity of the compound peak detected by the LC-MS. An unlabeled or mis-detected labeled metabolite would have a log (relative abundance) ≤ 0. (right) The violin plot demonstrates the distribution of 13C-labeled compounds with different relative abundance.
Supplementary Figure 8. Gene ontology enrichment results for highly-induced genes (log2(fold change) >5) in E. grandis roots in the pre-symbiotic interaction. Bar plot represent the number of genes involved in a gene-set of the enriched gene ontology term. The darkness of the color of the bar represent the significance (in terms of p-values) of the enriched gene-sets.
Supplementary Figure 9. Pathway graph to visualize the genes associated with the terpenoid backbone biosynthesis pathway and their expressions in E. grandis roots during direct physical interaction (coloration on left-hand side of each enzyme box) and pre-symbiotic interaction (coloration on the right-hand side of each enzyme box) with Pisolithus microcarpus in comparison to uninfected control roots. The Arabidopsis thaliana homologs of the E. grandis genes and their expression were mapped onto the pathway. The color of the boxes represents the log2(fold change) value of the gene.
Supplementary Figure 10. Pathway graph to visualize the genes associated with the fatty acid biosynthesis pathway and their expressions in E. grandis roots during direct physical interaction (coloration on left-hand side of each enzyme box) and pre-symbiotic interaction (coloration on the right-hand side of each enzyme box) with Pisolithus microcarpus in comparison to uninfected control roots. The Arabidopsis thaliana homologs of the E. grandis genes and their expression were mapped onto the pathway. The color of the boxes represents the log2(fold change) value of the gene.
Supplementary Figure 11. Pathway graph to visualize the genes associated with the diterpenoid biosynthesis pathway and their expressions in E. grandis roots during direct physical interaction (coloration on left-hand side of each enzyme box) and pre-symbiotic interaction (coloration on the right-hand side of each enzyme box) with Pisolithus microcarpus in comparison to uninfected control roots. The Arabidopsis thaliana homologs of the E. grandis genes and their expression were mapped onto the pathway. The color of the boxes represents the log2(fold change) value of the gene.
Supplementary Figure 12. Pathway graph to visualize the genes associated with the flavonoid biosynthesis pathway and their expressions in E. grandis roots during direct physical interaction (coloration on left-hand side of each enzyme box) and pre-symbiotic interaction (coloration on the right-hand side of each enzyme box) with Pisolithus microcarpus in comparison to uninfected control roots. The Arabidopsis thaliana homologs of the E. grandis genes and their expression were mapped onto the pathway. The color of the boxes represents the log2(fold change) value of the gene.
Supplementary Table 1. Raw Metabolite Data Matrix Used for Analyses. Data matrix of the normalized metabolite responses of samples used in this study and the details of the detected molecular features (neutral mass, retention time and composite spectra). The metabolite responses are normalized by the internal standard and sample weight.
Supplementary Table 2. Meta data for samples used for analyses.
Supplementary Table 3. Full list of metabolites specific to microbial lifestyles. Details of detected molecular features (neutral mass and retention time) and their response levels in microbe-associated root tips in comparison to untreated controls. FC, fold change; pval, p-value calculated with t-test; n.d., not detected; armi, Armillaria luteobubalina; piso, Pisolithus microcarpus; suil, Suillus granulatus; phyt, Phytophthora cinnamomi.
Supplementary Table 4. Full list of differentially expressed genes in E. grandis. Fold change (FC) of the differentially expressed genes and their details, including the gene name, transcript name, gene ontology (GO), and their homologs in Arabidopsis thaliana.
Supplementary Table 5. GO analysis of differentially expressed genes in E. grandis. GO enrichment result including the GO ID, description of the GO, condition, p-value (p.Val), false discovery rate (FDR), and the counts and names of genes involved in the GO set.
References
Akiyama, K., Matsuzaki, K.-I., and Hayashi, H. (2005). Plant sesquiterpenes induce hyphal branching in arbuscular mycorrhizal fungi. Nature 435, 824–827. doi: 10.1038/nature03608
Badri, D. V., and Vivanco, J. M. (2009). Regulation and function of root exudates. Plant Cell Environ. 32, 666–681. doi: 10.1111/j.1365-3040.2009.01926.x
Baetz, U., and Martinoia, E. (2014). Root exudates: the hidden part of plant defense. Trends Plant Sci. 19, 90–98. doi: 10.1016/j.tplants.2013.11.006
Baudoin, E., Benizri, E., and Guckert, A. (2003). Impact of artificial root exudates on the bacterial community structure in bulk soil and maize rhizosphere. Soil Biol. Biochem. 35, 1183–1192. doi: 10.1016/S0038-0717(03)00179-2
Beguiristain, T., and Lapeyrie, F. (1997). Host plant stimulates hypaphorine accumulation in Pisolithus tinctorius hyphae during ectomycorrhizal infection while excreted fungal hypaphorine controls root hair development. New Phytol. 136, 525–532. doi: 10.1046/j.1469-8137.1997.00753.x
Broeckling, C. D., Broz, A. K., Bergelson, J., Manter, D. K., and Vivanco, J. M. (2008). Root exudates regulate soil fungal community composition and diversity. Appl. Environ. Microbiol. 74, 738–744. doi: 10.1128/AEM.02188-07
Burgess, T., Dell, B., and Malajczuk, N. (1994). Variation in mycorrhizal development and growth stimulation by 20 Pisolithus isolates inoculated on to Eucalyptus grandis W. Hill ex Maiden. New Phytol. 127, 731–739.
Chapela, I. H., Osher, L. J., Horton, T. R., and Henn, M. R. (2001). Ectomycorrhizal fungi introduced with exotic pine plantations induce soil carbon depletion. Soil Biol. Biochem.33, 1733–1740. doi: 10.1016/S0038-0717(01)00098-0
Cho, H.-W., Kim, S. B., Jeong, M. K., Park, Y., Gletsu, N., Ziegler, T. R., et al. (2008). Discovery of metabolite features for the modelling and analysis of high-resolution NMR spectra. Int. J. Data Min. Bioinform. 2, 176–192. doi: 10.1504/IJDMB.2008.019097
Chong, J., Xia, J., and Stegle, O. (2018). MetaboAnalystR: an R package for flexible and reproducible analysis of metabolomics data. Bioinformatics 34, 4313–4314. doi: 10.1093/bioinformatics/bty528
Davis, V. W., Schiller, D. E., Eurich, D., and Sawyer, M. B. (2012). Urinary metabolomic signature of esophageal cancer and Barrett's esophagus. World J. Surg. Oncol. 10:271. doi: 10.1186/1477-7819-10-271
Deslippe, J. R., Hartmann, M., Grayston, S. J., Simard, S. W., and Mohn, W. W. (2016). Stable isotope probing implicates a species of Cortinarius in carbon transfer through ectomycorrhizal fungal mycelial networks in Arctic tundra. New Phytol. 210, 383–390. doi: 10.1111/nph.13797
Ditengou, F. A., and Lapeyrie, F. (2000). Hypaphorine from the ectomycorrhizal fungus Pisolithus tinctorius counteracts activities of indole-3-acetic acid and ethylene but not synthetic auxins in eucalypt seedlings. MPMI 13, 151–158. doi: 10.1094/MPMI.2000.13.2.151
Dunn, W. B., Erban, A., Weber, R. J. M., Creek, D. J., Brown, M., Breitling, R., et al. (2013). Mass appeal: metabolite identification in mass spectrometry-focused untargeted metabolomics. Metabolomics 9, 44–66. doi: 10.1007/s11306-012-0434-4
Facelli, E., McKay, S. F., Facelli, J. M., and Scott, E. S. (2018). A soil-borne generalist pathogen regulates complex plant interactions. Plant Soil 433, 101–109. doi: 10.1007/s11104-018-3828-x
Felix, G., Duran, J. D., Volko, S., and Boller, T. (1999). Plants have a sensitive perception system for the most conserved domain of bacterial flagellin. Plant J. 18, 265–276. doi: 10.1046/j.1365-313X.1999.00265.x
Felten, J., Legué, V., and Ditengou, F. A. (2010). Lateral root stimulation in the early interaction between Arabidopsis thaliana and the ectomycorrhizal fungus Laccaria bicolor. Plant Signal Behav. 5, 864–867. doi: 10.4161/psb.5.7.11896
Fries, N., Serck-Hanssen, K., Dimberg, L. H., and Theander, O. (1987). Abietic acid, and activator of basidiospore germination in ectomycorrhizal species of the genus Suillus (Boletaceae). Exp. Mycol. 11, 360–363. doi: 10.1016/0147-5975(87)90024-7
Genre, A., Chabaud, M., Balzergue, C., Puech-Pagès, V., Novero, M., Rey, T., et al. (2013). Short-chain chitin oligomers from arbuscular mycorrhizal fungi trigger nuclear Ca 2+ spiking in Medicago truncatula roots and their production is enhanced by strigolactone. New Phytol. 198, 190–202. doi: 10.1111/nph.12146
Gil de la Fuente, A., Godzien, J., Fernández López, M., Rupérez, F. J., Barbas, C., and Otero, A. (2018). Knowledge-based metabolite annotation tool: CEU Mass Mediator. J. Pharm. Biomed. Anal. 154, 138–149. doi: 10.1016/j.jpba.2018.02.046
Giovannetti, M., Mari, A., Novero, M., and Bonfante, P. (2015). Early Lotus japonicus root transcriptomic responses to symbiotic and pathogenic fungal exudates. Front. Plant Sci. 6:480. doi: 10.3389/fpls.2015.00480
Guo, J., McCulley, R. L., and McNear, D. H. J. (2015). Tall fescue cultivar and fungal endophyte combinations influence plant growth and root exudate composition. Front. Plant Sci. 6:183. doi: 10.3389/fpls.2015.00183
Hacquard, S., Spaepen, S., Garrido-Oter, R., and Schulze-Lefert, P. (2017). Interplay between innate immunity and the plant microbiota. Ann. Rev. Phytopathol. 55, 565–589. doi: 10.1146/annurev-phyto-080516-035623
Haichar, F., el, Z., Heulin, T., Guyonnet, J. P., and Achouak, W. (2016). Stable isotope probing of carbon flow in the plant holobiont. Curr. Opin. Biotechnol. 41, 9–13. doi: 10.1016/j.copbio.2016.02.023
Hartmann, A., Rothballer, M., and Schmid, M. (2008). Lorenz Hiltner, a pioneer in rhizosphere microbial ecology and soil bacteriology research. Plant Soil 312, 7–14. doi: 10.1007/s11104-007-9514-z
Hartmann, A., Schmid, M., Tuinen, D., and van Berg, G. (2009). Plant-driven selection of microbes. Plant Soil 321, 235–257. doi: 10.1007/s11104-008-9814-y
Huang, X., Chen, Y.-J., Cho, K., Nikolskiy, I., Crawford, P. A., and Patti, G. J. (2014). X13CMS: global tracking of isotopic labels in untargeted metabolomics. Anal. Chem. 86, 1632–1639. doi: 10.1021/ac403384n
Kamilova, F., Kravchenko, L. V., Shaposhnikov, A. I., Makarova, N., and Lugtenberg, B. (2006). Effects of the Tomato Pathogen Fusarium oxysporum f. sp. radicis-lycopersici and of the Biocontrol Bacterium Pseudomonas fluorescens WCS365 on the Composition of Organic Acids and Sugars in Tomato Root Exudate. MPMI 19, 1121–1126. doi: 10.1094/MPMI-19-1121
Kanehisa, M., Goto, S., Hattori, M., Aoki-Kinoshita, K. F., Itoh, M., Kawashima, S., et al. (2006). From genomics to chemical genomics: new developments in KEGG. Nucleic Acids Res. 34, D354–D357. doi: 10.1093/nar/gkj102
Kelly, S., Mun, T., Stougaard, J., Ben, C., and Andersen, S. U. (2018). Distinct Lotus japonicus transcriptomic responses to a spectrum of bacteria ranging from symbiotic to pathogenic. Front. Plant Sci. 9:1218. doi: 10.3389/fpls.2018.01218
Kile, G. A. (2000). “Woody root rots of eucalypts,” in Diseases and Pathogens of Eucalypts, eds P. J. Kean, G. A. Kile, F. D. Podger, and B. N. Brown (Collingwood, VIC: CSIRO Publishing), 293–306.
Kobori, H., Sekiya, A., Suzuki, T., Choi, J.-H., Hirai, H., and Kawagishi, H. (2015). Bioactive sesquiterpene aryl esters from the culture broth of Armillaria sp. J. Nat. Prod. 78, 163–167. doi: 10.1021/np500322t
Lagrange, H., Jay-Allgmand, C., and Lapeyrie, F. (2001). Rutin, the phenolglycoside from eucalyptus root exudates, stimulates Pisolithus hyphal growth at picomolar concentrations. New Phytol. 149, 349–355. doi: 10.1046/j.1469-8137.2001.00027.x
Lahrmann, U., Ding, Y., Banhara, A., Rath, M., Hajirezaei, M. R., Döhlemann, S., et al. (2013). Host-related metabolic cues affect colonization strategies of a root endophyte. Proc. Natl. Acad. Sci. U.S.A. 110, 13965–13970. doi: 10.1073/pnas.1301653110
López-Berges, M. S., Rispail, N., Prados-Rosales, R. C., and Pietro, A. D. (2010). A nitrogen response pathway regulates virulence functions in Fusarium oxysporum via the protein kinase TOR and the bZIP Protein MeaB. Plant Cell 22, 2459–2475. doi: 10.1105/tpc.110.075937
Love, M. I., Huber, W., and Anders, S. (2014). Moderated estimation of fold change and dispersion for RNA-seq data with DESeq2. Genome Biol. 15:550. doi: 10.1186/s13059-014-0550-8
Luo, W., Pant, G., Bhavnasi, Y. K., Blanchard, S. G., and Brouwer, C. (2017). Pathview Web: user friendly pathway visualization and data integration. Nucleic Acids Res. 45, W501–W508. doi: 10.1093/nar/gkx372
Maillet, F., Poinsot, V., André, O., Puech-Pagès, V., Haouy, A., Gueunier, M., et al. (2011). Fungal lipochitooligosaccharide symbiotic signals in arbuscular mycorrhiza. Nature 469, 58–63. doi: 10.1038/nature09622
Martin, F., Duplessis, S., Ditengou, F., Lagrange, H., Voiblet, C., and Lapeyrie, F. (2001). Developmental cross talking in the ectomycorrhizal symbiosis: signals and communication genes. New Phytol. 151, 145–154. doi: 10.1046/j.1469-8137.2001.00169.x
Merico, D., Isserlin, R., Stueker, O., Emili, A., and Bader, G. D. (2010). Enrichment Map: a network-based method for gene-set enrichment visualization and interpretation. PLoS ONE 5:e13984. doi: 10.1371/journal.pone.0013984
Miyata, K., Kozaki, T., Kouzai, Y., Ozawa, K., Ishii, K., Asamizu, E., et al. (2014). The bifunctional plant receptor, OsCERK1, regulates both chitin-triggered immunity and arbuscular mycorrhizal symbiosis in rice. Plant Cell Physiol. 55, 1864–1872. doi: 10.1093/pcp/pcu129
Myburg, A. A., Grattapaglia, D., Tuskan, G. A., Hellsten, U., Hayes, R. D., Grimwood, et al. (2014). The genome of Eucalyptus grandis. Nature 510, 356–362. doi: 10.1038/nature13308
Okazaki, S., Kaneko, T., Sato, S., and Saeki, K. (2013). Hijacking of leguminous nodulation signaling by the rhizobial type III secretion system. Proc. Natl. Acad. Sci. U.S.A. 110, 17131–17136. doi: 10.1073/pnas.1302360110
Pang, Q., Zhang, T., Wang, Y., Kong, W., Guan, Q., Yan, X., et al. (2018). Metabolomics of early stage plant cell–microbe interaction using stable isotope labeling. Front Plant Sci 9:760. doi: 10.3389/fpls.2018.00760
Plett, J. M., Kohler, A., Khachane, A., Keniry, K., Plett, K. L., Martin, F., et al. (2015). The effect of elevated carbon dioxide on the interaction between Eucalyptus grandis and diverse isolates of Pisolithus sp. is associated with a complex shift in the root transcriptome. New Phytol. 206, 1423–1436. doi: 10.1111/nph.13103
Radutoiu, S., Madsen, L. H., Madsen, E. B., Felle, H. H., Umehara, Y., Grønlund, M., et al. (2003). Plant recognition of symbiotic bacteria requires two LysM receptor-like kinases. Nature 425, 585–592. doi: 10.1038/nature02039
Raudaskoski, M., and Kothe, E. (2014). Novel findings on the role of signal exchange in arbuscular and ectomycorrhizal symbioses. Mycorrhiza 25, 243–252. doi: 10.1007/s00572-014-0607-2
Reimand, J., Kull, M., Peterson, H., Hansen, J., and Vilo, J. (2007). g:Profiler—a web-based toolset for functional profiling of gene lists from large-scale experiments. Nucleic Acids Res. 35, W193–W200. doi: 10.1093/nar/gkm226
Rey, T., Nars, A., Bonhomme, M., Bottin, A., Huguet, S., Balzergue, S., et al. (2013). NFP, a LysM protein controlling Nod factor perception, also intervenes in Medicago truncatula resistance to pathogens. New Phytol. 198, 875–886. doi: 10.1111/nph.12198
Schymanski, E. L., Jeon, J., Gulde, R., Fenner, K., Ruff, M., Singer, H. P., et al. (2014). Identifying small molecules via high resolution mass spectrometry: communicating confidence. Environ. Sci. Technol. 48, 2097–2098. doi: 10.1021/es5002105
Sena, K., Crocker, E., Vincelli, P., and Barton, C. (2018). Phytophthora cinnamomi as a driver of forest change: Implications for conservation and management. Forest Ecol. Manage. 409, 799–807. doi: 10.1016/j.foreco.2017.12.022
Shannon, P., Markiel, A., Ozier, O., Baliga, N. S., Wang, J. T., Ramage, D., et al. (2003). Cytoscape: a software environment for integrated models of biomolecular interaction networks. Genome Res. 13, 2498–2504. doi: 10.1101/gr.1239303
Shearer, B. L., and Smith, I. W. (2000). “Disease of eucalypts caused by soilborne species of Phytophthora and Pythium,” in Diseases and Pathogens of Eucalypts, eds P. J. Kean, G. A. Kile, F. D. Podger, and B. N. Brown (Collingwood, VIC: CSIRO Publishing), 259–291.
Simard, S. W., Perry, D. A., Jones, M. D., Myrold, D. D., Durall, D. M., and Molina, R. (1997). Net transfer of carbon between ectomycorrhizal tree species in the field. Nature 388, 579–582. doi: 10.1038/41557
Smith, C. A., Maille, G. O., Want, E. J., Qin, C., Trauger, S. A., Brandon, T. R., et al. (2005). METLIN: a metabolite mass spectral database. Therap. Drug Monitor.27, 747–751. doi: 10.1097/01.ftd.0000179845.53213.39
Smith, C. A., Want, E. J., O'Maille, G., Abagyan, R., and Siuzdak, G. (2006). XCMS: processing mass spectrometry data for metabolite profiling using nonlinear peak alignment, matching, and identification. Anal Chem. 78, 779–787. doi: 10.1021/ac051437y
St-Arnaud, M., Hamel, C., Vimard, B., Caron, M., and Fortin, J. A. (1996). Enhanced hyphal growth and spore production of the arbuscular mycorrhizal fungus Glomus intraradices in an in vitro system in the absence of host roots. Mycol. Res.100, 328–332. doi: 10.1016/S0953-7562(96)80164-X
Steinkellner, S., Lendzemo, V., Langer, I., Schweiger, P., Khaosaad, T., Toussaint, J.-P., et al. (2007). Flavonoids and strigolactones in root exudates as signals in symbiotic and pathogenic plant-fungus interactions. Molecules 12, 1290–1306. doi: 10.3390/12071290
Stracke, S., Kistner, C., Yoshida, S., Mulder, L., Sato, S., Kaneko, T., et al. (2002). A plant receptor-like kinase required for both bacterial and fungal symbiosis. Nature 417, 959–962. doi: 10.1038/nature00841
Stuttmann, J., Hubberten, H.-M., Rietz, S., Kaur, J., Muskett, P., Guerois, R., et al. (2011). Perturbation of arabidopsis amino acid metabolism causes incompatibility with the adapted biotrophic pathogen Hyaloperonospora arabidopsidis. Plant Cell 23, 2788–2803. doi: 10.1105/tpc.111.087684
Tellström, V., Usadel, B., Thimm, O., Stitt, M., Küster, H., and Niehaus, K. (2007). The lipopolysaccharide of Sinorhizobium meliloti suppresses defense-associated gene expression in cell cultures of the host plant Medicago truncatula. Plant Physiol. 143, 825–837. doi: 10.1104/pp.106.090985
Thomma, B. P. H. J., Nürnberger, T., and Joosten, M. H. A. J. (2011). Of PAMPs and Effectors: the blurred PTI-ETI dichotomy. Plant Cell 23, 4–15. doi: 10.1105/tpc.110.082602
van Brussel, A. A. N., Bakhuizen, R., van Spronsen, P. C., Spaink, H. P., Tak, T., Lugtenberg, B. J. J., et al. (1992). Induction of pre-infection thread structures in the leguminous host plant by mitogenic lipo-oligosaccharides of rhizobium. Science 257, 70–72.
Weljie, A. M., Bondareva, A., Zang, P., and Jirik, F. R. (2011). 1H NMR metabolomics identification of markers of hypoxia-induced metabolic shifts in a breast cancer model system. J. Biomol. NMR 49, 185–193. doi: 10.1007/s10858-011-9486-4
Wishart, D. S., Feunang, Y. D., Marcu, A., Guo, A. C., Liang, K., Vázquez-Fresno, R., et al. (2018). HMDB 4.0: the human metabolome database for 2018. Nucleic Acids Res. 46, D608–D617. doi: 10.1093/nar/gkx1089
Zhang, X., Dong, W., Sun, J., Feng, F., Deng, Y., He, Z., et al. (2015). The receptor kinase CERK1 has dual functions in symbiosis and immunity signalling. Plant J. 81, 258–267. doi: 10.1111/tpj.12723
Zipfel, C., Robatzek, S., Navarro, L., Oakeley, E. J., Jones, J. D. G., Felix, G., et al. (2004). Bacterial disease resistance in Arabidopsis through flagellin perception. Nature 428, 764–767. doi: 10.1038/nature02485
Keywords: plant-microbe interaction, chemical signaling, metabolomics, isotope, transcriptomics
Citation: Wong JWH, Lutz A, Natera S, Wang M, Ng V, Grigoriev I, Martin F, Roessner U, Anderson IC and Plett JM (2019) The Influence of Contrasting Microbial Lifestyles on the Pre-symbiotic Metabolite Responses of Eucalyptus grandis Roots. Front. Ecol. Evol. 7:10. doi: 10.3389/fevo.2019.00010
Received: 30 October 2018; Accepted: 14 January 2019;
Published: 01 February 2019.
Edited by:
Margot Schulz, Universität Bonn, GermanyReviewed by:
Ulrike Mathesius, Australian National University, AustraliaDavid Overy, Agriculture and Agri-Food Canada (AAFC), Canada
Copyright © 2019 Wong, Lutz, Natera, Wang, Ng, Grigoriev, Martin, Roessner, Anderson and Plett. This is an open-access article distributed under the terms of the Creative Commons Attribution License (CC BY). The use, distribution or reproduction in other forums is permitted, provided the original author(s) and the copyright owner(s) are credited and that the original publication in this journal is cited, in accordance with accepted academic practice. No use, distribution or reproduction is permitted which does not comply with these terms.
*Correspondence: Jonathan M. Plett, ai5wbGV0dEB3ZXN0ZXJuc3lkbmV5LmVkdS5hdQ==