Now You See Me, Now You Don't: Environmental Conditions, Signaler Behavior, and Receiver Response Thresholds Interact to Determine the Efficacy of a Movement-Based Animal Signal
- 1Animal Behaviour Group, Department of Ecology, Environment and Evolution, La Trobe University, Melbourne, VIC, Australia
- 2Faculty of Information Technology, Monash University, Caulfield East, VIC, Australia
Knowledge of the environment in which animals operate and the sensory processing demands that mediate behavior in an ecological context are crucial for understanding animal communication systems. Understanding how environmental factors constrain communication strategies requires quantifying both the signal and noise in detail, as has been demonstrated in studies of acoustic and color signals for some time. However, comparable investigations of movement-based animal signals and the signaling environment is limited. There is now growing evidence that the dynamics of motion noise, in the form of wind-blown plant-movement, are a major sensory constraint for movement-based signals. However, progress has been limited as traditional techniques for understanding the ecological constraints on movement-based signals have proven insufficient. Our study utilized an innovative approach to quantify motion ecology by simulating a signaling animal in a natural habitat using highly realistic 3D animations, which afforded us unprecedented control over the signal and the circumstances in which signaling takes place. Using the Jacky dragon Amphibolurus muricatus as a model species, we quantified the efficacy of signal in noise under different combinations of wind and light environments, and quantified the potential benefit of signaling faster, or in different orientations relative to the background. We also examined signal performance as a function of varying receiver operating characteristics. Our results suggest that prevailing environmental conditions at the time of signaling do indeed affect the efficacy of movement-based signals, with wind and light levels interacting to influence efficacy. We found that faster speeds and selecting particular orientations can be beneficial, but signal efficacy comes down to the interaction between wind conditions, the light environment, signaler orientation, and thresholds for receiver responses.
Introduction
The remarkable diversity of animal communication systems is a function of the complex interaction between social context, receiver sensory systems, and the signaling environment (Ryan et al., 1990; Endler, 1992; Ord et al., 2002). The sensory drive model of signal evolution suggests that the structure of the most effective signal is strongly influenced by habitat characteristics, promoting signal diversity as a consequence of niche selection by species and populations (Endler, 1992; Leal and Fleishman, 2003). For instance, the song structure of great tits (Parus major) varies between forest and open woodlands (Hunter and Krebs, 1979), allopatric populations of cricket frogs (Acris crepitans) produce different calling structures between pine wood and open grasslands (Ryan et al., 1990), and similarly, the divergence in vibrational signals produced by insects varies due to host plant selection (Cocroft and Rodriguez, 2005). The sensory drive model also highlights the role of receiver sensory systems. An effective signal must stimulate the sensory system of an intended receiver after it travels through often ecologically-complex environments (Endler, 1992). During transmission, signals inevitably degrade due to attenuation, diffraction, pattern loss, and other distorting effects of the transmission properties of the environment (Morton, 1975; Lythgoe, 1979; Endler, 1990, 1992). Therefore, it makes sense that natural selection will favor signaling behavior that maximizes signaling efficacy (Endler, 1992, 1993a; Fleishman, 1992), such that the specific transmission properties of different habitats determine the optimal structure of a signal (Wiley and Richards, 1983).
Another important constraint on effective communication is irrelevant sensory stimulations in the same modality (Fleishman, 1986; Brumm and Slabbekoorn, 2005; Witte et al., 2005). In order for a signal to be effective, intended receivers need to be able to distinguish it from competing stimulations from within the habitat that might interfere with reliable detection and processing by receivers (Endler, 1992; Fleishman, 1992). These irrelevant stimulations might have biotic or abiotic origins and can be broadly classed as environmental noise. The masking effects of environmental noise have been demonstrated widely in many signaling modalities including acoustic (Lengagne and Slater, 2002), seismic (Narins, 1990), chemosensory (Atema, 1995), electrical (Gabbiani et al., 1996), and visual signals (Fleishman and Persons, 2001; Peters et al., 2007). Variation in the ambient noise spectra across habitats can lead to divergence in signal structure (Slabbekoorn and Smith, 2002), while spectral characteristics of the relative light environments are suggested to contribute to divergence in reflectance patterns of different populations (Mccoy et al., 1997). However, the environmental conditions in which animals communicate are not constant, and the structure of a given habitat will affect moment-to-moment variation in signaling conditions (Fleishman, 1986; Peters, 2008). Therefore, to convey information effectively, animals may adjust signal structure or behavior, such as the timing of signaling in the presence of sympatric congeners (Greenfield, 1988), increase amplitude or intensity of the signal at times of increased environmental noise (Cynx et al., 1998; Ord et al., 2007) or change visual angle to enhance detectability of visual signals (Klomp et al., 2017). Therefore, understanding habitat structure and environmental conditions at the time of signaling is of fundamental importance to explain signal design and to identify the neural processing strategies that are used to detect these signals.
There is now growing interest in the role of habitat characteristics for visual signals, as visual signals are one of the most salient features in the animal kingdom and are taxonomically wide-spread, from mammals (Rundus et al., 2007) and reptiles (Ramos and Peters, 2016) to invertebrates (Elias et al., 2006). The detectability of visual signals is partly determined by the environment in which the signal is emitted (Endler, 1992) and the efficacy of the signal is influenced by different environmental conditions, such as light intensity or visual noise (e.g., windblown vegetation). For instance, some bird species have been shown to adjust their body orientation or selectively display in sunlight to increase iridescent coloration of their feathers (Bortolotti et al., 2011; Sicsu et al., 2013) or choose specific lekking positions to make best use of the light available in the environment (Endler and Thery, 1996). More recently, gliding lizards (Draco sumatranus) were shown to orientate themselves relative to the position of the sun to enhance their social displays to conspecifics (Klomp et al., 2017). While the effect of light environments is well-established in respect to color-based visual signals, whether and how it is relevant to motion based visual signals has been under-appreciated. The relevance of the light environment includes variation in light intensity (Endler, 1993b) and dappled light effects, which are a consequence of light passing through foliage. Foliage shadows cast onto substrates, swaying naturally with the wind, are an additional source of motion noise. Furthermore, the distracting effect of this noise might vary dynamically as light intensity varies. Variation in intensity might occur over a relatively short time period due to the influence of cloud cover, or over a long time period as a consequence of the position of the sun in the sky. The consequence of these variations is to alter the luminance profile of the scene, including the magnitude of changes from low to high contrast. This is relevant because the perception of motion by biological vision systems requires luminance information from the scene (Cropper and Wuerger, 2005), and plays an important role in grouping individual perceptual objects to facilitate the segmenting of scenes into distinct figures (Leonards and Singer, 1998). Therefore, we speculate that the luminance profile of the scene will impact the figure-ground segmentation mechanisms involved in detecting relevant movement-based visual signals of animals.
The main source of noise for motion signals is the physical movements of wind-blown plants (Fleishman, 1986; Ord et al., 2007; Peters et al., 2007). Fleishman (1986) was the first to experimentally address the potential masking effect of plant motion, showing that the responses of lizards to the salient movement of territorial displays was hindered by plant motion in the background. Using Fourier analysis to characterize the movement of single blades of grass, he subsequently showed that plant motion varied as a function of species and the prevailing wind conditions (Fleishman, 1988a). Several studies have extended Fleishman's early work by analyzing the motion of whole plants (Peters and Evans, 2003) and microhabitats (Peters et al., 2008; Fleishman and Pallus, 2010) using computational motion analysis.
However, movement-based animal signals and the plant environments they inhabit are particularly difficult to quantify in detail. The seemingly simple movements of plants in fact represents one of the most complex features of the environment. As the geometry of plants varies from one to another, the physical response to wind will likely be different even within the same species, while the topography of individual habitats will affect the plants' relative exposure to wind (Hannah et al., 1995). Different microhabitats are thus likely to reflect distinct “image motion environments” (Peters, 2013). Therefore, the first question guiding the present study was: how does variation in environmental conditions constrain the efficacy of a movement-based signal? Although we only have limited understanding of the sensory constraints plant-motion noise have on movement-based signals, the importance of such constraints is evident by watching the animals themselves. Multiple species of lizard have been shown to adjust their motion signaling strategies to compensate for the masking effect of plant motion (Ord et al., 2007; Peters et al., 2007; Ord and Stamps, 2008). For instance, the Australian Jacky dragon Amphibolurus muricatus prolongs introductory tail-flicking during their territorial displays in response to greater plant motion in the habitat (Peters et al., 2007), and male Anolis lizards were shown to include rapid body movements to enhance signal intensity in noisy environments (Ord and Stamps, 2008). While increasing the duration and speed of the display are known to be strategies that lizards use, which we infer enhances signal efficacy, changes in signal orientation might also have an effect on movement-based signals, as they seemingly do for color-based signals (Klomp et al., 2017). The detection of visual signals is highly dependent on viewer orientation. Indeed studies of visual color signals indicated that the detection of a signal is influenced by both visual angle (Giurfa et al., 1996) and distance (de Ibarra et al., 2001). With this in mind, we suspect that orientation relative to surrounding plant motion in the environment might also be relevant for the efficacy of movement-based signals. Therefore, the second question we address herein is: how effective at improving signal efficacy are adjustments in signaling speed and changing signaling orientation?
In order to address our questions above, we need to be able to assess the efficacy of movement-based signals in a variety of contexts. However, simultaneous measurements of plant movements, microhabitat structure, environmental variables and animal signals are technically challenging, and obtaining sufficient variation in the parameters of interest is impractical in nature. Instead, we recently argued that these questions could be approached using simulated natural environments using 3D animation, and demonstrated the potential utility of sophisticated 3D animation models as a tool for exploring the evolutionary constraints on movement-based signals (Bian et al., 2018). While it is crucial that the simulations created are based on data from real environments (Chouinard-Thuly et al., 2017), the ability to faithfully simulate lighting, shadows, geometry, motion, and time-based transitions within animations provides us with powerful tools to generate realistic environmental changes. Our strategy for building the 3D simulations makes use of real data on the signaling dynamics of lizards, and the environments in which they are generated. The end results are sophisticated animations with all parameters under our direct control (see Bian et al., 2018 for details). The selective agents for assessing signal effectiveness in this study are not real lizards, but computational models of visual selective attention. We employed the saliency-based computational model described by Itti et al. (1998) that predicts areas of a scene that captures attention. Saliency analysis models selective attention to objects by scanning a saliency map computed from local feature contrasts, including motion (Harel et al., 2007; Pike, 2018). By focusing on local features, like contrast, color, and motion, such models are considered to be adopting a bottom-up approach. We utilized the same computational approach to assessing the relative efficacy of animal motion signals in the present study and we describe our result in terms of signal efficacy, but in so doing do not dismiss the complexity involved in visual attention of natural scenes. As pointed out by Tatler et al. (2011), image saliency can explain certain aspects of visual attention, but explanatory power is limited because of several key assumptions inherent to saliency analysis, such as relying on simple features and bottom-up selection, as well as ignoring characteristics of visual systems that result in non-uniform sampling of scenes.
Natural environments are full of stochastic events, but only a small proportion of movement is relevant to animal receivers. The sensory and brain properties of receivers operate to filter out much of the seemingly irrelevant sensory stimulation (Eckert and Zeil, 2001). Furthermore, selective attention to a specific event, such as movement-based animal signals, results from a complex interaction between the observer's sensory capability, the specific context and potential payoffs of responding or not responding, past learning ability, and other visual distractions that might occur at the time of visual searching (Chapman and Underwood, 1998; Land et al., 1999; Kuhn and Tatler, 2005). As such, the same signal, under the same environmental conditions, might not attract the attention of every potential receiver, or might not always attract the attention of the same receiver at different times. As suggested by signal detection theory, it is useful to consider the process of attentional capture as utilizing a threshold for response, which influences the probability of correctly detecting a signal (success), missing a signal that was present (miss), responding when a signal was not present (false alarm), or correctly rejecting sensory stimulation in the absence of a signal (see Wiley, 2006, 2013, 2015 for application of signal detection theory to animal communication). Consequently, in order to quantify the efficacy of a given signal in noise in the present study, we have incorporated into our analysis variation in receiver thresholds. We operationally define low threshold values to represent receivers that are relatively non-discriminating in the cues that might attract attention, while high threshold values to represent receivers that will only attend to specific, highly salient cues. By incorporating variation in receiver thresholds, we considered whether signals are robust to variation in the operating characteristics of receivers (Swets, 1973) as well as in the environmental or ecological context we specify.
The model system chosen to address our questions of interest was the Australian Jacky dragon (A. muricatus), which can be found in a variety of habitats including coastal heath, rocky outcrops and dry woodlands. Coastal heath environments mask the movement-based signals of these lizards to a greater extent compared with rock or woodland habitats (Ramos and Peters, 2017), and was selected to be the environmental setting for our animations. Created using real-world data, we used the animation environment to address our questions of interest. To consider how variation in environmental conditions constrains the efficacy of a movement-based signal, we generated 100 animations in which we systematically manipulated the wind (plant movements) and light conditions, while holding the structure of the signal and the position of the receiver constant. To determine whether behavioral strategies might be able to mitigate the masking effect of environmental conditions, we held environmental variables and receiver position constant and, in separate sets of simulations, adjusted the speed of movement and varied the orientation of the signaler. Based on previous studies, we predicted that increased wind conditions will reduce signal efficacy (Fleishman, 1986; Ord et al., 2007; Peters et al., 2007; Ord and Stamps, 2008). The effect of the light environment on movement-based signaling has not been investigated previously, but given its relevance for motion vision systems we anticipate it will have a mediating effect. We also anticipated benefits to increasing signaling speed (following Ord et al., 2007) and speculated that signal efficacy would also be affected by changing orientation of the signaler.
Methods
Computer animation is an innovative approach to understand the sensory constraints inherent to dynamic environments (Bian et al., 2018). The ability of animation to faithfully simulate lighting, shadows, color, geometry, motion, and time-based transitions has made it a valuable tool in medical imaging, engineering, geophysics, and plant biology (Shinya and Fournier, 1992; Stam, 1997; Sakaguchi and Ohya, 1999; Ota et al., 2004; Wong and Datta, 2004; Akagi and Kitajima, 2006; Zhang et al., 2007; Habel et al., 2009; Khorloo et al., 2011). Using data from habitat surveys and video of the Jacky dragon's (A. muricatus) motion signaling sequence, we reconstructed the animal and its microhabitat using realistic 3D models in Maya©2015 (see Bian et al., 2018). We created three sets of simulations to address our research questions: manipulations of wind and light conditions, changing the speed of the signal, and varying the orientation of the signaler. Rendered videos of these simulations were analyzed using saliency models of selective attention in order to predict motion signal efficacy under a variety of contexts.
Creating 3D Lizard Model and Microhabitat
We provide here a quick overview of the construction process for 3D animation; see Bian et al. (2018) for detailed descriptions. The territorial displays of Jacky dragons were filmed in the field using a dual camera system (see Ramos and Peters, 2017), and one display was selected as representative of the set. Using a free Matlab (Mathworks Inc) toolbox (Hedrick, 2008), we digitized the position of multiple body parts throughout the sequence and reconstructed the signaling motion in 3D (see Bian et al., 2016; Peters et al., 2016 for applications of this technique). To begin creating the 3D animation, we built the lizard model using a subdivision-modeling technique, which involves sculpturing and texturing the model using real morphological measurements and photographs of skin textures. After building the model, we added a virtual skeleton that allows precise control of movement of the whole model and its parts. The lizard's signaling behavior was animated using a key-frame technique, where the position data from a frame-by-frame analysis of field recordings of the display were used to set the coordinates for each part of the body at relevant frames throughout the sequence. We finished with a display sequence to match our model species that comprises four distinct components including introductory tail-flicking followed by limb-waving, push-ups and whole-body movements.
Jacky dragons inhabit woodlands and coastal heath of southeast Australia and are often seen perched on fallen timber (Cogger, 1978). The habitat we created contains relevant features rather than a specific habitat (Figure 1). Landscape topography and plant models were created using the same method as the lizard model and were integrated with an inbuilt Maya script that provides physically accurate and realistic plant movements (Akagi and Kitajima, 2006). With the ground landscape completed, lighting and shadows were added to the scene. A single directional light was added to simulate the sun and thereby create naturally overcasting shadows from rocks, logs, and plants. The position and scale of lights was controlled using transformation tools within Maya.
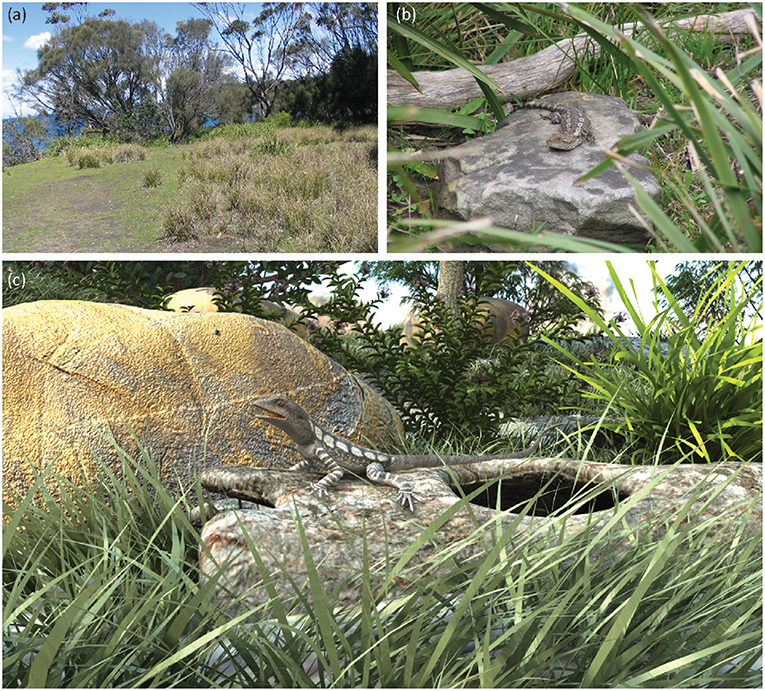
Figure 1. (a) Representative habitat of Jacky dragons Amphibolurus muricatus along the south-east coast of Australia, and (b) a basking adult Jacky dragon. (c) Rendered frame of the 3D animation environment showing the simulated habitat and lizard.
Changing Environmental Variables
Manipulations of Wind and Light
To test whether motion signal efficacy is influenced by prevailing environmental conditions, we varied the wind and light conditions systematically while keeping the signaling sequence constant. Our manipulation of light levels was not simply to adjust intensity values uniformly across the scene, but to alter the strength and range of dappled lighting surrounding the lizard (Figure 2A, top). This was done by adding a directional light integrated with a sequence of black and white moving foliage photographs as textures to create the effect of dappled light casting shadows onto surrounding objects. The outcome of these manipulations was to change the luminance profile of the scene, from relatively flat to strongly jagged luminance profiles (Figure 2A, bottom). For the remainder of the paper, we refer to differences in luminance between versions of our animations. It is important to note, however, that luminance is a function of light intensity, the reflective properties of the object and the angle of view.
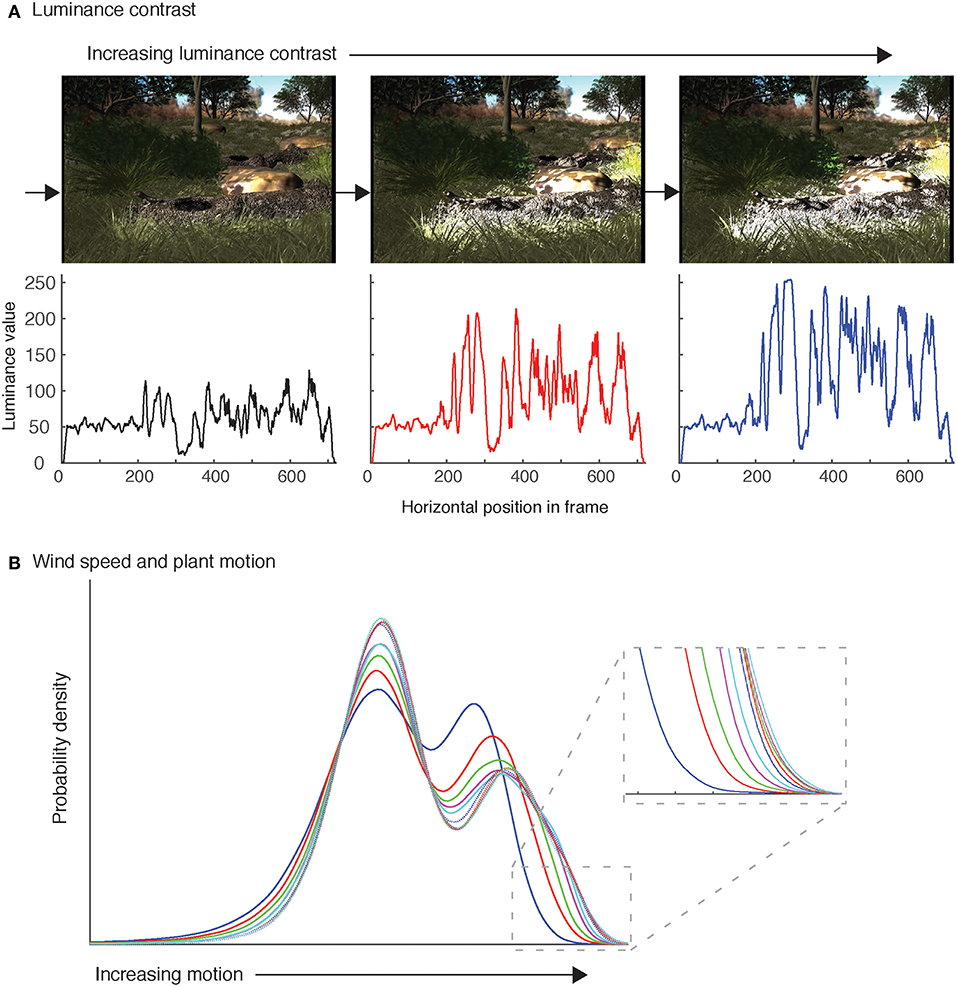
Figure 2. The animation environment enables control of light and wind-induced plant motion. (A) Representative frames for varying light conditions (levels 1, 4, and 7). Below each image is the horizontal luminance profile, corresponding to the position of the arrow in each frame. The luminance value is represented here by intensity values in the range [0–255] following grayscale conversion in Matlab. (B) Animation sequences featuring plant motion in the absence of lizard movements were analyzed using computational motion analysis (Peters et al., 2002). The resulting speed data for each frame was extracted and combined (ignoring time). We calculated kernel density estimates of these speeds using the ksdensity function in Matlab to generate a vector of relative probability of different image motion speeds (probability density). The probability density is plotted separately for all ten animation sequences (different line colors). Inset: Enlargement of the fast speed end of the distribution highlights the non-liner change across the set of animations.
In our manipulation of plant motion under wind, we systematically increased the extent of plant motion noise across the scene (Figure 2B). Plant models were integrated and controlled with an inbuilt script in Maya that guides plant movement based on physically accurate equations. We obtained the desired properties by manually adjusting the controllers for each plant, rendering animations of the scene and analyzing them using computational motion analysis techniques (see Peters et al., 2002). The end result was a unique set of 10 values for each plant that generated increasing levels of motion noise across the scene, albeit in a non-linear manner (Figure 2B). One hundred animation sequences were created from the factorial combination of 10 light levels and 10 wind conditions. An additional sequence was created featuring the displaying lizard in the absence of plant movement and featuring a flat luminance profile. This sequence provided a baseline against which the other sequences were compared, as explained below. All sequences were rendered with the lizard orientated sideways to the camera and exported as images (720 × 576 pixels) and composed into 8 second videos (200 frames at 25 frames/s).
Behavioral Strategies
Behavioral adjustments are well-documented in animals utilizing acoustic signals to enhance signal efficacy (reviewed in Brumm and Slabbekoorn, 2005). Animals are shown to increase the amplitude or intensity of their acoustic signals at times of increased environmental noise (Cynx et al., 1998; Brumm, 2004; Patricelli and Blickley, 2006). Similarly, animals using movement-based signals have been shown to increase signaling speed to overcome increased motion noise (e.g., Anolis lizards, Ord et al., 2007), and previous work on related species has shown that the detection of oscillating lures against a moving background is facilitated when the lure oscillates at higher frequencies (Fleishman, 1986). Interestingly, Peters et al. (2007) found that A. muricatus tailors its motion signal strategy not by increasing signal speed but the duration of the introductory tail flick under high levels of motion noise in the environment, and the angular speed of the tail flick did not differ between calm and windy conditions. However, if we could increase the signaling speed of the animal, would it help to facilitate signal efficacy in A. muricatus?
Increasing Signaling Speed
The first set of simulations indicated that moderate wind and scenes with a moderately jagged luminance profile affect signal efficacy. To explore whether increasing signaling speed would improve this outcome, we selected one sequence featuring the lizard orientated sideways to the camera under moderate wind and a moderately jagged luminance profile to represent “normal” signal speed. We then created two new animations in which we increased the signaling speed to both 1.5 and 2 times faster than that of the normal sequence. To achieve this, we compressed the total number of frames (n = 160) featuring lizard signaling movements to 120 frames (fast speed) and 80 frames (faster speed) respectively, while retaining the same sequence and amplitude of movements. Importantly, our manipulations of the timing of lizard movements has no effect on plant movements as all objects in the scene (lizard, plants etc.) are controlled independently. However, shorter signal durations mean the lizard movements will be viewed against only a small time window of plant movement relative to the normal sequence. To account for this, we created multiple replicates of each, shifting the starting position of the signal sequence by 10 frame increments (0.4 s in duration) within the animation to make sure that the signal overlaps with all background noise of the original sequence. This generated five and nine sequences for the fast and faster sequences, respectively (Figure 3). We also created separate baseline sequences for the fast and faster sequences, featuring no plant motion and a relatively flat luminance profile. All sequences were exported as images (720 × 576 pixels) and composed into 8 second videos (200 frames at 25 frames/s).
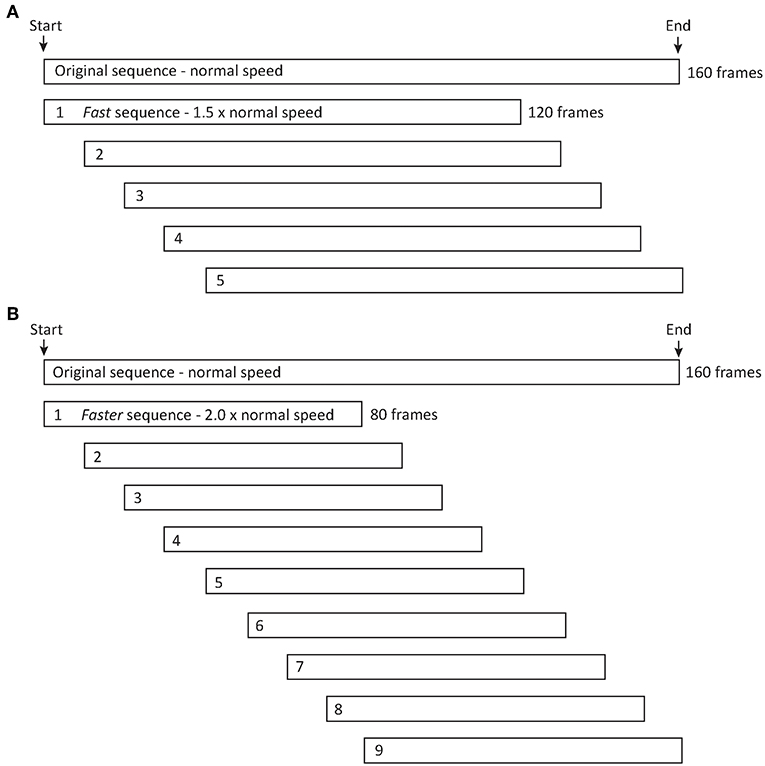
Figure 3. In order to investigate the potential benefit of signaling faster, we compressed the number of frames in the normal speed sequence to represent sequences (A) 1.5 × (fast) and (B) 2.0 × (faster) the normal speed. Importantly, the sequence and amplitude of movements is the same for each speed, and the process of changing the time-frame over which lizard displays occurs has no effect on other components in the animation, including plant movements. The fast and faster display sequences were completed in a shorter time frame than the normal sequence and therefore seen against different plant motion conditions. To account for this potential source of variation, we created multiple versions of each to ensure the new signal overlaps with all of the background noise of the normal sequence. This was achieved by shifting the starting point by increments of 10 frames (0.4 s), resulting in five and nine sequences for fast (A) and faster (B) lizard display speeds.
Changing Signaler Orientation
As the movement-based signals of Jacky dragons sweep 3D space, viewing angle is likely to impact which parts of the moving animal will be seen by the receiver. To explore whether this affects the efficacy of the signal, we created five sequences in which the orientation of the lizard relative to the background varied so as to be viewed from the front, side, angled left and right, and from behind (back; Figure 4a). The position of the camera remained the same as in all previous animations, and consequently the plant background was also unchanged. In this way we could compare the signal efficacy of different body orientations against the same motion background; the alternative of moving the camera around the lizard would have simultaneously changed the background as well (Figures 4b–d, see also Ramos and Peters, 2017). We created 10 animations for each body orientation featuring the factorial combination of three levels of wind and light, plus a baseline sequence with no plant motion. All sequences were exported as images (720 × 576) and composed into 8 s videos (200 frames at 25 frames/s).
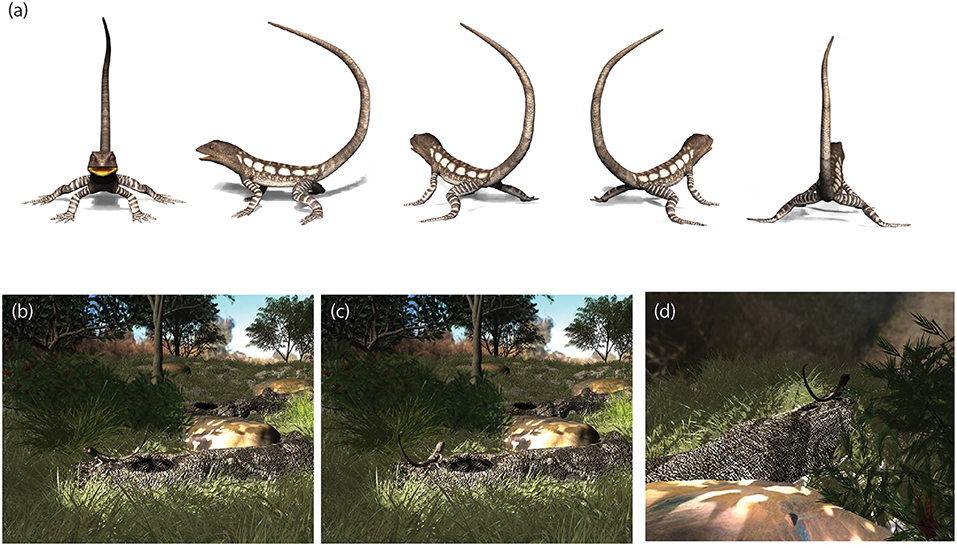
Figure 4. We manipulated the position of the lizard in the scene to examine whether signal effectiveness is mediated by signaler orientation. (a) Five different orientations were considered: front-on, side-on, angled left, angled right and from behind (back), respectively, from left to right. (b) We chose to change the orientation of the signaler rather than position of the viewer (camera position) as the latter includes concomitant changes in the background. For example, differences in signal effectiveness between the lizard in (b) and (c) under identical plant movements is attributable to the change in signaler orientation. However, the effect of changing orientation when comparing (b) and (d) is confounded by the very different plant movement backgrounds.
Sequence Analysis: Salience of Lizard Displays
Our analytical approach to determining the efficacy of lizard displays in noise is summarized in Figure 5 and was undertaken in Matlab (Mathworks Inc). Animation sequences were analyzed frame-by-frame (Figure 5A) to determine relative salience across the scene. We employed a saliency-driven computational model described by Itti et al. (1998) that involves scanning of local features such as contrast and motion to produce a saliency map for a given scene. The saliency map uses the computed values from local features as well as global information to generate a prediction on salient areas of the scene (Harel et al., 2007) in the range [0,1], with higher values representing the more salient areas (Figure 5C). Using the relevant baseline sequence for the animation in question, we computed a binary mask that specified where lizard movement is taking place within the scene. This was obtained by computing image motion in the baseline sequence, which contains only lizard display movements, using a gradient detector model (Peters et al., 2002) and thresholding the output such that all movement above 0.5 pixels was set to 1 (Figure 5D; image size is 720 × 576 pixels). Multiplying the salience score with the binary mask retained only the salience scores for lizard display movement in the scene (Figure 5E). This was repeated for all frames in the sequence to yield a vector of relative salience for each frame in the sequence (Figure 5F). We summarize these data further to determine the proportion of the sequence that exceeded a threshold value (dashed line in Figure 5F). For most analyses, we varied the value for this threshold by increments of 0.001 to provide a final estimate for the proportion of a given sequence as a function of the threshold for response (Figure 5G).
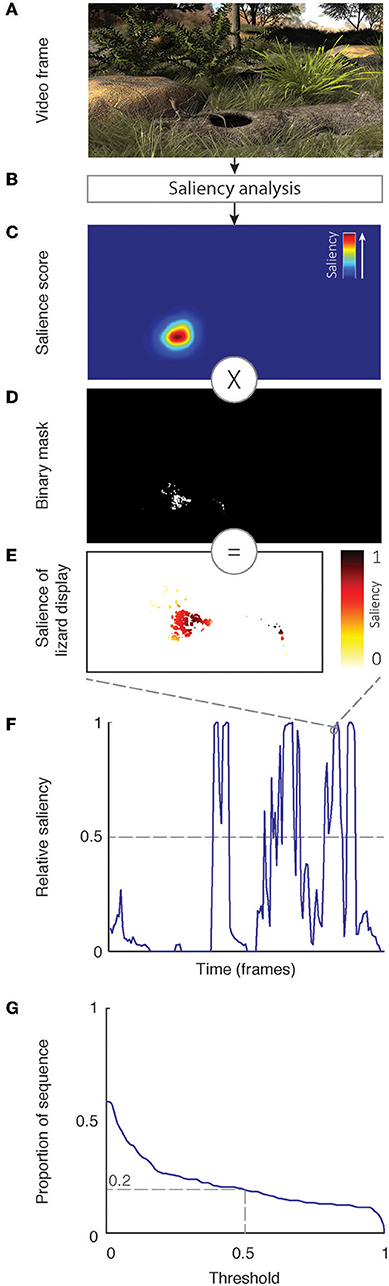
Figure 5. A schematic representation of our analytical approach to quantifying effectiveness of lizard displays in the animation sequences we created. Frames from the animation sequence (A) are analyzed using saliency analysis (B; see text for details) to yield a salience score in the range [0,1] for all areas of the frame (C). These scores are multiplied by a binary mask (D), which is derived separately and indicates where signal occurs [1] or does not occur [0]. This limits scores to areas of lizard display movement (E). The maximum value in this restricted set of salience scores represents the score for the respective frame in the sequence (F). The attention of receivers is predicted if the score exceeds a particular threshold; however, as explained in the text, we do not assume a single threshold for response. Rather we determine the (G) proportion of the sequence that exceeds a given threshold as a function of different threshold values.
Results
Changing Environmental Variables: Manipulations of Wind and Light
We tested the effect of environmental variables on signal efficacy by systematically varying wind and light conditions and computed the proportion of each sequence that exceeded a specified threshold value as a proxy for signal efficacy. Performance was subtly dependent on the interaction between prevailing wind conditions, the luminance profile, and receiver response thresholds. We summarize the results in Figure 6, focusing on three receiver thresholds. In general, performance declined as receivers became more discriminating (comparing across surface plots) and as prevailing wind and concomitant plant motion increased (arrow a in center plot). Rapid reductions in performance were apparent in the mid-range of wind speeds before performance flattens out. Performance improved to some degree with increased visual contrast due to changing luminance profiles in the scene (arrow b in center plot). This was particularly clear for moderately discriminating receivers; less discriminating receivers showed a rapid increase before it plateaus (values are close to 1), and highly discriminating receivers exhibited only a subtle increase. However, these outcomes were at low to moderate wind levels.
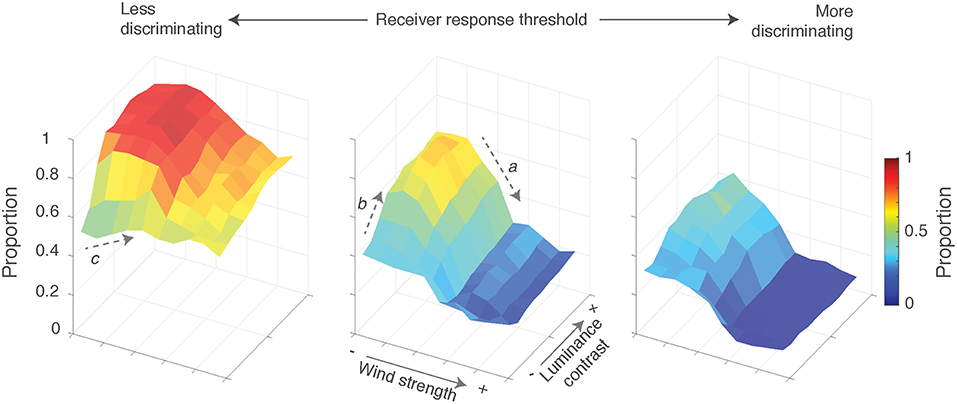
Figure 6. Signal effectiveness varied as a function of prevailing wind, the luminance profile of the scene and receiver response thresholds. Surface plots show the proportion of sequence duration that exceeds a specified threshold, as a function of wind strength and luminance contrast. Increased wind strength results in greater plant motion noise, while higher luminance contrast accentuates areas of light and dark. Separate plots are shown for different receiver response threshold: low (0.25), medium (0.5), and high (0.75) levels of discrimination (from left to right, respectively). The arrows labeled a, b, and c highlight the main outcomes referred to in the text: performance declines with increased wind speed (a), performance improves at low wind speeds with increased luminance contrast (b) and at low receiver thresholds, and flat luminance profiles, performance improves from low to moderate wind speeds (c).
Interestingly, when receivers are non-discriminating, and the luminance profile is flat, an increase in prevailing wind actually led to an improvement in performance (Figure 6, arrow c). We explored this further by examining the proportion above threshold as a function of changing thresholds, separating out the tail-flick and the rest of the display, for three levels each of wind and light conditions (Figure 7A). The beneficial effect of increased wind speed in this light environment is apparent for both the tail-flick component and the rest of the display (Figure 7A). Furthermore, it seems that this result is driven mostly by poor performance of both components of the signal in a scene with flatter luminance profiles and low wind conditions, as the same movement in the same wind conditions shows vast improvements when the luminance profile of the scene becomes more jagged (black line shifted to the right in middle and right sections under low luminance contrast in Figure 7A).
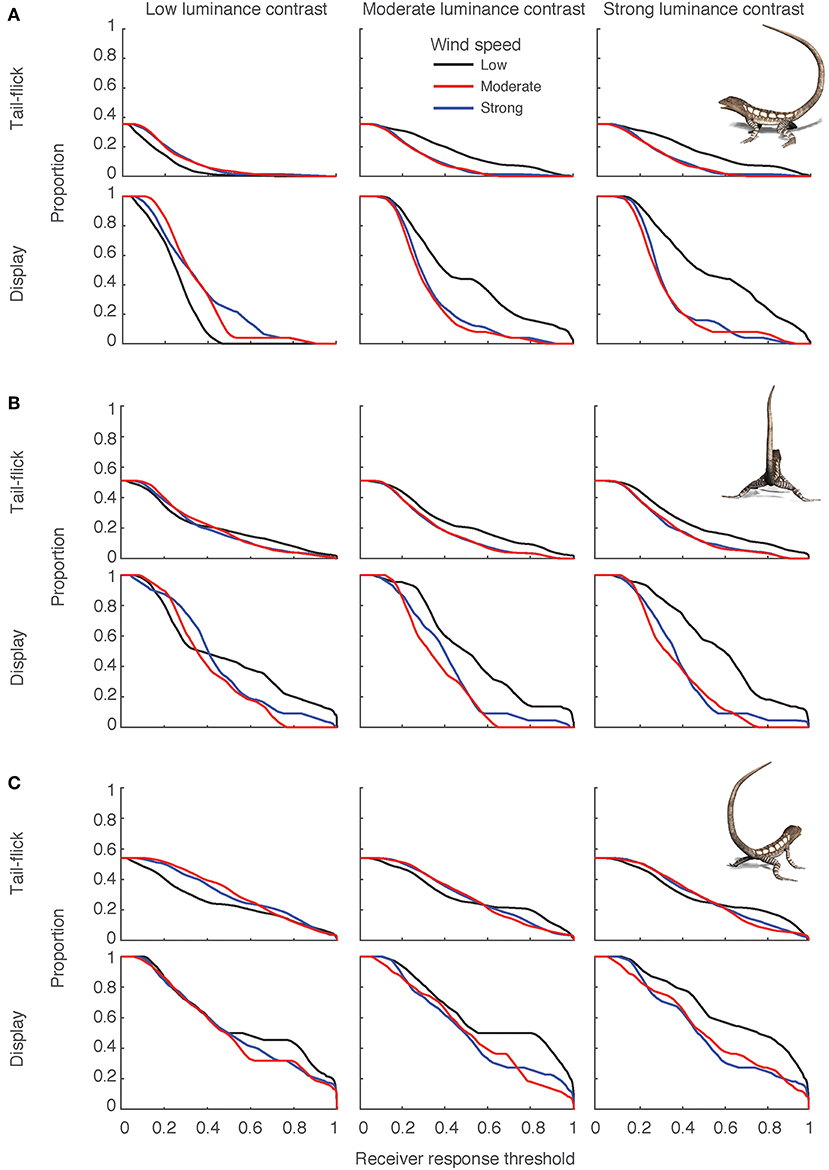
Figure 7. Signal effectiveness was influenced by signaler orientation, but was also mediated by the type of movement. Each plot shows the proportion of the lizard display with salience scores exceeding receiver thresholds for response, presented separately for the tail flick and rest of the display for three orientations: (A) side-on, (B) viewed from behind, (C) angled toward the right. Separate plots are shown for scenes with low (left column), moderate (middle column), and strong (right column) luminance contrast, while in each plot, lines represent low (black), moderate (blue), and strong (red) wind conditions.
Increasing Signaling Speed
To determine whether increasing signaling speed could improve signal efficacy, we produced two series of animations featuring the model lizard signaling at a fast (150%) and faster (200%) speed, using moderate wind conditions and a moderately jagged luminance profile. Figure 8A presents the proportion of sequence duration above threshold as a function of varying threshold levels for the original speed and the set of sequences exhibiting fast and faster speeds. In general, the data do not indicate an improvement when the original sequence duration is considered (8 s). There is, however, a slight improvement in performance for faster sequences relative to the normal speed over medium to high response thresholds (Figure 8A inset). Once again, sequences featuring faster movements were most effective under moderate to high receiver thresholds. We explored these data further by separating out the tail flick component and the rest of the display, and computed mean salience scores for the lizard movements (Figure 9). Fast and faster tail movements show modest improvement on the normal speed and were quite uniform across the range of backgrounds (Figure 9A). In contrast, performance by the rest of the display was more sensitive to the particular background against which it was viewed (Figure 9B). In Figure 8B, we use the time in motion as the denominator for calculating proportions rather than sequence duration. When considered in this way, we observed a substantial improvement in performance with increased signaling speed (Figure 8B).
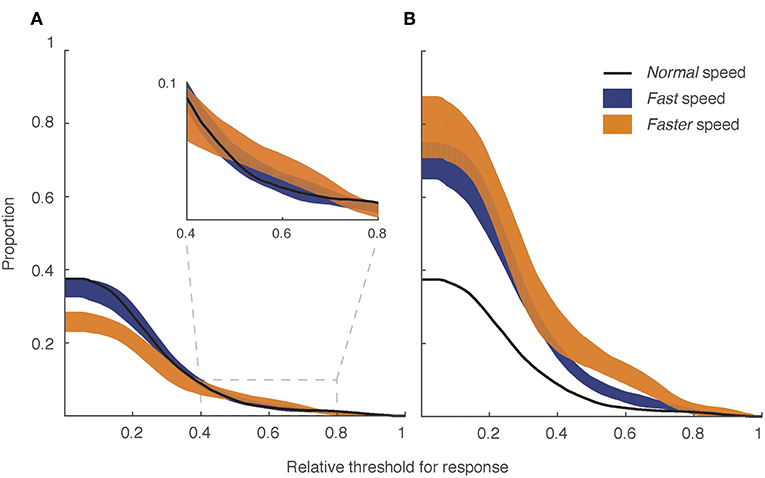
Figure 8. Signaling faster improves the salience of signal movements compared with plant movements, but reduces the time-frame over which the signal can be detected. The plots show the proportion of the lizard display with salience scores exceeding receiver thresholds for response in moderate wind (level 6) and luminance contrast (level 4). In each plot, the normal speed sequence (black line) is compared with the set of sequences in which display speed was increased by 1.5 × (blue; fast) and 2.0 ×(orange; faster) the normal speed. The shaded regions for the fast and faster sequences represent the minimum and maximum values for a given threshold. (A) Data is shown using the full 8 s sequence duration, with the reduction in performance for the fastest display movements a consequence of reduced time in motion. Despite this, the faster sequence was more effective at mid-high thresholds (see inset). (B) Data are shown as a function of time in motion and shows that both fast and faster movements are more salient than normal speed.
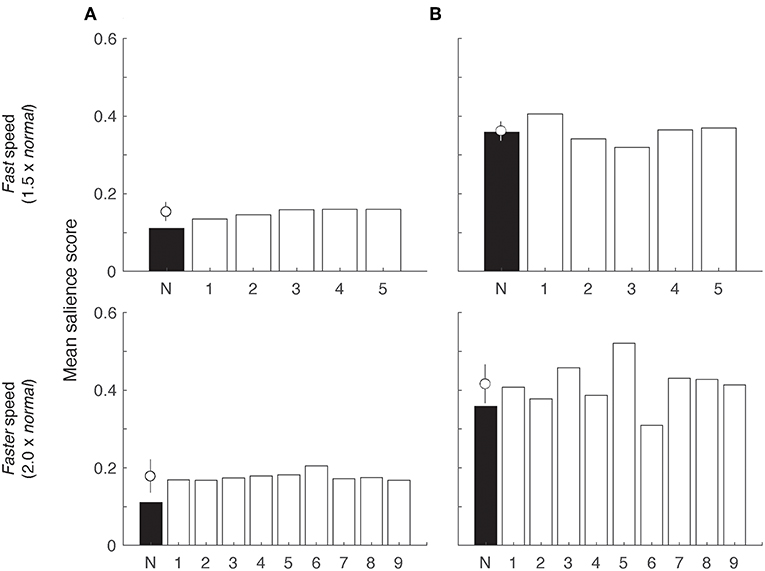
Figure 9. The salience scores of the tail flick component were lower than that of the rest of the display, but the rest of the display showed greater variability in response to background conditions. Bar charts show mean salience score of (A) tail flicks and (B) the rest of the display. Data are shown for the sequence at normal speed (N) and the set of animations at 1.5 × (top row) and 2.0 ×(bottom row) normal speed. In each plot, the normal speed sequence (N) is shown in black, with the open circle representing the mean (± SE) of the faster speed sequences to the right.
Changing Signaler Orientation
The proportion of the sequence that exceeded a threshold as a function of changing threshold in the baseline sequences is shown in Figure 10A, separately for each of the five different signaler orientations. For this particular display and in the absence of noise, performance was better when the signaler was oriented directly away from the receiver (back) or angled away (either left or right). Viewing this sequence with the signaler-oriented side on or facing the receiver were less effective. When we compared the salience of the whole display (Figure 10B) with different signal components (Figures 10C,D), the result showed that differences in signal efficacy due to signaler orientation is mainly driven by the tail flick component. For the non-tail-flick component of the display, varying the orientation of the signaler had a negligible effect on efficacy (Figure 10D).
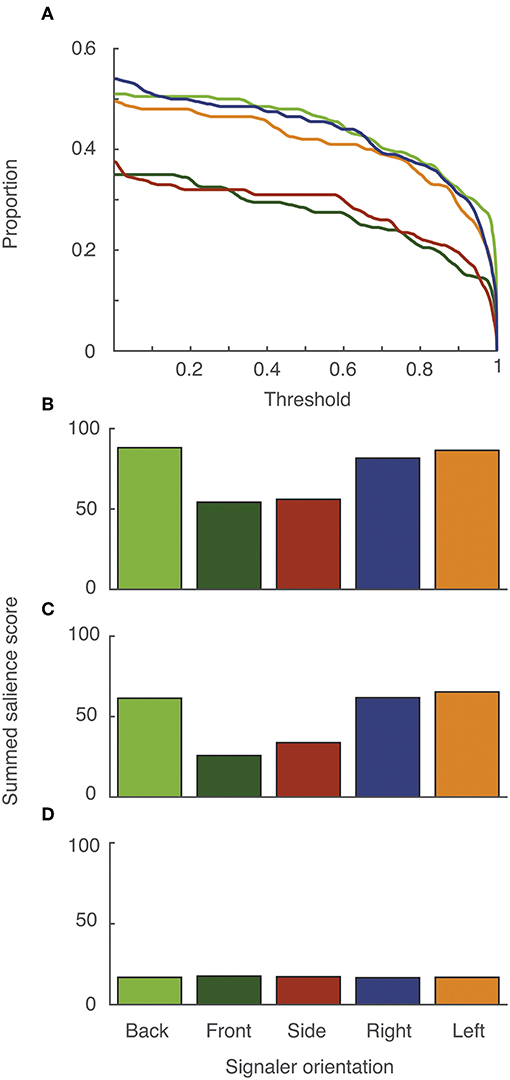
Figure 10. Signal effectiveness was mediated by signaler orientation, but only for tail-flicking. (A) The proportion of lizard displays with salience scores exceeding receiver thresholds for response, shown separately for five different signaler orientations. Summed salience scores for the whole display (B), and for the tail flick component (C) and rest of the display (D) separately. The color scheme in (A) is the same as in (B,D).
We then considered the effect of signaler orientation when we varied the environmental conditions and show that signaler orientation mediates signal performance. We previously showed that in scenes with a flat luminance profile, adding plant motion improved performance of both the tail flick and the rest of the display (Figure 7A). However, this applies when viewed from the side, and is not apparent when the signaler is facing away from the viewer (Figure 7B), and only applies to the tail flick component when the lizard is angled to the right (Figure 7C). It shows also that the detrimental effect of increasing plant motion varies according to signaler orientation.
Discussion
The movements of wind-blown plants are a major source of noise for movement-based signals and only rarely have been quantified in detail (but see Fleishman, 1986; Peters et al., 2008; Fleishman and Pallus, 2010). Such image motion backgrounds are likely to hinder detection of relevant visual motion signals in animals (Fleishman, 1986) and direct the evolution of effective communication strategies (sensu Endler, 1992). Knowledge of the environments in which animals operate and the sensory processing demands that mediate behavior in ecological contexts is crucial in understanding motion signal processing (e.g., Fleishman, 1988b). Physical measurements of plant-motion alone do not provide sufficient details for understanding the design of movement-based signal structure and the processing demands faced by animals. Our study presents an innovative approach using 3D animation to quantify motion signals and environmental characteristics simultaneously, with the ability to systematically manipulate one or more variables. The level of control within the animation environment gave us the opportunity to obtain a more complete picture of sensory constraints imposed by environmental conditions than previously attempted, and enabled us to assess whether changes to signal structure can be used to remain salient in different image motion environments. Although we could have varied many parameters relevant to the signaling context in our simulation environment, we opted to focus on just a few parameters in detail. The parameters that we varied in this study were shown empirically to be relevant (plant movements, signal speed) or that we suggested in the Introduction are relevant based on physical principles (light environment, signaler orientation). We discuss our results and their implications below; however, we acknowledge from the outset that we have modeled a single lizard display in a single habitat and thus our results are not suitable for inferential statistics and generalizations are made with caution. Nevertheless, our simulations break new ground for research into the motion ecology of animals and provides novel insight that can guide future work.
The results of the present set of simulations suggest that environmental conditions at the time of signaling do indeed affect signal efficacy and behavioral changes can mediate the masking effect of environmental noise. We acknowledge that although our agent for selection utilized well-established tools for measuring salience of scenes (Itti et al., 1998), there are limitations to its utility owing to inherent assumptions that do not reflect precisely the complex interactions involved in predicting attentional capture in natural scenes (Tatler et al., 2011). Much criticism of this approach centers on the use of static images, so adopting a model that incorporates dynamic information (Harel et al., 2007) at the feature layer is a step in the right direction, as well as being integral to our research question. Nevertheless, the set of animations we have created can be reanalyzed with different analytical approaches, which we are currently investigating. For example, incorporating top-down guidance as to where conspecifics are most likely to be located (Kanan et al., 2009) and explicitly modeling non-uniform sampling by visual systems of natural scenes (Wischnewski et al., 2010) will be useful extensions to the novel analysis we present herein, but require knowledge that is not yet in hand for lizards.
Environmental Conditions Affect Signal Efficacy
A number of previous studies have demonstrated that plant-motion is an important sensory constraint for the reception of movement-based signals in several lizard species including A. muricatus (Fleishman, 1986; Ord et al., 2007; Peters et al., 2007). Similarly, our analysis showed an overall decline in signal efficacy as we systematically increased wind strength in the simulated environment. However, our results offer an interesting caveat in that when receivers are less discriminating, the effect of prevailing wind might actually facilitate segmentation depending on the light environment and the orientation of the signaler. This effect is not present when receivers become moderately to highly discriminating. Likewise, the facilitating effect of increased luminance contrast at low wind levels flattens out when receivers are more discriminating (Figure 6).
Peters et al. (2008) has described in detail the image motion generated by different plant communities under a range of meteorological conditions across multiple sites. Their results demonstrated that plant-motion varied as a function of wind speed, plant structure and habitat topography. Fleishman and Pallus (2010) demonstrated the importance of local conditions for lizards when they modeled the signal detection task of receiver visual systems for multiple species of Anolis lizards. Changing noise levels had a detrimental effect for only one of five species, but the environmental conditions were moderate at worst. It would be interesting to know whether signal performance would be adversely affected for the other four species if noise levels spanned a greater range of conditions, as utilized in the present study. However, although we quantified signal performance under an unprecedented range of environmental conditions, our study focused on one signal in one habitat. Taking the lead from Fleishman and Pallus (2010), we need to consider multiple signals and multiple habitats to understand fully the environmental constraints on motion signaling. To this end, we are in the process of simulating multiple lizard species, which have very distinctive motor patterns, and the different habitats they occupy. We will then have the ability to compare signal performance in different habitats across different environmental conditions. More importantly, this involves “swapping” species between habitats to examine performance in a manner that would not be possible in nature due to government regulations preventing translocations outside of species' natural ranges.
Our study provides novel insights into the effect of the light environment on the efficacy of movement-based signals. As light passes through vegetated environments it casts shadows and creates areas of rapid changes in light intensity. These areas can change dynamically if the structures upon which the shadows are cast are moving, and furthermore, if the objects causing the shadows are themselves moving. These features of the environment are potentially very important for movement-based signals as the visual systems of receivers use luminance differences to detect motion, and the perception of movement is most reliable for areas of high-contrast. Matchette et al. (2018) recently demonstrated that detection of a moving prey item by human observers was more difficult when prey moved across dynamic, dappled areas of the scene. This potential anti-predator strategy is an option because it is a difficult visual processing task for the receiver. Similarly, we show here that the interaction between luminance profiles and wind-blown plants can mask a signal, however, this time the signaler wants to be seen. This is an underappreciated area of investigation and worthy of further consideration. The light environment is important for static visual signaling systems (Endler, 1993b; Endler and Thery, 1996) but the present study suggests it is also relevant in a dynamic world.
Behavioral Strategies Can Mediate the Masking Effect of Environmental Noise
Adjusting behavior and the structure of signals to enhance signal reception in “noisy” environments is widely observed in animals across diverse taxonomic groups (Ryan et al., 1990; Ord et al., 2007; Peters et al., 2007). In the present study, we showed quantitatively that simple behavioral modifications can indeed improve efficacy of movement-based signals, but whether these are actually useful strategies is more complicated. Animals that signal faster could enjoy efficacy benefits, and our results suggest that doubling the speed of movement is preferable. However, it is not clear whether such speeds are achievable in lizards, or what the energetic demands of this extra activity might entail (Read et al., 2013). The potential energetic demands are compounded when we consider that these faster movements would need to be sustained over a longer time period (Peters and Evans, 2003). Furthermore, signaling faster over sustained periods might also increase the risk of predation by predators that also detect the movement (Steinberg et al., 2014). Finally, while evaluating these extra costs, it is worth being mindful of the result that suggested displays (excluding tail-flicks) are quite sensitive to the level of noise at the time of signaling. In contrast, tail-flicks alone were less sensitive to noise conditions and might be better suited to sustained signaling (Peters et al., 2007).
Our results also show that orientation of the signaler relative to surrounding plant-motion noise has implications for signal efficacy (Figures 7, 10). The Jacky dragon display consists of tail-flicks, followed by a rapid sequence of foreleg waves and other movements centered on a push-up (Peters and Ord, 2003; Ramos and Peters, 2016). When we partitioned out the tail-flick from the rest of the display we observed that tail movements were susceptible to lizard orientation, but the rest of the display was more robust to variation in orientation. This can be explained by the nature of the two sets of movements. Tail movements in this species occur in random directions and can go in and out of view depending on the structure of the environment and the presence of occluding objects. In contrast, as the rest of the display has a far more restricted range of movement, and mostly delivered in full view, it is relatively robust to changes in orientation. Signaler orientation also appears to mediate performance in noise, and there are likely to be optimal orientations for a given movement type that might reduce the effects of noise.
The two strategies investigated for mediating the masking effect of plant motion thus seem to favor different components of the display. Tail-flicks show an improvement with faster speeds, are less susceptible to prevailing noise levels and, we suggest, are less costly during sustained signaling; yet they are susceptible to performance decrements due to signaler orientation. In contrast, the rest of the display is robust to signaler orientation and faster movements are beneficial. However, these must be weighed up against the energetic and predation costs of such movements, which are undetermined at this stage. How do these data and interpretations fit with what we know about Jacky dragon behavior? Our analysis shows that faster signaling might be a strategy they could adopt, but it is seemingly not what the lizards do in response to increased noise levels. Peters et al. (2007) showed that lizards lengthened the duration of tail flicking without changing speeds in windy conditions. They also switched to more intermittent movements. We held this component constant in the present analysis because we were explicitly testing the same display in different circumstances, but the efficacy benefit of structural variations of this kind should be investigated.
Concluding Remarks
Our study used an innovative approach to understand the complex effects of environmental conditions on movement-based signal performance. We have quantified in detail an interactive effect of changing environmental wind conditions and varying the luminance profile of the scene. In addition to novel insight into the role of the light environment, we also suggest that orientation of signalers relative to the surrounding plants is relevant, particularly for some motor patterns. Our approach to understanding animal communication is entirely novel, and the results herein are very informative; however, the greater benefit of simulations like ours comes from guiding work on living animals. On the basis of our results, we encourage others working on motion-signaling species to take a fresh look at the circumstances in which signaling takes place. For example, where do animals perform their display with respect to surrounding plants and the light environment? How do animals position themselves when signaling and how do they adjust positions during displays? Are there conditions in which signaling does not take place? Finally, the present analysis suggests that signal performance is very much a consequence of receiver thresholds for response. It is worthwhile to know whether potential receivers of these types of displays exhibit varying thresholds under different contexts. For example, when foraging and seeking out the movements of potential prey (Hoese et al., 2008), are they more, less, or equally likely to detect a conspecific territorial display?
Author Contributions
The project was designed by RP and XB. Animation work was undertaken by XB and AP. Manuscript was prepared by XB and RP with input from all authors.
Funding
This work was supported by an Australian Research Council Discovery Project Grant (DP170102370).
Conflict of Interest Statement
The authors declare that the research was conducted in the absence of any commercial or financial relationships that could be construed as a potential conflict of interest.
Acknowledgments
We are grateful to Nicole Butler and Jose Ramos for comments on the manuscript, Jose Ramos for providing field data, Chandara Ung for providing earlier versions of the lizard model and Warwick Laird for providing earlier versions of the habitat model.
References
Akagi, Y., and Kitajima, K. (2006). Computer animation of swaying trees based on physical simulation. Comput. Graphics 30, 529–539. doi: 10.1016/j.cag.2006.03.017
Atema, J. (1995). Chemical signals in the marine environment: dispersal, detection, and temporal signal analysis. Proc. Nat. Acad. Sci. Am. 92, 62–66. doi: 10.1073/pnas.92.1.62
Bian, X., Chandler, T., Laird, W., Pinilla, A., and Peters, R. (2018). Integrating evolutionary biology with digital arts to quantify ecological constraints on vision-based behaviour. Methods Ecol. Evol. 9, 544–559. doi: 10.1111/2041-210X.12912
Bian, X., Elgar, M. A., and Peters, R. A. (2016). The swaying behavior of Extatosoma tiaratum: motion camouflage in a stick insect? Behav. Ecol. 27, 83–92. doi: 10.1093/beheco/arv125
Bortolotti, G. R., Stoffel, M. J., and Galvan, I. (2011). Wintering Snowy Owls Bubo scandiacus integrate plumage color, behaviour and their environment to maximize efficacy of visual displays. IBIS 153, 134–142. doi: 10.1111/j.1474-919X.2010.01067.x
Brumm, H. (2004). The impact of environmental noise on song amplitude in a territorial bird. J. Anim. Ecol. 73, 434–440. doi: 10.1111/j.0021-8790.2004.00814.x
Brumm, H., and Slabbekoorn, H. (2005). Acoustic communication in noise. Adv. Study Behav. 35, 151–209. doi: 10.1016/S0065-3454(05)35004-2
Chapman, P. R., and Underwood, G. (1998). Visual search of driving situations: danger and experience. Perception 27, 951–964. doi: 10.1068/p270951
Chouinard-Thuly, L., Gierszewski, S., Rosenthal, G. G., Reader, S. M., Rieucau, G., Woo, K. L., et al. (2017). Technical and conceptual considerations for using animated stimuli in studies of animal behavior. Curr. Zool. 63, 5–19. doi: 10.1093/cz/zow104
Cocroft, R. B., and Rodriguez, R. L. (2005). The behavioral ecology of insect vibrational communication. Bioscience 55, 323–334. doi: 10.1641/0006-3568(2005)055[0323:TBEOIV]2.0.CO;2
Cogger, H. (1978). Reproductive cycles, fat body cycles and socio-sexual behaviour in the mallee dragon, Amphibolurus fordi (Lacertilia: Agamidae). Aust. J. Zool. 26, 653–672. doi: 10.1071/ZO9780653
Cropper, S. J., and Wuerger, S. M. (2005). The perception of motion in chromatic stimuli. Behav. Cogn. Neurosci. Rev. 4, 192–217. doi: 10.1177/1534582305285120
Cynx, J., Lewis, R., Tavel, B., and Tse, H. (1998). Amplitude regulation of vocalizations in noise by a songbird, Taeniopygia guttata. Anim. Behav. 56, 107–113. doi: 10.1006/anbe.1998.0746
de Ibarra, N. H., Giurfa, M., and Vorobyev, M. (2001). Detection of colored patterns by honeybees through chromatic and achromatic cues. J. Compar. Physiol. A 187, 215–224. doi: 10.1007/s003590100192
Eckert, M. P., and Zeil, J. (2001). “Towards an ecology of motion vision,” in Motion Vision: Computational, Neural and Ecological Constraints, eds J. M. Zanker and J. Zeil (Heidelberg: Springer), 333–369. doi: 10.1007/978-3-642-56550-2_18
Elias, D., Land, B., Mason, A., and Hoy, R. (2006). Measuring and quantifying dynamic visual signals in jumping spiders. J. Compar. Physiol. A 192, 785–797. doi: 10.1007/s00359-006-0116-7
Endler, J. A. (1990). On the measurement and classification of color in studies of animal color patterns. Biol. J. Linn. Soc. 41, 315–352. doi: 10.1111/j.1095-8312.1990.tb00839.x
Endler, J. A. (1992). Signals, signal conditions, and the direction of evolution. Am. Nat. 139, 125–153. doi: 10.1086/285308
Endler, J. A. (1993a). Some general comments on the evolution and design of animal communication systems. Philos. Trans. Biol. Sci. 340, 215–225. doi: 10.1098/rstb.1993.0060
Endler, J. A. (1993b). The color of light in forests and its implications. Ecol. Monogr. 63, 1–27. doi: 10.2307/2937121
Endler, J. A., and Thery, M. (1996). Interacting effects of lek placement, display behavior, ambient light, and color patterns in three neotropical forest-dwelling birds. Am. Nat. 148, 421–452. doi: 10.1086/285934
Fleishman, L., and Persons, M. (2001). The influence of stimulus and background color on signal visibility in the lizard Anolis cristatellus. J. Exp. Biol. 204, 1559–1575.
Fleishman, L. J. (1986). Motion detection in the presence and absence of background motion in an Anolis lizard. J. Compar. Physiol. A 159, 711–720. doi: 10.1007/BF00612043
Fleishman, L. J. (1988a). Sensory and environmental influences on display form in Anolis auratus, a grass anole from panama. Behav. Ecol. Sociobiol. 22, 309–316.
Fleishman, L. J. (1988b). Sensory influences on physical design of a visual display. Anim. Behav. 36, 1420–1424. doi: 10.1016/S0003-3472(88)80212-4
Fleishman, L. J. (1992). The influence of the sensory system and the environment on motion patterns in the visual-displays of anoline lizards and other vertebrates. Am. Nat. 139, S36–S61. doi: 10.1086/285304
Fleishman, L. J., and Pallus, A. C. (2010). Motion perception and visual signal design in Anolis lizards. Proc. Biol. Sci. 277, 3547–3554. doi: 10.1098/rspb.2010.0742
Gabbiani, F., Metzner, W., Wessel, R., and Koch, C. (1996). From stimulus encoding to feature extraction in weakly electric fish. Nature 384, 564–567. doi: 10.1038/384564a0
Giurfa, M., Vorobyev, M., Kevan, P., and Menzel, R. (1996). Detection of colored stimuli by honeybees: minimum visual angles and receptor specific contrasts. J. Compar. Physiol. A 178, 699–709. doi: 10.1007/BF00227381
Greenfield, M. D. (1988). Interspecific acoustic interactions among katydids Neoconocephalus: inhibition-induced shifts in diel periodicity. Anim. Behav. 36, 684–695. doi: 10.1016/S0003-3472(88)80151-9
Habel, R., Kusternig, A., and Wimmer, M. (2009). Physically guided animation of trees. Computer Graphics Forum 28, 533–540. doi: 10.1111/j.1467-8659.2009.01391.x
Hannah, P., Palutikof, J., and Quine, C. (1995). “Predicting windspeeds for forest areas in complex terrain,” in Wind and Trees, eds M. Coutts and J. Grace (Edinburgh; Cambridge: Cambridge University Press), 113–129. doi: 10.1017/CBO9780511600425.007
Harel, J., Koch, C., and Perona, P. (2007). Graph-based visual saliency. Adv. Nerual Informat. Process. Syst. 19, 545–552. doi: 10.7551/mitpress/7503.003.0073
Hedrick, T. L. (2008). Software techniques for two-and three-dimensional kinematic measurements of biological and biomimetic systems. Bioinspir. Biomim. 3:034001. doi: 10.1088/1748-3182/3/3/034001
Hoese, F., Peters, R. A., and Evans, C. S. (2008). The effect of variation in prey movement on the predatory response of jacky lizards (Amphibolurus muricatus). Ethology 114, 718–727. doi: 10.1111/j.1439-0310.2008.01517.x
Hunter, M. L., and Krebs, J. R. (1979). Geographical variation in the song of the great tit (Parus major) in relation to ecological factors. J. Anim. Ecol. 48, 759–785. doi: 10.2307/4194
Itti, L., Koch, C., and Niebur, E. (1998). A model of saliency-based visual attention for rapid scene analysis. IEEE Trans. Pattern Anal. Mach. Intell. 20, 1254–1259. doi: 10.1109/34.730558
Kanan, C., Tong, M. H., Zhang, L. Y., and Cottrell, G. W. (2009). SUN: Top-down saliency using natural statistics. Vis. cogn. 17, 979–1003. doi: 10.1080/13506280902771138
Khorloo, O., Gunjee, Z., Sosorbaram, B., and Chiba, N. (2011). Wind field synthesis for animating wind-induced vibration. Int. J. Virt. Reality 10, 53–60.
Klomp, D. A., Stuart-Fox, D., Das, I., and Ord, T. J. (2017). Gliding lizards use the position of the sun to enhance social display. Biol. Lett. 13:20160979. doi: 10.1098/rsbl.2016.0979
Kuhn, G., and Tatler, B. W. (2005). Magic and fixation: now you don't see it, now you do. Perception 34, 1155–1161. doi: 10.1068/p3409bn1
Land, M., Mennie, N., and Rusted, J. (1999). The roles of vision and eye movements in the control of activities of daily living. Perception 28, 1311–1328. doi: 10.1068/p2935
Leal, M., and Fleishman, L. J. (2003). Differences in visual signal design and detectability between allopatric populations of Anolis lizards. Am. Nat. 163, 26–39. doi: 10.1086/379794
Lengagne, T., and Slater, P. J. B. (2002). The effects of rain on acoustic communication: tawny owls have good reason for calling less in wet weather. Proc. R. Soc. B Biol. Sci. 269, 2121–2125. doi: 10.1098/rspb.2002.2115
Leonards, U., and Singer, W. (1998). Two segmentation mechanisms with differential sensitivity for color and luminance contrast. Vis. Res. 38, 101–109. doi: 10.1016/S0042-6989(97)00148-X
Matchette, S. R., Cuthill, I. C., and Scott-Samuel, N. E. (2018). Concealment in a dynamic world: dappled light and caustics mask movement. Anim. Behav. 143, 51–57. doi: 10.1016/j.anbehav.2018.07.003
Mccoy, J. K., Harmon, H. J., Baird, T., and Fox, S. F. (1997). Geographic variation in sexual dichromatism in the collared lizard, Crotaphytus collaris (Sauria: Crotaphytidae). Copeia 1997, 565–571. doi: 10.2307/1447560
Morton, E. S. (1975). Ecological sources of selection on avian sounds. Am. Nat. 109, 17–34. doi: 10.1086/282971
Narins, P. M. (1990). Seismic communication in anuran amphibians. Bioscience 40, 268–274. doi: 10.2307/1311263
Ord, T. J., Blumstein, D. T., and Evans, C. S. (2002). Ecology and signal evolution in lizards. Biol. J. Linn. Soc. 77, 127–148. doi: 10.1046/j.1095-8312.2002.00100.x
Ord, T. J., Peters, R. A., Clucas, B., and Stamps, J. A. (2007). Lizards speed up visual displays in noisy motion habitats. Proc. Biol. Sci. 274, 1057–1062. doi: 10.1098/rspb.2006.0263
Ord, T. J., and Stamps, J. A. (2008). Alert signals enhance animal communication in “noisy” environments. Proc. Natl. Acad. Sci. U.S.A. 105, 18830–18835. doi: 10.1073/pnas.0807657105
Ota, S., Tamura, M., Fujimoto, T., Muraoka, K., and Chiba, N. (2004). A hybrid method for real-time animation of trees swaying in wind fields. Vis. Comput. 20, 613–623. doi: 10.1007/s00371-004-0266-y
Patricelli, G. L., and Blickley, J. L. (2006). Avian communication in urban noise: causes and consequences of vocal adjustment. Auk Ornithol. Adv. 123, 639–649. doi: 10.1642/0004-8038(2006)123[639:ACIUNC]2.0.CO;2
Peters, R. A. (2008). Environmental motion delays the detection of movement-based signals. Biol. Lett. 4, 2–5. doi: 10.1098/rsbl.2007.0422
Peters, R. A. (2013). “Noise in visual communication: motion from wind-blown plants,” in Animal Communication and Noise, ed H. Brumm (Heidleberg, Springer), 311–330. doi: 10.1007/978-3-642-41494-7_11
Peters, R. A., Clifford, C. W. G., and Evans, C. S. (2002). Measuring the structure of dynamic visual signals. Anim. Behav. 64, 131–146. doi: 10.1006/anbe.2002.3015
Peters, R. A., and Evans, C. S. (2003). Design of the Jacky dragon visual display: signal and noise characteristics in a complex moving environment. J. Compar. Physiol. A 189, 447–459. doi: 10.1007/s00359-003-0423-1
Peters, R. A., Hemmi, J., and Zeil, J. (2008). Image motion environments: background noise for movement-based animal signals. J. Compar. Physiol. A 194, 441–456. doi: 10.1007/s00359-008-0317-3
Peters, R. A., Hemmi, J. M., and Zeil, J. (2007). Signaling against the wind: modifying motion-signal structure in response to increased noise. Curr. Biol. 17, 1231–1234. doi: 10.1016/j.cub.2007.06.035
Peters, R. A., and Ord, T. J. (2003). Display response of the Jacky Dragon, Amphibolurus muricatus (Lacertilia: Agamidae), to intruders: a semi-Markovian process. Austral. Ecol. 28, 499–506. doi: 10.1046/j.1442-9993.2003.01306.x
Peters, R. A., Ramos, J. A., Hernandez, J., Wu, Y., and Qi, Y. (2016). Social context affects tail displays by Phrynocephalus vlangalii lizards from China. Sci. Rep. 6:31573. doi: 10.1038/srep31573
Pike, T. W. (2018). Quantifying camouflage and conspicuousness using visual salience. Methods Ecol. Evol. 9, 1883–1895. doi: 10.1111/2041-210X.13019
Ramos, J. A., and Peters, R. A. (2016). Dragon wars: movement-based signalling by Australian agamid lizards in relation to species ecology. Austral. Ecol. 41, 302–315. doi: 10.1111/aec.12312
Ramos, J. A., and Peters, R. A. (2017). Quantifying ecological constraints on motion signaling. Front. Ecol. Evol. 5:9. doi: 10.3389/fevo.2017.00009
Read, J., Jones, G., and Radford, A. N. (2013). Fitness costs as well as benefits are important when considering responses to anthropogenic noise. Behav. Ecol. 25, 4–7. doi: 10.1093/beheco/art102
Rundus, A. S., Owings, D. H., Joshi, S. S., Chinn, E., and Giannini, N. (2007). Ground squirrels use an infrared signal to deter rattlesnake predation. Proc. Natl. Acad. Sci. U.S.A. 104, 14372–14376. doi: 10.1073/pnas.0702599104
Ryan, M. J., Cocroft, R. B., and Wilczynski, W. (1990). The role of environmental selection in intraspecific divergence of mate recognition signals in the cricket frog, Acris Crepitans. Evolution 44, 1869–1872. doi: 10.1111/j.1558-5646.1990.tb05256.x
Sakaguchi, T., and Ohya, J. (1999). Modeling and animation of botanical trees for interactive virtual environments. Sympos. Virt. Reality Softw. Technol. 99, 139–146. doi: 10.1145/323663.323685
Shinya, M., and Fournier, A. (1992). Stochastic motion—motion under the influence of wind. EuroGraphics 92, 119–128. doi: 10.1111/1467-8659.1130119
Sicsu, P., Manica, L. T., Maia, R., and Macedo, R. H. (2013). Here comes the sun: multimodal displays are associated with sunlight incidence. Behav. Ecol. Sociobiol. 67, 1633–1642. doi: 10.1007/s00265-013-1574-x
Slabbekoorn, H., and Smith, T. B. (2002). Habitat-dependent song divergence in the little greenbul: an analysis of environmental selection pressures on acoustic signals. Evolution 56, 1849–1858. doi: 10.1111/j.0014-3820.2002.tb00199.x
Stam, J. (1997). Stochastic dynamics: Simulating the effects of turbulence on flexible structures. EuroGraphics 97, 159–164.
Steinberg, D. S., Losos, J. B., Schoener, T. W., Spiller, D. A., Kolbe, J. J., and Leal, M. (2014). Predation-associated modulation of movement-based signals by a Bahamian lizard. Proc. Natl. Acad. Sci. 111, 9187–9192. doi: 10.1073/pnas.1407190111
Swets, J. A. (1973). The relative operating characteristic in psychology: a technique for isolating effects of response bias finds wide use in the study of perception and cognition. Science 182, 990–1000. doi: 10.1126/science.182.4116.990
Tatler, B. W., Hayhoe, M. M., Land, M. F., and Ballard, D. H. (2011). Eye guidance in natural vision: reinterpreting salience. J. Vis. 11:5. doi: 10.1167/11.5.5
Wiley, R. H. (2006). Signal detection and animal communication. Adv. Study Behav. 36, 217–247. doi: 10.1016/S0065-3454(06)36005-6
Wiley, R. H. (2013). Signal detection, noise, and the evolution of communication. Anim. Commun. Noise 2, 7–30. doi: 10.1007/978-3-642-41494-7_2
Wiley, R. H. (2015). Noise Matters - The Evolution of Communication. Cambridge, Harvard University Press. doi: 10.4159/9780674287044
Wiley, R. H., and Richards, D. G. (1983). “Adaptations for acoustic communication in birds: sound transmission and signal detection,” in Ecology and Evolution of Acoustic communication in birds, Vol. 1. eds D. E. Kroodsma and E. H. Miller (New York, NY: Academic Press), 131–181.
Wischnewski, M., Belardinelli, A., Schneider, W. X., and Steil, J. J. (2010). Where to look next? Combining static and dynamic proto-objects in a TVA-based model of visual attention. Cogn. Comput. 2, 326–343. doi: 10.1007/s12559-010-9080-1
Witte, K., Farris, H. E., Ryan, M. J., and Wilczynski, W. (2005). How cricket frog females deal with a noisy world: habitat-related differences in auditory tuning. Behav. Ecol. 16, 571–579. doi: 10.1093/beheco/ari032
Wong, J. C., and Datta, A. (2004). “Animating real-time realistic movements in small plants,” In Proceedings of the 2nd International Conference on GRAPHITE' 04 (New York, NY: ACM), 182–189. doi: 10.1145/988834.988866
Keywords: animal communication, background noise, movement-based signal, visual saliency, signal evolution, 3D animation
Citation: Bian X, Chandler T, Pinilla A and Peters RA (2019) Now You See Me, Now You Don't: Environmental Conditions, Signaler Behavior, and Receiver Response Thresholds Interact to Determine the Efficacy of a Movement-Based Animal Signal. Front. Ecol. Evol. 7:130. doi: 10.3389/fevo.2019.00130
Received: 17 December 2018; Accepted: 01 April 2019;
Published: 18 April 2019.
Edited by:
Martin Stevens, University of Exeter, United KingdomReviewed by:
Ximena J. Nelson, University of Canterbury, New ZealandKeith Tarvin, Oberlin College, United States
Russell Ligon, Cornell University, United States
Copyright © 2019 Bian, Chandler, Pinilla and Peters. This is an open-access article distributed under the terms of the Creative Commons Attribution License (CC BY). The use, distribution or reproduction in other forums is permitted, provided the original author(s) and the copyright owner(s) are credited and that the original publication in this journal is cited, in accordance with accepted academic practice. No use, distribution or reproduction is permitted which does not comply with these terms.
*Correspondence: Richard A. Peters, richard.peters@latrobe.edu.au