- 1US Forest Service Pacific Northwest Research Station, La Grande, OR, United States
- 2Eagle Environmental, Incorporated, Fort Collins, CO, United States
- 3Montana Fish, Wildlife & Parks, Bozeman, MT, United States
Modeling landscape use (i.e., estimating the probability or relative probability of use, occurrence, or selection in a given area and time) by ungulates is an increasingly common and important practice in research and management. Models of occupancy, distribution, movement, habitat use, and resource selection are formal approaches by which landscape use has been characterized and results published for a myriad of ungulate species. Understanding landscape use has benefited from a growing volume of data on animal locations and model covariates, and the ease of modeling with automated software. These models are particularly noteworthy in their potential to estimate use at multiple scales, characterize individual and population distributions, and predict spatiotemporal responses to environmental change. Despite these advantages, ecological processes can be secondary or forgotten. Models without a strong ecological foundation may perform well in case studies but fail to advance our understanding of a species’ habitat requirements and response to habitat change across a broad inference space. In response, we describe criteria, synthesized from the ecological literature, of direct relevance to modeling landscape use for advancing the ecological understanding and effective management of ungulates. Criteria include (1) a knowledge coproduction framework for scientist-manager collaborations; (2) an explicit inference space with supporting replication for broad inference; (3) process covariates and their ecological scaling to address habitat requirements; (4) ecologically plausible sets of competing models; (5) model evaluation to address objectives and hypotheses of ecological importance; (6) assessment of relationships with animal and population performance; and (7) reliable interpretations for ecological understanding and management uses. Contemporary modeling of landscape use has been challenged by large, disparate data sources and an emphasis on statistical methods. However, advances in knowledge and conservation of ungulates based on tenets of ecology, management, and inference are achievable with careful consideration of these criteria.
Introduction
Modeling landscape use, based on estimates of occupancy (Royle et al., 2012), distributions (Jarnevich et al., 2015), movement (Horne et al., 2007), frequency of use (Nielson and Sawyer, 2013), or resource selection (Boyce et al., 2002), is a common practice in contemporary ungulate research and management. While statistical assumptions and intended applications vary, models of landscape use estimate the probability or relative probability of species use, occurrence, or selection of a given area and time (per details by Lele et al., 2013), and provide valuable knowledge about animal behavior that presumably reflects a species’ habitat needs (Gaillard et al., 2010). These models are particularly appealing because they are highly flexible, employing a multivariate framework of potential covariates and interactions that can include predation, nutrition, human disturbance, vegetation, climate, weather, topography, and myriad other biotic and abiotic factors (Boyce et al., 2016). Well-designed models of landscape use provide a means to evaluate and predict how populations respond to habitat change from a variety of anthropogenic activities, management strategies, and ecological processes (e.g., Sawyer et al., 2006, 2019; White et al., 2018; Briscoe et al., 2019; Reinking et al., 2019).
Spatial data readily available from geographic information systems (GIS) provide continuous coverage, open-source layers for deriving many covariates (Turner et al., 2015), and current technologies provide voluminous data on animal use across large areas (Hebblewhite and Haydon, 2010; Burton et al., 2015; Middleton et al., 2019). Development and evaluation of landscape-use models can now be accomplished efficiently with canned statistical analysis routines in freely available software packages.
Landscape-use modeling, however, often is focused on statistical methods rather than on ecological processes (Wisdom et al., 2018a, b; Briscoe et al., 2019). Without a strong ecological framework, critics have argued that models of landscape use are not process-based (Morris, 2003; Gaillard et al., 2010), not developed with ecological rationale (McLoughlin et al., 2010; Laforge et al., 2015), fail to identify a clear inference space with appropriate replication (Nielsen et al., 2010; Wisdom et al., 2018a, b; Briscoe et al., 2019), not evaluated with independent data (Roberts et al., 2017) of ecological importance (Rykiel, 1996; Johnson, 2001), and lack connections with demographic consequences (Gaillard et al., 2010; Matthiopoulos et al., 2015, 2019). Without considering these issues, it has been argued that patterns of landscape use simply reflect animal behavior (Garshelis, 2000). And, if patterns of landscape use are not replicated over large areas and long time periods, robust predictions to variation in space and time are unknown.
Critics have voiced particular concerns about the use of model covariates that may not reflect ecological processes (Morris, 2003). Covariates often are assumed to index specific processes or habitat requirements (i.e., surrogate variables), but evidence for linkages to processes often is absent or unclear (Nielsen et al., 2010). Many vegetation and abiotic covariates, for example, are readily available in open-source formats world-wide (Chen et al., 2015; Shean et al., 2016). By contrast, covariates of nutrition, predation, and human disturbance, which explicitly reflect processes of energy acquisition and loss, typically require extensive field sampling (e.g., Cook et al., 2016; Proffitt et al., 2016, 2019). Consequently these process covariates are less likely to be considered (Wisdom et al., 2018a), in contrast to more available “convenience covariates” requiring little or no fieldwork. Uncritical use of convenience covariates for modeling, akin to “convenience sampling” in ecological studies, poses hidden problems that can unknowingly bias or inhibit knowledge gain (Anderson, 2001).
Ecological scaling of covariates to match scales of different life-history characteristics also is frequently ignored during model development and selection (McGarigal et al., 2016), and can result in models that are ecologically misleading or irrelevant (Mateo Sánchez et al., 2014). Explicit rationale for how covariates of energy acquisition (e.g., nutrition) and energy loss (e.g., human disturbance, predation, climatic stressors) are considered in modeling often is unstated or secondary to statistical paradigms that conventionally govern model development and selection (e.g., Burnham and Anderson, 2002; Hooten and Hobbs, 2015).
Finally, the level of spatial and temporal replication used to develop and evaluate models dictates an explicit inference space, yet often is undefined or not quantified for mapping (Yates et al., 2018). Most landscape-use models are based on case studies (one place, one time) with limited inference (Johnson, 2002; Yates et al., 2018). A growing suite of large but disparate datasets on ungulate landscape use, however, provides new opportunities for model replication across multiple study areas and time periods as a form of ecological meta-analysis (Gurevitch et al., 2018). Opportunistic use of disparate data sources poses new challenges for modeling because data are not collected under an a priori, unified sampling design, and problematic sources of variability must be addressed (Gurevitch et al., 2018).
These are daunting challenges for species with broad distributions, seasonal habitat needs, and diverse behavioral and evolutionary strategies, combined with complex management issues. These challenges necessitate modeling landscape use with a variety of covariates that address multiple spatiotemporal scales, ecological processes, and land uses (Apollonio et al., 2017). Many ungulates are migratory (Bolger et al., 2008; Sawyer et al., 2009; Barker et al., 2019), for example, requiring models that address seasonal ranges and multiple land ownerships. Their relatively large body size and dietary preferences also have led to human–wildlife conflicts (Gill, 1992; Sekhar, 1998; Ward et al., 2004), warranting models that accurately predict occurrence. Ungulates often are hunted, and harvest regulations depend on knowledge of landscape use (Krausman and Bleich, 2013). Many ungulates also respond negatively to anthropogenic disturbance by increasing movement rates (Spitz et al., 2019), shifting distributions (Sawyer et al., 2006), or even abandoning ranges (Hebblewhite, 2008). Models of landscape use that include covariates of human disturbance thus are essential to land-use planning (Proffitt et al., 2010; Dwinnell et al., 2019).
In response, we describe a landscape-use modeling framework for ungulates and other species that explicitly considers ecological and management criteria to frame and guide model design, development, and utility. We do not delve into statistical methods of modeling landscape use, which have been reviewed extensively (Boyce and McDonald, 1999; Boyce et al., 2002; Gillies et al., 2006; Lele et al., 2013; Muff et al., 2019). Instead, we provide ecological and management justification for modeling criteria, describe benefits of their consideration, and highlight examples of their use for ungulates, with obvious relevance to other vertebrate taxa.
Ecological and Management Criteria for Modeling Landscape Use
We identified seven criteria from the literature as tenets for modeling landscape use by ungulates (Table 1 and Figure 1). Similar criteria have been broadly described in the literature on sampling and experimental design for ecological and management studies, but have not been synthesized for modeling applications for ungulates or other vertebrates in one comprehensive source. We considered this need and integrated research and management considerations in each criterion.
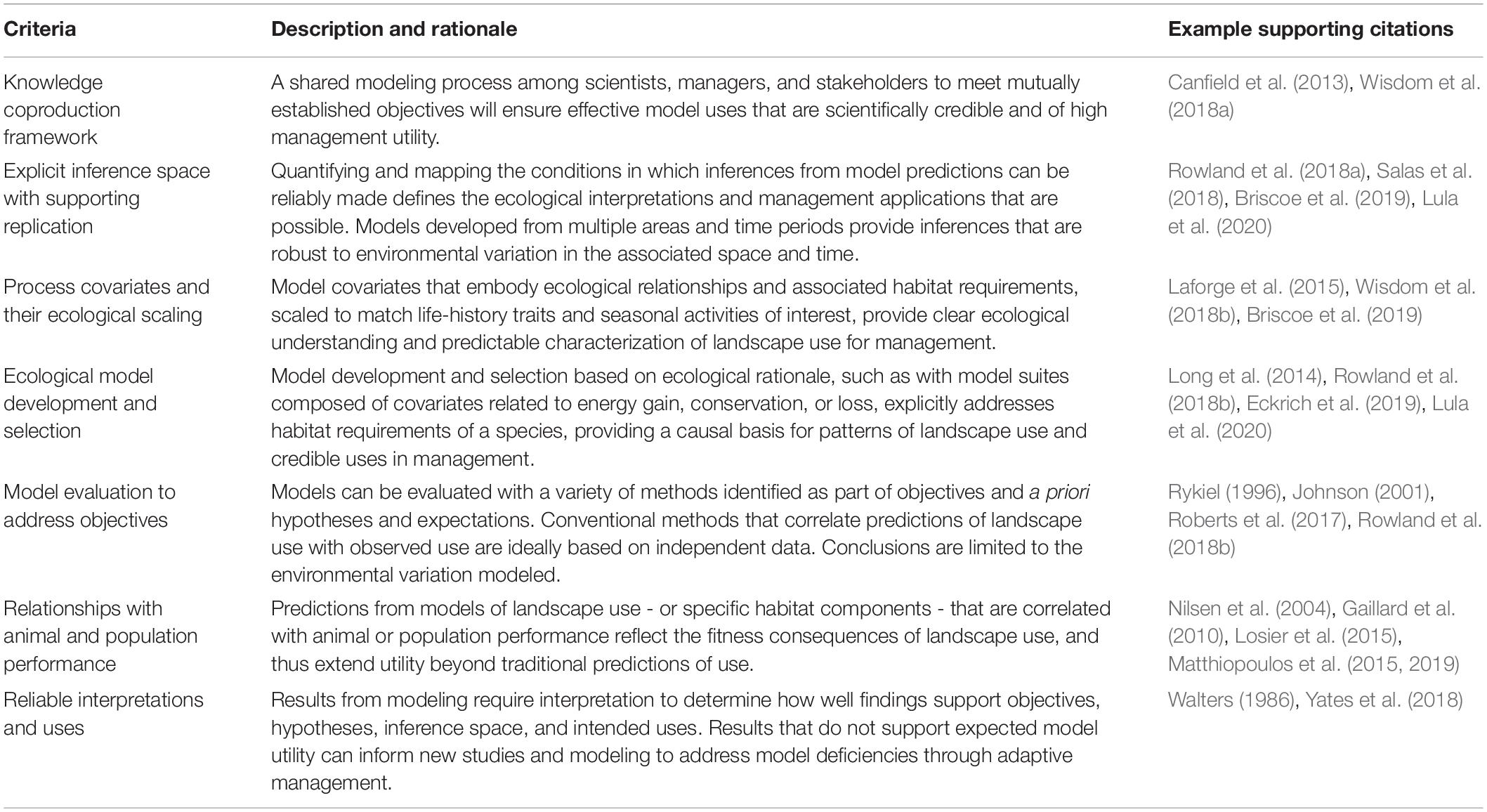
Table 1. Criteria to frame and guide the design, development, utility of landscape-use models for ungulates, and associated description and rationale with example supporting citations.
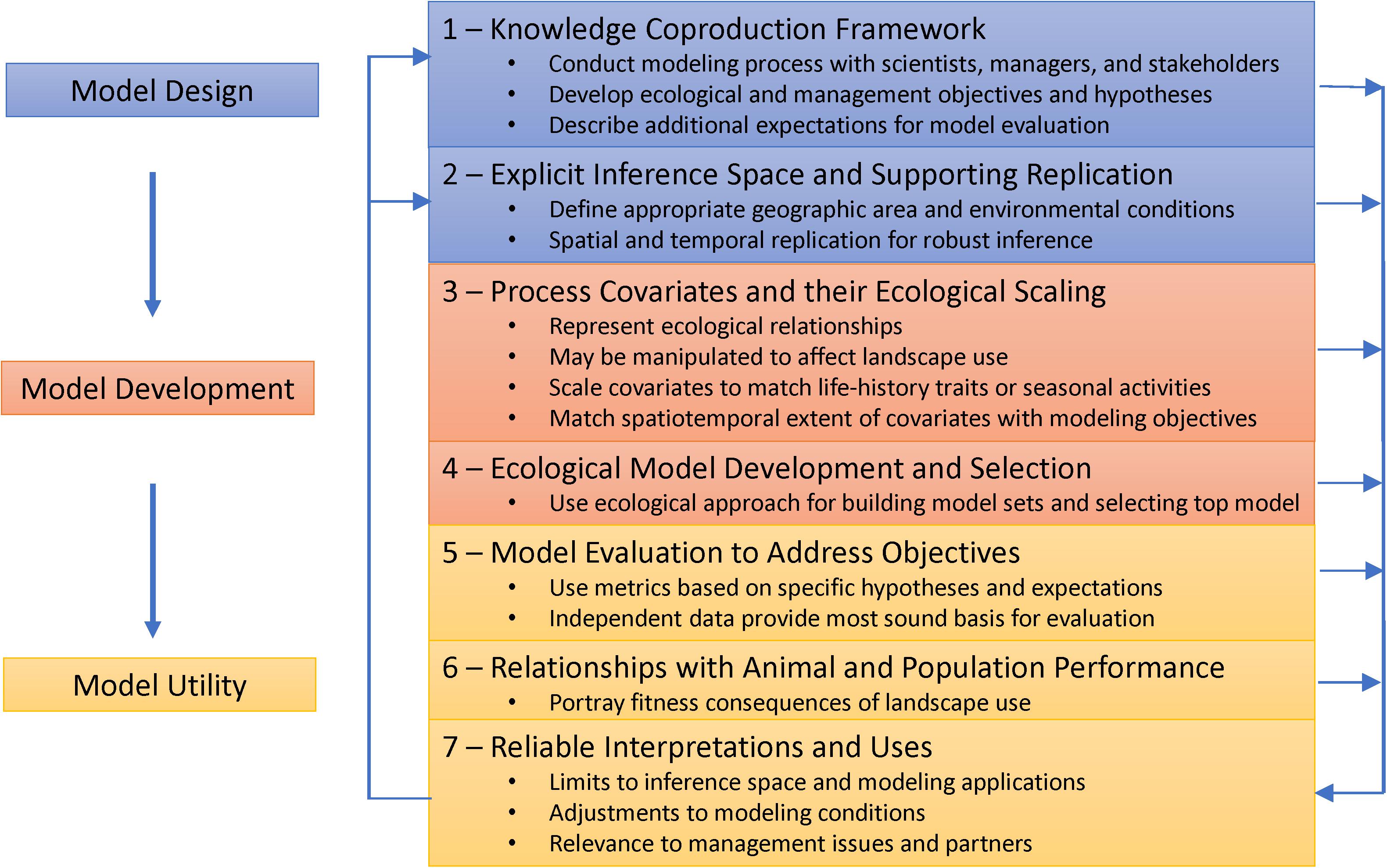
Figure 1. Modeling phases (left boxes) and associated design criteria (right boxes) that outline an ecological and management process (downward arrows) for model design, development, evaluation, and interpretation of landscape use models for ungulates. The primary feedback loop (upward arrows) is generated from interpretation of results from model development, selection, and evaluation, and relationships to performance to determine how well findings support objectives, hypotheses, inference space, and intended uses. Results that do not support model expectations can guide additional studies and modeling to address model deficiencies, using concepts of adaptive management (Walters, 1986).
We present the criteria as a linear process for modeling in three phases: (1) design, (2) development; and (3) utility (Figure 1). Model design includes all aspects of planning: establishing and using effective partnerships among scientists, managers, and stakeholders to define model purpose, objectives, and ecological hypotheses and expectations for testing (see the section “Knowledge Coproduction Framework”); and identifying the targeted geographic area, environmental conditions, and populations for inference (see the section “Explicit Inference Space with Supporting Replication”). Model development builds on design to address how covariates representing ecological relationships and habitat requirements are identified and scaled to match targeted life-history and seasonal-use activities (see the section “Process Covariates and Their Ecological Scaling”); and using these covariates in ecologically plausible, competing models that reflect life-history traits, habitat requirements, and evolutionary behavior (see the section “Ecological Model Development and Selection”). Model utility encompasses the final, critical phase that provides measures and interpretations of the ecological and management worth of a selected model. Criteria also include the evaluation of a model in relation to a priori hypotheses and expectations (see the section “Model Evaluation to Address Objectives”); identifying whether model predictions or components further relate to demographic performance of targeted populations (see the section “Relationships With Animal and Population Performance”); and the interpretations and uses of a model that provide essential context and direction for how results can advance ecological understanding and benefit management (see the section “Reliable Interpretations and Uses”).
The relevance of each criterion depends on objectives and associated hypotheses. Models developed for ecological understanding but not for explicit management applications, for example, do not require a large investment in knowledge coproduction with managers. Other models may have narrowly defined objectives for management, such as characterizing ungulate distributions by land ownership, without resources or need to expand inference space, formally evaluate the model, or relate predictions to demography. In that context, we consider the criteria as aspirational standards by which modelers of landscape use could evaluate the ecological and management worth of their work, not edicts to blindly follow. We offer the criteria to complement the well-developed and justified focus on statistically based modeling, to improve ecological understanding and management utility.
Knowledge Coproduction Framework
Knowledge coproduction is defined as the “process of producing usable, or actionable, science through collaboration between scientists and those that use science to make policy and management decisions” (Meadow et al., 2015). Coproduction is highly relevant to landscape-use modeling for meeting the dual purpose of improving ecological understanding of a species’ habitat requirements and using this knowledge to meet societal needs. Models created with this dual purpose are most effective when designed and implemented collaboratively among scientists, managers, and stakeholders (Irvine et al., 2009; Canfield et al., 2013; Wisdom et al., 2018b).
For ungulates, these collaborations are particularly important because complex management issues, potential for property damage and human conflicts, and important recreational values require scientists and managers to work closely with a diversity of stakeholders. Coproduction is a natural extension of the types of interactions among scientists, managers, and stakeholders on issues of harvest management, which are relevant to a high percentage of ungulates that are hunted (Heffelfinger et al., 2013; Krausman and Bleich, 2013).
Examples of effective coproduction modeling in ecology are growing (e.g., Davies and White, 2012; Reyers et al., 2015; Nel et al., 2016) but not currently standard practice (Addison et al., 2013; Meadow et al., 2015). The benefits of formal adoption of coproduction methods for modeling include: (1) better articulation of knowledge gaps impeding ecological understanding and management; (2) improved communication of modeling results, inference space, and proper application; and (3) increased acceptance and use of models in management. Coproduction as applied to landscape-use modeling involves collaboration in all phases of the scientific process: defining objectives and inference space, describing ecological and management hypotheses for testing, identifying analysis scales and potential covariates, developing and implementing appropriate modeling methods, interpreting results, and careful inference (Table 1 and Figure 1).
While deceptively simple, coproduction can be daunting and time-consuming (Voinov et al., 2016), requiring more resources than traditional research. However, by establishing a formal process of collaboration among scientists, managers, and stakeholders, ownership in the process and successful outcomes become more likely compared to traditional approaches led by scientists (Voinov et al., 2016; Merkle et al., 2019). Coproduction requires both researchers and managers to make a genuine, long-term commitment to thoughtfully consider each other’s viewpoints and objectives, and to work collaboratively for mutual benefit.
Examples
A long-term collaboration to model elk (Cervus canadensis) habitat used principles of knowledge coproduction from development (Canfield et al., 2013) to application (Ranglack et al., 2017; DeVoe et al., 2019; Lowrey et al., 2020). Following a series of stakeholder and legal challenges to elk habitat management in western Montana, wildlife scientists and managers from the U.S. Forest Service and Montana Fish, Wildlife and Parks formed a committee to discuss and compile recommendations for collective improvement of elk habitat. The committee spent 3 years sharing knowledge, discussing uncertainties in current science, and identifying modeling needed to improve elk habitat management in the region.
The resulting recommendations (Canfield et al., 2013) prompted a series of research and modeling projects to address management needs of both agencies. Ranglack et al. (2017) evaluated attributes of security areas used by nine elk populations and developed new management definitions for optimal canopy cover and distance from motorized routes that substantially improved elk security. Definitions are now used to inform management of vegetation and motorized access during archery and rifle elk seasons on public lands.
DeVoe et al. (2019) built on these definitions to evaluate the nutritional consequences of archery hunting on elk. Results included recommendations to integrate management of forage and security (i.e., canopy cover and motorized routes) to improve habitat and encourage elk to remain on public lands throughout the rifle season, the traditional period and method of population management. Lowrey et al. (2020) addressed an additional knowledge gap regarding elk responses to dramatic changes in forest structure and elk security brought about by severe infestations of mountain pine beetle (Dendroctonus ponderosae). Authors used their findings to define management thresholds for canopy cover and distance from motorized routes to maintain elk use during hunting seasons in areas experiencing severe conifer mortality from beetle infestations. Results are being used to inform management of elk security on public forests impacted by beetle-kill.
Explicit Inference Space With Supporting Replication
Clearly defining an inference space during model design ensures that sampling replication is appropriate for inference across the selected spatial and temporal extents (Hobbs, 2003). We define “inference space,” also referred to as the “target system” (Yates et al., 2018), as the geographic area and associated environmental conditions and their temporal variability to which interpretations, conclusions, and predictions of landscape use or other ecological relationships are made (also see Garton et al., 2001; Morrison, 2001, 2012). In spite of the importance of a clearly defined inference space, we noted few cases in the ecological literature where a target system was purposely identified and quantified for model applications (Yates et al., 2018). And yet, every model has its predictive limits, which have been well-defined for statistical inference (Chatfield, 1995). Understanding inferential limits from ecological and management perspectives reduces misapplication of the model (Garton et al., 2001).
A challenge in addressing inference space is the reliance on models of landscape use developed and evaluated with data from one study area as a case study lacking replication. By definition, case studies are based on local conditions, which limit knowledge gain and management utility to those specific conditions. As the number of study areas or similar units of spatial replication increases, the inference space for model predictions expands to an area larger than the replicates themselves (Yates et al., 2018). Inference space then represents conditions across the larger landscape, encompassing like variation among study areas. Broad scales of inference are possible, such as to habitats within an entire ecoregion or biome for widely distributed ungulate species [e.g., Acevedo et al., 2011 for landscape-use modeling of wild boar (Sus scrofa), red deer (Cervus elaphus), Iberian wild goat (Capra pyrenaica), and roe deer (Capreolus capreolus) in Spain; and Kanagaraj et al., 2019 for the sub-ungulate Asian elephant (Elephas maximus) in India and Nepal].
Inference space for modeling landscape use can be done in two ways, a priori or a posteriori. In the first, an explicit target system is identified when developing modeling objectives and hypotheses for testing. A formal sampling design is developed, with focus on the spatial and temporal replication needed for desired inference. If objectives call for development and validation of a landscape-use model across an extensive area, such as an ecoregion [e.g., Salas et al., 2018 for Marco Polo Sheep (Ovis ammon polii) across 4.1 million ha in eastern Tajikistan], the geographic extent of sample replicates needed for modeling will be substantially larger than those for a local population [e.g., Lula et al., 2020 for bighorn sheep (Ovis canadensis) restoration in the Madison Range of southwest Montana, United States]. The number and type of replicates needed will largely depend on the environmental variation considered in modeling (Figure 2), and classic methods of sampling design can be used to allocate units of replication to address the environmental variation in the defined inference space (Krebs, 1989).
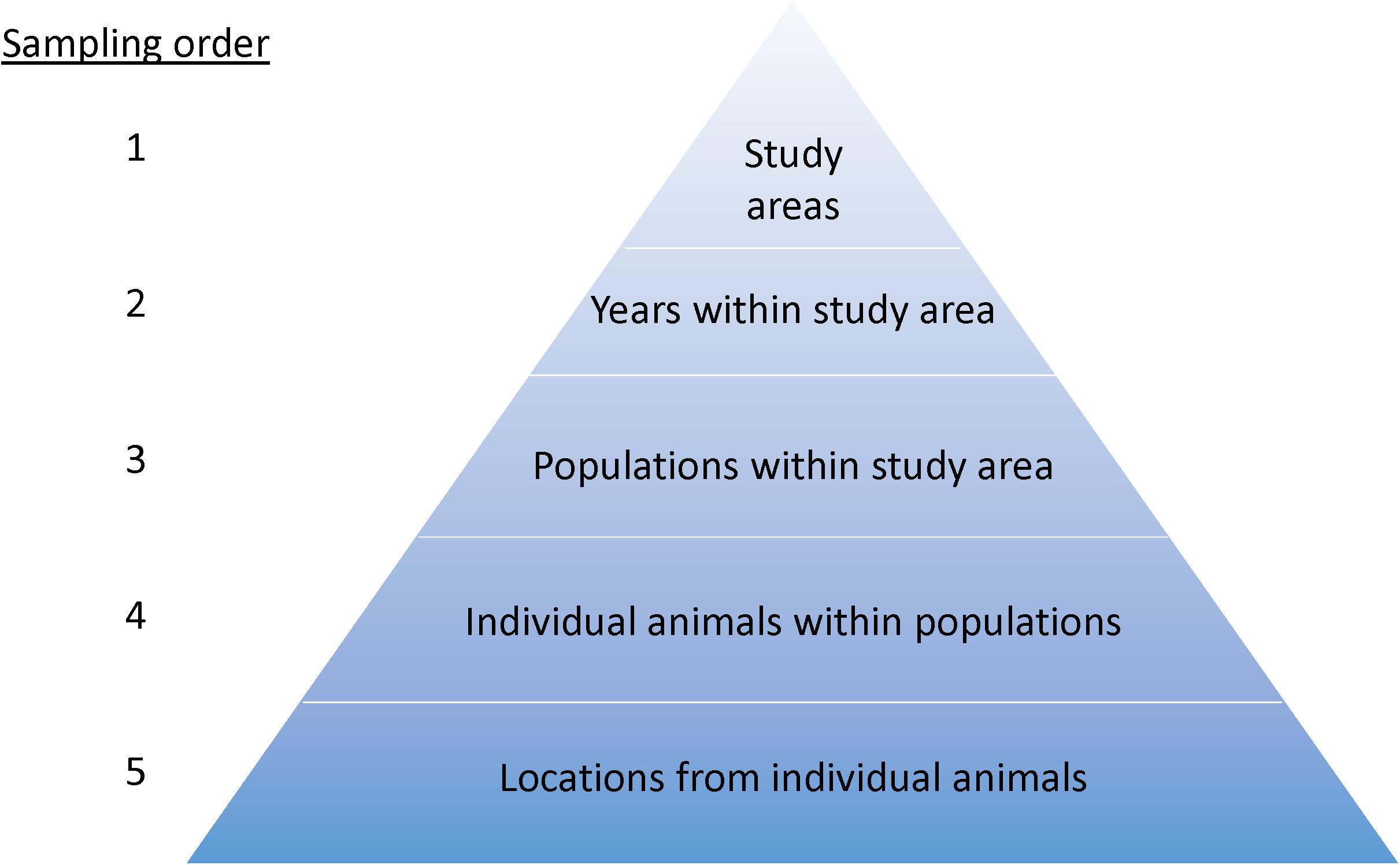
Figure 2. Sampling and inference hierarchy for landscape-use modeling. Model training and testing, including estimates of precision, should replicate this order. When making inference at one level (e.g., a population), the next level in the hierarchy is the appropriate unit of replication (e.g., individual animals) for estimating precision. Estimates of precision for a single study area should consider blocking at the next available level where replication occurs. For example, if within one study area (1), multiple years are studied (2), then model evaluation should use years (2), populations (3), or individual animals (4) for blocking, depending on the available sample size at each level. Estimates for a broader landscape require a sample (n > 1) of study areas.
Explicitly defining the inference space and units of replication before data collection and modeling under an a priori design can help avoid the “Modifiable Areal Unit Problem” (MAUP; Openshaw, 1984), in which the size and distribution of sampling (replication) units, and their aggregation, can lead to different results depending on how the units are combined for analysis (Jelinski and Wu, 1996; Bissonette, 2017). Study areas or other spatial replicates (polygons) are modifiable and setting arbitrary study extents based on mapping boundaries (e.g., ownership) can dramatically affect response variables. Thus, establishing spatial extents of these areas a priori to encompass variation of interest helps avoid the MAUP (Bissonette, 2017).
The a posteriori approach involves modeling landscape use opportunistically with available data and adapts inference space and related objectives and hypotheses to observed conditions [e.g., Rowland et al., 2018a for elk; Coe et al., 2018 and Eckrich et al., 2019 for mule deer (Odocoileus hemionus)]. Given the lack of a unified, consistent sampling design, this “default” inference space can be more limited than that under a priori allocation of replicates to match a desired target system. Despite these challenges, the emergence of large data sets on animal locations has provided new opportunities for expanding inference space. However, it is not always clear how well landscape-use models can be developed opportunistically a posteriori, with data from multiple replicate areas, as a form of spatially explicit meta-analysis (Gurevitch et al., 2018). Telemetry data collected across different areas and times, for example, often differ in number of animals collared, relocation frequency, fix success, and reasons for animal collaring (Rowland et al., 2018b). Moreover, few telemetry-based studies of landscape use explain how their capture protocols result in a set of collared animals that are representative of the population to which inferences will be made (Garton et al., 2001).
Differences in resource availability across time and space may also result in different patterns of landscape use, resulting in spurious conclusions (Holbrook et al., 2019), given the plethora of problems introduced by the MAUP (Bissonette, 2017). In addition, ungulate patterns of landscape use are typically density-dependent (McLoughlin et al., 2006; Godvik et al., 2009). Replication across increasingly large spatial extents and longer time periods may capture a variety of ungulate patterns of landscape use not otherwise revealed by accounting for functional responses in relation to population density (van Beest et al., 2012). At a minimum, basic knowledge of whether population density varies across replicates is needed to interpret potential variation in landscape use (McLoughlin et al., 2010). Otherwise, deriving a global model of landscape use, such as by averaging model coefficients among replicates, may result in an “average” model that represents none of the underlying areas (e.g., Russell et al., 2015). The same challenge exists for successfully building global models where management of ungulate harvest and predator complexes varies markedly across the inference space.
Despite these challenges, a combination of disparate sources of data used as replicates, both published and unpublished, has been integrated successfully in ecological meta-analysis (Gurevitch et al., 2018). Opportunistic syntheses of these data require clear ecological hypotheses to direct modeling efforts (Morrison, 2001, 2012). Opportunistic analyses further rely on integration of spatially and temporally replicated data that accounts for inherent data imprecision, and that constrains limits of inference accordingly (Johnson, 1999, 2002; Yates et al., 2018), rather than routinely rejecting the approach for lack of a unified, a priori design (Romesburg, 1981; Hurlbert, 1984).
We emphasize use of study areas as spatial replicates because they represent the most obvious and traditional form of replication that is geographically extensive and thus can encompass appropriate extents or targeted populations (Figure 2)—in contrast to more traditional use of animals as sample units and their locations as subsamples in case studies. A noteworthy example of spatial replication was the habitat-use model for mule deer developed by Coe et al. (2018), in which nine study areas were used as replicates for inference across three million ha of the sagebrush biome (Figure 3). Sampling designs, however, are evolving, and different forms of spatial and temporal replication can be used to support the associated inference space (Figure 2). New technologies have the potential for systematic sampling over broad areas of a species range, such as with geographically extensive camera traps arrays (Ahumada et al., 2011; Menkham et al., 2019) or broad-scale aerial sampling (Nielsen et al., 2010; Bristow et al., 2019). Species distribution modeling is a specific example of landscape-use modeling designed to address broad-scale patterns of occurrence and covariates accounting for those patterns, often without classical use of replicated study areas, but instead systematically sampling across the entire inference space (e.g., Zimmermann et al., 2010; Jarnevich et al., 2015).
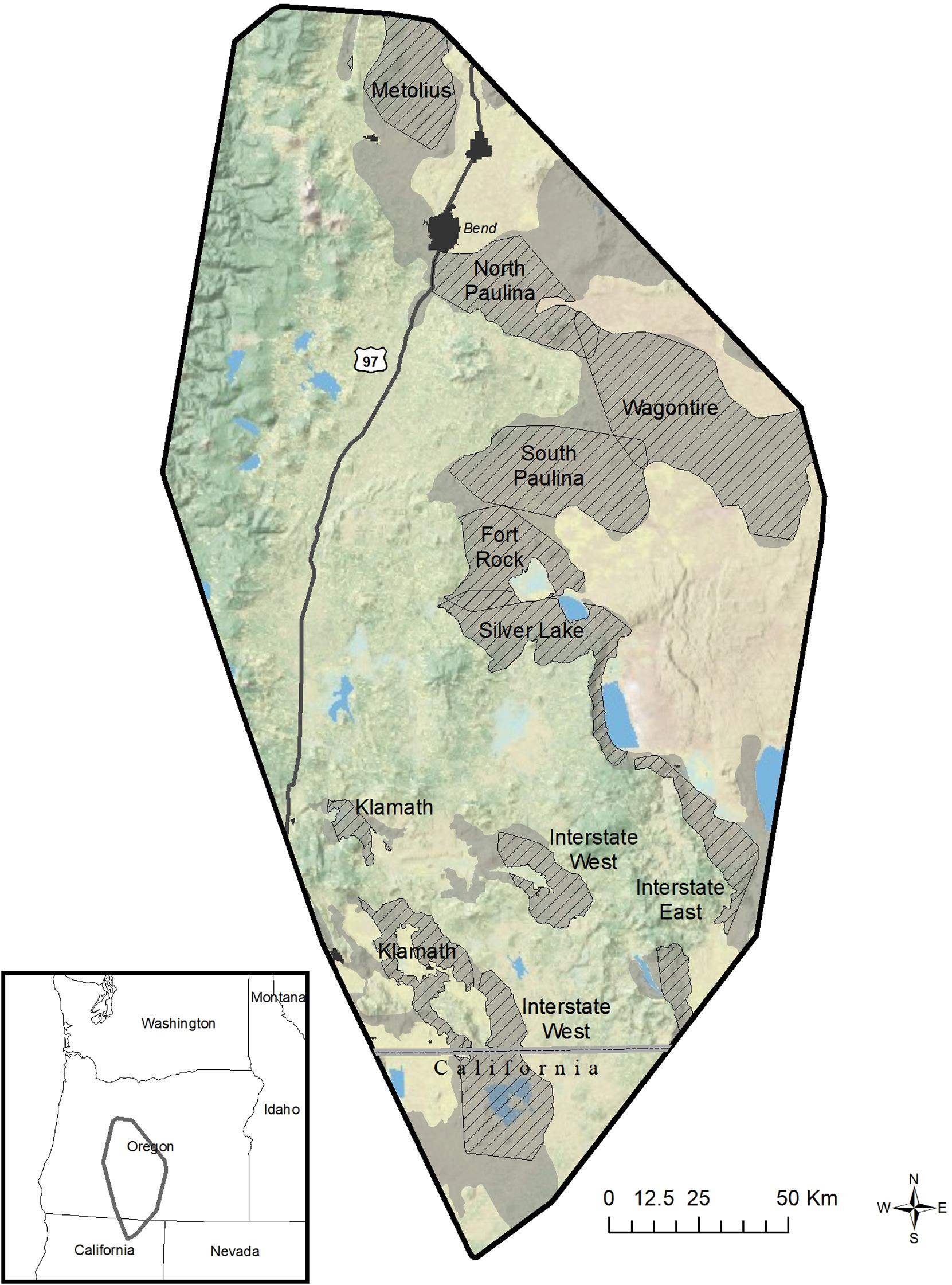
Figure 3. Nine study areas (hatched areas) were used by Coe et al. (2018) as spatial replicates to model habitat use by 452 mule deer during winter over 8 years (2005–2012) in southcentral Oregon and northern California, United States. As context, study areas were embedded within a minimum convex polygon of >3-million ha that defined the year-round range of telemetered animals (black boundary). Study areas were used as units of replication in developing and validating global models of habitat use for mule deer at three spatiotemporal scales: population, home range, and foraging. Competing models of habitat use at each scale were first developed and ranked for empirical support by study area. Rankings were then summed across study areas to identify the global model with highest overall support, and model coefficients averaged among study areas to derive a global model. Validation of the global model used locations from 95 mule deer withheld from model development in eight study areas, and results were reported by study area.
Consideration of an explicit inference space deserves much greater attention as part of landscape-use modeling, and is essential to robust predictions. Regardless of approach, the key steps are to identify the targeted area and conditions for desired inference, define the spatial and temporal replicates needed to support the range of conditions, and account for a variety of sources of sampling and environmental variation to enable robust predictions.
Examples
Modeling with an explicit inference space is particularly useful when evaluating future or potential conditions, such as projected effects of climate change (Salas et al., 2018), wildfire (Proffitt et al., 2019), or other disturbances on ungulate landscape use (Riggs et al., 2015). One exemplary study that defined an explicit inference space used resource selection functions (RSFs) to evaluate restoration potential for bighorn sheep in southwest Montana, United States (Figure 4) (Lula et al., 2020). Inference space was identified a priori as the historic sheep distribution across the Madison Range, with current sheep populations occupying the southwestern and northeastern portions (Figure 4). Model design included sampling in both populations, with the southwestern population used for model development, internal validation, and predictions and the northeastern population for external model validation. The authors carefully assessed the range of environmental variation within modeling areas, compared conditions to the targeted prediction area (i.e., the Madison Range), and limited predictions to areas with conditions similar to those used for model development and validation. Accordingly, the northern portion of the Madison Range was omitted from predictions because conditions differed from model development and validation areas, leading to potential for invalid predictions beyond the appropriate inference space.
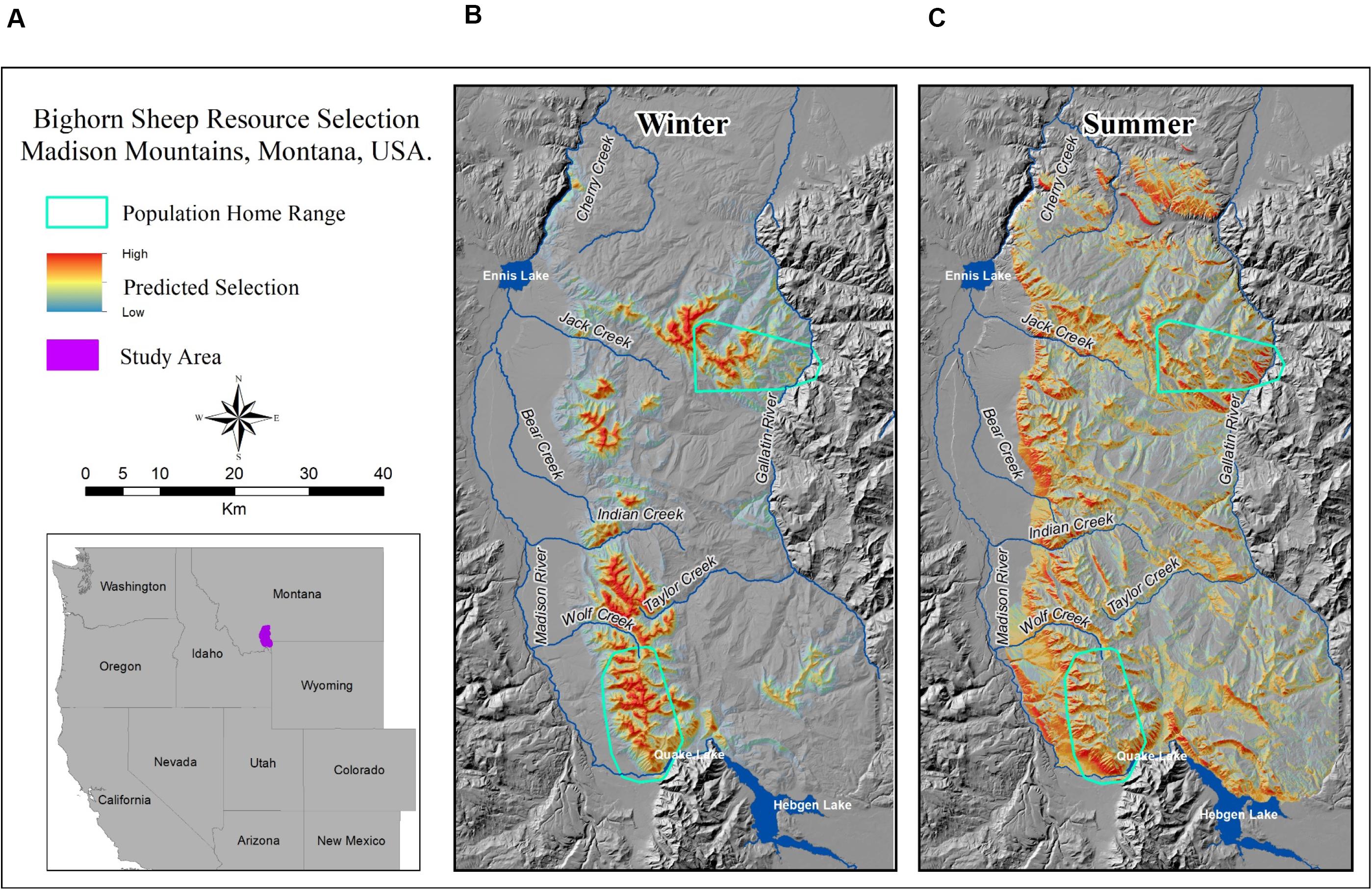
Figure 4. In southwest Montana, United States (A), predictions of bighorn sheep landscape use (B,C) from a resource selection function (RSF) were applied to an explicit inference space of historical but currently unoccupied bighorn sheep range to estimate potential winter and summer use (B,C, from Lula et al., 2020). Cool colors depict areas of low predicted selection, and warm colors areas of high predicted selection. The RSF was developed with telemetry data and validated with estimates of population abundance from established populations in nearby areas within the same historical range (summer and winter population home ranges, B,C).
Process Covariates and Their Ecological Scaling
Model covariates that embody ecological processes and the associated habitat requirements of a species have been referred to as process covariates (Nielsen et al., 2010). Examples in ungulate landscape-use modeling include nutrition (Rowland et al., 2018a, b, 2000; DeVoe et al., 2019); predation (Kittle et al., 2008; Ciuti et al., 2012); human disturbance (Jiang et al., 2008; Bonnot et al., 2013); land-use change (Acevedo et al., 2011; Schuette et al., 2016); climate change (Ciach and Pęksa, 2018; Salas et al., 2018); and weather (van Beest et al., 2012; Long et al., 2014). Many process covariates can also be managed to affect landscape use (Wisdom et al., 2018a, b). Because process covariates are drivers of ecological patterns, their emphasis in modeling is more likely to result in predictions of landscape use that are robust to spatiotemporal variation in the environment (Gaillard et al., 2010).
Other model covariates may provide important environmental context to enhance model predictions but may not represent explicit ecological processes, nor can they be actively managed. Examples of these contextual covariates include vegetation type, topography, soils, and geology. The specific role of contextual covariates in modeling can be unclear without empirical support. For example, ungulate selection for cool, mesic aspects during hot summers could indicate selection for areas of higher forage productivity, increased thermal relief, or both (e.g., Beck et al., 2013). Without knowledge of the specific role that aspect plays, understanding its relation to other covariates and processes is difficult.
Some covariates may also be used as surrogates for process covariates. Remotely sensed greenness metrics (Meier and Brown, 2014), for example, often are used as surrogates for ungulate nutrition (Pettorelli et al., 2007, 2011; Borowik et al., 2013). How these metrics index nutrition (e.g., forage quantity, quality) or animal productivity (e.g., pregnancy rates, first-year survival), however, is often unstated and implicitly assumed (Villamuelas et al., 2016). As with contextual covariates, the worth of a surrogate covariate relies on its documented relationship with a process covariate (Nielsen et al., 2010; Gautam et al., 2019).
Rationale for the types of covariates considered in landscape-use modeling—process, contextual, and surrogate—requires consideration of how such covariates are scaled (Wiens, 1989; McGarigal et al., 2016). Covariate scaling is the process of defining the spatial and temporal grain and extent (area or time) over which a covariate is derived, quantified, mapped, and used in model development and selection (Wheatley and Johnson, 2009; McGarigal et al., 2016). A related concept is that of the minimum mapping unit, which is the “minimum dimension of an element that can be displayed and analyzed” (Corsi et al., 2000, p. 410) and should be explicitly recognized when interpreting model results. Unfortunately, inconsistent use of scale-related terms permeates the ecological literature (Wheatley and Johnson, 2009). McGarigal et al. (2016) provide a comprehensive assessment of spatial and temporal scaling of covariates.
The scale used to derive spatial covariates can dramatically affect predicted landscape use by ungulates (e.g., Laforge et al., 2015, 2016), but often is not reported (McGarigal et al., 2016). One example of scale in this context is greenness metrics such as Normalized Difference Vegetation Index, currently delivered for 16-day periods at 250-m resolution (Meier and Brown, 2014) and often used as a surrogate to represent ungulate nutrition in modeling landscape use (e.g., see review by Villamuelas et al., 2016). An implicit assumption is that this spatial (i.e., 250 m) and temporal (16 days) grain matches that of the foraging behavior and associated landscape use by the species. For ungulates that forage in very small patches, however, a much smaller grain (e.g., 10 m) may be more appropriate, owing to the fine scale at which the ungulate selects foraging sites. The challenge lies with deriving a nutrition covariate that can be mapped accurately at this grain (Morris et al., 2016) but not exceed the accuracy of associated animal location data (Garton et al., 2001).
Evaluating each covariate at multiple scales during model development and selection is essential to identify optimal patterns of landscape use which otherwise can be missed (Mateo Sánchez et al., 2014). Although ecologists have recognized the need for multi-scale habitat models for decades, implementation of such models has been uncommon (McGarigal et al., 2016). The range in scales evaluated is based on ecological rationale and a priori knowledge about the covariate in relation to life-history traits and species activities being modeled (Wheatley and Johnson, 2009) or determined empirically post hoc (McGarigal et al., 2016). Different scales are customized for each spatial covariate based on this rationale and then formally evaluated for empirical support (see the section “Model Development and Selection”).
Importantly, defining and evaluating a range of scales for each covariate is different than hierarchical “levels” of landscape analysis (Mayor et al., 2009; Wheatley and Johnson, 2009), such as first, second, and third orders of selection (Johnson, 1980). Spatial orders of selection are now commonly incorporated in hierarchical modeling of landscape use (McGarigal et al., 2016) at the home range, population, study area, or species range (e.g., DeCesare et al., 2012). We do not address hierarchical modeling because of its common use, whereas covariate scaling to match ecological and management considerations of a species continues to be addressed superficially or not at all (McGarigal et al., 2016).
Examples
Rowland et al. (2018b) used a suite of process covariates to develop and validate elk nutrition and habitat-use models, each containing covariates representing sources of energy acquisition and loss that could be managed (Figure 5) (Wisdom et al., 2018a). The nutrition model addressed energy gain through overstory canopy cover, deemed a process covariate because it affects light penetration to the forest floor that directly influences biomass and quality of ungulate forage (e.g., Jenkins and Starkey, 1993; Peek et al., 2001, 2002; Cook et al., 2016, 2018), and because it can be managed through silvicultural prescriptions (Wisdom et al., 2018b). The response variable of the nutrition model, dietary digestible energy, was then used as a covariate in the habitat-use model that included a process covariate representing energy loss - distance to roads open to public motorized use (Figure 5) (Rowland et al., 2018a). Distance to roads served as an actively managed process covariate because public motorized routes are conduits for human activity that elk consistently avoid (e.g., Rowland et al., 2000; Ciuti et al., 2012; Ranglack et al., 2017). This example demonstrates the ecological underpinnings of the process covariates selected for modeling, and their manipulation through active management to affect desired landscape use.
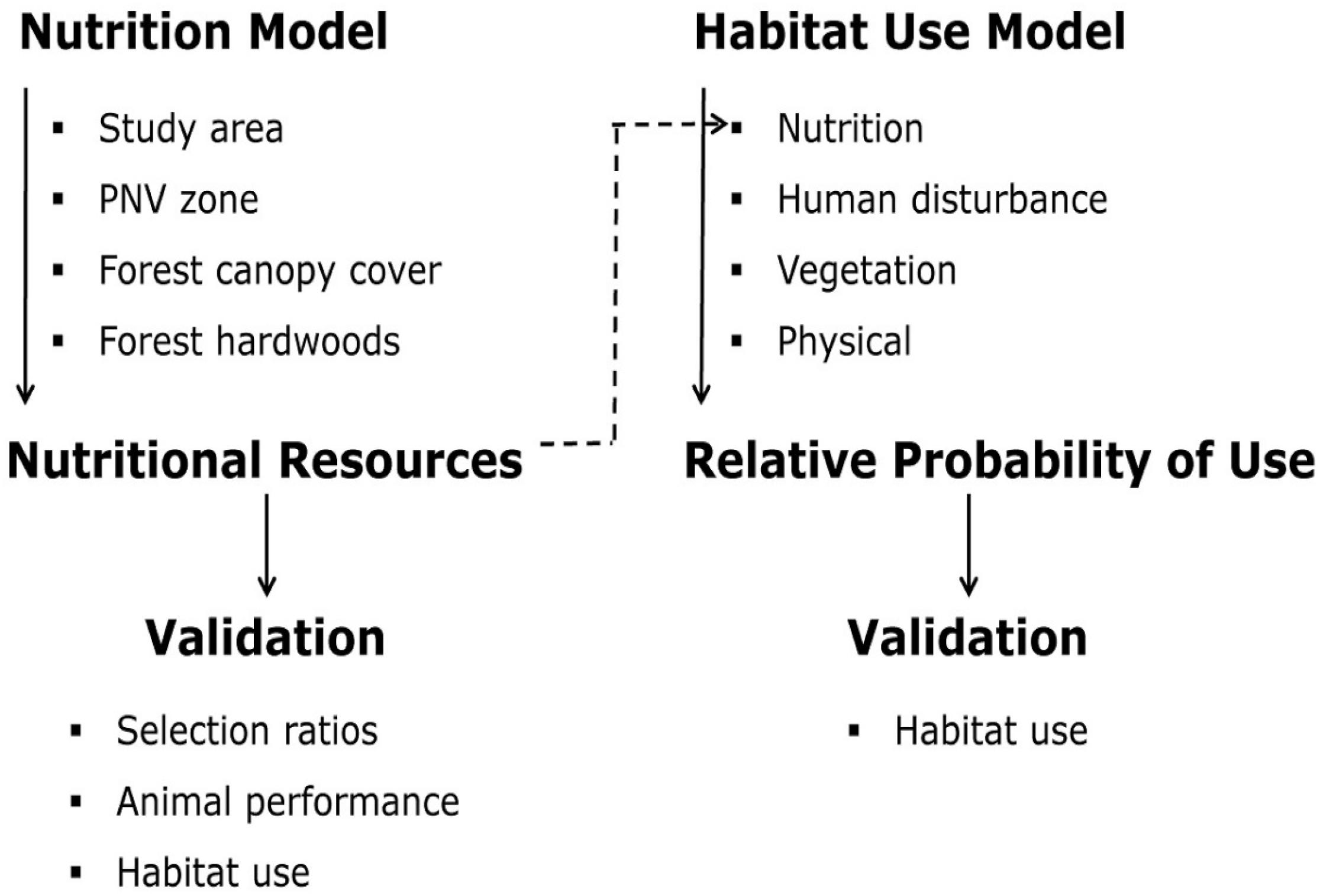
Figure 5. Conceptual framework of nutrition and habitat-use models developed and validated for elk in western Oregon and western Washington, United States (from Wisdom et al., 2018a). Categories of covariates considered during model development and types of data used for validation are shown below each model or type of validation. Covariates in the nutrition model included study area and potential natural vegetation (PNV) zone as contextual covariates, and percent overhead canopy cover (forest canopy cover) and percent composition of hardwoods (forest hardwoods) as process covariates to predict dietary digestible energy (nutritional resources). Nutrition model predictions were most strongly influenced by canopy cover. Predictions of the habitat-use model were influenced by process covariates of nutrition (dietary digestible energy), human disturbance (distance to roads open to public motorized use), and vegetation (distance to cover-forage edges), and the contextual covariate percent slope (physical category). The two models were designed as complementary applications: the nutrition model identified the spatial arrangement and quality of nutritional resources, and the habitat-use model quantified the probability that areas of higher nutrition would be used by elk, given the influence of all covariates that best predicted spatial distribution.
Ecological scaling of covariates was demonstrated by Laforge et al. (2015, 2016) in their landscape-use model for white-tailed deer (Odocoileus virginianus). They considered eight covariates in model selection, each derived at six grain sizes (Laforge et al., 2015), with grain defined as the area around used and available telemetry points. Deer responded uniquely to covariates at different grains. The slope of the functional response, measured as proportion of habitat used versus available, in relation to grain size for each covariate was strikingly different, indicating that deer response to covariates was scale-dependent. Had modeling proceeded with a single grain for all covariates, patterns of landscape use likely would have been substantially different—with the possibility that use associated with some covariates would have been undetected or substantially diluted (Laforge et al., 2015, 2016).
Ecological Model Development and Selection
Given the widespread availability of large datasets on animal locations and broad-scale spatial data, statistical models of landscape use may be complex, and in some cases their complexity may become the focus of the analysis. Contemporary models of landscape use thus often emphasize statistical methods and rote application of model selection criteria (e.g., Burnham and Anderson, 2002). A strong ecological framework for model formulation and selection will ensure that modeling is process-based and explicitly addresses the species life history, habitat relationships, and hypotheses of ecological and management interest.
Building an ecological framework for landscape-use modeling involves several steps. The first is developing ecologically plausible competing models or a list of potential covariates that align with the species’ life-history traits, habitat requirements, and evolutionary behavior (see the section “Process Covariates and Their Ecological Scaling”). In contrast to considering all possible combinations of covariates, recent approaches for developing sets of plausible competing models emphasize organizing covariates into model suites, such as nutrition, predation, and human disturbance (Figure 5) to address specific hypotheses (Franklin et al., 2000; DeVoe et al., 2015; Lowrey et al., 2017; Rowland et al., 2018b). Suites of covariates often are organized according to their roles as sources of energy acquisition (e.g., nutrition covariates or nutrition surrogates), energy loss (e.g., human disturbance, land use, or predation covariates), or energy conservation (e.g., cover or topographic covariates) per modeling concepts of Long et al. (2014) for ungulates. Some covariates may address more than one role, such as slope to indicate areas of higher nutrition and energy conservation (i.e., flatter slopes may have deeper soils that support higher forage biomass, but also are associated with decreased movement costs).
Organizing covariates into model suites that represent specific, often competing hypotheses helps simplify otherwise large and complex competing sets of models and fosters identification of the covariates best supported by the data within a model suite. This approach also allows for important process covariates to advance to later stages of model selection based on ecological rationale for their importance rather than solely on statistical criteria (Lula et al., 2020). Additionally, this approach allows different spatial grains or functional forms of covariates to be evaluated, such that only the most supported form of each is advanced in model selection (Franklin et al., 2000; Laforge et al., 2015; Eckrich et al., 2019; Lowrey et al., 2020). We suggest a tiered or similar approach (e.g., see Franklin et al., 2000; Lowrey et al., 2020) of organizing model suites that represent ecological processes or hypotheses to prioritize inclusion of ecologically meaningful and/or manageable covariates in the final model.
Second, the process of ranking and identifying a final model with highest empirical support from the data has a well-developed history based on information-theoretic (IT) methods (Burnham and Anderson, 2002). Nearly all models of landscape use, as cited here, have used Akaike’s Information Criterion (AIC), its variants (Burnham and Anderson, 2002), or other Bayesian IT statistics (Hooten and Hobbs, 2015) to guide model selection. When applied to all possible combinations of covariates or a model built solely on IT-based metrics (e.g., stepwise model building), the final landscape-use model may not be ecologically relevant or useful for management because a comparative ranking of models may overshadow ecological rationale and need for interpretable results (Arnold, 2010). However, when applied to a carefully developed process for modeling that represents ecological hypotheses of interest, statistical criteria such as AIC can help identify a plausible model with high management utility.
Examples
Lowrey et al. (2017) used an ecological framework and tiered model approach to develop and select models for mountain goat resource selection in the southwestern Greater Yellowstone Area, United States. The authors considered 12 covariates representing potential habitat attributes influencing mountain goat resource selection based on published research. To address specific hypotheses and simplify an otherwise potentially complex model list, covariates were grouped into four model suites representing terrain, vegetation, heat load, and snow. A tiered modeling and IT approach to model selection guided the progression from relatively simple univariate models focused on identifying the most explanatory functional form and grain of each covariate, to multivariate models that compared covariates within model suites. The final model suite contained supported covariates from each of the four model selection suites, from which the authors selected a final model of mountain goat resource selection. The authors’ approach placed covariates into categories representing specific ecological hypotheses, which strengthens interpretation of model outcomes and provides a more robust modeling foundation.
Rowland et al. (2018b) also used a strong ecological framework to develop and select models for elk habitat use in western Oregon and Washington, United States. To create a regional landscape-use model across sites differing in resource availability, the authors placed covariates to predict elk habitat use in one of four categories to represent specific hypotheses about their relative influence and associated ecological processes (Figure 5). As in the prior example, covariates were chosen from each suite for advancement and combined to create a set of candidate models for evaluation and identify a final global model that addressed specific ecological hypotheses and their use in management.
Model Evaluation to Address Objectives
Model evaluation can be defined as an examination of how a model performs in relation to a priori expectations (Rykiel, 1996; Johnson, 2001). The word “validation” is often used in the ecological modeling literature, but this term is misleading in that no model can be truly validated but only invalidated through repeated testing in space and time under various conditions (Holling, 1978; Walters, 1986). Moreover, the term validation implies a more absolute outcome than does exploring the value of a model; model evaluation thus has been recommended as a more appropriate term (Johnson, 2001).
Conceptually and in practice, model evaluation can occur in many ways (Rykiel, 1996; Morrison et al., 2006). Identifying the most appropriate method depends on objectives, targeted inference space (see the section “Explicit Inference Space with Supporting Replication”), study design and analysis methods, and available data. Assessing the accuracy and precision of model predictions to new areas is the most common objective for evaluating ungulate models of landscape use (Boyce et al., 2002; Morrison et al., 2006).
Evaluation often is narrowly focused on assessing model predictions, such as relative probability of use, versus a set of observed data to determine their correlation. Evaluation generally relies on some form of cross-validation (Hijmans, 2012; Hjorth, 2017), which separates the original dataset into estimation (aka training) versus test data (Figure 6) (Hjorth, 2017). Estimation data are used to develop predictions for the test data and evaluate the strength of their relationship. From a formal statistical view, “validation data” are used to evaluate the model multiple times throughout model training and help refine model hyperparameters, whereas “testing data” are the gold standard and used only after model “training” (Figure 6) (Hobbs and Hooten, 2015).
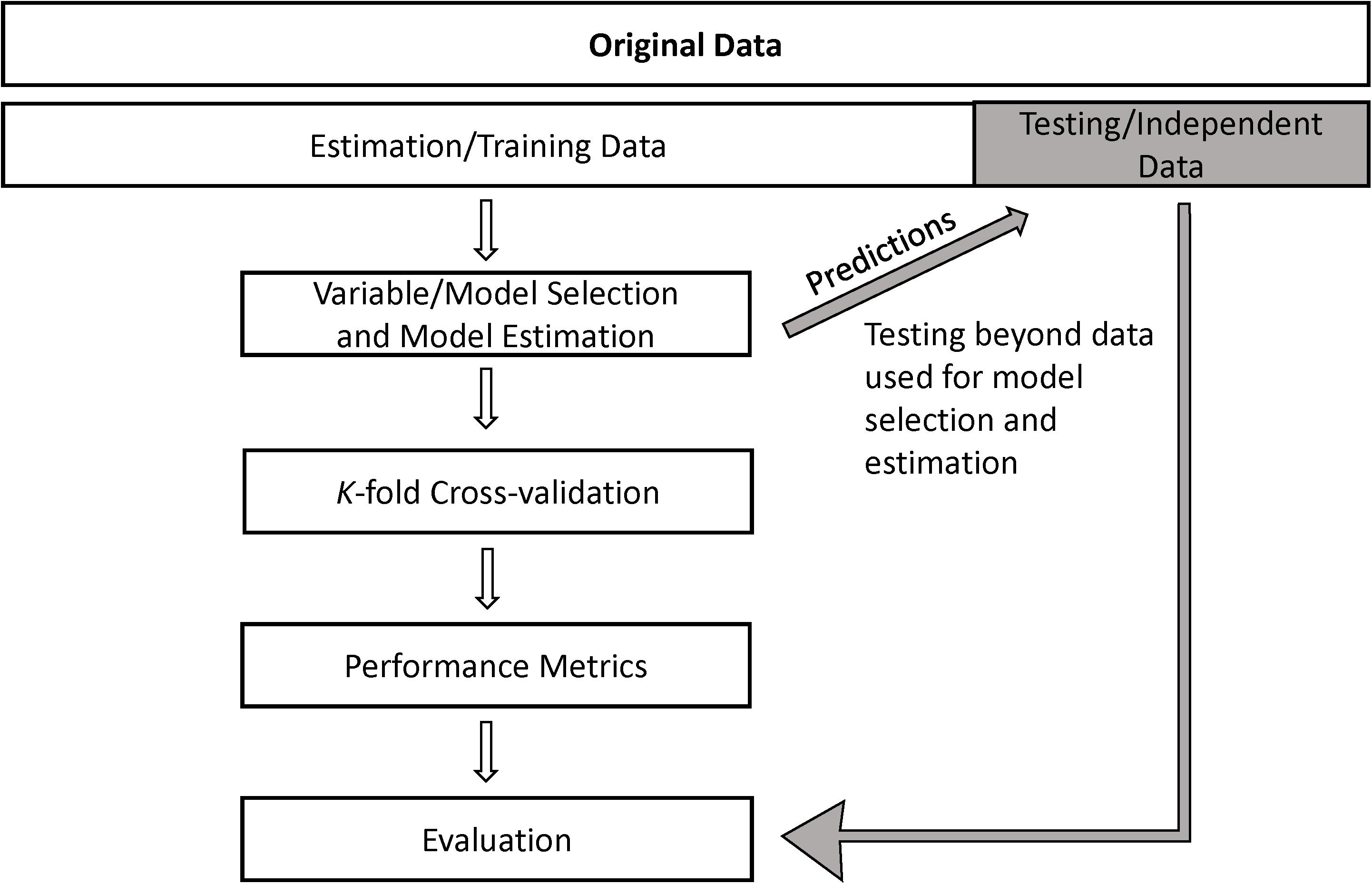
Figure 6. The original (full) data set is split into training (estimation) and testing sets, prior to variable and model selection and parameter estimation. Predictions from the model fit to the training data are then made for the testing data. Evaluation of the correspondence between predictions and testing data is combined with internal evaluation of the training data using k-fold cross-validation. In computer science and engineering disciplines like machine learning, k-fold cross-validation can lead to model refinements (Broms et al., 2016), which can then be re-tested using cross-validation. However, predictions should be made to the holdout testing data only after a final model has been reached, and not during the model selection or refinement process.
Models of landscape use have traditionally evaluated model predictions versus observed data in two ways. The first establishes training and test data from a common dataset (Fielding and Bell, 1997). The second evaluates model predictions with data independent of those used to develop the model. The first type, typically based on cross-validation, is commonly used to evaluate predictions of RSFs and habitat-use models (e.g., Beck et al., 2006; Laforge et al., 2015; Lowrey et al., 2017; Plante et al., 2017). Original data are typically split into k subsets after variable and model selection, and how the specific k subsets or holdout data are chosen can substantially affect results (Roberts et al., 2017). Random assignment of data to the k subsets in cross-validation will always overestimate model performance because evaluation is based on variability at the level of k used to assign the subsets (Figures 2, 6; e.g., study areas, animals; Gude et al., 2009). Whenever possible, assignment of data to the k subsets should be based on the primary sampling unit for inference (e.g., study area, Figure 2) to mimic the original data collection process and reduce bias in predictions (Roberts et al., 2017). This “blocking” (Roberts et al., 2017) to choose subsets is optimal because observations within blocks are not independent.
The second, preferred type of model evaluation uses holdout data that are spatially and temporally independent of those used for estimation (Roberts et al., 2017), and is especially valuable when making predictions to different environmental conditions. However, if data are only available from one study area or sample sizes are small (e.g., number of animals), all available data may be needed for model estimation and evaluation, resulting in optimistic performance (Roberts et al., 2017).
Use of training data to evaluate model predictions, be it from k-fold cross-validation or other methods, may be unreliable because the “model prediction parameters were selected with the estimation and test data sets, and thus might be biased” (Roberts et al., 2017). Thus, the holdout method is recommended for more insightful and realistic model evaluation. If the objective is to evaluate predictions for other areas and management scenarios, variable and model selection uncertainty must also be recognized (Hjorth, 2017). Differences in distributions of covariates between model estimation and evaluation data may point to model deficiencies or restrictions as to where or when the model can be applied with confidence.
Regardless of approach, the model evaluation process, including how to split the estimation and test datasets, should be developed before model selection and estimation (Figure 6) (Hjorth, 2017; Roberts et al., 2017). Evaluation can then proceed with a variety of metrics like r2, receiver operating characteristic (ROC) curves, area under the curve (AUC), classification tables, kappa, or Bayesian hierarchical approaches (Chatfield, 1995; Rykiel, 1996; Fielding and Bell, 1997; Allouche et al., 2006; Morrison et al., 2006; Peterson et al., 2008; Matthiopoulos et al., 2015; Broms et al., 2016). Other evaluation metrics may be based on the types of knowledge gained in relation to ecological hypotheses or expectations (Bunnell, 1989), or based on simulating future conditions under changing disturbance or climate regimes (e.g., Riggs et al., 2015; White et al., 2018).
For any evaluation that examines agreement between predicted and observed landscape use, the essential consideration is the degree of independence between data used for model development versus evaluation. Optimistic results generated by nearly all forms of k-fold cross-validation reflect their limitations for broad inference. Modeling approaches that hold out multiple datasets that are spatially and temporally independent of those used to develop a model lead to more robust evaluation and are increasingly common (see the section “Explicit Inference Space with Supporting Replication”).
Examples
Guan et al. (2015) developed landscape-use models for takin (Budorcas taxicolor) in the northern MinShan Mountains of Sichuan Province, China. The models were based on GPS coordinates of takin and sign of takin presence within sampled plots within a 38,000 km2 study region, and final predictions of takin presence were based on model averaging using AIC weights. The authors evaluated their model by comparing predictions to an independent data set of takin locations collected 2005–2010 across a network of 21 nature reserves in Sichuan Province. This example illustrates the most desirable form of model evaluation, i.e., using data independent and beyond the range of those used to develop the model, rather than subsetting model development data for evaluation with k-fold cross-validation or ROC-based classification matrices. Coe et al. (2018) likewise demonstrated a robust form of model evaluation, using data from 95 mule deer withheld from model development in eight study areas, with results reported in relation to varying environmental conditions across study areas (Figure 3).
Relationships With Animal and Population Performance
Predicting spatial and temporal distributions or probability of use is the primary objective of most ungulate landscape-use models; however, linking these predictions to measures of animal performance can identify the biological consequences of landscape use and extend model utility for conservation and management (Aldridge and Boyce, 2008; Gaillard et al., 2010). Here, we define performance as measures of survival and reproduction that reflect fitness, the expected contribution of individuals to future generations (Franklin and Morrissey, 2017) and often quantified as lifetime reproductive success (LRS). Indeed, the underlying motivation behind many habitat models is that “organisms have a reason for being where we find them” (Matthiopoulos et al., 2015, p. 414). Quantifying and understanding these connections, referred to as Habitat–Performance Relationships (HPR; Gaillard et al., 2010), can inform ungulate conservation in multiple ways.
If HPR have been quantified in an area, management can target specific habitat attributes and potentially improve performance, such as higher juvenile survival. Moreover, if a model covariate correlates well with some performance index and is a key driver of landscape-use model predictions (e.g., large standardized coefficient), we can assume that areas of highest predicted use are associated with greater fitness, and target management to influence ungulate distributions and benefit the population. However, HPR can change over time, for example with shifting densities of predators or alternate prey, and thus should be considered dynamic. These measures will be increasingly important in the future by enhancing our understanding of how populations respond to environmental change (Losier et al., 2015; Matthiopoulos et al., 2019).
Performance components linked to ungulate habitat can be either direct measures of reproduction and survival (e.g., fecundity, pregnancy status; Proffitt et al., 2016; Allen et al., 2017; Cook et al., 2018) or indirect metrics such as body fat (Proffitt et al., 2016; Cook et al., 2018; Merems et al., 2020). Outputs from ungulate landscape-use models have been related to performance measures in multiple ways (Table 2). A seminal study is the work of McLoughlin et al. (2006), in which LRS of red deer on the Isle of Rum, Scotland was associated with selection of vegetation communities (see “Examples” section below). A similar analysis by McLoughlin et al. (2007) for female roe deer in France demonstrated that LRS was greater for individuals that incorporated specific habitat components such as meadows and thickets in their home ranges.
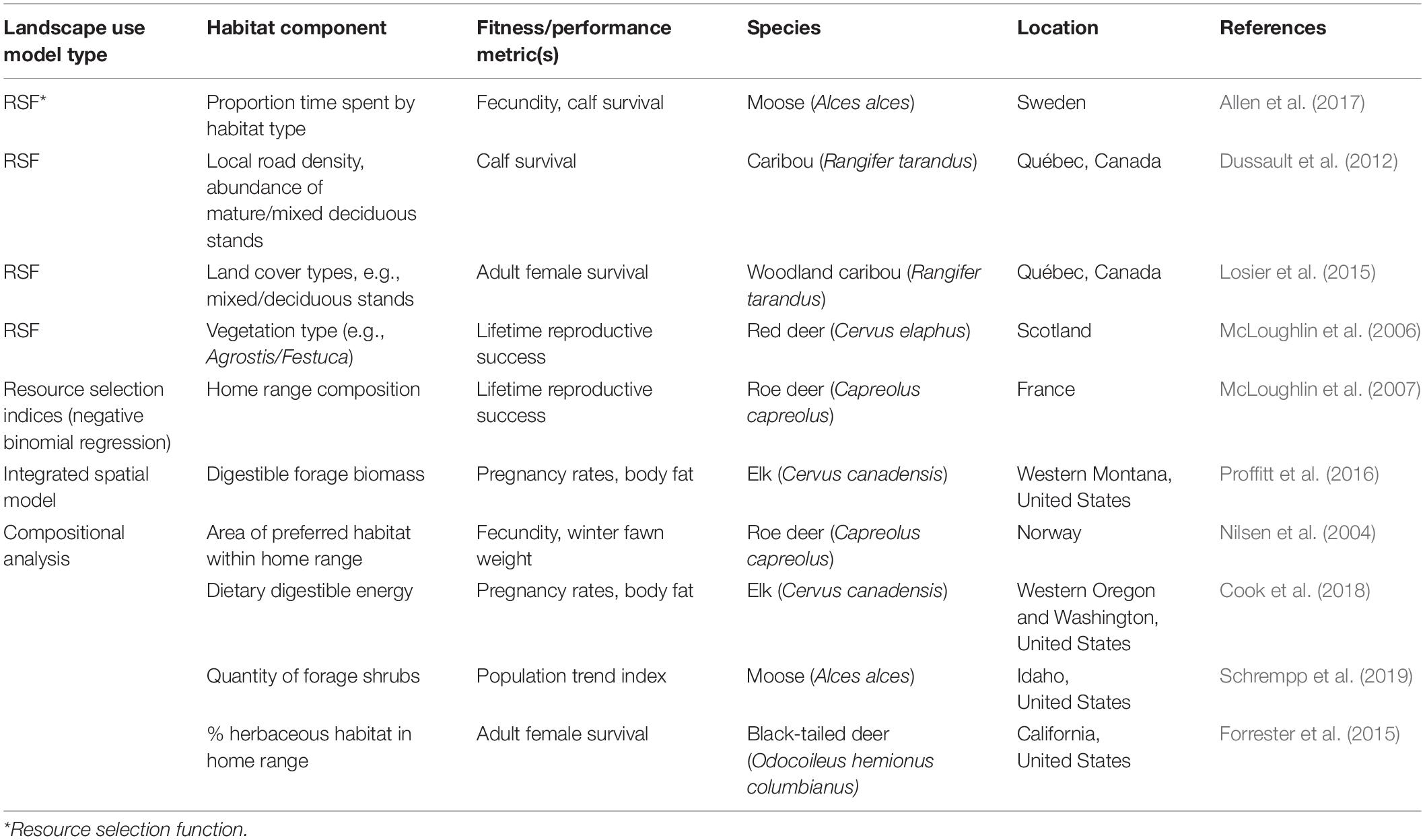
Table 2. Examples of ungulate landscape-use models or habitat components that demonstrated relationships between landscape use and animal or population performance.
Because landscape-use models are niche-based and multivariate, and generally predict distributions or habitat use, we cannot directly use their outputs (e.g., probability of use) to predict fitness (Gaillard et al., 2010). We can, however, explore how specific habitat features, whether as model covariates or independent of a formal modeling framework, relate to performance. For example, Nilsen et al. (2004) documented preference for woodland habitat by roe deer on winter ranges in Norway, and then evaluated covariation of the area in woodlands with litter size. Similarly, Schrempp et al. (2019) found that quantity of “forage shrubs” was correlated with population trends of moose in northern Idaho, United States.
One category of habitat-performance relationships especially relevant to ungulates is that between quantity and juxtaposition of “security” habitat to survival or other fitness indices during hunting seasons, both for hunted and non-hunted population segments. In Norway, Lone et al. (2015) found increased survival of male European red deer that moved into dense cover at the start of the hunting season. Caribou vulnerability to harvest in Canada was greater when animals were closer to infrastructure such as roads or hunting camps, and when in open or flat terrain (Plante et al., 2017). Spitz et al. (2019) documented lower body fat of adult female elk entering winter for individuals that markedly avoided roads during hunting seasons.
Considerations in relating performance to landscape use by ungulates and its interpretation include scale, density dependence, and predator-prey dynamics. The scale of model development is especially important in choosing appropriate performance metrics. At smaller geographic and temporal scales, individual energy gain or performance may be suitable measures, whereas at larger scales, population demography or persistence of local populations are more appropriate (Gaillard et al., 2010). Habitat-performance relationships that appear robust in one system may not be translatable to another if ungulate densities or resources vary widely between systems (Gaillard et al., 2000, 2010; McLoughlin et al., 2006, 2010; Matthiopoulos et al., 2015). Similarly, predator densities and distributions can strongly affect ungulate landscape use (e.g., Frair et al., 2005; Oates et al., 2019). Thus understanding these dynamics and quantifying their relative impact on ungulate habitat use and performance is requisite to reliably link habitat to performance.
Despite the potential value of establishing HPR in landscape-use models, acquiring sufficient data to do so is a daunting challenge (Garshelis, 2000; Gaillard et al., 2010). And not all landscape-use studies, depending on their objectives and applications, necessarily benefit from these linkages. The primary barrier is the long timespans required to comprehensively document HPR (Clutton-Brock, 1988; McLoughlin et al., 2006, 2007; Gaillard et al., 2010) and the costs of measuring performance – especially capture and handling of many animals. The ultimate measure of fitness, lifetime reproductive output, is seldom quantified for ungulates given their longevity and the difficulty in long-term monitoring of fecundity in individuals (Clutton-Brock, 1988) (but see McLoughlin et al., 2006 below).
Examples
Despite the difficulties in demonstrating habitat-performance linkages, some studies have produced ungulate landscape-use models that clearly establish HPR in a variety of settings. McLoughlin et al. (2006) used a dataset spanning >30 years on the Isle of Rum, Scotland for 270 free-ranging adult female red deer. They developed individual lifetime RSFs, as well as a pooled RSF, and quantified selection of primary vegetation types on the island. They measured LRS as the number of female calves surviving to 1 year for each adult female. Coefficients from the RSFs were used to predict LRS. The authors found that LRS was significantly related to use of Agrostis/Festuca grasslands, but that benefits of these grasslands decreased with increasing density of red deer.
In Canada, Losier et al. (2015) developed RSFs to model probability of occurrence of adult female woodland caribou (Rangifer tarandus caribou), and then used the top-ranked model to estimate probability of winter survival. They demonstrated that the functional responses of caribou in selecting risky habitats, i.e., clear-cuts preferred by moose, resulted in decreased survival due to increased mortality by wolves (Canis lupus) attracted to these habitats. This example highlights the complexities of HPR and the potential role of predators in affecting them.
Proffitt et al. (2016) developed an integrated spatial model to predict nutritional resources and evaluate their effects on elk in two study areas of different nutritional quality in Montana, United States. They found that elk exposed to lower digestible forage biomass, their index of nutritional resources, had lower body fat and pregnancy rates. The authors posited that nutritional limitations may predispose elk to predation, or limit population productivity and growth rate.
Reliable Interpretations and Uses
The final, critical steps in landscape-use modeling are to interpret results for ecological understanding and management uses, address unforeseen shortfalls in meeting objectives, and adjust inferences in relation to unmet objectives. Reliable interpretations and uses depend on successful completion of all three modeling phases: design, development, and utility (Figure 1).
Model design provides the baseline against which results can be interpreted in relation to collaboratively set a priori objectives and hypotheses. Unmet objectives or unsupported hypotheses may require adapting how and where the model is used, and inference space adjusted accordingly. New research may be initiated to augment the original spatial and temporal replication of data collection needed to support the desired inference space, or alternatively, to confirm the appropriateness of local models, each relevant to a smaller inference space. Results from model evaluation can also be used to design new modeling approaches and reformulate objectives (e.g., Walters, 1986; Hooten and Hobbs, 2015).
Model development provides further context for reliable interpretation and uses. For example, modeling ungulate nutrition and landscape use in response to climate change for an alpine ungulate (e.g., White et al., 2018) requires critical assumptions about how nutrition and other covariates are derived and mapped under climate change projections. These assumptions must be clearly articulated as covariates are developed, combined, and selected as plausible models that address climate change factors relevant to the species’ ecology and management. Further, ecological scaling of covariates, such as forage biomass and quality, with sufficient precision is needed to match the spatiotemporal scales of climate-change projections and habitat use. Low precision of model outcomes can muddy interpretation, emphasizing the need for improved empirical estimates of forage covariates and possibly their scaling as prerequisites to meet modeling objectives.
Model utility builds on these two prior phases with careful interpretation of model evaluation results to assess the ecological and management value of a model. How well model predictions are supported by independently observed data, withheld as spatial or temporal replicates for evaluation, often becomes critical to the interpretation of whether the desired inference space is justified or must be adjusted. Similarly, model covariates or predictions may be hypothesized to relate to pregnancy rates, survival, or other measures of animal performance. HPR linkages resulting from modeling can then be reliably interpreted in terms of value of the model as it relates to performance.
Models can ultimately be designed, developed, and applied as part of adaptive management (Walters, 1986; Varley and Boyce, 2006; Apollonio et al., 2017), which continues to provide a useful template that embodies the coproduction process (Figure 1) among scientists, managers, and stakeholders (Voinov et al., 2016), despite mixed application successes (Allen and Gunderson, 2011). Learning and improvement occurs throughout the process via studies and applications codeveloped and implemented incrementally and iteratively over time (Walters, 1986).
Modeling landscape use will continue to grow in scope and practice to benefit ungulate ecology and management with approaches that foster a continual learning process. No single model can ever serve the changing demands of knowledge gain and management applications, but the reliability of model interpretations and uses can be substantially improved with consideration of ecological and management criteria throughout the modeling process (Figure 1).
Conclusion
Models of landscape use have tremendous potential to advance ecological understanding and management of ungulates and other species worldwide. The growing volume of animal location data, accessibility of spatial data at broad scales, and advances in statistical modeling and software allow for the continued refinement and expanded application of landscape-use models. Without a sound ecological and management framework, however, contemporary modeling of landscape use may continue to rely on “convenience sampling,” statistical methods, and case studies lacking the spatial and temporal replication needed for broad inference. Although many of these concepts have been previously described in a variety of publications, we synthesized trends and ideas from the disparate literature to develop a coherent ecological framework that included seven criteria for designing, developing, and applying landscape-use models to advance ecological understanding and effective management of ungulates (Table 1 and Figure 1). We highlighted recent examples that represent successes in this advancement and described future avenues for research to fill gaps in current understanding of ungulate modeling, such as linking landscape use to animal performance.
We view the criteria as aspirational standards, not strict rules to uniformly follow without careful consideration of modeling objectives. Not all landscape-use models will include all criteria, owing to a wide spectrum of modeling objectives and available resources. In that light, we offer our criteria as a complement to the traditional focus on statistically based methods, to help ensure a modeling process that advances ecological understanding and management utility.
Author Contributions
MW developed the initial concepts and criteria for the manuscript and wrote early rough drafts. MW, RN, MR, and KP contributed equally to detailed refinement of the criteria and the intellectual thinking behind the concepts and criteria. All authors helped to write the manuscript, contributed to the article, and approved the submitted version.
Funding
This review was supported by Eagle Environmental, Inc.; Montana Fish, Wildlife, and Parks; and USDA Forest Service Pacific Northwest Research Station.
Conflict of Interest
The authors declare that the research was conducted in the absence of any commercial or financial relationships that could be construed as a potential conflict of interest.
Acknowledgments
We thank Topic Editors V. Bleich, R. Bowyer, J. Gaillard, and P. Krausman for their guidance on this work, particularly P. Krausman for directing the peer review and revision process. We are indebted to J. Gude, B. Lowrey, D. Vales, S. Boutin, and J. Bissonette, whose comments substantially improved the intellectual rigor of concepts presented here. We thank E. Lula for preparing Figure 4, and L. Dick for establishing and managing the automated entry of References.
References
Acevedo, P., Farfán, M. A., Márquez, A. L., Delibes-Mateos, M., Real, R., and Vargas, J. M. (2011). Past, present and future of wild ungulates in relation to changes in land use. Landsc. Ecol. 26, 19–31. doi: 10.1007/s10980-010-9538-2
Addison, P. F., Rumpff, L., Bau, S. S., Carey, J. M., Chee, Y. E., Jarrad, F. C., et al. (2013). Practical solutions for making models indispensable in conservation decision-making. Divers. Distrib. 19, 490–502. doi: 10.1111/ddi.12054
Ahumada, J. A., Silva, C. E., Gajapersad, K., Hallam, C., Hurtado, J., Martin, E., et al. (2011). Community structure and diversity of tropical forest mammals: data from a global camera trap network. Philos. Trans. R. Soc. B Biol. Sci. 366, 2703–2711. doi: 10.1098/rstb.2011.0115
Aldridge, C. L., and Boyce, M. S. (2008). Accounting for fitness: combining survival and selection when assessing wildlife-habitat relationships. Israel J. Ecol. Evol. 54, 389–419. doi: 10.1560/ijee.54.3-4.389
Allen, A. M., Dorey, A., Malmsten, J., Edenius, L., Ericsson, G., and Singh, N. J. (2017). Habitat–performance relationships of a large mammal on a predator-free island dominated by humans. Ecol. Evol. 7, 305–319. doi: 10.1002/ece3.2594
Allen, C. R., and Gunderson, L. H. (2011). Pathology and failure in the design and implementation of adaptive management. J. Environ. Manag. 92, 1379–1384. doi: 10.1016/j.jenvman.2010.10.063
Allouche, O., Tsoar, A., and Kadmon, R. (2006). Assessing the accuracy of species distribution models: prevalence, kappa and the true skill statistic (TSS). J. Appl. Ecol. 43, 1223–1232. doi: 10.1111/j.1365-2664.2006.01214.x
Anderson, D. R. (2001). The need to get the basics right in wildlife field studies. Wildl. Soc. Bull. 29, 1294–1297.
Apollonio, M., Belkin, V. V., Borkowski, J., Borodin, O. I., Borowik, T., Cagnacci, F., et al. (2017). Challenges and science-based implications for modern management and conservation of European ungulate populations. Mamm. Res. 62, 209–217. doi: 10.1007/s13364-017-0321-5
Arnold, T. W. (2010). Uninformative parameters and model selection using Akaike’s Information Criterion. J. Wildl. Manag. 74, 1175–1178. doi: 10.1111/j.1937-2817.2010.tb01236.x
Barker, K. J., Mitchell, M. S., and Proffitt, K. M. (2019). Native forage mediates influence of irrigated agriculture on migratory behavior of Elk. J. Anim. Ecol. 88, 1100–1110. doi: 10.1111/1365-2656.12991
Beck, J. L., Peek, J. M., and Strand, E. K. (2006). Estimates of elk summer range nutritional carrying capacity constrained by probabilities of habitat selection. J. Wildl. Manag. 70, 283–294. doi: 10.2193/0022-541x(2006)70[283:eoesrn]2.0.co;2
Beck, J. L., Smith, K. T., Flinders, J. T., and Clyde, C. L. (2013). Seasonal habitat selection by elk in north central Utah. Western North Am. Nat. 73, 442–456. doi: 10.3398/064.073.0414
Bissonette, J. A. (2017). Avoiding the scale sampling problem: a consilient solution. J. Wildl. Manag. 81, 192–205. doi: 10.1002/jwmg.21187
Bolger, D. T., Newmark, W. D., Morrison, T. A., and Doak, D. F. (2008). The need for integrative approaches to understand and conserve migratory ungulates. Ecol. Lett. 11, 63–77.
Bonnot, N., Morellet, N., Verheyden, H., Cargnelutti, B., Lourtet, B., Klein, F., et al. (2013). Habitat use under predation risk: hunting, roads and human dwellings influence the spatial behaviour of roe deer. Eur. J. Wildl. Res. 59, 185–193. doi: 10.1007/s10344-012-0665-8
Borowik, T., Pettorelli, N., Sönnichsen, L., and Jędrzejewska, B. (2013). Normalized difference vegetation index (NDVI) as a predictor of forage availability for ungulates in forest and field habitats. Eur. J. Wildl. Res. 59, 675–682. doi: 10.1007/s10344-013-0720-0
Boyce, M. S., Johnson, C. J., Merrill, E. H., Nielsen, S. E., Solberg, E. J., and Van Moorter, B. (2016). Can habitat selection predict abundance? J. Anim. Ecol. 85, 11–20. doi: 10.1111/1365-2656.12359
Boyce, M. S., and McDonald, L. L. (1999). Relating populations to habitats using resource selection functions. Trends Ecol. Evol. 14, 268–272. doi: 10.1016/s0169-5347(99)01593-1
Boyce, M. S., Vernier, P. R., Nielsen, S. E., and Schmiegelow, F. K. (2002). Evaluating resource selection functions. Ecol. Model. 157, 281–300. doi: 10.1016/s0304-3800(02)00200-4
Briscoe, N. J., Elith, J., Salguero-Gómez, R., Lahoz-Monfort, J. J., Camac, J. S., Giljohann, K. M., et al. (2019). Forecasting species range dynamics with process-explicit models: matching methods to applications. Ecol. Lett. 22, 1940–1956. doi: 10.1111/ele.13348
Bristow, K. D., Clement, M. J., Crabb, M. L., Harding, L. E., and Rubin, E. S. (2019). Comparison of aerial survey methods for elk in Arizona. Wildl. Soc. Bull. 43, 77–92. doi: 10.1002/wsb.940
Broms, K. M., Hooten, M. B., and Fitzpatrick, R. M. (2016). Model selection and assessment for multi-species occupancy models. Ecol. 97, 1759–1770. doi: 10.1890/15-1471.1
Bunnell, F. L. (1989). Alchemy and Uncertainty: What Good are Models?. Portland, OR: USDA Forest Service.
Burnham, K. P., and Anderson, D. R. (2002). A Practical Information-Theoretic Approach. Model Selection and Multimodel Inference, 2nd Edn. New York: Springer.
Burton, A. C., Neilson, E., Moreira, D., Ladle, A., Steenweg, R., Fisher, J. T., et al. (2015). Wildlife camera trapping: a review and recommendations for linking surveys to ecological processes. J. Appl. Ecol. 52, 675–685. doi: 10.1111/1365-2664.12432
Canfield, J. E., Grove, A., Tomasik, E., Pengeroth, D., and Kujala, Q. (2013). U.S Forest Service and Montana Department of Fish Wildlife and Parks collaborative overview and recommendations for elk habitat management on the Custer, Gallatin, Helena, and Lewis and Clark National Forests. Bozeman, MT: Gallatin National Forest Supervisor’s Office.
Chatfield, C. (1995). Model uncertainty, data mining and statistical inference. J. R. Stat. Soc. Ser. A 158, 419–444.
Chen, J., Chen, J., Liao, A., Cao, X., Chen, L., Chen, X., et al. (2015). Global land cover mapping at 30 m resolution: a POK-based operational approach. ISPRS J. Photogram. Remote Sens. 103, 7–27. doi: 10.1016/j.isprsjprs.2014.09.002
Ciach, M., and Pęksa, Ł (2018). Human-induced environmental changes influence habitat use by an ungulate over the long term. Curr. Zool. 65, 129–137. doi: 10.1093/cz/zoy035
Ciuti, S., Northrup, J. M., Muhly, T. B., Simi, S., Musiani, M., Pitt, J. A., et al. (2012). Effects of humans on behaviour of wildlife exceed those of natural predators in a landscape of fear. PLoS One 7:e50611. doi: 10.1371/journal.pone.0050611
Clutton-Brock, T. H. (1988). Reproductive Success: Studies of Individual Variation in Contrasting Breeding Systems. Chicago, IL: University of Chicago Press.
Coe, P. K., Clark, D. A., Nielson, R. M., Gregory, S. C., Cupples, J. B., Hedrick, M. J., et al. (2018). Multiscale models of habitat use by mule deer in winter. J. Wildl. Manag. 82, 1285–1299. doi: 10.1002/jwmg.21484
Cook, J. G., Cook, R. C., Davis, R. W., and Irwin, L. L. (2016). Nutritional ecology of elk during summer and autumn in the Pacific Northwest. Wildl. Monogr. 195, 1–81. doi: 10.1002/wmon.1020
Cook, J. G., Cook, R. C., Davis, R. W., Rowland, M. M., Nielson, R. M., Wisdom, M. J., et al. (2018). Development and evaluation of a landscape nutrition model for elk in western Oregon and Washington. Wildl. Monogr. 199, 13–30.
Corsi, F., De Leeuw, J., and Skidmore, A. (2000). “Modeling species distribution with GIS,” in Research Techniques in Animal Ecology: Controversies and Consequences, eds L. Boitani and T. K. Fuller (New York: Columbia University Press), 389–434.
Davies, A. L., and White, R. M. (2012). Collaboration in natural resource governance: reconciling stakeholder expectations in deer management in Scotland. J. Environ. Manag. 112, 160–169. doi: 10.1016/j.jenvman.2012.07.032
DeCesare, N. J., Hebblewhite, M., Schmiegelow, F., Hervieux, D., McDermid, G. J., Neufeld, L., et al. (2012). Transcending scale dependence in identifying habitat with resource selection functions. Ecol. Appl. 22, 1068–1083. doi: 10.1890/11-1610.1
DeVoe, J. D., Garrott, R. A., Rotella, J. J., Challender, S., White, P. J., O’Reilly, M., et al. (2015). Summer range occupancy modeling of non-native mountain goats in the greater Yellowstone area. Ecosphere 6, 1–20.
DeVoe, J. D., Proffitt, K. M., Mitchell, M. S., Jourdonnais, C. S., and Barker, K. J. (2019). Elk forage and risk tradeoffs during the fall archery season. J. Wildl. Manag. 83, 801–816. doi: 10.1002/jwmg.21638
Dussault, C., Pinard, V., Ouellet, J.-P., Courtois, R., and Fortin, D. (2012). Avoidance of roads and selection for recent cutovers by threatened caribou: fitness-rewarding or maladaptive behaviour? Proc. R. Soc. B Biol. Sci. 279, 4481–4488. doi: 10.1098/rspb.2012.1700
Dwinnell, S. P., Sawyer, H., Randall, J. E., Beck, J. L., Forbey, J. S., Fralick, G. L., et al. (2019). Where to forage when afraid: does perceived risk impair use of the foodscape? Ecol. Appl. 29:e01972.
Eckrich, C. A., Coe, P. K., Clark, D. A., Nielson, R. M., Lombardi, J., Gregory, S. C., et al. (2019). Summer habitat use of female mule deer in oregon. J. Wildl. Manag. 83, 576–587. doi: 10.1002/jwmg.21806
Fielding, A. H., and Bell, J. F. (1997). A review of methods for the assessment of prediction errors in conservation presence/absence models. Environ. Conserv. 24, 38–49. doi: 10.1017/s0376892997000088
Forrester, T. D., Casady, D. S., and Wittmer, H. U. (2015). Home sweet home: fitness consequences of site familiarity in female black-tailed deer. Behav. Ecol. Sociobiol. 69, 603–612. doi: 10.1007/s00265-014-1871-z
Frair, J. L., Merrill, E. H., Visscher, D. R., Fortin, D., Beyer, H. L., and Morales, J. M. (2005). Scales of movement by elk (Cervus elaphus) in response to heterogeneity in forage resources and predation risk. Landsc. Ecol. 20, 273–287. doi: 10.1007/s10980-005-2075-8
Franklin, A. B., Anderson, D. R., Gutiérrez, R. J., and Burnham, K. P. (2000). Climate, habitat quality, and fitness in northern spotted owl populations in northwestern California. Ecol. Monogr. 70, 539–590. doi: 10.1890/0012-9615(2000)070[0539:chqafi]2.0.co;2
Franklin, O. D., and Morrissey, M. B. (2017). Inference of selection gradients using performance measures as fitness proxies. Methods Ecol. Evol. 8, 663–677. doi: 10.1111/2041-210x.12737
Gaillard, J.-M., Festa-Bianchet, M., Yoccoz, N., Loison, A., and Toigo, C. (2000). Temporal variation in fitness components and population dynamics of large herbivores. Annu. Rev. Ecol. Syst. 31, 367–393. doi: 10.1146/annurev.ecolsys.31.1.367
Gaillard, J.-M., Hebblewhite, M., Loison, A., Fuller, M., Powell, R., Basille, M., et al. (2010). Habitat-performance relationships: finding the right metric at a given spatial scale. Philos. Trans. R. Soc. B Biol. Sci. 365, 2255–2265. doi: 10.1098/rstb.2010.0085
Garshelis, D. L. (2000). “Delusions in habitat evaluation: measuring use, selection, and importance,” in Research Techniques in Animal Ecology: Controversies and Consequences, eds L. Boitani and T. K. Fuller (New York, NY: Columbia University Press), 111–164.
Garton, E. O., Wisdom, M. J., Leban, F. A., and Johnson, B. K. (2001). “Experimental design for radiotelemetry studies,” in Radio Tracking and Animal Populations, eds J. Marzluff and J. Millspaugh (Amsterdam: Elsevier), 15–42. doi: 10.1016/b978-012497781-5/50003-7
Gautam, H., Arulmalar, E., Kulkarni, M. R., and Vidya, T. (2019). NDVI is not reliable as a surrogate of forage abundance for a large herbivore in tropical forest habitat. Biotropica 51, 443–456. doi: 10.1111/btp.12651
Gill, R. (1992). A review of damage by mammals in north temperate forests: 1. Deer For. Int. J. For. Res. 65, 145–169. doi: 10.1093/forestry/65.2.145
Gillies, C. S., Hebblewhite, M., Nielsen, S. E., Krawchuk, M. A., Aldridge, C. L., Frair, J. L., et al. (2006). Application of random effects to the study of resource selection by animals. J. Anim. Ecol. 75, 887–898. doi: 10.1111/j.1365-2656.2006.01106.x
Godvik, I. M. R., Loe, L. E., Vik, J. O., Veiberg, V., Langvatn, R., and Mysterud, A. (2009). Temporal scales, trade-offs, and functional responses in red deer habitat selection. Ecology 90, 699–710. doi: 10.1890/08-0576.1
Guan, T., Wang, F., Li, S., and McShea, W. J. (2015). Nature reserve requirements for landscape-dependent ungulates: the case of endangered takin (Budorcas taxicolor) in Southwestern China. Biol. Conserv. 182, 63–71. doi: 10.1016/j.biocon.2014.11.041
Gude, J. A., Mitchell, M. S., Ausband, D. E., Sime, C. A., and Bangs, E. E. (2009). Internal validation of predictive logistic regression models for decision-making in wildlife management. Wildl. Biol. 15, 352–369. doi: 10.2981/08-057
Gurevitch, J., Koricheva, J., Nakagawa, S., and Stewart, G. (2018). Meta-analysis and the science of research synthesis. Nature 555:175.
Hebblewhite, M. (2008). A literature review of the effects of energy development on ungulates: implications for central and eastern Montana. Wildl. Biol. Faculty Publ. 48, 1–125.
Hebblewhite, M., and Haydon, D. T. (2010). Distinguishing technology from biology: a critical review of the use of GPS telemetry data in ecology. Philos. Trans. R. Soc. B Biol. Sci. 365, 2303–2312. doi: 10.1098/rstb.2010.0087
Heffelfinger, J. R., Geist, V., and Wishart, W. (2013). The role of hunting in North American wildlife conservation. Int. J. Environ. Stud. 70, 399–413. doi: 10.1080/00207233.2013.800383
Hijmans, R. J. (2012). Cross-validation of species distribution models: removing spatial sorting bias and calibration with a null model. Ecology 93, 679–688. doi: 10.1890/11-0826.1
Hjorth, J. U. (2017). Computer Intensive Statistical Methods: Validation, Model Selection, and Bootstrap. Abingdon: Routledge.
Hobbs, N. T. (2003). Challenges and opportunities in integrating ecological knowledge across scales. For. Ecol. Manag. 181, 223–238. doi: 10.1016/S0378-1127(03)00135-X
Hobbs, N. T., and Hooten, M. B. (2015). Bayesian Models: A Statistical Primer for Ecologists. Princeton, NJ: Princeton University Press.
Holbrook, J. D., Olson, L. E., DeCesare, N. J., Hebblewhite, M., Squires, J. R., and Steenweg, R. (2019). Functional responses in habitat selection: clarifying hypotheses and interpretations. Ecol. Appl. 29:e01852.
Holling, C. S. (1978). Adaptive Environmental Assessment and Management. Hoboken, NJ: John Wiley & Sons.
Hooten, M. B., and Hobbs, N. T. (2015). A guide to Bayesian model selection for ecologists. Ecol. Monogr. 85, 3–28. doi: 10.1890/14-0661.1
Horne, J. S., Garton, E. O., Krone, S. M., and Lewis, J. S. (2007). Analyzing animal movements using Brownian bridges. Ecology 88, 2354–2363. doi: 10.1890/06-0957.1
Hurlbert, S. H. (1984). Pseudoreplication and the design of ecological field experiments. Ecol. Monogr. 54, 187–211. doi: 10.2307/1942661
Irvine, R., Fiorini, S., Yearley, S., McLeod, J., Turner, A., Armstrong, H., et al. (2009). Can managers inform models? Integrating local knowledge into models of red deer habitat use. J. Appl. Ecol. 46, 344–352. doi: 10.1111/j.1365-2664.2009.01626.x
Jarnevich, C. S., Stohlgren, T. J., Kumar, S., Morisette, J. T., and Holcombe, T. R. (2015). Caveats for correlative species distribution modeling. Ecol. Inform. 29, 6–15. doi: 10.1016/j.ecoinf.2015.06.007
Jelinski, D. E., and Wu, J. (1996). The modifiable areal unit problem and implications for landscape ecology. Landsc. Ecol. 11, 129–140. doi: 10.1007/BF02447512
Jenkins, K. J., and Starkey, E. E. (1993). Winter forages and diets of elk in old-growth and regenerating coniferous forests in western Washington. Am. Midland Nat. 130, 299–313.
Jiang, G., Zhang, M., and Ma, J. (2008). Habitat use and separation between red deer and roe deer in relation to human disturbance in the Wandashan mountains, northeastern China. Wildl. Biol. 14, 92–100. doi: 10.2981/0909-6396(2008)14[92:huasbr]2.0.co;2
Johnson, D. H. (1980). The comparison of usage and availability measurements for evaluating resource preference. Ecology 61, 65–71. doi: 10.2307/1937156
Johnson, D. H. (1999). The insignificance of statistical significance testing. J. Wildl. Manag. 225, 763–772.
Johnson, D. H. (2001). Validating and Evaluating Models. Modeling in Natural Resource Management. Covelo: Island Press, 105–119.
Johnson, D. H. (2002). The importance of replication in wildlife research. J. Wildl. Manag. 66, 919–932.
Kanagaraj, R., Araujo, M. B., Barman, R., Davidar, P., De, R., Digal, D. K., et al. (2019). Predicting range shifts of Asian elephants under global change. Divers. Distrib. 25, 822–838.
Kittle, A. M., Fryxell, J. M., Desy, G. E., and Hamr, J. (2008). The scale-dependent impact of wolf predation risk on resource selection by three sympatric ungulates. Oecologia 157, 163–175. doi: 10.1007/s00442-008-1051-9
Krausman, P. R., and Bleich, V. C. (2013). Conservation and management of ungulates in North America. Int. J. Environ. Stud. 70, 372–382. doi: 10.1080/00207233.2013.804748
Laforge, M. P., Brook, R. K., van Beest, F. M., Bayne, E. M., and McLoughlin, P. D. (2016). Grain-dependent functional responses in habitat selection. Landsc. Ecol. 31, 855–863. doi: 10.1007/s10980-015-0298-x
Laforge, M. P., Vander Wal, E., Brook, R. K., Bayne, E. M., and McLoughlin, P. D. (2015). Process-focussed, multi-grain resource selection functions. Ecol. Model. 305, 10–21. doi: 10.1016/j.ecolmodel.2015.03.003
Lele, S. R., Merrill, E. H., Keim, J., and Boyce, M. S. (2013). Selection, use, choice and occupancy: clarifying concepts in resource selection studies. J. Anim. Ecol. 82, 1183–1191. doi: 10.1111/1365-2656.12141
Lone, K., Loe, L. E., Meisingset, E. L., Stamnes, I., and Mysterud, A. (2015). An adaptive behavioural response to hunting: surviving male red deer shift habitat at the onset of the hunting season. Anim. Behav. 102, 127–138. doi: 10.1016/j.anbehav.2015.01.012
Long, R. A., Bowyer, R. T., Porter, W. P., Mathewson, P., Monteith, K. L., and Kie, J. G. (2014). Behavior and nutritional condition buffer a large-bodied endotherm against direct and indirect effects of climate. Ecol. Monogr. 84, 513–532. doi: 10.1890/13-1273.1
Losier, C. L., Couturier, S., St-Laurent, M. H., Drapeau, P., Dussault, C., Rudolph, T., et al. (2015). Adjustments in habitat selection to changing availability induce fitness costs for a threatened ungulate. J. Appl. Ecol. 52, 496–504. doi: 10.1111/1365-2664.12400
Lowrey, B., Devoe, J., Proffitt, K. M., and Garrott, R. A. (2020). Hiding without cover? Defining elk security in a beetle-killed forest. J. Wildl. Manag. 84, 138–149. doi: 10.1002/jwmg.21781
Lowrey, B., Garrott, R. A., Miyasaki, H. M., Fralick, G., and Dewey, S. R. (2017). Seasonal resource selection by introduced mountain goats in the southwest greater yellowstone area. Ecosphere 8:e01769. doi: 10.1002/ecs2.1769
Lula, E. S., Lowrey, B., Proffitt, K. M., Litt, A. R., Cunningham, J. A., Butler, C. J., et al. (2020). Is habitat constraining bighorn sheep restoration? A case study. J. Wildl. Manag. 84, 588–600. doi: 10.1002/jwmg.21823
Mateo Sánchez, M. C., Cushman, S. A., and Saura, S. (2014). Scale dependence in habitat selection: the case of the endangered brown bear (Ursus arctos) in the Cantabrian Range (NW Spain). Int. J. Geogr. Inform. Sci. 28, 1531–1546. doi: 10.1080/13658816.2013.776684
Matthiopoulos, J., Fieberg, J., Aarts, G., Beyer, H. L., Morales, J. M., and Haydon, D. T. (2015). Establishing the link between habitat selection and animal population dynamics. Ecol. Monogr. 85, 413–436. doi: 10.1890/14-2244.1
Matthiopoulos, J., Field, C., and MacLeod, R. (2019). Predicting population change from models based on habitat availability and utilization. Proc. R. Soc. B 286:20182911. doi: 10.1098/rspb.2018.2911
Mayor, S. J., Schneider, D. C., Schaefer, J. A., and Mahoney, S. P. (2009). Habitat selection at multiple scales. Ecoscience 16, 238–247.
McGarigal, K., Wan, H. Y., Zeller, K. A., Timm, B. C., and Cushman, S. A. (2016). Multi-scale habitat selection modeling: a review and outlook. Landsc. Ecol. 31, 1161–1175. doi: 10.1007/s10980-016-0374-x
McLoughlin, P., Gaillard, J.-M., Boyce, M., Bonenfant, C., Messier, F., Duncan, P., et al. (2007). Lifetime reproductive success and composition of the home range in a large herbivore. Ecology 88, 3192–3201. doi: 10.1890/06-1974.1
McLoughlin, P. D., Boyce, M. S., Coulson, T., and Clutton-Brock, T. (2006). Lifetime reproductive success and density-dependent, multi-variable resource selection. Proc. R. Soc. B Biol. Sci. 273, 1449–1454. doi: 10.1098/rspb.2006.3486
McLoughlin, P. D., Morris, D. W., Fortin, D., Vander Wal, E., and Contasti, A. L. (2010). Considering ecological dynamics in resource selection functions. J. Anim. Ecol. 79, 4–12. doi: 10.1111/j.1365-2656.2009.01613.x
Meadow, A. M., Ferguson, D. B., Guido, Z., Horangic, A., Owen, G., and Wall, T. (2015). Moving toward the deliberate coproduction of climate science knowledge. Weather Clim. Soc. 7, 179–191. doi: 10.1175/WCAS-D-14-00050.1
Meier, G., and Brown, J. F. (2014). Remote Sensing of Land Surface Phenology. Reston: US Geol. Surv.
Menkham, K., Sukmasuang, R., Pla-Ard, M., Charaspet, K., Panganta, T., Trisurat, Y., et al. (2019). Population and habitat use of Asian elephants (Elephas maximus) and five ungulate species in Khao Ang Rue Nai Wildlife Sanctuary, Chachoengsao Province, Thailand. Biodivers. J. Biol. Divers. 20, 2213–2221.
Merems, J. L., Shipley, L. A., Levi, T., Ruprecht, J., Clark, D. A., Wisdom, M. J., et al. (2020). Nutritional-landscape models link habitat use to condition of mule deer (Odocoileus hemionus). Front. Ecol. Evol. 8:98. doi: 10.3389/fevo.2020.00098
Merkle, J. A., Anderson, N. J., Baxley, D. L., Chopp, M., Gigliotti, L. C., Gude, J. A., et al. (2019). A collaborative approach to bridging the gap between wildlife managers and researchers. J. Wildl. Manag. 83, 1644–1651. doi: 10.1002/jwmg.21759
Middleton, A. D., Sawyer, H., Merkle, J. A., Kauffman, M. J., Cole, E. K., Dewey, S. R., et al. (2019). Conserving transboundary wildlife migrations: recent insights from the greater yellowstone ecosystem. Front. Ecol. Environ. 18, 83–91. doi: 10.1002/fee.2145
Morris, D. W. (2003). Toward an ecological synthesis: a case for habitat selection. Oecologia 136, 1–13. doi: 10.1007/s00442-003-1241-4
Morris, L. R., Proffitt, K. M., and Blackburn, J. K. (2016). Mapping resource selection functions in wildlife studies: concerns and recommendations. Appl. Geogr. 76, 173–183. doi: 10.1016/j.apgeog.2016.09.025
Morrison, M. L. (2001). A proposed research emphasis to overcome the limits of wildlife-habitat relationship studies. J. Wildl. Manag. 65, 613–623.
Morrison, M. L. (2012). The habitat sampling and analysis paradigm has limited value in animal conservation: a prequel. J. Wildl. Manag. 76, 438–450. doi: 10.1002/jwmg.333
Morrison, M. L., Marcot, B. G., and Mannan, R. W. (2006). Wildlife-Habitat Relationships: Concepts and Applications. Washington, DC: Island Press.
Muff, S., Signer, J., and Fieberg, J. (2019). Accounting for individual-specific variation in habitat-selection studies: efficient estimation of mixed-effects models using Bayesian or frequentist computation. J. Anim. Ecol. 89, 80–92. doi: 10.1111/1365-2656.13087
Nel, J. L., Roux, D. J., Driver, A., Hill, L., Maherry, A. C., Snaddon, K., et al. (2016). Knowledge co-production and boundary work to promote implementation of conservation plans. Conserv. Biol. 30, 176–188. doi: 10.1111/cobi.12560
Nielsen, S. E., McDermid, G., Stenhouse, G. B., and Boyce, M. S. (2010). Dynamic wildlife habitat models: seasonal foods and mortality risk predict occupancy-abundance and habitat selection in grizzly bears. Biol. Conserv. 143, 1623–1634. doi: 10.1016/j.biocon.2010.04.007
Nielson, R. M., and Sawyer, H. (2013). Estimating resource selection with count data. Ecol. Evol. 3, 2233–2240. doi: 10.1002/ece3.617
Nilsen, E. B., Linnell, J. D., and Andersen, R. (2004). Individual access to preferred habitat affects fitness components in female roe deer Capreolus capreolus. J. Anim. Ecol. 73, 44–50. doi: 10.1111/j.1365-2656.2004.00790.x
Oates, B. A., Merkle, J. A., Kauffman, M. J., Dewey, S. R., Jimenez, M. D., Vartanian, J. M., et al. (2019). Antipredator response diminishes during periods of resource deficit for a large herbivore. Ecology 100:e02618. doi: 10.1002/ecy.2618
Openshaw, S. (1984). The Modifiable Areal Unit Problem Concepts and Techniques in Modern Geography. Norwich: Geobooks.
Peek, J. M., Dennis, B., and Hershey, T. (2002). Predicting population trends of mule deer. J. Wildl. Manag. 66, 729–736. doi: 10.2307/3803138
Peek, J. M., Korol, J. J., Gay, D., and Hershey, T. (2001). Overstory–understory biomass changes over a 35-year period in southcentral Oregon. For. Ecol. Manag. 150, 267–277. doi: 10.1016/S0378-1127(00)00585-5
Peterson, A. T., Papeş, M., and Soberón, J. (2008). Rethinking receiver operating characteristic analysis applications in ecological niche modeling. Ecol. Model. 213, 63–72. doi: 10.1016/j.ecolmodel.2007.11.008
Pettorelli, N., Pelletier, F., Hardenberg, A. V., Festa-Bianchet, M., and Côté, S. D. (2007). Early onset of vegetation growth vs. rapid green-up: impacs on juvenile mountain ungulates. Ecology 88, 381–390. doi: 10.1890/06-0875
Pettorelli, N., Ryan, S., Mueller, T., Bunnefeld, N., Jędrzejewska, B., Lima, M., et al. (2011). The normalized difference vegetation index (NDVI): unforeseen successes in animal ecology. Clim. Res. 46, 15–27. doi: 10.3354/cr00936
Plante, S., Dussault, C., and Côté, S. D. (2017). Landscape attributes explain migratory caribou vulnerability to sport hunting. J. Wildl. Manag. 81, 238–247. doi: 10.1002/jwmg.21203
Proffitt, K. M., DeVoe, J., Barker, K., Durham, R., Hayes, T., Hebblewhite, M., et al. (2019). A century of changing fire management alters ungulate forage in a wildfire-dominated landscape. For. Int. J. For. Res. 92, 523–537. doi: 10.1093/forestry/cpz017
Proffitt, K. M., Graigg, J. L., Garrott, R. A., Hamlin, K. L., Cunningham, J., Gude, J. A., et al. (2010). Changes in Elk resource selection and distributions associated with a late-season elk hunt. J. Wildl. Manag. 74, 210–218. doi: 10.2193/2008-593
Proffitt, K. M., Hebblewhite, M., Peters, W., Hupp, N., and Shamhart, J. (2016). Linking landscape-scale differences in forage to ungulate nutritional ecology. Ecol. Appl. 26, 2156–2174. doi: 10.1002/eap.1370
Ranglack, D. H., Proffitt, K. M., Canfield, J. E., Gude, J. A., Rotella, J., and Garrott, R. A. (2017). Security areas for elk during archery and rifle hunting seasons. J. Wildl. Manag. 81, 778–791. doi: 10.1002/jwmg.21258
Reinking, A. K., Smith, K. T., Mong, T. W., Read, M. J., and Beck, J. L. (2019). Across scales, pronghorn select sagebrush, avoid fences, and show negative responses to anthropogenic features in winter. Ecosphere 10:e02722. doi: 10.1002/ecs2.2722
Reyers, B., Nel, J. L., O’Farrell, P. J., Sitas, N., and Nel, D. C. (2015). Navigating complexity through knowledge coproduction: mainstreaming ecosystem services into disaster risk reduction. Proc. Natl. Acad. Sci. U.S.A. 112, 7362–7368. doi: 10.1073/pnas.1414374112
Riggs, R. A., Keane, R. E., Cimon, N., Cook, R., Holsinger, L., Cook, J., et al. (2015). Biomass and fire dynamics in a temperate forest-grassland mosaic: integrating multi-species herbivory, climate, and fire with the FireBGCv2/GrazeBGC system. Ecol. Model. 296, 57–78. doi: 10.1016/j.ecolmodel.2014.10.013
Roberts, D. R., Bahn, V., Ciuti, S., Boyce, M. S., Elith, J., Guillera-Arroita, G., et al. (2017). Cross-validation strategies for data with temporal, spatial, hierarchical, or phylogenetic structure. Ecography 40, 913–929. doi: 10.1111/ecog.02881
Romesburg, H. C. (1981). Wildlife science: gaining reliable knowledge. J. Wildl. Manag. 45, 293–313.
Rowland, M. M., Nielson, R. M., Wisdom, M. J., Coe, P. K., Cook, J. G., Hafer, J. M., et al. (2018a). Linking nutrition with landscape features in a regional habitat-use model for elk in Western Oregon and Washington. Wildl. Monogr. 199, 31–49.
Rowland, M. M., Wisdom, M. J., Johnson, B. K., and Kie, J. G. (2000). Elk distribution and modeling in relation to roads. J. Wildl. Manag. 64, 672–684.
Rowland, M. M., Wisdom, M. J., Nielson, R. M., Cook, J. G., Cook, R. C., Johnson, B. K., et al. (2018b). Modeling elk nutrition and habitat use in Western Oregon and Washington. Wildl. Monogr. 199, 1–102.
Royle, J. A., Chandler, R. B., Yackulic, C., and Nichols, J. D. (2012). Likelihood analysis of species occurrence probability from presence-only data for modelling species distributions. Methods Ecol. Evol. 3, 545–554. doi: 10.1111/j.2041-210X.2011.00182.x
Russell, R. E., Gude, J. A., Anderson, N. J., and Ramsey, J. M. (2015). Identifying priority chronic wasting disease surveillance areas for mule deer in Montana. J. Wildl. Manag. 79, 989–997. doi: 10.1002/jwmg.914
Rykiel, E. J. (1996). Testing ecological models: the meaning of validation. Ecol. Model. 90, 229–244. doi: 10.1016/0304-3800(95)00152-2
Salas, E. A. L., Valdez, R., Michel, S., and Boykin, K. G. (2018). Habitat assessment of Marco Polo sheep (Ovis ammon polii) in Eastern Tajikistan: modeling the effects of climate change. Ecol. Evol. 8, 5124–5138. doi: 10.1002/ece3.4103
Sawyer, H., Kauffman, M. J., Nielson, R. M., and Horne, J. S. (2009). Identifying and prioritizing ungulate migration routes for landscape-level conservation. Ecol. Appl. 19, 2016–2025. doi: 10.1890/08-2034.1
Sawyer, H., LeBeau, C. W., McDonald, T. L., Xu, W., and Middleton, A. D. (2019). All routes are not created equal: an ungulate’s choice of migration route can influence its survival. J. Appl. Ecol. 56, 1860–1869.
Sawyer, H., Nielson, R. M., Lindzey, F., and McDonald, L. L. (2006). Winter habitat selection of mule deer before and during development of a natural gas field. J. Wildl. Manag. 70, 396–403. doi: 10.2193/0022-541x(2006)70[396:whsomd]2.0.co;2
Schrempp, T. V., Rachlow, J. L., Johnson, T. R., Shipley, L. A., Long, R. A., Aycrigg, J. L., et al. (2019). Linking forest management to moose population trends: the role of the nutritional landscape. PLoS One 14:e0219128. doi: 10.1371/journal.pone.0219128
Schuette, P., Creel, S., and Christianson, D. (2016). Ungulate distributions in a rangeland with competitors, predators and pastoralists. J. Appl. Ecol. 53, 1066–1077. doi: 10.1111/1365-2664.12610
Sekhar, N. U. (1998). Crop and livestock depredation caused by wild animals in protected areas: the case of Sariska Tiger Reserve, Rajasthan, India. Environ. Conserv. 25, 160–171. doi: 10.1017/s0376892998000204
Shean, D. E., Alexandrov, O., Moratto, Z. M., Smith, B. E., Joughin, I. R., Porter, C., et al. (2016). An automated, open-source pipeline for mass production of digital elevation models (DEMs) from very-high-resolution commercial stereo satellite imagery. ISPRS J. Photogram. Remote Sens. 116, 101–117. doi: 10.1016/j.isprsjprs.2016.03.012
Spitz, D. B., Rowland, M. M., Clark, D. A., Wisdom, M. J., Smith, J. B., Brown, C. L., et al. (2019). Behavioral changes and nutritional consequences to elk (Cervus canadensis) avoiding perceived risk from human hunters. Ecosphere 10:e02864. doi: 10.1002/ecs2.2864
Turner, W., Rondinini, C., Pettorelli, N., Mora, B., Leidner, A. K., Szantoi, Z., et al. (2015). Free and open-access satellite data are key to biodiversity conservation. Biol. Conserv. 182, 173–176. doi: 10.1016/j.biocon.2014.11.048
van Beest, F. M., Van Moorter, B., and Milner, J. M. (2012). Temperature-mediated habitat use and selection by a heat-sensitive northern ungulate. Anim. Behav. 84, 723–735. doi: 10.1016/j.anbehav.2012.06.032
Varley, N., and Boyce, M. S. (2006). Adaptive management for reintroductions: updating a wolf recovery model for Yellowstone National Park. Ecol. Model. 193, 315–339. doi: 10.1016/j.ecolmodel.2005.09.001
Villamuelas, M., Fernández, N., Albanell, E., Gálvez-Cerón, A., Bartolomé, J., Mentaberre, G., et al. (2016). The Enhanced Vegetation Index (EVI) as a proxy for diet quality and composition in a mountain ungulate. Ecol. Indic. 61, 658–666. doi: 10.1016/j.ecolind.2015.10.017
Voinov, A., Kolagani, N., McCall, M. K., Glynn, P. D., Kragt, M. E., Ostermann, F. O., et al. (2016). Modelling with stakeholders – Next generation. Environ. Model. Softw. 77, 196–220. doi: 10.1016/j.envsoft.2015.11.016
Walters, C. J. (1986). Adaptive Management of Renewable Resources. New York, NY: Macmillan Publishers Ltd.
Ward, A. I., White, P. C., Smith, A., and Critchley, C. H. (2004). Modelling the cost of roe deer browsing damage to forestry. For. Ecol. Manag. 191, 301–310. doi: 10.1016/j.foreco.2003.12.018
Wheatley, M., and Johnson, C. (2009). Factors limiting our understanding of ecological scale. Ecol. Complex. 6, 150–159. doi: 10.1016/j.ecocom.2008.10.011
White, K. S., Gregovich, D. P., and Levi, T. (2018). Projecting the future of an alpine ungulate under climate change scenarios. Glob. Chang. Biol. 24, 1136–1149. doi: 10.1111/gcb.13919
Wisdom, M. J., Rowland, M. M., Nielson, R. M., Cook, J. G., Johnson, B. K., Coe, P. K., et al. (2018a). Modeling to evaluate elk habitat: contemporary approaches for Western Oregon and Washington. Wildl. Monogr. 199, 8–12.
Wisdom, M. J., Rowland, M. M., Nielson, R. M., Cook, J. G., Johnson, B. K., Hafer, J. M., et al. (2018b). Nutrition and habitat-use models for elk management in western Oregon and Washington. Wildl. Monogr. 199, 50–69.
Yates, K. L., Bouchet, P. J., Caley, M. J., Mengersen, K., Randin, C. F., Parnell, S., et al. (2018). Outstanding challenges in the transferability of ecological models. Trends Ecol. Evol. 33, 790–802. doi: 10.1016/j.tree.2018.08.001
Keywords: habitat use, model development, covariates, evaluation, inference space, occupancy modeling, resource selection, ungulate management
Citation: Wisdom MJ, Nielson RM, Rowland MM and Proffitt KM (2020) Modeling Landscape Use for Ungulates: Forgotten Tenets of Ecology, Management, and Inference. Front. Ecol. Evol. 8:211. doi: 10.3389/fevo.2020.00211
Received: 01 February 2020; Accepted: 08 June 2020;
Published: 30 June 2020.
Edited by:
Paul Richard Krausman, University of Arizona, United StatesReviewed by:
Stan Boutin, University of Alberta, CanadaJohn Alfred Bissonette, Utah State University, United States
Copyright © 2020 Wisdom, Nielson, Rowland and Proffitt. This is an open-access article distributed under the terms of the Creative Commons Attribution License (CC BY). The use, distribution or reproduction in other forums is permitted, provided the original author(s) and the copyright owner(s) are credited and that the original publication in this journal is cited, in accordance with accepted academic practice. No use, distribution or reproduction is permitted which does not comply with these terms.
*Correspondence: Michael J. Wisdom, bWljaGFlbC53aXNkb21AdXNkYS5nb3Y=