- 1Department of Anthropology and Heritage Studies, University of California, Merced, Merced, CA, United States
- 2Bioarchaeology and Stable Isotope Research Laboratory, Department of Anthropology, Vanderbilt University, Nashville, TN, United States
- 3Metals, Environmental and Terrestrial Analytical Laboratory, School of Earth and Space Exploration, Arizona State University, Tempe, AZ, United States
- 4Department of Anthropology, University of Toronto, Toronto, ON, Canada
- 5Archaeology Centre, University of Toronto, Toronto, ON, Canada
- 6Center for Bioarchaeological Research, School of Human Evolution and Social Change, Arizona State University, Tempe, AZ, United States
- 7Department of Anthropology, University of California, Santa Cruz, Santa Cruz, CA, United States
The analysis of 87Sr/86Sr has become a robust tool for identifying non-local individuals at archeological sites. The 87Sr/86Sr in human bioapatite reflects the geological signature of food and water consumed during tissue development. Modeling relationships between 87Sr/86Sr in human environments, food webs, and archeological human tissues is critical for moving from identifying non-locals to determining their likely provenience. In the Andes, obstacles to sample geolocation include overlapping 87Sr/86Sr of distant geographies and a poor understanding of mixed strontium sources in food and drink. Here, water is investigated as a proxy for bioavailable strontium in archeological human skeletal and dental tissues. This study develops a water 87Sr/86Sr isoscape from 262 samples (220 new and 42 published samples), testing the model with published archeological human skeletal 87Sr/86Sr trimmed of probable non-locals. Water 87Sr/86Sr and prediction error between the predicted and measured 87Sr/86Sr for the archeological test set are compared by elevation, underlying geology, and watershed size. Across the Peruvian Andes, water 87Sr/86Sr ranges from 0.7049 to 0.7227 (M = 0.7081, SD = 0.0027). Water 87Sr/86Sr is higher in the highlands, in areas overlying older bedrock, and in larger watersheds, characteristics which are geographically correlated. Spatial outliers identified are from canals, wells, and one stream, suggesting those sources could show non-representative 87Sr/86Sr. The best-fit water 87Sr/86Sr isoscape achieves prediction errors for archeological samples ranging from 0.0017 – 0.0031 (M = 0.0012, n = 493). The water isoscape explains only 7.0% of the variation in archeological skeletal 87Sr/86Sr (R2 = 0.07), but 90.0% of archeological skeleton 87Sr/86Sr fall within the site isoscape prediction ± site prediction standard error. Due to lower sampling density and higher geological variability in the highlands, the water 87Sr/86Sr isoscape is more useful for ruling out geographic origins for lowland dwellers than for highlanders. Baseline studies are especially needed in the highlands and poorly sampled regions. Because the results demonstrate that a geostatistical water model is insufficient for fully predicting human 87Sr/86Sr variation, future work will incorporate additional substrates like plants, fauna, soils, and dust, aiming to eventually generate a regression and process-based mixing model for the probabilistic geolocation of Andean samples.
Introduction
Over the past few decades, the analysis of radiogenic strontium isotopes (87Sr/86Sr) in skeletal and dental tissues has become a robust tool for identifying probable non-local individuals among archeological samples and for geo-locating them by matching sample 87Sr/86Sr to landscape 87Sr/86Sr. While mass-dependent isotopic fractionation does occur between dietary and water sources of strontium as they are processed by the human body, the effects of fractionation are negligible after laboratory normalization (Graustein, 1989, p. 492; Beard and Johnson, 2000; Knudson et al., 2010, p. 2; Lewis et al., 2017; Bataille et al., 2020). Due to this small magnitude of diet-body offset of strontium isotopes, the 87Sr/86Sr in animal tissues reflect the geological signature of underlying bedrock, which is incorporated into the mixed sources of food, water, and atmospheric elements in the food chain that are consumed and imbibed throughout the course of tissue development (Graustein, 1989; Bentley, 2006; Grimstead et al., 2017; Bataille et al., 2020). Strontium isotope analysis is typically employed by archeologists to examine questions of past migration and mobility: identifying first-generation migrants in a skeletal sample, constraining areas of possible provenience for those non-locals, and assessing the proportion of geologically local to non-local individuals in skeletal assemblages (e.g., Ericson, 1985; Price et al., 1994, 2002, 2015; Evans et al., 2006; Knudson and Price, 2007; Slovak et al., 2009; Montgomery, 2010; Tung and Knudson, 2011; Frei and Price, 2012; Knudson et al., 2012b). Assuming mostly local foods were consumed, individuals with 87Sr/86Sr that differs from most other individuals in a sample, or from the measured 87Sr/86Sr of the local environmental baseline, were likely non-local during the period when the sampled tissue was developing.
Understanding how much difference in 87Sr/86Sr across the sample is required for a non-local designation and then reconstructing the likely provenience of those suspected non-locals depends on accurately characterizing the 87Sr/86Sr expected throughout the region(s) where they might have lived or traveled. Methods for defining the expected local 87Sr/86Sr range have shifted over the last few decades from statistically parsing human skeletal 87Sr/86Sr around the sample mean (e.g., Wright, 2005; Slovak et al., 2009; Price et al., 2015) to testing archeological and modern fauna with small home ranges (Price et al., 2002, 2007; Evans and Tatham, 2004; Hedman et al., 2009, 2018; Knudson and Tung, 2011). More recently, many scholars have argued that the only reliable way to determine the local 87Sr/86Sr range is by analyzing the bioavailable strontium in local environmental reference or baseline materials like soils, plants, and local fauna, and then aggregating those reference 87Sr/86Sr to geological units, soil units, or statistical groupings of baseline and/or skeletal materials (Valentine et al., 2008; Evans et al., 2010; Maurer et al., 2012; Knudson et al., 2014; Kootker et al., 2016; Grimstead et al., 2017; Willmes et al., 2018; Barberena et al., 2019; Pacheco-Forés et al., 2020; Snoeck et al., 2020). Unfortunately, because underlying bedrock geology is highly variable even at a short distance, and because rock weathers unevenly throughout catchments, these methods do not enable the sourcing of a sample to any location beyond the immediate vicinity of the sampling location. This is in part because the geological units the isotopic catchments are aggregated to are themselves models of the underlying bedrock that do not account for uncertainty error. Furthermore, because mammalian tissues derive their strontium content from the mixing of plants, fauna, water, and atmospheric particles ingested from the diet, sampling any one reference material or overreliance on bedrock geology will inevitably fail to capture the mixing of strontium in the human diet or in the diets of other animal end-members (Bentley, 2006; Grupe et al., 2011; Bataille et al., 2012; Burton and Price, 2013; Grimstead et al., 2017).
Because the 87Sr/86Sr of mixed-source isotopic catchments vary predictably across the landscape, models of baseline or reference landscape 87Sr/86Sr have become essential for reconstructing movements across the landscape throughout human history (Fisher et al., 2010; Frei and Frei, 2011; Laffoon et al., 2012; Knudson et al., 2014; Grupe et al., 2017a,b; Bataille et al., 2018; Willmes et al., 2018; Adams et al., 2019; Hoogewerff et al., 2019; Lengfelder et al., 2019; Scaffidi and Knudson, 2020). Isoscapes are spatially explicit models of isotope variability (Bowen, 2010; Ehleringer et al., 2010; Wunder, 2010; West et al., 2014; Pellegrini et al., 2016). Archeological and anthropological isoscapes have most commonly utilized a geostatistical kriging method to model isotopic variability across the landscape, which relies on underlying spatial auto-correlation in GPS-located isotope measurements to predict isotope values at unsampled locations (Copeland et al., 2016; Pellegrini et al., 2016; Wang et al., 2018; Willmes et al., 2018; Adams et al., 2019; Scaffidi and Knudson, 2020). Geostatistical models have been improved on in recent years by process-based isoscapes that rely on regression or machine learning methods to predict isotope variability as a function of environmental variables (Bataille and Bowen, 2012; Bataille et al., 2014, 2020; Hoogewerff et al., 2019)—these are rare in archeological and anthropological applications but are becoming more common (Bataille et al., 2012, 2018; Hoogewerff et al., 2019; Zimmer-Dauphinee et al., 2020). In either case, when predictive isoscapes are generated from samples reflecting the bioavailable strontium in the dietary catchment rather than bedrock outcroppings or soils containing weathered bedrock, they have the distinct advantage of capturing and predicting the mix of foods, water, and dust at every geographic location. This is important since studies have generally found that soil and bedrock 87Sr/86Sr alone are poor predictors for human tissue 87Sr/86Sr (Price et al., 2002, pp. 120–122; Knudson et al., 2014; Grimstead et al., 2017; Snoeck et al., 2020). Establishing accurate isoscapes of bioavailable 87Sr/86Sr variation in the natural environment and understanding what proxy materials best reflect human 87Sr/86Sr are prerequisites for accurately identifying non-locals in archeological assemblages and constraining their likely geographic origins to the most probable locations.
Isoscape modeling is notoriously tricky in mountainous landscapes due to their highly variable geology, hydrology, weather, and climate patterns (Bowen and Revenaugh, 2003; Yamanaka et al., 2015). In the Andes Mountains, seismic activity, droughts, flooding, landslides, and intensive mining and agricultural practices are additional factors that alter 87Sr/86Sr distributions around the landscape. This is expected to contribute to high heterogeneity of 87Sr/86Sr in higher-elevation zones. The study region of southern Peru is located in the Central Volcanic Zone of the Andes, where lava flows across a broad geographic area tap the same magma sources, homogenizing surface materials across regions within the volcanic arc (Scott et al., 2018). Similarly, recent findings by Serna et al. (2020, p. 10) confirm that in volcanic zones of Northern Patagonia are influenced away from bedrock 87Sr/86Sr by the deposition and mixing of “unradiogenic volcanic tephra”… and “reworked loess mixing Quaternary and Jurassic volcaniclastic sediments.” Serna et al. (2020) conclude that dust and sediment reworking processes dominate the geomorphology of bioavailable Sr in some volcanic zones—sampling in these areas is critical for correctly characterizing expected 87Sr/86Sr.
Another obstacle to accurate geolocation that is particularly pronounced in the Andes is the problem of equifinality (Price et al., 2002)—the fact that 87Sr/86Sr of geographically distant regions are often identical, so that samples with the same 87Sr/86Sr, recovered from the same location, may have actually originated in distinct places. For example, the recent meta-analysis of published 87Sr/86Sr from Andean archeological sites by Scaffidi and Knudson (2020) show that archeological human and animal tissue 87Sr/86Sr ranges from 0.7038 to 0.7239. The upper and lower ends of the human and animal range are slightly higher than the theoretical Andean range based on bedrock, where 87Sr/86Sr = 0.7050 to 0.7200 (Knudson et al., 2014). Variation follows an east-west gradient corresponding to increasing elevation, longitude, and distance from the coast, so that locally born individuals from similar longitudes might display identical 87Sr/86Sr even though they grew up thousands of miles apart latitudinally (Scaffidi and Knudson, 2020). Yet another obstacle is that the mixing of geological strontium sources in Andean food webs prior to their incorporation into human tissues—between soils, crops, fertilizers, animal protein, different water sources, dust, and sea spray—is poorly understood, both in the past and the present.
There is considerable debate over the use of relatively simplistic geostatistical kriging models for predicting 87Sr/86Sr variability across the landscape. Kriging assumes data are normally distributed, continuous, stationary, and spatially auto-correlated—nearer points should be more similar than farther points (Cressie, 1993; Oliver and Webster, 2014, 2015). Because bedrock units are discrete blocks, Sr reservoirs are not continuous in 87Sr/86Sr across the landscape and 87Sr/86Sr are often non-normally distributed. Therefore, geostatistical methods “can give ambiguous and inaccurate results due to the challenges of incorporating non-normal and skewed distributions” which limit the utility of kriged isoscapes for determining provenience (Bataille et al., 2014, 2020, p. 8). Process and regression-based methods are ultimately necessary for developing high-resolution models of human 87Sr/86Sr variability (Hoogewerff et al., 2019; Bataille et al., 2020; Serna et al., 2020). Notwithstanding these limitations, kriging has been used to develop coarse, preliminary predictions of bioavailable 87Sr/86Sr for hydrological (e.g., Varouchakis et al., 2012; Johnson et al., 2018) and anthropological and archeological applications (Frei and Frei, 2011; Copeland et al., 2016; Wang et al., 2018; Willmes et al., 2018; Adams et al., 2019). Published bedrock and volcanic tephra 87Sr/86Sr are abundant throughout the Andes (Mamani et al., 2008; Scott et al., 2018), but these samples are limited in various parts of the Peruvian Andes, which limits the accuracy of existing bedrock 87Sr/86Sr models (e.g., Schenk et al., 1999). Therefore, this paper argues that modeling a geostatistical isoscape of bioavailable 87Sr/86Sr is appropriate as an initial step to characterizing 87Sr/86Sr variability in these sparsely sampled regions, an effort which will be improved on by subsequent sampling campaigns and future regression-based models.
This paper follows recent ecological and archeological studies to argue that because water averages strontium from throughout the dietary catchment (Frei and Frei, 2011; Bataille and Bowen, 2012; Frei and Frei, 2013; Zitek et al., 2015; Crowley et al., 2017), imbibed water from the dietary catchment may contribute to a portion of 87Sr/86Sr variation in archeological skeletal tissues. We report 220 new water 87Sr/86Sr from GPS-located water samples throughout the Peruvian Andes. We plot these together with 46 published Peruvian water 87Sr/86Sr (Flusche et al., 2005; Alaica et al., 2020), and generate a geostatistical water 87Sr/86Sr isoscape from this dataset trimmed of spatial outlier values (n = 249). We then test the validity of the model for geolocating previously compiled human archeological samples considered local to their burial location (from Scaffidi and Knudson, 2020)1. Ultimately, a mismatch (beyond the model error range) between the model predictions and a measured archeological sample will enable that individual to be identified as non-local during the time of tissue development and allow archeologists and other researchers in the Andes to constrain possible provenience matches along the modeled 87Sr/86Sr gradient.
Materials and Methods
Strontium Isotopes in the Andes: From Dietary Catchments to the Human Skeleton
Strontium enters the body through food and water consumed, and then substitutes for Ca ions in the bioapatite crystals of bone and enamel. While diet-body fractionation throughout the process of digestion and tissue incorporation is minimal, a trophic level effect called biopurification has been well documented throughout ecological systems. The Sr/Ca is reduced by approximately a factor of five in each trophic level, so that plants contain lower Sr/Ca ratios than soils, but higher Sr/Ca than meat (Comar et al., 1957a,b; Wasserman et al., 1958; Sillen and Kavanagh, 1982; Burton and Wright, 1995; Bentley, 2006). Because strontium follows calcium pathways into skeletal tissues, calcium-enriched foods like salt and seafood can be disproportionately reflected in tissue 87Sr/86Sr, which could override the signature of the isotopic catchment for individuals with significantly more consumption of salty or marine foods (Sillen and Kavanagh, 1982; Hodell et al., 2004; Slovak et al., 2009; Knudson et al., 2010). Thus, the Sr/Ca ratio and related 87Sr/86Sr in human body tissues are largely impacted by the proportion and types of foods in the diet. Assuming these foods were mostly locally procured, skeletal 87Sr/86Sr reflects the geological 87Sr/86Sr of the dietary catchment of residence during the period of tissue formation (Ericson, 1985; Price et al., 1994, 2002; Montgomery, 2010).
The 87Sr/86Sr in the food chain at a given sampling location is a mix of weathered bedrock eroded and mixed into soils, surface and groundwater carrying strontium from different weathered sources, atmospheric sources of strontium like sea spray, ash, and dust, and faunal and plant strontium reservoirs (Bentley, 2006; Bataille et al., 2020). The proportion of these different sources varies across the landscape, and is best understood by localized, multi-source mixing models (Montgomery et al., 2007; Bataille et al., 2012, 2018, 2020; Willmes et al., 2018). In the Andes, 87Sr/86Sr baseline data from plants, fauna, and water are relatively limited compared to other world regions (but see Barberena et al., 2017, 2019). Expectations for catchment 87Sr/86Sr are primarily derived from models based on bedrock outcroppings. Bedrock models illustrate that patterns in 87Sr/86Sr in the Peruvian Andes are influenced by the age and lithology of the two sub-ranges: the Cordillera Occidental (Western Cordillera), which rises sharply out of the coastal plain into a lower-elevation range, and the Cordillera Oriental (Eastern Cordillera), which is the highest elevation range to the east that gradually descends into the Amazonian basin (Figure 1). Coastal areas throughout the north and south of Peru are comprised by Quaternary sediments with contributions from various alluvial flows from the highlands (Bellido et al., 1956; Schenk et al., 1999), and thus, bioavailable 87Sr/86Sr is expected to be relatively heterogeneous at the country scale. The near-coastal Cordillera Occidental is comprised of late Cenozoic volcanic rocks, which are older in the south-central Andes than in the north-central Andes. For example, bedrock outcropping 87Sr/86Sr in this front range varies from a mean of 0.7043 in Ecuador, to 0.7074 in Arequipa, to 0.7065 in northern Chile (see summary in Knudson et al., 2014, p. 206). In contrast, in the Cordillera Oriental, bedrock is dominated by Paleozoic andesites with more radiogenic (higher) 87Sr/86Sr, although bedrock has not been well-sampled in this region. In areas between the two ranges, like Lake Titicaca, 87Sr/86Sr should reflect a mix of alluvial deposits from both ranges, but again, bedrock samples are lacking (see summary in Knudson et al., 2014, pp. 206–207). Finally, the Amazon basin is comprised of Precambrian-Devonian sediments and alluvial contributions from the Cordillera Oriental (Bellido et al., 1956; Schenk et al., 1999). To the far east, Amazonian rivers show a broad range of 87Sr/86Sr, from 0.7056 to 0.7438, which reflect drainage of highly variable highland geologies in the eastern sub-range (Santos et al., 2015).
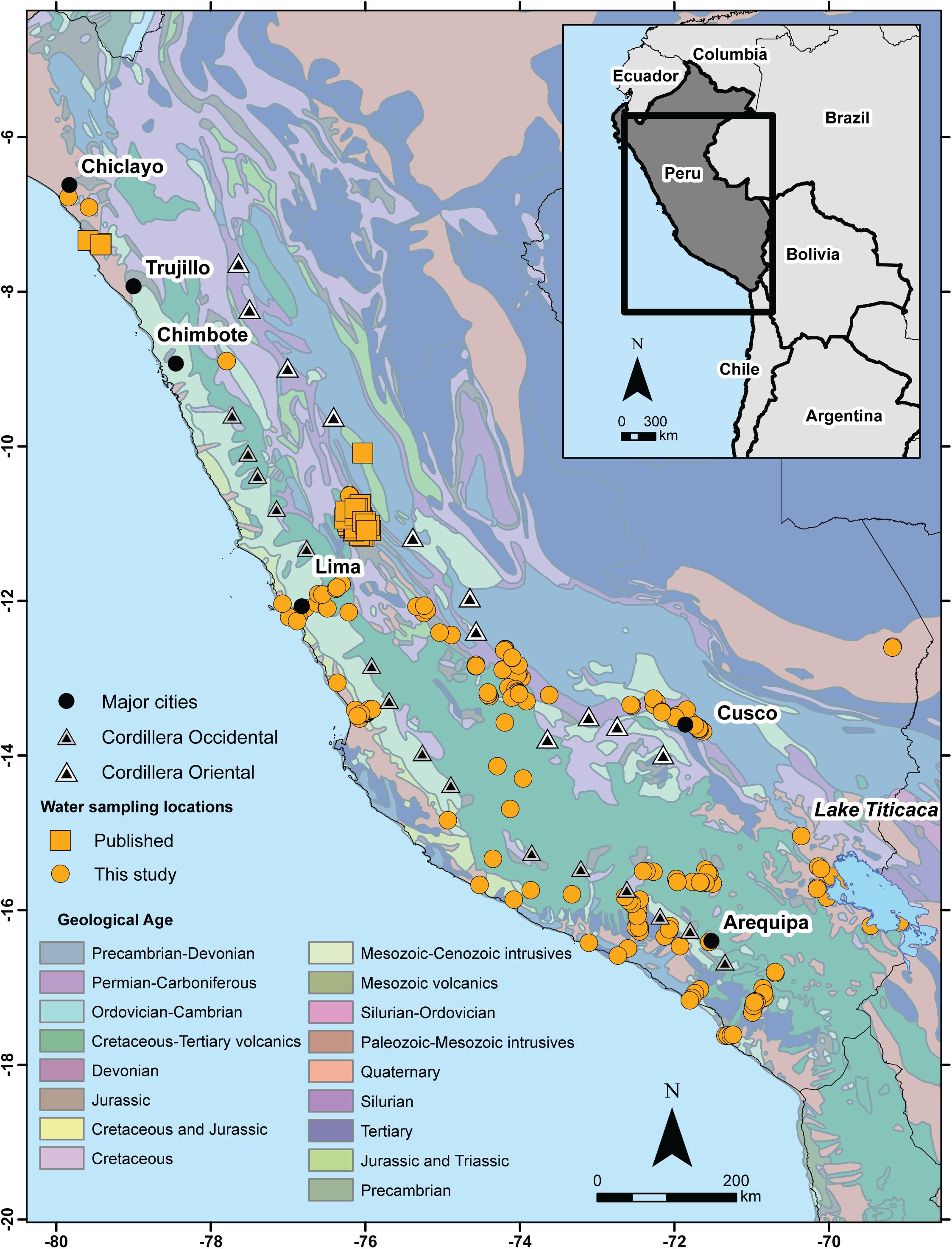
Figure 1. Water sampling locations throughout Peru plotted in decimal degrees (WGS 1984 datum), relative to bedrock geology modeled by Schenk et al. (1999). Major cities and the general location of mountains in the Cordillera Occidental (Western Cordillera) and the Cordillera Oriental (Eastern Cordillera) are indicated in the legend.
At present, it is unclear how bedrock 87Sr/86Sr variability is integrated into the human dietary catchment in the Andes. Generally, bedrock has broader 87Sr/86Sr ranges than the catchment-averaged bioavailable 87Sr/86Sr from biological substrates (Bataille et al., 2020), and of those substrates, omnivorous skeletons show broader ranges than soils, herbivorous fauna, and plants (Valentine et al., 2008; Ladegaard-Pedersen et al., 2020). However, in geologically active areas in the highlands like those around Cusco, Knudson et al. (2014) found wider 87Sr/86Sr variability in soils than in published bedrock. In coastal locations, soil 87Sr/86Sr matched bedrock 87Sr/86Sr but very few coastal bedrock samples were available for comparison. This study shows promise for establishing local bioavailable 87Sr/86Sr expectations through soil analysis but is limited by its geographic coverage—soils were tested from only 17 locations (n = 114), all but three of which were coastal. Knudson et al. (2014) also found that archeological human and faunal samples were consistently offset from soil 87Sr/86Sr in many locations. This makes sense since soils and rocks are generally not directly consumed, although rock contributions to the diet might be nominal in groups that process food with grinding stones (Johnson et al., 2019), practice geophagy, or consume geological materials as digestive aids—all were done in the ancient Andes (Johns, 1990). These inconsistencies between bedrock, soil, and skeletal 87Sr/86Sr could be due to differential weathering of bedrock throughout the steep Andes and/or the overall paucity of bedrock and soil samples from the region; nonetheless, this illustrates the need for a deeper examination of different substrates for the analysis of bioavailable Sr in the region.
Indeed, across the globe, researchers have debated which environmental baseline materials incorporated into the food web are the best proxy for human tissue 87Sr/86Sr. Baseline studies have accordingly varied in their reference material of focus according to the different landscapes of archeological study locations. Using small modern or archeological fauna to establish a baseline was the earliest approach, which remains one of the most common approaches. Because small animals tend to have small homes ranges, they should average many of the same elements of the isotopic catchment as human end members do (Price et al., 2002; Bentley and Knipper, 2005; Hedman et al., 2009; Kootker et al., 2016; Fernández-Crespo et al., 2020). However, fauna may not be local at all, and this is especially true for livestock (Knudson et al., 2012a; Grimstead et al., 2016). Furthermore, the home ranges of small animals can be larger than expected and may crosscut discrete 87Sr/86Sr catchments (Copeland et al., 2010). Or, faunal substrate animals may have consumed non-local food, especially when they fed at or near archeological settlements that relied to some extent on imported or fertilized foods. Furthermore, animal behavior and physiology can also lead to results that do not represent the dietary catchment well; for example, land snails burrow into discrete geological pockets (Thornton, 2011) and have an extraordinarily high rainwater contribution to their shell (Evans et al., 2009, 2010), leading to abnormal 87Sr/86Sr compared to other fauna or plants from the same area.
Plants are another common reference material for bioavailable strontium. When sampled from various root depths, they can provide a more representative mix of the dietary catchment (Åberg, 1995; Knipper et al., 2012; Grimstead et al., 2017), but caution still must be exercised when relying on plants for several reasons. First due to the process of biopurification, 87Sr/86Sr can vary throughout different plant organs and according to plant age (Bailey et al., 1996; Drouet et al., 2005; Drouet and Herbauts, 2008). Second, different species can also show distinct 87Sr/86Sr from the same location, due in part to their root systems accessing discrete pockets of strontium in the soil (Dijkstra, 2003; Poszwa et al., 2004). Third, differential contributions from sea spray and the acidity or other molecular differences of the microhabitat can cause plant 87Sr/86Sr to differ even when the same organ and species are sampled from the same location (Snoeck et al., 2020). A fourth complicating factor is the use of non-local fertilizers in either modern or ancient times, which can contribute a non-local geological signature to plant and soil samples (Bentley et al., 2004; Bentley, 2006; Evans et al., 2012; Thomsen and Andreasen, 2019). Sea bird guano (Julien et al., 1985; Szpak et al., 2012) and manure (Finucane, 2007) were commonly applied to agricultural fields throughout the pre-Hispanic Andes. Avoiding sampling from fertilized fields is therefore essential for accurately characterizing local bioavailable strontium throughout pre-Hispanic Andes (Knudson et al., 2014). However, in many parts of the Andes, unfertilized fields are rare to non-existent, so that systematic sampling of soils and plants to avoid contamination can be challenging if not impossible. Knudson et al.’s (2014) study suggests there is much more localized variability in bioavailable Sr that could be captured through systematic sampling of plants and other reference materials in the geologically and hydrologically complex dietary catchments of the Andes.
To that end, efforts are currently underway to develop local baseline models from environmental reference materials in discrete parts of the Andes, by a variety of research teams (e.g., Barberena et al., 2017; Barberena et al., 2019, inter alia; Serna et al., 2020). However, these efforts are largely localized to sub-regions of the Andes and constrained to sampling locations near targeted archeological sites. The only supra-regional analysis of Andean 87Sr/86Sr for archeological provenience published as of June 2020 is that of the Andean Paleomobility Unification (APU) project directed by Scaffidi and Knudson (2020). Arguing that humans provide the ultimate mixing model for 87Sr/86Sr in dietary catchments, this research shows that at a supra-regional scale, archeological tissue 87Sr/86Sr alone can be used to distinguish provenience between nearby areas, provided that suspected mobility routes are between discrete isoscape areas, along an east-west or coastal-highland gradient. However, this archeological isoscape does not handle equifinality well, given that people living at a similar distance from the coast but at different latitudes show similar 87Sr/86Sr. Therefore, the ongoing aims of the APU project include developing broader regional coverage and exploring variability in different 87Sr/86Sr substrates to further refine expected local baselines in the Andes.
Skeletal tissue 87Sr/86Sr at a sampling location reflects the averaging of plant and animal foods I the diet. In prehistoric communities, these are assumed to have been mostly locally procured, with the plant portion of the diet dominating the 87Sr/86Sr in the tissues of omnivorous humans (Beard and Johnson, 2000; Montgomery, 2010). In the pre-Hispanic Andes, however, the local food assumption may not hold true, and may not hold true uniformly across different individual’s diets within a community. As far back as the Preceramic, reciprocal exchange of maritime products and highland grains was practiced over robust long-distance trade networks throughout the Andes (Quilter and Stocker, 1983; Hastorf, 2003; see contributions in Dillehay, 2011; Cuéllar, 2013). Particularly with the rise of the first Andean states and empires, distinct diets developed between elites and subelites, so that access to imported foods varied between individuals in communities where social inequality was manifested through differential food consumption (Hastorf and Johannessen, 1993; Hastorf, 2003; Cuéllar, 2013). For communities or individuals with access to imported foods, diet-derived 87Sr/86Sr of human tissues might depart significantly from local plant, animal, and soil inputs. Therefore, it would not be surprising for archeological human 87Sr/86Sr in the Andes to deviate substantially from any mixed model of local soil, plant, and faunal 87Sr/86Sr. However, given that the human body is up to 60% water, with up to 31% of skeletal tissues comprised by water (Mitchell et al., 1945), the 87Sr/86Sr of imbibed water may also contribute significantly to skeletal tissues. If this is the case, local drinking water 87Sr/86Sr analysis could help diminish the effect of imported foods. Therefore, this paper examines the potential for water 87Sr/86Sr to predict 87Sr/86Sr in archeological human tissues, comparing water 87Sr/86Sr and measured vs. predicted 87Sr/86Sr between samples from different elevations, watershed sizes, and underlying geologies.
Assessing Water as a Proxy for Local Skeletal 87Sr/86Sr
Water is influential in the 87Sr/86Sr of a dietary catchment and varies somewhat predictably throughout watersheds and flowing surface water networks (Aubert et al., 2002; Bataille and Bowen, 2012; Frei and Frei, 2013; Bataille et al., 2014; Zitek et al., 2015; Crowley et al., 2017). Because the Sr/Ca entering food chains in the form of plants are directly related to the Sr/Ca of the water they consume (Sillen and Kavanagh, 1982), dissolved strontium in surface and groundwater heavily influences plant and faunal 87Sr/86Sr (Beard and Johnson, 2000; Bentley, 2006; Bataille and Bowen, 2012). Fast-flowing rivers might be suspected to carry a greater proportion of strontium from distant rocks and soils relative to slower-moving rivers, but the 87Sr/86Sr of river water has repeatedly been observed as consistent regardless of flow rate (see summary in Bentley, 2006, p. 8), seasonal, or long-term temporal variation (Voerkelius et al., 2010). In geologically active, higher elevations or during seasons of low discharge, flowing water 87Sr/86Sr is higher (more radiogenic), reflecting faster weathering of higher 87Sr/86Sr rock from higher elevation water sources throughout the watershed’s headwaters, depositing high-altitude sediments on floodplains (Bentley, 2006). The 87Sr/86Sr of these sediments is then mixed into the waters consumed throughout the dietary catchment at a given sampling location (see summary in Bataille et al., 2020, pp. 5–6). Hodell et al. (2004) report that water body depth also influences 87Sr/86Sr—locations with shallower water tables reflect upstream mixing, while locations with deeper water tables reflect more of the underlying bedrock signature. Watershed size also influenced 87Sr/86Sr variability, with larger watersheds leading to more homogenized 87Sr/86Sr in rivers (Brennan et al., 2019).
There are several limitations to using water 87Sr/86Sr as a reference material. First, sampling locations low in a watershed might also have greater pollution levels from upstream agricultural runoff, so higher elevation waters more closely reflect the weathering of underlying bedrock (Buhl et al., 1991; Tricca et al., 1999; Eikenberg et al., 2001; Thomsen and Andreasen, 2019). However, a recent study of Danish surface waters (Frei et al., 2020) shows that much of agricultural lime is retained by soils, so agricultural contamination may not influence surface water 87Sr/86Sr as much as previously thought. Second, proximity to the ocean also impacts water 87Sr/86Sr. Because modern ocean water is uniform across the planet (87Sr/86Sr = 0.7092) (Veizer, 1989), sea spray and brackish water can drive up otherwise low 87Sr/86Sr in coastal plains (Whipkey et al., 2000; Bentley, 2006; Snoeck et al., 2020), particularly for water samples closest to the ocean. This can lead 87Sr/86Sr in coastal regions to deviate from expectations based on bedrock. Third, Sr concentrations are generally low in global surface waters (0.06 ppm on average) (Capo et al., 1998), so water samples can be difficult to measure. Finally, at a global scale, river water 87Sr/86Sr is less variable than other substrates (Peucker-Ehrenbrink and Fiske, 2019; Bataille et al., 2020), which may average out some of the 87Sr/86Sr variability needed for identifying non-locals in zones with homogeneous bedrock geology.
Notwithstanding these potential drawbacks, surficial and groundwaters have been used as 87Sr/86Sr reference materials for provenience human samples. The earliest approach involved testing 87Sr/86Sr of water sources and comparing those to people known or suspected to reside at those locations. Over four decades ago, Nixon and Helsby (1976) showed a relationship between strontium concentrations of tap water in 12 English towns and tooth enamel of their human residents. However, a more recent study found no relationship with modern tooth enamel 87Sr/86Sr and tap water 87Sr/86Sr throughout the Netherlands (Kootker et al., 2020). In another study, Tipple et al. (2018) found that modern human hair 87Sr/86Sr reflected tap water 87Sr/86Sr. They analyzed 41 student hair samples alongside 99 tap water samples collected from sites around three schools in Salt Lake City, Utah, and found that only 6 hair samples fell outside of the tap water ranges for those locations (Tipple et al., 2018, p. 4, inferred from Figure 4). However, all tap water and hair 87Sr/86Sr fell within 0.0070 of each other (inferred from Figure 4), and the three schools shared overlapping 87Sr/86Sr, so that without known provenience data it would be impossible to distinguish between origin sites for all but individuals with the highest 87Sr/86Sr. In contrast with the Nixon and Helsby (1976) enamel study, the Tipple et al. (2018) study shows that 87Sr/86Sr in hair reflects uptake from bathing waters rather than from imbibed water.
Recent studies suggest that dietary Sr dominates human skeletal tissue 87Sr/86Sr rather than drinking water or atmospheric contributions. For example, a recent porcine controlled feeding study (Lewis et al., 2017) shows that there is little correlation between drinking water 87Sr/86Sr and skeletal 87Sr/86Sr compared to high correlations between dietary 87Sr/86Sr and skeletal 87Sr/86Sr. However, this study is limited by a small sample size (n = 19 pigs) and the assumption that human water metabolism is like that of pigs. Similarly, a large study of French children (Glorennec et al., 2016) shows that Sr concentrations are dominated by dietary Sr contributions rather than drinking water or atmospheric dust. However, this study is limited in that tissues were not directly tested for 87Sr/86Sr—instead, dietary Sr concentrations were estimated from known values of foods reported in national survey data. Furthermore, the sources of water were not controlled for. Bottled water was widely consumed by study participants, so the Sr concentrations may not reflect local water. Lewis et al. (2017) further clarify that drinking water can, in fact, contribute to skeletal 87Sr/86Sr in animals that drink frequently or in landscapes with Sr-rich waters. Additional testing of human tissues relative to known food and water sources is needed to further clarify how much water 87Sr/86Sr is integrated into human bioapatite relative to dietary sources in Andean landscapes.
The potential of using water 87Sr/86Sr for archeological skeleton provenience has varied according to study location and approach. Many baseline studies including water do not directly compare water and archeological human 87Sr/86Sr (Hodell et al., 2004; Bentley and Knipper, 2005; Frei and Frei, 2011, 2013; Lengfelder et al., 2019; Ladegaard-Pedersen et al., 2020; Pacheco-Forés et al., 2020), although some incorporate water into a mixed or predictive model and evaluate archeological data points against that model (e.g., Montgomery et al., 2007; Snoeck et al., 2020). Among studies in mountainous regions comparing skeletal 87Sr/86Sr directly to local water measurements, a relationship is reported between water elevation, sample variability, and fit of archeological samples to local water. In the Rhine Valley of the Alps, Hoogewerff et al. (2001) found that high elevation stream water 87Sr/86Sr in an area comprised of old, high Rb/Sr gneisses the river’s headwaters matched historic skeletal 87Sr/86Sr better than waters overlying younger, lower Rb/Sr limestone. After excluding outlier archeological pig 87Sr/86Sr, Bentley and Knipper (2005) report a similar finding for Rhine Valley sites—archeological samples overlapped with water 87Sr/86Sr from published studies, and showed more variability in the uplands than the lowlands. So far, direct comparisons of water and archeological 87Sr/86Sr have not been published for the Andes, but similar highland vs. lowland trends are to be expected for the similarly geologically complex river valleys of the Andes mountains.
The second approach to water 87Sr/86Sr sourcing involves testing water 87Sr/86Sr throughout a region, generating an isoscape predicting 87Sr/86Sr at unmeasured regions, and then validating that model with 87Sr/86Sr measurements from tissue samples. Testing how well a water strontium isoscape for the United States derived from bedrock weathering projections and river 87Sr/86Sr measurements (Bataille and Bowen, 2012) predicts mammalian body tissues, Crowley et al. (2017) found that that large-bodied mammalian tissues were more accurately predicted by the surface water model than vegetation and fish skeletons. They also report that the surface water model predicted mammalian tissues better for small, relatively non-complex watersheds than for larger watersheds. Frei and Frei (2011) found that surface water 87Sr/86Sr predictions in Denmark were consistently 15% higher in modern snail shell and soil leachates than in water samples from the same location, but modern sheep wool coincided with water 87Sr/86Sr. Lengfelder et al. (2019) used water 87Sr/86Sr in the Alps to construct an environmental mixing model which predicted 87Sr/86Sr 87Sr/86Sr in 70% (80/115) zooarchaeological samples. Lengfelder et al. (2019) considered the prediction matching if it fell within the isoscape prediction ± (2 SD + mean offset between water and archeological fauna, which was ∼0.001 for their study area). These appear to be the only studies that validate predictive models based on water 87Sr/86Sr for modern mammalian or archeological tissues. It is important to note that none of these studies tested water 87Sr/86Sr models with human skeletal 87Sr/86Sr; in light of this and recent feeding studies critiqued above (Glorennec et al., 2016; Lewis et al., 2017), understanding how much water contributes to skeletal 87Sr/86Sr is an ongoing research question.
In the present study, water was selected as a potential baseline proxy for the bioavailable strontium in archeological human tissues for several reasons. First, water samples are relatively quick to process (as described in section “Sample Collection and Strontium Isotope Analysis Methods” below). Unlike for stable oxygen isotope analysis, which is impacted by evaporation and storage of water samples, there is no evidence that 87Sr/86Sr is impacted by water storage and evaporation, so old, un-parafilmed samples can still be analyzed. Second, agricultural fields in many parts of the Andes are rarely planted up to the river line, to secure crops from seasonal flooding. Due to this, water samples from rivers are suspected to be less likely than plants or soils to be contaminated by non-local fertilizer (although this may not be the case for stationary water bodies). Third, unlike foods that were sometimes exchanged across great distances in the prehistoric Andes, water was difficult to transport and would have been largely imbibed from local sources. Particularly in dry coastal valleys of the Peruvian Andes, settlements were located within walking distance of rivers, which comprised some of the only sources of drinking water in many places2. While the composition and geographic origin of diets often varied within and between neighboring archeological sites due to social status differences, gender, occupation, or other social identities, drinking water should have been sourced mostly from the same nearby locations. Finally, surface water provides an inherently mixed model of local and upriver bioavailable strontium catchments that averages bedrock weathering, soil runoff, atmospheric, and upriver water contributions as it moves throughout the watershed. When local foods are consumed, the overall contribution of local water to the human diet may be further amplified—i.e., directly imbibed water is disproportionately represented in human tissues, and in the tissues of local plants and animals consumed. Based on the studies above, it is hypothesized that 87Sr/86Sr will vary according to elevation, watershed size, and underlying geological age. It is also hypothesized that homogenous water at lower elevations will better predict human archeological 87Sr/86Sr compared to geologically complex higher elevation zones.
Sample Collection and Strontium Isotope Analysis Methods
Water samples were collected by co-authors and collaborators3 during the North American summer and early fall of the 2009–2019 field seasons that comprise the drier months of the Austral winter and spring (Supplementary Table S1). We focused on collecting surface water from Southern Peru, where the majority of the co-authors’ archeological samples were excavated. Because lakes, rivers, and streams would have been the most commonly used drinking water sources for archeological communities, we focused our collection on flowing surface water near archeological sites, far from fertilized fields (following the prior methods of BSIRL and APU described in Zimmer-Dauphinee et al., 2020). Since much of the municipal water stores in Peru come from diverted surficial water, we also tested tap and fountain water samples to understand how accurately they reflect surface water 87Sr/86Sr, following Chesson et al.’s (2012) investigation of tap water in the United States. Between 15 and 50 mL of sediment-free water was collected from each location and each was categorized as surface water (rivers, streams/tributaries, lakes/ponds), ground or subsurface water (springs/wells), or man-made storage or diversion of surface water (reservoirs, canals, tap water/fountains). Non-acid washed collection vials were parafilmed to prevent contamination.
The geographic coordinates, water temperature, speed, location of sampling within the water body were collected by APU collaborators in a database developed by Scaffidi using the cloud-based and collaborative Collector app (ESRI) deployed on mobile devices equipped with Bad Elf precision GPS receivers accurate to within 2 m. Coordinates collected by the ACL and BSIRL were collected with consumer to technical grade GPS receivers ranging from 10 m to 1 m in locational accuracy, and limited hydrological data was collected from sampling sites. Finally, a literature search for published water 87Sr/86Sr in the Andes was completed. Several publications list 87Sr/86Sr for water sources in and around the Andes (Coudrain et al., 2002; Grove et al., 2003; Santos et al., 2015), but only one study published sample collection coordinates necessary for addition to this study’s database (Flusche et al., 2005). Coordinates were determined for published water 87Sr/86Sr points from the North Coast of Peru (Alaica et al., 2020). Sampling locations are illustrated relative to the bedrock geological units modeled by Schenk et al. (1999) in Figure 1.
Water samples were prepared for analysis in the Archaeological Chemistry Laboratory (Center for Bioarchaeological Research) at Arizona State University following established protocols (Pacheco-Forés et al., 2020). Water was strained into metal-free vials through a 2.5 μm pore size filter paper and acidified with 5% HCl to prevent microbial growth. In the Metals, Environmental, and Terrestrial Analytical Laboratory (METAL Lab) at Arizona State University, water was evaporated and precipitates were re-dissolved in concentrated nitric acid and diluted to a 2 M stock solution. From the stock, a 1 mL aliquot was measured for elemental concentrations on the Thermo Fisher Scientific iCAP quadrupole inductively coupled plasma mass spectrometer (Q-ICP-MS). Strontium was separated through ion chromatography using a Sr-Ca ion exchange resin either with manual columns using Eichrom brand Sr Spec resin (Horwitz et al., 1992) or TODGA resin on the prepFAST automated low pressure chromatography system (Pourmand and Dauphas, 2010; Romaniello et al., 2015). For samples separated on the prepFAST system, elemental concentrations were compared for sample aliquots taken before and after strontium separation, to verify consistent yields were achieved. After separation, the strontium-containing sample was completely evaporated in a Teflon beaker and refluxed with concentrated nitric acid and 30% hydrogen peroxide to oxidize organic complexes eluted from the resin. Samples were then evaporated and reconstituted with 0.32 M nitric acid. Finally, samples were diluted with 0.32 M nitric acid in the amount necessary to achieve a concentration of 50 ppb Sr, based on the elemental concentrations of each sample from analysis on the Q-ICP-MS.
Strontium isotope ratios were measured on the Thermo-Finnigan Neptune multicollector inductively coupled plasma mass spectrometer (MC-ICP-MS) using an Apex sample introduction system. Measurements were made after on-peak blank correction, rejection of voltage outliers greater than 2 standard deviations outside the mean and normalization of 87Sr/86Sr ratios to 86Sr/88Sr of 0.1194 (Romaniello et al., 2015). Prior to any sample analysis, a sequence of SRM 987 from five to 50 ppb was analyzed to ensure measured 87Sr/86Sr was accurate within 0.00002 over the entire range. The analytical sequence included bracketing concentration-matched SRM 987 standard every six samples, and frequent secondary check standards. The SRM 987 bracketing standard was measured as 0.710247 ± 0.000019 (2 SD, n = 63) with a standard error of 0.000001. Purified IAPSO seawater gave an accurate value of 0.709169 compared to the known value of 0.709187 (Romaniello et al., 2015). A secondary check standard was created to simulate a sample with poor chemical recovery, SRM 987 run at 50% of the concentration of the bracketing standard and doped with calcium ICP solution to a Ca/Sr ratio of 500. This provided both an accurate and precise value of 0.710231 ± 0.00004 (2 SD, n = 28) with a standard error of 0.000004. In addition to these analytical check standards, NIST 1400 was purified and measured in parallel to samples to evaluate user and laboratory accuracy and precision. Aliquots of NIST 1400 similar in amount to samples was processed with each batch of samples; over the course of this project, the measured value was 0.713118 ± 0.000053 (2 SD, n = 10) with a standard error of 0.000008. This was in good agreement with the GeoREM value of 0.71314 (Romaniello et al., 2015).
87Sr/86Sr was rounded to four decimal places before spatial and statistical analyses, as this has been found to be sufficient for discriminating between origins for human tissues (Bentley, 2006; Knudson et al., 2016). Then, point values were divided into subgroupings for statistical analysis. Following prior work (Scaffidi and Knudson, 2020), groups were assigned to geo-ecological zones of Pulgar Vidal (1981): coastal (0 – 500 masl), yunga (500 – 2300 masl) and highland (2300+ masl). Point values were also divided into subgroups by geological era (Cenozoic, Mesozoic, and Paleozoic). Finally, point values were divided into groups based on watershed size, which was arbitrarily defined as small (<500,000 ha), medium (500,000 – 1 million ha), and large (>1 million ha). Because 87Sr/86Sr and the residuals were non-normally distributed according to Shapiro–Wilks tests and could not be transformed into normal distributions (see results), the non-parametric Kruskal–Wallis test was used to compare central tendencies and variability between three subgroupings. Mann–Whitney U was used to compare central tendencies between two subgroupings.
Spatial Analysis and Geostatistical Methods
All spatial and geostatistical analysis was completed with the Geostatistical Analyst tools in ArcMap 10.6.2 following prior Andean isoscape modeling methods (Scaffidi and Knudson, 2020; Zimmer-Dauphinee et al., 2020). Sampling location points (n = 263) recorded in degrees, minutes, seconds, or UTM were projected to decimal degrees (WGS, 1984) as a common coordinate space. One sample was from the Maipo Valley in Chile, and due to its distance from the main geographic focus area, it was excluded from geostatistical analysis, leaving 262 Peruvian samples. Points were plotted in ArcMap 10.6.2 and projected to the Albers Equal Area Conic coordinate system, which is necessary for accurate modeling of metric distances with the least distortion possible at the regional scale in South America. The name, distance in meters, and type of the nearest surface water body (i.e., primary river, secondary river, or lake/pond) was determined using the near tool in ArcMap. Following Scaffidi and Knudson (2020, p. 6), elevation at each sampling location was extracted from the 250 m resolution Aster Digital Elevation Model (version 2) (Tachikawa et al., 2011) and coded as coastal (0–500 masl), yunga (mid-elevation, 500–2300 masl), or highland (2300 masl and higher). Finally, each sampling location was spatially joined to DEM-derived drainage basins/watershed boundaries created by the HydroSHEDS project (Lehner and Grill, 2013), primary rivers (Instituto Geográfico Nacional de Perú, 2015), and the bedrock geology model of Schenk et al. (1999).
Before generating the isoscape, an analysis for suspected aberrant 87Sr/86Sr values was carried out. This is important, as a high percentage of the highland Colca Valley samples were collected from puquios or wells made with non-local cements and/or quarried stone. Furthermore, some highland samples were collected from canals flowing from higher to lower field terraces, or from areas with a history of mining contamination. These factors contributed to suspicion of non-representative 87Sr/86Sr in some parts of the highlands, necessitating analytical scrutiny. In order to assess the dataset for the possibility of contamination, following prior archeological isoscape studies (Pellegrini et al., 2016; Scaffidi and Knudson, 2020), spatial outliers identified as high-low or low-high clusters were screened for with the Anselin Local Moran’s I statistic in ArcMap and were trimmed from the dataset prior to generating the isoscape.
Because the trend analysis tool in ArcMap identified a strong east-west trend from less to more radiogenic, Universal Kriging with first order trend removal was used to detrend the dataset, model the residuals, and add the trend back in to the final model. Due to the non-normal distribution (see results), 87Sr/86Sr was modeled using trans-Gaussian kriging and first transformed with the arcsin transformation. The best fit model was selected based on the following criteria: lowest mean standard error between predicted and measured 87Sr/86Sr, a root-mean square and average standard error closest to each other, a mean standardized error closest to 0, and a root-mean-square standardized error closest to 1 (Oliver and Webster, 1990; Oliver and Webster, 2014, 2015). Coincidental points were not averaged before kriging and Supplementary Table S2 presents the parameters used to achieve the best fit model.
The best fit model was assessed in two ways. First, a random 20% subset of the water measurement points was removed, the remaining 80% training subset was modeled on the same parameters as the best fit model and measured vs. predicted 87Sr/86Sr was cross-validated for the 20% test subset. Second, the models were tested with published 87Sr/86Sr archeological human tissue measurements at known geographic coordinates from the archeological meta-analysis compiled by Scaffidi and Knudson (2020). The archeological test set was limited to 12 sites spanning the coast, yunga, and highlands, with samples of 10 or more individuals. Trophy heads and sacrificial victims were excluded, given the likelihood that they represented non-local individuals. The archeological dataset was also limited to sites with a low proportion of inter-quartile range (IQR) outliers relative to the sample size analyzed (see Scaffidi and Knudson, 2020); specifically, sites with an IQR outlier proportion more than 10.0%4 were excluded (n = 493 archeological human 87Sr/86Sr). Each site’s IQR outliers were trimmed from the archeological test dataset prior to plotting the points and extracting predicted 87Sr/86Sr from the kriged isoscape raster, elevation, geological units, and watershed size at each site.
Cross-validation and model tests produced two metrics that are emphasized here: prediction error, which is the difference between measured and predicted 87Sr/86Sr at a given location, and prediction standard error (PSE or uncertainty) at each sampling location or archeological site. Linear regressions were also used to assess the fit between measured water and predicted 87Sr/86Sr and between measured archeological 87Sr/86Sr from the Scaffidi and Knudson (2020) dataset and water isoscape predicted 87Sr/86Sr. The 87Sr/86Sr at each site was considered a match if it fell within the isoscape 87Sr/86Sr prediction ± prediction standard error at that location. Kruskal–Wallis tests were then used to compare 87Sr/86Sr prediction standard errors between site groupings based on elevation, geology, and watershed characteristics. Finally, Chi-squared was used to compare the proportions of archeological samples falling within and beyond the geostatistical prediction standard error at each site to understand whether higher prediction rates were associated with an elevational zone.
Results
Andean Water 87Sr/86Sr Is Related to Elevation, Bedrock Geology, and Watershed Size
Plotting water sampling locations (n = 262)5 demonstrates that water was collected from a wide range of watersheds and underlying geologies. Water was collected from 31 distinct watersheds, reflecting sampling coverage of approximately one-quarter (24.4%) of the 127 watersheds within Peru (Figure 2). The geographic coordinates, elevation, and watershed characteristics of sampling locations are reported in Supplementary Tables S1, S4. Water was collected from locations corresponding to 10 bedrock geological age units (according to the Schenk et al., 1999 model), reflecting sampling coverage of approximately two-thirds (66.7%) of the 15 bedrock age units in Peru.
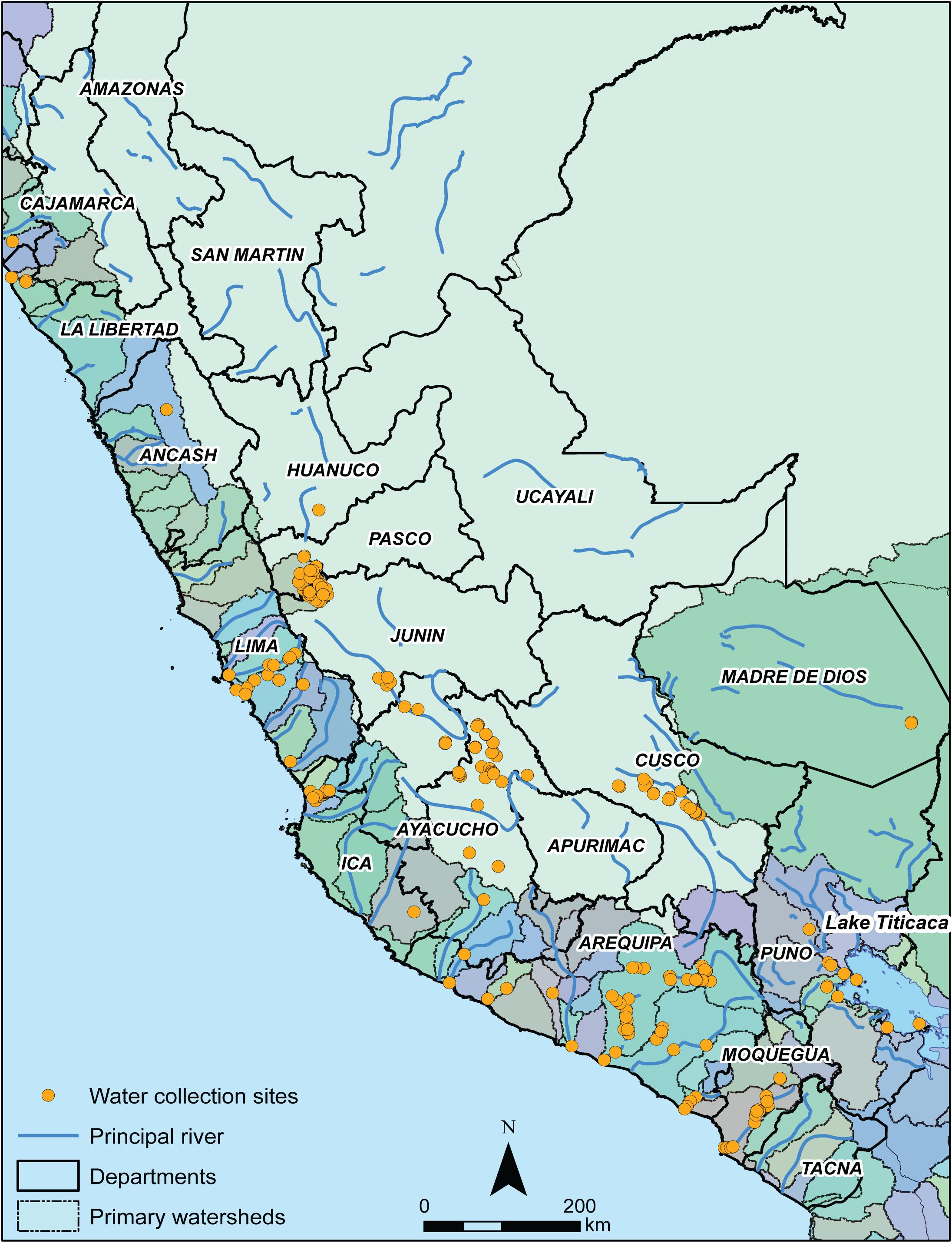
Figure 2. Water sampling locations (n = 262) throughout Peruvian departments (labeled with solid outline) relative to principal rivers and primary watersheds (colored fill with dashed outline).
Elemental concentrations of 88Sr are sufficient to measure 87Sr/86Sr in all samples, ranging from 0.0001 – 0.0113 (M = 0.0007, SD = 0.0019, n = 220) (Supplementary Table S3). New 87Sr/86Sr from this study (n = 220) are reported in Supplementary Table S4. Throughout the entire sample water 87Sr/86Sr ranges from 0.7049 to 0.7227 (M = 0.7081, SD = 0.0027, n = 262). Water 87Sr/86Sr and residuals are non-normally distributed according to Shapiro–Wilk, W(261) = 0.672, p < 0.001. Trend analysis in ArcMap shows a strong east-west trend where 87Sr/86Sr increases longitudinally with elevation and distance from the coast. Water 87Sr/86Sr is lowest near the coasts of Lima, Moquegua, and Nasca. Water 87Sr/86Sr increases to the highest (most radiogenic) around the eastern portion of Lake Junín, the eastern Ayacucho Basin, and the northwestern part of the Cusco Valley near Machu Picchu (Figure 3).
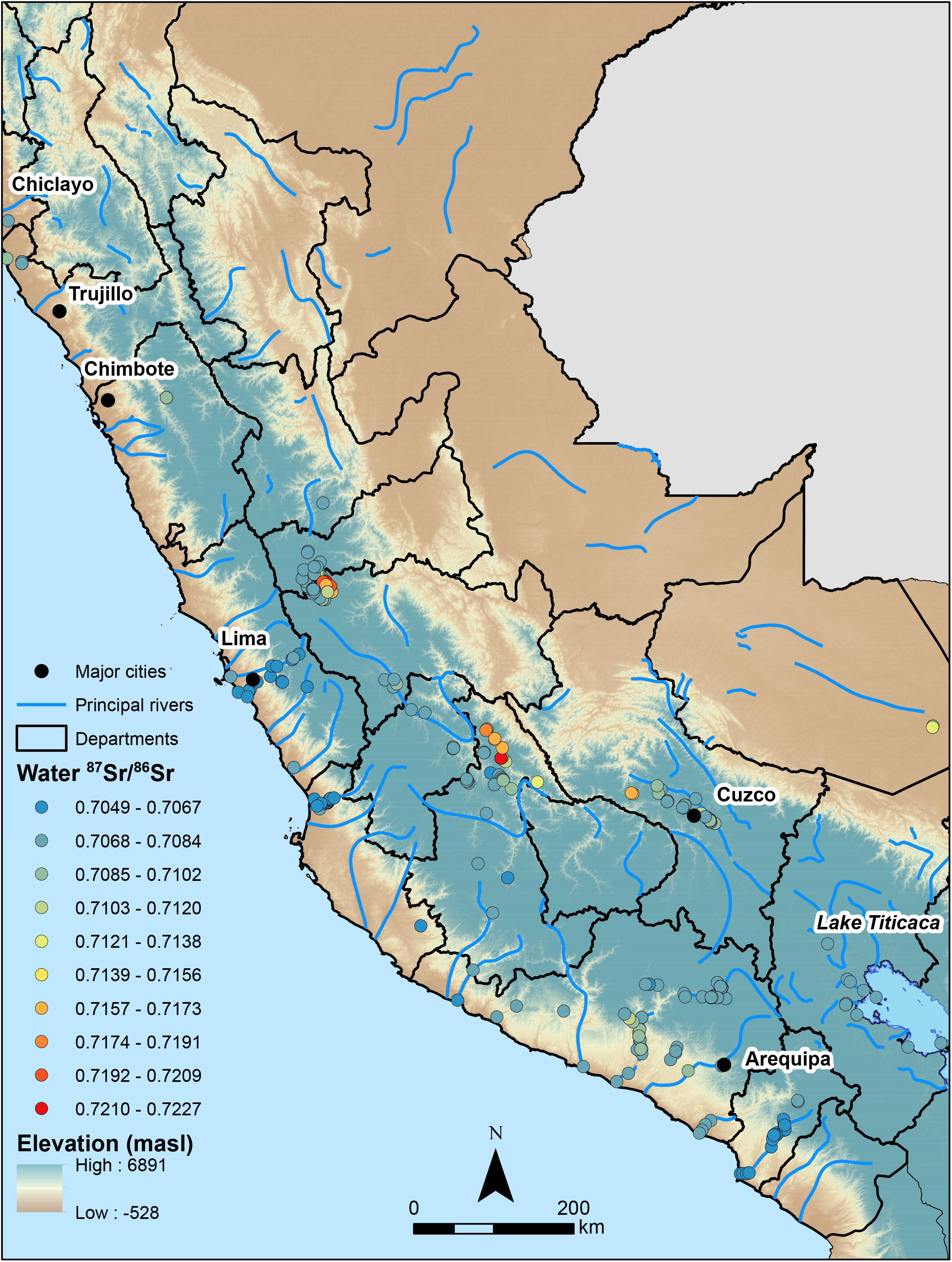
Figure 3. Peruvian water 87Sr/86Sr results, arbitrarily divided into 10 equally sized bins, relative to the locations of major cities, rivers, and elevation at sampling locations (n = 262).
Water 87Sr/86Sr varies according to elevation, watershed size, and underlying bedrock geological age. Highland samples show a significantly different median 87Sr/86Sr (Median = 0.7080, SD = 0.0031, n = 162) than those from the coast (Median = 0.7067, SD = 0.0016, n = 56) and yunga (Median = 0.7068, SD = 0.0013, n = 44), Kruskal–Wallis H(2) = 35.97, p < 0.001 (Figure 4A). Similarly, larger watersheds show significantly higher and more variable 87Sr/86Sr (Median = 0.7080, SD = 0.0029, n = 135) than small ones (Median = 0.7067, SD = 0.0011, n = 62) and medium ones [Median = 0.7070, SD = 0.0029, n = 65, Kruskal–Wallis H(2) = 50.47, p < 0.001] (Figure 4B). Watershed size (hectares) accounts for nearly 60% of the variability in water 87Sr/86Sr (Adjusted R2 = 0.60); as watershed size increases, so does the standard deviation within the watershed. Watershed size accounts for 43.6% of 87Sr/86Sr variation (SD) across a watershed (Adjusted R2 = 0.42). These differences in water 87Sr/86Sr also correspond to the geological age of the underlying bedrock geological units (Supplementary Table S5). Water overlying units deposited in the most distant past, the Paleozoic, are significantly more radiogenic (Median = 0.7087, SD = 0.0034, n = 31) compared to those from the Mesozoic (Median = 0.7076, SD = 0.0027, n = 134) and the most recent Cenozoic [Median = 0.7076, SD = 0.0022, n = 97, Kruskal–Wallis H(2) = 26.45, p < 0.001].
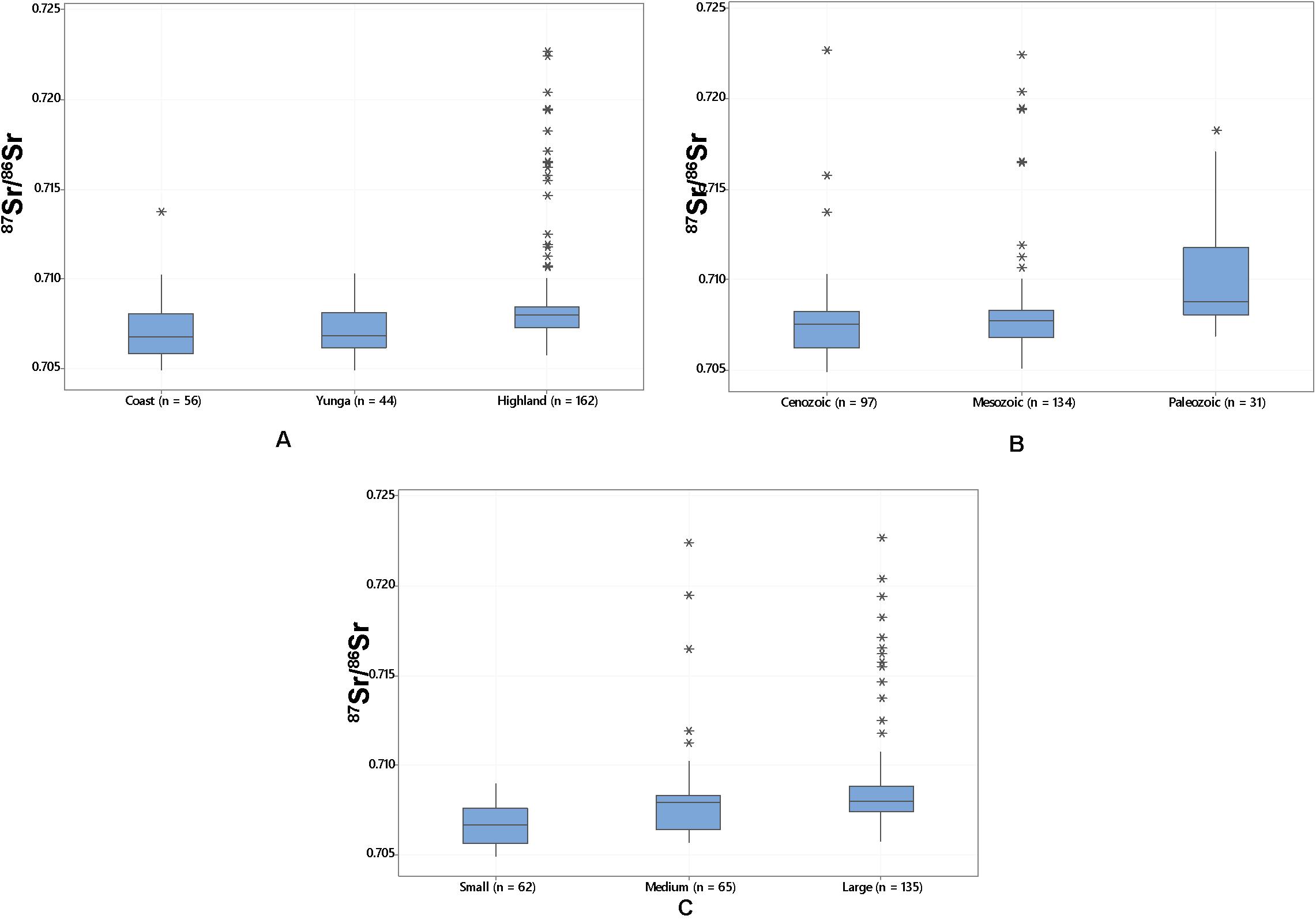
Figure 4. (A) Boxplot of 87Sr/86Sr (n = 262) from coastal, yunga, and highland elevations; (B) Boxplot of 87Sr/86Sr from small, medium, and large watersheds (hectares); and (C) Boxplot of water 87Sr/86Sr overlying geological units from the Cenozoic, Mesozoic, and Paleozoic eras (from most recently to oldest deposited bedrock).
Anselin Local Moran’s I test generates 13 spatial outlier 87Sr/86Sr that were excluded from the isoscape model, since they were suspected to reflect contamination from agricultural runoff or storage in non-native stone wells at those sampling locations. Of these outliers, nine are low 87Sr/86Sr surround by abnormally high 87Sr/86Sr. One of these is an abnormally low value from a high-elevation (3900 masl) stream in the Ayacucho Basin. The remaining eight abnormally low 87Sr/86Sr are from around the western and northern Lake Junín area collected by the Flusche et al. (2005) study. There are also four points with abnormally high 87Sr/86Sr surrounded by more typical low 87Sr/86Sr. Three of these are from the high-elevation Colca Valley of the Department of Arequipa—one is from a natural spring, and two are from man-made irrigation canals. The final high-low outlier is from a stream in Lucanas in the Ayacucho Basin. Interestingly, none of the tap water samples (n = 5) are outliers relative to surrounding sample 87Sr/86Sr, and none of the larger surface water bodies—rivers (n = 133) and lakes (n = 34)—produced spatial outliers.
Geostatistical Water 87Sr/86Sr Model
The trimmed 87Sr/86Sr model (n = 249, Figure 5A) shows a range of 87Sr/86Sr = 0.7049 – 0.7227. Leave-one-out validation of the best-fit isoscape achieves a mean error = < 0.0001 (other model parameters and metrics are reported in Supplementary Table S2). Measured water 87Sr/86Sr explains 99.1% of the variability in predicted 87Sr/86Sr (Adjusted R2 = 0.99, n = 249). As anticipated, the 80% training model (n = 199) performs more poorly, with the random 20% test set (n = 50) of measured 87Sr/86Sr explaining only 31.0% (R2 = 0.31) of the variability in predicted 87Sr/86Sr at corresponding locations. The model semivariogram (Figure 5B) shows no spatial autocorrelation of water 87Sr/86Sr beyond pairwise distances of approximately 10 km apart.
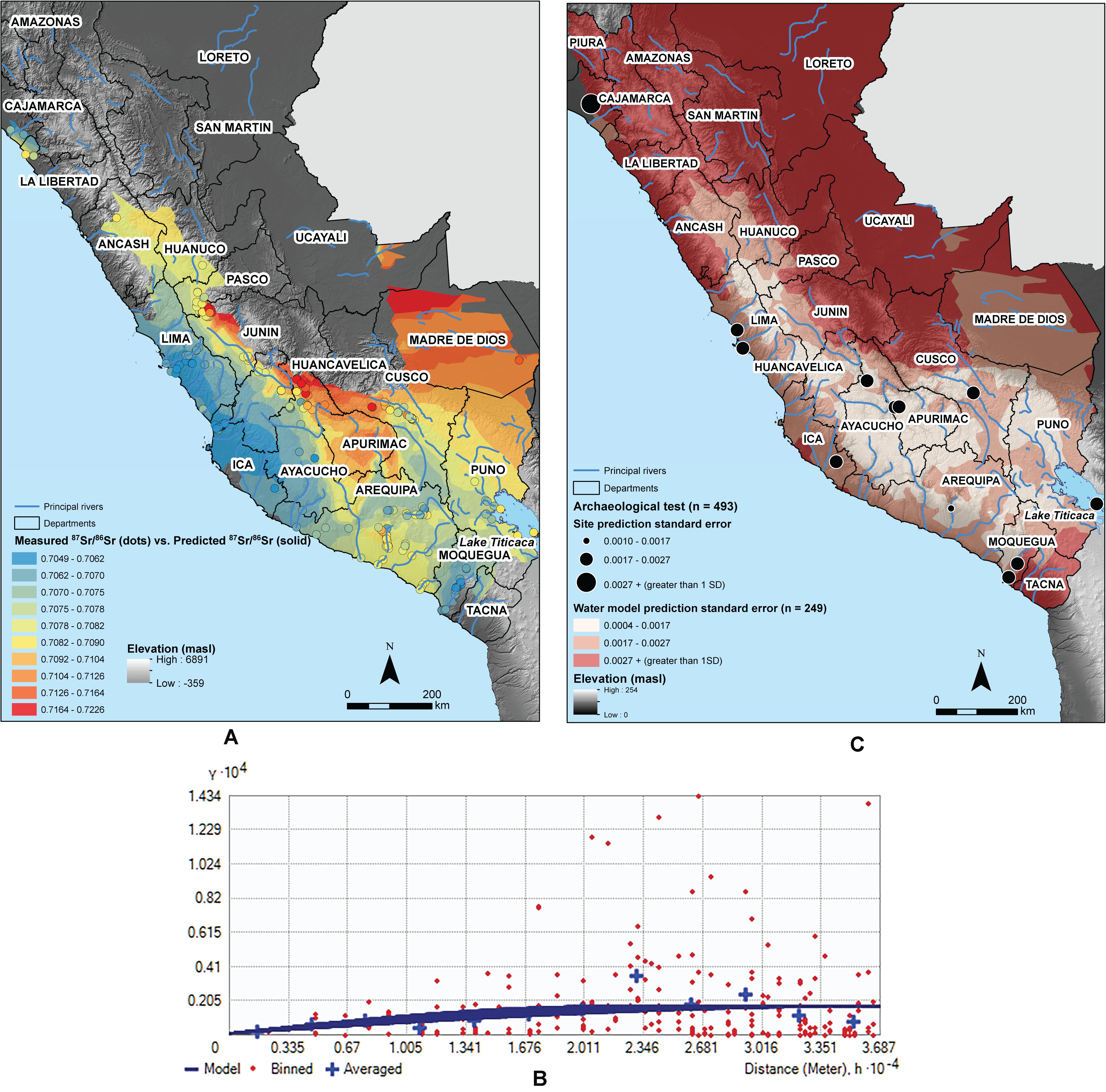
Figure 5. (A) Predictive 87Sr/86Sr isoscape of the trimmed water dataset (n = 249) (solid fill) compared to measured water 87Sr/86Sr (points in graduated colors), clipped to areas where the prediction standard error is less than 1 SD of the dataset (SD = 0.0027), with digital elevation model (250 m ASTER) for reference. Data values are arbitrarily classified into 10 equal bins. (B) The kriging semivariogram shows the absence of spatial autocorrelation between points further away from each other than 10 km. (C) Solid fill indicates error between predicted vs. measured 87Sr/86Sr at water sampling sites (n = 249). Predicted vs. measured 87Sr/86Sr is illustrated by increasing point size at the 12 archaeological test sites (n = 493 test points trimmed of IQR outliers).
Prediction errors for the model range from 87Sr/86Sr = 0.0004 to 0.0052 (Supplementary Table S6; prediction standard error is illustrated in Figure 5C). The geographic distribution shows a lower prediction standard error at highland sites in Cusco, Ayacucho, and Huancavelica, as well as around the Lima coast (Supplementary Table S6). The geographic distribution of prediction standard errors is partially a function of sampling coverage, with lowest errors in the well-sampled Department of Arequipa and neighboring departments in southern Peru (Figure 5B). There are no statistically significant differences in the measured-predicted 87Sr/86Sr errors between groupings based on watershed size, elevational zone, or underlying bedrock geology age.
Testing the isoscape with the archeological samples from 12 sites trimmed of IQR outliers (n = 493) shows mediocre performance of the surface water-only model, explaining about one-tenth (7.0%) of the variation in human skeletal tissue 87Sr/86Sr (Adjusted R2 = 0.07) (Supplementary Table S7). Error between the isoscape predicted and measured 87Sr/86Sr for the archeological test set ranges from 0.0000 – 0.0089 (M = 0.0012, n = 493, see Supplementary Table S7). Prediction standard error (uncertainty) at each site ranges from 0.0001 – 0.0052. The only site with a prediction standard error larger than 1 SD (SD = 0.0027) is the north coast Moche settlement of Huaca Colorada (PSE = 0.0031, n = 16).
Yunga archeological samples have a significantly smaller prediction error (Median = 0.0007, n = 103) than coastal (Median = 0.0012, n = 251) and highland (Median = 0.0014, n = 139) archeological samples according to a Kruskal–Wallis test H(2) = 8.42, p = 0.015 (Figure 6). Prediction error is also significantly lower for samples from sites in large watersheds (Median = 0.0004, n = 222) than for samples from medium (Median = 0.0013, n = 102) and small watersheds (Median = 0.0013, n = 169), according to Kruskal–Wallis [H(2) = 30.35, p < 0.001] (Figure 6). Prediction error is also lower for samples from sites with more recent underlying bedrock deposition dating to the Cenozoic (Median = 0.0004, n = 185) and Paleozoic (Median = 0.0004, n = 74) than for samples with underlying Mesozoic bedrock (Median = 0.0014, n = 234) according to a Kruskal–Wallis, [F(2) = 112.68, p < 0.001] (Figure 6).
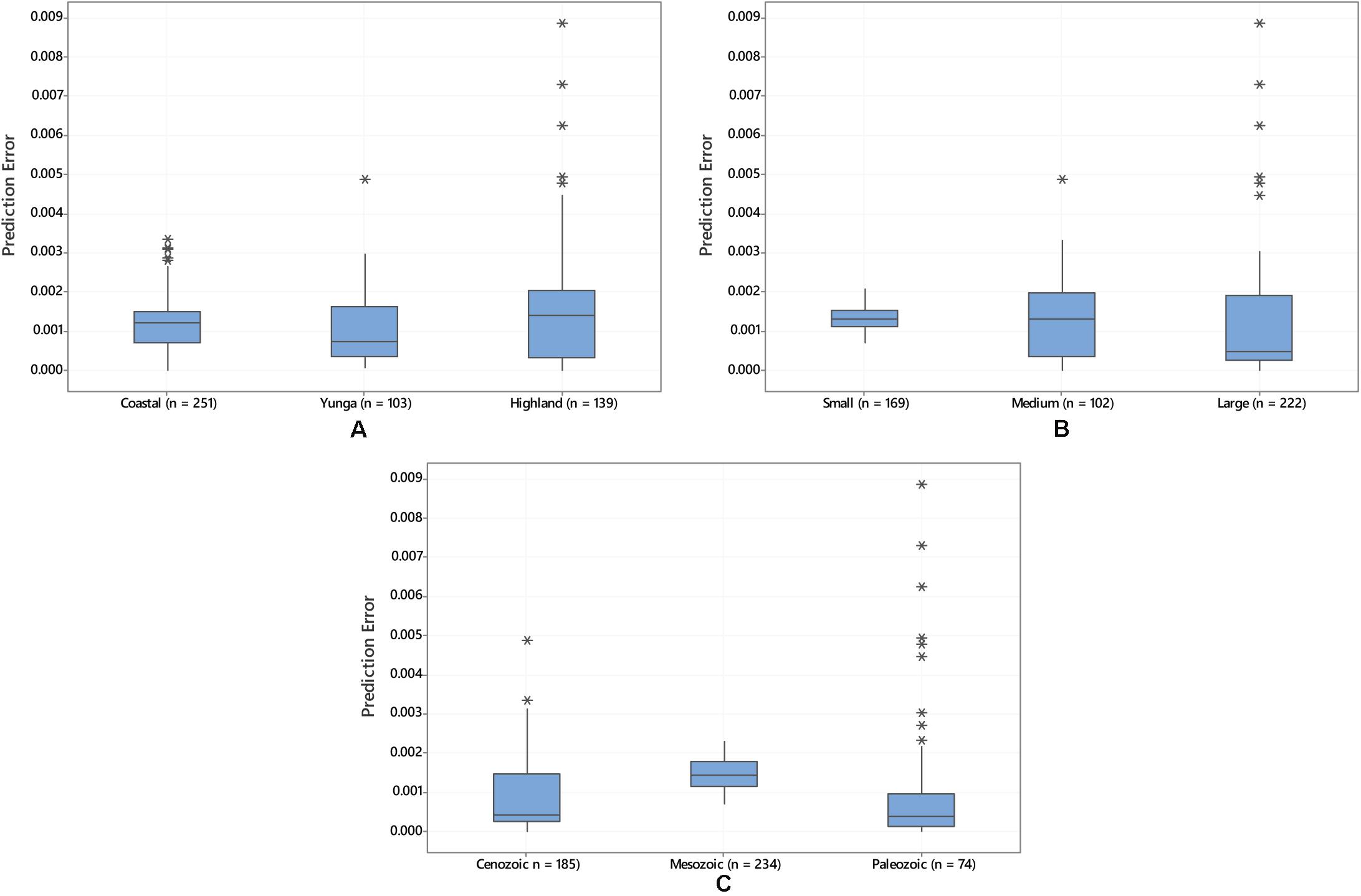
Figure 6. (A) Boxplot of predicted-measured error in 87Sr/86Sr for archeological samples from coastal, yunga, and highland elevations; (B) Boxplot of predicted-measured error in 87Sr/86Sr for archeological samples from small, medium, and large watersheds (in hectares); and (C) predicted-measured error in 87Sr/86Sr for archeological samples overlying geological units dating to the Cenozoic, Mesozoic, and Paleozoic eras (from most recently to oldest deposited bedrock). The predicted-measured errors plotted are from 493 published archeological samples trimmed of IQR outliers.
The archeological 87Sr/86Sr (trimmed of their IQR outliers) are within the site’s prediction standard error of the water 87Sr/86Sr isoscape prediction for 90.0% (451/493) of the test samples (Supplementary Table S8). More archeological samples from the coast (248/251 = 98.9%) fall within their site’s prediction standard error range of the water isoscape than yunga (87/103 = 84.5%) or highland samples (117/139 = 84.2%), and this difference is statistically significant, X2(2) = 35.081, p < 0.001, n = 493. One highland site has a particularly low proportion of skeletal 87Sr/86Sr within the model uncertainty range—none of the nine samples from Turpo fall within the prediction of 0.7092 ± 0.0019 (they are all less radiogenic than expected based on the isoscape prediction) (Supplementary Table S8).
Discussion
Overall, the water isoscape mirrors the east-west distribution of 87Sr/86Sr from less to more radiogenic illustrated by the Scaffidi and Knudson (2020) archeological isoscape, as well as the bedrock geology model of Schenk et al. (1999). Both this water model and the Scaffidi and Knudson (2020) archeological isoscape lack adequate sampling coverage in the north coast and highlands, all rainforest departments (e.g., Loreto, Ucayali, and most of the Madre de Dios), and within regions in between major cities in Peru. Nonetheless, the water 87Sr/86Sr isoscape illustrates important points about the heterogeneity of water in the highlands compared to coastal and yunga water, which has critical implications for understanding the suitability of sampling likely drinking water sources as a reference material for 87Sr/86Sr geolocation of archeological individuals.
Water 87Sr/86Sr Varies According to Elevation, Watershed Size, and Geology
Measured water 87Sr/86Sr fits with expectations from previous water studies in mountainous zones that show more variable 87Sr/86Sr at higher elevations (Frei and Frei, 2011; Bataille and Bowen, 2012; Crowley et al., 2017). The isoscape predictions vary from less to more radiogenic from east to west, with a few areas of highly radiogenic predictions around the highlands of Junín, Cusco, the north coast of La Libertad, and around the northern jungles of the Madre de Dios. The lowest 87Sr/86Sr is predicted for the Lima, Nasca, and Moquegua desert coasts (Figure 5A). Pockets of the highest and lowest projected 87Sr/86Sr are a function of poor sampling in some regions (e.g., the north coast, the Eastern slopes and the jungle, and the central and northern highlands), and should be ground-truthed by future sampling in those areas. Indeed, the isoscape is not reliable in areas where the prediction standard error is higher than the SD of the water samples (SD = 0.0027, Figure 5B). Furthermore, the fact that the water model only explains 30% of the variance in measured water 87Sr/86Sr on cross-validation demonstrates that sampling coverage should be further improved, and also that geostatistical methods may not produce accurate models in these hydrogeologically complex mountainous areas.
Notwithstanding the poor cross-validation results, important patterns emerge that can inform our understanding of bioavailable 87Sr/86Sr in Andean waters. Highland water samples displayed distinct 87Sr/86Sr than those from mid-elevation (yunga) and coastal zones, which demonstrates the homogenization of water strontium sources as rivers drain into the Pacific Ocean. Heterogeneity in water 87Sr/86Sr increases with watershed size, a finding that differs from the findings of Brennan et al. (2019). In the Andes, the largest watersheds are located along the eastern slopes of the Andes and the Amazon Rainforest (Figure 2). These watersheds drain some of the oldest and most variable Precambrian-Devonian geologies (Figure 1) from the Cordillera Oriental, which produce highly variable 87Sr/86Sr water. This is important for several reasons. First, according to this isoscape, 87Sr/86Sr in the Amazon Rainforest should not be homogenous, as has been often suggested by archeologists contemplating 87Sr/86Sr results for items traded into communities from Amazonian groups. In fact, and consistent with recent hydrological analyses that report distinct 87Sr/86Sr for Amazonian rivers (Bouchez et al., 2010; Santos et al., 2015), this study suggests that 87Sr/86Sr signatures are likely to be more heterogeneous on the eastern slopes of the Andes than on the western slopes. On the other hand, these water 87Sr/86Sr results suggest that within smaller watersheds, river and stream water averages strontium from fewer distinct headwaters, so that mixed, large water bodies of lower elevation watersheds should be better proxies for local 87Sr/86Sr than the higher elevation, large watersheds. Of course, it is most prudent to generate localized and mixed models for any region, but this study shows that care should be taken to systematically sample the hydrological system particularly, in the Andean highlands and in the largest watersheds.
Water type should also be considered carefully when devising baseline sampling campaigns. As other studies have argued, it is critical to sample the most likely drinking water sources for a given archeological site (Hodell et al., 2004; Montgomery et al., 2007; Pacheco-Forés et al., 2020; Snoeck et al., 2020). This study’s examination of spatial outlier water 87Sr/86Sr generally agrees with this proposition. Of the 13 spatial outliers detected with possibly aberrant 87Sr/86Sr, none were large water bodies like rivers and lakes. Most were canals and one small stream, which may have been contaminated with fertilizer from agricultural runoff, as some studies have shown (Frei and Frei, 2013; Thomsen and Andreasen, 2019). However, a more recent study by Frei et al. (2020) shows that Danish waters were not contaminated by agricultural liming, and fertilizers were fully contained in the soils. Modern and ancient Peruvian fertilization practices and their impact on soil and water 87Sr/86Sr need to be studied in-depth to understand whether this impacts the local baseline. The other spatial outliers in the present study were from natural springs or man-made wells. Springs might reflect distinct groundwater 87Sr/86Sr, while stored waters from wells or stone-lined canals may take on the geological signature of their containers, which may themselves not be local. The fact that these outliers were all from highland locations could reflect the intensity of agriculture and the proximity of fields to water sources in the highlands that make them more likely to be contaminated. Mining contamination is certainly another factor that could contribute to abnormal 87Sr/86Sr around Lake Junín (Rodbell et al., 2014), although it does not explain the unusually high 87Sr/86Sr in the Colca Canyon. Spatial outlier analysis suggests that springs, wells, and canals could reflect non-representative 87Sr/86Sr. However, it is possible that seemingly abnormal well and canal 87Sr/86Sr represents true variability of bedrock strontium contributions—these areas need to be more systematically sampled to understand the complexity of the local geohydrological system.
The fact that none of the five tap water samples were spatial outliers demonstrates that tap-derived drinking water 87Sr/86Sr is close to that of the source waters they are diverted from. This pattern may have been different if tap waters from very large cities like Lima, Arequipa, and Trujillo were sampled, but in Chiclayo, Chincha, and Cusco, tap samples are within the range of nearby river 87Sr/86Sr variation. Because there were no archeological samples in the archeological test dataset that were directly comparable at the tap water sampling sites, it remains unclear whether tap water could be useful for geolocation of human skeletons. A thorough understanding of Peruvian water management practices and water transportation materials would be necessary to conduct a large-scale tap water provenience study like those conducted in the US by Chesson et al. (2012) and Tipple et al. (2018). Another consideration is that wild animals would likely have consumed primarily water from rivers or lakes, and not the tap water, smaller streams, springs, and canals closer to human settlements. Therefore, it is suspected that water contributions of wild faunal protein sources in the human food web would be better reflected by preferentially sampling large water bodies outside of anthropogenically altered settlements. Further testing of different modern and ancient drinking water sources throughout the Andes is needed to assess the utility of these dietary substrates for provenience human skeletons.
Peruvian Water 87Sr/86Sr Isoscape Predicts One-Tenth of the Variation in Archeological Human Tissues
At a broad scale, the variation in water 87Sr/86Sr from the Peruvian Andes is similar to that observed in the meta-analysis of human archeological tissues across the Andes by Scaffidi and Knudson (2020). The median 87Sr/86Sr of the water samples from this study (Median = 0.7077, n = 262) is not statistically distinct from the archeological human 87Sr/86Sr from the Andes aggregated by Scaffidi and Knudson (2020) [Median = 0.7077, n = 1390, Mann–Whitney U W(1), p = 0.315]. The present study is limited as it does not model rainwater in areas with significant precipitation, or groundwater or fog-water 87Sr/86Sr, which may have been the source of a significant amount of imbibed water in arid coastal regions where rivers frequently run dry or underground. The water-only isoscape performs well overall at predicting archeological 87Sr/86Sr—archeological 87Sr/86Sr falls within the water isoscape prediction ± prediction standard error for 90% of the IQR-outlier-trimmed archeological samples. This 90% prediction accuracy exceeds the 70% reported by Lengfelder et al. (2019) for a mixing model in the Alps based on soil, dust, groundwater, drinking water, vegetation, and fauna. The high prediction accuracy of the water-only model makes sense given that the drinking water from riverine sources reflects a mix of upstream bedrock weathering, soil and alluvial runoff, and atmospheric strontium (Whipkey et al., 2000; Hodell et al., 2004; Bentley, 2006; Bataille and Bowen, 2012; Crowley et al., 2017). However, the poor correlation between the measured and predicted 87Sr/86Sr still leaves most of the variation in human archeological 87Sr/86Sr to be accounted for from dietary sources of strontium.
Since R2 values rarely approach a one-to-one correlation when modeling complex human phenomena, it is informative that 87Sr/86Sr alone accounts for nearly one-tenth of the variation in the archeological human test set 87Sr/86Sr trimmed of IQR outliers. The poor test performance could be explained by the fact that the water model accounts for 30% of the variation in water 87Sr/86Sr across the landscape when cross-validated with random water points in the 20% validation set. These low R2 values are undoubtedly influenced by shortcomings of the geostatistical approach for modeling bioavailable strontium, including its reliance on Euclidean distances to predict 87Sr/86Sr (Bataille et al., 2018, 2020). However, it is important to note that the fit of isoscapes to archeological or human data is always assessed based on an interval from the prediction—linear fit statistics (R2) are generally not reported for human studies. This is because, as Lengfelder et al. (2019, p. 246) discuss with respect to assessing the fit between isoscape predictions and archeological values, “isotopes in bioarchaeology will always remain proxy data and how much of this ‘noise’ can be tolerated depends on the specific hypothesis.” Nonetheless, regression-based methods are necessary to improve higher accuracy predictions than are allowed by geostatistical models (Bataille et al., 2018, 2020; Hoogewerff et al., 2019). Predicting a continuous surface from linear features like rivers is also problematic, as spatial autocorrelation is constrained by distance to those rivers (Brennan et al., 2016). Particularly in the yunga and coastal Andes where settlements were constrained to river valleys, methods that constrain predictions to networks within specific watersheds (Bataille et al., 2014; Brennan et al., 2016, 2019) would likely improve prediction accuracy for sites located within a walking-distance proximity to the river networks accessed by archeological human residents.
The model will also be improved with the incorporation of bioavailable 87Sr/86Sr from faunal and plant sources in local food webs that were likely consumed by inhabitants at different sites, as well as collection of baseline data from under-sampled areas. Care must be used in modeling animal and plant strontium sources, however, given that non-local plants and animals likely played an important role in many Andean groups with access to far-reaching and complex exchange networks. In particular, camelids like alpacas and llamas are common protein sources in the Andes—and many of these moved from the highlands to the coast and/or vice-versa throughout their lifetimes (Núñez and Dillehay, 1979; Browman, 1980; Thornton et al., 2011; deFrance et al., 2016; Tripcevich and Capriles, 2016; Mader et al., 2018), so that individuals with diets high in camelid protein might demonstrate correspondingly complex 87Sr/86Sr that does not necessarily echo the landscape 87Sr/86Sr of origin for human end-members. Similarly, marine proteins like dried fish were often exchanged over great distances into mid-elevations and highlands (Lynch, 1983; Quilter and Stocker, 1983; Hastorf, 2003), which could drive radiogenic isotope signatures upwards in communities with sustained and significant access to coastal exchange routes. Even in places with access to traded foods, though, community members may not have uniformly consumed those imported goods. Furthermore, communities may have altered their local production and import of different foods based on cultural or environmental conditions. In other words, faunal distributions and subsistence practices in many parts of the Andes varied at different temporal resolutions: seasonally, from year to year, or over many generations. At present, macrobotanical and stable isotopic reconstructions of local diets are limited from the 12 archeological test sites. To more accurately model the bioavailable Sr of local dietary catchments, it is critical that archeological evidence-based local food webs and water sources are identified, sampled, and integrated into multi-scalar models. In practice, this means that future efforts at 87Sr/86Sr mixing models in the Andes will best be achieved through local and collaborative, supra-regional scale process-based models, informed and contextualized by combining ethnohistoric and archeological information on diets with geological and hydrological data. While beyond the scope of this study, multiple stable isotope data points are essential for developing appropriately weighted 87Sr/86Sr models of past dietary catchments for addressing bioarchaeological and zooarchaeological questions about mobility practices.
The water 87Sr/86Sr isoscape improves equifinality in well-sampled regions that look homogeneous in the archeological isoscape, like within Arequipa, Cusco, and the Lake Titicaca areas (Scaffidi and Knudson, 2020, p. 13). In particular, the model has a low uncertainty (Figure 5B) and a low prediction standard error (Supplementary Tables S7, S8) for samples at Beringa in the Majes Valley, and at Chokepukio in Cusco, where both the number of archeological samples and water samples are high. In these areas, water 87Sr/86Sr and the model predictions can be relied on to identify non-locals, but in areas with uncertainty higher than the water 87Sr/86Sr standard deviation (Figure 5B), the model cannot be relied on for this and further sampling is needed. The high uncertainty in most of the Andes reflects the absence of sampling throughout areas that are more difficult to access and beyond the research focus of this study’s authors in southern Peru. Due to this high uncertainty, it is emphasized here that this preliminary model cannot be relied on for geolocation, and future models will seek to incorporate additional dietary proxies for bioavailable Sr, increased the geographic scope sampling, and incorporate regression-based methods to improve the model.
The fit between archeological samples and the water isoscape prediction is better in coastal and yunga regions than in the highlands. Yunga waters show the lowest prediction standard error (Supplementary Table S6) due to homogenized, well-mixed water and high sample numbers at these elevations. Coastal water is also well-mixed, but sea-spray contributions and the preponderance of salt-rich seafood in diets may have masked local drinking water signatures in several communities (Sillen and Kavanagh, 1982; Bentley, 2006). At the coastal settlements of Ancón and Huaca Colorada where a few individuals show 87Sr/86Sr not within the site prediction standard error range (Supplementary Tables S7, S8), reliance on seafood may have driven human 87Sr/86Sr higher than the local drinking water prediction. Indeed, carbon and nitrogen data from Ancón show they consumed primarily marine proteins (Slovak and Paytan, 2011). Carbon and nitrogen data are not yet published for Huaca Colorada, but given iconographic evidence for reliance of north coastal Moche people on seafood (Donnan and McClelland, 1999; see, e.g., Bourget and Jones, 2009), it is likely that Huaca Colorada’s inhabitants relied heavily on marine products, just as other coastal people did (Knudson et al., 2007, 2015; Slovak and Paytan, 2011). In contrast, at the coastal site of Armatambo, all of the human samples fell within the isoscape prediction ± prediction standard error, even though they consumed a high proportion of marine foods (Marsteller et al., 2017). At Armatambo, measured 87Sr/86Sr have a mean error of 0.0012 more radiogenic than the drinking water prediction, which may reflect a uniform elevation of 87Sr/86Sr consistent with greater consumption of marine products with 87Sr/86Sr around 0.7092. Reliance on water storage and underground aquifers may also have contributed to higher 87Sr/86Sr at these sites. Since coastal sites are generally located along arid desert seashores with little year-round freshwater, coastal and desert-dwelling people often relied on stored water or used underground wells or aqueducts (Netherly, 1984; Denevan, 2001; Schreiber and Lancho Rojas, 2003; Lane, 2014; Scaffidi, 2019). Furthermore, the use of guano fertilizers at might have driven 87Sr/86Sr up. Differences in the proportion of marine foods, and/or guano, and/or variable amounts of sea spray could contribute to prediction error, and these factors should be examined in future models.
Highland waters have the highest prediction error rates (Supplementary Table S6), which likely reflects true heterogeneity in the 87Sr/86Sr of water and underlying geology. At Ch’isi on Lake Titicaca, the fact that all archeological samples were within the isoscape prediction ± prediction standard error could confirm that most people obtained drinking water from the lake. In contrast, the failure of the water isoscape to predict any of the archeological samples from the highland site of Turpo in Andahuaylas might reflect that these people consumed water primarily from a spring or small lake that deviates from the local river system. The poor fit is to be expected when there were no water samples from this area, but the limits of geostatistical modeling and the absence of dietary substrate materials in the model are also likely at play. Overall, the higher variability of highland water 87Sr/86Sr and the potential of high-87Sr/86Sr seafood masking to impact coastal 87Sr/86Sr demonstrates that localized conditions and diets must be modeled alongside of supra-regional patterns in bioavailable substrates. Given their greater variability, localized highland water models have immense potential to discriminate between different geographic origins if geo-hydrological systems, other isotopic proxies for diet are analyzed, and likely drinking water sources are understood and systematically sampled.
Sample Collection and 87Sr/86Sr Mixing Model Development in the Andes
Systematic sampling and coordinating data collected in different coordinate systems with varying techniques remain the most significant deficiencies in baseline isoscape modeling in the Andes. Particularly along Andean coastal valleys, it is often impossible to travel in a grid, sampling at every 500 km2, as recommended by prior studies (Garrett et al., 1990; Fordyce et al., 1993). Furthermore, modern roads do not always pass where ancient ones like the Qhapaq Ñan (the Inca and pre-Inca road system) did, which makes many archeological sites and their isotopic catchments difficult to access. Due to these factors, more accessible archeological sites around larger cities are disproportionately well-sampled compared to more remote sites (Figure 1).
To advance regional and localized baseline models, a unified, cloud-based approach would maximize efficiency in the data collection and data aggregation phases and predictive accuracy of the isoscape products. One challenge to this project was aggregating spreadsheets from multiple field sampling excursions which collected different types of locational attributes in different coordinate systems and at different spatial resolutions—points not collected in the APU relational database required manual spreadsheet consolidation, which amplified opportunities for error. Another challenge is that environmental co-variates that could be analyzed for process-based modeling, such as temperature, soil type, or contamination levels, were not collected. Widespread adoption of a single cloud-based, collaborative database with environmental co-variates suitable for the region, such as the APU database generated by Scaffidi with the ArcCollector app (ESRI), is one excellent option for multi-user collection. With an inexpensive external GPS receiver, this database can be deployed on mobile IOS and Android devices, standardizing data collection and aggregation. Data is uploaded to the cloud whenever a wi-fi signal is detected, so users can plan routes around extant points. The 2019 APU team found that even if base maps were not downloaded, Peruvian cellular data enabled sufficient bandwidth for caching base map tiles. Data is stored in a web map at ArcGIS Online, which can be viewed, edited, and downloaded by users with institutional ESRI subscriptions. The APU water sampling map can be viewed at: https://arcg.is/1bj4CT; prospective users can be added to the web map by emailing Scaffidi. Collaborative databases like those developed in other world regions (Salesse et al., 2018; Willmes et al., 2018; Hermann et al., 2020) are absolutely critical for fully characterizing isotopic variability in this difficult-to-traverse region.
This study shows that a water 87Sr/86Sr isoscape is useful at a coarse scale for discerning between samples from highland, mid-elevation, and coastal provenience in the Peruvian Andes—particularly when known trade and migration networks are in well-sampled areas, pass between elevational zones, and are implicated by the research question of a given study. The greater variability in highland locations and larger watersheds calls for proportionally more baseline sampling when investigating archeological populations from these geo-hydrologically complex high-elevation zones. This study demonstrates the need for a mixing model to explain the other nine-tenths of variation in archeological human 87Sr/86Sr, and the need for standardized, collaborative sampling and data sharing. It also demonstrates the importance of analyzing environmental materials alongside paleodietary evidence to understand dietary-driven deviance from the environmental baseline. Future research will analyze bioavailable strontium from plant and faunal baselines throughout the Peruvian Andes, with the eventual goal of incorporating these multiple bioavailable strontium sources into a new, process-based mixing model for the probabilistic geolocation of archeological human 87Sr/86Sr.
Data Availability Statement
All datasets presented in this study are included in the article/Supplementary Material.
Author Contributions
BS funded (through grants to BS and to BS, TT, and KK) and analyzed the majority of the samples, collected the samples, and wrote the manuscript. TT collected the samples and revised the manuscript. GG assisted with isotopic analysis and contributed paragraphs on analytical and instrument methods. AA and LG funded (through a grant to AA) and analyzed the some samples. AA revised the manuscript. SM, AD, and ES collected the samples and revised the manuscript. All authors contributed to the article and approved the submitted version.
Funding
The National Science Foundation SBE Postdoctoral Fellowship (award # 18090470) to BS funded collection and isotopic analysis of water samples. A Vanderbilt University Research Scholar Grant to TT funded additional field collection of samples. A Collaborative Research Grant from the Center for Bioarchaeological Research at Arizona State University to BS, TT, and KK funded analysis of a portion of the water samples. The analysis of seven other samples was supported by the Wenner-Gren Dissertation Fieldwork Grant to AA (award # 9580). The collection of Ilo Valley samples was funded by the Graduate and Professional Student Association Graduate Research Support Program Grant at Arizona State University to ES. Finally, the open access fee was funded by BS, KK, and TT.
Conflict of Interest
The authors declare that the research was conducted in the absence of any commercial or financial relationships that could be construed as a potential conflict of interest.
Acknowledgments
Water sample collection and analysis was supported primarily by: a National Science Foundation SBE Postdoctoral Fellowship to BS (award number 1809470), a Collaborative Research Grant from the Center for Bioarchaeological Research at Arizona State University to BS, TT, and KK, and a Vanderbilt University Research Scholar Grant to TT. The analysis of seven samples was supported by the Wenner-Gren Dissertation Fieldwork Grant to AA (award number 9580). The collection of Ilo Valley samples was funded by the Graduate and Professional Student Association Graduate Research Support Program Grant at Arizona State University to ES. Vanderbilt University BSIRL and Natasha Vang facilitated a great deal of the sample collection, organization, and shipping to Scaffidi for analysis. In addition to BSIRL, ACL, and APU students, the following individuals also graciously assisted with water sampling: Manuel Mamaní Calloapaza, Jacob Bongers, Dave Reid, Jake Dean, Alex Menaker, Matt Biwer, Donna Nash, and Kirk Costion. Furthermore, the following individuals allowed us to use their archeological research projects as home bases for sample collection: Rebecca Bria, Véronique Bélisle, Willy Yépez Álvarez, Justin Jennings, and Donna Nash. We thank researchers at the METAL Laboratory at Arizona State University for assisting with instrumentation and data processing: Trevor Martin, Tyler Goepfert, and Natasha Zolotova. Andrew Zipkin of the Archaeological Chemistry Laboratory at Arizona State University was a limitless source of feedback about chemical and spatial analysis of strontium, and the SPATIAL short course (Isotopes in Spatial Systems) from the Inter-university Training for Continental-scale Ecology group at the University of Utah provided isoscape training for Scaffidi. Michael Scaffidi assisted with figure creation. Finally, we appreciate the invitation to participate in this special edition; we also thank the two reviewers and the editors for their feedback which greatly improved the manuscript.
Supplementary Material
The Supplementary Material for this article can be found online at: https://www.frontiersin.org/articles/10.3389/fevo.2020.00281/full#supplementary-material
Footnotes
- ^ This study focuses on predicting human 87Sr/86Sr from water 87Sr/86Sr measurements, but the water model could also be applied to flora and non-human fauna if the water physiology and strontium biogeochemistry of those organisms is well-understood.
- ^ Water management practices in the pre-Hispanic Andes were intensive—coastal and highland regions utilized expansive irrigation systems to divert water into agricultural fields and settlements (Netherly, 1984; Denevan, 2001; Billman, 2002; Lane, 2014) or to tap underground water sources (see discussion of Nasca coastal aqueducts and well systems in Schreiber and Lancho Rojas, 2003). Regardless of this hydraulic expertise, in many areas, much of diverted water would have been sourced from local surface or groundwater. Thus, even if ancient Peruvians consumed most of their water from irrigation-fed fountains, water 87Sr/86Sr should largely reflect local drinking water sources.
- ^ As reflected in Supplementary Table S1, samples were collected by researchers from the Archaeological Chemistry Laboratory (Center for Bioarchaeological Research, School of Human Evolution and Social Change, Arizona State University), the Vanderbilt University Bioarchaeological and Stable Isotope Research Laboratory (Department of Anthropology), and the Andean Paleomobility Unification Project directed by the first author, in collaboration with both laboratories.
- ^ Sites with high proportions of IQR outliers include places like the Inca site of Machu Picchu, where studies have shown high variation in 87Sr/86Sr because they were settled by people from diverse geographic origins (Turner et al., 2009; see discussion in Scaffidi and Knudson, 2020). Samples from settlements comprised of a high relative proportion of probable first-generation migrants would not be expected to match the local environmental baseline as communities comprised of mostly locally born individuals, and were thus excluded from the archeological test set.
References
Åberg, G. (1995). The use of natural strontium isotopes as tracers in environmental studies. Water Air Soil Pollut. 79, 309–322. doi: 10.1007/BF01100444
Adams, S., Grün, R., McGahan, D., Zhao, J. X., Feng, Y., Nguyen, A., et al. (2019). A strontium isoscape of north-east Australia for human provenance and repatriation. Geoarchaeology 34, 231–251. doi: 10.1002/gea.21728
Alaica, A. K., González La Rosa, L. M., and Knudson, K. J. (2020). Creating a body-subject in the Late Moche Period (CE 650-850). Bioarchaeological and biogeochemical analyses of human offerings from Huaca Colorada, Jequetepeque Valley, Peru. World Archaeol. 52, 49–70. doi: 10.1080/00438243.2019.1743205
Aubert, D., Probst, A., Stille, P., and Viville, D. (2002). Evidence of hydrological control of Sr behavior in stream water (Strengbach catchment, Vosges mountains, France). Appl. Geochem. 17, 285–300. doi: 10.1016/S0883-2927(01)00080-4
Bailey, S. W., Hornbeck, J. W., Driscoll, C. T., and Gaudette, H. E. (1996). Calcium inputs and transport in a base-poor forest ecosystem as interpreted by Sr isotopes. Water Resourc. Res. 32, 707–719. doi: 10.1029/95WR03642
Barberena, R., Durán, V. A., Novellino, P., Winocur, D., Benítez, A., Tessone, A., et al. (2017). Scale of human mobility in the southern Andes (Argentina and Chile): a new framework based on strontium isotopes. Am. J. Phys. Anthropol. 164, 305–320. doi: 10.1002/ajpa.23270
Barberena, R., Tessone, A., Cagnoni, M., Gasco, A., Durán, V., Winocur, D., et al. (2019). Bioavailable strontium in the Southern Andes (Argentina and Chile): a tool for tracking human and animal movement. Environ. Archaeol. doi: 10.1080/14614103.2019.1689894
Bataille, C. P., and Bowen, G. J. (2012). Mapping 87Sr/86 Sr variations in bedrock and water for large scale provenance studies. Chem. Geol. 304, 39–52. doi: 10.1016/j.chemgeo.2012.01.028
Bataille, C. P., Brennan, S. R., Hartmann, J., Moosdorf, N., Wooller, M., and Bowen, G. J. (2014). A geostatistical framework for predicting variations in strontium concentrations and isotope ratios in Alaskan rivers. Chem. Geol. 389, 1–15. doi: 10.1016/j.chemgeo.2014.08.030
Bataille, C. P., Crowley, B. E., Wooller, M. J., and Bowen, G. J. (2020). Advances in global bioavailable strontium isoscapes. Palaeogeogr. Palaeoclimatol. Palaeoecol. 2020:109849. doi: 10.1016/j.palaeo.2020.109849
Bataille, C. P., Laffoon, J., and Bowen, G. J. (2012). Mapping multiple source effects on the strontium isotopic signatures of ecosystems from the circum-Caribbean region. Ecosphere 3, 1–24. doi: 10.1890/ES12-00155.1
Bataille, C. P., von Holstein, I. C., Laffoon, J. E., Willmes, M., Liu, X.-M., and Davies, G. R. (2018). A bioavailable strontium isoscape for Western Europe: a machine learning approach. PLoS One 13:e0197386. doi: 10.1371/journal.pone.0197386
Beard, B., and Johnson, C. (2000). Strontium isotope composition of skeletal material can determine the birth place and geographic mobility of humans and animals. J. Forensic Sci. 45, 1049–1061. doi: 10.1520/JFS14829J
Bellido, B. E., Navarez, S., and Simons, F. D. (1956). Mapa Geológica del Perú. Lima: La Sociedad Geológica del Peru.
Bentley, R. A. (2006). Strontium isotopes from the earth to the archaeological skeleton: a review. J. Archaeol. Method Theory 13, 135–187. doi: 10.1007/s10816-006-9009-x
Bentley, R. A., and Knipper, C. (2005). Geographical patterns in biologically available strontium, carbon and oxygen isotope signatures in prehistoric SW Germany. Archaeometry 47, 629–644. doi: 10.1111/j.1475-4754.2005.00223.x
Bentley, R. A., Price, T. D., and Stephan, E. (2004). Determining the ‘local’87Sr/86Sr range for archaeological skeletons: a case study from Neolithic Europe. J. Archaeol. Sci. 31, 365–375. doi: 10.1016/j.jas.2003.09.003
Billman, B. R. (2002). Irrigation and the origins of the southern Moche state on the north coast of Peru. Latin Am. Antiq. 13, 371–400. doi: 10.2307/972222
Bouchez, J., Lajeunesse, E., Gaillardet, J., France-Lanord, C., Dutra-Maia, P., and Maurice, L. (2010). Turbulent mixing in the Amazon River: the isotopic memory of confluences. Earth Planet. Sci. Lett. 290, 37–43. doi: 10.1016/j.epsl.2009.11.054
Bourget, S., and Jones, K. L. (2009). The Art and Archaeology of the Moche: An Ancient Andean Society of the Peruvian North Coast. Austin, TX: University of Texas Press.
Bowen, G. (2010). Isoscapes: spatial patterns in isotopic biogeochemistry. Annu. Rev. Earth Planet. Sci. 38, 161–187. doi: 10.1146/annurev-earth-040809-152429
Bowen, G. J., and Revenaugh, J. (2003). Interpolating the isotopic composition of modern meteoric precipitation. Water Resour. Res. 39, 1–13. doi: 10.1029/2003WR002086
Brennan, S. R., Schindler, D. E., Cline, T. J., Walsworth, T. E., Buck, G., and Fernandez, D. P. (2019). Shifting habitat mosaics and fish production across river basins. Science 364, 783–786. doi: 10.1126/science.aav4313
Brennan, S. R., Torgersen, C. E., Hollenbeck, J. P., Fernandez, D. P., Jensen, C. K., and Schindler, D. E. (2016). Dendritic network models: improving isoscapes and quantifying influence of landscape and in-stream processes on strontium isotopes in rivers. Geophys. Res. Lett. 43, 5043–5051. doi: 10.1002/2016GL068904
Browman, D. L. (1980). Tiwanaku expansion and altiplano economic patterns. Estud. Arqueológ. 5, 107–120.
Buhl, D., Neuser, R., Richter, D., Riedel, D., Roberts, B., Strauss, H., et al. (1991). Nature and nurture: environmental isotope story of the river Rhine. Naturwissenschaften 78, 337–346. doi: 10.1007/BF01131605
Burton, J. H., and Price, T. D. (2013). “Seeking the local 87Sr/86Sr ratio to determine geographic origins of humans,” in Across the Alps in Prehistory, eds R. A. Armitage and J. H. Burton (Cham: Spring), 27–48. doi: 10.1021/bk-2013-1147.ch018
Burton, J. H., and Wright, L. E. (1995). Nonlinearity in the relationship between bone Sr/Ca and diet: paleodietary implications. Am. J. Phys. Anthropol. 96, 273–282. doi: 10.1002/ajpa.1330960305
Capo, R. C., Stewart, B. W., and Chadwick, O. A. (1998). Strontium isotopes as tracers of ecosystem processes: theory and methods. Geoderma 82, 197–225. doi: 10.1016/S0016-7061(97)00102-X
Chesson, L. A., Tipple, B. J., Mackey, G. N., Hynek, S. A., Fernandez, D. P., and Ehleringer, J. R. (2012). Strontium isotopes in tap water from the coterminous USA. Ecosphere 3, 1–17. doi: 10.1890/ES12-00122.1
Comar, C., Russell, R. S., and Wasserman, R. (1957a). Strontium-calcium movement from soil to man. Science 126, 485–492. doi: 10.1126/science.126.3272.485
Comar, C., Wasserman, R., Ullberg, S., and Andrews, G. (1957b). Strontium metabolism and strontium-calcium discrimination in man. Proc. Soc. Exper. Biol. Med. 95, 386–391. doi: 10.3181/00379727-95-23231
Copeland, S. R., Cawthra, H. C., Fisher, E. C., Lee-Thorp, J. A., Cowling, R. M., Le Roux, P. J., et al. (2016). Strontium isotope investigation of ungulate movement patterns on the pleistocene paleo-agulhas plain of the greater cape floristic region, South Africa. Q. Sci. Rev. 141, 65–84. doi: 10.1016/j.quascirev.2016.04.002
Copeland, S. R., Sponheimer, M., Lee-Thorp, J. A., de Ruiter, D. J., le Roux, P. J., Grimes, V., et al. (2010). Using strontium isotopes to study site accumulation processes. J. Taphon. 8, 115–127.
Coudrain, A., Loubet, M., Condom, T., Talbi, A., Ribstein, P., Pouyaud, B., et al. (2002). Données isotopiques (8786/Sr) et changements hydrologiques depuis 15 000 ans sur l’Altiplano andin. Hydrol. Sci. J. 47, 293–306. doi: 10.1080/02626660209492931
Cressie, N. (1993). Statistics for Spatial Data. New York, NY: John Wiley and Sons, Inc. doi: 10.1002/9781119115151
Crowley, B. E., Miller, J. H., and Bataille, C. P. (2017). Strontium isotopes (87Sr/86Sr) in terrestrial ecological and palaeoecological research: empirical efforts and recent advances in continental-scale models. Biol. Rev. Camb. Philos. Soc. 92, 43–59. doi: 10.1111/brv.12217
Cuéllar, A. M. (2013). The archaeology of food and social inequality in the Andes. J. Archaeol. Res. 21, 123–174. doi: 10.1007/s10814-012-9061-x
deFrance, S. D., Capriles, J. M., and Tripcevich, N. (2016). “Pastoralism through time in Southern Peru,” in The Archaeology of Andean Pastoralism, eds J. M. Capriles and N. Tripcevich (Albuquerque, NM: University of New Mexico Press), 119–138.
Denevan, W. M. (2001). Cultivated Landscapes of Native Amazonia and the Andes. Oxford: Oxford University Press.
Dijkstra, F. A. (2003). Calcium mineralization in the forest floor and surface soil beneath different tree species in the northeastern US. For. Ecol. Manag. 175, 185–194. doi: 10.1016/S0378-1127(02)00128-7
Dillehay, T. D. (2011). From Foraging to Farming in the Andes: New Perspectives on Food Production and Social Organization. Cambridge: Cambridge University Press. doi: 10.1017/CBO9780511793790
Donnan, C. B., and McClelland, D. (1999). Moche Fineline Painting: Its Evolution and Its Artists. Los Angeles, CA: University of California Los Angeles Fowler Museum Publications.
Drouet, T., and Herbauts, J. (2008). Evaluation of the mobility and discrimination of Ca, Sr and Ba in forest ecosystems: consequence on the use of alkaline-earth element ratios as tracers of Ca. Plant Soil 302, 105–124. doi: 10.1007/s11104-007-9459-2
Drouet, T., Herbauts, J., Gruber, W., and Demaiffe, D. (2005). Strontium isotope composition as a tracer of calcium sources in two forest ecosystems in Belgium. Geoderma 126, 203–223. doi: 10.1016/j.geoderma.2004.09.010
Ehleringer, J. R., Thompson, A. H., Podlesak, D. W., Bowen, G. J., Chesson, L. A., Cerling, T. E., et al. (2010). “A framework for the incorporation of isotopes and isoscapes in geospatial forensic investigations,” in Isoscapes, eds G. J. Bowen and J. B. West (New York, NY: Springer), 357–387. doi: 10.1007/978-90-481-3354-3_17
Eikenberg, J., Tricca, A., Vezzu, G., Stille, P., Bajo, S., and Ruethi, M. (2001). 228Ra/226Ra/224Ra and 87Sr/86Sr isotope relationships for determining interactions between ground and river water in the upper Rhine valley. J. Environ. Radioact. 54, 133–162. doi: 10.1016/S0265-931X(00)00171-5
Ericson, J. E. (1985). Strontium isotope characterization in the study of prehistoric human ecology. J. Hum. Evol. 14, 503–514. doi: 10.1016/S0047-2484(85)80029-4
Evans, J. A., Chenery, C. A., and Fitzpatrick, A. P. (2006). Bronze Age childhood migration of individuals near Stonehenge, revealed by strontium and oxygen isotope tooth enamel analysis. Archaeometry 48, 309–321. doi: 10.1111/j.1475-4754.2006.00258.x
Evans, J. A., Chenery, C. A., and Montgomery, J. (2012). A summary of strontium and oxygen isotope variation in archaeological human tooth enamel excavated from Britain. J. Analyt. Atom. Spectrom. 27, 754–764. doi: 10.1039/c2ja10362a
Evans, J. A., Montgomery, J., and Wildman, G. (2009). Isotope domain mapping of 87Sr/86Sr biosphere variation on the Isle of Skye, Scotland. J. Geol. Soc. 166, 617–631. doi: 10.1144/0016-76492008-043
Evans, J. A., Montgomery, J., Wildman, G., and Boulton, N. (2010). Spatial variations in biosphere 87Sr/86Sr in Britain. J. Geol. Soc. 167, 1–4. doi: 10.1144/0016-76492009-090
Evans, J. A., and Tatham, S. (2004). Defining ‘local signature’in terms of Sr isotope composition using a tenth-to twelfth-century Anglo-Saxon population living on a Jurassic clay-carbonate terrain, Rutland, UK. Geol. Soc. Lond. Spec. Public. 232, 237–248. doi: 10.1144/GSL.SP.2004.232.01.21
Fernández-Crespo, T., Snoeck, C., Ordoño, J., de Winter, N., Czermak, A., Mattielli, N., et al. (2020). Multi-isotope evidence for the emergence of cultural alterity in Late Neolithic Europe. Sci. Adv. 6:eaay2169. doi: 10.1126/sciadv.aay2169
Finucane, B. C. (2007). Mummies, maize, and manure: multi-tissue stable isotope analysis of late prehistoric human remains from the Ayacucho Valley, Peru. J. Archaeol. Sci. 34, 2115–2124. doi: 10.1016/j.jas.2007.02.006
Fisher, E. C., Bar-Matthews, M., Jerardino, A., and Marean, C. W. (2010). Middle and Late Pleistocene paleoscape modeling along the southern coast of South Africa. Q. Sci. Rev. 29, 1382–1398. doi: 10.1016/j.quascirev.2010.01.015
Flusche, M. A., Seltzer, G., Rodbell, D., Siegel, D., and Samson, S. (2005). Constraining water sources and hydrologic processes from the isotopic analysis of water and dissolved strontium, Lake Junin, Peru. J. Hydrol. 312, 1–13. doi: 10.1016/j.jhydrol.2005.02.021
Fordyce, F. M., Green, P., and Simpson, P. (1993). Simulation of regional geochemical survey maps at variable sample density. J. Geochem. Explor. 49, 161–175. doi: 10.1016/0375-6742(93)90043-L
Frei, K. M., and Frei, R. (2011). The geographic distribution of strontium isotopes in Danish surface waters-A base for provenance studies in archaeology, hydrology and agriculture. Appl. Geochem. 26, 326–340. doi: 10.1016/j.apgeochem.2010.12.006
Frei, K. M., and Price, T. D. (2012). Strontium isotopes and human mobility in prehistoric Denmark. Archaeol. Anthropol. Sci. 4, 103–114. doi: 10.1007/s12520-011-0087-7
Frei, R., and Frei, K. M. (2013). The geographic distribution of Sr isotopes from surface waters and soil extracts over the island of Bornholm (Denmark)-A base for provenance studies in archaeology and agriculture. Appl. Geochem. 38, 147–160. doi: 10.1016/j.apgeochem.2013.09.007
Frei, R., Frei, K. M., and Jessen, S. (2020). Shallow retardation of the strontium isotope signal of agricultural liming-implications for isoscapes used in provenance studies. Sci. Total Environ. 706:135710. doi: 10.1016/j.scitotenv.2019.135710
Garrett, R. G., Banville, R. M., and Adcock, S. W. (1990). Regional geochemical data compilation and map preparation, Labrador, Canada. J. Geochem. Explor. 39, 91–116. doi: 10.1016/0375-6742(90)90070-Q
Glorennec, P., Lucas, J.-P., Mercat, A.-C., Roudot, A.-C., and Le Bot, B. (2016). Environmental and dietary exposure of young children to inorganic trace elements. Environ. Int. 97, 28–36. doi: 10.1016/j.envint.2016.10.009
Graustein, W. C. (1989). “87Sr/86Sr ratios measure the sources and flow of strontium in terrestrial ecosystems,” in Stable Isotopes in Ecological Research, eds P. Rundle, J. Ehleringer, and K. Nagy (New York, NY: Springer-Verlag), 491–512. doi: 10.1007/978-1-4612-3498-2_28
Grimstead, D. N., Nugent, S., and Whipple, J. (2017). Why a standardization of strontium isotope baseline environmental data is needed and recommendations for methodology. Adv. Archaeol. Pract. 5, 184–195. doi: 10.1017/aap.2017.6
Grimstead, D. N., Quade, J., and Stiner, M. C. (2016). Isotopic evidence for long-distance mammal procurement, chaco canyon, New Mexico, USA. Geoarchaeology 31, 335–354. doi: 10.1002/gea.21545
Grove, M. J., Baker, P. A., Cross, S. L., Rigsby, C. A., and Seltzer, G. O. (2003). Application of strontium isotopes to understanding the hydrology and paleohydrology of the Altiplano, Bolivia-Peru. Palaeogeogr. Palaeoclimatol. Palaeoecol. 194, 281–297. doi: 10.1016/S0031-0182(03)00282-7
Grupe, G., Hölzl, S., Kocsis, B., Kröger, P., Mauder, M., Mayr, C., et al. (2017a). “Isotopic mapping and migration research based on bioarchaeological finds,” in ResourceCultures: Sociocultural Dynamics and the Use of Resources – Theories, Methods, and Perspectives, eds A. Scholz, M. Bartleheim, R. Hardenberg, and J. Staecker (Tübingen: University of Tübingen), 195–208.
Grupe, G., Hölzl, S., Mayr, C., and Söllner, F. (2017b). “The concept of isotopic landscapes: modern ecogeochemistry versus bioarchaeology,” in Across the Alps in Prehistory, eds G. Grupe, A. Grigat, and G. McGlynn (Cham: Springer), 27–48. doi: 10.1007/978-3-319-41550-5_2
Grupe, G., von Carnap-Bornheim, C., and Söllner, F. (2011). Stable strontium isotope mapping for provenance studies in archaeology: different material, different signals. Bull. Soc. Suisse Anthropol. 17, 67–76.
Hastorf, C. A. (2003). Andean luxury foods: special food for the ancestors, deities and the élite. Antiquity 77, 545–554. doi: 10.1017/S0003598X00092607
Hastorf, C. A., and Johannessen, S. (1993). Pre-Hispanic political change and the role of maize in the Central Andes of Peru. Am. Anthropol. 95, 115–138. doi: 10.1525/aa.1993.95.1.02a00060
Hedman, K. M., Curry, B. B., Johnson, T. M., Fullagar, P. D., and Emerson, T. E. (2009). Variation in strontium isotope ratios of archaeological fauna in the Midwestern United States: a preliminary study. J. Archaeol. Sci. 36, 64–73. doi: 10.1016/j.jas.2008.07.009
Hedman, K. M., Slater, P. A., Fort, M. A., Emerson, T. E., and Lambert, J. M. (2018). Expanding the strontium isoscape for the American midcontinent: identifying potential places of origin for Cahokian and Pre-Columbian migrants. J. Archaeol. Sci. Rep. 22, 202–213. doi: 10.1016/j.jasrep.2018.09.027
Hermann, A., Forkel, R., McAlister, A., Cruickshank, A., Golitko, M., Kneebone, B., et al. (2020). Pofatu, a curated and open-access database for geochemical sourcing of archaeological materials. Sci. Data 7, 1–9. doi: 10.1038/s41597-020-0485-8
Hodell, D. A., Quinn, R. L., Brenner, M., and Kamenov, G. (2004). Spatial variation of strontium isotopes (87Sr/86Sr) in the Maya region: a tool for tracking ancient human migration. J. Archaeol. Sci. 31, 585–601. doi: 10.1016/j.jas.2003.10.009
Hoogewerff, J., Papesch, W., Kralik, M., Berner, M., Vroon, P., Miesbauer, H., et al. (2001). The last domicile of the Iceman from Hauslabjoch: a geochemical approach using Sr, C and O isotopes and trace element signatures. J. Archaeol. Sci. 28, 983–989. doi: 10.1006/jasc.2001.0659
Hoogewerff, J. A., Reimann, C., Ueckermann, H., Frei, R., Frei, K. M., Van Aswegen, T., et al. (2019). Bioavailable 87Sr/86Sr in European soils: a baseline for provenancing studies. Sci. Total Environ. 672, 1033–1044. doi: 10.1016/j.scitotenv.2019.03.387
Horwitz, P., Chiarizia, R., and Dietz, M. L. (1992). A novel strontium-selective extraction chromatographic resin. Solvent Extract. Ion Exchang. 10, 313–336. doi: 10.1080/07366299208918107
Instituto Geográfico Nacional de Perú (2015). Información Hidrográfica de Perú. Perú: Instituto Geográfico Nacional de Perú.
Johns, T. (1990). With Bitter Herbs They Shall Eat It: Chemical Ecology and the Origins of Human Diet and Medicine. Tucson, AZ: University of Arizona Press.
Johnson, C. D., Nandi, A., Joyner, T. A., and Luffman, I. (2018). Iron and manganese in groundwater: using Kriging and GIS to locate high concentrations in Buncombe County, North Carolina. Groundwater 56, 87–95. doi: 10.1111/gwat.12560
Johnson, L., Montgomery, J., Evans, J., and Hamilton, E. (2019). Contribution of strontium to the human diet from querns and millstones: an experiment in digestive strontium isotope uptake. Archaeometry 61, 1366–1381. doi: 10.1111/arcm.12485
Julien, C. J., Shozo, M., Isumi, S., and Craig, M. (1985). “Guano and resource control in sixteenth-century Arequipa,” in Andean Ecology and Civilization, eds S. Masuda and M. C. Shimada (Tokyo: University of Tokyo Press), 185–231.
Knipper, C., Maurer, A.-F., Peters, D., Meyer, C., Brauns, M., Galer, S. J., et al. (2012). “Mobility in thuringia or mobile thuringians: a strontium isotope study from early Medieval central Germany,” in Population Dynamics in Prehistory and Early History: New Approaches Using Stable Isotopes and Genetics, eds E. Kaiser, J. Burger, and W. Schier (Berlin: de Gruyter), 287–310.
Knudson, K. J., Aufderheide, A. E., and Buikstra, J. E. (2007). Seasonality and paleodiet in the Chiribaya polity of southern Peru. J. Archaeol. Sci. 34, 451–462. doi: 10.1016/j.jas.2006.07.003
Knudson, K. J., Gardella, K. R., and Yaeger, J. (2012a). Provisioning Inka feasts at tiwanaku, bolivia: the geographic origins of camelids in the pumapunku complex. J. Archaeol. Sci. 39, 479–491. doi: 10.1016/j.jas.2011.10.003
Knudson, K. J., O’Donnabhain, B., Carver, C., Cleland, R., and Price, T. D. (2012b). Migration and Viking Dublin: paleomobility and paleodiet through isotopic analyses. J. Archaeol. Sci. 39, 308–320. doi: 10.1016/j.jas.2011.09.014
Knudson, K. J., Peters, A. H., and Cagigao, E. T. (2015). Paleodiet in the Paracas Necropolis of Wari Kayan: carbon and nitrogen isotope analysis of keratin samples from the south coast of Peru. J. Archaeol. Sci. 55, 231–243. doi: 10.1016/j.jas.2015.01.011
Knudson, K. J., and Price, T. D. (2007). Utility of multiple chemical techniques in archaeological residential mobility studies: case studies from Tiwanaku-and Chiribaya-affiliated sites in the Andes. Am. J. Phys. Anthropol. 132, 25–39. doi: 10.1002/ajpa.20480
Knudson, K. J., Stanish, C., Lozada, M. C., Faull, K. M., and Tantaleán, H. (2016). Intra-individual variability and strontium isotope measurements: a methodological study using 87 Sr/86 Sr data from Pampa de los Gentiles, Chincha Valley, Peru. J. Archaeol. Sci. Rep. 5, 590–597. doi: 10.1016/j.jasrep.2016.01.016
Knudson, K. J., and Tung, T. A. (2011). Investigating regional mobility in the southern hinterland of the Wari Empire: biogeochemistry at the site of Beringa, Peru. Am. J. Phys. Anthropol. 145, 299–310. doi: 10.1002/ajpa.21494
Knudson, K. J., Webb, E., White, C., and Longstaffe, F. J. (2014). Baseline data for Andean paleomobility research: a radiogenic strontium isotope study of modern Peruvian agricultural soils. Archaeol. Anthropol. Sci. 6, 205–219. doi: 10.1007/s12520-013-0148-1
Knudson, K. J., Williams, H. M., Buikstra, J. E., Tomczak, P. D., Gordon, G. W., and Anbar, A. D. (2010). Introducing δ88/86 Sr analysis in archaeology: a demonstration of the utility of strontium isotope fractionation in paleodietary studies. J. Archaeol. Sci. 37, 2352–2364. doi: 10.1016/j.jas.2010.04.009
Kootker, L., Plomp, E., Ammer, S., Hoogland, V., and Davies, G. (2020). Spatial patterns in 87Sr/86Sr ratios in modern human dental enamel and tap water from the Netherlands: implications for forensic provenancing. Sci. Total Environ. 729:138992. doi: 10.1016/j.scitotenv.2020.138992
Kootker, L. M., van Lanen, R. J., Kars, H., and Davies, G. R. (2016). Strontium isoscapes in the Netherlands: Spatial variations in 87 Sr/86 Sr as a proxy for palaeomobility. J. Archaeol. Sci. Rep. 6, 1–13. doi: 10.1016/j.jasrep.2016.01.015
Ladegaard-Pedersen, P., Achilleos, M., Dörflinger, G., Frei, R., Kristiansen, K., and Frei, K. M. (2020). A strontium isotope baseline of Cyprus. Assessing the use of soil leachates, plants, groundwater and surface water as proxies for the local range of bioavailable strontium isotope composition. Sci. Total Environ. 708:134714. doi: 10.1016/j.scitotenv.2019.134714
Laffoon, J. E., Davies, G. R., Hoogland, M. L., and Hofman, C. L. (2012). Spatial variation of biologically available strontium isotopes (87 Sr/86 Sr) in an archipelagic setting: a case study from the Caribbean. J. Archaeol. Sci. 39, 2371–2384. doi: 10.1016/j.jas.2012.02.002
Lane, K. (2014). “Water technology in the Andes,” in Encyclopaedia of the History of Science, Technology, and Medicine in Non-Western Cultures, ed. H. Selin (Dordrecht: Springer), 1–24. doi: 10.1007/978-94-007-3934-5_10182-1
Lehner, B., and Grill, G. (2013). Global river hydrography and network routing: baseline data and new approaches to study the world’s large river systems. Hydrol. Process. 27, 2171–2186. doi: 10.1002/hyp.9740
Lengfelder, F., Grupe, G., Stallauer, A., Huth, R., and Söllner, F. (2019). Modelling strontium isotopes in past biospheres-assessment of bioavailable 87Sr/86Sr ratios in local archaeological vertebrates based on environmental signatures. Sci. Total Environ. 648, 236–252. doi: 10.1016/j.scitotenv.2018.08.014
Lewis, J., Pike, A., Coath, C., and Evershed, R. (2017). Strontium concentration, radiogenic (87Sr/86Sr) and stable (δ 88Sr) strontium isotope systematics in a controlled feeding study. Sci. Technol. Archaeol. Res. 3, 45–57. doi: 10.1080/20548923.2017.1303124
Lynch, T. F. (1983). Camelid pastoralism and the emergence of Tiwanaku civilization in the south-central Andes. World Archaeol. 15, 1–14. doi: 10.1080/00438243.1983.9979881
Mader, C., Hölzl, S., Heck, K., Reindel, M., and Isla, J. (2018). The llama’s share: highland origins of camelids during the Late Paracas period (370 to 200 BCE) in south Peru demonstrated by strontium isotope analysis. J. Archaeol. Sci. Rep. 20, 257–270. doi: 10.1016/j.jasrep.2018.04.032
Mamani, M., Tassara, A., and Wörner, G. (2008). Composition and structural control of crustal domains in the central Andes. Geochem. Geophys. Geosyst. 9:2007GC001925. doi: 10.1029/2007GC001925
Marsteller, S. J., Zolotova, N., and Knudson, K. J. (2017). Investigating economic specialization on the central Peruvian coast: a reconstruction of Late Intermediate Period Ychsma diet using stable isotopes. Am. J. Phys. Anthropol. 162, 300–317. doi: 10.1002/ajpa.23117
Maurer, A.-F., Galer, S. J., Knipper, C., Beierlein, L., Nunn, E. V., Peters, D., et al. (2012). Bioavailable 87Sr/86Sr in different environmental samples—Effects of anthropogenic contamination and implications for isoscapes in past migration studies. Sci. Total Environ. 433, 216–229. doi: 10.1016/j.scitotenv.2012.06.046
Mitchell, H., Hamilton, T., Steggerda, F., and Bean, H. (1945). The chemical composition of the adult human body and its bearing on the biochemistry of growth. J. Biol. Chem. 158, 625–637.
Montgomery, J. (2010). Passports from the past: investigating human dispersals using strontium isotope analysis of tooth enamel. Ann. Hum. Biol. 37, 325–346. doi: 10.3109/03014461003649297
Montgomery, J., Evans, J. A., and Cooper, R. E. (2007). Resolving archaeological populations with Sr-isotope mixing models. Appl. Geochem. 22, 1502–1514. doi: 10.1016/j.apgeochem.2007.02.009
Netherly, P. J. (1984). The management of late Andean irrigation systems on the north coast of Peru. Am. Antiq. 49, 227–254. doi: 10.2307/280017
Nixon, G., and Helsby, C. (1976). The relationship between strontium in water supplies and human tooth enamel. Arch. Oral. Biol. 21, 691–695. doi: 10.1016/0003-9969(76)90144-8
Núñez, L., and Dillehay, T. (1979). Movilidad Giratoria, Armonía y Desarrollo en los Andes Meridionales, Patrones de tráfico e Interacción Económica, Dirección General de Investigaciones Tecnológicas. Antofagasta: Universidad del Norte.
Oliver, M., and Webster, R. (2014). A tutorial guide to geostatistics: computing and modelling variograms and kriging. Catena 113, 56–69. doi: 10.1016/j.catena.2013.09.006
Oliver, M. A., and Webster, R. (1990). Kriging: a method of interpolation for geographical information systems. Intern. J. Geograp. Inform. Syst. 4, 313–332. doi: 10.1080/02693799008941549
Oliver, M. A., and Webster, R. (2015). Basic Steps in Geostatistics: The Variogram and Kriging. Cham: Springer Nature. doi: 10.1007/978-3-319-15865-5
Pacheco-Forés, S. I., Gordon, G. W., and Knudson, K. J. (2020). Expanding radiogenic strontium isotope baseline data for central Mexican paleomobility studies. PLoS One 15:e0229687. doi: 10.1371/journal.pone.0229687
Pellegrini, M., Pouncett, J., Jay, M., Pearson, M. P., and Richards, M. P. (2016). Tooth enamel oxygen “isoscapes” show a high degree of human mobility in prehistoric Britain. Sci. Rep. 6:34986. doi: 10.1038/srep34986
Peucker-Ehrenbrink, B., and Fiske, G. J. (2019). A continental perspective of the seawater 87Sr/86Sr record: a review. Chem. Geol. 510, 140–165. doi: 10.1016/j.chemgeo.2019.01.017
Poszwa, A., Ferry, B., Dambrine, E., Pollier, B., Wickman, T., Loubet, M., et al. (2004). Variations of bioavailable Sr concentration and 87 Sr/86 Sr ratio in boreal forest ecosystems. Biogeochemistry 67, 1–20. doi: 10.1023/B:BIOG.0000015162.12857.3e
Pourmand, A., and Dauphas, N. (2010). Distribution coefficients of 60 elements on TODGA resin: application to Ca, Lu, Hf, U and Th isotope geochemistry. Talanta 81, 741–753. doi: 10.1016/j.talanta.2010.01.008
Price, T. D., Burton, J. H., and Bentley, R. A. (2002). The characterization of biologically available strontium isotope ratios for the study of prehistoric migration. Archaeometry 44, 117–135. doi: 10.1111/1475-4754.00047
Price, T. D., Burton, J. H., Fullagar, P. D., Wright, L. E., Buikstra, J. E., and Tiesler, V. (2015). “Strontium isotopes and the study of human mobility among the ancient Maya,” in Archaeology and Bioarchaeology of Population Movement Among the Prehispanic Maya, ed. A. Cucina (New York, NY: Springer), 119–132. doi: 10.1007/978-3-319-10858-2_11
Price, T. D., Burton, J. H., and Stoltman, J. B. (2007). Place of origin of prehistoric inhabitants of Aztalan, Jefferson Co., Wisconsin. Am. Antiq. 72, 524–538. doi: 10.2307/40035859
Price, T. D., Johnson, C. M., Ezzo, J. A., Ericson, J., and Burton, J. H. (1994). Residential mobility in the prehistoric southwest United States: a preliminary study using strontium isotope analysis. J. Archaeol. Sci. 21, 315–330. doi: 10.1006/jasc.1994.1031
Pulgar Vidal, J. (1981). Geografiìa del Perú: Las Ocho Regiones Naturales del Perú. Lima: PUCP- Fondo Editorial.
Quilter, J., and Stocker, T. (1983). Subsistence economies and the origins of Andean complex societies. Am. Anthropol. 85, 545–562. doi: 10.1525/aa.1983.85.3.02a00030
Rodbell, D. T., Delman, E. M., Abbott, M. B., Besonen, M. T., and Tapia, P. M. (2014). The heavy metal contamination of Lake Junín National Reserve, Peru: an unintended consequence of the juxtaposition of hydroelectricity and mining. GSA Today 24, 4–10. doi: 10.1130/GSATG200A.1
Romaniello, S., Field, M., Smith, H., Gordon, G., Kim, M., and Anbar, A. (2015). Fully automated chromatographic purification of Sr and Ca for isotopic analysis. J. Analyt. Atom. Spectrom. 30, 1906–1912. doi: 10.1039/C5JA00205B
Salesse, K., Fernandes, R., de Rochefort, X., Brůžek, J., Castex, D., and Dufour, É (2018). IsoArcH. eu: an open-access and collaborative isotope database for bioarchaeological samples from the Graeco-Roman world and its margins. J. Archaeol. Sci. Rep. 19, 1050–1055. doi: 10.1016/j.jasrep.2017.07.030
Santos, R. V., Sondag, F., Cochonneau, G., Lagane, C., Brunet, P., Hattingh, K., et al. (2015). Source area and seasonal 87Sr/86Sr variations in rivers of the Amazon basin. Hydrol. Process. 29, 187–197. doi: 10.1002/hyp.10131
Scaffidi, B. K. (2019). Spatial paleopathology: a geographic approach to the etiology of cribrotic lesions in the prehistoric Andes. Intern. J. Paleopathol. 29, 102–116. doi: 10.1016/j.ijpp.2019.07.002
Scaffidi, B. K., and Knudson, K. J. (2020). An archaeological strontium isoscape for the prehistoric Andes: understanding population mobility through a geostatistical meta-analysis of archaeological 87Sr/86Sr values from humans, animals, and artifacts. J. Archaeol. Sci. 117, 105–121. doi: 10.1016/j.jas.2020.105121
Schenk, C. J., Viger, R. J., and Anderson, C. P. (1999). Maps Showing Geology, Oil and Gas Fields, and Geological Provinces of South America: U.S. Geological Survey Open-File Report 97-470-D. Available online at: https://pubs.er.usgs.gov/publication/ofr97470D doi: 10.3133/ofr97470D (accessed May 1, 2020).
Schreiber, K., and Lancho Rojas, J. (2003). Irrigation and Society in the Peruvian Desert: The Puquios of Nasca. Lanham, MD: Lexington Books.
Scott, E. M., Allen, M. B., Macpherson, C. G., McCaffrey, K. J. W., Davidson, J. P., Saville, C., et al. (2018). Andean surface uplift constrained by radiogenic isotopes of arc lavas. Nat. Commun. 9, 969–977. doi: 10.1038/s41467-018-03173-4
Serna, A., Prates, L., Mange, E., Salazar-García, D. C., and Bataille, C. P. (2020). Implications for paleomobility studies of the effects of quaternary volcanism on bioavailable strontium: a test case in North Patagonia (Argentina). J. Archaeol. Sci. 121:105198. doi: 10.1016/j.jas.2020.105198
Sillen, A., and Kavanagh, M. (1982). Strontium and paleodietary research: a review. Am. J. Phys. Anthropol. 25, 67–90. doi: 10.1002/ajpa.1330250505
Slovak, N. M., and Paytan, A. (2011). Fisherfolk and farmers: carbon and nitrogen isotope evidence from Middle Horizon Ancón, Peru. Intern. J. Osteoarchaeol. 21, 253–267. doi: 10.1002/oa.1128
Slovak, N. M., Paytan, A., and Wiegand, B. A. (2009). Reconstructing Middle Horizon mobility patterns on the coast of Peru through strontium isotope analysis. J. Archaeol. Sci. 36, 157–165. doi: 10.1016/j.jas.2008.08.004
Snoeck, C., Ryan, S., Pouncett, J., Pellegrini, M., Claeys, P., Wainwright, A. N., et al. (2020). Towards a biologically available strontium isotope baseline for Ireland. Sci. Total Environ. 712:136248. doi: 10.1016/j.scitotenv.2019.136248
Szpak, P., Millaire, J.-F., White, C. D., and Longstaffe, F. J. (2012). Influence of seabird guano and camelid dung fertilization on the nitrogen isotopic composition of field-grown maize (Zea mays). J. Archaeol. Sci. 39, 3721–3740. doi: 10.1016/j.jas.2012.06.035
Tachikawa, T., Hato, M., Kaku, M., and Iwasaki, A. (2011). “Characteristics of ASTER GDEM version 2,” in Proceedings of the 2011 IEEE International Geoscience and Remote Sensing Symposium (IGARSS): Institute of Electrical and Electronics Engineers, Piscataway, NJ. doi: 10.1109/IGARSS.2011.6050017
Thomsen, E., and Andreasen, R. (2019). Agricultural lime disturbs natural strontium isotope variations: implications for provenance and migration studies. Sci. Adv. 5, 1–11. doi: 10.1126/sciadv.aav8083
Thornton, E. K. (2011). Reconstructing ancient Maya animal trade through strontium isotope (87Sr/86Sr) analysis. J. Archaeol. Sci. 38, 3254–3263. doi: 10.1016/j.jas.2011.06.035
Thornton, E. K., Defrance, S. D., Krigbaum, J., and Williams, P. R. (2011). Isotopic evidence for Middle Horizon to 16th century camelid herding in the Osmore Valley, Peru. Intern. J. Osteoarchaeol. 21, 544–567. doi: 10.1002/oa.1157
Tipple, B. J., Valenzuela, L. O., and Ehleringer, J. R. (2018). Strontium isotope ratios of human hair record intra-city variations in tap water source. Sci. Rep. 8, 1–10. doi: 10.1038/s41598-018-21359-0
Tricca, A., Stille, P., Steinmann, M., Kiefel, B., Samuel, J., and Eikenberg, J. (1999). Rare earth elements and Sr and Nd isotopic compositions of dissolved and suspended loads from small river systems in the Vosges mountains (France), the river Rhine and groundwater. Chem. Geol. 160, 139–158. doi: 10.1016/S0009-2541(99)00065-0
Tripcevich, N., and Capriles, J. M. (2016). The Archaeology of Andean Pastoralism. Albuquerque: University of New Mexico Press.
Tung, T. A., and Knudson, K. J. (2011). Identifying locals, migrants, and captives in the Wari heartland: a bioarchaeological and biogeochemical study of human remains from Conchopata, Peru. J. Anthropol. Archaeol. 30, 247–261. doi: 10.1016/j.jaa.2011.06.005
Turner, B. L., Kamenov, G. D., Kingston, J. D., and Armelagos, G. J. (2009). Insights into immigration and social class at Macchu Picchu, Peru, based on oxygen, strontium, and lead isotopic analysis. J. Archaeol. Sci. 36, 317–332. doi: 10.1016/j.jas.2008.09.018
Valentine, B., Kamenov, G. D., and Krigbaum, J. (2008). Reconstructing Neolithic groups in Sarawak, Malaysia through lead and strontium isotope analysis. J. Archaeol. Sci. 35, 1463–1473. doi: 10.1016/j.jas.2007.10.016
Varouchakis, E., Hristopulos, D., and Karatzas, G. (2012). Improving kriging of groundwater level data using nonlinear normalizing transformations—a field application. Hydrol. Sci. J. 57, 1404–1419. doi: 10.1080/02626667.2012.717174
Veizer, J. (1989). Strontium isotopes in seawater through time. Annu. Rev. Earth Planet. Sci. 17, 141–167. doi: 10.1146/annurev.ea.17.050189.001041
Voerkelius, S., Lorenz, G. D., Rummel, S., Quétel, C. R., Heiss, G., Baxter, M., et al. (2010). Strontium isotopic signatures of natural mineral waters, the reference to a simple geological map and its potential for authentication of food. Food Chem. 118, 933–940. doi: 10.1016/j.foodchem.2009.04.125
Wang, X., Tang, Z., and Dong, X. (2018). Distribution of strontium isotopes in river waters across the Tarim Basin: a map for migration studies. J. Geol. Soc. 175, 967–973. doi: 10.1144/jgs2018-074
Wasserman, R., Lengemann, F., and Comar, C. (1958). Comparative metabolism of calcium and strontium in lactation. J. Dairy Sci. 41, 812–821. doi: 10.3168/jds.S0022-0302(58)91004-X
West, A., February, E., and Bowen, G. (2014). Spatial analysis of hydrogen and oxygen stable isotopes (“isoscapes”) in ground water and tap water across South Africa. J. Geochem. Explorat. 145, 213–222. doi: 10.1016/j.gexplo.2014.06.009
Whipkey, C., Capo, R., Chadwick, O., and Stewart, B. (2000). The importance of sea spray to the cation budget of a coastal Hawaiian soil: a strontium isotope approach. Chem. Geol. 168, 37–48. doi: 10.1016/S0009-2541(00)00187-X
Willmes, M., Bataille, C. P., James, H. F., Moffat, I., McMorrow, L., Kinsley, L., et al. (2018). Mapping of bioavailable strontium isotope ratios in France for archaeological provenance studies. Appl. Geochem. 90, 75–86. doi: 10.1016/j.apgeochem.2017.12.025
Wright, L. (2005). Identifying immigrants to Tikal, Guatemala: defining local variability in strontium isotope ratios of human tooth enamel. J. Archaeol. Sci. 32, 555–566. doi: 10.1016/j.jas.2004.11.011
Wunder, M. B. (2010). “Using isoscapes to model probability surfaces for determining geographic origins,” in Isoscapes: Understanding Movement, Pattern, and Process on Earth Through Isotope Mapping, eds J. B. West, G. J. Bowen, T. E. Dawson, and K. P. Tu (Dordrecht: Springer), 251–270. doi: 10.1007/978-90-481-3354-3_12
Yamanaka, T., Makino, Y., Wakiyama, Y., Kishi, K., Maruyama, K., Kano, M., et al. (2015). How reliable are modeled precipitation isoscapes over a high-relief mountainous region? Hydrol. Res. Lett. 9, 118–124. doi: 10.3178/hrl.9.118
Zimmer-Dauphinee, J., Scaffidi, B., and Tung, T. (2020). “Finding family using forensic isoscapes: The application of stable oxygen isotope analysis in Peru,” in Humanitarian Forensic Science: Interacting with the Dead and the Living, eds D. Ubelaker and R. Parra (Hoboken, NJ: John Wiley & Sons), 311–329. doi: 10.1002/9781119482062.ch21
Zitek, A., Tchaikovsky, A., Irrgeher, J., Waidbacher, H., and Prohaska, T. (2015). “The 87Sr/86Sr river water isoscape of the Danube catchment,” in Joint Danube Survey 3: A Comprehensive Analysis of Danube Water Quality, eds I. Liška, F. Wagner, M. Sengl, K. Deutsch, and J. Slobodnik (Vienna: ICPDR - International Commission for the Proteccion of the Danube River), 349–354.
Keywords: pre-Hispanic Andes, strontium isotope analysis, geostatistical analysis, isoscape, archeological geolocation
Citation: Scaffidi BK, Tung TA, Gordon G, Alaica AK, González La Rosa LM, Marsteller SJ, Dahlstedt A, Schach E and Knudson KJ (2020) Drinking Locally: A Water 87Sr/86Sr Isoscape for Geolocation of Archeological Samples in the Peruvian Andes. Front. Ecol. Evol. 8:281. doi: 10.3389/fevo.2020.00281
Received: 04 June 2020; Accepted: 10 August 2020;
Published: 29 September 2020.
Edited by:
Clement Pierre Bataille, University of Ottawa, CanadaReviewed by:
Ramiro Barberena, CONICET Mendoza, ArgentinaAlejandro Serna, National University of La Plata, Argentina
Copyright © 2020 Scaffidi, Tung, Gordon, Alaica, González La Rosa, Marsteller, Dahlstedt, Schach and Knudson. This is an open-access article distributed under the terms of the Creative Commons Attribution License (CC BY). The use, distribution or reproduction in other forums is permitted, provided the original author(s) and the copyright owner(s) are credited and that the original publication in this journal is cited, in accordance with accepted academic practice. No use, distribution or reproduction is permitted which does not comply with these terms.
*Correspondence: Beth K. Scaffidi, Y3NjYWZmaWRpQHVjbWVyY2VkLmVkdQ==