- Department of Psychology, Rutgers University, New Brunswick, NJ, United States
Although genetically heterogeneous laboratory mice express individual differences in general cognitive ability (c.f., “intelligence”), it is unknown whether these differences are translated into behaviors that would promote survival. Here, genetically heterogeneous laboratory CD-1 mice were administered a series of cognitive tests from which their aggregate general cognitive ability was estimated. Subsequently, all animals were tested on nine (unlearned) tasks designed to assess behaviors that could contribute to survival in the wild. These tests included nest building (in the home and a novel environment), exploration, several indices of food finding, retrieval, and preference, and predator avoidance. Like general cognitive ability, a principal component analysis of these measures of survival-related behaviors (survival-readiness) yielded a general factor that accounted for ∼25% of the variance of mice across all of the tasks. An aggregate metric of general cognitive ability predicted an aggregate metric of general survival-readiness (r = 0.64), suggesting that more intelligent animals would be more suited for survival in natural environments. The nature of the pattern of correlations between general cognitive ability and performance on individual tests of survival-readiness (where tests conducted in previously unexplored contexts were more closely related to general cognitive ability) suggests the possibility that heightened attention (which is taxed in a novel environment) may be the common mediator of both of these classes of abilities, although other potential mediators are discussed. In total, these results suggest that performance on tasks that are explicitly intended to assess the likelihood of survival can be impacted by cognitive abilities.
Introduction
In response to the question “how do we know that our [IQ] tests are ‘good’ measures of intelligence?,” Wechsler (1944) wrote:
“The only honest answer we can make is that our own experience has shown them to be so. If this seems to be a very tenuous answer we need only remind the reader that it has been practical experience which has given (or denied) final validity to every intelligence test. Regrettable as it may seem, empirical judgements, here as elsewhere, play the role of ultimate arbiter.”
To an empiricist, Wechsler’s comment might seem to lack quantitative substance, instead relying on anecdotal observations to support the utility of the intelligence test. However, to the extent that IQ tests should predict functionally important life outcomes, the decades since Wechsler’s statement have filled in the empirical gaps. It is now well-established that IQ tests predict a range of measures of academic success, such as grades, years of education, and performance on other standardized tests (such as the SAT or GRE; for review, see Gottfredson, 1998). A skeptic might be concerned that these correlations are to be expected, since all of these outcomes are dependent (to varying degrees) on test-taking abilities. Thus it is much more impressive that IQ test performance predicts outcomes that are not dependent on formal test-taking abilities, such as rank and performance ratings obtained in military service (Gottfredson, 2003), job performance ratings and satisfaction (Schmidt and Hunter, 1998), income and life-long earnings (Murray, 1998), and even such distantly related outcomes as the inverse relationship between IQ and racist beliefs (Dhont and Hodson, 2014), obesity (Richards et al., 2009), clinical depression (Gale et al., 2009), the likelihood of developing cancer, and even death by automobile accident (Leon et al., 2009). Given these observations, it is not surprising that IQ is directly related to longevity (Wilson et al., 2009). This list of outcomes predicted by performance on the IQ test has been necessarily truncated, but the predictive validity of IQ tests have been discussed more extensively elsewhere (e.g., Gottfredson, 1998, 2003).
Like humans, it is now established that variations in general cognitive performance can be detected across individuals in a variety of animal species including mice (Galsworthy et al., 2002; Matzel et al., 2003; Sauce et al., 2018), rats (Anderson, 1993; Locurto and Scanlon, 1998), birds (Shaw et al., 2015; but see Sorato et al., 2018), dogs (Arden and Adams, 2016), and several non-human primates (Herndon et al., 1997; Banerjee et al., 2009; Herrmann et al., 2010; Beran and Hopkins, 2018; Eisenreich and Hayden, 2018; Damerius et al., 2019). Notably, while the literature on human intelligence is replete with examples of the relationship between IQ test performance and real-life outcomes, very few such demonstrations have been reported in non-human animals. The paucity of predictive validation of these studies of animal “general intelligence” has been noted by Burkart et al. (2016) and Locurto (2017), who wrote that “an important, even critical limitation of such studies is that they lack something that is commonplace in studies of human g [general intelligence] – namely, what is called predictive validity,” and this paucity of evidence compromises any assessment or conclusions about the nature of general intelligence in non-human species.
To the extent that it validates the utility of an intelligence test, the absence of data related to the predictive capacity of tests of non-human animal (hereafter “animal”) intelligence is certainly problematic. It is notable though that some limited data suggests that at least specific cognitive abilities predict outcomes that would have apparent survival value. For instance, mice with characteristically high intelligence (assessed as aggregate performance across a battery of cognitive tests) exhibit more effective foraging for food (Wass et al., 2012) and better avoidance of contact with aversive stimulation (Matzel et al., 2006), and mice that are more intelligent exhibit more exploratory behaviors in what is determined to be a “safe” environment (a behavior that would promote better contact with critical environmental contingencies; Light et al., 2011). Likewise, tests of general intelligence in cotton-top tamarin monkeys have included measures that have clear implications for effective social interactions (Banerjee et al., 2009). In the wild (where more direct evidence of survival skills can sometimes be obtained), similar relationships have been observed. For instance, male New Zealand robins with superior spatial memory have greater breeding success and provide an increased proportion of larger prey items to offspring (Shaw et al., 2019). Similarly, Cole et al. administered problem solving tests to great tits (Parus major), and successful problem solvers produced larger broods of chicks and were more efficient foragers for food (Cole et al., 2012; also see Ashton et al., 2018). Cauchard et al. (2017) demonstrated that the link between cognitive abilities and brood size was causal in nature, i.e., while birds of higher cognitive abilities tended to maintain larger broods, direct manipulation of brood size did not in itself promote increases in cognitive ability. Other than reproductive behaviors, cognitive abilities sometimes predict other survival-related skills. For instance, the performance of mountain chickadees in a spatial learning task was predictive of the likelihood of surviving the birds’ first winter (Sonnenberg et al., 2019), and longevity was predicted by spatial learning in male African striped mice, while in females of the species, performance on the same spatial task predicted the speed of their response to predators (Maille and Schradin, 2016).
Despite the seeming relationship between specific cognitive skills and survival, in other instances, specific cognitive abilities have not always predicted important functional outcomes such as song repertoire in birds that are dependent on these songs for reproductive success (MacKinlay and Shaw, 2019), and performance on a problem solving task did not predict mating success in male spotted bowerbirds (Isden et al., 2013). In one instance, pheasants that learned a reversal task more quickly were found to be less likely to survive in the wild (Madden et al., 2018). Thus consistent with Locurto’s (2017) concern, the assessment of the predictive validity of tests of animal intelligence has been non-systematic and has yielded inconclusive results. In part, this may be a reflection of the limited nature of the cognitive tests that have previously been used to assess these relationships. Other than in monkeys, studies of the relationship between survival-related behaviors and cognitive abilities have tended to be limited to the assessment of animals’ performance on single domain-specific abilities, e.g., spatial memory. (For a review of these and other relevant issues, see Orr, 2009; Thornton et al., 2014; Shaw et al., 2015).
A difficulty for the assessment of the predictive validity of tests of animal intelligence is that the administration of well-controlled and sensitive cognitive test batteries are facilitated by their administration to animals that are maintained in captivity, and this has been common in tests of mice, rats, dogs, and primates (although some exceptions have been reported in birds; e.g., Shaw et al., 2015). However, these captive (or protected) animals will not typically face the same demands on survival that would present themselves to wild animals, thus mitigating the study of ethologically-relevant survival-related outcomes. Moreover, many of the outcomes predicted by human intelligence tests are a product of the impact of intelligence on the integration and evaluation of available evidence and relevant information, e.g., the better diet selection (and less obesity/Type II diabetes) exhibited by more intelligent individuals is most certainly the product of more acquired awareness of dietary risks in addition to the better development of plans for mitigating those risks (for discussion, see Mackintosh, 1998). These same outcomes would not reasonably be anticipated in captive (or laboratory) animals, where things like diet availability are intentionally controlled (and a history of differential experiences cannot be drawn upon by the animals).
The present study represented our preliminary effort to assess the “functional” consequences of variations in mouse intelligence while still maintaining the laboratory conditions necessary to administer a controlled assessment of general cognitive abilities. Tests of survival-readiness were chosen that did not explicitly depend on prior experience and which had previously been suggested to have survival benefits (and/or which could reasonably be expected to impact the likelihood of survival in the wild, e.g., Deacon, 2006a,b). To this end, 56 genetically heterogeneous CD-1 mice were raised (under homogeneous conditions) in captivity and administered a diverse battery of cognitive tests (designed to characterize general cognitive ability) as young adults. Subsequently, these animals were administered a series of tests to assess unlearned skills related to survival, e.g., nest building in home and novel environments, foraging efficiency, exploration and food source discovery, food preference, and predator avoidance. Since these survival skills were nominally unlearned (a reasonable expectation in laboratory-reared animals), performance on these tests would provide an index of the extent to which general cognitive ability predicted functional (and survival-related) skills.
Materials and Methods
Subjects
A total of 56 CD-1 outbred male mice from Harlan Laboratories (Indianapolis, IN, United States) were used. Animals were housed individually in standard shoebox home cages in a temperature-controlled colony room using a standard 12 h light-dark cycle. These animals are well-suited for studies of individual differences as the CD-1 mouse genome displays patterns of linkage disequilibrium and heterogeneity similar to wild-caught mice (Aldinger et al., 2009). In this, our first attempt to assess survival-related behaviors, we focused on only male animals as we have extensive previous experience in the assessment of general cognitive abilities in these animals. Animals arrived in the laboratory at approximately 8–10 weeks of age and were given ad libitum access to food and water except during testing that required food deprivation, when animals were given 120 min access to food starting on the day prior to testing, then each day following data collection. Prior to the start of testing (which began at approximately 12–14 weeks of age), animals were handled (i.e., held by an experimenter while walking throughout the laboratory test rooms) for 60 s/day for 7 days to minimize any stress that arises from handling. All procedures were conducted with approval with the Institutional Care and Use Committee (IACUC) at Rutgers University.
Procedures
Two phases of testing were administered to all animals. The first phase of testing was designed to assess general cognitive ability and was comprized of three distinct cognitive tasks (that yielded seven measures of cognitive performance) that depended on different underlying processes. Performance measures (indicative of rate of learning or problem resolution) from these tests were entered into a principal component analysis, to (1) determine the degree to which a general factor influenced performance across all cognitive tests, and (2) to generate factor scores for each animal. A factor score is essentially an average z-score of each animal’s performance across all cognitive tests (where the individual tests are weighted according to their loading on the general factor). Thus these factor scores represent each animal’s general cognitive performance relative to all of the animals that contributed to this sample.
Upon completion of the cognitive assessment, all animals were then subjected to a series of tests intended to assess performance on tasks with clear implications for animals’ survival.
Cognitive Tests (Seven Dependent Measures)
The battery of cognitive tests employed here to assess general cognitive ability is somewhat different in nature than batteries that have been previously used in our laboratory, and notably, the performance in all of the cognitive tests in the current battery was motivated by food deprivation (whereas in prior batteries, several different motivational states were represented). It should be noted, however, that the current battery was compared to prior batteries and it was determined that the amount of cognitive variance accounted for by the present battery of tests was comparable in magnitude and structure to what has previously been reported (Crawford et al., 2020).
The sequence and nature of the cognitive tests are illustrated in Table 1.
The first three cognitive tests (that yielded six dependent measures) were conducted in a single piece of apparatus constructed as a convertible hybrid-style straight alley/Lashley maze. An illustration of this maze is provided in Figure 1. The tests administered in this maze included a simple discrimination task, egocentric navigation in a Lashley maze, a reversal of path direction in the Lashley maze, and two simple object-permanence puzzles.
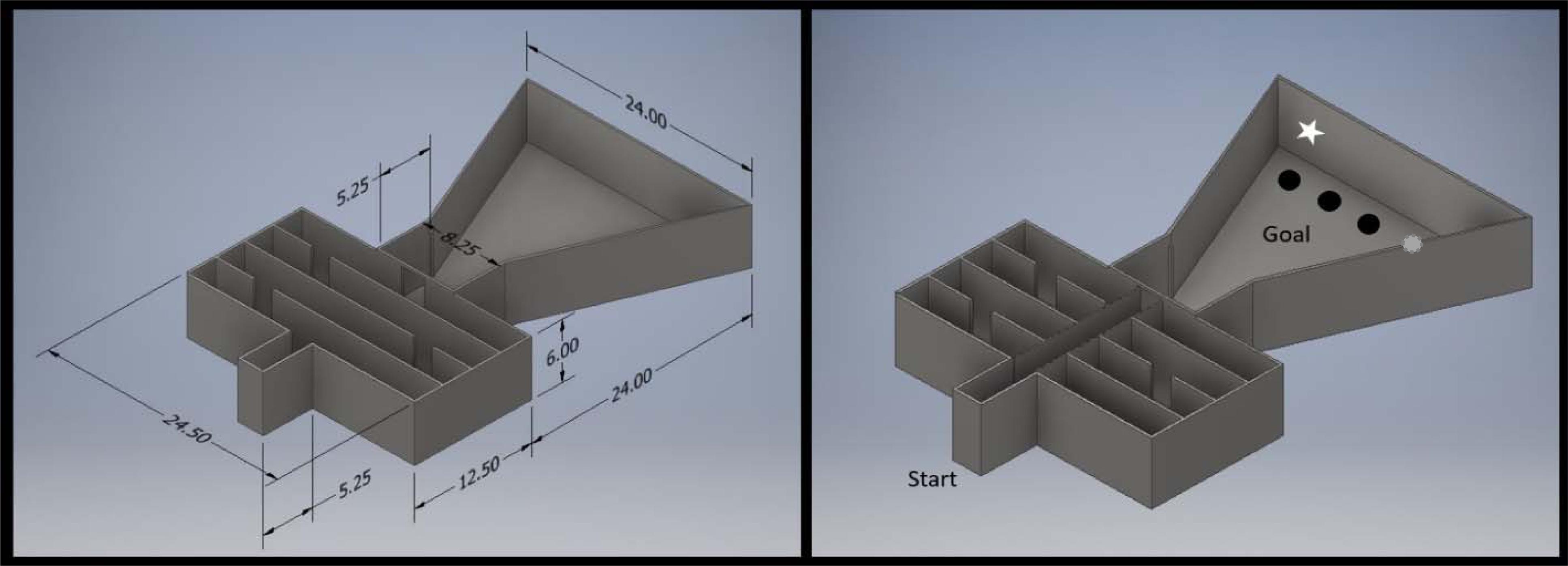
Figure 1. The straight alley and Lashley maze configurations of a single test chamber. The left panel illustrates the maze configuration, and the right illustrates the straight alley configuration. The start box is located near the left side of the maze, and the goal location is near the right. Depending on the task, either 1, 2, or 4 food cups were present at the goal location. In some tasks, discriminative cues were mounted on the wall behind the goal cups (illustrated by the star over Position 1). The apparatus was constructed of black Plexiglas and measurements are in cm.
Puzzle Solving in a Straight Alley (Yielding One Measure of Cognitive Performance)
Mice were placed in the start box of the maze for 5 s, after which the exit door was opened and the mice were free to traverse the alley. When mice reached the goal area of the maze, access to the alley was blocked to enclose them in the goal area. The goal area contained a single platform with a food dish holding one piece of accessible food and one piece of inaccessible food. Time taken by the mouse to retrieve the food was recorded. This was repeated for a total of five trials with a 6–10 min ITI for each animal. The first five trials in this maze were merely intended to establish directed approach to the food cup and an expectation that food would be located there. Trial 6 served as the critical measure of cognitive performance and proceeded as previous trials, with the exception of an added hexagonal lid (a plastic weigh boat) placed on top of the food cup. Of interest were the number of failures (“errors”) to remove the lid in order to collect the food reward. An error was scored if an animal placed at least two paws on platform and withdrew from the platform or if the animal made contact with the lid and failed to remove it. This trial was considered complete when the animal removed the lid and retrieved the food reward.
Simple Discrimination in Straight Alley (One Measure of Cognitive Performance)
Mice were again placed in the start box of the straight alley for 5 s and then released. On these trials, the goal box contained four platforms, each with one food cup on it. Each cup was baited with inaccessible food, while the cup marked by a discriminative cue also contained accessible food. During Trial 1, one cup (in position #3, counted from left to right) was covered with a hexagonal lid and baited with accessible food. This trial proceeded as previous trials in the straight alley, counting errors as previously described. Trials 2–12 had four cups in the goal area, all covered with hexagonal lids. One of the four cups were marked by a white star (35 mm diameter) on the wall behind and 20 mm above the food cup. This star served as the discriminative cue and marked the location of accessible food. Position of the target cup was randomly selected for each of these trials and standardized for all animals. Lid errors were recorded as previously described, and errors were also counted any time that the mouse made contact with a non-target lid. Errors could occur multiple times at a single lid provided that the animal stepped off the platform after making initial error (i.e., attempts at same lid without leaving the platform count as a single error). Average number of errors on Trials 6–11 served as each animal’s index of performance.
Lashley Maze (Yielding Four Measures of Cognitive Performance)
For this portion of testing the straight alley maze was converted to its Lashley maze configuration. A single platform with an uncovered food cup baited with reinforcer was placed in the center back of the goal area. This phase of training took place over 3 days in total, with 2 days of acquisition testing (Trials 1–12) followed by 1 day of testing (Trials 13–18) in which the learned response requirements were reversed.
For Trials 1–6 (Day 1), animals were placed in the start box, released, and allowed to traverse maze until it entered the arena and food was retrieved. Errors were recorded for the following actions: (1) back-tracking (complete reversal involving movement), (2) missed turn, i.e., passing a door without entering, or (3) wrong direction of turn (i.e., exiting a door and turning in the wrong direction). Errors were not compounded, i.e., if an animal missed a door (error), then back-tracked to return to that door, the back-track was “necessary,” and thus was not scored as an error. Likewise, if an animal made a wrong turn (error), it must back-track to return to the proper course and thus back-tracking was not an error. Errors could only occur if an animal was moving toward the goal, i.e., if the animal made an error that leads back through several doors before correcting and moving toward the goal, only the first error in the series was counted. Once the animal again progressed toward the goal, errors were again counted. Trials 7–12 (Day 2) followed this same procedure. A 20 min ITI intervened between each trial. The average number of errors committed on Trials 3–5 (Day 1) served as each animal’s index of acquisition. Trials 3–5 were used for this analysis as we have previously found that performance on these trials effectively capture differences between slow and faster learners (A complete description and rationale for our scoring methods can be found in Kolata et al., 2008).
On Trial 6 only, the food cup was covered by a blue ping-pong ball constituting a “puzzle” to be solved in order to obtain food. We recorded errors to enter the arena (as on all trials), then errors committed in solving the puzzle. An error was scored if an animal placed at least two paws on platform and withdrew from the platform or made contact with the ball and failed to remove it. Thus, errors were recorded if an animal approached a cup or made contact with the lid without retrieving the food. The trial was completed (and no error scored) if an animal successfully removed the ball to retrieve the food reward. Trials 7–12 were identical to Trials 1–5.
At the start of Day 3 in the Lashley maze, two “refresher” trials were administered (Trials 13–14) that followed the same procedure as the first 12 acquisition trials. The subsequent four trials (Trial 15–18) required that the animal reverse its previously learned path in order to obtain the food reward. A baited food cup was placed in what was previously the start box. Animals began the trial placed in the center of the goal area facing an empty food cup in the location of the previously baited cup. Two types of errors were recorded: (1) Approach errors were recorded every time the animal approached the old (now empty) food cup. This was constituted any time that an animal placed at least two paws on the platform and withdrew or when its nose crossed the plane of the cup wall (in cases where the animal did not step on to the platform). (2) Maze errors were recorded as the animal traversed the maze toward the new goal location (in what was previously the start box). These errors were scored as they were during forward Lashley maze training, although in this instance, the correct route was reversed. Animals were allowed to find and consume the food, and then removed to begin their inter-trial interval.
Decision Tree Maze (One Measure of Cognitive Performance)
Upon completion of testing in the Lashley maze, an additional cognitive test was administered in a distinct piece of apparatus (a decision tree). The Decision Tree maze is a “tree” shaped maze constructed from black Plexiglass with a start box and series of bifurcating arms at seven symmetric locations, “nodes,” after an initial split dividing the maze in two symmetrical halves (see Wass et al., 2012, for an illustration of the maze). Before the initial division in the maze sits an alley that originates from a starting box with a removable door where mice begin the test. At each of the 14 nodes within the maze (located at splits and at the end of arms), a small hole (3 mm wide × 3 mm deep) was drilled to hold a recessed 14 mg Noyes pellet that serves as the food reward, a random selection of which were baited on any given trial. This test involves mice navigating the branch-like structured maze to inspect each node for food. The object of this test is for mice learn the overall structure of the maze and formulate the optimally efficient path through which it can search each potential food deposit while using the lowest amount of time and energy possible. Mice with high general intelligence will explore the maze in efficient paths (i.e., cross the same node only en route to an unexplored node) while mice with lower intelligence will often take meandering paths and make many errors (unnecessarily crossing a node) in exploring the maze. This maze has previously been shown to load heavily (0.49) on a factor analysis describing a general intelligence factor, and the efficiency with which an animal searches the maze has been said to be emblematic of inductive reasoning (Wass et al., 2012).
Animals were food deprived for approximately 16 h before testing began. Testing in this maze lasted 5 days, with animals being tested in two trials per day for a total of 10 trials. On Trial 1, mice were placed in the start box for 10 s before opening the gate to the maze. On this trial, all 14 nodes were baited with food. The path taken by the animal was recorded until all food pellets were retrieved, and then the mice remained in the maze for 12 min to allow further exploration. Mice were then removed and placed back in its home cage in the testing room for the 10 min ITI.
Trials 2–10 involved a similar procedure to trial one, with two exceptions. The first exception is that during these trials, only four to eight of the nodes were baited with food. Standard arrangements of the food baiting were used to ensure consistency among animals. The number and location of nodes to be baited during each trial were selected randomly. The second exception from Trial 1 was that these trials were not subjected to the 12 min time requirement. Rather, these trials ended when all available food had been eaten and all nodes had been explored.
The path an animal takes to explore each node in the maze were recorded. On each trial we recorded the “streak,” or number of node crossings an animal made before making an unnecessary node crossing. For the present purpose, the average streak length on best two of Trials 7–10 served as the dependent measure of each animal’s performance.
Survival-Related Tests (Nine Measures)
Survival-related measures were obtained in the animals’ home cages as well as three novel environments. In total, nine measures of survival-readiness were obtained. The sequence and nature of these tasks are illustrated in Table 2.
Nest Building in the Home Cage (One Measure)
This procedure is based on one described by Deacon (2006a), who argued that proper nest building (which is performed by both male and female mice) conserves body heat and protects animals from predation, both of which facilitate survival in the wild.
Mice in this study had no prior experience with nesting material other than the shredded cob bedding that lined their home cages. Approximately 60 min prior to the onset of the dark cycle, a 3 g compressed cotton pad (Oasis Shred-a-Bed) was placed against the front wall of the animals’ home cages. Even with no prior experience, mice characteristically shred these pads and use the material to construct nests. The pad remained in the home cage throughout the ensuing dark cycle. 2 h after the onset of the light cycle, the quality of the nest was recorded using the 5-point scale recommended by Deacon (2006a), where a score of 1 is assigned to an animal that has not noticeably touched the pad (more than 90% intact), and a score of 5 is assigned to an animal that has constructed a near-perfect nest with more than 90% of the pad shredded, and the nest forming a crater occupying 25% or less of the cage floor, with at least 50% of the walls higher than the prone mouse’ body height. Intermediate scores (i.e., between two whole numbers) were used when a nest was judged to be intermediate between any two points on the rating scale.
Exploration and Food Retrieval in a Burrowing Box (Two Measures)
Two adjoining white Plexiglas boxes (20 cm l × 13 cm w × 10 cm h) were connected by a 2.5 cm diameter ×10 cm long tube that emerged through the floor of each box on either side of the adjoining wall. The wall that divided the two boxes was perforated to facilitate the transmission of odors between the two sides of the box. Testing in this box was performed on two consecutive days. On Day 1, each mouse was placed in one box and allowed to freely explore throughout a 12 min session. The dependent measure obtained was the latency for the animal to first cross the tube and enter to other box (recorded when all four paws made contact with the floor of the box). This measure was essentially an index of exploratory tendencies in a novel environment. Upon completion of Day 1 testing, each animal began a 20 h period of food deprivation. Day 2 of testing was similar to Day 1 with two exceptions. First, the tube that connected the two boxes was filled with shredded cob bedding. Second, the side of the box opposite to the start side had in it one gram of standard lab chow. The mouse was started in the empty side of the box, and of interest was the latency to burrow through the occluded connecting tube and retrieve a piece of food in the opposite box. This trial had no time constraint and was ended when the mouse retrieved the piece of food.
Food Retrieval and Nesting in a Novel Hoarding Box (Four Measures)
Given access to a food supply outside of the home environment, mice will typically retrieve that food and return it to their nesting area. Moreover, when given a choice, mice tend to reject less attractive food in favor of more attractive food. These species-typical behaviors are said to have obvious survival value (Deacon, 2006b). To assess these behaviors in laboratory mice, Deacon (2006b) developed a procedure in which mice are introduced to and housed in a novel environment, and are given access to a tunnel (that protrudes from the nest area) that terminates in a batch of familiar food. In Deacon’s description of this task, the mice were mildly food deprived and allowed approximately 12 h to engage in food retrieval, after which was recorded the amount of food (from a 100 g source) that was returned to the home box. In a preliminary attempt to employ this procedure, we observed that all mice returned all food by the end of the 12 h interval. Consequently, we made several modifications to the procedure and also collected several other measures related to hoarding and survival in a novel environment. First, we assessed nest building shortly after introduction to the novel box. (This procedure was identical to the procedure used in the home box except that the quality of the nest was assessed 2 h after introduction in the novel box, a behavior that would have important survival benefits when an animal is moved from its familiar home nest.) Second, for the first hoarding test animals were not food deprived and two measures were obtained, including the latency to first contact food after given access to the tunnel, and the amount of food retrieved was recorded after 2 h of access. (In preliminary studies we observed significant variability across animals in the amount of food retrieved at this interval). Access to the tunnel was then blocked. 20 h after the initial bout of hoarding, the mice were again given access to the tunnel and again allowed to retrieve food. However, in this instance the animals had access to 16 food pellets, eight of which were 500 mg, and eight of which were 100 mg of interest was any observed preference for the large pellet among the first eight pellets returned to the home cage.
Apparatus was similar to that described by Deacon (2006b). A test box (30 × 13× 15 cm) was constructed of white Plexiglas with a clear lid covered in orange acetate (which darkened the interior of the box). The floor of the box was lined with shredded cob bedding. A 50 cm long ×5 cm diameter wire mesh (6 mm grid size) tunnel extended from the end of the home box and terminated in an 10 cm long black Plexiglas tube with a sealed end (serving as a food cup). Access to the tunnel could be blocked with a black rubber stopper.
On Day 1 (approximately 4 h after the start of the light cycle), each mouse was transferred from its home cage to the test apparatus (where it would remain for three consecutive days). The home cage bedding was transferred to the test apparatus along with 400 ml of additional fresh bedding and a Shred-a-Bed nest pad. The test box contained the animal’s regular food (four pellets) and a water spout. Four pieces of novel food (Hartz Small Animal Diet for Guinea Pigs; two 100 mg pellets, and two 500 mg pellets, designated “small” and “large” test pellets) were also present. (This food type would be used in a later test and was provided at this time to mitigate any neophobic responses). Entrance to wire tunnel was blocked. 2 h after introduction of a mouse to the test box, nest quality was scored in the manner described above.
On Day 2, 100 g of the animals’ standard diet (pellet size 1.5–3.0 g) was placed in food cup at end of the wire tunnel. Mid-way through the light cycle, the rubber plug was removed allowing the animal access to the tunnel. The latency for each animal to traverse the tunnel to reach the food was then recorded. 2 h later, the tunnel was again blocked and food pellets that remained in the tunnel were weighed; this weight was subtracted from the original 100 g to yield a measure of successfully hoarded food pellets (i.e., the pellets that had been returned to the nest area). After this test, all food was removed from the nest box. Each animal was then provided one large (500 mg) and one small (100 mg) test food pellet (Hartz Small Animal Diet).
On Day 3 (18 h after removal of food from the home test box) two columns of 8 test food pellets were placed in the food cup at the end of the wire tunnel. The rows of the two columns alternated between small and large pellets. The rubber plug was then removed from the wire tunnel allowing each mouse access, and the size of the first eight pellets retrieved was recorded. For purposes of scoring, each large pellet was worth 1 point, whereas small pellets were worth 0 points, yielding a maximum score of “8” for each animal.
Predator Avoidance in a Social Box (Two Measures)
In their natural environments, mice are the target of predation by rats. Even without prior experience with the predator, mice will avoid areas marked by the odor of rats (Papes et al., 2010). This native defensive tendency was assessed here where mice could approach or withdraw from a live rat. A mouse was placed in the center chamber of a 3-chamber box, in which the mouse could freely move between the chambers. One of the chambers that adjoined the center contained a rat restrained in a wire tube, while the opposite chamber adjoining the center contained a cotton wad similar in size to that of the rat inside a wire tube. Two behaviors were of interest. First, we recorded the latency to first exit the center chamber when both adjoining chambers were empty (Day 1), and second (on Day 2), we recorded the difference in time spent in the chamber containing the rat relative to the chamber containing the cotton wad.
This test was conducted on two successive days, the first of which was intended to acclimate the mouse to the test chambers and to obtain a measure of exploration in a novel environment. Each mouse (in its home cage) was placed in the testing room under dim light for 10 min prior to testing. The predator avoidance box was a 60 × 40 × 24 cm clear Plexiglas box divided into three 20 × 40 × 24 cm sections. A small door (15 cm square) allowed access from the center chamber into each of the adjoining chambers. Each of the chambers that adjoined the center contained a wire mesh container (6 mm grid, 18 cm diameter ×22 cm high). These containers were empty on the acclimation day. Each mouse was placed in the center chamber and allowed to explore the box freely for 10 min. We recorded the latency (in sec) for the animal to leave the center chamber and enter either of the two adjoining chambers (the mouse was considered to have entered a new chamber when all four paws were on its floor). At the conclusion of the 10 min of acclimation, the mouse was returned to its home cage.
On Day 2, the box was arranged as during Day 1, with the addition of a live Sprague-Dawley (Crl:SD) male rat in the wire mesh container in one of the side chambers, and a rat-sized white cotton bundle in the container in the opposite side chamber. The mouse was again placed in the center chamber and allowed to explore the box for 10 min. Of interest was the time spent in the chamber with the cotton wad minus the time spent in the chamber with the rat. A score approaching 10 min would reflect maximum avoidance of the rat. After the 10 min test the mouse was returned to its home cage.
Statistical Analyses
All statistical analyses were completed using IBM Statistics Version 25. The dependent measures from each of the cognitive tasks were entered into a principal component analysis to obtain an estimate of the influence of a general cognitive factor and to compute factors scores which reflect the aggregate (general) cognitive performance of each mouse relative to the other mice in this sample. Next, the performance measures of survival-readiness were entered into a principal component analysis to determine the existence of a “general survival-readiness factor” and to compute factor scores on this dimension (indicative of an animal’s relative survival-readiness). The degree of correlation between general survival-readiness and general cognitive ability could then be assessed. We also examined the correlations between general cognitive factor scores and each measure of survival-readiness to determine which, if any, of those individual behaviors were predicted by general cognitive ability.
In some instances, better performance on a task was indicated by a lower score (e.g., fewer errors), whereas in other instances, better performance on a task was indicated by a higher score (e.g., more food retrieved). This complicates the interpretation of correlations, as although better performance on one task might predict better performance on a second task, in one instance this would be reflected in a negative correlation whereas in another instance this would be reflected in a positive correlation. To simplify the presentation (for instance, the correlation matrix presented in Table 3), in all cases, correlations are presented such that positive values mean better performance on one value predicts better performance on the other. This was accomplished by inverting raw values in some instances.
Results
Fifty-six male mice contributed to this analysis. All 56 contributed data on all cognitive tests. For tests of survival-readiness, one animal was removed from the study due to illness, yielding ns = 55 on all tests except “Hoarded weight,” where due to a procedural error, data from eight animals was lost, yielding an n = 47 on that test. Principal component analyses require that all subjects contribute to all dependent variables. Consequently, the principal component analysis of cognitive abilities was based on an n = 56, whereas the analysis of survival abilities was based on an n = 47. In one instance, we compared aggregate performance on the cognitive tests (based on factor scores) to aggregate performance on survival-readiness tests (see Figure 2, described below). For this purpose, only the 47 animals that were represented in both sets of tests were included in the analysis.
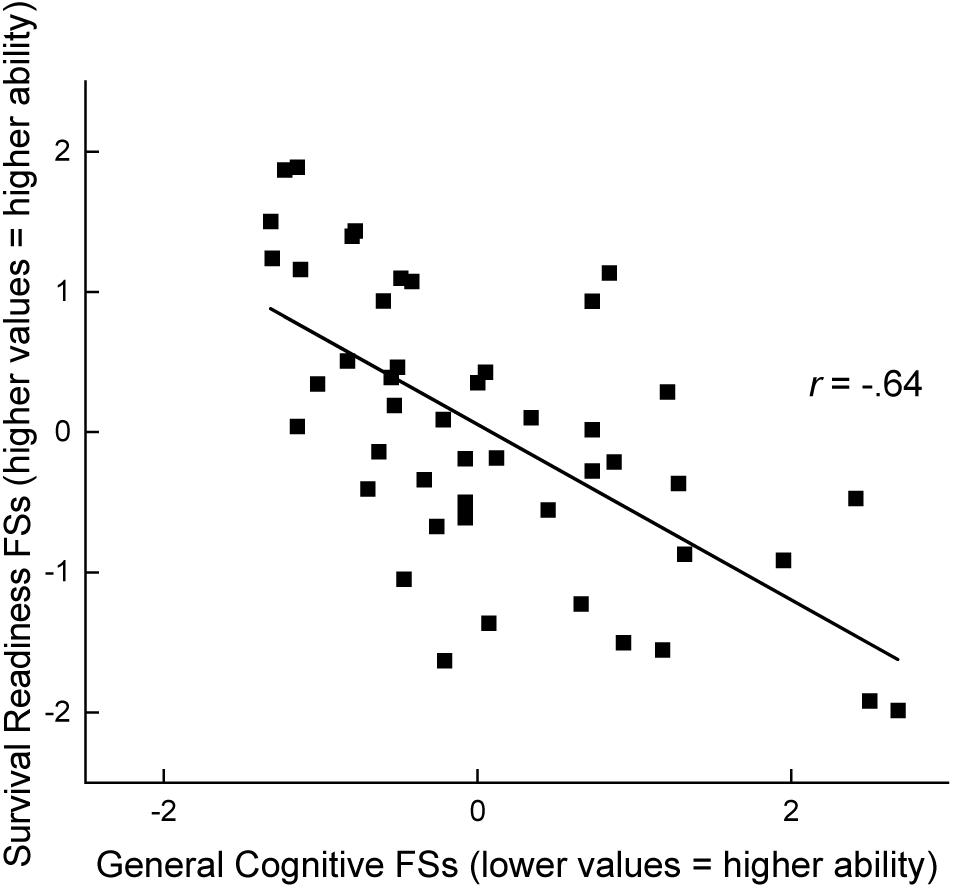
Figure 2. Factor scores for general cognitive ability and general survival-readiness are compared. A correlation was observed indicating that better overall cognitive ability predicted better overall performance on tests of survival skills (n = 47).
General Cognitive Ability
Table 3 presents the results of the principal component analysis of all seven cognitive measures.
As evident from Table 3, the performance on all cognitive tests loaded moderately to strongly on a principal factor, indicative of a common influence on performance across all tests. This general factor accounted for ∼29% of the variance in performance across all tasks. This degree of variance is comparable to that observed using batteries of tests that were very dissimilar to the one used here (e.g., Kolata et al., 2008; Sauce et al., 2018). This is notable since in the present battery, all of the cognitive tests were motivated by food rewards, which could make it difficult to distinguish between a general cognitive factor and a general motivational factor. However, the results obtained from other test batteries were based on tasks that depended on at least three different motivational systems. Thus it is reasonable to conclude that the present results are a reflection more of cognitive ability rather than a common motivational state.
General Survival-Readiness
Table 4 presents the results of the principal component analysis of all nine measures of survival-related behaviors.
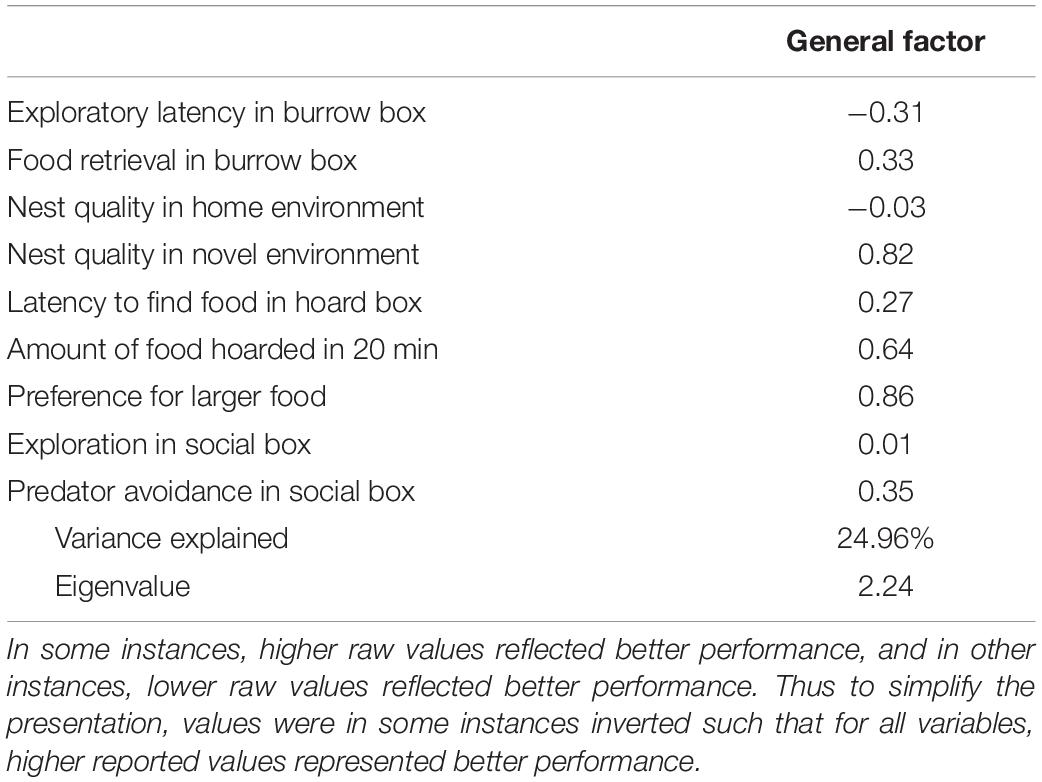
Table 4. Principal component analysis of nine survival-related tests (eight animals did not contribute a score on the amount of food hoarded, resulting in 47 animals that contributed scores to all tests).
As evident from Table 4, the performance on many measures of survival-readiness loaded moderately to strongly on a single factor that accounted for ∼25% of the variance in performance across all tests. This suggests the existence of a “general survival-readiness” ability that would influence an individual’s capacity for survival. Notably though, three of the measures loaded negligibly or in the opposite direction on this factor, indicating a less than universal influence on these survival-related behaviors.
The Relationship Between General Cognitive Ability and General Survival-Readiness
Forty-seven animals contributed scores on all of the cognitive tests and all of the survival-readiness tests. Of particular interest was the relationship between general cognitive abilities and general survival-readiness. To understand this relationship, we assessed the correlation between factor scores obtained on the cognitive test battery and factor scores derived from tests of survival-related behaviors, yielding r (46) = -0.64, p < 0.01. This correlation indicates that higher cognitive abilities (reflected in lower values of performance, e.g., fewer errors, and thus lower factor scores) predicts better performance in tests of survival-readiness (reflected in higher values of performance, e.g., a higher quality nest, thus higher factor scores). The relationship of these two variables is illustrated in Figure 2.
To demonstrate the consistency of animals across tasks in the cognitive battery and the survival-readiness battery, as well as the relationship of these two trait, in Figure 3 we illustrate the relative performances of two animals, one of which (#29) was one of the best performers in the cognitive battery, and one of which (#61) was the worst performer in the cognitive battery. These designations were based on factor scores extracted from performance on the battery of cognitive tests. These scores ranged from -1.83 [best general cognitive performance] to +2.67 [worst general cognitive performance]. Subject #29 received a factor score of -1.31, and Subject #61 received the factor score of +2.67. The subject that performed best on the cognitive battery (factor score of -1.83) could not be used for this analysis as it did not contribute one score on the survival-readiness battery of tests). Subjects #29 and 61 were assigned a rank based on its performance relative to all other animals on each of the cognitive tasks and each of the survival-readiness tasks. These ranks are illustrated in Figure 3. As this figure illustrates, Subject #29 performed near above the median (near the top of the distribution) on each of the cognitive tests, and also performed above the median on each of the survival-readiness tests. Subject #61 performed below the median on all of the cognitive tests, and also tended to perform poorly on most of the survival-readiness tests.
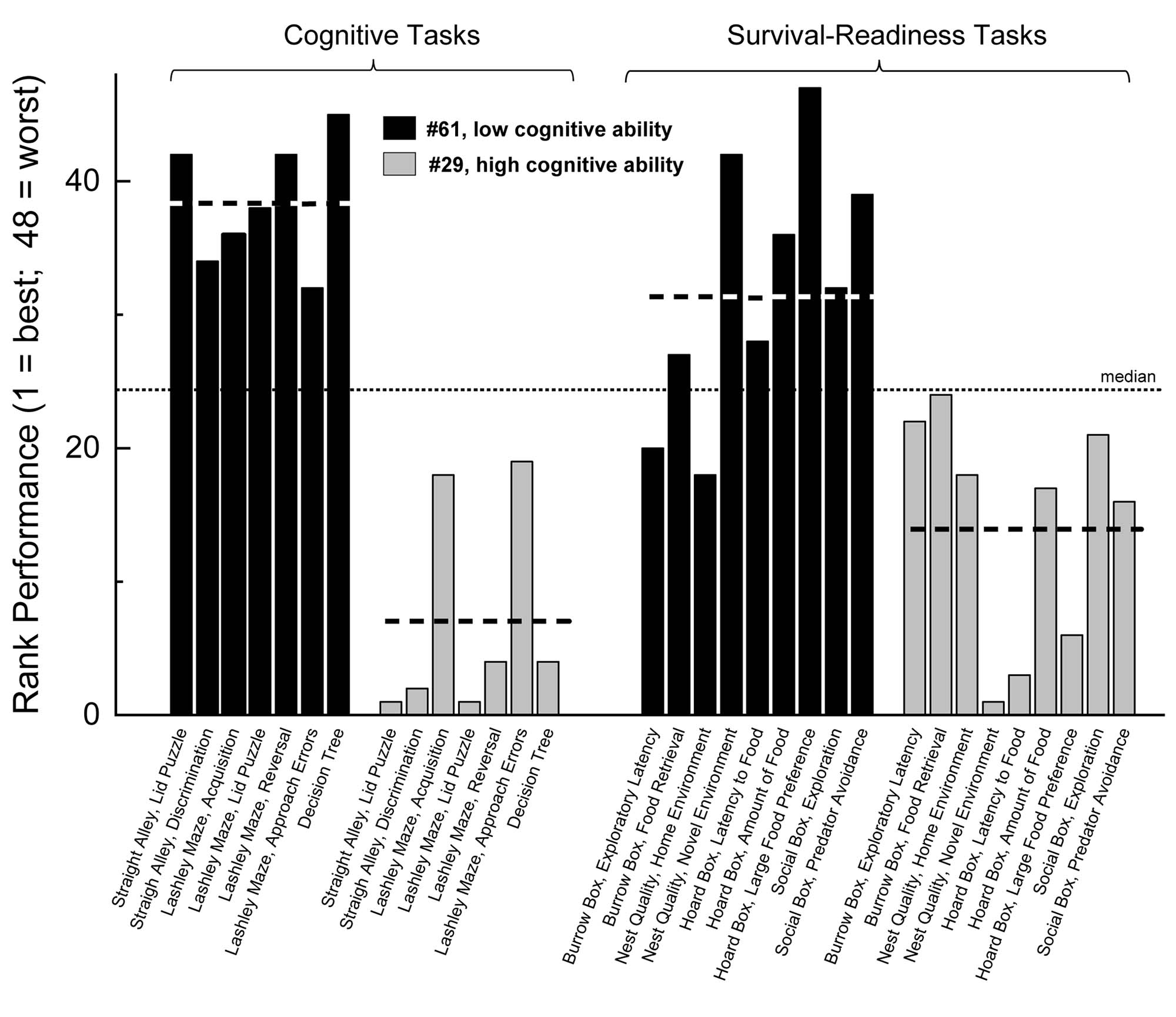
Figure 3. Subject #29 (high general cognitive abilities, as determined by factor scores obtained from the PCA of cognitive tasks) and Subject #61 (low general cognitive abilities) were ranked relative to other animals in this sample (n = 47) on each cognitive (left sets of bars) and survival-readiness task (right sets of bars). The heavy dashed line represents each animal’s mean rank on that set of tasks, and the light dashed line is the expected median for the sample of 47 animals. Subject #29 performed well above the median on each of the cognitive tasks, and also performed well on each of the survival readiness tasks. Subject #61 performed around the median or poorly on each class of tests.
General Cognitive Ability and Performance on Individual Tests of Survival-Readiness
Finally, we examined the relationship between general cognitive ability and performance on individual tests of survival-related behaviors, as well the relationship between the various measures of survival-readiness. A matrix of correlations of all relevant variables (i.e., general cognitive factor scores and all measures of performance on tests of survival-readiness) is presented in Table 5.
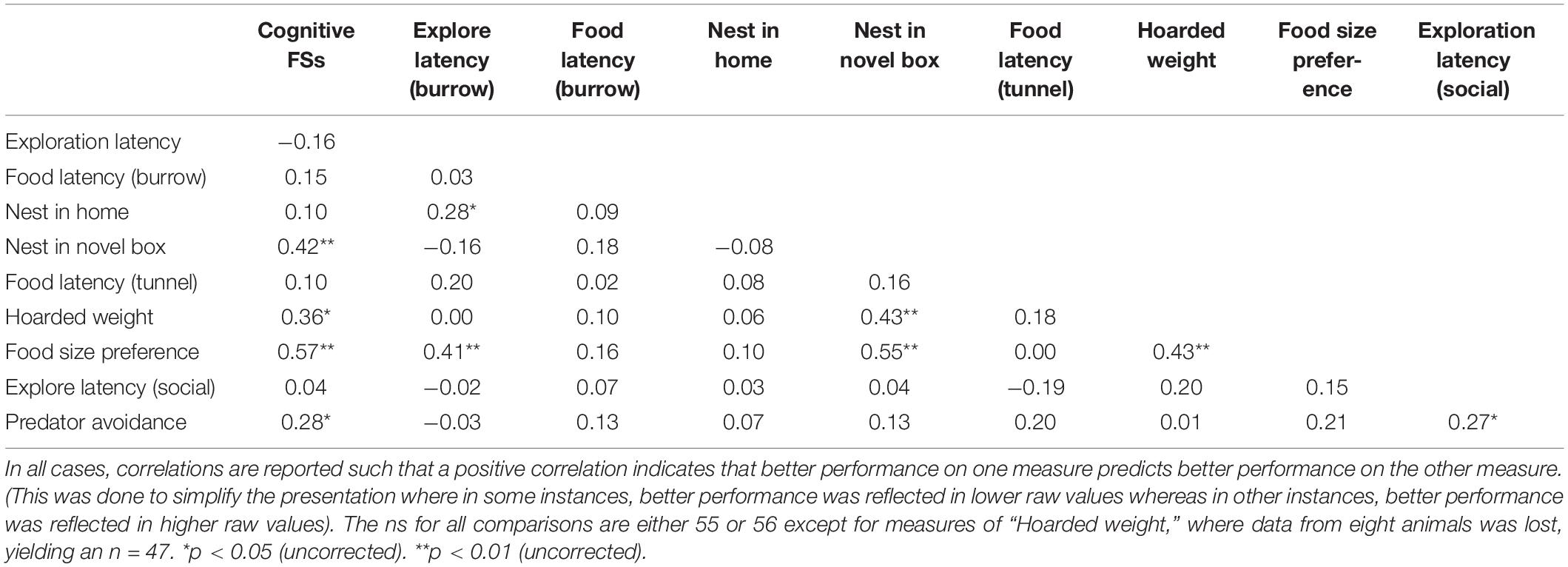
Table 5. A correlation matrix of cognitive factor scores (indicative of general cognitive ability) and all measures of survival-related behaviors.
The correlation matrix presented in Table 5 is a mixed set of results. Most correlations were positive (suggesting a common influence on general cognitive ability and all measures of survival-readiness), although several negative correlations were observed and most correlations were not significant (and weak). However, general cognitive ability did significantly predict several measures of survival-readiness, including nest building in a novel environment, the amount of food hoarded in a 20 min interval, the degree of preference for larger portions of food, and predator avoidance. Notably, most of the strong correlations between cognition and measures of survival-readiness were observed in those cases where survival-related behaviors were assessed in an environment that was unfamiliar to the mouse. This suggests the possibility that distractions that arise in a new environment (but which are minimal in a familiar environment) are more easily overcome in animals with higher cognitive abilities. This and other possibilities are considered more fully below.
General Discussion
It had been noted by several authors (e.g., Burkart et al., 2016; Locurto, 2017) that measures of “intelligence” in non-human animals have suffered from the lack of independent verification of the impact of those measures on outcomes that were independent of the intelligence test itself. While some exceptions were noted above, this criticism was certainly true of laboratory assessments performed on mice, is thus it is an important concern to address. An impediment to such an analysis arises when working with laboratory mice, from which is difficult (or impossible) to assess obvious practical outcomes of variations in intelligence, e.g., the relationship of intelligence to survival in the wild (which could be expected to be impacted by variations in cognitive abilities). Here we took an intermediate approach, i.e., intelligence was assessed in laboratory mice, and then these same mice were assessed for performance on unlearned behaviors that could be reasonably expected to impact survival in the wild.
In the present study, genetically heterogeneous laboratory mice were assessed on a battery of cognitive tests (where individual’s aggregate performance served as an index of “intelligence”) and were then assessed on a number of tasks relevant to nest building (in familiar and unfamiliar environments), exploration in novel/familiar environments, food discovery, efficiency of food retrieval and preference, and predator avoidance. Better performance on these tasks might promote survival-readiness under more natural (non-laboratory) conditions. Several key observations arose from this analysis. First (and as in many previous reports, e.g., Matzel et al., 2006, 2008; Kolata et al., 2007, 2008), a single factor was found to contribute to performance on all cognitive tests. Similarly (and second), a general “survival-readiness” trait was also identified, i.e., a single source of variance was found to exert an influence on some measures of unlearned survival-related skills, accounting for 24.96% of the variance among individuals across nine different tasks. Thirdly, and of principal relevance to our intentions here, general cognitive abilities were strongly predictive of general survival-readiness (r = -0.64). This latter observation suggests that animals with higher cognitive abilities (all other things equal) would indeed exhibit a higher likelihood of survival in the wild.
The analysis of the relationship between general cognitive ability and specific survival-related tasks is not entirely straightforward. For instance, nest building in the home cage was unrelated to general cognitive ability (r = 0.10), while nest building in a novel environment was significantly predicted by an individual’s general cognitive ability (r = 0.42). Although many differences characterize these different tasks, a distinguishing feature is that general cognitive ability appears to have a stronger influence on tests of survival-readiness when those later tests are administered in unfamiliar settings. We have previously suggested that attentional abilities may be a principal determinant of variations in mouse intelligence (Kolata et al., 2005, 2007; Sauce et al., 2014; for review, see Matzel and Kolata, 2010; Matzel and Sauce, 2017), a relationship that may also exist among humans (Engle, 2002, 2018; Cowan et al., 2005, 2006; Shipstead and Engle, 2013). Lapses in attention (which could be exacerbated in unfamiliar environments with new distractions) might explain the relationship between general cognitive ability (and its dependence on attention) and survival skills.
In addition to variations in general cognitive ability, other “general” influences might account for the relationship between performance on the cognitive battery and survival readiness. Notably, it is conceivable that variations in stress reactivity or anxiety might contribute to general cognitive performance and thus might underly the relationship to survival readiness. However, our prior work suggests that this is unlikely. In a series of papers, general influences such as stress reactivity, exploratory tendencies, and anxiety have been dissociated from the principal factor in three ways. First, nominal measures of fear/anxiety/stress do not load (or load weakly) on the principal factor that captures general cognitive performance (Matzel et al., 2006). Second, pharmacological reductions in anxiety or stress reactivity do not promote increases in general cognitive performance or change the pattern of loadings on the general cognitive factor (where the cognitive tasks are differentially dependent on fear-motivated responses; Grossman et al., 2007). Lastly, Light et al. (2008) found that behavioral interventions that reduce fear/stress/anxiety do not change the pattern of loading on the principal factor that represents general cognitive performance. These results have led us to favor the interpretation that the general factor that describes performance across our batteries of cognitive tests is reflective of a general influence on cognitive abilities.
The potential relationship between attention and survival-readiness might be best described with an anecdotal description of mouse behavior. We have consistently observed that mice that are classified as expressing low general cognitive abilities seem to have difficulty maintaining directed behavior (an observation that is consistent with empirical evidence, e.g., Kolata et al., 2007; Matzel et al., 2008; Light et al., 2010). For example, in a straight alley (a very simple test of learned behavior in which a mouse runs in a straight line to a food reward), dull mice often appear to lose focus, e.g., in the course of a run they may stop and rear or engage in bouts of grooming. Thus even on such a simple task, a dull mice will exhibit more between-trial variability, and “worst” performance might best distinguish between high and low intelligence individuals (a tendency that has been repeatedly observed and quantified in humans’ performance on many cognitive tasks; Juhel, 1993; Coyle, 2003). Relatedly, it is possible that in a new environment, an animal could be more distractible (and thus less directed) than would be the case in a familiar environment. This suggests the possibility that distractions inherent to a new environment (but which are absent from a familiar environment) are more easily overcome in animals with higher cognitive abilities. In total, the possibility that attentional variation is the common factor that mediates the relationship between intelligence and expression of survival skills seems both plausible and parsimonious with the range of available data.
Of the nine tests of survival-readiness used here, three of those tests (the latency to cross sides in the burrowing box, the latency to traverse a tunnel in the hoarding box, and the latency to leave the center compartment in the social box) were thought to be primarily dependent on the propensity for exploration (which of course can be influenced by stress, fear, or anxiety; Matzel et al., 2006; Grossman et al., 2007). While other tests of survival-readiness used here had obvious survival benefits, the relationship of exploration to survival is more complex. Exploration (or “novelty seeking”) could be beneficial under some circumstances, but could be detrimental in others (necessitating a balance between “too little” and “too much”). In this regard, we have reported that variations in “exploration” may in fact be a consequence of differences in rate of learning, such that more competent animals evaluate their environment more quickly and are thus more likely to engage in exploration when conditions have been deemed appropriate (Light et al., 2008, 2011). This interpretation is consistent with our regular observation that exploration covaries with general cognitive ability (e.g., Matzel et al., 2003). In this regard, it is quite surprising that none of the measures of exploration employed here were related to general cognitive performance and did not load appreciably on the principal component analysis of survival-related behaviors. One possible explanation of this result is consistent with previous data of Light et al. (2011) who reported that the relationship of exploration and general cognitive ability is diminished as animals spend more time in a novel environment, i.e., after the environment becomes familiar. In the present study, animals had extensive experience in environments (during tests of cognitive abilities) similar to those utilized for later tests of exploration, and thus their novelty might have been minimized. Regardless of the explanation, the results obtained here suggest that the relationship between cognitive abilities and exploration might be less universal than we had previously believed. Importantly, we note that previous research suggests general cognitive abilities of individuals may be differentially impacted by socialization paradigms present in natural environments (e.g., Fitchett et al., 2005; Chida et al., 2006). One of us has previously reported that predisposition to submission within a social hierarchy predicts superior cognitive performance (Matzel et al., 2017). One possible explanation for this result was that survival-readiness strategies reliant on cognitive abilities may have evolved to facilitate the survival of those not predisposed to benefit from strategies that favor dominant physical or social abilities. As such, the social environment of an animal likely plays an integral role in its survival strategy. Based on the results reported in the present study it is possible, for example, that an animal with a predisposition for high cognitive ability reared in an environment free of socially dominant peers may enjoy a high degree of survival-readiness (perhaps even enhanced by socialization, given the relationship between socialization and improved cognitive ability; see Voiker et al., 2005; Chida et al., 2006), while that same animal reared with exposure to social submission may experience decreased survival-readiness (given that social submission can impair cognitive performance; see Fitchett et al., 2005; Colas-Zelin et al., 2012).
In a general sense, it is unlikely that genes common to both survival skills and intelligence could mediate the relationship between these two sets of variables. In any nominal way, it seems unlikely that the same genes could regulate tasks as dissimilar as those that constitute tests of cognition (e.g., the Lashley Maze) and tests of survival skills (e.g., nest building). However, while these different traits are almost surely regulated independently, they may also be regulated in common by networks of genes that could impact processes like attention. While the heritability of mouse intelligence (e.g., Galsworthy et al., 2002, 2005; Sauce et al., 2018; Matzel et al., 2019) suggests a strong genetic influence on the expression of this trait, the heritability of survival skills in mice is presently unknown (but is currently under investigation in our laboratory).
Data Availability Statement
The datasets generated for this study are available on request to the corresponding author.
Ethics Statement
The animal study was reviewed and approved by Rutgers IACUC.
Author Contributions
LM and DC performed data analyses and wrote the manuscript. LM, DC, HP, MP, MM, and AT maintained animals and collected data. All authors contributed to the article and approved the submitted version.
Conflict of Interest
The authors declare that the research was conducted in the absence of any commercial or financial relationships that could be construed as a potential conflict of interest.
References
Aldinger, K. A., Sokoloff, G., Rosenberg, D. M., Palmer, A. A., and Millen, K. J. (2009). Genetic variation and population substructure in outbred CD-1 mice: implications for genome-wide association studies. PLoS One 4:e4729. doi: 10.1371/journal.pone.0004729
Anderson, B. (1993). Evidence in the rat for a general factor that underlies cognitive performance and that relates to brain size: intelligence? Neurosci. Lett. 153, 98–102. doi: 10.1016/0304-3940(93)90086-z
Arden, R., and Adams, M. J. (2016). A general intelligence factor in dogs. Intelligence 55, 79–85. doi: 10.1016/j.intell.2016.01.008
Ashton, B. J., Ridley, A. R., Edwards, E. K., and Thornton, A. (2018). Cognitive performance is linked to group size and affects fitness in Australian magpies. Nature, 554, 364–367. doi: 10.1038/nature25503
Banerjee, K., Chabris, C. F., Johnson, V. E., Lee, J. J., Tsao, F., and Hauser, M. D. (2009). General intelligence in another primate: individual differences across cognitive task performance in a New World monkey (Saguinus oedipus). PLoS One 4:e5883. doi: 10.1371/journal.pone.0005883
Beran, M. J., and Hopkins, W. D. (2018). Self-Control in Chimpanzees Relates to General Intelligence. Curr. Biol. 28, 574.e–579.e.
Burkart, J. M., Schubiger, M. N., and van Schaik, C. P. (2016). The evolution of general intelligence. Behav. Brain Sci. 40, 1–65.
Cauchard, L., Angers, B., Boogert, N. J., Lenarth, M., Bize, P., and Doligez, B. (2017). An experimental test of a causal link between problem-solving performance and reproductive success in wild great tits. Front. Ecol. Evol. 5:107. doi: 10.3389/fevo.2017.00107
Chida, Y., Sudo, N., Mori, J., and Kubo, C. (2006). Social isolation stress impairs passive avoidance learning in senescence-accelerated mouse (SAM). Brain Res. 1067, 201–208. doi: 10.1016/j.brainres.2005.10.042
Colas-Zelin, D., Light, K. R., Kolata, S., Wass, C., Denman-Brice, A., Rios, C., et al. (2012). The imposition of, but not the propensity for, social subordination impairs exploratory behaviors and general cognitive abilities. Behav. Brain Res. 232, 294–305. doi: 10.1016/j.bbr.2012.04.017
Cole, E. F., Morand-Ferron, J., Hinks, A. E., and Quinn, J. L. (2012). Cognitive ability influences reproductive life history variation in the wild. Current Biology, 22, 1808–1812. doi: 10.1016/j.cub.2012.07.051
Cowan, N., Elliott, E. M., Scott, S. J., Morey, C. C., Mattox, S., Hismjatullina, A., et al. (2005). On the capacity of attention: its estimation and its role in working memory and cognitive aptitudes. Cogn. Psychol. 51, 42–100. doi: 10.1016/j.cogpsych.2004.12.001
Cowan, N., Fristoe, N. M., Elliot, E. M., Saults, J. S., Morey, C. C., Mattox, S., et al. (2006). Scope of attention, control of attention, and intelligence in children and adults. Mem. Cognit. 34, 1754–1768. doi: 10.3758/bf03195936
Coyle, T. R. (2003). A review of the worst performance rule: Evidence, theory, and alternative hypotheses. Intelligence 31, 567–587. doi: 10.1016/s0160-2896(03)00054-0
Crawford, D. W., Bendrath, S., Manzano, M. D., Mehta, A., Patel, H. M., Piela, M., et al. (2020). Intelligence demands flexibility: individual differences in attentional disengagement strongly predict the general cognitive ability of mice. Learn. Motiv. 71:101657 doi: 10.1016/j.lmot.2020.101657
Damerius, L. A., Burkart, J. M., van Noordwijk, M. A., Haun, D. B. M., Kosonen, Z. K., Galdikas, B. M. F., et al. (2019). General cognitive abilities in orangutans (Pongo abelii and Pongo pygmaeus). Intelligence 74, 3–11. doi: 10.1016/j.intell.2018.10.008
Deacon, R. M. J (2006a). Assessing hoarding in mice. Nat. Protoc. 1, 2828–2830. doi: 10.1038/nprot.2006.171
Deacon, R. M. J. (2006b). Assessing nest building in mice. Nat. Protoc. 1, 1117–1119. doi: 10.1038/nprot.2006.170
Dhont, K., and Hodson, G. (2014). Does lower cognitive ability predict greater prejudice? Curr. Direct. Psychol. Sci. 23, 454–459. doi: 10.1177/0963721414549750
Eisenreich, B. R., and Hayden, B. Y. (2018). Cognitive science: persistent apes are intelligent apes. Curr. Biol. 28, R160–R162.
Engle, R. W. (2002). Working memory capacity as executive attention. Curr. Direct. Psychol. Sci. 11, 19–23. doi: 10.1111/1467-8721.00160
Engle, R. W. (2018). Working memory and executive attention: a revisit. Perspect. Psychol. Sci. 13, 190–193. doi: 10.1177/1745691617720478
Fitchett, A. E., Collins, S. A., Barnard, C. J., and Cassaday, H. J. (2005). Subordinate male mice show long-lasting differences in spatial learning that persist when housed alone. Neurobiol. Learn. Mem. 84, 247–251. doi: 10.1016/j.nlm.2005.08.004
Gale, C. R., Hatch, S. L., Batty, G. D., and Deary, I. J. (2009). Intelligence in childhood and risk of psychological distress in adulthood: the 1958 National Child Development Survey and the 1970 British Cohort Study. Intelligence 37, 592–599. doi: 10.1016/j.intell.2008.09.002
Galsworthy, M. J., Paya-Cano, J. L., Liu, L., Monleon, S., Gregoryan, G., Fernandes, C., et al. (2005). Assessing reliability, heritability and general cognitive ability in a battery of cognitive tasks for laboratory mice. Behav. Genet. 35, 675–692. doi: 10.1007/s10519-005-3423-9
Galsworthy, M. J., Paya-Cano, J. L., Monleón, S., and Plomin, R. (2002). Evidence for general cognitive ability (g) in heterogeneous stock mice and an analysis of potential confounds. Genes Brain Behav. 1, 88–95. doi: 10.1034/j.1601-183x.2002.10204.x
Gottfredson, L. S. (2003). “Jobs, and life,” in The Scientific Study of General Intelligence: Tribute to Arthur R. Jensen, ed. H. Nyborg (Pergamon: Oxford).573–597
Grossman, H., Hale, G., Light, K., Kolata, S., and Matzel, L. D. (2007). Pharmacological modulation of stress reactivity dissociates its role in the determination of the relationship of exploration and general cognitive abilitiies. Behav. Neurosci. 121, 949–964. doi: 10.1037/0735-7044.121.5.949
Herndon, J. G., Moss, M. B., Rosene, D. L., and Killiany, R. J. (1997). Patterns of cognitive decline in aged rhesus monkeys. Behav. Brain Res. 87, 25–34. doi: 10.1016/s0166-4328(96)02256-5
Herrmann, E., Hare, B., Call, J., and Tomasello, M. (2010). Differences in the cognitive skills of bonobos and chimpanzees. PLoS One 5:e12438. doi: 10.1371/journal.pone.0012438
Isden, J., Panayi, C., Dingle, C., and Madden, J. (2013). Performance in cognitive and problem-solving tasks in male spotted bowerbirds does not correlate with mating success. Animal Behaviour, 86, 829–838. doi: 10.1016/j.anbehav.2013.07.024
Juhel, J. (1993). Should we take the shape of reaction time distributions into account when studying the relationship between RT and psychometric intelligence? Personal. Indiv. Differ. 15, 357–360. doi: 10.1016/0191-8869(93)90231-q
Kolata, S., Light, K., Grossman, H. C., Hale, G., and Matzel, L. D. (2007). Selective attention is a primary determinant of the relationship between working memory and general learning ability in outbred mice. Learn. Mem. 14, 22–28. doi: 10.1101/lm.408507
Kolata, S., Light, K., and Matzel, L. D. (2008). Domain-specific and domain-general learning factors are expressed in genetically heterogeneous CD-1 mice. Intelligence 36, 619–629. doi: 10.1016/j.intell.2007.12.001
Kolata, S., Light, K., Townsend, D. A., Hale, G., Grossman, H., and Matzel, L. D. (2005). Variations in working memory capacity predict individual differences in general learning abilities among genetically diverse mice. Neurobiol. Learn. Mem. 84, 242–246.
Leon, D. A., Lawlor, D. A., Clark, H., Batty, G. D., and Macintyre, S. (2009). The association of childhood intelligence with mortality risk from adolescence to middle age: findings from the Aberdeen Children of the 1950s cohort study. Intelligence 37, 520–528. doi: 10.1016/j.intell.2008.11.004
Light, K., Kolata, S., Townsend, D. A., Grossman, H., Hale, G., and Matzel, L. D. (2008). Up-regulation of exploratory tendencies does not enhance general learning abilities in juvenile or young-adult outbred mice. Neurobiol. Learn. Mem. 90, 317–329. doi: 10.1016/j.nlm.2008.04.007
Light, K., Kolata, S., Wass, C., Denman-Brice, A., Zagalsky, R., and Matzel, L. D. (2010). Working memory training promotes general cogntive abilities in genetically heterogeneous mice. Curr. Biol. 20, 777–782 doi: 10.1016/j.cub.2010.02.034
Light, K. R., Grossman, Y., Kolata, S., Wass, C. D., and Matzel, L. D. (2011). General learning ability regulates exploration through its influence on rate of habituation. Behav. Brain Res. 223, 297–309. doi: 10.1016/j.bbr.2011.04.050
Locurto, C. (2017). G and g: two markers of a general cognitive ability, or none? Behav. Brain. Sci. 40:e211.
Locurto, C., and Scanlon, C. (1998). Individual differences and a spatial learning factor in two strains of mice. J. Comp. Psychol. 112:352.
MacKinlay, R. D., and Shaw, R. C. (2019). Male New Zealand robin (Petroica longipes) song repertoire size does not correlate with cognitive performance in the wild. Intelligence 74, 25–33. doi: 10.1016/j.intell.2018.10.009
Madden, J. R., Langley, E. J. G., Whiteside, M. A., Beardsworth, C. E., and Horik, J. O. v (2018). The quick are the dead: pheasants that are slow to reverse a learned association survive for longer in the wild. Philos. Trans. R. Soc. B Biol. Sci. 373:20170297. doi: 10.1098/rstb.2017.0297
Maille, A., and Schradin, C. (2016). Survival is linked with reaction time and spatial memory in African striped mice. Biology Letters, 12:20160346. doi: 10.1098/rsbl.2016.0346
Matzel, L. D., Bendrath, S., Herzfeld, M., Crawford, D. W., and Sauce, B. (2019). Mouse twins separated when young: a history of exploration doubles the heritability of boldness and differentially affects the heritability of measures of learning. Intelligence 74, 34–42. doi: 10.1016/j.intell.2019.01.005
Matzel, L. D., Grossman, H., Light, K., Townsend, D. A., and Kolata, S. (2008). Variations in age-related declines in general cogntiive abilities of Balb/C mice are associated with disparities in working memory span/capacity and body weight. Learn. Mem. 15, 733–746. doi: 10.1101/lm.954808
Matzel, L. D., Han, Y. R., Grossman, H., Karnik, M. S., Patel, D., Scott, N., et al. (2003). Individual differences in the expression of a “general” learning ability in mice. J. Neurosci. 23, 6423–6433.
Matzel, L. D., and Kolata, S. (2010). Selective attention, working memory, and animal intelligence. Neurosci. Biobehav. Rev. 34, 23–30. doi: 10.1016/j.neubiorev.2009.07.002
Matzel, L. D., Kolata, S., Light, K., and Sauce, B. (2017). The tendancy for social submission predicts cognitive performance in previously isolate male mice. Behav. Proces. 134, 12–21. doi: 10.1016/j.beproc.2016.07.011
Matzel, L. D., and Sauce, B. (2017). Individual differences: case studies of rodent and primate intelligence. J. Exp. Psychol. Anim. Learn. Cogn. 43, 325–340. doi: 10.1037/xan0000152
Matzel, L. D., Townsend, D. A., Grossman, H., Han, Y. R., Hale, G., Zappulla, M., et al. (2006). Exploration in outbred mice covaries with general learning abilities irrespective of stress reactivity, emotionality, and physical attributes. Neurobio. Learn. Mem. 86, 228–240. doi: 10.1016/j.nlm.2006.03.004
Orr, H. A. (2009). Fitness and its role in evolutionary genetics. Nature Reviews Genetics, 10, 531–539. doi: 10.1038/nrg2603
Papes, F., Logan, D. W., and Stowers, L. (2010). The vomeronasal organ mediates interspecies defensive behaviors through detection of protein pheromone homologs. Cell 141, 692–703. doi: 10.1016/j.cell.2010.03.037
Richards, M., Black, S., Mishra, G., Gale, C. R., Deary, I. J., and Batty, D. G. (2009). IQ in childhood and the metabolic syndrome in middle age: Extended follow-up of the 1946 British Birth Cohort Study. Intelligence 37, 567–572. doi: 10.1016/j.intell.2008.09.004
Sauce, B., Wass, C., Smith, A., Kwan, S., and Matzel, L. D. (2014). The external-internal loop of interference: two types of attention and their influence on the learning abilities of mice. Neurobiol. Learn Mem. 116, 181–192. doi: 10.1016/j.nlm.2014.10.005
Sauce, B., Bendrath, S., Herzfeld, M., Siegel, D., Style, C., Rab, S., et al. (2018). The impact of environmental interventions among mouse siblings on the heritability and malleability of general cognitive ability. Philos. Trans. R. Soc. Lond. B Biol. Sci. 373:20170289. doi: 10.1098/rstb.2017.0289
Schmidt, F. L., and Hunter, J. E. (1998). The validity and utility of selection methods in personnel psychology: Practical and theoretical implications of 85 years of research findings. Psychol. Bull. 124, 262–274. doi: 10.1037/0033-2909.124.2.262
Shaw, R. C., Boogert, N. J., Clayton, N. S., and Burns, K. C. (2015). Wild psychometrics: evidence for ‘general’ cognitive performance in wild New Zealand robins. Petroica longipes. Anim. Behav. 109, 101–111. doi: 10.1016/j.anbehav.2015.08.001
Shaw, R. C., MacKinlay, R. D., Clayton, N. S., and Burns, K. C. (2019). Memory performance influences male reproductive success in a wild bird. Curr. Biol. 29:2773. doi: 10.1016/j.cub.2019.07.049
Shipstead, Z., and Engle, R. W. (2013). Interference within the focus of attention: working memory tasks reflect more than temporary maintenance. J. Exp. Psychol. Learn. Mem. Cogn. 39, 277–289. doi: 10.1037/a0028467
Sonnenberg, B. R., Branch, C. L., Pitera, A. M., Bridge, E., and Pravosudov, V. V. (2019). Natural selection and spatial cognition in wild food-caching mountain chickadees. Curr. Biol. 29, 670–676.e. doi: 10.1016/j.cub.2019.01.006
Sorato, E., Zidar, J., Garnham, L., Wilson, A., and Lovlie, H. (2018). Heritabilities and co-variation among cognitive traits in red junglefowl. Philos. Trans. R. Soc. Lond. B Biol. Sci. 373:20170285. doi: 10.1098/rstb.2017.0285
Thornton, A., Isden, J., and Madden, J. R. (2014). Toward wild psychometrics: linking individual cognitive differences to fitness. Behav. Ecol. 25, 1299–1301. doi: 10.1093/beheco/aru095
Voiker, V., Polus, P., Vasar, E., and Rauvala, H. (2005). Long-term individual housing in C57BL/6J and DBA/2 mice: assessment of behavioral consequences. Genes Brain Behav. 4, 240–252. doi: 10.1111/j.1601-183x.2004.00106.x
Wass, C., Denman-Brice, A., Rios, C., Light, K. R., Kolata, S., Smith, A. M., et al. (2012). Covariation of learning and “reasoning” abilities in mice: evolutionary conservation of the operations of intelligence. J. Exp. Psychol. Anim. Behav. Proces. 38, 109–124. doi: 10.1037/a0027355
Keywords: intelligence, survival, fitness, nest building, hoarding, foraging, mice
Citation: Matzel LD, Patel HM, Piela MC, Manzano MD, Tu A and Crawford DW (2020) General Cognitive Ability Predicts Survival-Readiness in Genetically Heterogeneous Laboratory Mice. Front. Ecol. Evol. 8:531014. doi: 10.3389/fevo.2020.531014
Received: 30 January 2020; Accepted: 22 September 2020;
Published: 05 November 2020.
Edited by:
Laure Cauchard, University of Aberdeen, United KingdomReviewed by:
Rachael Caroline Shaw, Victoria University of Wellington, New ZealandKenneth R. Light, Columbia University, United States
Copyright © 2020 Matzel, Patel, Piela, Manzano, Tu and Crawford. This is an open-access article distributed under the terms of the Creative Commons Attribution License (CC BY). The use, distribution or reproduction in other forums is permitted, provided the original author(s) and the copyright owner(s) are credited and that the original publication in this journal is cited, in accordance with accepted academic practice. No use, distribution or reproduction is permitted which does not comply with these terms.
*Correspondence: Louis D. Matzel, bWF0emVsQHBzeWNoLnJ1dGdlcnMuZWR1