- 1Co-Innovation Center for Sustainable Forestry in Southern China, Nanjing Forestry University, Nanjing, China
- 2Jiangsu Province Key Laboratory of Soil and Water Conservation and Ecological Restoration, Nanjing Forestry University, Nanjing, China
To protect endangered species and restore their habitats, it is important to be able to predict their potential geographic distributions. The Dendrobium plant is important in traditional Chinese medicine, but urban expansion and over-exploitation have led to a decrease of Dendrobium resources. To achieve sustainable development of Dendrobium resources, the spatial and temporal distribution of two Dendrobium species were systematically analyzed based on the distribution points of D. moniliforme (104) and D. nobile (87). Maximum entropy modeling (MaxEnt) was used to predict the distribution of suitable habitats for these Dendrobium species, both currently and in the future, under different representative concentration pathways (RCPs). Three RCPs included RCP2.6, RCP4.5, and RCP8.5. The results show that D. moniliforme and D. nobile are widely distributed in the south of China (Anhui, Guangdong, Jiangxi, and Hunan provinces). Worldwide, Japan and North Korea were identified as major distribution areas for D. moniliforme and D. nobile. Based on the MaxEnt model, the mean diurnal range and the minimum temperature of the coldest month were identified as the most significant bioclimatic variables controlling the distribution of D. moniliforme and D. nobile. Future climate change will likely result in an increase of suitable habitat areas for D. moniliforme (by around 16%), and a decrease for D. nobile (by around 1–10%), but climate change is unlikely to have much impact on the distribution of suitable habitats for D. moniliforme and D. nobile in Japan and North Korea. Based on our findings, measures should be taken to protect these precious medicinal plant resources, and the sites used for the artificial cultivation of Dendrobium will need to shift as the climate changes.
Introduction
Climate is the most important factor determining the distribution of species on Earth, and changes in species distributions reflect changes in climate (Tanaka et al., 2012; Faleiro et al., 2013; Li et al., 2013). There have been many studies addressing the relationship between climate change and the distributions of the herbs used in traditional Chinese medicine (Szakiel et al., 2011; Li et al., 2015; Guo et al., 2016). Dendrobium is the second-largest Orchid genus (∼1,500 species). Although Dendrobium species are native to China, Japan, the Philippines, Thailand, and other countries, they are mainly distributed in the tropical and subtropical regions of Asia and Oceania; there are only 80 species and two varieties of Dendrobium in China (Wu et al., 2009; Zheng et al., 2018). The fresh or dried stems of many Dendrobium species are one of the most expensive tonics in traditional Chinese medicine, and these tonics are considered a “superior grade” herbal medicine in the ancient text “Shen Nong’s Herbal Classic” (Xu et al., 2013). The main chemical components of Dendrobium are alkaloids, aromatic compounds, sesquiterpenoids, and polysaccharides, with multiple biological activities, including immunomodulatory, neuroprotective, and anti-tumor effects, with high research and development value (Xu et al., 2013). The world’s medicinal Dendrobium is almost exclusively sourced from the wild. A huge and persisting market demand has led to the overharvesting of wild Dendrobium, which is now endangered. Also, indiscriminate collection is destroying the natural environment that Dendrobium depends on.
The climatic conditions of Dendrobium are relatively special, and the Dendrobium species found differ among regions. Generally, the average annual temperature in the distribution area is 8.5–21.7°C, the annual extreme maximum temperature is up to 35.7°C, the annual extreme minimum temperature is −1.6°C, the optimal temperature in the growing period is 20–30°C, and the average temperature in winter is 1–12.2°C (Baker and Baker, 1996; Xiang et al., 2016). Although Dendrobium has some resistance to adverse temperatures, the survival of Dendrobium is expected to be affected by changing climatic conditions. Therefore, it is important to analyze and evaluate the likely migration and change of Dendrobium growing areas under climate change. Determining the specific distribution points and population density of species through field sampling information and database sample information is the most important tool we have for determining species distribution and habitat. However, it is difficult to objectively measure species distributions and to make reasonable predictions about future species distributions and habitat conditions.
In recent years, species distribution models based on geographic information systems and ecological principles have been widely used in efforts to predict the potential distribution of species. Maximum entropy (MaxEnt), genetic algorithm for rule-set production (GARP), artificial neural network (ANN), species distribution models (SDM), and generalized additive model (GAM) approaches have been used to study the distribution of wild plants under current and future climatic conditions (Raimundo et al., 2007; Haverkost et al., 2010; Tanaka et al., 2012; De Souza and De Marco, 2014; Motloung et al., 2014). MaxEnt is a habitat suitability model based on a niche principle, where species distribution data and environmental data are used to analyze the distribution of species at maximum entropy (Remya et al., 2015). Compared with other niche models, MaxEnt is most robust when relatively few distribution points are available, and because of this, MaxEnt has been widely used by scholars throughout the world (Elith et al., 2006; Phillips et al., 2006; Petitpierre et al., 2012; Zhang M.G. et al., 2016).
Many scholars have studied the relationship between environmental factors (climate and soil) and the potential distribution of Dendrobium plants (Khanum et al., 2013; Yang et al., 2013). However, most current Dendrobium research is focused on artificial cultivation, genomics, pharmacology, clinical application, and extraction of chemical components (Teixeira da Silva et al., 2016; Cheng et al., 2019; Yuan et al., 2020). There have been few attempts to describe the distribution of suitable Dendrobium habitats, or to predict how future climate is likely to affect this. To address this, here we describe the distribution of two representative Dendrobium species (D. moniliforme and D. nobile) and predict potentially suitable habitats for these species, for both current and under future climate change scenarios. This will provide a theoretical basis for the protection and reasonable introduction of Dendrobium resources under current climate conditions. Meanwhile, scientific measures should be taken to protect Dendrobium according to the influence of climate factors on the distribution of Dendrobium in the future.
Materials and Methods
Study Species and Occurrence Data
D. nobile was listed in the Pharmacopia of the People’s Republic of China and D. moniliforme has a high medicinal value. These two species were planted more widely in the world and have greater potential for promotion and cultivation. So, we selected these two representative species to study the current and future distributions of the Dendrobium species. The current distribution of suitable habitats for D. moniliforme and D. nobile is shown in Figure 1. The distribution point records of two different Dendrobium species were derived from the Global Biodiversity Information Network Database1 (GBIF), China Digital Herbarium2 (CVH), China’s eFlora website3, and the China National Knowledge Infrastructure4.
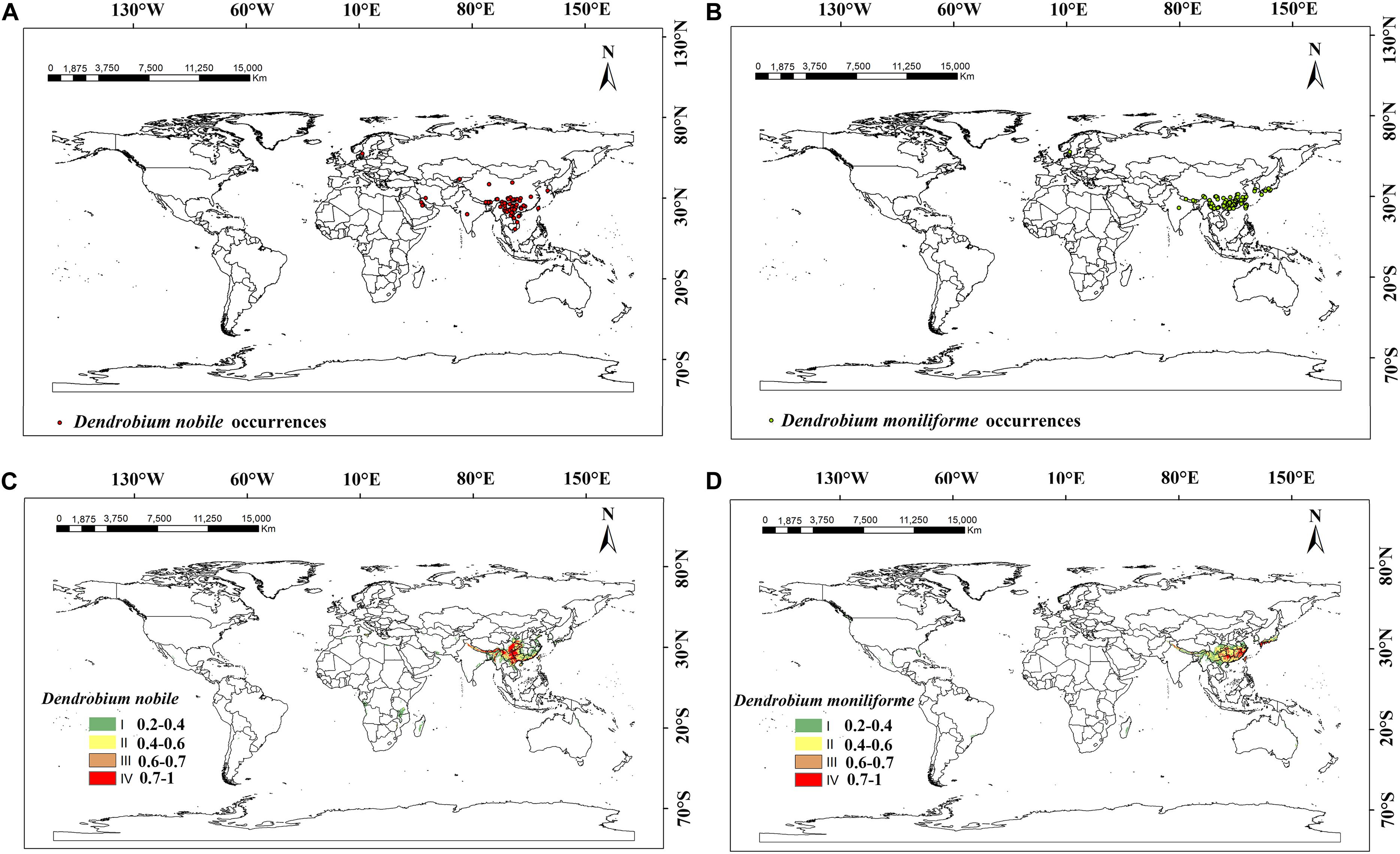
Figure 1. Global sample occurrence records and suitable habitat areas of Dendrobium nobile and Dendrobium moniliforme at present. (A) Dendrobium nobile occurrences; (B) Dendrobium moniliforme occurrences; (C) suitable habitat areas of Dendrobium nobile; (D) suitable habitat areas of Dendrobium moniliforme.
To ensure the accuracy of the Dendrobium distribution points, the obtained distribution point records were strictly screened. First, we selected the Latin name and accurate latitude and longitude points, eliminating any Latin name errors, and incomplete or repeated latitude and longitude points. Next, for the points with exact place names but no longitude and latitude, this data was gathered using Google Earth5 7.0. Ultimately, we gathered 104 distribution points for D. moniliforme and 87 distribution points for D. nobile. The distribution points data of Dendrobium were stored in a.csv format for later use, sorted by species name, longitude, and latitude. ArcGIS 10.5 (Esri, Redlands, CA, United States) and.csv files of the two Dendrobium distribution points data were used to map the distribution points of the two Dendrobium species. The time span of distribution points in the database was 1930–2018, mainly distributed at 25–50°N and 80–150°E. Considering possible climate change within the early distribution points, we removed the distribution points with earlier recorded times. From the distribution range of the occurrence data, China and Japan have a more concentrated set of distribution points, while India, Iran, and Saudi Arabia also have small numbers of distribution points.
Predictor Environmental Variables
Nineteen bioclimatic variables (bio01–bio19) of current times and the future (the 2050s and 2070s) were downloaded from the Global Climate Databases6 with 5-min spatial resolution (Table 1). Biological variables came from three different representative concentration pathways (RCPs), including RCP2.6, RCP4.5, and RCP8.5, which represented low, moderate, and high greenhouse gas emission scenarios, respectively. The elevation data (ALT) of topographic variables extracted using ArcGIS 10.5 (Esri, Redlands, CA, United States) were derived from the National Geophysical Data Center7. As the coordinate system, we used WGS84, which includes indicators such as annual average, seasonal average and monthly average temperature/humidity, annual average sunshine, and annual average precipitation. CCSM4 is an efficient global climate tool for the simulation of future climatic conditions, which has been thoroughly evaluated in China and successfully applied to predict the influence of future climatic changes on the distribution of plant species in similar environments (Vertenstein et al., 2010; Meehl et al., 2012, 2013; Li et al., 2020; Yan et al., 2020). Four RCPs were established, ranging from RCP 2.6 (aggressive mitigation/lowest emission scenario) to RCP 8.5 (highest emission scenario) in the fifth report of the Intergovernmental Panel on Climate Change (IPCC), and the RCP2.6, RCP 4.5, and RCP 8.5 scenarios were selected to model the prospective potential distribution of Dendrobium under a maximum emissions scenario hypothesis (Li et al., 2020).
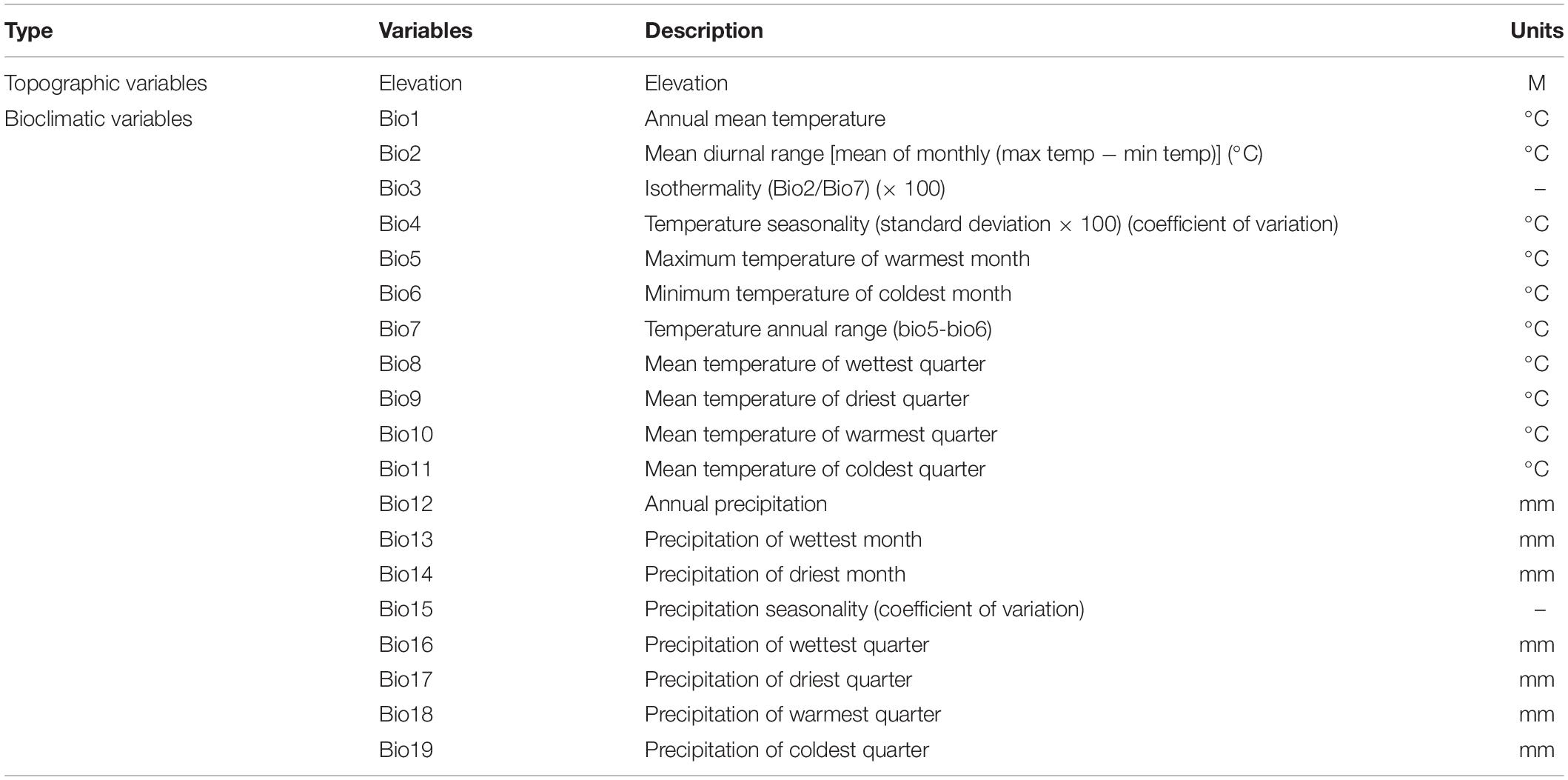
Table 1. Selected environmental variables in the MaxEnt model and their units in predicting the current and future distribution of Dendrobium nobile and Dendrobium moniliforme.
The prediction result of the MaxEnt model will be over-fitted due to the collinearity between various ecological factors (Graham, 2003). To exclude the over-fitting of model prediction results, the Spearman coefficient method is used to detect the correlation of 20 ecological factors (Figure 2). If the correlation coefficient of the two factors is greater than or equal to 0.8, the factors with a contribution rate are retained, and factors with a correlation coefficient of less than 0.8 are retained (Zhang H. et al., 2016). Based on the analysis of the MaxEnt model and the Spearman coefficient method, this study ultimately selected eight and ten environmental factors for D. moniliforme and D. nobile, respectively, and these data were used to map the potential distributions of Dendrobium and identify the key variables. The digital elevation model was resampled using ArcGIS 10.5 (Esri, Redlands, CA, United States) to make the spatial resolution the same as the 19 bioclimatic variables. All variables were converted into the ASCII format via ArcGIS 10.5 (Esri, Redlands, CA, United States) for use with the MaxEnt software.
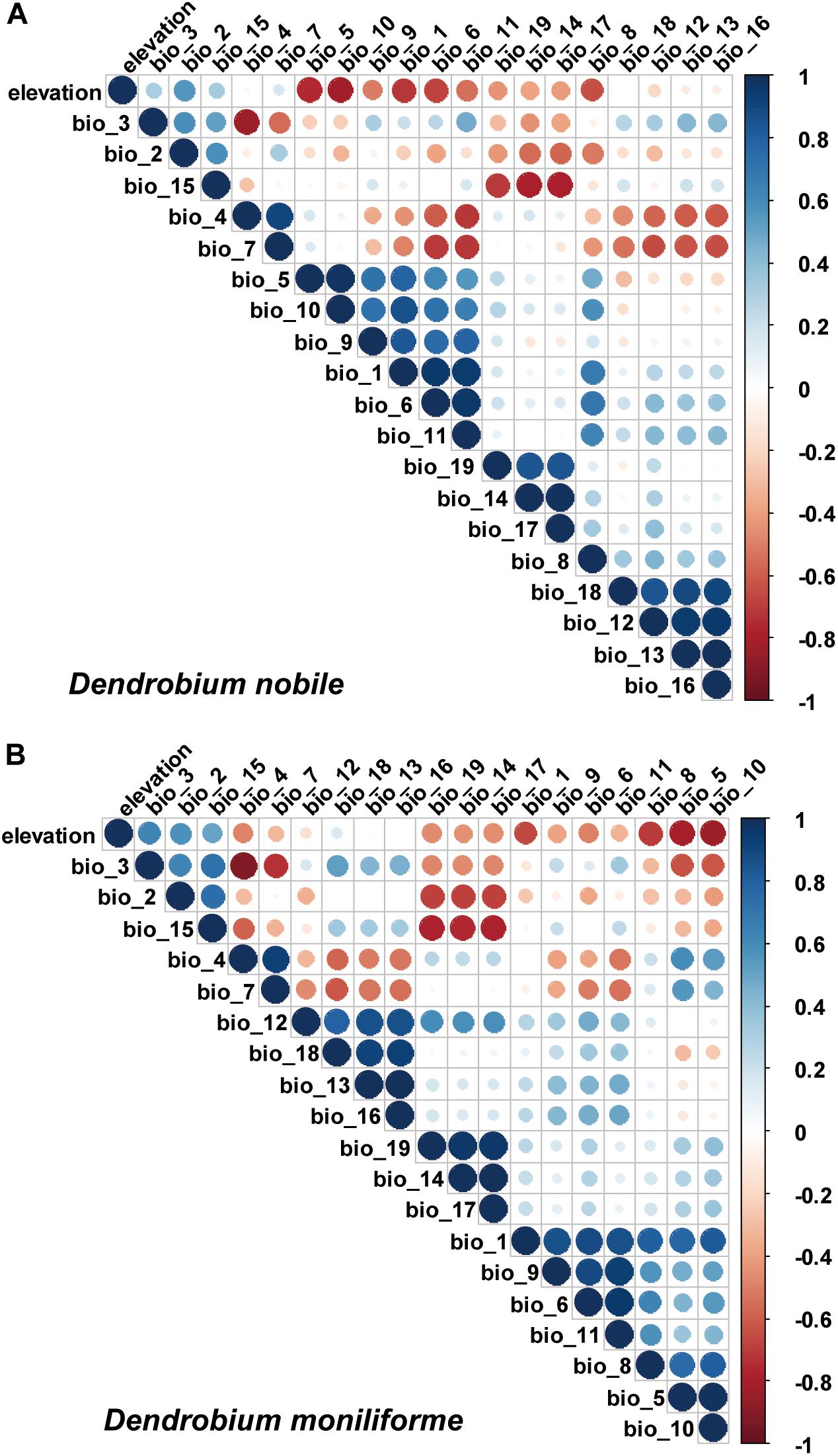
Figure 2. Pearson correlation coefficients of important environmental variables using a multicollinearity test. (A) Dendrobium nobile; (B) Dendrobium moniliforme.
Maximum Information Entropy Modeling Analysis
At present, the MaxEnt model has successfully predicted the potential distribution areas of various plants and achieved good results based on the sampling point information of the species distribution and environmental variables (Haverkost et al., 2010; De Souza and De Marco, 2014; Motloung et al., 2014; Zhang et al., 2018). So, in this study, we intend to use the MaxEnt model for analysis. After analyzing the contribution of environmental factors to the growth and distribution of Dendrobium with the MaxEnt 3.4.1 software, a MaxEnt prediction model based on the actual distribution records and ecological variables was established. The overall probability of Dendrobium occurrence was calculated, and its suitable habitat area was predicted. The potentially suitable habitats of the two Dendrobium species at current and future periods in the world were obtained using ArcGIS 10.5 (Esri, Redlands, CA, United States). The specific operation method is as follows: first, the selected ecological variables and the occurrence data of D. moniliforme and D. nobile were respectively optimized by R, the performance of different models was evaluated to obtain the best-fitting model using the R package “ENMeval” and the package “dismo” was used to establish a model of species distribution (Hijmans et al., 2013; Muscarella et al., 2014). The features and regularization multiplier for the MaxEnt model were obtained by optimizing relevant data through R. Next, the ecological variables and the occurrence data were loaded into the MaxEnt model; 75% of the location data were used for training, and the remaining 25% were used to test the predictive ability of the model. The model ran either 500 iterations of these processes or continued until a convergence threshold of 0.00001 was met. The logistic output was chosen as an estimate of the probability of the presence conditioned by the ecological variables, per grid cell. The features and regularization multiplier were set according to the optimization result of R; other parameters were kept as the default.
Modeling Evaluation
The accuracy of the prediction of the MaxEnt model under different conditions needs to be quantified by calculating the area under the receiver operating characteristic (ROC) curve (AUC) (Lobo et al., 2008). AUC values below 0.7 were considered as poor; values between 0.7 and 0.9 were moderate, and >0.9 were considered as high (Franklin, 2010). The Jackknife method is an analytical method to evaluate the reliability of the model, and the ROC curve of the knife-cut method is a commonly used model in the evaluation of the potential distribution model of species (Dorfman et al., 1992; Townsend Peterson et al., 2007). A larger value of the AUC indicates a more accurate prediction. These values are typically between 0.8 and 0.9, and values between 0.9 to 1.0 are considered very good (Swets, 1988). There are many ways to divide suitable habitat areas when studying the relationship between the environments of plant-suitable habitat areas. To do this, we synthesized the existence probability of Dendrobium and used an artificial classification method with a good division effect to divide the suitable habitat area of Dendrobium into four levels: high habitat suitability (existence probability of 0.7–1), moderate-high habitat suitability (existence probability of 0.6–0.7), moderate habitat suitability (existence probability of 0.4–0.6), and low habitat suitability (existence probability of 0.2–0.4) (Yang et al., 2013). In the climate factor response map generated by the knife-cut method, the resulting values with a probability of existence greater than 0.5 were taken as the most suitable ecological factor range for the species in this study (Hemery et al., 2016).
Results
Model Performance for Dendrobium Species
At present, ROC analysis is recognized as the best evaluation index for diagnostic tests and the area under the ROC curve (AUC value) is used to measure the accuracy of model prediction (Wang et al., 2007; Peterson et al., 2008; Yuan et al., 2015). The larger the AUC value, the larger the true positive rate and the smaller the false positive rate, which means that the prediction result of the model is more accurate. When the AUC value is greater than 0.8, the accuracy of the model prediction is high. When the AUC value is greater than 0.9, the model prediction area matches the actual species distribution and has excellent performance (Howell et al., 2011; Pourghasemi et al., 2017).
The AUC results of the MaxEnt model are shown in Figure 3. In D. nobile, the AUC value of the training set reached 0.976, and the AUC value of the test set reached 0.989 in the current situation. In D. moniliforme, the AUC values of both the training and testing sets reached 0.991 in the current situation. In the future (the 2050s and 2070s), under different greenhouse gas emission models, the AUC values of training and testing of the D. nobile and D. moniliforme sets both exceeded 0.975. These results show that the predicted distribution area in the current period was highly consistent with the natural distribution area, and the MaxEnt model has high credibility and accuracy in calculating suitable habitat areas for Dendrobium for current and various future periods.
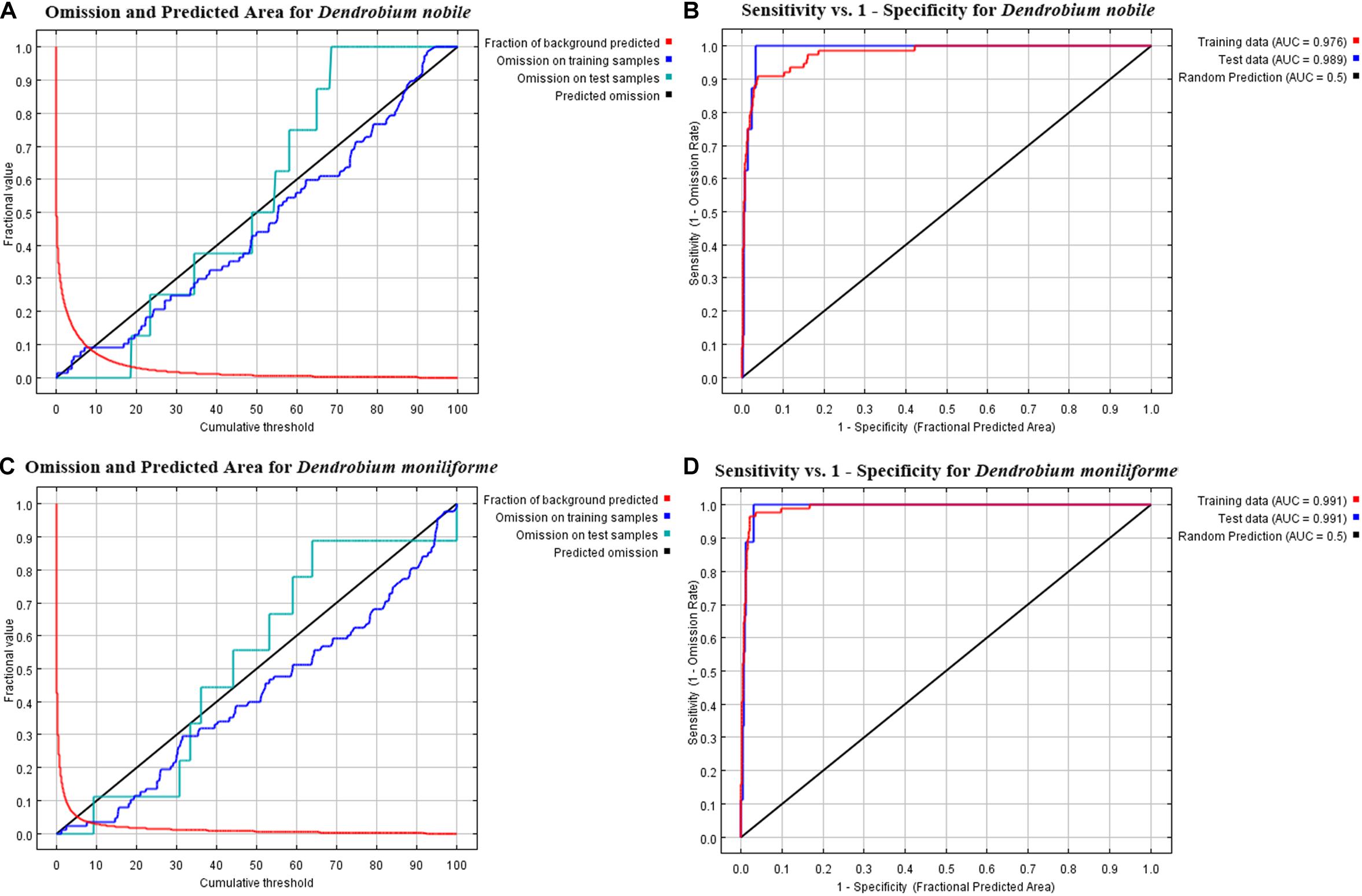
Figure 3. Omission and predicted area curves for two Dendrobium species. (A,B) ROC for the training and test data with the area under the AUC values. The red line represents the fraction of background predicted, the blue line represents the omission on training samples, the indigo line represents the omission on test samples, and the black line indicates the predicted omission. (A,B) Dendrobium nobile; (C,D) Dendrobium moniliforme.
Environments That Constrain the Model
The MaxEnt model obtains the contribution rate of each ecological factor through iterative calculation and normalization. It essentially provides a measure of the relative importance of each ecological variable, and the greater the contribution rate, the greater the impact on plant growth and distribution. The Jackknife test can analyze each environmental variable with a knife-cut method, and can distinguish that different variables have different effects on the potential distribution area of Dendrobium plants (Figure 4). The relative importance of the various ecological variables showed that the mean diurnal range (bio2), temperature seasonality (bio4), minimum temperature of the coldest month (bio6), annual precipitation (bio12), precipitation of the driest month (bio14), precipitation seasonality (bio15), precipitation of the warmest quarter (bio18), and elevation were important factors in predicting the geographic distribution for D. moniliforme, and that the cumulative contribution rate of temperature seasonality (bio4), minimum temperature of the coldest month (bio6), and precipitation of the warmest quarter (bio18) reached 77.3% (Table 2).
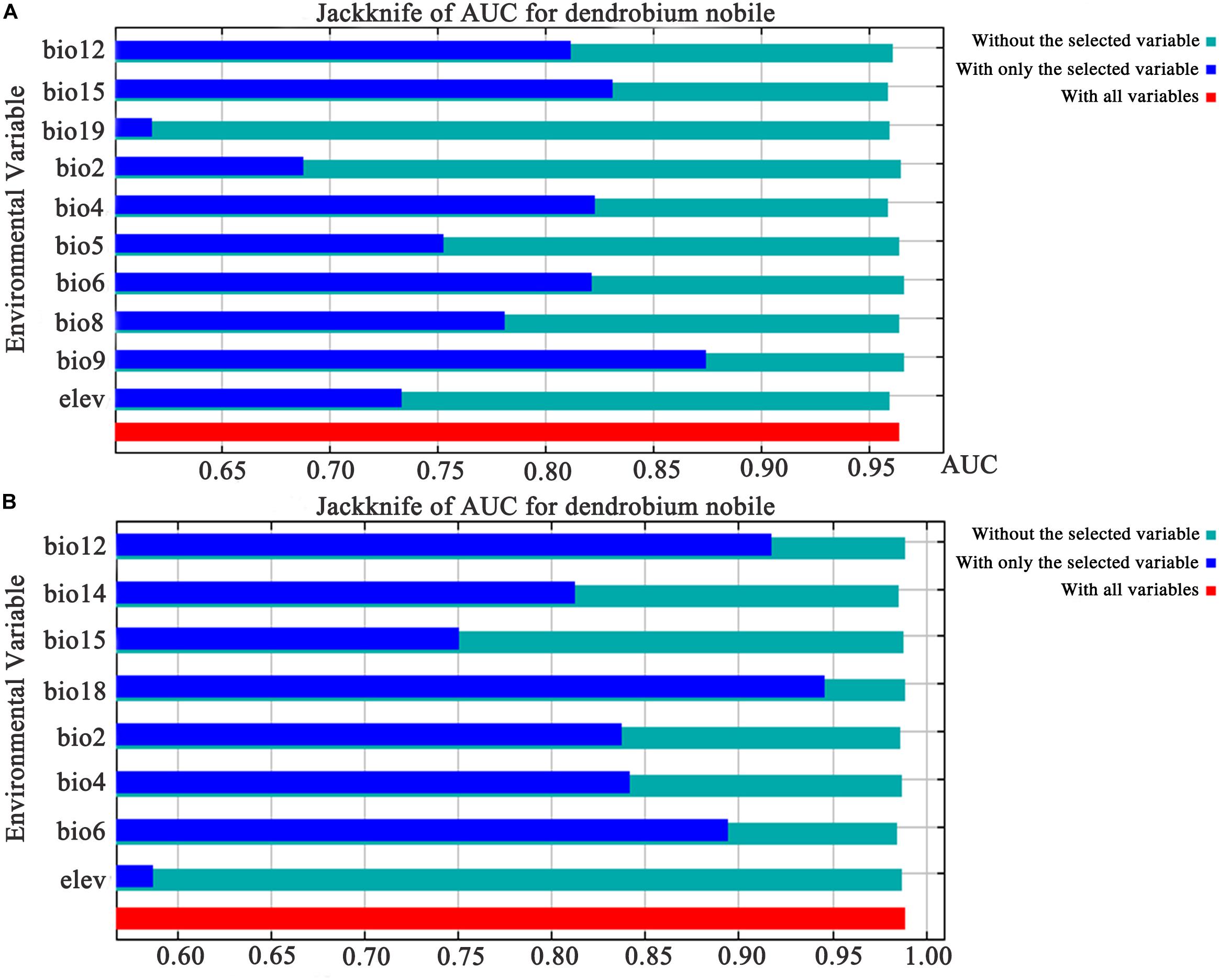
Figure 4. The results of the Jackknife procedure on AUC for Dendrobium worldwide. (A) Dendrobium nobile. (B) Dendrobium moniliforme.
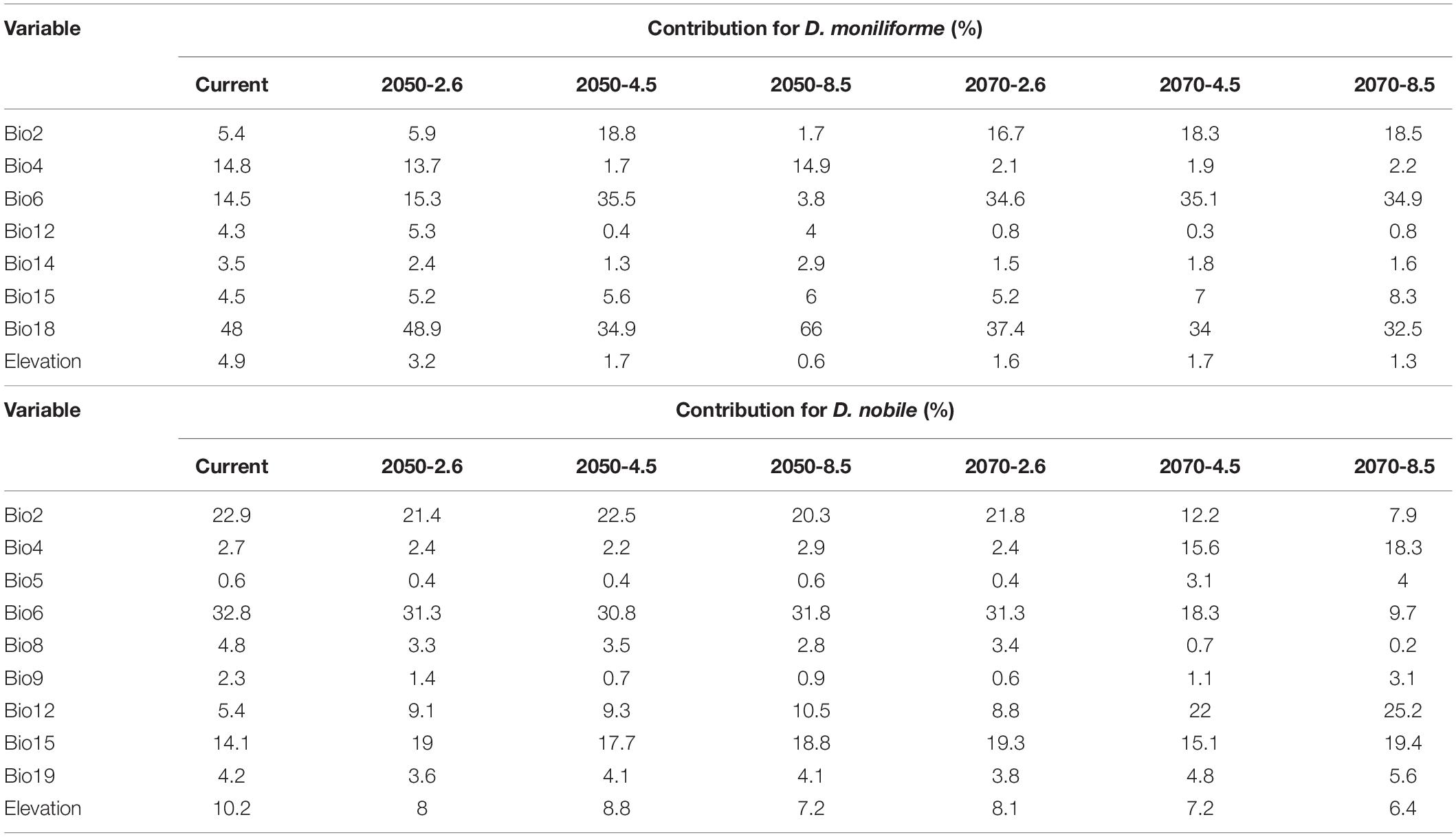
Table 2. Relative contribution of selected environmental variables in the MaxEnt model for two Dendrobium species.
Similarly, among the ten ecological variables that are highly correlated with the geographical distribution of D. nobile, the cumulative contribution rate of the mean diurnal range (bio2), minimum temperature of the coldest month (bio6), and precipitation seasonality (bio15) reached 69.8% (Table 2). As shown in Figures 5 and 6, the response curves of the distribution model of D. nobile and D. moniliforme indicated a high probability of occurrence (probability of presence >0.6) of this species in these regions. In the current period, D. moniliforme and D. nobile are both greatly affected by the minimum temperature of the coldest month (bio6), and its effect on D. nobile is greater than other ecological factors, which indicates that the two Dendrobium species have greater sensitivity to low temperatures. The difference is that the largest ecological factor affecting D. moniliforme is the precipitation of the warmest quarter (bio18), which indicates that the two Dendrobium species of the same genus have different environmental requirements.
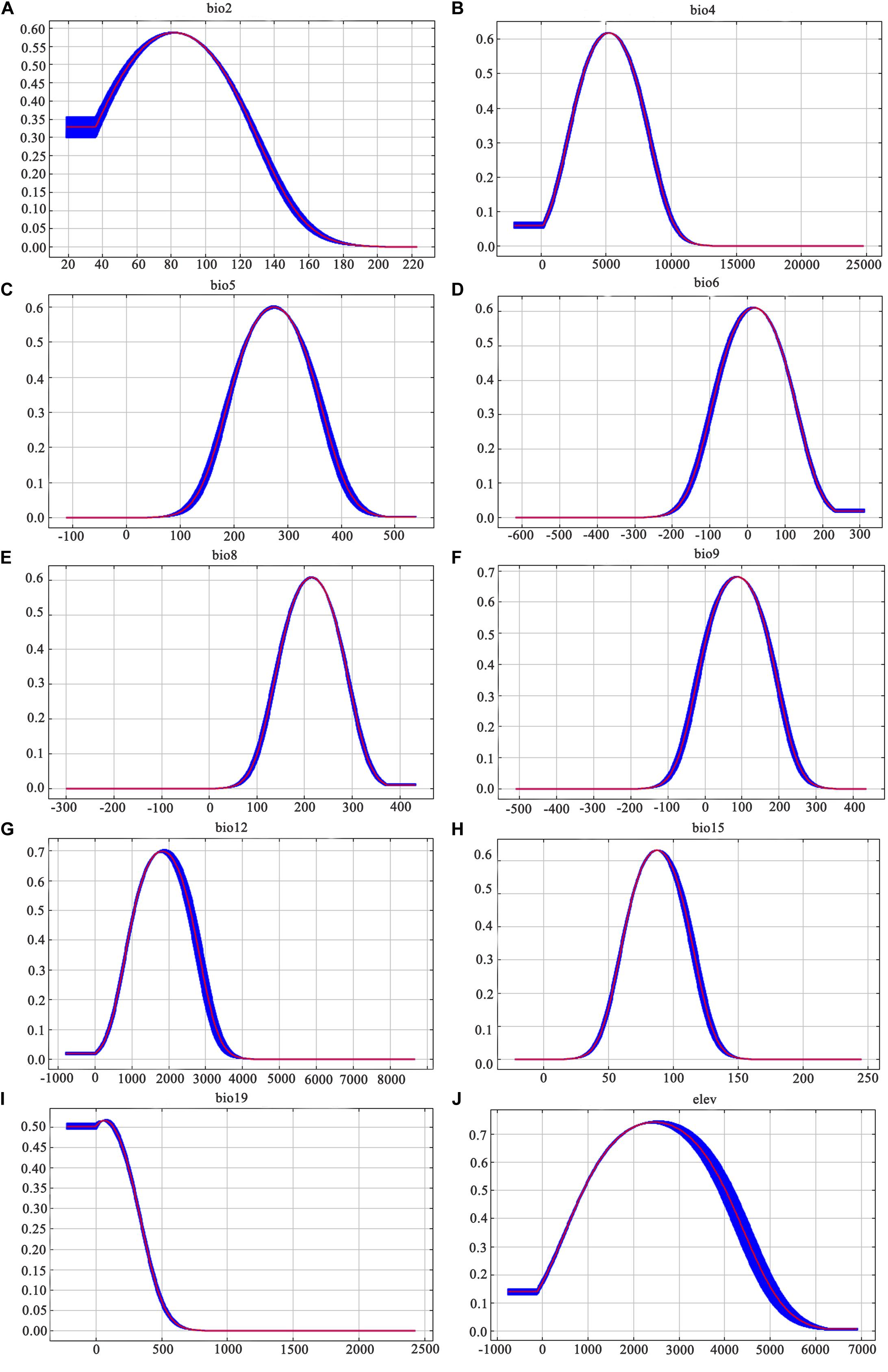
Figure 5. Response curves of the ten ecological variables affecting the growth of Dendrobium nobile: (A) mean diurnal range; (B) temperature seasonality; (C) maximum temperature of warmest month; (D) minimum temperature of the coldest month; (E) mean temperature of the wettest quarter; (F) mean temperature of driest quarter; (G) annual precipitation; (H) precipitation seasonality; (I) precipitation of coldest quarter; and (J) elevation. Values shown are the average of 10 replicate runs: blue margins show ± SD calculated over ten replicates.
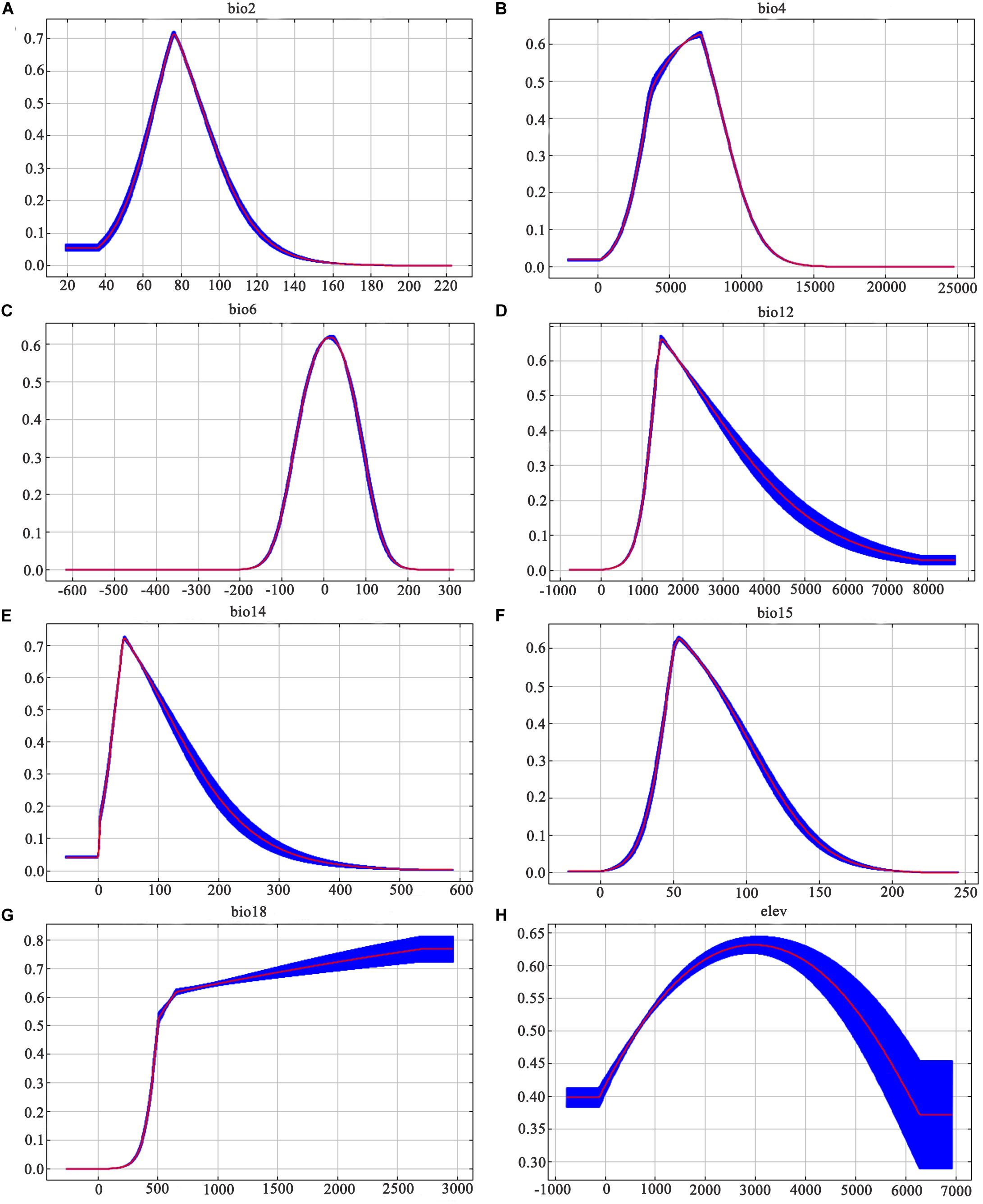
Figure 6. Response curves of the fifteen ecological variables affecting the growth of Dendrobium moniliforme: (A) mean diurnal range; (B) temperature seasonality; (C) minimum temperature of the coldest month; (D) annual precipitation; (E) precipitation of driest month; (F) precipitation seasonality; (G) precipitation of warmest quarter; and (H) elevation. Values are shown are the average of 10 replicate runs: blue margins show ± SD calculated over 10 replicates.
In the future (the 2050s and 2070s), under different greenhouse gas emission scenarios, the contribution rate of ecological factors affecting the growth and distribution of D. moniliforme and D. nobile will change to some extent. With the change of time, space, and climate, some ecological factors gradually increase their contribution rate to Dendrobium, while some ecological factors have a smaller impact on Dendrobium, which indicates that Dendrobium is affected by different factors in different situations, and also has a certain ecological adaptability under climate change.
Predicting the Distribution of Suitable Habitats Under Current Conditions
The suitable habitats (from high suitability to low suitability) of D. moniliforme and D. nobile are mainly distributed in the southeastern and southwestern regions of China and the regions around the Tibetan plateau, including Guangdong, Guangxi, Yunnan, Guizhou, and Taiwan. At the same time, D. moniliforme is also distributed in the southern islands of Japan, the coast of South Korea, eastern and northeastern Australia, the eastern coast of Canada, eastern Madagascar, and western Norway. Compared with D. moniliforme, the suitable habitat areas of D. nobile in southern Japan and western Canada are significantly reduced, which indicates that these areas did not meet the climatic conditions required for D. nobile to grow. In contrast, suitable habitat areas for D. nobile exist on the Mediterranean coast and parts of Mozambique and Zimbabwe and the distribution area of D. nobile in China is significantly larger than D. moniliforme. According to the actual distribution and prediction results of Dendrobium, the suitable habitat areas of D. nobile and D. moniliforme are basically consistent with the current actual distribution area and are mainly concentrated in the coastal areas of all continents, which indicates the accuracy of the prediction results and the importance of temperature and moisture to the growth and distribution of Dendrobium.
Predicted Future Potential Species Distribution
The analysis and prediction of the potential suitable distribution of D. nobile and D. moniliforme under the three greenhouse gas emission scenarios (RCP 2.6, RCP 4.5, and RCP 8.5) in the 2050s and 2070s are shown in Figures 7 and 8. Under the RCP 2.6 and RCP 4.5 greenhouse gas emission scenarios, the suitable habitat areas for D. moniliforme will be mainly concentrated in central and eastern Zhejiang, northern Fujian, northwestern Guangdong, Guangxi, Taiwan, and most of Guizhou province. It is also suitable for growth in southern Japan, Canada, and the west coast of Sweden. Under RCP 8.5, the suitable habitat areas for D. moniliforme will be expanded to Anhui, Jiangxi, southeastern Shanxi Province, and parts of Chongqing. Compared with RCP 2.6 and RCP 4.5, the suitable habitat area of D. moniliforme in Guizhou Province will be greatly reduced, which indicates that the climatic conditions of Guizhou Province in the 2050s will be unsuitable for the growth of D. moniliforme under RCP 8.5 conditions. The distribution area of D. moniliforme is roughly the same under the three greenhouse gas emission scenarios in the 2070s. Compared with the 2050s, the suitable area in the coastal areas of eastern Fujian and Zhejiang and parts of Hubei have been significantly reduced. In the long-term, the suitable habitat area of D. moniliforme will be continuously reduced in the future, and is predicted to be reduced by 16% by the 2070s (Table 3). Therefore, it is expected that D. moniliforme resources will face greater challenges in the future.
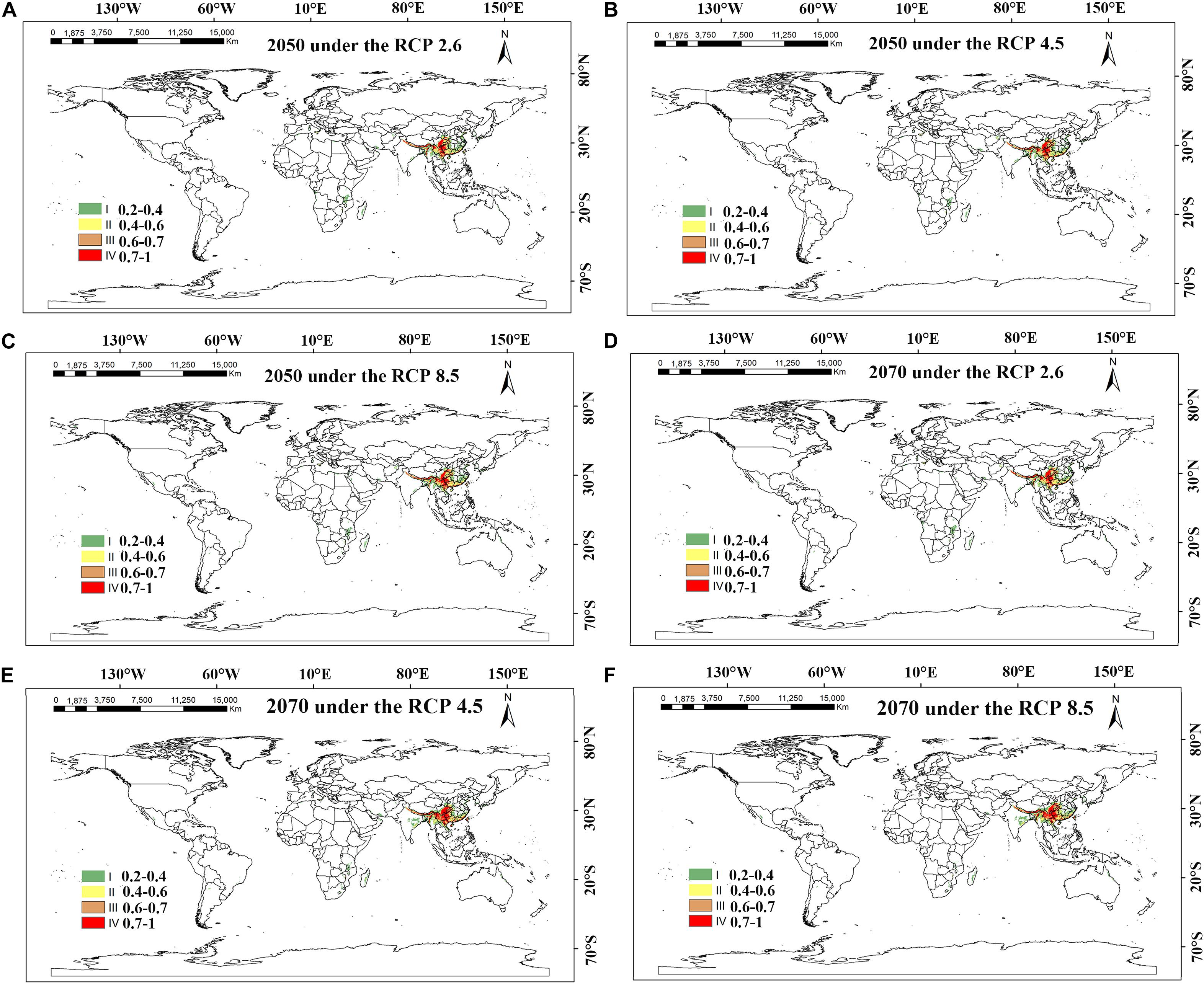
Figure 7. MaxEnt-predicted future species distribution models of Dendrobium nobile on a global scale under different climate scenarios. (A–C) RCP 2050-2.6; RCP 2050-4.5; RCP 2050-8.5; (D–F) CP 2070-2.6; RCP 2070-4.5; RCP 2070-8.5.
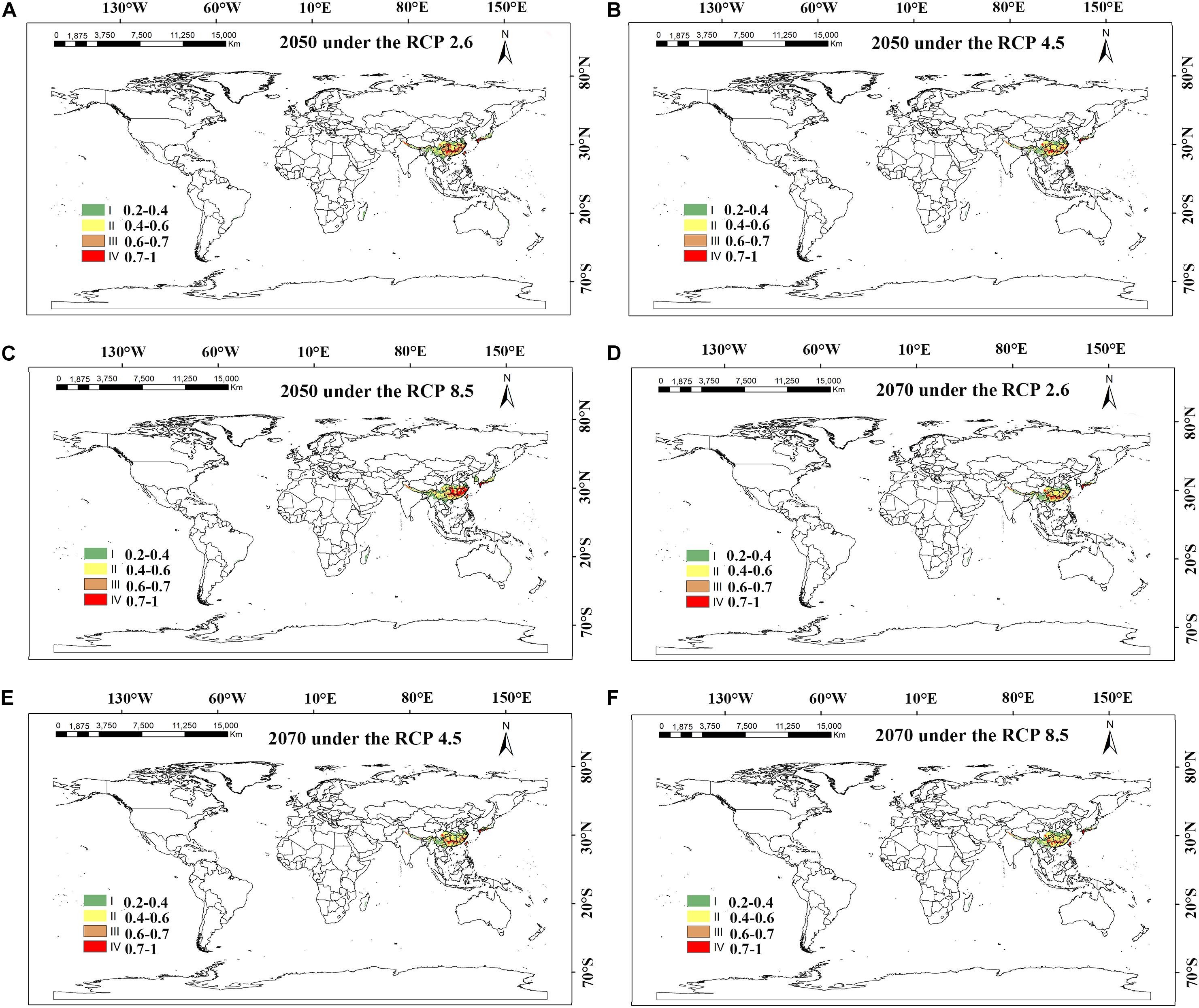
Figure 8. MaxEnt-predicted future species distribution models of Dendrobium moniliforme on a global scale under different climate scenarios. (A–C) RCP 2050-2.6; RCP 2050-4.5; RCP 2050-8.5; (D–F) CP 2070-2.6; RCP 2070-4.5; RCP 2070-8.5.
The potential future distribution of habitats suitable for D. nobile was analyzed. These predictions were largely under the three tested greenhouse gas emission scenarios. In China, D. nobile will be mainly distributed in Guangxi, Fujian, Guizhou, Chongqing, southwestern Taiwan, east coast of Guangdong, and the southern Tibet Plateau of Qinghai Province. Worldwide, it is distributed mainly in a small part of the Mediterranean coast, Zimbabwe, and Zambia. Compared with the 2050s, the area of suitable habitats for D. nobile in the 2070s period will continue to expand. Under RCP4.5, the area suitable for D. nobile is expected to increase by 10% (Table 3).
Dendrobium nobile and D. moniliforme show different changing trends in the context of future climate change. During the period of the 2050s, the area of suitable habitats of D. nobile and D. moniliforme changed under different greenhouse gas emission patterns, but generally made steady progress, which indicates that the two Dendrobium species have some adaptability to climate change. In the 2070s, the suitable habitat area of D. nobile will continue to increase, but that of D. moniliforme will continue to decrease, indicating that D. nobile has better adaptability to environmental changes than D. moniliforme under future climate change scenarios.
Discussion
Significance of Model Predictions and Comparison With Previously Recorded Distributions
Environmental factors affect the growth, quality, and efficacy of Chinese herbal medicines (Grimm et al., 2008; Yang et al., 2011; Huang et al., 2012; Shang et al., 2015). The overexploitation of wild Chinese herbal medicines damages the environment and leads to shortages (Zhang and Li, 2008; Wu et al., 2011). As a traditional Chinese herbal medicine with great medicinal value, Dendrobium needs further development and utilization (Bi et al., 2004; Shao et al., 2004; Xu and Wang, 2005; Zhang et al., 2007; Hsieh et al., 2008; Ng et al., 2012; Pan et al., 2014). However, the destruction of the ecological environment and excessive excavation are increasing the strain on the wild resources of Dendrobium and shrinking the area available for its growth (He et al., 2008). Therefore, to protect Dendrobium supplies, it will be important to understand where it can be grown, both now and in the future (Kolanowska and Konowalik, 2014; Jalal, 2019). In recent years, model methods have been continuously optimized in practice. The MaxEnt model has been widely used as a model to predict the potential geographical distribution of species, and has achieved good prediction results.
As a machine learning model, the MaxEnt model does not require prior data conversion and the elimination of outliers (Boutaba et al., 2018; Liu et al., 2018; Kalinski, 2019). It is also possible to combine data from multiple measurement scales without making statistical assumptions, thus helping to stimulate natural phenomena with non-linear relationships (Phillips, 2005; Kramer-Schadt et al., 2013; Phillips et al., 2017). In recent years, the MaxEnt model has been widely used in the analysis of invasive alien species and the study of the suitability of Chinese medicinal materials, and has achieved good prediction results (Fan et al., 2019; Huang et al., 2019; Tang et al., 2019; Wei et al., 2019). Although the MaxEnt model has some shortcomings, it has high performance and accuracy in modeling, high operational modeling capabilities, and a very friendly interactive interface. Because of these advantages, the MaxEnt model is becoming increasingly and more widely used (Warren and Seifert, 2011; Renner and Warton, 2013; Yuan et al., 2015; West et al., 2016; Yi et al., 2016).
Given the pressures on Dendrobium resources and the importance of estimating the current and future distributions of suitable Dendrobium habitats, the MaxEnt model is well-suited to this task. To our knowledge, this is the first time that the MaxEnt model and ArcGIS 10.5 (Esri, Redlands, CA, United States) have been used to model the distribution of Dendrobium species. According to available records, Dendrobium is mainly distributed in tropical and subtropical regions, such as southeast and southwest China, the areas surrounding the Qinghai-Tibet Plateau, Japan, India, Nepal, Sikkim, Bhutan, Myanmar, Thailand, Laos, Vietnam, and Europe and America (Baker and Baker, 1996; Lo et al., 2004; Zhang et al., 2005; Asahina et al., 2010). The current simulation results of Dendrobium are basically the same as the actual distribution, which also illustrates the accuracy of the MaxEnt prediction results from another aspect.
Prospective Change of Distribution Ranges in the Future
Dendrobium is a perennial epiphytic herb with unique habitats and strict microclimate environmental requirements (Ding et al., 2018). Dendrobium is mainly distributed in warm and cool environments with an average annual temperature of 18–23°C, annual precipitation of 100–1200 mm, and relative air humidity of more than 80% (Wood, 2006; Hao L.L. et al., 2012; Sun et al., 2018). Strictly limited by water, wild Dendrobium has a low reproduction rate, poor natural regeneration ability, slow growth, and a narrow distribution range (Hu, 1970; Dressler, 1993; Ng et al., 2012; Xu et al., 2013). Orchidacea plants have higher richness at higher temperatures and are more stable in humid regions, which decrease with increasing latitude, indicating that the geographical distribution of orchidaceous plants is closely related to climate factors, such as precipitation and temperature (Teng et al., 2016).
The MaxEnt prediction results show that, at present, D. nobile is mainly distributed in southeastern and southwestern China, northern India and southeastern Asia, southeastern Africa, the Mediterranean coast, and the south coast of South Korea, and Japan. Some inland areas of India will be suitable for its survival under future climate change conditions. Unlike D. nobile, the current distribution of D. moniliforme is mainly concentrated in China, South Korea, and Japan, and D. moniliforme is absent from regions outside of Asia. We predict that the distribution area of D. moniliforme is unlikely to change much in the future, but also predict that the fitness of D. moniliforme will decrease as the climate changes. The predictions suggest that D. nobile and D. moniliforme have different environmental adaptabilities. The impact of climate change on plant growth and distribution is complex and multifaceted, leading to differences in the distribution and area of plants in suitable habitats in response to climate change (Qin et al., 2015; Cao et al., 2016; Tang et al., 2017; Li et al., 2020).
Li et al. (2019) that predicted the future suitable habitats for Tuber mustard, the predicted area of suitable habitats was increased, but were different in comparison between the 2050s and 2070s. The distribution areas of the two species of Dendrobium are mainly concentrated in the coastal areas of tropical and subtropical regions, which is consistent with the literature (Zhang et al., 2005; Xiang et al., 2013). The distribution and planting of Orchidacea plants in India have been studied, with the authors reporting that India has climatic conditions suitable for the growth of orchid plants (Jennings, 1875). There are also related studies on the distribution of Dendrobium in Japan, Korea, Southeast Asia, and other countries and regions, which are consistent with the results of our MaxEnt prediction (Ichihashi, 1997; Lavarack et al., 2000; Kim et al., 2015). But findings on the seasonal flowering of Dendrobium in Hawaii is inconsistent with the distribution area of our simulation results, which might be related to the choice of climate variables (Paull et al., 1995).
In summary, the two Dendrobium distribution areas are concentrated in the coastal areas of tropical and subtropical areas, and the warm and humid air from the ocean provides sufficient moisture for these areas (Sarntinoranont and Wannakrairoj, 2010; Li et al., 2017). Compared with inland areas, the coastal areas have high winter temperatures and low summer temperatures, and the daily and monthly changes in temperature differences are relatively small, providing suitable climate conditions for the growth of Dendrobium leaves and flowers (He et al., 1998). The greater silicon elements in coastal areas might also benefit Dendrobium species by improving the photosynthetic capacity and drought tolerance (Carvalho et al., 2013; Reiners Carvalho et al., 2013). As an important distribution area with more than 70 Dendrobium species, China should rationally develop wild Dendrobium resources based on the suitable habitat data presented here, and artificial cultivation should be strengthened to meet growing demand. It might also be beneficial to attempt to cultivate Dendrobium in the humid and warm coastal areas of all continents, such as the west coast of North America and the east coast of Africa, especially D. nobile, which has relatively better adaptability to environmental change.
Environmental Variables Affecting the Geographical Distribution of Dendrobium
The suitable habitat area of D. moniliforme in China will decrease as the temperature rises, and the area of D. nobile will gradually increase, which shows that the impact of climate change on the growth and distribution of Dendrobium is nuanced and the distribution pattern of these plants will be the result of long-term adaptation to climate (Watson et al., 1998; Macel et al., 2007; Franks et al., 2014; Christmas et al., 2016). Future climate change will surely alter the distribution of plant species throughout the world (Woodward and Williams, 1987; Wu, 2011), and many species will be limited by their inability to adapt to climate change (Leemans and Eickhout, 2004), resulting in instability, decreasing continuity, and increasing fragmentation (Ashcroft et al., 2009). Climate change is expected to reduce the area of suitable habitat for U. lamellosa, and this will cause local extinctions (Yan et al., 2018). Related research on other species found that future climate change will affect the distribution of Sinopodophyllum hexandrum and Amygdalus mongolica adaptive areas, leading to the extinction of these two species (Guo et al., 2014; Ma et al., 2014).
Differences in adaptive ecological factors might account for the opposite trends we report for the suitable habitat areas of Dendrobium under future climate conditions. Here we report that the mean diurnal range (bio2), temperature seasonality (bio4), minimum temperature of the cold month (bio6), precipitation seasonality (bio15), and precipitation of the warmest quarter (bio18) have important effects on the growth and distribution of D. moniliforme and D. nobile. Our analyses suggest that Dendrobium can grow at sites with limited variations in temperature, which are warm and humid, and where the rainy and hot periods overlap. This might be mainly related to the effect of the temperature cycle on the flowering of Dendrobium and endogenous hormones (Campos and Kerbauy, 2004; Mandal et al., 2004; Sarathum et al., 2010; Davies, 2013). Rainfall will also affect the germination rate of Dendrobium seeds (Vendrame et al., 2007; Huehne and Bhinija, 2012).
The leaves and flowers of Dendrobium Sonia were studied in response to strong light and high temperature under natural conditions, and it was found that excessive temperature causes damage to the leaves and flowers, and that the flowers were especially sensitive to strong light (He et al., 1998). For annual and biennial D. nobile, 25°C is the optimum temperature for plant growth, which has a significant positive impact on its reproductive ability (Ch et al., 2001; Hao L. et al., 2012). For D. officinale, photosynthesis is optimal at 30°C, but polysaccharide content was optimal at 20°C (Ai et al., 2010), which likely accounts for why Dendrobium is widely distributed in the shade under trees in the forest.
Dendrobium species form unique symbiotic relationships with mycorrhizal fungi (Zhang et al., 2012). Orchid mycorrhizal fungi promote seed germination of Dendrobium orchids (Swangmaneecharern et al., 2012). Endophytic fungi affect the growth and polysaccharide and alkaloid content of D. nobile by increasing the number of aerial roots (Chen and Guo, 2005). Lanthanum nitrate and orchid mycorrhizal fungi promote the growth of D. nobile, including underground and aboveground biomass, and increase the content of active components (Wei et al., 2014). The growth and distribution of mycorrhizal fungi are largely affected by temperature and humidity, and at the same time can improve plant tolerance to temperature changes (Hacskaylo et al., 1965; Koske, 1987; Bunn et al., 2009). Taken together, temperature and moisture affect the distribution of Dendrobium either directly, by affecting the growth of Dendrobium, or indirectly, by affecting mycorrhizal fungi.
The Application Prospect of MaxEnt in Endangered Species
The MaxEnt model is a model that predicts the spatial distribution and geographic scale of species based on the theory of maximum entropy, which was written using the JAVA language (Phillips et al., 2006; Baldwin, 2009). The MaxEnt model calculates the possible distribution of the target species in the predicted area based on the actual distribution points of the species and the environmental variables of the species distribution area (Elith et al., 2011). Because the potential distribution area of the predicted species distribution is close to the actual distribution area of the species, the MaxEnt model has been widely used (Cao et al., 2013). In the future, the Maxent model has extensive prospects in predicting the potential distribution and suitability of endangered species. Sustainable and efficient environmental monitoring, scientific protection and management, and restriction of human activities are recommended for endangered plants according to the results of the MaxEnt model. In particular, we should consider the impact of future rapid climate change on the distribution of endangered plant resources, strengthen climate monitoring and genetic diversity protection, and build an early warning system for future climate change.
The sample capacity has an influence on the prediction accuracy and stability of the MaxEnt model. At the same time, there is a lack of research on soil factors and human factors when simulating the future suitable areas of plants with the MaxEnt model. At present, soil science research focuses on a single soil component and a small geographic range, without research on future trends of large-scale soil changes (Walter et al., 2003). Human influences affect the future direction of climate and soil changes, but they are complex and difficult to simulate with models. Another study discussed the impact of climate change on the distribution of plants, but did not discuss the impact of soil and human factors on the future distribution of these plants (Khanum et al., 2013). We believe that the influence of soil and human influences on the distribution of species will be an important direction for future research.
Conclusion
To our knowledge, this is the first study to systematically investigate the geographic distribution and present and future potential distributions of D. moniliforme and D. nobile. D. moniliforme and D. nobile are abundant in the south of China. In other parts of the world, D. moniliforme and D. nobile are mainly distributed in parts of Japan and South Korea with tropical and subtropical monsoon climates.
In the future, the coastal areas of multiple continents are expected to become suitable for individual species of Dendrobium. In the 2050s and 2070s, we tested three greenhouse gas emission scenarios [high emission (RCP 8.5), medium emission (RCP 4.5), and low emission (RCP 2.6)]. Our model predicts that, under climate warming, the distribution and area of the adaptive zones of D. moniliforme and D. nobile will remain relatively stable in the 2050s. In the 2070s, the area of the adaptive zone of D. moniliforme will shrink (by up to 16%), while the area of the adaptive zone of D. nobile is expected to expand by 1 to 10%, suggesting that D. nobile has better adaptability to climate change than D. moniliforme.
In recent years, the intensification of conversion of farmland to forest and urbanization has seriously damaged the environment of Dendrobium, many species have become extremely rare, and some endemic species with narrow habitat and small geographical distribution are close to extinction. Dendrobium species have medicinal and edible value. Relevant research shows that wild Dendrobium has greater medicinal activities than greenhouse cultivated Dendrobium. Considering the impact of predicted climate change on Dendrobium plants, we should carefully investigate the growth characteristics and cultivation methods of each plant, and consider the development of sustainable cultivation methods for the cultivation of the most valuable plants.
Data Availability Statement
The original contributions presented in the study are included in the article/supplementary material, further inquiries can be directed to the corresponding author.
Author Contributions
XT and YY: conception and design of the research. XT: acquisition of data and statistical analysis. YY: analysis and interpretation of data. YY and JZ: drafting the manuscript. All authors read and approved the final manuscript.
Conflict of Interest
The authors declare that the research was conducted in the absence of any commercial or financial relationships that could be construed as a potential conflict of interest.
Funding
This project was funded by the Priority Academic Program Development of Jiangsu Higher Education Institutions (PAPD), the National Foundation of Forestry Science and Technology Popularization (Grant No. [2015]17), and the Major Fund for Natural Science of Jiangsu Higher Education Institutions (Grant No. 15KJA220004). The funders had no role in study design, data collection and analysis, decision to publish, or preparation of the manuscript. We thank TopEdit (www.topeditsci.com) for its linguistic assistance during the preparation of this manuscript.
Footnotes
- ^ http://www.gbif.org/
- ^ http://www.cvh.org.cn/
- ^ http://www.eflora.cn
- ^ http://www.cnki.net/
- ^ http://earth.google.com
- ^ http://www.worldclim.org
- ^ http://www.ngdc.noaa.gov/mgg/topo/globe.html
References
Ai, J., Yan, N., Hu, H., and Li, S. (2010). Effects of temperature on the growth and physiological characteristics of Dendrobium officinale (Orchidaceae). Acta Bot. Yunnanica 32, 420–426.
Asahina, H., Shinozaki, J., Masuda, K., Morimitsu, Y., and Satake, M. (2010). Identification of medicinal Dendrobium species by phylogenetic analyses using matK and rbcL sequences. J. Nat. Med. 64, 133–138. doi: 10.1007/s11418-009-0379-8
Ashcroft, M. B., Chisholm, L. A., and French, K. O. (2009). Climate change at the landscape scale: predicting fine−grained spatial heterogeneity in warming and potential refugia for vegetation. Glob. Change Biol. 15, 656–667. doi: 10.1111/j.1365-2486.2008.01762.x
Baldwin, R. A. (2009). Use of maximum entropy modeling in wildlife research. Entropy 11, 854–866. doi: 10.3390/e11040854
Bi, Z.-M., Wang, Z.-T., and Xu, L.-S. (2004). Chemical constituents of Dendrobium moniliforme. Acta Botanica Sin. Eng. Edit. 46, 124–126.
Boutaba, R., Salahuddin, M. A., Limam, N., Ayoubi, S., Shahriar, N., Estrada-Solano, F., et al. (2018). A comprehensive survey on machine learning for networking: evolution, applications and research opportunities. J. Int. Ser. Appl. 9:16.
Bunn, R., Lekberg, Y., and Zabinski, C. (2009). Arbuscular mycorrhizal fungi ameliorate temperature stress in thermophilic plants. Ecology 90, 1378–1388. doi: 10.1890/07-2080.1
Campos, K. O., and Kerbauy, G. B. (2004). Thermoperiodic effect on flowering and endogenous hormonal status in Dendrobium (Orchidaceae). J. Plant Physiol. 161, 1385–1387. doi: 10.1016/j.jplph.2004.07.008
Cao, B., Bai, C., Zhang, L., Li, G., and Mao, M. (2016). Modeling habitat distribution of Cornus officinalis with Maxent modeling and fuzzy logics in China. J. Plant Ecol. 9, 742–751. doi: 10.1093/jpe/rtw009
Cao, Y., DeWalt, R. E., Robinson, J. L., Tweddale, T., Hinz, L., and Pessino, M. (2013). Using Maxent to model the historic distributions of stonefly species in Illinois streams: the effects of regularization and threshold selections. Ecol. Model. 259, 30–39. doi: 10.1016/j.ecolmodel.2013.03.012
Carvalho, P. R., Faria, R. T., de Batista Fonseca, I. C., and de Andrade Junior, O. (2013). Efeito do silício na qualidade de Àores de Dendrobium nobile (Orchidaceae). Ciências Agrárias 34, 1615–1621.
Ch, I. O. M., Zhu, L., Zhang, Y., Zhang, M., Bie, Z., Chen, S., et al. (2001). Effect of light intensities and temperatures on growth of dendrobium nobile lindl. Acta Phytoecol. Sin. 25, 325–330.
Chen, X., and Guo, S. (2005). Effects of four species of endophytic fungi on the growth and polysaccharide and alkaloid contents of Dendrobium nobile. Zhongguo Zhong Yao Za Zhi 30, 253–257.
Cheng, J., Dang, P. P., Zhao, Z., Yuan, L. C., Zhou, Z. H., Wolf, D., et al. (2019). An assessment of the Chinese medicinal Dendrobium industry: Supply, demand and sustainability. J. Ethnopharmacol. 229, 81–88. doi: 10.1016/j.jep.2018.09.001
Christmas, M. J., Breed, M. F., and Lowe, A. J. (2016). Constraints to and conservation implications for climate change adaptation in plants. Conserv. Genet. 17, 305–320. doi: 10.1007/s10592-015-0782-5
Davies, P. J. (2013). Plant Hormones: Physiology, Biochemistry and Molecular Biology. Berline: Springer Science and Business Media.
De Souza, R. A., and De Marco, P. Jr. (2014). The use of species distribution models to predict the spatial distribution of deforestation in the western Brazilian Amazon. Ecol. Model. 291, 250–259. doi: 10.1016/j.ecolmodel.2014.07.007
Ding, J. T., Hang-Yao, T., Ze-Lin, Z., Min, H., and Sheng-Jun, Z. (2018). Precise control and prediction of the greenhouse growth environment of Dendrobium candidum. Comput. Electron. Agric. 151, 453–459. doi: 10.1016/j.compag.2018.06.037
Dorfman, D. D., Berbaum, K. S., and Metz, C. E. (1992). Receiver operating characteristic rating analysis: generalization to the population of readers and patients with the jackknife method. Investigat. Radiol. 27, 723–731. doi: 10.1097/00004424-199209000-00015
Dressler, R. L. (1993). Phylogeny and Classification of the Orchid Family. Cambridge: Cambridge University Press.
Elith, J., Graham, C. H., Anderson, P. R., Dudík, M., Ferrier, S., Guisan, A., et al. (2006). Novel methods improve prediction of species’ distributions from occurrence data. Ecography 29, 129–151.
Elith, J., Phillips, S. J., Hastie, T., Dudík, M., Chee, Y. E., and Yates, C. J. (2011). A statistical explanation of MaxEnt for ecologists. Div. Dis. 17, 43–57. doi: 10.1111/j.1472-4642.2010.00725.x
Faleiro, F. V., Machado, R. B., and Loyola, R. D. (2013). Defining spatial conservation priorities in the face of land-use and climate change. Biol. Conserv. 158, 248–257. doi: 10.1016/j.biocon.2012.09.020
Fan, D., Zhong, H., Hu, B., Tian, Z., Sun, L., Fischer, G., et al. (2019). Agro-ecological suitability assessment of Chinese Medicinal Yam under future climate change. Environ. Geochem. Health 42, 1–14.
Franklin, J. (2010). Mapping Species Distributions: Spatial Inference and Prediction. Cambridge: Cambridge University Press.
Franks, S. J., Weber, J. J., and Aitken, S. N. (2014). Evolutionary and plastic responses to climate change in terrestrial plant populations. Evol. Appl. 7, 123–139. doi: 10.1111/eva.12112
Graham, M. H. (2003). Confronting multicollinearity in ecological multiple regression. Ecology 84, 2809–2815. doi: 10.1890/02-3114
Grimm, N. B., Faeth, S. H., Golubiewski, N. E., Redman, C. L., Wu, J., Bai, X., et al. (2008). Global change and the ecology of cities. Science 319, 756–760.
Guo, Y., Wei, H., Lu, C., Gao, B., and Gu, W. (2016). Predictions of potential geographical distribution and quality of Schisandra sphenanthera under climate change. PeerJ 4:e2554. doi: 10.7717/peerj.2554
Guo, Y., Wei, H., Lu, C., Zhang, H., and Gu, W. (2014). Predictions of potential geographical distribution of Sinopodophyllum hexandrum under climate change. Chin. J. Plant Ecol. 38, 249–261. doi: 10.3724/sp.j.1258.2014.00022
Hacskaylo, E., Palmer, J. G., and Vozzo, J. (1965). Effect of temperature on growth and respiration of ectotrophic mycorrhizal fungi. Mycologia 57, 748–756. doi: 10.2307/3757010
Hao, L., Zhang, X., Zhang, W., and Yi, Y. (2012). Effect of temperature on growth and metabolism of Dendrobium nobile. Zhongcaoyao 43, 372–374.
Hao, L. L., Zhang, X. M., and Zhang, W. F. (2012). Effect of temperature on growth and metabolism of Dendrobium nobile. Zhongcaoyao 43, 372–374
Haverkost, T. R., Gardner, S. L., and Peterson, A. T. (2010). Predicting the distribution of a parasite using the ecological niche model. GARP. Rev. Mex. Biodiv. 81, 895–902.
He, J., Khoo, G., and Hew, C. (1998). Susceptibility of CAM Dendrobium leaves and flowers to high light and high temperature under natural tropical conditions. Environ. Exper. Bot. 40, 255–264. doi: 10.1016/s0098-8472(98)00042-2
He, T., Chun, Z., Luo, A., Fan, Y., Liu, J., and Hu, M. (2008). Wild resources and conservation of dendrobium in Sichuan. China. Chin. J. Appl. Envir. Biol. 14, 710–715.
Hemery, L. G., Marion, S. R., Romsos, C. G., Kurapov, A. L., and Henkel, S. K. (2016). Ecological niche and species distribution modelling of sea stars along the Pacific Northwest continental shelf. Diver. Distrib. 22, 1314–1327. doi: 10.1111/ddi.12490
Hijmans, R. J., Phillips, S., Leathwick, J., and Elith, J. (2013). dismo: Species distribution modeling R package version 0.8-17.
Howell, K. L., Holt, R., Endrino, I. P., and Stewart, H. (2011). When the species is also a habitat: comparing the predictively modelled distributions of Lophelia pertusa and the reef habitat it forms. Biol. Conserv. 144, 2656–2665. doi: 10.1016/j.biocon.2011.07.025
Hsieh, Y. S.-Y., Chien, C., Liao, S. K.-S., Liao, S.-F., Hung, W.-T., Yang, W.-B., et al. (2008). Structure and bioactivity of the polysaccharides in medicinal plant Dendrobium huoshanense. Bioorg. Med. Chem. 16, 6054–6068. doi: 10.1016/j.bmc.2008.04.042
Huang, L., Fu, J., and Chen, S. (2012). Academic study on ecological variation of traditional Chinese medicinal materials. Zhong Cao Yao 43, 1249–1258.
Huang, Z., Xie, L., Wang, H., Zhong, J., Li, Y., Liu, J., et al. (2019). Geographic distribution and impacts of climate change on the suitable habitats of Zingiber species in China. Ind. Crops Prod. 138:111429. doi: 10.1016/j.indcrop.2019.05.078
Huehne, P. S., and Bhinija, K. (2012). Application of cryoprotectants to improve low temperature storage survival of orchid seeds. Sci. Hortic. 135, 186–193. doi: 10.1016/j.scienta.2011.11.026
Jalal, J. S. (2019). Diversity and distribution of orchids of Goa. Western Ghats, India. J. Threat. Taxa 11, 15015–15042. doi: 10.11609/jott.4499.11.15.15015-15042
Jennings, S. (1875). Orchids and how to Grow them in India and Other Tropical Climates. London: L. Reeve and Company.
Kalinski, C. E. (2019). Building Better Species Distribution Models with Machine Learning: Assessing the Role of Covariate Scale and Tuning in Maxent Models. California: University of Southern California.
Khanum, R., Mumtaz, A., and Kumar, S. (2013). Predicting impacts of climate change on medicinal asclepiads of Pakistan using Maxent modeling. Acta Oecol. 49, 23–31. doi: 10.1016/j.actao.2013.02.007
Kim, Y.-K., Kang, K.-W., and Kim, K.-J. (2015). Genetic differences between Korean-Japanese and Chinese-Taiwanese Dendrobium moniliforme (L.) Sw. Korean J. Plant Taxon. 45, 145–157. doi: 10.11110/kjpt.2015.45.2.145
Kolanowska, M., and Konowalik, K. (2014). Niche conservatism and future changes in the potential area coverage of Arundina graminifolia, an invasive orchid species from Southeast Asia. Biotropica 46, 157–165. doi: 10.1111/btp.12089
Koske, R. (1987). Distribution of VA mycorrhizal fungi along a latitudinal temperature gradient. Mycologia 79, 55–68. doi: 10.1080/00275514.1987.12025370
Kramer−Schadt, S., Niedballa, J., Pilgrim, J. D., Schröder, B., Lindenborn, J., Reinfelder, V., et al. (2013). The importance of correcting for sampling bias in MaxEnt species distribution models. Divers. Distrib. 19, 1366–1379.
Lavarack, B., Harris, W., and Stocker, G. (2000). Dendrobium and its Relatives. Portland: Timber Press.
Leemans, R., and Eickhout, B. (2004). Another reason for concern: regional and global impacts on ecosystems for different levels of climate change. Glob. Environ. Change 14, 219–228. doi: 10.1016/j.gloenvcha.2004.04.009
Li, H., Liu, X., Wang, J., Xing, L., and Fu, Y. (2019). Maxent modelling for predicting climate change effects on the potential planting area of tuber mustard in China. J. Agric. Sci. 157, 375–381. doi: 10.1017/s0021859619000686
Li, J.-L., Zhao, Z., Liu, H.-C., Luo, C.-L., and Wang, H.-L. (2017). Influence of light intensity and water content of medium on total dendrobine of Dendrobium nobile Lindl. Asian Pac. J. Trop. Med. 10, 1095–1100. doi: 10.1016/j.apjtm.2017.10.015
Li, J., Fan, G., and He, Y. (2020). Predicting the current and future distribution of three Coptis herbs in China under climate change conditions, using the MaxEnt model and chemical analysis. Sci. Total Environ. 698:134141. doi: 10.1016/j.scitotenv.2019.134141
Li, L.-p, Zhang, B.-g, Xiao, P.-g, Zhang, Z., Qi, Y.-d, Li, X.-j, et al. (2015). Native medicinal plant richness distribution patterns and environmental determinants of Xinjiang, Northwest China. Chin. Herb. Med. 7, 45–53. doi: 10.1016/s1674-6384(15)60019-3
Li, X., Tian, H., Wang, Y., Li, R., Song, Z., Zhang, F., et al. (2013). Vulnerability of 208 endemic or endangered species in China to the effects of climate change. Reg. Environ. Change 13, 843–852. doi: 10.1007/s10113-012-0344-z
Liu, C., White, M., and Newell, G. (2018). Detecting outliers in species distribution data. J. Biogeogr. 45, 164–176. doi: 10.1111/jbi.13122
Lo, S.-F., Nalawade, S. M., Kuo, C.-L., Chen, C.-L., and Tsay, H.-S. (2004). Asymbiotic germination of immature seeds, plantlet development and ex vitro establishment of plants of Dendrobium tosaense makino—A medicinally improrant orchid. Vitro Cell. Dev. Biol. Plant 40, 528–535. doi: 10.1079/ivp2004571
Lobo, J. M., Jiménez−Valverde, A., and Real, R. (2008). AUC: a misleading measure of the performance of predictive distribution models. Glob. Ecol. Biogeogr. 17, 145–151. doi: 10.1111/j.1466-8238.2007.00358.x
Ma, S., Nie, Y., Geng, Q., and Wang, R. (2014). Impact of climate change on suitable distribution range and spatial pattern in Amygdalus mongolica. Chin. J. Plant Ecol. 38, 262–269. doi: 10.3724/sp.j.1258.2014.00023
Macel, M., Lawson, C. S., Mortimer, S. R., Šmilauerova, M., Bischoff, A., Crémieux, L., et al. (2007). Climate vs. soil factors in local adaptation of two common plant species. Ecology 88, 424–433. doi: 10.1890/0012-9658(2007)88[424:cvsfil]2.0.co;2
Mandal, T., Munsi, P., and Roychowdhury, N. (2004). “Evaluation of different Dendrobium spp. under polyhouse in North-East Indian hills,” in VII International Symposium on Protected Cultivation in Mild Winter Climates: Production, Pest Management and Global Competition 659, (Kissimmee, FL: ISHS Acta Horticulturae), 491–498. doi: 10.17660/actahortic.2004.659.65
Meehl, G. A., Washington, W. M., Arblaster, J. M., Hu, A., Teng, H., Kay, J. E., et al. (2013). Climate change projections in CESM1 (CAM5) compared to CCSM4. J. Clim. 26, 6287–6308. doi: 10.1175/jcli-d-12-00572.1
Meehl, G. A., Washington, W. M., Arblaster, J. M., Hu, A., Teng, H., Tebaldi, C., et al. (2012). Climate system response to external forcings and climate change projections in CCSM4. J. Clim. 25, 3661–3683. doi: 10.1175/jcli-d-11-00240.1
Motloung, R., Robertson, M., Rouget, M., and Wilson, J. (2014). Forestry trial data can be used to evaluate climate-based species distribution models in predicting tree invasions. NeoBiota 20:31. doi: 10.3897/neobiota.20.5778
Muscarella, R., Galante, P. J., Soley−Guardia, M., Boria, R. A., Kass, J. M., Uriarte, M., et al. (2014). ENM eval: An R package for conducting spatially independent evaluations and estimating optimal model complexity for Maxent ecological niche models. Methods Ecol. Evol. 5, 1198–1205. doi: 10.1111/2041-210x.12261
Ng, T. B., Liu, J., Wong, J. H., Ye, X., Sze, S. C. W., Tong, Y., et al. (2012). Review of research on Dendrobium, a prized folk medicine. Appl. Microbiol. Biotechnol. 93, 1795–1803. doi: 10.1007/s00253-011-3829-7
Pan, L.-H., Li, X.-F., Wang, M.-N., Zha, X.-Q., Yang, X.-F., Liu, Z.-J., et al. (2014). Comparison of hypoglycemic and antioxidative effects of polysaccharides from four different Dendrobium species. Int. J. Biol. Macromol. 64, 420–427. doi: 10.1016/j.ijbiomac.2013.12.024
Paull, R. E., Leonhardt, K. W., Higaki, T., and Imamura, J. (1995). Seasonal flowering of Dendrobium ‘Jaquelyn Thomas’ in Hawaii. Sci. Hortic. 61, 263–272. doi: 10.1016/0304-4238(94)00744-z
Peterson, A. T., Papeş, M., and Soberón, J. (2008). Rethinking receiver operating characteristic analysis applications in ecological niche modeling. Ecol. Model. 213, 63–72. doi: 10.1016/j.ecolmodel.2007.11.008
Petitpierre, B., Kueffer, C., Broennimann, O., Randin, C., Daehler, C., and Guisan, A. (2012). Climatic niche shifts are rare among terrestrial plant invaders. Science 335, 1344–1348. doi: 10.1126/science.1215933
Phillips, S. J., Anderson, R. P., Dudík, M., Schapire, R. E., and Blair, M. E. (2017). Opening the black box: An open−source release of Maxent. Ecography 40, 887–893. doi: 10.1111/ecog.03049
Phillips, S. J., Anderson, R. P., and Schapire, R. E. (2006). Maximum entropy modeling of species geographic distributions. Ecol. Model. 190, 231–259. doi: 10.1016/j.ecolmodel.2005.03.026
Pourghasemi, H. R., Yousefi, S., Kornejady, A., and Cerdà, A. (2017). Performance assessment of individual and ensemble data-mining techniques for gully erosion modeling. Sci. Total Environ. 609, 764–775. doi: 10.1016/j.scitotenv.2017.07.198
Qin, Z., Zhang, J.-e, DiTommaso, A., Wang, R.-l, and Wu, R.-s (2015). Predicting invasions of Wedelia trilobata (L.) Hitchc. with Maxent and GARP models. J. Plant Res. 128, 763–775. doi: 10.1007/s10265-015-0738-3
Raimundo, R. L. G., Fonseca, R. L., Schachetti-Pereira, R., Peterson, A. T., and Lewinsohn, T. M. (2007). Native and exotic distributions of siamweed (Chromolaena odorata) modeled using the genetic algorithm for rule-set production. Weed Sci. 55, 41–48. doi: 10.1614/ws-06-083.1
Reiners Carvalho, P., Tadeu Faria, R., Cristina de Batista Fonseca, I., and de Andrade Junior, O. (2013). Effect of silicon on the quality of flowers of Dendrobium nobile (Orchidaceae). Semina. Ciênc. Agrár. 34, 1615–1622.
Remya, K., Ramachandran, A., and Jayakumar, S. (2015). Predicting the current and future suitable habitat distribution of Myristica dactyloides Gaertn. using MaxEnt model in the Eastern Ghats. Ind. Ecol Eng. 82, 184–188. doi: 10.1016/j.ecoleng.2015.04.053
Renner, I. W., and Warton, D. I. (2013). Equivalence of MAXENT and Poisson point process models for species distribution modeling in ecology. Biometrics 69, 274–281. doi: 10.1111/j.1541-0420.2012.01824.x
Sarathum, S., Tantiviwat, S., Nanakorn, M., Hegele, M., and Wünsche, J. (2010). “Effect of low temperature on flowering and endogenous hormonal status in Dendrobium scabrilingue L,” in XI International Symposium on Plant Bioregulators in Fruit Production 884, (Bologna: ISHS Acta Horticulturae), 651–656. doi: 10.17660/actahortic.2010.884.87
Sarntinoranont, V., and Wannakrairoj, S. (2010). The relationship between environmental factors during rainy season and un-opened floret yellowing in Dendrobium sonia ‘Ear-sakul’. Nat. Sci. 44, 1016–1025.
Shang, Z., Wei, H., Gu, W., Mao, Y., Zhu, L., and Sang, M. (2015). Potential ecological suitability regionalization analysis of Angelica sinensis based on GIS and fuzzy matter element model. J. Chin. Med. Mater. 38, 1370–1374.
Shao, H., Zhang, L., Li, J., and Wei, R. (2004). Advances in research of Dendrobium officinale. Chin. Trad. Herb. Drugs 1:45.
Sun, L., Zheng, Z., and Zhu, J. (2018). Mining Spatio-Temporal Knowledge of Climate for Dendrobium Officinale in Greenhouse Cultivation. Rec. Adv. Electric. Electronic Eng. 11, 160–166. doi: 10.2174/2352096510666170921162448
Swangmaneecharern, P., Serivichyaswat, P., and Nontachaiyapoom, S. (2012). Promoting effect of orchid mycorrhizal fungi Epulorhiza isolates on seed germination of Dendrobium orchids. Sci. Hortic. 148, 55–58. doi: 10.1016/j.scienta.2012.09.013
Swets, J. A. (1988). Measuring the accuracy of diagnostic systems. Science 240, 1285–1293. doi: 10.1126/science.3287615
Szakiel, A., Pączkowski, C., and Henry, M. (2011). Influence of environmental abiotic factors on the content of saponins in plants. Phytochem. Rev. 10, 471–491. doi: 10.1007/s11101-010-9177-x
Tanaka, N., Nakao, K., Tsuyama, I., Higa, M., Nakazono, E., and Matsui, T. (2012). Predicting the impact of climate change on potential habitats of fir (Abies) species in Japan and on the East Asian continent. Proc. Environ. Sci. 13, 455–466. doi: 10.1016/j.proenv.2012.01.039
Tang, C., Wen, J., Zhang, W., Su, J., Xie, C., and Zhang, Y. (2017). Potential distribution of the traditional Tibetan herb Pterocephalus hookeri by Maxent model. Zhongguo Zhong yao za zhi 42, 1871–1876.
Tang, J., Li, J., Lu, H., Lu, F., and Lu, B. (2019). Potential distribution of an invasive pest. Eu platypus parallelus, in China as predicted by Maxent. Pest Manag. Sci. 75, 1630–1637. doi: 10.1002/ps.5280
Teixeira da Silva, J. A., Jin, X., Dobranszki, J., Lu, J., Wang, H., Zotz, G., et al. (2016). Advances in Dendrobium molecular research: Applications in genetic variation, identification and breeding. Mol. Phylog. Evol. 95, 196–216. doi: 10.1016/j.ympev.2015.10.012
Teng, S., He, Y., Shi, J., Lei, C., Zhong, S., Ma, H., et al. (2016). “Effect of ecological factors in different latitudes on the quality of 2-year-old Dendrobium officinale Kimura et Migo,” in Proceedings of the 2015 International Conference on Sustainable Development (ICSD2015), (Singapore: World Scientific.), 974–982.
Townsend Peterson, A., Papeş, M., and Eaton, M. (2007). Transferability and model evaluation in ecological niche modeling: a comparison of GARP and Maxent. Ecography 30, 550–560. doi: 10.1111/j.0906-7590.2007.05102.x
Vendrame, W. A., Carvalho, V., and Dias, J. (2007). In vitro germination and seedling development of cryopreserved Dendrobium hybrid mature seeds. Sci. Hortic. 114, 188–193. doi: 10.1016/j.scienta.2007.06.006
Vertenstein, M., Craig, T., Middleton, A., Feddema, D., and Fischer, C. (2010). CCSM4. 0 User’s Guide. National Center for Atmospheric Research, Boulder. Boulder: community earth system model.
Walter, C., Rossel, R., and McBratney, A. (2003). Spatio-temporal simulation of the field-scale evolution of organic carbon over the landscape. Soil Sci. Soc. Am. J. 67, 1477–1486. doi: 10.2136/sssaj2003.1477
Wang, Y., Xie, B., Wan, F., Xiao, Q., and Dai, L. (2007). Application of ROC curve analysis in evaluating the performance of alien species potential distribution models. Biodiv. Sci. 15, 365–372. doi: 10.1360/biodiv.060280
Warren, D. L., and Seifert, S. N. (2011). Ecological niche modeling in Maxent: the importance of model complexity and the performance of model selection criteria. Ecol. Appl. 21, 335–342. doi: 10.1890/10-1171.1
Watson, R. T., Zinyowera, M. C., Moss, R. H., and Dokken, D. J. (1998). The Regional Impacts Of Climate Change. Geneva: IPCC.
Wei, J., Peng, L., He, Z., Lu, Y., and Wang, F. (2019). Potential distribution of two invasive pineapple pests under climate change. Pest. Manag. Sci. 76, 1652–1663. doi: 10.1002/ps.5684
Wei, M., Yang, C.-Y., Li, A.-J., and Tai, Y.-H. (2014). Effects of Lanthanum Nitrate and Orchid Mycorrhizal Fungi on the Growth and Active Component Accumulation in Dendrobium nobile. Plant Physiol. J. 50, 1059–1064.
West, A. M., Kumar, S., Brown, C. S., Stohlgren, T. J., and Bromberg, J. (2016). Field validation of an invasive species Maxent model. Ecol. Inform. 36, 126–134. doi: 10.1016/j.ecoinf.2016.11.001
Woodward, F. I., and Williams, B. (1987). Climate and plant distribution at global and local scales. Vegetatio 69, 189–197. doi: 10.1007/978-94-009-4061-1_19
Wu, J. (2011). The potential effects of climate change on the distributions of 7 plants in China. Guangxi Zhiwu Guihaia 31, 595–607.
Wu, Q.-y, He, Y.-x, Chen, H.-y, Ji, F.-x, Hu, W., Wang, J., et al. (2011). Unchecked excavation of wild herbal medicines causes the damages of the ecological environment in the Gannan grassland. Pharm. Biol. 56, 465–484.,Google Scholar
Xiang, X.-G., Schuiteman, A., Li, D.-Z., Huang, W.-C., Chung, S.-W., Li, J.-W., et al. (2013). Molecular systematics of Dendrobium (Orchidaceae, Dendrobieae) from mainland Asia based on plastid and nuclear sequences. Mol. Phylogen. Evol. 69, 950–960. doi: 10.1016/j.ympev.2013.06.009
Xiang, X. G., Mi, X. C., Zhou, H. L., Li, J. W., Chung, S. W., Li, D. Z., et al. (2016). Biogeographical diversification of mainland Asian Dendrobium (Orchidaceae) and its implications for the historical dynamics of evergreen broad−leaved forests. J. Biogeogr. 43, 1310–1323. doi: 10.1111/jbi.12726
Xu, J., Han, Q.-B., Li, S.-L., Chen, X.-J., Wang, X.-N., Zhao, Z.-Z., et al. (2013). Chemistry, bioactivity and quality control of Dendrobium, a commonly used tonic herb in traditional Chinese medicine. Phytochem. Rev. 12, 341–367. doi: 10.1007/s11101-013-9310-8
Yan, D., Chen, W., Liu, L., Li, J., Liu, L., and Wang, Y. (2018). Change in current and future geographic distributions of Ulmus lamellosa in China. J. Forest. Res. 29, 1147–1156. doi: 10.1007/s11676-017-0503-7
Yan, H., Feng, L., Zhao, Y., Feng, L., Wu, D., and Zhu, C. (2020). Prediction of the spatial distribution of Alternanthera philoxeroides in China based on ArcGIS and MaxEnt. Glob. Ecol. Conserv. 21:e00856. doi: 10.1016/j.gecco.2019.e00856
Yang, S., Li, J., Zhao, Y., Chen, B., and Fu, C. (2011). Harpagoside variation is positively correlated with temperature in Scrophularia ningpoensis Hemsl. J. Agric. Food Chem. 59, 1612–1621. doi: 10.1021/jf104702u
Yang, X.-Q., Kushwaha, S., Saran, S., Xu, J., and Roy, P. (2013). Maxent modeling for predicting the potential distribution of medicinal plant. Justicia adhatoda L. in Lesser Himalayan foothills. Ecol. Eng. 51, 83–87. doi: 10.1016/j.ecoleng.2012.12.004
Yi, Y.-j, Cheng, X., Yang, Z.-F., and Zhang, S.-H. (2016). Maxent modeling for predicting the potential distribution of endangered medicinal plant (H. riparia Lour) in Yunnan, China. Ecol. Eng. 92, 260–269. doi: 10.1016/j.ecoleng.2016.04.010
Yuan, H.-S., Wei, Y.-L., and Wang, X.-G. (2015). Maxent modeling for predicting the potential distribution of Sanghuang, an important group of medicinal fungi in China. Fungal Ecol. 17, 140–145. doi: 10.1016/j.funeco.2015.06.001
Yuan, Y., Tang, X., Jia, Z., Li, C., Ma, J., and Zhang, J. (2020). The Effects of Ecological Factors on the Main Medicinal Components of Dendrobium officinale under Different Cultivation Modes. Forests 11:94. doi: 10.3390/f11010094
Zhang, H., Luo, D., Mu, X., Xu, M., Wei, H., Luo, J., et al. (2016). Predicting the potential suitable distribution area of the apple snail Pomacea canaliculata in China based on multiple ecological niche models. J. Appl. Ecol. 27, 1277–1284.
Zhang, M.-G., Slik, J. F., and Ma, K.-P. (2016). Using species distribution modeling to delineate the botanical richness patterns and phytogeographical regions of China. Sci. Rep. 6, 1–9. doi: 10.3724/sp.j.1148.2011.00001
Zhang, L., Chen, J., Lv, Y., Gao, C., and Guo, S. (2012). Mycena sp., a mycorrhizal fungus of the orchid Dendrobium officinale. Mycol. Prog. 11, 395–401. doi: 10.1007/s11557-011-0754-1
Zhang, Q., Wen, J., Chang, Z.-Q., Xie, C.-X., and Song, J.-Y. (2018). Evaluation and prediction of ecological suitability of medicinal plant American ginseng (panax quinquefolius). Chin. Herb. Med. 10, 80–85. doi: 10.1016/j.chmed.2018.01.003
Zhang, X., Xu, J.-K., Wang, J., Wang, N.-L., Kurihara, H., Kitanaka, S., et al. (2007). Bioactive bibenzyl derivatives and fluorenones from Dendrobium nobile. J. Nat. Prod. 70, 24–28. doi: 10.1021/np060449r
Zhang, Y.-B., But, P. P.-H., Wang, Z.-T., and Shaw, P.-C. (2005). Current approaches for the authentication of medicinal Dendrobium species and its products. Plant Genet. Resour. 3, 144–148. doi: 10.1079/pgr200578
Zhang, Z.-m, and Li, S.-t (2008). Present situation and thinking of grassland ecological protection and construction in Gansu pastoral area. Grassland Turf 2:812
Keywords: Dendrobium, ArcGIS, MaxEnt, ecologically suitable distributions, climate change
Citation: Tang X, Yuan Y and Zhang J (2020) How Climate Change Will Alter the Distribution of Suitable Dendrobium Habitats. Front. Ecol. Evol. 8:536339. doi: 10.3389/fevo.2020.536339
Received: 06 April 2020; Accepted: 03 September 2020;
Published: 23 October 2020.
Edited by:
Ruscena P. Wiederholt, Everglades Foundation, United StatesReviewed by:
Sharif A. Mukul, University of the Sunshine Coast, AustraliaHossein Bashari, Isfahan University of Technology, Iran
Copyright © 2020 Tang, Yuan and Zhang. This is an open-access article distributed under the terms of the Creative Commons Attribution License (CC BY). The use, distribution or reproduction in other forums is permitted, provided the original author(s) and the copyright owner(s) are credited and that the original publication in this journal is cited, in accordance with accepted academic practice. No use, distribution or reproduction is permitted which does not comply with these terms.
*Correspondence: Jinchi Zhang, emhhbmc4ODExQG5qZnUuZWR1LmNu; emhhbmdqYzg4MTFAZ21haWwuY29t
†These authors have contributed equally to this work