Standards and Best Practices for Monitoring and Benchmarking Insects
- 1Department of Ecology and Evolutionary Biology, University of California, Los Angeles, Los Angeles, CA, United States
- 2Florida Museum of Natural History, University of Florida, Gainesville, FL, United States
- 3Department of Biology, University of Florida, Gainesville, FL, United States
Benchmark studies of insect populations are increasingly relevant and needed amid accelerating concern about insect trends in the Anthropocene. The growing recognition that insect populations may be in decline has given rise to a renewed call for insect population monitoring by scientists, and a desire from the broader public to participate in insect surveys. However, due to the immense diversity of insects and a vast assortment of data collection methods, there is a general lack of standardization in insect monitoring methods, such that a sudden and unplanned expansion of data collection may fail to meet its ecological potential or conservation needs without a coordinated focus on standards and best practices. To begin to address this problem, we provide simple guidelines for maximizing return on proven inventory methods that will provide insect benchmarking data suitable for a variety of ecological responses, including occurrence and distribution, phenology, abundance and biomass, and diversity and species composition. To track these responses, we present seven primary insect sampling methods—malaise trapping, light trapping, pan trapping, pitfall trappings, beating sheets, acoustic monitoring, and active visual surveys—and recommend standards while highlighting examples of model programs. For each method, we discuss key topics such as recommended spatial and temporal scales of sampling, important metadata to track, and degree of replication needed to produce rigorous estimates of ecological responses. We additionally suggest protocols for scalable insect monitoring, from backyards to national parks. Overall, we aim to compile a resource that can be used by diverse individuals and organizations seeking to initiate or improve insect monitoring programs in this era of rapid change.
Introduction
“The best time to plant a tree is 20 years ago. The second best time is now.” -Unattributed proverb
The threat of widespread insect declines, supported by accumulating evidence across the globe (Conrad et al., 2006; Forister et al., 2011; Hallmann et al., 2017; van Klink et al., 2020), has sparked broad and outspoken concern. But even this general pattern of insect decline is heterogeneous in time and space, and drivers of declines in particular taxa and locations remain unclear, though they are likely myriad (Fox, 2013; Wagner, 2020). To better understand insect declines in the face of data gaps and other challenges (Didham et al., 2020), researchers need more systematic and long-term monitoring of insect abundance and diversity. Though many monitoring schemes already exist, relatively few have been operating long enough to draw robust, independent conclusions about insect populations and diversity over time (e.g., Shortall et al., 2009), and these monitoring schemes are necessarily limited in their geographic and taxonomic coverage. As scientists, we can and should lament our severely limited data on insect declines—long-term monitoring efforts should have been underway long before now, but were prevented for many reasons, including a lack of funding, motivation, and organization. Given increased societal interest in insects, there is the potential for widespread, long-term monitoring at the scale necessary to benchmark and track insect trends moving forward.
Many new monitoring efforts have been recently initiated, motivated by reports of insect declines. Researchers, managers, and community scientists are currently increasing efforts to document insects, whether photographing insects at porch lights, counting pollinators on transects, or establishing structured malaise trap programs. These efforts are a crucial first step toward broadly tracking trends in insect abundance and diversity. To maximize the information gain from these largely independent efforts, we recommend integration with established insect monitoring methods to coordinate sharing data that are accessible and interoperable. As much as possible, new monitoring efforts should align methods, metadata, and data access with those that already exist to increase explanatory power, streamline analysis, and facilitate the development of a global insect monitoring network. This network is already beginning to form through the efforts of organizations like PollardBase (Taron and Ries, 2015), the National Moth Recording Scheme (Fox et al., 2011), the Global Malaise Program (Geiger et al., 2016), as well as regional efforts, taxon-specific programs (e.g., for monarch butterflies and lady beetles), and even groups of Twitter users organizing nights to check their porch lights for insects1. Recently, Woodard et al. (2020) took the important step of proposing a national bee monitoring network in the United States. However, the urgency of insect declines requires even more rapid development and integration in an era of purported “insect apocalypse.”
To reach the goal of a long-term monitoring network on a global scale, we will need data that, through standardization and well-defined metadata, can be integrated across monitoring efforts. Without standardized data and metadata collection, researchers will assemble datasets that are difficult or impossible to integrate, hindering synthesis. In other words, the efforts of thousands working independently are most valuable when those efforts can be assembled into a collective whole.
To meet this challenge, our aim here is to inform new monitoring projects with standardized data collection and metadata collection practices, facilitating future integration. We present a standardized toolbox for monitoring methods and metadata practices, aimed as a starting point for non-specialists and a reference point for specialists. Specifically, we provide: (1) overviews of common insect monitoring methods, including malaise, light, pan, and pitfall trapping, beating sheet, and audio and active visual surveys; (2) specific recommendations for how to carry out each method in the field; (3) an overview of metadata considerations; (4) recommendations for standardized metadata collection for each method (Table 1); and (5) a forecast of emerging methods that can complement and extend existing methods.
Our audience is anyone interested in insect monitoring, from community members motivated to contribute to science, to entomological specialists who want to make their data more broadly useful. We especially hope these recommendations will aid those interested in insect monitoring but are not sure where to start. Workers can choose monitoring methods from the toolbox we present, then modify as needed for their goals and systems. We make these recommendations with scalability in mind—the methods we discuss are generally low cost, field-tested, and can be performed by a single individual. We generally organize these methods by following the framework presented by Ferro and Summerlin (2019), while our summaries and recommendations are especially influenced by Southwood and Henderson (2000), Samways et al. (2010), and previous efforts to advocate for sampling alignment for bees (Droege et al., 2017) and birds (Ralph et al., 1993). Along these lines, we do not advocate for existing monitoring networks to change their methods even if they are not easy to integrate with other efforts. Though methodological standardization is ultimately a goal, we pragmatically advocate as much for alignment with and among existing monitoring efforts as we do for standardization.
In the following sections, we outline recommended methods for sampling a given set of insect taxa for monitoring purposes. These methods are generally suitable for a variety of key benchmarking goals, including the measurement of occurrence and distribution, phenology, abundance and biomass, and diversity and species composition (Box 1). This is not a guide for conducting exhaustive species inventories (e.g., BioBlitzes or site lists), which often emphasize maximizing species counts, nor is it a guide for maximizing insect catches. This guide also does not aim to eliminate bias—no method is free of bias, but if methods and metadata are documented carefully and are consistent over time, then bias can largely be estimated and controlled. Additionally, monitoring insects in sites with rare and endangered species also requires unique considerations that must be site- and species-specific, and we do not cover the complexities of those considerations here, nor do we go into details of how to analyze data once collected. Finally, we do not recommend these sampling methods for entomologists with highly specific taxonomic goals; the class Insecta is simply too diverse in its niches and behaviors to be comprehensively assessed by one or even a few sampling methods. Here, we attempt to create a balance between being too general or too specific, by presenting a variety of methods for sampling broad taxa and guilds, each with its own strengths and weaknesses (Figure 1). If you find yourself wanting to monitor more specific insect groups, your sampling methods may need to be modified from those discussed here or may not be covered.
Box 1. Measurement goals of benchmarking studies.
The goals of benchmarking and monitoring studies typically aim to measure change in at least one of the following: (1) occurrence and distribution, (2) phenology, (3) abundance and biomass, or (4) diversity and species composition. The seven monitoring methods we highlight can be used with the goal of measuring changes in as many of these responses as desired. Each of these four categories of response is important for different reasons, requires a different minimum spatiotemporal scale of sampling, and is currently used to study how insect populations and communities change over time.
Occurrence and distribution: Changes in occurrence and distribution are important indicators of how shifts in underlying processes affect organisms. Occurrence can also sometimes serve as proxies for abundance (Royle and Nichols, 2003). Estimating occurrence and distribution requires, at minimum, the formal identification of a taxon at a location (i.e., a presence), but the addition of data on what taxa were not present (i.e., an absence), allows for a more powerful analysis of occurrence. For insects, occurrence and distribution monitoring is perhaps the most widespread benchmarking method (e.g., Chen et al., 2009; Boyes et al., 2019; Outhwaite et al., 2019), especially for invasive species and in the context of shifting ranges due to climate change.
Phenology: Shifts in phenology can indicate changes in the factors governing the timing of insect life cycles, from temperature and precipitation patterns, to flowering periods in plants. Shifts in insect phenology can cause mismatches with other taxa in their communities, from plants to birds, and can have demographic consequences for those taxa (Visser and Gienapp, 2019). Estimating phenology typically requires, at minimum, presence data for a taxon at a location repeatedly over a short time span (i.e., a “season”), but presence and absence data together allow for stronger inference. Changes in insect phenology are poorly documented in most taxa, but recent interest in the effects of climate change has spurred a larger focus on insect phenology in monitoring efforts (Gimesi, 2012).
Abundance and biomass: Changes in abundance and biomass, both measures of ecosystem function, are important for understanding the health of the ecosystem as well as for conservation and management. Estimating abundance and biomass typically requires presence and absence data in addition to accurate counts of individuals of each taxa. This form of monitoring is historically rare but has been perhaps the most influential in spurring recent interest in insect declines (Hallmann et al., 2017; Wepprich et al., 2019).
Diversity and species composition: Changes in measures of biodiversity, such as species diversity and composition, indicate how communities respond to environmental change. Estimating diversity and species composition typically requires presence and absence data for multiple taxa in a community, with some expectation that sampling is equally likely across taxa. Monitoring insect diversity and composition is relatively common compared to abundance, biomass, and phenological monitoring (e.g., Brooks et al., 2012; Valtonen et al., 2017).
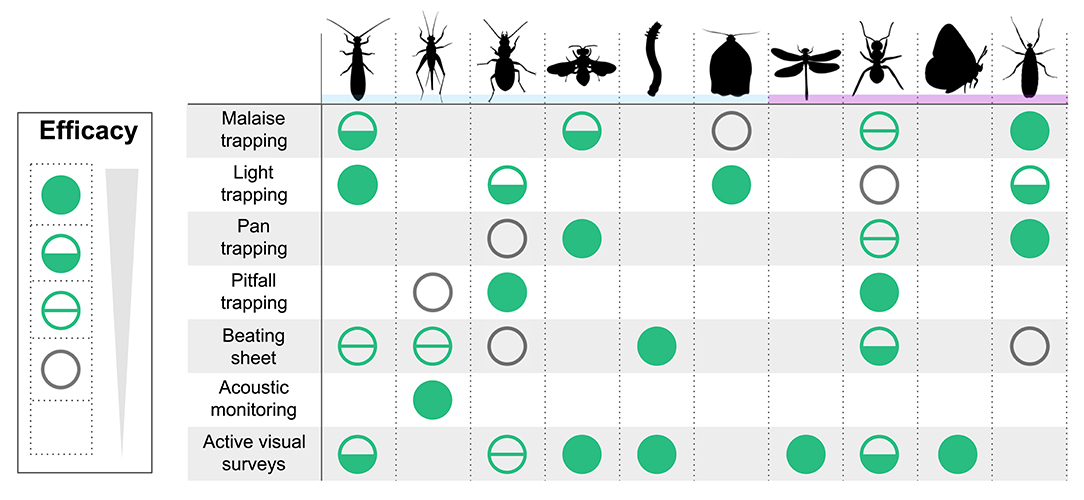
Figure 1. Commonly-monitored insect guilds and taxa and the efficacy for each of seven benchmarking methods. Efficacy of each method for a given insect group is scored as follows: filled green circles indicate optimal suitability; half-filled circles indicate possible suitability; divided, unfilled circles indicate marginal suitability; unfilled gray circles indicate bycatch only; and no circle indicates general unsuitability. Insect groupings are defined by ecological traits (blue bar) or taxonomic clades (purple bar). In order by column, insect groupings are: adult semi-aquatic insects (Plecoptera, Ephemeroptera, and Trichoptera); singing insects (Orthoptera & Hemiptera: Cicadoidea); ground-dwelling beetles (Coleoptera: Carabidae and Staphylinidae); non-lepidopteran pollinators (Hymenoptera, Diptera, Coleoptera); leaf-chewing larvae (Lepidoptera and Hymenoptera: Symphyta); night-active moths (Lepidoptera); dragonflies and damselflies (Odonata); ants (Hymenoptera: Formicidae); butterflies (Lepidoptera: Papilionoidea); and flies (Diptera).
Standardized Monitoring Practices for Different Taxa and Methods
Malaise Trapping
Overview
Malaise traps (Malaise, 1937) are large tent-like structures made of netting meant to funnel insects to a common area (Figure 2A). In essence, an insect flies into a vertical wall of netting, responds by flying upwards, then is gradually funneled by sloped netting into a collecting vial. This vial is then checked and emptied periodically over days or weeks. The Townes-type malaise trap is the most common style used, but at least four other types—Gressitt malaise, Schact malaise, Sea, land, and air malaise (SLAM), and Cornell malaise—are also in use (Matthews and Matthews, 1983; van Achterberg, 2009). For detailed accounts of history and methodology, see van Achterberg (2009). Exemplar malaise trapping programs include the School Malaise Trap Program in Canada (Steinke et al., 2017) and the Swedish Malaise Trap Program (Karlsson et al., 2020). For those interested in joining an existing network, the Global Malaise Trap Program/BIOSCAN (Geiger et al., 2016) is accepting new members.
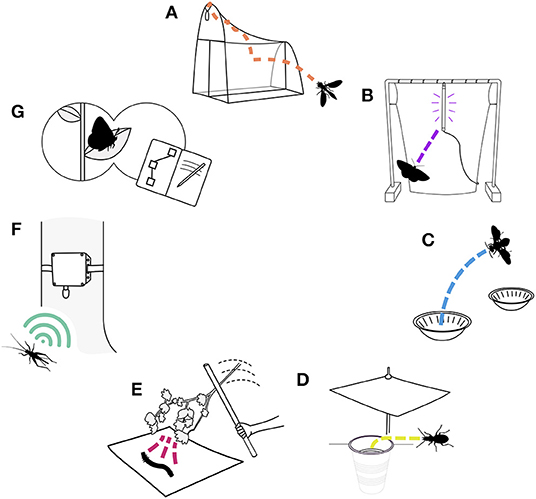
Figure 2. A visual overview of the seven insect benchmarking methods summarized here: (A) malaise trapping, (B) light trapping, (C) pan trapping, (D) pitfall trapping, (E) beating sheet, (F) acoustic monitoring, and (G) active visual surveys.
Taxonomic Considerations
Malaise trapping is only appropriate for monitoring flying insects (Figure 1). Many flies (Diptera) and some wasps, flying ants, bees (Hymenoptera), bugs (Hemiptera), moths (Lepidoptera), and semi-aquatic taxa are effectively sampled by malaise traps (Matthews and Matthews, 1970; Noyes, 1989; Campbell and Hanula, 2007; Fraser et al., 2008; Mazon and Bordera, 2008; Diserud et al., 2013; Schmidt et al., 2019). Within these groups, malaise trapping is especially appropriate for Tenthredinidae, Ichneumonoidea, Scelionidae, Mymaridae, and other hymenopterans with similar life histories, as well as Cicadellidae and Cercopidae (Hemiptera), microlepidopterans (Lepidoptera), and the semi-aquatic orders Plecoptera, Ephemeroptera, and Trichoptera—if traps are placed alongside aquatic habitats. It is important to note that malaise trap efficacy for a taxon can depend on habitat. For example, bees (Apoidea) are sampled well in some habitats, like tallgrass prairie (Geroff et al., 2014), but pan-trapping is generally more effective for sampling this superfamily (Campbell and Hanula, 2007). The narrower the taxon of interest, the more necessary it is to customize these recommendations to your own system.
Methodological Considerations
Location
Spatial placement is extremely important for malaise trapping; it is critical to document the trap's exact position and microhabitat by photograph, written description (particularly for habitat), and precise coordinates (see Table 1 for additional metadata needed). Choice of placement will vary based on the particular taxon of interest, but malaise traps should generally be placed along natural flight corridors (e.g., streams or gaps between bushes) to maximize catch. Amount of wind exposure should also be recorded, as wind can limit efficacy. The vertical panel of netting (i.e., the interception area) that insects hit should be oriented to be perpendicular to the expected movement corridor.
Design
We recommend Townes-type malaise traps due to their broad efficacy and already widespread use. Multiple sizes are available, with a 165 × 110 cm interception area being most common. The vertical wall of netting is commonly black to reduce visibility, while the dome of the tent is white to increase insects' propensity to fly upwards (i.e., toward “the sky”), which increases catch. We recommend 95% ethanol (as is used by BIOSCAN) as a killing agent that preserves DNA, but lower concentrations (down to 80%) can be used if evaporation or cost prohibits use of 95%. Alternative approaches to collection include cyanide (hazardous to humans), ethyl acetate (destroys DNA), and live collecting (needs to be checked daily, and specimens are often damaged). Ethanol, however, does remove scales from taxa like lepidopterans. Mesh size and shape are also important to note: holes that are too wide are less effective at sampling small flies, wasps, and other microfauna, but large mesh sizes can better sample groups like stinging hymenopterans (Darling and Packer, 1988). Some insects, such as many beetles, drop after hitting the mesh screen, rather than trying to escape by flying higher. To take advantage of this, pans with collecting liquid can be placed underneath the mesh wall, creating what is called a flight-intercept trap (traditional malaise traps are a specialized flight-intercept trap that only samples upward-moving insects). If this is done, pan color should be recorded.
Scalability
Commercial malaise traps can be expensive (usually > US $230). Costs can be reduced by constructing home-made malaise traps (e.g., Blackmon, 2010). Mosquito netting can be used for the interception area (Lamarre et al., 2012), but mesh size and shape should be considered. Setup effort is generally low (up to 1 h the first time). Sampling effort is minimal once a malaise trap is set up (10 min per week once at the site): the sample vial simply needs to be emptied into a storage vial. Post-sampling effort can be high, however, since malaise trapping can yield large numbers of insects (e.g., up to 10,000 specimens per week depending on the site). DNA metabarcoding of the sample is a faster post-sampling identification method for measuring diversity (though abundance is lost), and non-destructive methods to sample from fixative fluids show promise (Marquina et al., 2019; Nielsen et al., 2019; Zizka et al., 2019). Although metabarcoding lowers the time costs off post-sampling identification, metabarcoding does come with increased costs related to DNA sequencing and additional genetic expertise is needed. See Hausmann et al. (2020) for a recent example of a malaise trap study employing metabarcoding. Finally, because malaise traps are sensitive to microhabitat variation—like most stationary sampling methods—multiple traps at a site are better than a single trap.
Light Trapping
Overview
Light traps are one of the most common and efficient methods for surveying insect that fly at night. At their most basic, light traps simply consist of a light attractant and a viewing surface, often a bedsheet (Figure 2B). More structured light traps commonly consist of a funnel, vanes (which deflect insects toward the funnel), and a collection container, which together are used in conjunction with the light source to form a structured trap. In either case, light-attracted insects fly toward the light source, hit a surface or vanes surrounding the light, and can then be observed and recorded or sampled and collected. Common styles of vaned light traps include Robinson traps and Heath traps (Macgregor et al., 2017). Light traps provide an opportunity to gather standardized and comparable data, but many factors influence the abundance and composition of light trapped insects, including trap type, season, time of day, lunar phase, duration of sampling, and light attractant (Jonason et al., 2014). Consequently, these details are all important to track (Table 1). Mercury vapor bulbs are the most commonly used attractant and have consistently caught a higher abundance and diversity of insects than other standard bulbs due to the powerful low-wavelength light emitted (Jonason et al., 2014; White et al., 2016). Other commonly used bulb types include UV, metal halide, and LED (Ferro and Summerlin, 2019). Although many commercial light traps are available and can be deployed in remote locations, light trapping can be as simple as documenting the moths that are attracted to your porch light. Individuals interested in joining the Discover Life's Mothing project can join a network of people working to photograph and identify moths that come to their porch light (Pickering, 2015). Exemplar long term light-trapping programs include the Hungarian Light-trap Network (Szentkirályi, 2002) and the Rothamsted Insect Survey (Macgregor et al., 2019), both of which have been surveying phototactic insects for over 50 years.
Taxonomic Considerations
Light trapping is appropriate for monitoring phototactic (i.e., light-attracted) night-flying insects in both terrestrial and aquatic habitats and is used for surveying a wide range of insect taxa (Figure 1), including flies (Diptera), true bugs (Hemiptera), beetles (Coleoptera), caddisflies (Trichoptera), parasitic wasps (Hymenoptera, and moths (Lepidoptera), among other groups. Light trapping is especially appropriate for moths (Lepidoptera) (Macgregor et al., 2019), caddisflies (Trichoptera) (Waringer, 2003), and many beetle taxa (Coleoptera) (Liu et al., 2007).
Methodological Considerations
Location
Spatial placement is extremely important for light trapping. Although light traps can attract insects from the surrounding environment, insects are rarely attracted at distances >30 meters (Truxa and Fiedler, 2012). Therefore, the microhabitat of the trap location will influence what organisms are trapped, making it important to describe the trap location in field notes and record the precise coordinates of the trap location (Table 1). Light pollution can decrease the flight-to-light behavior of moth populations (Altermatt and Ebert, 2016), so light sensors (low quality light sensors are available as smart phone apps) should be used to note the lumens/m2 of light pollution at the trap location.
Design
The wavelength and brightness of light attractants differs dramatically among different light bulbs and are important to consider when designing light trapping projects. If the goal is to sample the greatest abundance and diversity of insects, then we recommend using mercury vapor bulbs, as these are consistently found to attract the most moths (Jonason et al., 2014; White et al., 2016). However, mercury vapor traps may not be the optimal tool because of their cost and the logistics required to deploy them (e.g., an outlet or automotive battery is needed). Therefore, low-cost, light-weight, and easy to deploy light traps offer convenient alternatives and facilitate insect trapping at more sites and in more diverse settings (White et al., 2016). If light traps can be checked early in the morning, insects can be trapped alive by having traps lined with egg cartons to provide areas for the insects to hide (Jonason et al., 2014). Live specimens can be photographed in the field or later in the lab, after cooling in a refrigerator and then released the following night (Ford et al., 2020). If observation alone is undesirable, light trap containers can be lined with pest strips (18.6% dichlorvos [2,2-dichlorovinyl dimethyl phosphate]) or filled with ethanol.
Scalability
Commercial light traps can be expensive (between US $75 and US $500). Additionally, commercial light traps often require outlets or car batteries, making carrying them into remote locations challenging. Homemade light traps can be inexpensively constructed and can greatly reduce weight by running efficient LED strips using small 12V batteries (White et al., 2016). Effort required for field sampling can be high if insects are live trapped and identified in the field or transferred to jars to be photographed. If insects are lethally trapped, sampling effort can be low, with just a few minutes spent setting the light trap each sampling event and a few minutes spent collecting the specimens the following morning. However, post processing costs can be high, as light traps can yield a high diversity and abundance of insects. Technological advances in “smart light traps,” where insects attracted to lights are automatically photographed throughout the night, offer great promise to increase the scalability of light trap surveys (Hogeweg et al., 2019).
Pan Trapping
Overview
Pan traps (Moericke, 1951) are trays filled with liquid set out to collect insects. Pan traps often rely on color as an attractant and are effective primarily because insects mistake them for food resources. An insect flies to a pan, attempts to land, then becomes trapped in the liquid solution—often soapy water, propylene glycol, or saline (Figure 2). Pan traps can be made from nearly any object that holds liquid—i.e., a disposable plate filled with water and a few drops of dish soap—and this accessibility has made them more popular than more training-intensive methods that may sample more diversity (e.g., standardized sweep-netting; Cane et al., 2000). Like all sampling methods, there is no doubt that pan traps have considerable sampling bias for certain taxa (Portman et al., 2020). For detailed accounts of history and methodology, see Droege et al. (2017), LeBuhn et al. (2003), Vrdoljak and Samways (2012), and Southwood and Henderson (2000). An exemplar pan trap monitoring networks is the UK Pollinator Monitoring Scheme, and a data-recording scheme designed for bee monitoring can be found in LeBuhn et al. (2003).
Taxonomic Considerations
Pan trapping is appropriate for monitoring flying insects (Figure 1C). It is effective at sampling aphids (Hemiptera), thrips (Thysanoptera), bees and parasitic wasps (Hymenoptera), flies (Diptera), some beetles (Coleoptera), and even some grasshoppers (Orthoptera) (Evans and Bailey, 1993; Westphal et al., 2008; Vrdoljak and Samways, 2012). Trap efficacy for each taxon varies strongly with pan color (Vrdoljak and Samways, 2012). Yellow is most commonly used, as yellow traps often collect the largest catches and highest total insect diversity, but other common colors include blue, white, red, and green. As with other monitoring methods, habitat and geographic region can affect the trap efficacy for a given group (Vrdoljak and Samways, 2012; Saunders and Luck, 2013). For those with broad taxonomic interests for their monitoring programs, we recommend what has become a common standard: yellow pan traps in conjunction with white and blue pan traps (Vrdoljak and Samways, 2012; Sircom et al., 2018), as is done in the UK Pollinator Monitoring Scheme. If needed, traps can be painted using colors defined by the Bee Inventory Plot program (LeBuhn et al., 2003).
Methodological Considerations
Location
Pan traps are typically placed in open areas where they can be seen by target insects. Traps can be placed together as close as 5 m—the minimum distance at which they do not influence each other (Droege et al., 2017). Although a large diversity of spatial arrangements exist, we recommend one of two methods. The first method, used by the UK Pollinator Monitoring Scheme, places 1 trap per square km, and is suitable for sampling large geographic regions. The second method, from the Bee Inventory Plot (LeBuhn et al., 2003), uses 15 traps in a single array, each separated by 5 m and placed in two perpendicular lines forming an “X.” This arrangement is suitable for targeted monitoring, with two arrays (30 traps) demonstrated as being adequate for sampling local bee diversity (Shapiro et al., 2014).
Design
For discussion of pan trap color, see Taxonomic considerations. Pan traps can be placed on the ground (most common), elevated above the ground, or placed flush with the substrate (i.e., essentially modified pitfall traps). Elevated pan traps sometimes yield larger numbers of specimens (Tuell and Isaacs, 2009), and pan traps flush with the ground can also attract ground-dwelling species (Ernst et al., 2016). Trap size may not affect catch (Gonzalez et al., 2020), so small pan traps are desirable to minimize costs; circular pans with a 7 cm diameter are common. In arid areas, the trap solution may evaporate too quickly between visits, so larger pan traps can be used, for example, 2-gallon buckets. The amount of liquid in a trap can affect trap efficacy and should be recorded when setting up and checking traps. We recommend premixing the liquid solution recipe of LeBuhn et al. (2003): 1 part dish soap to 750 parts water (approximately 1 teaspoon soap for a gallon of water).
Because pan color is the main attractant, it is important to maintain trap color through frequent cleaning and eventual trap replacement when color fades. Scented water like rose water can be used to increase catches for some taxa (Laubertie et al., 2006) but since maximizing the number of individuals caught is not necessarily a goal of standardized monitoring, we recommend against using scents or baits for benchmarking. Small amounts of preservative chemicals can be added to prevent fungal growth when the time between visits is necessarily long, but chemical safety precautions should be taken.
Scalability
Pan traps are low cost but require frequent (often daily) trap visits and maintenance. For an estimated time budget for a 24-pan transect, see Droege et al. (2017). Specimen processing times can be high depending on target taxon abundance. A typical pan in a field could yield only a few specimens over 24 h, but even with low abundances, numbers can rise quickly if using multiple traps over long time periods. Sieving with nylon mesh (e.g., an aquarium net) is a common practice that speeds up specimen processing, but can damage small, fragile taxa such as aphids.
Pitfall Trapping
Overview
Pitfall traps (Hertz, 1927) are containers placed flush with ground level to capture ground-dwelling (epigeic) insects. In essence, an insect walks to the trap edge, loses balance, and falls in (Figure 2D). The container is then checked, the catch collected or documented, and reset. Several recent reviews have discussed pitfall trapping (Skvarla et al., 2014; Brown and Matthews, 2016; Hohbein and Conway, 2018), and standardized traps have been proposed by Brown and Matthews (2016). Like any insect sampling method, pitfall traps produce taxonomically biased samples (Topping and Sunderland, 1992; Lang, 2000), but are inexpensive and popular for monitoring. For detailed methodological accounts, see Southwood and Henderson (2000), Brown and Matthews (2016), and Hoekman et al. (2017). Existing pitfall trap monitoring networks include the US National Ecological Observatory Network (NEON; Hoekman et al., 2017), and the UK Environmental Change Network (Brooks et al., 2012).
Taxonomic Considerations
Pitfall traps are most appropriate for sampling ground-dwelling beetles (Coleoptera)—especially Carabidae and Staphylinidae—and ants (Hymenoptera: Formicidae) (Baars, 1979; Skvarla et al., 2014). They may incidentally collect flying taxa, especially if the trap is roofless and white or yellow (Buchholz et al., 2013), but are not an effective sampling method for most other groups (Figure 1).
Methodological Considerations
Location
Pitfall traps can be placed nearly anywhere with suitable substrate for digging. There is some controversy over how far apart traps should be placed; some studies have found that traps provide independent samples even when only 1 m apart (Ward et al., 2001), while others recommend 10 m (Hohbein and Conway, 2018). NEON, which conducts standardized trapping across North American sites, separates traps by at least 25 m. Digweed et al. (1995) found that population depletion occurs when traps are separated by 10 m or less, but not if separated by distances of <25 m. Until there is more consensus, a 25 m distance between traps should be adequate to ensure independence of samples.
Design
Pitfall traps can be made of glass, plastic, or metal, but disposable plastic cups have become perhaps the most widespread trap container. The container should be placed with its lip flush with the soil surface. As might be expected, the diameter of the trap affects catch (Abensperg-Traun and Steven, 1995). Collecting fluid should generally be used to avoid damage to specimens from other trapped insects and to prevent escapes, but the type of collecting fluid used can affect the taxa attracted (Skvarla et al., 2014). Ethylene glycol has been traditionally used but is toxic for wildlife if consumed and can be easily substituted with propylene glycol, which we recommend for most uses. Propylene glycol evaporates more slowly than ethanol and adequately preserves most DNA, at least over the short-term (Nakamura et al., 2020). Traps with baits will be more readily disturbed by vertebrates (Vandenberghe, 1992), and should be avoided. Fences, or guidance barriers that direct insects toward the trap, can increase catch (Boetzl et al., 2018) but require more effort to set up. Pitfall color affects taxonomic composition of the catch (Buchholz et al., 2013) and should be recorded; we recommend using transparent containers as described in Brown and Matthews (2016). Using funnels increases catch efficiency while simultaneously reducing vertebrate bycatch (Radawiec and Aleksandrowicz, 2013), although low roofs also reduce vertebrate bycatch (Hoekman et al., 2017). Roofs also prevent rain from diluting the collecting fluid and appear to not influence the composition or magnitude of insect catch (Buchholz and Hannig, 2013). Containers should be nested to allow fast and easy removal of samples. Disturbance from trap placement can affect catch, so a latent period of 1–2 weeks before trap monitoring begins should be observed if possible (Greenslade, 1973).
Calls for pitfall trapping standardization have a longer history than other monitoring methods (Brown and Matthews, 2016), and we recommend alignment with existing programs. Given the broad extent of NEON, we recommend that new monitoring programs (at least those in North America) adopt the NEON pitfall trapping protocol when practical (Hoekman et al., 2017). This protocol involves nested clear plastic cups (diameter: 11 cm, depth: 7 cm, volume: 473 mL) and a roof made of hard plastic raised 1.5 cm above the trap entrance. Each trap is filled with 150 mL of an equal ratio of propylene glycol to distilled water. Traps are placed in arrays of 4, arranged in a square with sides 25 m long.
Scalability
Pitfall trapping using disposable plastic cups is relatively cheap and easily scalable. Set-up can be labor intensive depending on the design (and the inclusion of fences), but under the NEON protocol is limited to simply digging an appropriately sized hole and placing the trap and roof. Checking traps is also a low time commitment, especially when using a nested cup design, which allows for easy removal.
Beating Sheets
Overview
A beating or beat sheet is a piece of fabric supported by a frame, which is placed below a substrate of interest (e.g., a tree branch). An insect rests or feeds on the substrate, which is then shaken or hit (“beat”), dislodging the insect so that it falls on the sheet where it can be collected or recorded (Figure 2E). This active sampling method is often used in conjunction with an aspirator to suck up fast-moving taxa. Currently, the most common design is two pieces of wood or PVC pipe forming an “X,” with a piece of white fabric (e.g., bedsheet) stretched behind. Alternative designs include simply placing a sheet on the ground, or even using an umbrella. Beating sheets are cheap, easy to build, and straightforward to use. Insects can be recorded visually or collected for further identification depending on project goals. The Caterpillars Count! citizen science program (Hurlbert et al., 2019) is an example of a beating sheet monitoring network in North America.
Taxonomic Considerations
Beating sheets are appropriate for sampling tree and shrub dwelling insects, such as caterpillars (Lepidoptera), some true bugs (e.g., aphids and scale insects; Hemiptera), some beetles (Coleoptera), and other plant-feeding insects (Figure 1). It is not a good method for sampling flying insects; they will often fly away when the branch is hit, or hit the sheet, then quickly escape.
Methodological Considerations
Location
Beating sheets can be used anywhere vegetation is found for beating: typically shrubs and trees, but also groundcover in some cases.
Design
The standard size and shape for a beating sheet is a square with sides of about 90 cm (3 feet), using two pieces of PVC or wood 1.3 m (51 inches) long for crossbars. A cloth can then be stapled or glued to the crossbars. White cloth should be used to maximize visibility of insects that fall on the sheet. The object used for hitting the substrate can vary, but dimensions should be recorded; a stick of about 2.5 cm (1 inch) diameter and 60 cm (2 feet) long works well. The surveyor should strongly hit the branch 10 times, but not so strongly that the plant is damaged. Because many insect species have some degree of host specificity, substrate type (e.g., tree species) strongly predicts insect species diversity and abundance. This will affect sampling decisions depending on your goals; sampling plants of only one species or of multiple species are both reasonable. Either way, we strongly recommend always recording the plant species (Table 1). If collecting specimens, using an aspirator helps capture fast-moving or flying insects.
Scalability
A simple beating sheet can be constructed in <15 min using materials that cost < US $102. As an active sampling method, using beating sheets can be more time consuming than passive methods, but usually not prohibitively so. Sampling a substrate and collecting the specimens can take <5 min. Visual surveys can be substituted for specimen collection to reduce time spent on post-sampling identification, but at the likely cost of taxonomic resolution. Only one beating sheet is needed per person sampling. Some research suggests that three plants of the same species is the minimum necessary to accurately estimate insect abundance (Harris et al., 1972).
Acoustic Monitoring
Overview
Acoustic monitors provide a passive, non-destructive method to detect and identify insects (Ganchev et al., 2007; Mankin et al., 2011). Insects may generate bioacoustic signals as a means of communication (Penone et al., 2013), or as a by-product of locomotion (Kawakita and Ichikawa, 2019). These bioacoustic signals may be captured as sounds with microphones or as vibrations with contact sensors (Figure 2F). Although contact and ultrasonic sensors have been successful in detecting insect pests that live inside agricultural products (Mankin et al., 2011), we focus here on acoustic recording units and their use for surveying the relative abundance and diversity of insects. Many factors influence the efficacy of acoustic devices in identifying and estimating density of insects, including the frequency range, substrate (air or water), type of sensor, the size and behavior of the insect, and the distance between the insects and the sensors (Gibb et al., 2019). These factors should be considered and noted when conducting surveys using acoustic monitoring. Large-scale acoustic monitoring has been successfully coordinated by the French National Museum of Natural History to assess the impacts of anthropogenic stressors on Orthoptera communities (Penone et al., 2013; Jeliazkov et al., 2016).
Taxonomic Considerations
Acoustic monitoring is an appropriate method for monitoring insects that use sounds or vibrations in communications. Some of these noises, such as cicada and cricket songs can be detected over long distances. If the bioacoustic signal produced by insects follows a consistent species-specific pattern, it can be extracted from background noise for identification purposes (Ganchev et al., 2007). Therefore, passive acoustic monitoring is particularly well-suited for loud terrestrial insects such as Orthoptera or Cicadoidea because they produce species-specific mating calls (Penone et al., 2013) (Figure 1), but may also be useful for a variety of other insects including bees (Galen et al., 2019; Kawakita and Ichikawa, 2019) and aquatic Hemiptera (Desjonquères et al., 2020; Gottesman et al., 2020).
Methodological Considerations
Location
The distance at which acoustic signals can be detectable above ambient noise varies depending on the sound's amplitude and frequency, landscape heterogeneity such as topography and vegetation, and weather (Gibb et al., 2019). Additionally, anthropogenic sounds or sounds from other animals can mask target sounds. The precise GPS coordinate of where static sensors are deployed must be recorded and potential sources of sound pollution should be noted (Table 1). Bioacoustic devices can also be used while traveling along transects, but we recommend keeping bioacoustic devices in fixed locations to collect data that is easier to standardize across sites and replicate across visits.
Design
Commercially available acoustic monitors can be flexibly programed to collect acoustic signals and on-board metadata for long intervals across a variety of sampling regimes (Hill et al., 2019). The use of inexpensive components (e.g., microelectromechanical systems microphones) may decrease financial barriers to initiating multisensor surveys but can lower data quality by having lower signal-to-noise ratios and inconsistent frequency response (Gibb et al., 2019). Critical to successful acoustic surveys is the development of efficient pipelines to process sound files and output annotated data. Manually annotating data is time consuming and can be biased by the analyst's knowledge level. Developing automated machine learning pipelines to process individual sound files—which can each include more than 10 min of ambient sound recording—can both increase the efficiency of data processing while also making data processing more reproducible and interoperable.
Scalability
Recent advances in custom built electronics and the lowering costs of small but usable microphones provide novel opportunities to monitor select insect taxa across greater spatial and temporal scales. Passive acoustic monitors can automatically collect data over long periods (e.g., a month), with minimal maintenance needed to replace batteries and digital memory cards (Hill et al., 2019). Typically, they are programmed to record periodically during a window of interest. Recent developments in customizable acoustic devices have dramatically lowered costs closer to US $50 (Hill et al., 2018). Developing automatic identification pipelines using machine learning algorithms is critical to scaling acoustic monitoring and discussed further in section looking forward.
Active Visual Surveys
Overview
Visual surveys are commonly used to document the abundance and diversity of insects that can easily be visually identified in the field, often with the aid of close-focus binoculars and nets (Figure 2G). These surveys typically involve researchers documenting the presence of a species or counting the total number of individuals of each species observed during a standardized survey. The most frequently used methods include (1) transects, (2) point counts, and (3) area counts. Although mark/recapture is another frequently used visual survey technique to document insect population dynamics, we do not consider that a viable benchmarking technique as it takes enormous effort and would not be tractable to do simultaneously for large numbers of insect species.
All three of the commonly used methods have extensive histories of standardized protocols. Transect counts use visual identification while searching along predefined transects with specified search distances. Pollard walks are a commonly used transect method used in butterfly research, in which an observer visualizes a box that extends 5 m ahead and 5 m to the sides as they walk a transect counting butterflies (Pollard, 1977). Point counts, where an observer stands still and identifies and counts the number of individuals of the target taxa around them during a set period of time, provides an alternative to transects in sites that are difficult to walk in or where habitats are fragile or at-risk (Henry et al., 2015). Distance sampling techniques, where observers note their distance from the observed insect, can be implemented with both transects and point counts to estimate densities (Isaac et al., 2011; Henry et al., 2015). By incorporating imperfect detection, distance sampling allows for density and absolute population size to be estimated in closed populations (Buckland, 2001). Area counts, such as the North American Butterfly Association's count circle (Taron and Ries, 2015), consist of surveyors counting each species within a delimited study plot during a certain time period. Insects that are challenging to identify quickly or in flight can be netted and transferred into vials and placed in a cooler to chill until the end of the survey period (Loffland et al., 2017). Photos of chilled individuals can then be taken for later identification before releasing these individuals. Exemplar visual survey programs include multiple butterfly monitoring schemes (BMS) such as the UK BMS, the Dutch BMS (Schmucki et al., 2016), and the Ohio Lepidopterists BMS (Wepprich et al., 2019).
Taxonomic Considerations
Visual surveys are only appropriate for large insects that can be easily detected and identified or photographed in the field. Butterflies (Papilionoidea), dragonflies and damselflies (Odonata), and large bees (Apidae) such as bumblebees are effectively sampled using visual surveys (Figure 1). When species cannot be identified, individuals can be netted and then identified or photographed (Loffland et al., 2017; Holtmann et al., 2018). However, not all species can be identified in the field; for some species, microscopic examination of the genitalia and abdominal appendages is necessary for identification. Visual surveys generally focus on generating a complete list of species observed (with or without counts), and therefore, visual surveys must focus on a select target group of insects (e.g., butterflies or bumblebees).
Methodological Considerations
Location
The location of the visual survey is important as many insects are habitat specialists. After selecting sites for visual surveys, multiple transects, points, or areas should be randomly selected to ensure sampling across the heterogeneity of a site. It is important to document the coordinates of the survey location and note the habitat type. Visual surveys occurring in difficult terrain or in at-risk ecosystems may consider choosing point counts to limit trampling or allow for more flexible walking routes.
Design
Care should be taken when selecting the location of visual surveys, as we recommend these locations remain fixed to enable surveys to be compared from year to year. Transect surveys should be at least 1 km in length, although visual survey methods allow for the correction of survey effort by adjusting by the length of the transect or by time of survey (Taron and Ries, 2015). Thus, detailed information must be documented on the length of transects and the start and end times of surveys (Table 1). Consistent and repeated surveys are needed to capture the seasonal abundance of individual species and to fully capture the diversity of the community. Therefore, surveys should begin before the first adult individuals of the target group are presumed to be active and terminate after the final adult activity. During this period of activity, visual surveys are recommended to occur weekly when conditions meet the time of day and weather criteria suggested by Pollard and Yates (1993). Surveys should occur between the midday hours of 1,000 and 1,700 when air temperature exceeds 13°C (although this may be reduced to 11°C in polar, upland areas) and there is at least 60% sun or 17°C in any conditions, providing it is not raining and wind speeds are below a six on the Beaufort scale (Pollard and Yates, 1993).
Scalability
Visual surveys can require extensive field effort with the potential of a single survey taking multiple hours complete. Due to the field effort required to complete visual surveys across numerous sites, many successful visual survey programs rely on the dedication of numerous trained volunteers (Schmucki et al., 2016; Wepprich et al., 2019). Critical to the success of visual surveys is a rigid observer training protocol, as untrained observers tend to have biased distance estimates and observer experience can significantly affect detection functions (Buckland, 2001). Visual surveys generally have limited post-sampling effort, with the main effort being transcribing field notes and data collection sheets. This process can be further enhanced by using GPS handheld tablets to record data in the field (Hackett et al., 2019).
General Methodological Considerations
Replication
Four types of replication are especially important when sampling: spatial, inter-annual, intra-annual, and within-sample replication. All types of replication are important and broaden the inferential scale of any monitoring program while also expanding the analytical options and flexibility. We caution, however, that when sampling lethally, large-scale replication (particularly, spatially and temporally intense sampling at a local scale) could theoretically lead to abundance declines, especially perhaps in rare taxa (Minteer et al., 2014, but see Gezon et al., 2015). Lethal sampling should only be done when scientifically and ethically justified (Drinkwater et al., 2019).
The required amount of spatial replication for accurate and precise monitoring is still unknown for many insect monitoring methods, and contentious for others. We provide specific discussion in each method section, but in general, the more spatial replication, the more accurate and precise the estimates of measured responses. One way to increase the degree of spatial replication without increasing individual effort is to join an existing monitoring network, thereby increasing the network's degree of spatial replication.
A high degree of inter-annual replication is important for monitoring of most taxa (Wauchope et al., 2019), but is especially important for measuring insect abundance, where large year-to-year fluctuations are common (Didham et al., 2020). Failure to account for high inter-annual variability has led to disagreements over whether some insect populations are truly declining or not (see Willig et al., 2019 in response to Lister and Garcia, 2018).
A high degree of intra-annual replication is also important because insect phenology is complex and variable. For example, the week of peak abundance for a species one year could be different the next year, and even the number of generations produced by a species can vary across years. Even if only interested in studying occurrence or abundance, intra-annual variation in phenology of insect activity and generations can lead to strong biases in these responses if samples are only collected once per year (i.e., the “groundhog effect”; Didham et al., 2020). To account for this, we strongly recommend that monitoring efforts be carried out for the entire season of activity for the taxon of interest, allowing easier comparison across years.
Finally, within-sample replication—that is, multiple samples of a response at a single site during a period when the occurrence and abundance of target taxa are assumed to be constant—can be important for the statistical analysis of trends when trying to account for imperfect detection of individuals and species, such as with occupancy modeling (MacKenzie et al., 2006). Within-sample replication can be achieved either through spatial sub-samples—for example, using “array” designs, as discussed above for pitfall and pan traps—or temporal sub-samples—such as conducting visual or acoustic surveys at the same location multiple days in a row. In general, the ability to collect within-sample replication of monitoring data depends on the design and scalability of a chosen method. Although within-sample replication can substantially increase effort of monitoring, the ability of increased samples to account for sampling noise can be extremely powerful when detection probabilities of target taxa are low. For recent examples of occupancy modeling using some means of sampling replication for insects, see Isaac et al. (2014), Loffland et al. (2017), Outhwaite et al. (2019), Szewczyk and McCain (2019), and Powney et al. (2019).
Curation of Specimens
Many of the monitoring methods discussed require collecting insect specimens for subsequent identification. After identification, it is generally up to the scientist whether specimens should be kept or discarded, although vouchering of representative taxa can be key to the long-term value of datasets, particularly given taxonomic revisions and new technologies. To that end, great care should be taken to ensure specimens are preserved properly, if kept. For an overview of insect specimen storage, see Heraty et al. (2020). It is becoming increasingly common to preserve specimens in 80–95% ethanol to better preserve DNA. Regardless of the preservation method, it is worth developing a plan for deposition and cataloging of specimens prior to beginning a monitoring project. If discussed ahead of time with university and museum insect curators or collection managers, specimens that are properly preserved and curated should be donated. Optimally, archival deposition should include all survey notes, along with specimens, to enhance long-term re-use value.
Curation of Data and Metadata
Just like physical specimens, data are valuable resources for future science. Just as important as data are metadata, that is, the collective information about how the monitoring data were collected. Each method of insect monitoring has its own unique set of critical metadata (Table 1). Monitoring programs should strive to meet FAIR data principles to ensure their data and metadata are: findable, accessible, interoperable, and reusable (Wilkinson et al., 2016). Findable and accessible data will require that collected data are digitized and uploaded to websites or online databases that are constructed to hold data about the collecting method. There is much work on this topic that needs to be done, but some resources are developing rapidly.
Interoperable data may be the most challenging aspect of FAIR principles, given that each monitoring program often develops its own reporting standards, even for the same monitoring methods, which ultimately places the burden on downstream users to reintegrate, often with loss of key information. Recent efforts have called for unified, global monitoring standards, such as the Humboldt Core metadata standard (Guralnick et al., 2018). While it is unlikely that one data standard will fit all insect monitoring, the Humboldt Core provides a typology of different survey and inventorying processes, such as restricted or open searches, along with key definitions of taxonomic, spatial, and temporal scopes, that strongly aid in discovery of monitoring datasets. More specific metadata describing particulars of different monitoring schemes can and should be accommodated (Table 1). We argue that rather than assume an improbable utopia of full data integration, monitoring programs should work in federations and take seriously the production of detailed metadata, and, as much as possible, develop standardization for metadata that can be as easily linked as possible into existing frameworks. The end result will be FAIR data that can most easily be integrated into flexible modeling frameworks that allow statistical integration of well-described data to better answer broad-scale ecological questions.
As with specimens, it is critical that a plan for data management be considered prior to beginning a monitoring project. Data standards are a key part of that plan since monitoring metadata is crucial for generating insights from monitoring outcomes. However, other factors are also critical, including developing local data storage solutions, deciding on a longer-term repository for data, assuring appropriate credit models for those involved with data collection, and licensing and use agreements of data products. Each of these issues deserves its own longer contribution and we point readers to Michener (2015) and Hardisty et al. (2019) for further reading. Here we make two broad recommendations. First, we strongly suggest development of a coherent data storage and sharing plan that has community buy-in. A best-case approach is development of an internal content management system that provides tools for data access and curation for program participants along with a broad, coherent, and multipronged, data sharing policy that assures long-term access. One part of this sharing policy should focus on best practices for archiving data in community repositories such as Zenodo and Dryad. A second part should focus on publication to aggregators that specialize in biodiversity data mobilization, such as the Global Biodiversity Information Facility (GBIF). The value of publishing to GBIF is enhanced discoverability, since it acts as a single, global access point to biodiversity data and information. However, it can still be challenging to properly publish all survey metadata given GBIF's reliance on standards that were built for incidental records (Guralnick et al., 2018). Finally, we also encourage monitoring programs to explicitly state data collection and review policies, including how individuals within the programs are credited for the work they do. Such credit models may include attribution for use of data, which can be supported by both licensing mechanisms such as creative commons licenses, and data use policies.
We also encourage development of digital tools to support the capture of field data. Digital tools (e.g., phone apps) can limit transcription errors and are sometimes easier to manage in the field. However, physical data sheets still play an important role in most monitoring programs and are a reliable backup over the very long-term. For these, archival-quality paper and ink should be used to maximize longevity. Though the cost and effort are not trivial, undigitized data can be digitized increasingly easily via scanning and optical character recognition (OCR) capture. Finally, the entoGEM project3 (Grames et al., 2019) is soliciting unpublished insect abundance and diversity time series for inclusion in a global systematic map and meta-analysis. EntoGEM is a database, not a repository, but can serve as a temporary mechanism for archiving until a suitable repository is found and is a way to increase the utility of your data.
Looking Forward
Traditional survey methods are limited by being labor and time intensive, but ecological monitoring of animals has recently undergone a dramatic transformation with the development of technologies that expand the spatial, temporal, and taxonomic scales possible to monitor biodiversity (Pimm et al., 2015). These technological advances could facilitate the collection and availability of vast quantities of data by reducing the effort and expense of insect monitoring. No single method will be able to monitor multiple different insect groups across diverse landscapes. However, a combination of emerging technologies in surveying methods, processing, and data sharing pipelines will allow insect trends to be extracted at currently unprecedented scales.
We are entering an era where passive automated monitoring is already augmenting the traditional methods discussed above. Passive acoustic monitoring using arrays of acoustic sensors are already being deployed and tested. Such new methods have enormous promise, but also produce enormous volumes of data. A single acoustic recording unit can easily generate hundreds of gigabytes of data, with much of the data consisting of non-target (i.e., non-insect) sounds. Algorithms to automatically locate and identify target sounds within audio recordings are being developed (Gibb et al., 2019), and machine learning approaches can substantially improve detection and classification accuracies by discriminating spectro-temporal information directly from annotated spectrograms. These algorithms have been demonstrated to outperform alternative detection and classification methods in a variety of settings (Fairbrass et al., 2019). Unfortunately, one of the greatest barriers to detecting and classifying species using passive acoustic monitoring is the limited availability of expert-verified sound databases for reference and training data (Gibb et al., 2019). This problem may be especially exacerbated for insects, given their vast diversity, the paucity of audio libraries, and that only 5% of published terrestrial acoustic monitoring research has been on invertebrates (Sugai et al., 2019).
Camera traps are another emerging surveying tool that are being used to monitor a variety of wildlife (Burton et al., 2015). Networks of many camera traps allow for data to be collected across greater spatial and temporal scales (Kissling et al., 2018). Like with acoustic monitoring, deep learning convolutional neural networks are being developed to automatically count and identify wildlife (Norouzzadeh et al., 2018). The relatively small size of insects compared to wildlife typically captured using camera traps provides unique challenges to monitoring insects with cameras. However, recent studies have shown the potential of camera traps to monitor the overall abundance of flying nocturnal insects (Ruczyński et al., 2020). Additionally, a portable computer vision light trap has been developed to attract and identify live moths (Bjerge et al., 2020), and a monitoring network of camera traps that are made with smart image processing has been proposed to monitor light-attracted insects in the Netherlands (Hogeweg et al., 2019). Continued effort into developing camera traps designed to monitor insects has great potential for passive surveying of non-acoustically detected insects at greater spatiotemporal scales.
Environmental metabarcoding is an emerging tool that provides rapid and cost-effective means for taxonomic identification of many organisms in terrestrial and aquatic environments (Piper et al., 2019). These approaches can provide detection/non-detection data for insects collected from a variety of methods, especially if specimens are stored in 80–95% ethanol. Metabarcoding insect feces (e.g., frass) offer another non-lethal surveying tool, as caterpillars have been identified to species by amplifying larval DNA from caterpillar feces (Rytkönen et al., 2019). Unfortunately, most insects have insufficient reference sequences in public archives such as GenBank, making genetically identifying insects challenging. Still, environmental metabarcoding provides a cost-effective and efficient option to identify insects collected in large quantities.
Radar can also create standardized monitoring data for insects at broad spatial and temporal scales (Didham et al., 2020). Filtering insects from meteorological data can provide previously unused datasets to monitor insects through time and have been used to document the decline of burrowing mayflies (Hexagenia spp.) across North America (Stepanian et al., 2020). In most cases, species-level identification cannot be accomplished using radar approaches, but specialized entomological radar shows promise in monitoring insects that may migrate in large abundances at heights difficult to monitor using traditional approaches.
New methods do not need to work in isolation nor are they replacements for traditional monitoring methods. Rather, these new approaches are ways to augment existing ones, and to lower costs for onerous activities that may be partially or wholly automated. We envision passive monitoring tools that can be deployed in conjunction with traditional trap or restricted search methods. For example, acoustic monitors and camera-loaded light traps could be controlled by one device and augment, for example, pitfall trapping, to capture a broader spectrum of insects at a single site. If these sensor approaches also have means to easily share data across a network of sensors and people, it may speed up necessary steps to create the most usable data resources for broad-scale insect monitoring.
Conclusion
We live in an era of rapid change that affects nearly all life on Earth. We can only understand this change and its effects on insects by pooling effort, integrating projects, and working together. Methods standardization is a relatively simple first step, but what challenges come next? For one, we urgently have to coordinate our use of these tools. Networks of networks need to be built for data collection. This means incentivizing participation, coordinating new and existing projects, and organizing efforts on a trans-national scale. Large networks should communicate with each other to increase complementarity, and smaller networks should seek to fit in with what is already being done while maximizing the utility of their own data. But coordination is likely not the greatest challenge.
The largest bottleneck for insect monitoring is getting from trap to accessible data—we need to accelerate the time-consuming stage spent processing and identifying specimens and build tools for the efficient capture of all data and metadata associated with an observation. Improving identification is an area where we have immense potential for advancement over the near term. Bringing more automation to this stage will result in much shorter lag times between data collection and analysis and increase scalability of new and existing projects. Tools for automating identification include metabarcoding, computer vision, and machine learning. Efficient expansion of these identification tools will not only facilitate the broader participation of individuals in insect monitoring (e.g., those without specific skills in taxonomy), but the digital nature of automated and semi-automated identification will speed up data accessibility and metadata capture.
We also need analytical advances for integrating data collected by multiple sampling methods. Assimilating data collected using the same benchmarking method into a composite database is the first step, but ultimately, integrating data collected by multiple different means will vastly improve our ability to understand the broader insect community. But even if we arrive at the point where all the necessary data are being collected on a global scale, the best data in the world are useless if they are not made available—where availability means in a digital format that adheres to all of the FAIR principles. Consequently, we also need the infrastructure to aggregate, store, and share data widely–existing databases such as GBIF are paving the way—while also recognizing the importance of attributing credit (e.g., Chavan and Penev, 2011) to incentivize participation in the process of infrastructure development and data curation.
Every one of these challenges will require collective action to overcome. In the face of rapid Anthropogenic change, there is an intense urgency to this effort. These are no small tasks, and the timeline for completing them is short. Benchmarking has no point when there is nothing left to benchmark. Appropriate foresight and funding would have developed large-scale insect monitoring long ago, but the second best option is to rapidly build capacity now.
Author Contributions
GM and MT conceived the idea for the manuscript and proposed the initial outline. GM and MB led writing of the first draft. All authors contributed critically to drafts and gave final approval for publication.
Funding
This research was supported by the US National Science Foundation (Grants EF 1703048 and 2033263) to MT and RG. Additional support was provided to GM through the UCLA Department of Ecology and Evolutionary Biology and to MB through a University of Florida Biodiversity Institute Fellowship.
Conflict of Interest
The authors declare that the research was conducted in the absence of any commercial or financial relationships that could be construed as a potential conflict of interest.
Acknowledgments
We thank David Wagner for providing useful comments that significantly improved the manuscript, as well as the valued input of two reviewers.
Footnotes
References
Abensperg-Traun, M., and Steven, D. (1995). The effects of pitfall trap diameter on ant species richness (Hymenoptera: Formicidae) and species composition of the catch in a semi-arid eucalypt woodland. Aust. J. Ecol. 20, 282–287. doi: 10.1111/j.1442-9993.1995.tb00540.x
Altermatt, F., and Ebert, D. (2016). Reduced flight-to-light behaviour of moth populations exposed to long-term urban light pollution. Biol. Lett. 12:20160111. doi: 10.1098/rsbl.2016.0111
Baars, M. A. (1979). Catches in pitfall traps in relation to mean densities of carabid beetles. Oecologia 41, 25–46. doi: 10.1007/BF00344835
Bjerge, K., Nielsen, J. B., Videbaek Sepstrup, M., Helsing-Nielsen, F., and Høye, T. T. (2020). A light trap and computer vision system to detect and classify live moths (Lepidoptera) using tracking and deep learning. Ecology. doi: 10.1101/2020.03.18.996447
Blackmon, H. (2010). DIY Malaise Net for $69.00. Available online at: http://coleoguy.blogspot.com/2010/04/diy-malaise-net-for-6900.html (accessed 30 June, 2020).
Boetzl, F. A., Ries, E., Schneider, G., and Krauss, J. (2018). It's a matter of design—how pitfall trap design affects trap samples and possible predictions. PeerJ. 6:e5078. doi: 10.7717/peerj.5078
Boyes, D. H., Fox, R., Shortall, C. R., and Whittaker, R. J. (2019). Bucking the trend: the diversity of Anthropocene ‘winners’ among British moths. Front. Biogeogr. 11:3862. doi: 10.21425/F5FBG43862
Brooks, D. R., Bater, J. E., Clark, S. J., Monteith, D. T., Andrews, C., Corbett, S. J., et al. (2012). Large carabid beetle declines in a United Kingdom monitoring network increases evidence for a widespread loss in insect biodiversity. J. Appl. Ecol. 49, 1009–1019. doi: 10.1111/j.1365-2664.2012.02194.x
Brown, G. R., and Matthews, I. M. (2016). A review of extensive variation in the design of pitfall traps and a proposal for a standard pitfall trap design for monitoring ground-active arthropod biodiversity. Ecol. Evol. 6, 3953–3964. doi: 10.1002/ece3.2176
Buchholz, S., and Hannig, K. (2013). Do covers influence the capture efficiency of pitfall traps? EJE 106, 667–671. doi: 10.14411/eje.2009.083
Buchholz, S., Jess, A.-M., Hertenstein, F., and Schirmel, J. (2013). Effect of the colour of pitfall traps on their capture efficiency of carabid beetles (Coleoptera: Carabidae), spiders (Araneae) and other arthropods. EJE 107, 277–280. doi: 10.14411/eje.2010.036
Buckland, S. T. (2001). Introduction to Distance Sampling: Estimating Abundance of Biological Populations. Oxford University Press.
Burton, A. C., Neilson, E., Moreira, D., Ladle, A., Steenweg, R., Fisher, J. T., et al. (2015). REVIEW: Wildlife camera trapping: a review and recommendations for linking surveys to ecological processes. J. Appl. Ecol. 52, 675–685. doi: 10.1111/1365-2664.12432
Campbell, J. W., and Hanula, J. L. (2007). Efficiency of Malaise traps and colored pan traps for collecting flower visiting insects from three forested ecosystems. J. Insect Conserv. 11, 399–408. doi: 10.1007/s10841-006-9055-4
Cane, J. H., Minckley, R. L., and Kervin, L. J. (2000). Sampling bees (Hymenoptera: Apiformes) for pollinator community studies: pitfalls of pan-trapping. J. Kans. Entomol. Soc. 73, 225–231.
Chavan, V., and Penev, L. (2011). The data paper: a mechanism to incentivize data publishing in biodiversity science. BMC Bioinformat. 12”S2. doi: 10.1186/1471-2105-12-S15-S2
Chen, I.-C., Shiu, H.-J., Benedick, S., Holloway, J. D., Chey, V. K., Barlow, H. S., et al. (2009). Elevation increases in moth assemblages over 42 years on a tropical mountain. Proc. Natl. Acad. Sci. U.S.A. 106, 1479–1483. doi: 10.1073/pnas.0809320106
Conrad, K. F., Warren, M. S., Fox, R., Parsons, M. S., and Woiwod, I. P. (2006). Rapid declines of common, widespread British moths provide evidence of an insect biodiversity crisis. Biol. Conserv. 132, 279–291. doi: 10.1016/j.biocon.2006.04.020
Darling, D. C., and Packer, L. (1988). Effectiveness of Malaise traps in collecting Hymenoptera: the influence of trap design, mesh size, and location. Can. Entomol. 120, 787–796. doi: 10.4039/Ent120787-8
Desjonquères, C., Rybak, F., Ulloa, J. S., Kempf, A., Hen, A. B., and Sueur, J. (2020). Monitoring the acoustic activity of an aquatic insect population in relation to temperature, vegetation and noise. Freshw. Biol. 65, 107–116. doi: 10.1111/fwb.13171
Didham, R. K., Basset, Y., Collins, C. M., Leather, S. R., Littlewood, N. A., Menz, M. H. M., et al. (2020). Interpreting insect declines: seven challenges and a way forward. Insect Conserv. Divers. 13, 103–114. doi: 10.1111/icad.12408
Digweed, S. C., Currie, C. R., Carcamo, H. A., and Spence, J. R. (1995). Digging out the “digging-in effect” of pitfall traps: influences of depletion and disturbance on catches of ground beetles (Coleoptera: Carabidae). Pedobiologia 39, 561–576.
Diserud, O. H., Stur, E., and Aagaard, K. (2013). How reliable are Malaise traps for biomonitoring? – A bivariate species abundance model evaluation using alpine Chironomidae (Diptera). Insect Conserv. Divers. 6, 561–571. doi: 10.1111/icad.12012
Drinkwater, E., Robinson, E. J. H., and Hart, A. G. (2019). Keeping invertebrate research ethical in a landscape of shifting public opinion. Methods Ecol. Evolut. 10, 1265–1273. doi: 10.1111/2041-210X.13208
Droege, S., Engler, J. D., Sellers, E. A., and O'Brien, L. (2017). U.S. National protocol framework for the inventory and monitoring of bees, Version 2.0. Inventory Monit. Natl. Wildl. Refuge Syst. US Fish Wildl. Serv. Fort Collins CO.
Ernst, C. M., Loboda, S., and Buddle, C. M. (2016). Capturing northern biodiversity: diversity of arctic, subarctic and north boreal beetles and spiders are affected by trap type and habitat. Insect Conserv. Divers. 9, 63–73. doi: 10.1111/icad.12143
Evans, E. W., and Bailey, K. W. (1993). Sampling Grasshoppers (Orthoptera: Acrididae) in Utah Grasslands: Pan Trapping versus Sweep Sampling. J. Kans. Entomol. Soc. 66, 214–222.
Fairbrass, A. J., Firman, M., Williams, C., Brostow, G. J., Titheridge, H., and Jones, K. E. (2019). CityNet—Deep learning tools for urban ecoacoustic assessment. Methods Ecol. Evol. 10, 186–197. doi: 10.1111/2041-210X.13114
Ferro, M. L., and Summerlin, M. (2019). Developing a standardized list of entomological collection methods for use in databases. ZooKeys 861, 145–156. doi: 10.3897/zookeys.861.32347
Ford, S., Kidd, P., Nash, K., and Rietveld, A. (2020). Artificial light and moth biodiversity: a comparison of moth diversity across different habitats on Lundy to investigate the effect of artificial light. J. Lundy Field Soc. 7, 53–68
Forister, M. L., Jahner, J. P., Casner, K. L., Wilson, J. S., and Shapiro, A. M. (2011). The race is not to the swift: long-term data reveal pervasive declines in California's low-elevation butterfly fauna. Ecology 92, 2222–2235. doi: 10.1890/11-0382.1
Fox, R. (2013). The decline of moths in Great Britain: a review of possible causes: the decline of moths in Great Britain. Insect Conserv. Divers. 6, 5–19. doi: 10.1111/j.1752-4598.2012.00186.x
Fox, R., Randle, Z., Hill, L., Anders, S., Wiffen, L., and Parsons, M. S. (2011). Moths count: recording moths for conservation in the UK. J. Insect Conserv. 15, 55–68. doi: 10.1007/s10841-010-9309-z
Fraser, S. E. M., Dytham, C., and Mayhew, P. J. (2008). The effectiveness and optimal use of Malaise traps for monitoring parasitoid wasps. Insect Conserv. Divers. 1, 22–31. doi: 10.1111/j.1752-4598.2007.00003.x
Galen, C., Miller, Z., Lynn, A., Axe, M., Holden, S., Storks, L., et al. (2019). Pollination on the dark side: acoustic monitoring reveals impacts of a total solar eclipse on flight behavior and activity schedule of foraging bees. Ann. Entomol. Soc. Am. 112, 20–26. doi: 10.1093/aesa/say035
Ganchev, T., Potamitis, I., and Fakotakis, N. (2007). Acoustic Monitoring of Singing Insects. In: 2007 IEEE International Conference on Acoustics, Speech and Signal Processing - ICASSP'07, IV-721-IV−724. doi: 10.1109/ICASSP.2007.367014
Geiger, M., Moriniere, J., Hausmann, A., Haszprunar, G., Wägele, W., Hebert, P., et al. (2016). Testing the global malaise trap program – how well does the current barcode reference library identify flying insects in Germany? Biodivers. Data J. 4:e10671. doi: 10.3897/BDJ.4.e10671
Geroff, R. K., Gibbs, J., and McCravy, K. W. (2014). Assessing bee (Hymenoptera: Apoidea) diversity of an Illinois restored tallgrass prairie: methodology and conservation considerations. J. Insect Conserv. 18, 951–964. doi: 10.1007/s10841-014-9703-z
Gezon, Z. J., Wyman, E. S., Ascher, J. S., Inouye, D. W., and Irwin, R. E. (2015). The effect of repeated, lethal sampling on wild bee abundance and diversity. Methods Ecol. Evolution 6, 1044–1054. doi: 10.1111/2041-210X.12375
Gibb, R., Browning, E., Glover-Kapfer, P., and Jones, K. E. (2019). Emerging opportunities and challenges for passive acoustics in ecological assessment and monitoring. Methods Ecol. Evol. 10, 169–185. doi: 10.1111/2041-210X.13101
Gimesi, L. (2012). The effect of climate change on the phenology of moth abundance and diversity. Appl. Ecol. Environ. Res. 10, 349–363. doi: 10.15666/aeer/1003_349363
Gonzalez, V. H., Osborn, A. L., Brown, E. R., Pavlick, C. R., Enríquez, E., Tscheulin, T., et al. (2020). Effect of pan trap size on the diversity of sampled bees and abundance of bycatch. J. Insect Conserv. 24, 409–420. doi: 10.1007/s10841-020-00224-4
Gottesman, B. L., Francomano, D., Zhao, Z., Bellisario, K., Ghadiri, M., Broadhead, T., et al. (2020). Acoustic monitoring reveals diversity and surprising dynamics in tropical freshwater soundscapes. Freshw. Biol. 65, 117–132. doi: 10.1111/fwb.13096
Grames, E., Montgomery, G. A., Haddaway, N. R., Dicks, L. V., Elphick, C. S., Matson, T. A., et al. (2019). Trends in Global Insect Abundance and Biodiversity: A Community-Driven Systematic Map Protocol. OSF.
Greenslade, P. J. M. (1973). Sampling ants with pitfall traps: digging-in effects. Insectes Sociaux 20, 343–353. doi: 10.1007/BF02226087
Guralnick, R., Walls, R., and Jetz, W. (2018). Humboldt Core – toward a standardized capture of biological inventories for biodiversity monitoring, modeling and assessment. Ecography 41, 713–725. doi: 10.1111/ecog.02942
Hackett, R. A., Belitz, M. W., Gilbert, E. E., and Monfils, A. K. (2019). A data management workflow of biodiversity data from the field to data users. Appl. Plant Sci. 7:e11310. doi: 10.1002/aps3.11310
Hallmann, C. A., Sorg, M., Jongejans, E., Siepel, H., Hofland, N., Schwan, H., et al. (2017). More than 75 percent decline over 27 years in total flying insect biomass in protected areas. PLoS ONE 12:e0185809. doi: 10.1371/journal.pone.0185809
Hardisty, A. R., Michener, W. K., Agosti, D., Alonso García, E., Bastin, L., Belbin, L., et al. (2019). The Bari Manifesto: an interoperability framework for essential biodiversity variables. Ecol. Inform. 49, 22–31. doi: 10.1016/j.ecoinf.2018.11.003
Harris, J. W. E., Collis, D. G., and Magar, K. M. (1972). Evaluation of the tree-beating method for sampling defoliating forest insects. Can. Entomol. 104, 723–729. doi: 10.4039/Ent104723-5
Hausmann, A., Segerer, A. H., Greifenstein, T., Knubben, J., Morinière, J., Bozicevic, V., et al. (2020). Toward a standardized quantitative and qualitative insect monitoring scheme. Ecol. Evol. 10, 4009–4020. doi: 10.1002/ece3.6166
Henry, E. H., Haddad, N. M., Wilson, J., Hughes, P., and Gardner, B. (2015). Point-count methods to monitor butterfly populations when traditional methods fail: a case study with Miami blue butterfly. J. Insect Conserv. 19, 519–529. doi: 10.1007/s10841-015-9773-6
Heraty, J., Yanega, D., and Triapitsyn, S. (2020). Museum Specimen Preparation Guidelines (incl. Vouchers). Available online at: https://entmuseum.ucr.edu/specimen_preparation/index.html (accessed June 30, 2020).
Hill, A. P., Prince, P., Covarrubias, E. P., Doncaster, C. P., Snaddon, J. L., and Rogers, A. (2018). AudioMoth: evaluation of a smart open acoustic device for monitoring biodiversity and the environment. Methods Ecol. Evol. 9, 1199–1211. doi: 10.1111/2041-210X.12955
Hill, A. P., Prince, P., Snaddon, J. L., Doncaster, C. P., and Rogers, A. (2019). AudioMoth: a low-cost acoustic device for monitoring biodiversity and the environment. HardwareX 6:e00073. doi: 10.1016/j.ohx.2019.e00073
Hoekman, D., LeVan, K. E., Ball, G. E., Browne, R. A., Davidson, R. L., Erwin, T. L., et al. (2017). Design for ground beetle abundance and diversity sampling within the National Ecological Observatory Network. Ecosphere 8:e01744. doi: 10.1002/ecs2.1744
Hogeweg, L., Zeegers, T., Katramados, I., and Jongejans, E. (2019). Smart Insect Cameras. Biodivers. Inf. Sci. Stand. 3:e39241. doi: 10.3897/biss.3.39241
Hohbein, R. R., and Conway, C. J. (2018). Pitfall traps: a review of methods for estimating arthropod abundance. Wildl. Soc. Bull. 42, 597–606. doi: 10.1002/wsb.928
Holtmann, L., Juchem, M., Brüggeshemke, J., Möhlmeyer, A., and Fartmann, T. (2018). Stormwater ponds promote dragonfly (Odonata) species richness and density in urban areas. Ecol. Eng. 118, 1–11. doi: 10.1016/j.ecoleng.2017.12.028
Hurlbert, A., Hayes, T., McKinnon, T., and Goforth, C. (2019). Caterpillars Count! A citizen science project for monitoring foliage arthropod abundance and phenology. Citiz. Sci. Theory Pract. 4:1. doi: 10.5334/cstp.148
Isaac, N. J. B., Cruickshanks, K. L., Weddle, A. M., Rowcliffe, J. M., Brereton, T. M., Dennis, R. L. H., et al. (2011). Distance sampling and the challenge of monitoring butterfly populations. Methods Ecol. Evol. 2, 585–594. doi: 10.1111/j.2041-210X.2011.00109.x
Isaac, N. J. B., van Strien, A. J., August, T. A., de Zeeuw, M. P., and Roy, D. B. (2014). Statistics for citizen science: extracting signals of change from noisy ecological data. Methods Ecol Evol. 5, 1052–1060. doi: 10.1111/2041-210X.12254
Jeliazkov, A., Bas, Y., Kerbiriou, C., Julien, J.-F., Penone, C., and Le Viol, I. (2016). Large-scale semi-automated acoustic monitoring allows to detect temporal decline of bush-crickets. Glob. Ecol. Conserv. 6, 208–218. doi: 10.1016/j.gecco.2016.02.008
Jonason, D., Franzén, M., and Ranius, T. (2014). Surveying moths using light traps: effects of weather and time of year. PLoS ONE 9:e92453. doi: 10.1371/journal.pone.0092453
Karlsson, D., Hartop, E., Forshage, M., Jaschof, M., and Ronquist, F. (2020). The Swedish Malaise trap project: a 15 year retrospective on a countrywide insect inventory. Biodivers. Data J. 8. Available online at: https://bdj.pensoft.net/article/47255/ (accessed June 19, 2020).
Kawakita, S., and Ichikawa, K. (2019). Automated classification of bees and hornet using acoustic analysis of their flight sounds. Apidologie 50, 71–79. doi: 10.1007/s13592-018-0619-6
Kissling, W. D., Ahumada, J. A., Bowser, A., Fernandez, M., Fernández, N., García, E. A., et al. (2018). Building essential biodiversity variables (EBVs) of species distribution and abundance at a global scale. Biol. Rev. 93, 600–625. doi: 10.1111/brv.12359
Lamarre, G., Molto, Q., Fine, P., and Baraloto, C. (2012). A comparison of two common flight interception traps to survey tropical arthropods. ZooKeys 216, 43–55. doi: 10.3897/zookeys.216.3332
Lang, A. (2000). The pitfalls of pitfalls: a comparison of pitfall trap catches and absolute density estimates of epigeal invertebrate predators in Arable Land. Anz. Für Schädlingskunde J. Pest Sci. 73, 99–106. doi: 10.1007/BF02956438
Laubertie, E. A., Wratten, S. D., and Sedcole, J. R. (2006). The role of odour and visual cues in the pan-trap catching of hoverflies (Diptera: Syrphidae). Ann. Appl. Biol. 148, 173–178. doi: 10.1111/j.1744-7348.2006.00046.x
LeBuhn, G., Griswold, T., Minckley, R., Droege, S., Roulston, T., Cane, J., et al. (2003). A Standardized Method for Monitoring Bee Populations - The Bee Inventory (BI) Plot.
Lister, B. C., and Garcia, A. (2018). Climate-driven declines in arthropod abundance restructure a rainforest food web. Proc. Natl. Acad. Sci. U.S.A. 115, E10397–E10406. doi: 10.1073/pnas.1722477115
Liu, Y., Axmacher, J. C., Li, L., Wang, C., and Yu, Z. (2007). Ground beetle (Coleoptera: Carabidae) inventories: a comparison of light and pitfall trapping. Bull. Entomol. Res. 97, 577–583. doi: 10.1017/S0007485307005299
Loffland, H. L., Polasik, J. S., Tingley, M. W., Elsey, E. A., Loffland, C., Lebuhn, G., et al. (2017). Bumble bee use of post-fire chaparral in the central Sierra Nevada. J. Wildl. Manag. 81, 1084–1097. doi: 10.1002/jwmg.21280
Macgregor, C. J., Evans, D. M., Fox, R., and Pocock, M. J. O. (2017). The dark side of street lighting: impacts on moths and evidence for the disruption of nocturnal pollen transport. Glob. Change Biol. 23, 697–707. doi: 10.1111/gcb.13371
Macgregor, C. J., Williams, J. H., Bell, J. R., and Thomas, C. D. (2019). Moth biomass increases and decreases over 50 years in Britain. Nat. Ecol. Evol. 3, 1645–1649. doi: 10.1038/s41559-019-1028-6
MacKenzie, D., Nichols, J. D., Royle, J. A., Pollock, K. H., Bailey, L., and Hines, J. E. (2006). Occupancy Estimation and Modeling: Inferring Patterns and Dynamics of Species Occurrence. 1st ed. Elsevier.
Mankin, R. W., Hagstrum, D. W., Smith, M. T., Roda, A. L., and Kairo, M. T. K. (2011). Perspective and promise: a century of insect acoustic detection and monitoring. Am. Entomol. 57, 30–44. doi: 10.1093/ae/57.1.30
Marquina, D., Esparza-Salas, R., Roslin, T., and Ronquist, F. (2019). Establishing arthropod community composition using metabarcoding: Surprising inconsistencies between soil samples and preservative ethanol and homogenate from Malaise trap catches. Mol. Ecol. Resour. 19, 1516–1530. doi: 10.1111/1755-0998.13071
Matthews, R. W., and Matthews, J. R. (1970). Malaise trap studies of flying insects in a New York mesic forest I. Ordinal composition and seasonal Abundance. J. N. Y. Entomol. Soc. 78, 52–59.
Matthews, R. W., and Matthews, J. R. (1983). Malaise traps: the Townes model catches more insects. Contrib. Am. Entomol. Inst. 20, 428–432.
Mazon, M., and Bordera, S. (2008). Effectiveness of two sampling methods used for collecting ichneumonidae (Hymenoptera) in the Cabaneros National Park (Spain). Eur. J. Entomol. 105, 879–888. doi: 10.14411/eje.2008.116
Michener, W. K. (2015). Ecological data sharing. Ecol. Inform. 29, 33–44. doi: 10.1016/j.ecoinf.2015.06.010
Minteer, B. A., Collins, J. P., Love, K. E., and Puschendorf, R. (2014). Avoiding (Re) extinction. Science. 344, 260–261. doi: 10.1126/science.1250953
Moericke (1951). Eine Farbafalle zur Kontrolle des Fluges von Blattlausen, insbesondere der Pfirsichblattlaus, Myzodes persicae (Sulz.). Nachrichtenblatt Dtsch. Pflanzenschutzdiensten 3, 23–24.
Nakamura, S., Tamura, S., Taki, H., and Shoda-Kagaya, E. (2020). Propylene glycol: a promising preservative for insects, comparable to ethanol, from trapping to DNA analysis. Entomol. Exp. Appl. 168, 158–165. doi: 10.1111/eea.12876
Nielsen, M., Gilbert, M. T. P., Pape, T., and Bohmann, K. (2019). A simplified DNA extraction protocol for unsorted bulk arthropod samples that maintains exoskeletal integrity. Environ. DNA 1, 144–154. doi: 10.1002/edn3.16
Norouzzadeh, M. S., Nguyen, A., Kosmala, M., Swanson, A., Palmer, M. S., Packer, C., et al. (2018). Automatically identifying, counting, and describing wild animals in camera-trap images with deep learning. Proc. Natl. Acad. Sci. U.S.A. 115, E5716–E5725. doi: 10.1073/pnas.1719367115
Noyes, J. S. (1989). A study of five methods of sampling Hymenoptera (Insecta) in a tropical rainforest, with special reference to the Parasitica. J. Nat. Hist. 23, 285–298. doi: 10.1080/00222938900770181
Outhwaite, C. L., Powney, G. D., August, T. A., Chandler, R. E., Rorke, S., Pescott, O. L., et al. (2019). Annual estimates of occupancy for bryophytes, lichens and invertebrates in the UK, 1970–2015. Sci. Data 6:259. doi: 10.1038/s41597-019-0269-1
Penone, C., Viol, I. L., Pellissier, V., Julien, J.-F., Bas, Y., and Kerbiriou, C. (2013). Use of large-scale acoustic monitoring to assess anthropogenic pressures on orthoptera communities. Conserv. Biol. 27, 979–987. doi: 10.1111/cobi.12083
Pickering, J. (2015). Find your dark side: invitation to join discover life's mothing project. South. Lepidopterists News 37, 205–208.
Pimm, S. L., Alibhai, S., Bergl, R., Dehgan, A., Giri, C., Jewell, Z., et al. (2015). Emerging technologies to conserve biodiversity. Trends Ecol. Evol. 30, 685–696. doi: 10.1016/j.tree.2015.08.008
Piper, A. M., Batovska, J., Cogan, N. O. I., Weiss, J., Cunningham, J. P., Rodoni, B. C., et al. (2019). Prospects and challenges of implementing DNA metabarcoding for high-throughput insect surveillance. GigaScience 8:giz092. doi: 10.1093/gigascience/giz092
Pollard, E. (1977). A method for assessing changes in the abundance of butterflies. Biol. Conserv. 12, 115–134. doi: 10.1016/0006-3207(77)90065-9
Pollard, E., and Yates, T. J. (1993). Monitoring Butterflies for Ecology and Conservation. Chapman and Hall, London, UK.
Portman, Z. M., Bruninga-Socolar, B., and Cariveau, D. P. (2020). The state of bee monitoring in the United States: a call to refocus away from bowl traps and towards more effective methods. Ann. Entomol. Soc. Am. 113, 337–342. doi: 10.1093/aesa/saaa010
Powney, G. D., Carvell, C., Edwards, M., Morris, R. K. A., Roy, H. E., Woodcock, B. A., et al. (2019). Widespread losses of pollinating insects in Britain. Nat. Commun. 10, 1018. doi: 10.1038/s41467-019-08974-9
Radawiec, B., and Aleksandrowicz, O. (2013). A modified pitfalltrap for capturing ground beetles (Coleoptera: Carabidae). Coleopt. Bull. 67, 473–480. doi: 10.1649/0010-065X-67.4.473
Ralph, C. J., Geupel, G. R., Pyle, P., Martin, T. E., and DeSante, D. F. (1993). Handbook of Field Methods for Monitoring Landbirds. Albany, CA: U.S. Department of Agriculture, Forest Service, Pacific Southwest Research Station. doi: 10.2737/PSW-GTR-144
Royle, J. A., and Nichols, J. D. (2003). Estimating abundance from repeated presence–absence data or point counts. Ecology 84, 777–790. doi: 10.1890/0012-9658(2003)084[0777:EAFRPA]2.0.CO;2
Ruczyński, I., Hałat, Z., Zegarek, M., Borowik, T., and Dechmann, D. K. N. (2020). Camera transects as a method to monitor high temporal and spatial ephemerality of flying nocturnal insects. Methods Ecol. Evol. 11, 294–302. doi: 10.1111/2041-210X.13339
Rytkönen, S., Vesterinen, E. J., Westerduin, C., Leviäkangas, T., Vatka, E., Mutanen, M., et al. (2019). From feces to data: a metabarcoding method for analyzing consumed and available prey in a bird-insect food web. Ecol. Evol. 9, 631–639. doi: 10.1002/ece3.4787
Samways, M. J., McGeoch, M. A., and New, T. R. (2010). Insect Conservation: A Handbook of Approaches and Methods. Oxford: Oxford University Press.
Saunders, M. E., and Luck, G. W. (2013). Pan trap catches of pollinator insects vary with habitat. Aust. J. Entomol. 52, 106–113. doi: 10.1111/aen.12008
Schmidt, O., Schmidt, S., Hauser, C., Hausmann, A., and Vu, L. V. (2019). Using Malaise traps for collecting Lepidoptera (Insecta), with notes on the preparation of Macrolepidoptera from ethanol. Biodivers. Data J. 7:e32192. doi: 10.3897/BDJ.7.e32192
Schmucki, R., Pe'er, G., Roy, D. B., Stefanescu, C., Swaay, C. A. M. V., Oliver, T. H., et al. (2016). A regionally informed abundance index for supporting integrative analyses across butterfly monitoring schemes. J. Appl. Ecol. 53, 501–510. doi: 10.1111/1365-2664.12561
Shapiro, L. H., Tepedino, V. J., and Minckley, R. L. (2014). Bowling for bees: optimal sample number for “bee bowl” sampling transects. J. Insect Conserv. 18, 1105–1113. doi: 10.1007/s10841-014-9720-y
Shortall, C. R., Moore, A., Smith, E., Hall, M. J., Woiwod, I. P., and Harrington, R. (2009). Long-term changes in the abundance of flying insects. Insect Conserv. Divers. 2, 251–260. doi: 10.1111/j.1752-4598.2009.00062.x
Sircom, J., Arul Jothi, G., and Pinksen, J. (2018). Monitoring bee populations: are eusocial bees attracted to different colours of pan trap than other bees? J. Insect Conserv. 22, 433–441. doi: 10.1007/s10841-018-0071-y
Skvarla, M. J., Larson, J. L., and Dowling, A. P. G. (2014). Pitfalls and preservatives: a review. J. Entomol. Soc. Ont. 145. Available at: https://journal.lib.uoguelph.ca/index.php/eso/article/view/3734 (accessed June 21, 2020).
Southwood, T. R. E., and Henderson, P. A. (2000). Ecological Methods 3rd Edn. Oxford: Blackwell Science.
Steinke, D., Breton, V., Berzitis, E., and Hebert, P. D. N. (2017). The school malaise trap program: coupling educational outreach with scientific discovery. PLoS Biol. 15:e2001829. doi: 10.1371/journal.pbio.2001829
Stepanian, P. M., Entrekin, S. A., Wainwright, C. E., Mirkovic, D., Tank, J. L., and Kelly, J. F. (2020). Declines in an abundant aquatic insect, the burrowing mayfly, across major North American waterways. Proc. Natl. Acad. Sci. U.S.A. 117, 2987–2992. doi: 10.1073/pnas.1913598117
Sugai, L. S. M., Silva, T. S. F., Ribeiro, J. W., and Llusia, D. (2019). Terrestrial passive acoustic monitoring: review and perspectives. BioScience 69, 15–25. doi: 10.1093/biosci/biy147
Szentkirályi, F. (2002). Fifty-year-long insect survey in Hungary: T. Jermy's contributions to light-trapping. Acta Zool. Acad. Sci. Hung. 1:85–105.
Szewczyk, T. M., and McCain, C. M. (2019). Disentangling elevational richness: a multi-scale hierarchical Bayesian occupancy model of Colorado ant communities. Ecography 42, 977–988. doi: 10.1111/ecog.04115
Taron, D., and Ries, L. (2015). “Butterfly monitoring for conservation,” in Butterfly Conservation in North America, ed J. C. Daniels (Springer: Dordrecht), 35–57. doi: 10.1007/978-94-017-9852-5_3
Topping, C. J., and Sunderland, K. D. (1992). Limitations to the use of pitfall traps in ecological studies exemplified by a study of spiders in a field of winter wheat. J. Appl. Ecol. 29, 485–491. doi: 10.2307/2404516
Truxa, C., and Fiedler, K. (2012). Attraction to light - from how far do moths (Lepidoptera) return to weak artificial sources of light? Eur. J. Entomol. 109, 77–84. doi: 10.14411/eje.2012.010
Tuell, J. K., and Isaacs, R. (2009). Elevated pan traps to monitor bees in flowering crop canopies. Entomol. Exp. Appl. 131, 93–98. doi: 10.1111/j.1570-7458.2009.00826.x
Valtonen, A., Hirka, A., Szocs, L., Ayres, M. P., Roininen, H., and Csóka, G. (2017). Long-term species loss and homogenization of moth communities in Central Europe. J. Anim. Ecol. 86, 730–738. doi: 10.1111/1365-2656.12687
van Achterberg, K. (2009). Can townes type malaise traps be improved? some recent developments. Entomol. Ber. 69, 129–135.
van Klink, R., Bowler, D. E., Gongalsky, K. B., Swengel, A. B., Gentile, A., and Chase, J. M. (2020). Meta-analysis reveals declines in terrestrial but increases in freshwater insect abundances. Science 368, 417–420. doi: 10.1126/science.aax9931
Visser, M. E., and Gienapp, P. (2019). Evolutionary and demographic consequences of phenological mismatches. Nat. Ecol. Evol. 3, 879–885. doi: 10.1038/s41559-019-0880-8
Vrdoljak, S. M., and Samways, M. J. (2012). Optimising coloured pan traps to survey flower visiting insects. J. Insect Conserv. 16, 345–354. doi: 10.1007/s10841-011-9420-9
Wagner, D. L. (2020). Insect declines in the anthropocene. Annu. Rev. Entomol. 65, 457–480. doi: 10.1146/annurev-ento-011019-025151
Ward, D. F., New, T. R., and Yen, A. L. (2001). Effects of pitfall trap spacing on the abundance, richness and composition of invertebrate catches. J. Insect Conserv. 5, 47–53. doi: 10.1023/A:1011317423622
Waringer, J. A. (2003). Light-trapping of Caddisflies at the Thaya (Lower Austria), a river influenced by pulsating hypolimnetic water release. Int. Rev. Hydrobiol. 88, 139–153. doi: 10.1002/iroh.200390010
Wauchope, H. S., Amano, T., Sutherland, W. J., and Johnston, A. (2019). When can we trust population trends? A method for quantifying the effects of sampling interval and duration. Methods Ecol. Evol. 10, 2067–2078. doi: 10.1111/2041-210X.13302
Wepprich, T., Adrion, J. R., Ries, L., Wiedmann, J., and Haddad, N. M. (2019). Butterfly abundance declines over 20 years of systematic monitoring in Ohio, USA. PLoS ONE 14:e0216270. doi: 10.1371/journal.pone.0216270
Westphal, C., Bommarco, R., Carré, G., Lamborn, E., Morison, N., Petanidou, T., et al. (2008). Measuring bee diversity in different European habitats and biogeographical regions. Ecol. Monogr. 78, 653–671. doi: 10.1890/07-1292.1
White, P. J. T., Glover, K., Stewart, J., and Rice, A. (2016). The technical and performance characteristics of a low-cost, simply constructed, black light moth trap. J. Insect Sci. 16:25. doi: 10.1093/jisesa/iew011
Wilkinson, M. D., Dumontier, M., Aalbersberg, I.j,., J., Appleton, G., Axton, M., et al. (2016). The FAIR guiding Principles for scientific data management and stewardship. Sci. Data 3:160018. doi: 10.1038/sdata.2016.18
Willig, M. R., Woolbright, L., Presley, S. J., Schowalter, T. D., Waide, R. B., Scalley, T. H., et al. (2019). Populations are not declining and food webs are not collapsing at the Luquillo Experimental Forest. Proc. Natl. Acad. Sci. U.S.A. 116, 12143–12144. doi: 10.1073/pnas.1820456116
Woodard, S. H., Federman, S., James, R. R., Danforth, B. N., Griswold, T. L., Inouye, D., et al. (2020). Towards a U.S. national program for monitoring native bees. Biol. Conserv. 252, 108821. doi: 10.1016/j.biocon.2020.108821
Keywords: survey, methodology, metadata, entomology, insect decline
Citation: Montgomery GA, Belitz MW, Guralnick RP and Tingley MW (2021) Standards and Best Practices for Monitoring and Benchmarking Insects. Front. Ecol. Evol. 8:579193. doi: 10.3389/fevo.2020.579193
Received: 01 July 2020; Accepted: 18 December 2020;
Published: 15 January 2021.
Edited by:
W. Douglas Robinson, Oregon State University, United StatesReviewed by:
Ek Del-Val, National Autonomous University of Mexico, MexicoKatherine LeVan, National Ecological Observatory Network, United States
Copyright © 2021 Montgomery, Belitz, Guralnick and Tingley. This is an open-access article distributed under the terms of the Creative Commons Attribution License (CC BY). The use, distribution or reproduction in other forums is permitted, provided the original author(s) and the copyright owner(s) are credited and that the original publication in this journal is cited, in accordance with accepted academic practice. No use, distribution or reproduction is permitted which does not comply with these terms.
*Correspondence: Morgan W. Tingley, mtingley@ucla.edu