- 1Hubei Field Observation and Scientific Research Stations for Water Ecosystem in Three Gorges Reservoir, China Three Gorges University, Yichang, China
- 2Pearl River Hydraulic Research Institute, Pearl River Water Resources Commission of the Ministry of Water Resources, Guangzhou, China
- 3Institute for Environmental Sciences, University of Koblenz-Landau, Landau, Germany
- 4Hubei Key Laboratory of River-lake Ecological Restoration and Algal Utilization, Hubei University of Technology, Wuhan, China
Algal blooms have been reported in some tributary bays since the initial impoundment of Three Gorges Reservoir, which has seriously affected the water ecology and drinking water safety. Hydrodynamics plays a crucial role in algae growth. The recent numerical models of hydrodynamics and water quality are effective to identify the effects of hydrodynamics on phytoplankton and find potential strategies for controlling algal blooms. In this study, the CE-QUAL-W2 model was applied to simulate the hydrodynamics and algal blooms in the Xiangxi Bay (XXB) of the Three Gorges Reservoir. The model performed well in simulating flow patterns, water temperature profile, and algal blooms. The results indicated that the hydrodynamics showed the spatial and temporal differences in the XXB. In the mouth area, the intensity and plunge depth of density currents were dynamic and characterized by a typical seasonal pattern. The transformation of density currents from interflow to overflow will provide more opportunities for vertical mixing, resulting in un-stratification and reducing of algal blooms. However, in the middle and upper areas, strong stratification and low velocity at upstream provide enough favorable conditions for the growth of algae and increase algal blooms. The simulation results revealed that the variation of mixing depth explains the spatial and temporal differences of Chl.a. It played a vital role in seasonal stratification and the dynamics of phytoplankton succession in XXB.
Introduction
The act of damming and impounding a river imposes fundamental physical changes upon the river continuum, which in turn fragments habitats (Grill et al., 2014; Winemiller et al., 2016; Anderson et al., 2018), disrupts the hydrological cycle of floodplain (Hu et al., 2008; Zeilhofer and De Moura, 2009; Guo et al., 2012), releases large amounts of methane (Lima et al., 2008; Fearnside and Pueyo, 2012; DelSontro et al., 2016), and causes eutrophication and even harmful algal blooms (Wang et al., 2013; Rafiee and Jahangirirad, 2015). The Three Gorges Reservoir (TGR) is a typical flooded river valley reservoir and the world's largest reservoir. It has produced substantial social and economic benefits in flood control, power generation, shipping, and storage of water resources for irrigation and potable water supplies, since the operation of the Three Gorges Dam (TGD) at full capacity at the end of 2008. However, it cannot be ignored that TGR has also caused new hydrodynamic conditions (Bie et al., 2013; Long et al., 2020), water temperature stratification (Long et al., 2016a; Liu et al., 2018), water eutrophication and frequent occurrence of algal blooms (Dai et al., 2010; Xu et al., 2013; Wu et al., 2017; Yang et al., 2017) in tributaries, threatening the downstream water ecology.
A large number of studies show that algal blooms in the tributaries of TGR are mostly affected by hydrodynamics (Liu et al., 2012; Xiong et al., 2013; Yang et al., 2018a), thermal stratification (Cui et al., 2012; Yang et al., 2012; Zhou et al., 2016), and nutrient loads (Zheng et al., 2009a; Yang et al., 2018b; Nwankwegu et al., 2020). From the long-term water quality monitoring in the tributaries of TGR, it was found that the nutrient levels in TGR are high and difficult to control because nitrogen and phosphorus loads in several major tributaries are mainly from non-point source pollution (Zheng et al., 2009b). In addition, it is known that bidirectional density currents are a common flow pattern in the tributaries of TGR, which are caused by the density difference due to the water temperature and the sediment concentrations, for TGR water temperature is the dominant factor (Liu et al., 2016). It is a consensus that the significant change in hydrodynamic condition is a key factor to control algal blooms by affecting the thermal stratification, nutrient transport, and the relationship between the mixing depth and euphotic depth (Ji et al., 2017; Yang et al., 2018a; Chuo et al., 2019).
It is a huge challenge and of great significance to understand the ecological effects produced by density currents in the tributaries of TGR. Compared with the expensive and time-consuming field monitoring method, it is acceptable to use a numerical model to simulate the development and progression of hydrodynamics and water quality. At the present, many authors have successfully applied the CE-QUAL-W2 model to simulate the density currents in the tributaries of TGR (Ma et al., 2015; Ji et al., 2017; Jin et al., 2019; Long et al., 2019). However, it is hardly used to simulate the hydrodynamic effects on the outbreak of algal blooms. Therefore, in this study, a two-dimensional laterally averaged hydrodynamic and ecological model (CE-QUAL-W2) was implemented to (1) simulate density currents, water temperature, and algal blooms in a typical tributary of the TGR and (2) investigate the mechanism that affect algal blooms.
Materials and Methods
Study Area
The Xiangxi River (XXR), a typical tributary in the lower reach of the Three Gorges Reservoir (32 km from the Three Gorges Dam), runs from north to south and drains a watershed whose area is about 3,099 km2. It has a total length of 97.3 km and extends from 110°25′E to 111°06′E and from 30°57′N to 31°34′N (Figure 1). After initial filling in June 2003 to a water level of 135 m, a deep riverine bay formed in the Xiangxi Bay (XXB), with the lower 24 km submerged by a backwater reach. The backwater of the XXR reach extended to 30 and 40 km when the TGR was filled to a water level (156 m) and a normal water level (175 m), respectively.
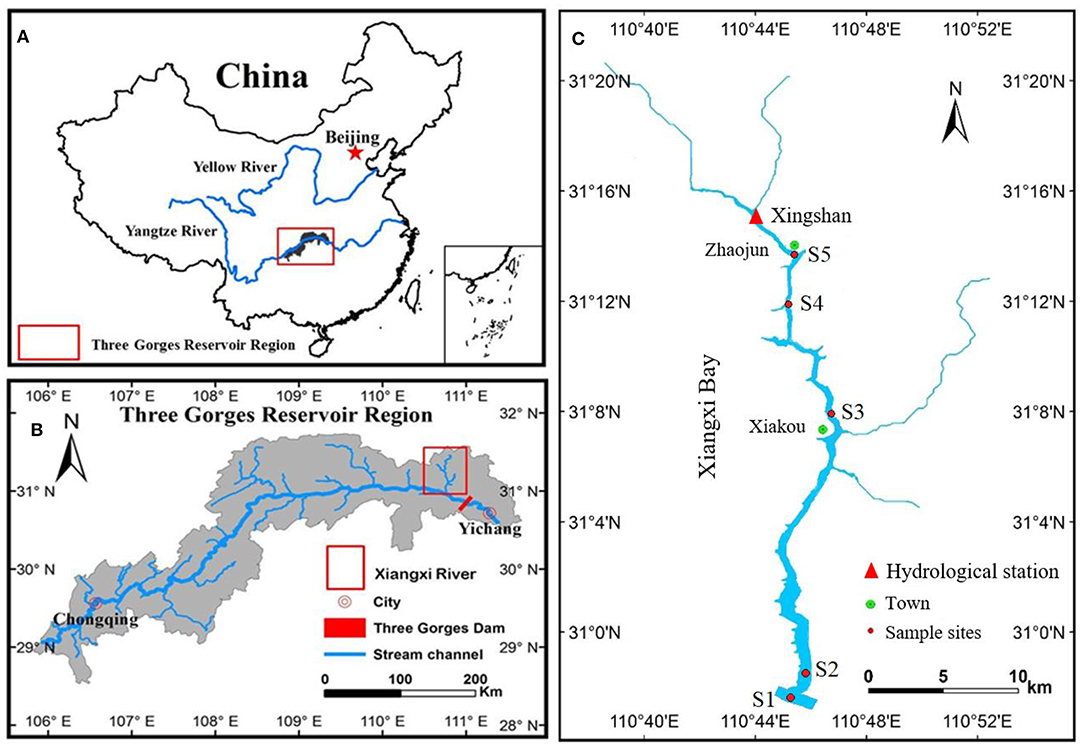
Figure 1. (A) Location of the Three Gorges Reservoir (TGR) in China; (B) location of the Xiangxi Bay (XXB) in the TGR outlined in red and its drainage system; and (C) location of the sampling sites in the XXB.
Model Application
CE-QUAL-W2 is a two-dimensional, longitudinal/vertical, hydrodynamic, and water quality model, and it is best suited for relatively long and narrow waterbodies exhibiting longitudinal and vertical water quality gradients under the assumption that lateral gradients are negligible (Wells, 2019). It is a coupled model system in which a hydrodynamic module is combined with a water quality module. The model has been successfully and widely applied to rivers, lakes, reservoirs, estuaries, and combinations thereof including entire river basins with multiple reservoirs and river segments (Berger and Wells, 2008; Afshar et al., 2011, 2013; Park et al., 2014; Zou et al., 2014; Noori et al., 2015; Glubt, 2017). The CE-QUAL-W2 model was selected for this study because it can well-reproduce the dominant density currents and water temperature profiles in the tributaries of the TGR (Ma et al., 2015; Long et al., 2016b, 2019; Ji et al., 2017).
Model Design and Data Collection
This study covers a total length of 30.7 km, and five sampling sites (S1~S5) were set from the mouth of the Xiangxi River to the vicinity of Zhaojun town (Figure 1C). Field data in this study were collected from January 2010 to December 2011, and the model was used to input files, calibration, and validation. The outputs in 2012 are used to analyze the spatiotemporal dynamics of hydrodynamics, stratification, and algal blooms. The hydrodynamic and algal modeling required the following input data:
① Bathymetry data. The computational grid of the XXB developed from bathymetric and geometric data was 500 m in length and 1 m in thickness, with a total of 64 longitudinal segments and 109 vertical layers. The accuracy of the bathymetry data was confirmed by comparing the observed and simulated storage water elevation curves.
② The meteorological parameters (i.e., air temperatures, cloud cover, wind, direct solar radiation, et al.). Daily meteorological data were available from measurements obtained from a hydrological station at Xingshan (Figure 1C), approximately 36 km upstream from the confluence of XXB and the Yangtze River.
③ Upstream inflow temperature, discharge, and water quality. Daily upstream inflow rates and weekly inflow temperature data were obtained from Xingshan station. Data gaps for water temperature were determined by statistical regression equations of water temperature and air temperature (Ma et al., 2015). Surface water samples at S5 were collected for water quality parameters. Monitored water quality variables included phosphate phosphorus (PO4-P), nitrate nitrogen (NO3-N), ammonium nitrogen (NH4-N), dissolved silicate (D-Si), dissolved oxygen (DO), chlorophyll a (Chl.a), and phytoplankton species and biomass.
④ Downstream temperature and water quality profile. Downstream temperatures profiles (4-m vertical spacing) were collected weekly at the river mouth (S1) of the XXB using a multiprobe sensor (Hydrolab DS 5X). Monthly water samples at S1 were collected from the surface, mid-depth, and near-bottom layers for obtaining vertical profiles.
⑤ Water level data. Daily water level data were obtained from the China Three Gorges Corporation (https://www.ctg.com.cn/).
⑥ Calibration data. Monthly water temperature and velocity profiles measured at S2, S3, and S4 were used for the calibration. Surface algal density and Chl.a concentration were measured at S3 for calibration.
Model Calibration
Model performance was evaluated based on the absolute mean error (AME) and the root mean square error (RMSE) statistics. The AME provides an indication of model performance and is one measure of average error. The RMSE is statistically well-behaved and is another indicator of the average difference between observations and predictions.
Based on previous CE-QUAL-W2 model studies in XXB (Ma et al., 2015; Chuo et al., 2019; Jin et al., 2019), the most sensitive model parameters are the longitudinal eddy viscosity, longitudinal eddy diffusivity, Manning's roughness coefficient, wind sheltering coefficient, dynamic shading coefficient, the fraction of incident solar radiation absorbed at the water surface, and the light extinction for pure water (Table 1). The parameter values are listed in Table 1. The model performed well in simulating the hydrodynamics, including density current patterns (overflow, underflow, or interflow), velocities profiles (Figure 2). Predictions of water temperature in 2010–2011 yielded a good match to the measured profiles at three sites: S2 (downstream), S3 (middle reaches), and S4 (upstream) (Figure 3). The model performance was also evaluated by comparing the simulated results and observations at sites S2–S4, and these comparisons showed excellent model–data agreement. The overall averaged absolute mean error (AME) and averaged root mean square error (RMSE) were 0.21°C and 0.35°C, respectively, at site S2, 0.30°C and 0.53°C, respectively, at site S3, and 0.37°C and 0.47°C, respectively, at site S4. Most of the vertical profiles have AMEs <0.7°C and RMSEs <0.8°C. The error statistics show that the model effectively captured the characteristics of thermal stratification.
This study added water quality and algal module in the previous hydrodynamic model (Ma et al., 2015; Long et al., 2019). Ten controllable parameters affecting the growth of phytoplankton species are selected in the study. Taking into account the algal occurrences in XXB, five common algal species ALG1-5 (peridiniopsis, peridinium, diatoms, chlorophyta, and cyanophyta) were selected for simulation (Table 2). Observed chlorophyll a (Chl.a) at S3 and four kinds of algal blooms (pyrrophyta, diatom, chlorophyta, and cyanophyta) were used for model calibration (Figure 2). All parameters for each species are repeatedly calibrated and listed in Table 2.
As shown in Figure 4, the simulated four algal blooms (chlorophyta, pyrrophyta, diatom, and cyanophyta), and Chl.a concentration were used to compare field data to model predictions. The simulated results indicated that the algal model can capture the temporal dynamics of each phytoplankton biomass and algal blooms events from Chl.a concentration (Figure 4). Four algal densities have a relatively high level in spring and summer and nearly narrow almost to vanishing point. Figure 4 shows that there are good agreements between the model predictions and the field data on the occurrence time. Occasionally, the algal peaks vary considerably, especially in pyrrophyta, but the model can simulate the seasonal distribution of each phytoplankton. For example, the biomass of pyrrophyta increased in the spring, and cyanophyta prefer stratified water with high temperature. On the whole, predictions of Chl.a concentration in 2010–2011 yielded a good match to the measured variations, indicating that the model effectively captured the growth and disappearance of phytoplankton.
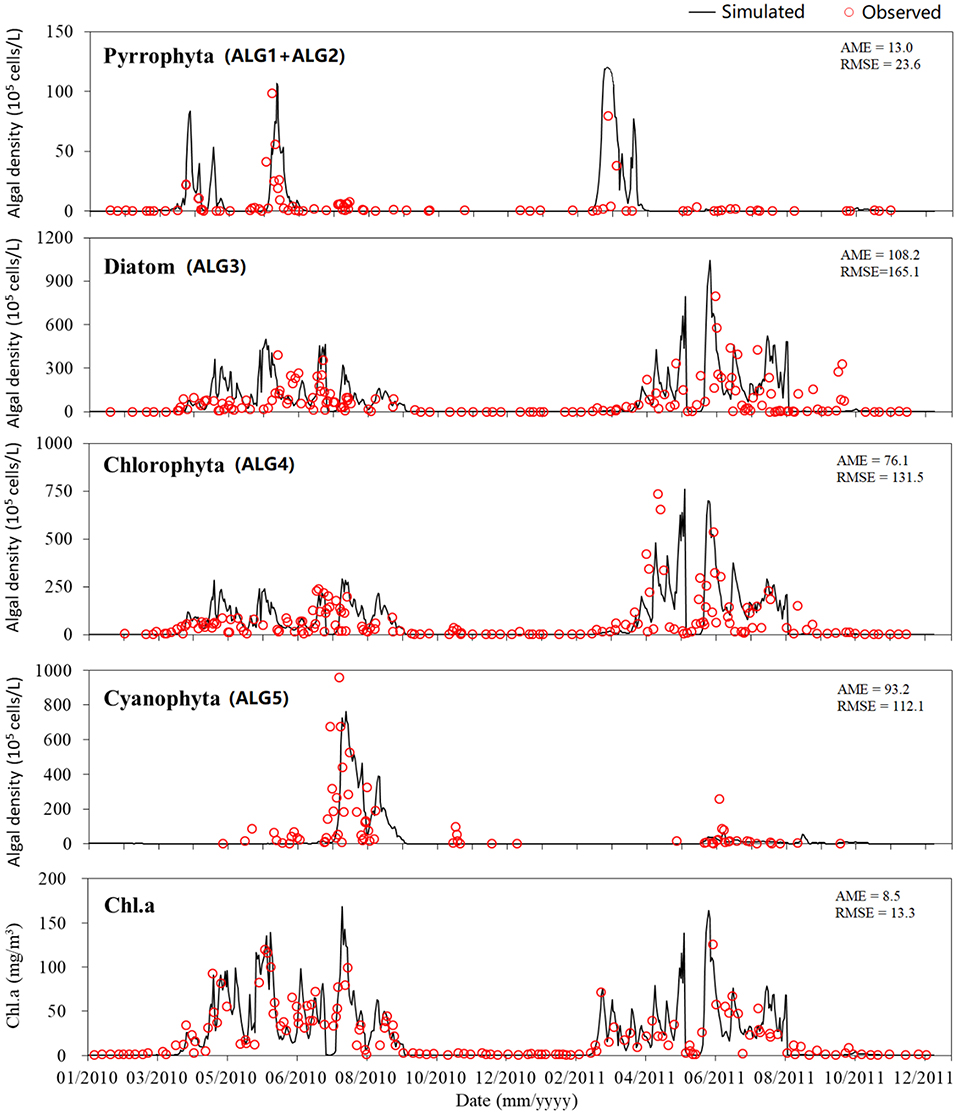
Figure 4. Observed and simulated surface four algal density (Pyrroptata, diatom and cyanophyta, chlorophyta) and Chl.a at S3 in the Xiangxi bay.
Model Output and Post-processing
In this model, segments 63, 25, and 09 represent the three field measurement sites [mouth site (S1), middle site (S3), and upper site (S2)] in Figure 1C. The daily simulated velocity, temperature, and Chl.a concentration profiles at these three segments were outputted and processed in Matlab 6.0 for further analysis. In addition, the euphotic depth (Zeu), which is the suitable light zone for the phytoplankton photosynthesis, was estimated when the solar radiation intensity was attenuated to 1% of that at the surface. The mixed layer depth (Zmix) was determined as the depth at which the water temperature was 0.5°C lower than that of the surface water (Montegut et al., 2004).
Results
Dynamics of Density Currents in the Xiangxi Bay
The temporal and spatial dynamics of flow velocity at the mouth, middle, and upper sites of XXB and upstream inflow discharge rate are shown in the Figure 5. The positive velocity indicates water flowing into the tributary bay from mainstream, and the reverse represents the water flowing out from the tributary bay. A two-layered flow structure of density currents was clearly visible at three sites (S2–S4), but the magnitude and direction of the velocity differed. It can be seen that the intensity and the plunge depth of the density current were dynamic and characterized by a typical seasonal pattern. The density currents can be distinguished by overflow, interflow, and underflow according to the plunge depth. As shown in Figure 5A, the water from the mainstream started to flow into the XXB from the middle layer (interflow) at early February and gradually moved to the bottom (underflow) until mid-March. Then, the intrusion water suddenly ran up to the upper layer, ~5 m below the surface, until the end of August. Meanwhile, it increased in strength with the double magnitude of the flow rate, but twice upon a time it turned into overflow, e.g., in mid-May and mid-August. Another sharp and significant shift occurred in the end of July, a short downward flow in the middle of the long overflow period.
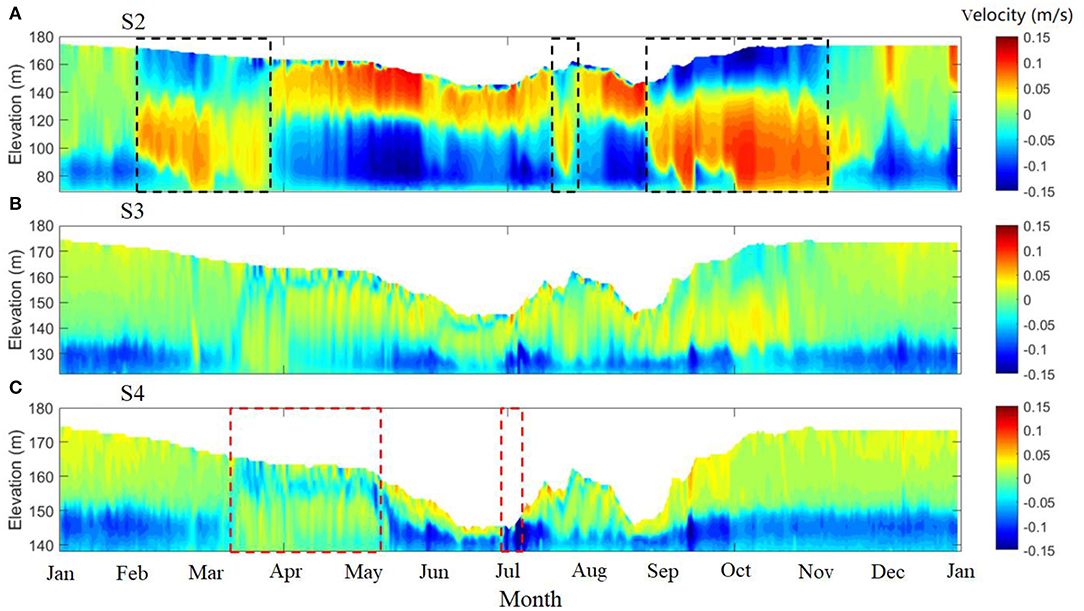
Figure 5. Temporally simulated horizontal velocities at the mouth (A, S2), middle (B, S3), and upper (C, S4) sites of Xiangxi Bay in 2012. Positive flow velocity corresponds to upstream flow (from TGR into the Xiangxi Bay); negative velocity is in the direction of the river flow. The black and red dotted boxes show the sharp shift of density currents, respectively.
With the impoundment of TGR in September, the depth of intrusion water moved down over again, and it was transformed into underflow in October, then gradually weakened after November. This longer-term variability is most likely related to changes in mainstream water density (mainly due to the temperature change) and the seasonal fluctuation of water level in TGR. Compared to the mouth site, the flow structures at the middle and upper sites are more stable and simple (Figures 5B,C); nevertheless, the velocity magnitudes of intrusion water were significantly decreased. The features of flow velocity at S3 and S4 were similar in spite of the large differences in water depth. The cold river inflow along the bottom (underflow) occurred within a maximum thick layer of 10 (S3)−15 m (S4) most of the time. However, it was also seen that upstream inflow entered into the bay as an interflow, and even as an overflow, e.g., from mid-March to early May. This short-time shift of inflow density can be caused by the warming of air temperature and reducing of inflow discharge in spring.
Spatiotemporal Variation of Stratification
Figure 6A shows temporally simulated water temperature at the mouth, middle, and upper reach of the Xiangxi Bay from January 1, 2012 to December 31, 2012. The results show that stratification varied in space and time. In space, the vertical thermal structure at the mouth is markedly different from that of the upstream sites (mid and upper site). There is no persistent stratification at the mouth site, even in summer when mixing goes through the bottom layer (Figure 6A). The mixing depths (Zmix) have consistently exceeded 90 m from the end of September to the middle of March, with the highest water level operation in TGR. In spring and summer, the short-time and weak stratification were broken, exactly as the frequent up-and-down jumps of Zmix. The bottom water column was energetic and active without visible hypolimnion. Compared to the mouth area, the middle and upper part of the tributary bay had a 6-months period of thermal layering (Figures 6B,C). It started from the late of March when the surface layer got warmer due to the increase in solar radiation. With the vertical transfer of heat to deeper water layers, the thickness of the bottom cold layer gradually decreased, while the water temperature differences between the surface and bottom gradually increased until May. Stratification reached its maximum level during May–August when the temperature difference of >6°C between the top and the bottom layers occurred. It is worthy to note that the strong thermal laying in summer also showed short-time synoptic variability, e.g., short-time mixing over all depths due to intensification of river inflow at the end of June (Figure 6C). However, this break of stratification caused by storms only occurred locally in the upstream area, and its effects in the middle part of the river (Figure 6B) were notably weakened. By the beginning of September, the mixing layer increased rapidly with the rise in water levels. From early September to mid-March, the water column was nearly completely mixed and had no significant vertical temperature differences (MTD <2°C). During this period, there was little spatial difference in water temperature indicating a complete mixed regime of the tributary.
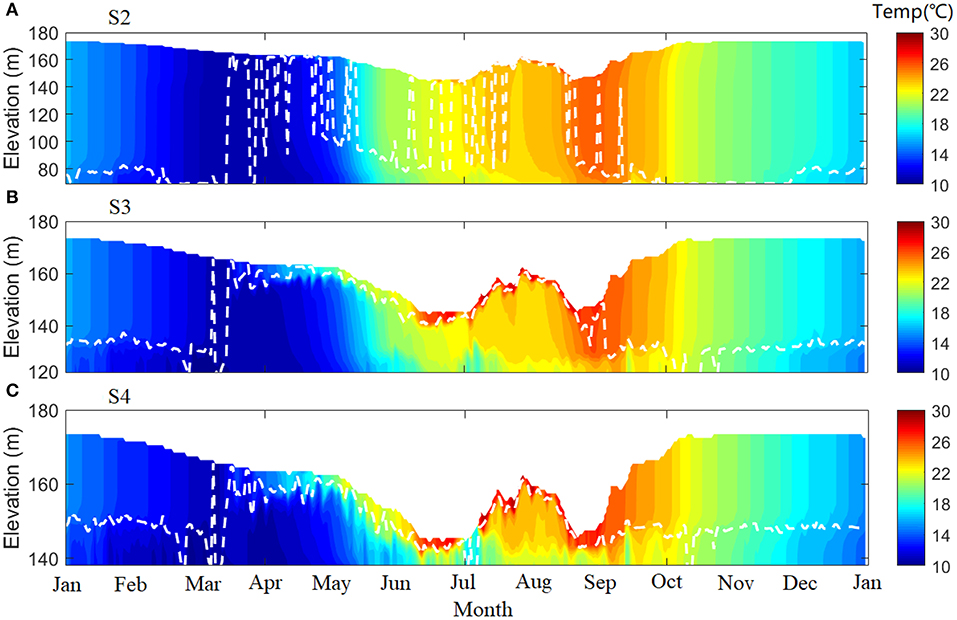
Figure 6. Time series of simulated water temperature at the mouth (S2), middle (S3), and upper (S4) sites of the Xiangxi Bay in 2012 (A–C). The white dash line shows the mixing depth (<0.5°C below surface temperature).
Dynamics of Chl.a and Phytoplankton Composition
Figure 7A shows the seasonal variation of Chl.a during a stratified period from April 1 to September 31, when surface Chl.a > 1 μg/L. The results indicated five algal bloom events when Chl.a > threshold (30 μg/L) in the XXB, in April 6–11 (~37 μg/L), June 2–28 (~79 μg/L), July 7–21 (~65 μg/L), August 2–15 (~79 μg/L), and August 8 to September 8 (~55 μg/L), respectively. High levels in Chl.a mainly occurred within 5 m below the water surface, except at the end of the bloom events, e.g., in early September. Figure 7B displays the seasonal succession of surface phytoplankton, which was associated with changes in water temperature. Diatoms and chlorophyta were the widespread species of algal over the entire stratification period. Diatoms predominated in April–May (>50%) and chlorophyta in June–July, especially in mid-June (>90%). There were two brief periods with pyrroptata cells (~5–10%) in mid-April and September. In addition, a significant increase in cyanophyta cells occurred after July. Cyanophyta, diatom, and chlorophyta were evenly distributed from the end of August to mid-September. Variations in phytoplankton biomass and succession were both associated with seasonal stratification and Zmix. Figure 7C shows the spatial distribution of surface Chl.a at one algal bloom event (June 12) in the XXB, indicating that the algal blooms were prone to occur in the intermediate and upstream zones of the bay (16–28 km distance from the mouth of the river). The downstream zones where the hydrodynamics were mainly affected by the mainstream were not propitious for the formation of algal blooms. The riverine hydrodynamic conditions at the upstream end of the river were also unfavorable for the growth of phytoplankton. The spatial distributions of phytoplankton were associated with longitudinal differences in hydrodynamics.
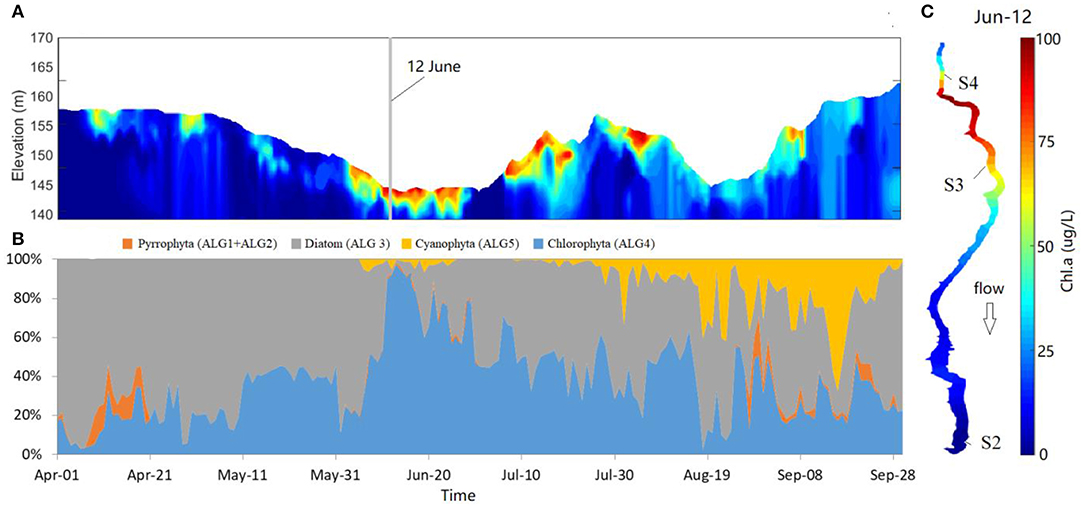
Figure 7. (A) Temporally simulated Chl.a at S4 in stratified period (April–September). (B) Seasonal succession of phytoplankton composition at S4 at the same period as in panel (A). (C) Space distribution of surface Chl.a on June 12, 2012, corresponding to the gray background line in panel (A).
Effect of Hydrodynamics on Seasonal Chl.a Variation
Figure 8 displays the seasonal variations of Chl.a that were associated with changes in the vertical mixing driven by density current and water level fluctuations. As mentioned above, the algal bloom events always occurred in the stratification period (April–September) when external environment conditions were appropriate, e.g., temperature, flow, and nutrition. In the stratification period, the Zmix at the upper site gradually decreased with the increasing stratification (Figure 8B), except in the end of July. It can be seen that Zmix varied from >Zeu to < Zeu, corresponding, respectively, to the lower level of Chl.a in spring and the high level in summer, although the relative depths of intrusion water from the estuary had little change (Figure 6C). Two short-term and rapid decreases in Chl.a are highlighted by two narrow gray filled boxes in July (Figure 8). One occurred in the end of June with lower Chl.a concentration, which can contribute to an upstream flood. In the flood period, surface water temperature decreased by ~10°C and caused a completed mixing over the entire depths and phytoplankton extinction (Figures 8A,B). Another reduction in Chl.a happened in the end of July when the water level quickly increased. Compared to the impacts of upstream flood, the Zmix also slightly increased, which is caused by the moving down of the plunge depth at the end of July. As a result, the growth of phytoplankton was inhibited when Zmix > Zeu. Then the algal bloom erupted again subsequent to the decrease in Zmix in August. However, the depth variation between Zmix and Zeu cannot explain all increases in Chl.a in XXB. For example, in early September, the short-time and sharp increase of Chl.a may be just a scum of alga due to the reservoir filling. The Zmix rapidly increased to a range well over Zeu due to the filling in early September. The unfavorable mixing regime and dilution effect of the intrusion water resulted in the decrease in Chl.a and phytoplankton extinction (Figure 8A). The strong mixing was driven by the kinetic energy of the intrusion water in early September and then maintained by winter convective overturn.
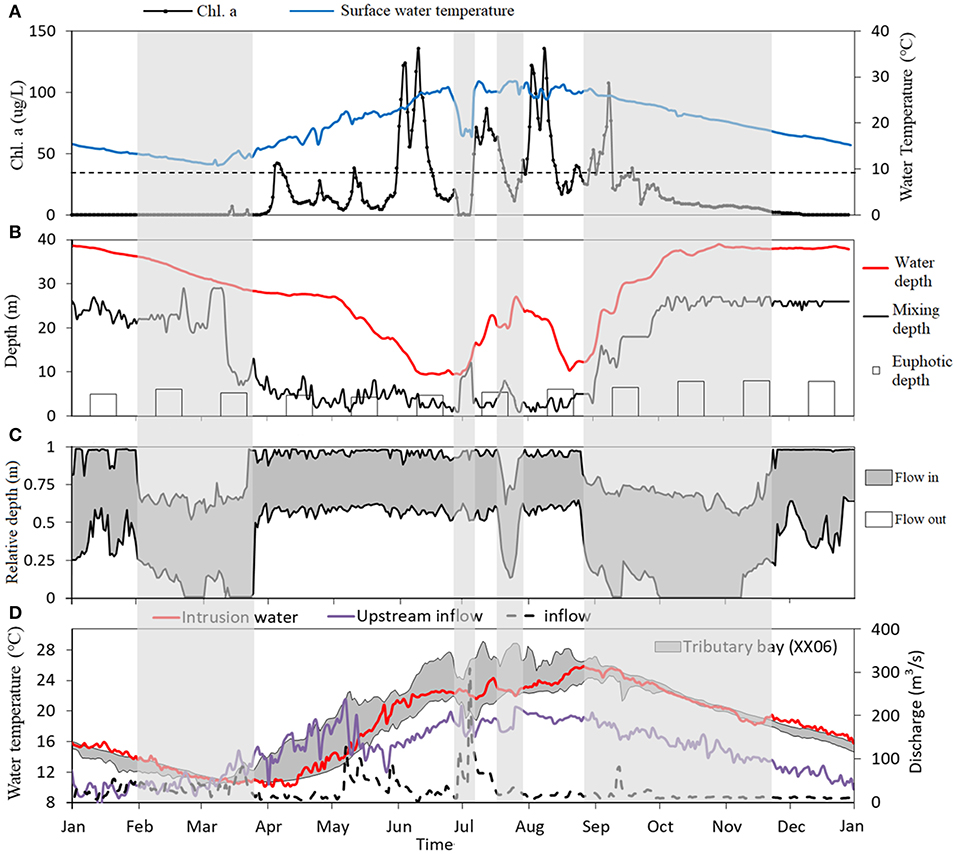
Figure 8. Annual variation of each parameter in 2012. (A) Daily surface Chl.a concentration and water temperature at upper site (S4). The dash line is the threshold of algal bloom (30 μg/L). (B) Daily water depth, mixing depth, and monthly measured euphotic depth at upper site (S4). (C) Relative depth of intrusion water from mainstream at mouth site (S2). (D) Daily water temperature at mainstream, tributary bay (S3), and inflow and upstream inflow. Gray filled box represents the four special periods differed to the other period.
Discussion
The Effects of Density Currents on the Stratification and Algal Blooms
In this study, the two-dimensional CE-QUAL-W2 model successfully captured the dynamics of density currents, stratification, and phytoplankton. Through numerical simulation, the results show the spatiotemporal difference of hydrodynamics and stratification in the tributary bay of the Three Gorges Reservoir (Figures 5, 6). At the mouth area, frequent water exchange driven by a dynamic density current led to complete mixing over the entire water column. The changes in intensity and plunge depth of density current created more vertical mixing in this area. Even during summer, the bottom water was energetic and active. The mixing driven by dynamic density current was sufficient to break down the vertical stratification and inhibit algal growth in the downstream area (0–16 km), consistent with previous reports (Dai et al., 2013). Temperature differences (resulting in density differences) have been identified as the main cause of density currents in the tributary of the Three Gorges Reservoir (Ma et al., 2015; Long et al., 2019). In rivers and reservoirs, the density variation can result in density flow intrusion that sometimes sinks and sometimes rises up. The simulation results also showed that temperature differences between the mainstream and the tributary bay (Figure 8D) had a good agreement with the frequent shift of density flow intrusion (Figure 5A). In addition, the results revealed that the intensities (magnitude of velocity) of the density currents were larger in summer than in winter (Figure 5A). The greater the temperature difference, the energy of intrusion water would be larger (Ma et al., 2015). The temperature differences frequently varied due to the changes in water temperature in mainstream, which is affected by reservoir operation, e.g., rapid filling and massive flood discharge (Figure 8D). Ma et al. (2015) and Ji et al. (2017) had examined the rapid filling in September and found that it can increase flow rates of intrusion water and create more mixing to break down the stratification. Thus, the reservoir operation can be a controllable way to affect the density currents and further change the hydrodynamics in the tributary.
By contrast to the downstream area, the upstream area (16–28 km) is the most sensitive and high-risk area for algal blooms due to the favorable conditions for phytoplankton growth, e.g., right temperature, flow, and enough nutrients (Paerl et al., 2001; Paerl and Paul, 2012). Many studies have reported that the hydrodynamics play a vital role in seasonal dynamics of phytoplankton succession in the TGR (Ji et al., 2017, Liu et al., 2012). In the shallower bay, it presented a period of ~5 months of stratifications with nearly complete mixing from September to the following February. We found that most of the time, the upper layer water was pushed forward upstream by a downstream intrusion water (Figure 5C), and the bottom cold water flowed out along the riverbed as an underflow (except during spring). When the upper density flow intrusion and bottom inflow were separated, they formed a two-layered flow structure of density current. With the upstream movement of the surface water, flow rates gradually slowed down, and the stratification intensified. The development of flow created a favorable environment for phytoplankton and resulted in the increases in the Chl.a concentration (Figure 7). It can be seen that the hydrodynamics of the upper water was dominated by the downstream density flow intrusion. However, the upstream inflow still cannot be ignored. For example, in March–April, the warmer upstream inflow entered into the bay from middle or surface layers and met the density flow intrusion from downstream (Figure 5C). Nevertheless, this spring shifts of inflow water from upstream failed to inhibit the diatom growth of phytoplankton because the inflow discharge was small enough so that it did not create a large area of mixing. However, in flood season, a mass of cold inflow water can easily completely break density flow and stratification, such as in the end of June (Figure 8D). As a consequence of floodwaters, water temperatures rapidly dropped, and phytoplankton vanished suddenly. When the floodwater receded, a favorable environment was created again with a rapid warming in water temperature and stratification, and then algal blooms occurred again. Thus, upstream inflows are an important factor to affect fast the stratification and phytoplankton. Considering this, the cascade dams at the upstream of the XXB can provide a controllable inflow condition, either the discharge or temperature.
Main Factors Affecting the Vertical Mixing in the Tributary
A lot of studies have revealed that vertical mixing is an important physical parameter, which can affect algal growth in eutrophic water (Elliott et al., 2001; Liu et al., 2012; Gao et al., 2017). That is true from our simulation results that show that the variation of mixing depth can reveal seasonal stratification in XXB, and it plays a crucial role in the growth of phytoplankton in the XXB. The mixing regimes can be affected by density current, including intrusion water from the mainstream and inflow water from upstream. This explanation was confirmed earlier by Liu et al. (2012) from field measurement. However, in lakes or reservoirs, the mixing regime changes in spatial or temporal scales can also be driven by the other forces, such as heat balance, wind stress, seiching, or internal waves (Serra et al., 2007; Preusse et al., 2010). The surface stratification can inhibit vertical mixing in spring and summer, while convective overturn occurring in autumn and winter promotes deeper mixing, even to the bottom. Similar to natural lakes in the temperate zone (Ullyott and Holmes, 1936; Boehrer and Schultze, 2008), the XXB undergoes seasonal variations in stratification and shows a dimictic annual stratification pattern caused by climate. However, the stratification in the XXB breaks down rapidly, even within 1 week, and has a longer complete mixing period, resulting in a strong artificial property. The water level fluctuations occupy a pivotal position in the annual cycle of stratification (Jin et al., 2019). In lakes and reservoirs, wind stress has also been demonstrated to be the other key factor in the mixing and circulation processes (Bengtsson, 1978; Findikakis and Law, 1999). By increasing the surface mixing, it not only influences the intensity and duration of stratification but also contributes to the heat storage of water bodies (Zhang et al., 2020). In this study, the frequent changes in surface flow direction could be driven by periodic wind speed (Figure 5). Nonetheless, it is difficult to identify the wind effect in detail because the sub-daily or small-scale variation cannot be resolved with daily averaged boundary conditions and 1-m vertical cells. High-frequency wind and fine vertical flow measurements in the surface layer are necessary to quantify the influence of wind on surface mixing in density current flow.
Internal waves are a universal feature in stratified aquatic system, which are the most important driving force for horizontal and vertical transport of energy and matter below the surface mixed layer during the stratified period (Hodges et al., 2000; Bocaniov et al., 2014). Yu et al. (2012) first reported from numerical modeling that the existence of internal wave generated by tidal flow can have a remarkable effect on hydrodynamic and stratification in the XXB. Soon afterward, the artificial tide flow and its effects on the water environment were further studied by Sha et al. (2015). Driven by tide flow, the water level and flow velocity in the tributary bay can represent a so far overlooked high-frequency oscillated feature. Recently, Long et al. (2020) first reported the high-frequency bay oscillations with a period of ~2 h in the XXB, which contributed to flow velocity and caused a frequent reversal of the density current, resulting in more mixing at the interface (Long et al., 2020). It indicates that the oscillation seiching is also an important factor to affect the mixing regimes in the tributary bay of the Three Gorges Reservoir. Under the effects of external forces, e.g., density current, wind, surface heat exchange, or seiching, the temporal and spatial scale of mixing are complex and flexible, particularly in space and time. This study highlights the importance of considering both high-frequency temporal and spatial measurements to better understand the hydrodynamics of reservoirs.
Conclusions
The two-dimensional hydrodynamic and algal model of XXB based on CE-QUAL-W2 was able to adequately simulate the shift in density current, seasonal stratification, and algal blooms. The CE-QUAL-W2 model performed well in hydrodynamics and algal bloom forecasts in XXB. The hydrodynamics show significant temporal and spatial differences in XXB. In the mouth area, the intensity and plunge depth of the density current were dynamic and characterized by a seasonal pattern. The frequent transformations of plunge depth provide more opportunities for vertical mixing, resulting in no stratification and low risk of algal blooms at the mouth. However, in the middle and upper areas, a two-layered flow structure of density current (upper layer driven by density flow intrusion from mainstream, lower layer driven by upstream cold inflow) is long standing. Strong stratification and low velocity at upstream provide enough favorable conditions for the growth of algae and increase the risk of algal blooms. The rapid variations in upstream inflow caused by floodwater can have a short-term change in the stratification and phytoplankton. The study further proves that the mixing depth plays a vital role in seasonal dynamics of phytoplankton succession in the XXB, which can be affected by density current, reservoir operation in the water level, upstream inflow, weather, etc. High-frequency fine vertical flow measurements in the surface layer are necessary to quantify the influence of multifactorial on surface mixing in density current flow. To improve the understanding of mixing will be helpful to the physical mechanism of algal bloom elimination.
Data Availability Statement
The raw data supporting the conclusions of this article will be made available by the authors, without undue reservation.
Author Contributions
HX: first writing. DJ and ZY: field measurement and data collection. DL, ZY, and DJ: fund. MY and JM: model design. HX and LL: data analysis. All authors contributed to the article and approved the submitted version.
Funding
This work was financially supported by the Natural Science Foundation of China (Grant Nos. 91647207, 51779128, 51879099, 51909135, 52009066, 52079075, and 51809298). The China Three Gorges Corporation provided the necessary hydrologic data.
Conflict of Interest
The authors declare that the research was conducted in the absence of any commercial or financial relationships that could be construed as a potential conflict of interest.
Acknowledgments
We also extend their thanks to all of the members of the Engineering Research Center of Eco-Environment in the Three Gorges Reservoir Region, Ministry of Education, China Three Gorges University for participating in the field monitoring.
References
Afshar, A., Kazemi, H., and Saadatpour, M. (2011). Particle swarm optimization for automatic calibration of large scale water quality model (CE-QUAL-W2): application to karkheh reservoir, Iran. Water Resourc. Manage. 25, 2613–2632. doi: 10.1007/s11269-011-9829-7
Afshar, A., Shojaei, N., and Sagharjooghifarahani, M. (2013). Multiobjective calibration of reservoir water quality modeling using multiobjective particle swarm optimization (MOPSO). Water Resourc. Manage. 27, 1931–1947. doi: 10.1007/s11269-013-0263-x
Anderson, E. P., Jenkins, C. N., Heilpern, S. A., Maldonadoocampo, J. A., Carvajalvallejos, F. M., Encalada, A. C., et al. (2018). Fragmentation of andes-to-amazon connectivity by hydropower dams. Sci. Adv. 4, 1–7. doi: 10.1126/sciadv.aao1642
Bengtsson, L. (1978). Wind induced circulation in lakes. Hydrol. Res. 9, 75–94. doi: 10.2166/nh.1978.0007
Berger, C., and Wells, S. A. (2008). Modeling the effects of macrophytes on hydrodynamics. J. Environ. Eng. 134, 778–788. doi: 10.1061/(ASCE)0733-9372(2008)134:9(778)
Bie, Y., Jiang, D., Tang, M., Kong, F., and Wang, Y. (2013). Influence of the scheduling of the three gorges reservoir on the hydrodynamic field in xiangxi river. Adv. Mater. Res. 726–731, 3429–3433. doi: 10.4028/www.scientific.net/AMR.726-731.3429
Bocaniov, S. A., Ullmann, C., Rinke, K., Lamb, K. G., and Boehrer, B. (2014). Internal waves and mixing in a stratified reservoir: insights from three-dimensional modeling. Limnologica 49, 52–67. doi: 10.1016/j.limno.2014.08.004
Boehrer, B., and Schultze, M. (2008). Stratification of lakes. Rev. Geophys. 46, 1–27. doi: 10.1029/2006RG000210
Chuo, M., Ma, J., Liu, D., and Yang, Z. (2019). Effects of the impounding process during the food season on algal blooms in xiangxi bay in the three gorges reservoir, China. Ecol. Modell. 392, 236–249. doi: 10.1016/j.ecolmodel.2018.11.017
Cui, X., Liu, S., and Wei, X. (2012). Impacts of forest changes on hydrology: a case study of large watersheds in the upper reaches of minjiang river watershed in China. Hydrol. Earth Syst. Sci. 16, 4279–4290. doi: 10.5194/hess-16-4279-2012
Dai, H., Mao, J., Jiang, D., and Wang, L. (2013). Longitudinal hydrodynamic characteristics in reservoir tributary embayments and effects on algal blooms. PLoS ONE 8:e68186. doi: 10.1371/journal.pone.0068186
Dai, H., Zheng, T., and Liu, D. (2010). Effects of reservoir impounding on key ecological factors in the three gorges region. Proc. Environ. Sci. 2, 15–24. doi: 10.1016/j.proenv.2010.10.004
DelSontro, T., Perez, K. K., Sollberger, S., and Wehrli, B. (2016). Methane dynamics downstream of a temperate run-of-the-river reservoir. Limnol. Oceanogr. 61, S188–S203. doi: 10.1002/lno.10387
Elliott, J. A., Irish, A. E., and Reynolds, C. S. (2001). The effects of vertical mixing on a phytoplankton community: a modelling approach to the intermediate disturbance hypothesis. Freshw. Biol. 46, 1291–1297. doi: 10.1046/j.1365-2427.2001.00754.x
Fearnside, P. M., and Pueyo, S. (2012). Greenhouse-gas emissions from tropical dams. Nat. Clim. Chang. 2, 382–384. doi: 10.1038/nclimate1540
Findikakis, A. N., and Law, A. W. K. (1999). Wind mixing in temperature simulations for lakes and reservoirs. J. Environ. Eng. 125, 420–428. doi: 10.1061/(ASCE)0733-9372(1999)125:5(420)
Gao, Q., He, G., Fang, H., and Huang, L. (2017). Effects of vertical mixing on algal growth in the tributary of three gorges reservoir. J. Hydraulic Eng. 48, 96–103.
Glubt, S. V. (2017). Hydrodynamic and water quality modeling of the chehalis river using CE-QUAL-W2. (Dissertations/master's Theses). Portland State University: Portland. doi: 10.1061/9780784480601.019
Grill, G., Dallaire, C. O., Chouinard, E. F., Sindorf, N., and Lehner, B. (2014). Development of new indicators to evaluate river fragmentation and flow regulation at large scales: a case study for the mekong river basin. Ecol. Indic. 45, 148–159. doi: 10.1016/j.ecolind.2014.03.026
Guo, H., Hu, Q., Zhang, Q., and Feng, S. (2012). Effects of the three gorges dam on yangtze river flow and river interaction with poyang lake, China: 2003-2008. J. Hydrol. 416, 19–27. doi: 10.1016/j.jhydrol.2011.11.027
Hodges, B. R., Imberger, J., Saggio, A., and Winters, K. B. (2000). Modeling basin-scale internal waves in a stratified lake. Limnol. Oceanogr. 45, 1603–1620. doi: 10.4319/lo.2000.45.7.1603
Hu, W., Wang, G., Deng, W., and Li, S. (2008). The influence of dams on ecohydrological conditions in the huaihe river basin, China. Ecol. Eng. 33, 233–241. doi: 10.1016/j.ecoleng.2008.04.003
Ji, D., Wells, S. A., Yang, Z., Liu, D., Huang, Y., Ma, J., et al. (2017). Impacts of water level rise on algal bloom prevention in the tributary of three gorges reservoir, China. Ecol. Eng. 98, 70–81. doi: 10.1016/j.ecoleng.2016.10.019
Jin, J., Wells, S. A., Liu, D., Yang, G., and Yang, Z. (2019). Effects of water level fluctuation on thermal stratification in a typical tributary bay of three gorges reservoir, China. PeerJ 7:6925. doi: 10.7717/peerj.6925
Lima, I. B. T., Ramos, F. M., Bambace, L. A. W., and Rosa, R. R. (2008). Methane emissions from large dams as renewable energy resources: a developing nation perspective. Mitig. Adapt. Strateg. Glob. Chang. 13, 193–206. doi: 10.1007/s11027-007-9086-5
Liu, D., Yang, Z., Ji, D., Ma, J., Cui, Y., and Song, L. (2016). A review on the mechanism and its controlling methods of the algal blooms in the tributaries of Three Gorges Reservoir. J. Hydr. Eng. 47, 443–454. doi: 10.13243/j.cnki.slxb.20151304
Liu, J., Xu, Y., Ma, J., Liu, D., and Yang, Z. (2018). Model of water temperature stratification in Xiangxi bay of the Three Gorges Reservoir with different density currents. J. Yangtze River Sci. Res. Inst. 35, 37–42.
Liu, L., Liu, D., Johnson, D. M., Yi, Z., and Huang, Y. (2012). Effects of vertical mixing on phytoplankton blooms in xiangxi bay of three gorges reservoir: implications for management. Water Res. 46, 2121–2130. doi: 10.1016/j.watres.2012.01.029
Long, L., Ji, D., Liu, D., Yan, M., Cui, Y., and Song, L. (2016b). Characteristics of flow velocity and water temperature in Shennong bay using CE-QUAL-W2 model. J. Yangtze River Sci. Res. Inst. 33, 28–35. doi: 10.11988/ckyyb.20140927
Long, L., Ji, D., Yang, Z., Cheng, H., Yang, Z., Liu, D., et al. (2020). Tributary oscillations generated by diurnal discharge regulation in three gorges reservoir. Environ. Res. Lett. 15:084011. doi: 10.1088/1748-9326/ab8d80
Long, L., Ji, D., Yang, Z., Ma, J., Wells, S. A., Liu, D., et al. (2019). Density-driven water circulation in a typical tributary of the three gorges reservoir, China. River Res. Appl. 35, 833–843. doi: 10.1002/rra.3459
Long, L., Xu, H., Ji, D., Cui, Y., Liu, D., and Song, L. (2016a). Characteristic of the water temperature lag in three gorges reservoir and its effect on the water temperature structure of tributaries. Environ. Earth Sci. 75:1459. doi: 10.1007/s12665-016-6266-1
Ma, J., Liu, D., Wells, S. A., Tang, H., Ji, D., and Yang, Z. (2015). Modeling density currents in a typical tributary of the three gorges reservoir, China. Ecol. Modell. 296, 113–125. doi: 10.1016/j.ecolmodel.2014.10.030
Montegut, C. D. B., Madec, G., Fischer, A. S., Lazar, A., and Iudicone, D. (2004). Mixed layer depth over the global ocean: an examination of profile data and a profile-based climatology. J. Geophys. Res. 109, 1978–2012. doi: 10.1029/2004JC002378
Noori, R., Yeh, H., Ashrafi, K., Rezazadeh, N., Bateni, S. M., Karbassi, A. R., et al. (2015). A reduced-order based CE-QUAL-W2 model for simulation of nitrate concentration in dam reservoirs. J. Hydrol. 530, 645–656. doi: 10.1016/j.jhydrol.2015.10.022
Nwankwegu, A. S., Li, Y., Huang, Y., Wei, J., Norgbey, E., Lai, Q., et al. (2020). Nutrient addition bioassay and phytoplankton community structure monitored during autumn in xiangxi bay of three gorges reservoir, China. Chemosphere 247:125960. doi: 10.1016/j.chemosphere.2020.125960
Paerl, H. W., Fulton, R. S., Moisander, P. H., and Dyble, J. (2001). Harmful freshwater algal blooms, with an emphasis on cyanobacteria. ScientificWorldJournal 1:139109. doi: 10.1100/tsw.2001.16
Paerl, H. W., and Paul, V. J. (2012). Climate change: links to global expansion of harmful cyanobacteria. Water Res. 46, 1349–1363. doi: 10.1016/j.watres.2011.08.002
Park, Y., Cho, K. H., Kang, J., Lee, S. W., and Kim, J. H. (2014). Developing a flow control strategy to reduce nutrient load in a reclaimed multi-reservoir system using a 2D hydrodynamic and water quality model. Sci. Total Environ. 466, 871–880. doi: 10.1016/j.scitotenv.2013.07.041
Preusse, M., Peeters, F., and Lorke, A. (2010). Internal waves and the generation of turbulence in the thermocline of a large lake. Limnol. Oceanogr. 55, 2353–2365. doi: 10.4319/lo.2010.55.6.2353
Rafiee, M., and Jahangirirad, M. (2015). Artificial neural network approaches to the prediction of eutrophication and algal blooms in aras dam, Iran. Iran. J. Health Sci. 3, 25–32. doi: 10.7508/ijhs.2015.01.004
Serra, T., Vidal, J., Casamitjana, X., Soler, M., and Colomer, J. (2007). The role of surface vertical mixing in phytoplankton distribution in a stratified reservoir. Limnol. Oceanogr. 52, 620–634. doi: 10.4319/lo.2007.52.2.0620
Sha, Y., Wei, Y., Li, W., Fan, J., and Cheng, G. (2015). Artificial tide generation and its effects on the water environment in the backwater of three Gorges Reservoir. J. Hydrol. 528, 230–237. doi: 10.1016/j.jhydrol.2015.06.020
Ullyott, P., and Holmes, P. (1936). Thermal stratification in lakes. Nature 138, 971–971. doi: 10.1038/138971a0
Wang, L., Liu, L., and Zheng, B. (2013). Eutrophication development and its key regulating factors in a water-supply reservoir in North China. J. Environ. Sci. China 25, 962–970. doi: 10.1016/S1001-0742(12)60120-X
Wells, S. A. (2019). CE-QUAL-W2: A Two-Dimensional, Laterally Averaged, Hydrodynamic and Water Quality Model, Version 4.2, User Manual: Part 1 Introduction, Department of Civil and Environmental Engineering. Portland, OR: Portland State University.
Winemiller, K. O., Mcintyre, P. B., Castello, L., Fluetchouinard, E., Giarrizzo, T., Nam, S., et al. (2016). Balancing hydropower and biodiversity in the Amazon, Congo, and Mekong. Science 351, 128–129. doi: 10.1126/science.aac7082
Wu, D., Yan, H., Shang, M., Shan, K., and Wang, G. (2017). Water eutrophication evaluation based on semi-supervised classification: a case study in three gorges reservoir. Ecol. Indic. 81, 362–372. doi: 10.1016/j.ecolind.2017.06.004
Xiong, C. J., Liu, D. F., Zheng, B. H., Zhang, J. L., Hu, N. S., Zhang, Y., et al. (2013). The Influence of hydrodynamic conditions on algal bloom in the three gorges reservoir tributaries. Appl. Mech. Mater. 295–298, 1981–1990. doi: 10.4028/www.scientific.net/AMM.295-298.1981
Xu, X., Tan, Y., and Yang, G. (2013). Environmental impact assessments of the three gorges project in China: Issues and interventions. Earth Sci. Rev. 124, 115–125. doi: 10.1016/j.earscirev.2013.05.007
Yang, Z., Cheng, B., Xu, Y., Liu, D., Ma, J., and Ji, D. (2018b). Stable isotopes in water indicate sources of nutrients that drive algal blooms in the tributary bay of a subtropical reservoir. Sci. Total Environ. 634, 205–213. doi: 10.1016/j.scitotenv.2018.03.266
Yang, Z., Liu, D., Ma, J., Chen, Y., Fang, X., and Ji, D. (2012). Effects of special vertical layered water temperatures on algal bloom in Xiangxi bay of Three Gorges Reservoir. Eng. J. Wuhan Univ. 45, 1–9.
Yang, Z., Xu, P., Liu, D., Ma, J., Ji, D., and Cui, Y. (2018a). Hydrodynamic mechanisms underlying periodic algal blooms in the tributary bay of a subtropical reservoir. Ecol. Eng. 120, 6–13. doi: 10.1016/j.ecoleng.2018.05.003
Yang, Z., Yu, Y., Chen, Z., and Ma, J. (2017). Mechanism of eutrophication and phytoplankton blooms in three gorges reservoir, China: a research review. Eng. J. Wuhan Univ. 50, 507–516.
Yu, Z., Wang, L., Zhu, H., Lang, Y., Dai, H., and Williams, J. (2012). Numerical simulation of internal waves generation by tidal flow in Xiangxi Bay of the three Gorges reservoir. J. Sichuan Univ. 44, 26–30.
Zeilhofer, P., and De Moura, R. M. (2009). Hydrological changes in the northern pantanal caused by the manso dam: impact analysis and suggestions for mitigation. Ecol. Eng. 35, 105–117. doi: 10.1016/j.ecoleng.2008.09.011
Zhang, F., Zhang, H., Bertone, E., Stewart, R., Lemckert, C., and Cinque, K. (2020). Numerical study of the thermal structure of a stratified temperate monomictic drinking water reservoir. J. Hydrol. 30:100699. doi: 10.1016/j.ejrh.2020.100699
Zheng, B., Cao, C., Zhang, J., Huang, M., and Chen, Z. (2009a). Analysis of algal blooms in Da-Ning River of three gorges reservoir. Environ. Sci. 30, 3218–3226.
Zheng, B., Wang, L., and Gong, B. (2009b). Load of non-point source pollutants from upstream rivers into three gorges reservoir. Res. Environ. Sci. 22, 125–131.
Zhou, C., Yu, J., Li, F., Cui, Y., Liu, D., Jiang, W., et al. (2016). Temporal and spatial distribution of environmental factors and phytoplankton during algal bloom season in Pengxi River, Three Gorges Reservoir. Environ. Sci. 37, 873–883. doi: 10.13227/j.hjkx.2016.03.011
Keywords: density current, flow patterns, algal blooms, CE-QUAL-W2, three gorges reservoir
Citation: Xu H, Yan M, Long L, Ma J, Ji D, Liu D and Yang Z (2021) Modeling the Effects of Hydrodynamics on Thermal Stratification and Algal Blooms in the Xiangxi Bay of Three Gorges Reservoir. Front. Ecol. Evol. 8:610622. doi: 10.3389/fevo.2020.610622
Received: 26 September 2020; Accepted: 23 November 2020;
Published: 15 January 2021.
Edited by:
Yinjun Zhou, Changjiang River Scientific Research Institute (CRSRI), ChinaReviewed by:
Wang Wen, Xi'an University of Technology, ChinaYonghong Bi, Chinese Academy of Sciences, China
Liu Xiao Qing, Xihua University, China
Yanjun Zhang, Wuhan University, China
Copyright © 2021 Xu, Yan, Long, Ma, Ji, Liu and Yang. This is an open-access article distributed under the terms of the Creative Commons Attribution License (CC BY). The use, distribution or reproduction in other forums is permitted, provided the original author(s) and the copyright owner(s) are credited and that the original publication in this journal is cited, in accordance with accepted academic practice. No use, distribution or reproduction is permitted which does not comply with these terms.
*Correspondence: Lianghong Long, bG9uZ0B1bmktbGFuZGF1LmRl; Zhengjian Yang, eWFuZ3pqMTk4NEBjdGd1LmVkdS5jbg==