Introduction
Citizen science datasets are becoming increasingly important means by which researchers can study ecological systems on geographic and temporal scales that would be otherwise impossible (Kullenberg and Kasperowski, 2016). Birds are both a tractable study taxa for citizen science efforts, and an indicator of broad ecological and evolutionary themes such a climate change and anthropogenic habitat modification, invasive species dynamics and disease ecology (Bock and Root, 1981; Link and Sauer, 1998; Bonney et al., 2009), to name a few. Enjoying birds around one's home may seem like an ephemeral pastime, but in the context of citizen science, such a pastime has built a multi-decade long, continent-wide dataset of bird abundance through the program Project FeederWatch (hereafter, FeederWatch).
FeederWatch is a place-based citizen science program that asks participants to identify and count the birds that visit the area around their home, particularly focused around supplementary feeding stations (i.e., bird feeders). Place-based datasets provide a unique view of change through time and engage participants in long-term data collection from a single location, inspiring them to engage more deeply in the preservation of the place they study (Loss et al., 2015; Haywood et al., 2016). The concept of FeederWatch began when Erica Dunn of Canada's Long Point Bird Observatory established the Ontario Bird Feeder Survey in 1976 (Dunn, 1986). Ten years later, in 1986, the organizers expanded the survey to cover all provinces in Canada and states in the United States by partnering with the Cornell Lab of Ornithology to create the program now called Project FeederWatch (Wells et al., 1998). In the winter of 1987-88, more than 4,000 people enrolled and began counting birds following the current counting protocol. Since then, the number of project participants has grown to > 25,000 annually across the U.S. and Canada, approximately half of which submit bird checklists (Figure 1A). The program collates ~180,000 checklists annually (as of the 2019-2020 season) with submissions increasing over time (Figure 1B). FeederWatch continues to be a cooperative research project of the Cornell Lab of Ornithology and Birds Canada (formerly the Long Point Bird Observatory and later Bird Studies Canada) and has an inter-annual participant retention rate of ~60–70%.
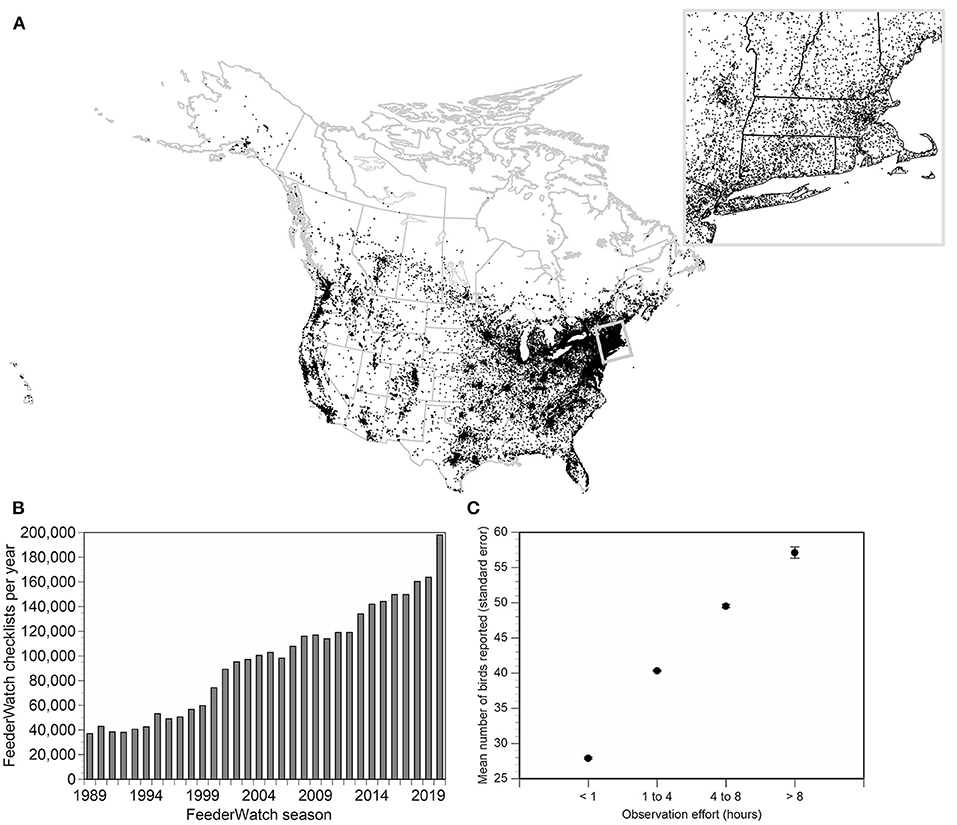
Figure 1. (A) Map of locations from which Project FeederWatch participants have submitted data (all sites, 1989–2020, N = 65,237 locations). The inset box provides detail of an example area of northeastern North America to better illustrate the density of sampling locations. (B) Total number of checklists submitted to Project FeederWatch by year. (C) Mean (± standard error) number of birds reported per checklist as a function of observation effort (categorical: < 1 h of effort, 1–4 h, 4–8 h, > 8 h). All years and sites combined.
Data from FeederWatch have been used in dozens of scientific publications, ranging in topic from invasive species dynamics (Bonter et al., 2010), disease ecology (Hartup et al., 2001), irruptive movements (Dunn, 2019), predator-prey interactions (McCabe et al., 2018), range expansions (Greig et al., 2017), dominance hierarchies (Leighton et al., 2018) and climate change (Zuckerberg et al., 2011; Prince and Zuckerberg, 2014). Studies use either the standard protocol bird count dataset, which is the dataset we describe here, or supplementary data protocols such as reports of signs of disease (Hartup et al., 2001), reports of behavioral interactions (Miller et al., 2017) or reports of window strike mortality (Dunn, 1993). Irrespective of the exact data type being collected, the strength of the FeederWatch dataset lies in the repeated observations made from the same location over time, which creates a data structure perfectly suited to occupancy modeling or repeated measures analyses. It also cultivates long-term participation in the project, which is predicted to increase data accuracy because participants are expected to improve their data collection skills the longer they participate (Kelling et al., 2015).
Methods
Data Collection Protocol
Participants follow a standardized counting protocol to record all the bird species they see around their count site, typically their home, and typically in the proximity of supplementary feeding stations or other resources (e.g., water or plantings). Specifically, participants count the maximum number of each bird species seen in their count site over a 2-day checklist period. By requiring that participants only report the maximum number of each species in view simultaneously during the checklist period, the protocol ensures that participants are not repeatedly recording the same individuals multiple times within a single checklist. Further, the protocol requires that participants submit complete checklists of all bird species observed, allowing for the inference of zeros (i.e., both detection and non-detection) in all checklists. These checklists are conducted from late fall through early spring in the northern hemisphere (November to April each year, the FeederWatch “season”). Participants can submit checklists as often as once per week within this time frame. For each checklist, participants are required to report two categorical measures of observation effort (detailed below). Participants also record a categorical estimate of snow cover. Historically, participants were asked to record additional weather variables during their checklist periods, but with the availability of large-scale climate datasets, collection of additional weather data has been discontinued. The protocol instructions provided to participants are available on the project web site (https://feederwatch.org/about/detailed-instructions/).
Because the FeederWatch protocol is a repeated measures design, participants are reporting from the same location as often as weekly, with many people reporting for many years. As such, it is useful to capture a description of the participant's count site and supplementary feeding procedures and how those change over time. Annually, participants can describe their count site on a form that records information about habitat, resources, and threats to birds. Completing the site description is not compulsory, so not every location has a complete site description for every year of participation (site description data were provided for 72% of count sites during the 2019-2020 season). Although the site description information is not available for all locations, this information can be useful for addressing specific research questions. For example, researchers may be interested in the effects of supplementary food type or amount on the detectability or occupancy of bird species in the community (e.g., Greig et al., 2017). Details of the 57 data fields recorded by participants on the site description form are available in the data repository.
Data Validation
All FeederWatch checklists are passed through geographically and temporally explicit filters to flag observations that are unexpected for any species in a particular state/province or month (Bonter and Cooper, 2012). The flagging system takes into account the FeederWatch protocol which instructs that participants record the maximum number of each species in view simultaneously. Because the territorial and flocking behavior of species limits the maximum number of each species that is likely to be viewed in a single location at the same time, the system filters were set to trigger a flag if the count reported exceeded three standard deviations from the mean for each species/state or province combination. Count limits were originally calculated based on FeederWatch data submitted prior to the 2006 season and have been manually adjusted over time (e.g., to allow for range expansions). Therefore, the flagging system is not only triggered by a species reported outside of its typical geographic range (e.g., 1 Verdin, Auriparus flaviceps, in Maine), but also by unusually high counts (e.g., 30 Black-capped Chickadees, Poecile atricapillus) and by species rarely seen in the context of backyard bird feeding (e.g., waterfowl and migratory warblers). Over time the flagging system has become more sophisticated. Since 2014, a real-time data entry trigger has been used to flag suspect observations, whereby the participant entering the count is immediately asked to review and confirm that their entry is correct. This provides an opportunity for participants to correct typographical errors or identification mistakes before they are entered into the database. If the participant chooses to enter their flagged observation into the database, it is automatically entered into the manual review system to be checked by an expert reviewer before being accepted as valid, corrected, or left flagged as an unexpected observation. Flagged observations are identified in the database as “0” in the VALID field and their status in the review process is described using a combination of the VALID field and the REVIEWED field as defined here:
VALID = 0; REVIEWED = 0; Interpretation: Observation triggered a flag by the automated system and awaits the review process. Note that such observations should only be used with caution.
VALID = 0; REVIEWED = 1; Interpretation: Observation triggered a flag by the automated system and was reviewed; insufficient evidence was provided to confirm the observation. Note that such observations should not be used for most analyses.
VALID = 1; REVIEWED = 0; Interpretation: Observation did not trigger the automatic flagging system and was accepted into the database without review.
VALID = 1; REVIEWED = 1; Interpretation: Observation triggered the flagging system and was approved by an expert reviewer.
The decisions of expert reviewers are based on a knowledge of bird biology and supporting information from the participant in the form of a description, photo, or confirmation that they are following the counting protocol correctly. All reports irrespective of their VALID or REVIEWED status are included in the full dataset, because incorrect identifications may themselves be of interest to researchers. For example, this dataset could be used to study longitudinal changes through time in participant data collection accuracy. It is up to researchers to appropriately remove invalid and unreviewed sightings from their analysis. Note that the overall proportion of flagged records is small relative to the entire dataset; of the 34,074,558 observations submitted from 1988 to 2020, only 516,614 (1.52%) were flagged for review, and only 48,417 (0.14%) were permanently flagged following review due to lack of supporting evidence.
Undoubtedly, some of the presumed valid reports in the database involve incorrect identifications that have not triggered a flag (e.g., misidentification of one common species for another), or reports by participants who do not correctly follow the FeederWatch protocol but whose incorrect counts are within the range permitted by the filter system. Researchers may want to consider lumping similar-looking species in some analyses depending on their questions, for example Black-capped and Carolina Chickadees (Poecile carolinensis) in the areas where populations overlap and hybridize, or Cooper's and Sharp-shinned Hawks (Accipiter cooperii and A. striatus), which are difficult to distinguish throughout their ranges. Despite the fact that a dataset of this temporal and geographic scale must contain some imperfections, there is consistency in avian population trends found with FeederWatch and other indices of bird abundance (e.g., Christmas Bird Counts; Lepage and Francis, 2002). This suggests that unidentified errors do not drive broad patterns in the data, and that FeederWatch data provide biologically meaningful insights.
Dataset
Dataset Structure
There are two datasets that are the primary Project FeederWatch data: (1) the checklists (i.e., the bird counts) and (2) the site descriptions. The key data fields associated with these datasets are listed in Table 1, with a complete dictionary of data fields included with the raw data files in the open access data repository. The “data level” column in Table 1 defines levels of organization of the dataset, of which there are four levels: (1) “site level,” referring to fixed data associated with the site, or location, at which the observations are made (e.g., the latitude and longitude); (2) “season level,” which are site-level descriptors that may (or may not) change from one season to the next (e.g., number of feeders maintained), (3) “checklist level,” referring to variables shared across a single checklist (e.g., date and sampling effort), and (4) “observation level,” referring to aspects of an individual species count within a checklist (e.g., the number of Black-capped Chickadees observed). When combining raw data from the checklists and site descriptions, researchers should link datasets using location (LOC_ID) and year (PROJ_PERIOD_ID). The data are organized for easy incorporation into a occupancy modeling framework (Fiske and Chandler, 2011). Specifically, the site-level variables are static across seasons and equivalent to site-level covariates. Season-level variables are dynamic across seasons and equivalent to season-level covariates. The season-level also includes the year in which a series of checklists were made, equivalent to the primary sampling period. Checklist- and observation-level variables are equivalent to “visits” or “observations” using the occupancy modeling terminology in Fiske and Chandler (2011).
Data are either binary (e.g., whether or not cats are present at the site), categorical (e.g., the approximate depth of snow cover), continuous (e.g., the number of chickadees observed on a checklist), or a date, indicated by the “data type” column in Table 1. The data are either entered by participants (e.g., the number of suet feeders provided) or assigned automatically by the database (e.g., the unique LOC_ID for every location), indicated by the “data entry” column in the data dictionary housed with the raw data. Categorical variables entered by participants are constrained by drop-down menu options or check boxes at the time of data entry.
The dataset is stored with all observations of presence recorded, but observations of absence are not recorded. Because the FeederWatch protocol instructs participants to record all species seen within the count area, researchers can infer absence for any species of interest by assuming that if it was not reported on a particular checklist (i.e., a particular SUB_ID), it was not observed. It is necessary for researchers to zero-fill the data themselves for their species of interest. This zero-filling can be accomplished by extracting a list of unique checklists (SUB_ID values), filling the HOW_MANY field for the species of interest with zeros, then overwriting the zeros with actual counts for the species on the checklists (SUB_ID values) in which the species was observed.
Interpretation and Use
The content of most data fields is self-explanatory from Table 1, but there are a few details to be aware of when interpreting some fields. The latitude and longitude fields are identified with varying degrees of accuracy depending upon how participants submitted their data and how locations were estimated. Prior to 2000, all data were submitted on paper forms (identified as “paper” in the DATA_ENTRY_METHOD field) and all sites were given the latitude and longitude of the centroid of the ZIP code (United States) or postal code (Canada), and identified as “POSTCODE LAT/LONG LOOKUP” in the ENTRY_TECHNIQUE field. The online data entry system was developed in late 1999 and, since then, a series of mapping tools with varying degrees of location accuracy have been implemented, most of which tie into Google Maps Application Programming Interfaces (APIs). These systems are identified in the ENTRY_TECHNIQUE field. Researchers seeking high spatial accuracy should exclude sites created using the centroid of the ZIP/postal code (e.g., when linking observations to high resolution land cover and weather datasets). Locations are subject to some degree of error because participants are responsible for inputting their site location and any changes in that location over time (e.g., if the participant moves). However, participants are likely self-motivated to maintain the accuracy of their site location, because they themselves wish to accurately monitor their site's birds through time using the data outputs provided on the FeederWatch website.
While the data collection protocols have remained fixed over time, data entry methods have changed, with implications for data interpretation. Before 2004, the paper data forms had boxes that only accommodated values up to 9, 99, or 999 for some species (the maximum value allowed varied depending on the typical flocking behavior of the species). If the participant observed a larger number of a species than could be accommodated on the paper forms, then they recorded the maximum number permitted on the data form and marked the “Plus_Code” field as “1.” These observations should be interpreted with caution because there is no way to know the true number of birds observed by the participant.
In 2018, the Cornell Lab of Ornithology released a mobile phone application for FeederWatch data entry. The mode of data entry is documented in the DATA_ENTRY_METHOD field. The codes are continuously evolving with new releases of the web and mobile apps but should be self-explanatory and can be functionally distilled to the three modes of data entry (web vs. mobile vs. paper). Because the mobile app is a new development, we have not yet attempted to quantify any potential differences in observations submitted using the mobile vs. web-based apps.
Previous research clearly demonstrates the importance of including sampling effort in analyses of FeederWatch data (e.g., Zuckerberg et al., 2011; Prince and Zuckerberg, 2014; Greig et al., 2017). There are two measures of effort within the dataset. The EFFORT_HRS_ATLEAST field records a 4-level categorical measure of observation effort (< 1, 1–4, 4–8, > 8 h). The second measure of effort divides the 2-day observation period into 4 half days, with the observer recording whether or not they observed their feeders during each of the four half-day periods. The series of four fields, labeled DAY1_AM, DAY1_PM, DAY2_AM, DAY2_PM, is often aggregated into a derived metric of the number of half-days that the participant spent observing during one checklist. Typically, the greater the sampling effort, the greater the number of species and individuals observed (Figure 1C).
Researchers may want to consider using occupancy modeling frameworks (e.g., Fiske and Chandler, 2011) when analyzing FeederWatch data because the data structure is well-suited to this form of analysis. Occupancy modeling allows for inferences about both presence/absence, abundance, and behavior. For example, finding complementary patterns in occupancy and detectability for a species across some environmental gradient may suggest changes in abundance (e.g., Zuckerberg et al., 2011). However, finding contrasting patterns in occupancy and detectability over an environmental gradient may suggest changes in behavior (e.g., Greig et al., 2017). As always, researchers should interpret data with care and within the context of the biological system being studied. Other modeling approaches can also be appropriate, such as generalized linear mixed models (GLMMs) because of the repeated counts from the same locations (e.g., Bonter and Harvey, 2008), as well as general algebraic modeling system (GAMS) approaches.
Data Access
Raw data from 1989-present are available in the Mendeley data repository with the most permissive open access level (doi: 10.17632/cptx336tt5.1). Data are also available with open access from the FeederWatch website maintained by the Cornell Lab of Ornithology (https://feederwatch.org/explore/raw-dataset-requests/). FeederWatch is an ongoing program and future data updates will be added to the Cornell Lab of Ornithology website. Data are updated annually around June 1.
Data Availability Statement
The datasets presented in this study can be found in online repositories. The names of the repository/repositories and accession number(s) can be found at: https://data.mendeley.com/datasets/cptx336tt5/1.
Ethics Statement
The animal study was reviewed and approved by Cornell University Institutional Animal Care and Use Committee (protocol #2008-0083).
Author Contributions
DB conceptualized the manuscript, prepared and revised the presentation of raw data, and revised the manuscript. EG conceptualized the manuscript, wrote and revised the manuscript, and revised the presentation of raw data. All authors contributed to the article and approved the submitted version.
Funding
Project FeederWatch was primarily supported by fees paid by project participants to the Cornell Lab of Ornithology and Birds Canada. Support is also provided by these two host institutions.
Conflict of Interest
The authors declare that the research was conducted in the absence of any commercial or financial relationships that could be construed as a potential conflict of interest.
Acknowledgments
We thank Dr. Erica Dunn for her vision in founding the project, and the more than 70,000 participants who have contributed their time, observations, and financial support since Project FeederWatch began. Special thanks to Kerrie Wilcox, Denis LePage, and Danielle Ethier (Birds Canada), and Anne Marie Johnson, Holly Faulkner-Grant, Wesley Hochachka, and Lisa Larson (Cornell Lab of Ornithology).
References
Bock, C. E., and Root, T. L. (1981). The christmas bird count and avian ecology. Stud. Avian Biol. 6, 17–23.
Bonney, R., Cooper, C. B., Dickinson, J., Kelling, S., Phillips, T., Rosenberg, K. V., et al. (2009). Citizen science: a developing tool for expanding science knowledge and scientific literacy. Bioscience 59, 977–984. doi: 10.1525/bio.2009.59.11.9
Bonter, D. N., and Cooper, C. B. (2012). Data validation in citizen science: a case study from project FeederWatch. Front. Ecol. Environ. 10:305307. doi: 10.1890/110273
Bonter, D. N., and Harvey, M. G. (2008). Winter survey data reveal rangewide decline in evening grosbeak populations. Condor 110, 376–381. doi: 10.1525/cond.2008.8463
Bonter, D. N., Zuckerberg, B., and Dickinson, J. L. (2010). Invasive birds in a novel landscape: habitat associations and effects on established species. Ecography 33, 494–502. doi: 10.1111/j.1600-0587.2009.06017.x
Dunn, E. H. (1993). Bird mortality from striking residential windows in winter. J. Field Ornithol. 64, 302–309.
Dunn, E. H. (2019). Dynamics and population consequences of irruption in the Red-breasted Nuthatch (Sitta canadensis). Auk 136:2.
Fiske, I., and Chandler, R. (2011). Unmarked: an R package for fitting hierarchical models of wildlife occurrence and abundance. J. Stat. Softw. 43, 1–23. doi: 10.18637/jss.v043.i10
Greig, E. I., Wood, E. M., and Bonter, D. N. (2017). Winter range expansion of a hummingbird is associated with urbanization and supplementary feeding. Proc. R. Soc. B 284:20170256. doi: 10.1098/rspb.2017.0256
Hartup, B. K., Dhondt, A. A., Sydenstricker, K., Hochachka, W. M., and Kollias, G. V. (2001). Host range and dynamics of mycoplasmal conjunctivitis among birds in North America. J. Wildlife Dis. 37, 72–81. doi: 10.7589/0090-3558-37.1.72
Haywood, B. K., Parrish, J. K., and Dolliver, J. (2016). Place-based and data-rich citizen science as a precursor for conservation action. Conserv. Biol. 30, 476–486. doi: 10.1111/cobi.12702
Kelling, S., Johnston, A., Hochachka, W. M., Iliff, M., Fink, D., Gerbracht, J., et al. (2015). Can observation skills of citizen scientists be estimated using species accumulation curves? PLoS ONE 10:e0139600. doi: 10.1371/journal.pone.0139600
Kullenberg, C., and Kasperowski, D. (2016). What is citizen science? – A scientometric meta- analysis. PLoS ONE 11:e0147152. doi: 10.1371/journal.pone.0147152
Leighton, G. M., Lees, A. C., and Miller, E. T. (2018). The hairy-downy game revisited: an empirical test of the interspecific social dominance mimicry hypothesis. Anim. Behav. 137, 141–148. doi: 10.1016/j.anbehav.2018.01.012
Lepage, D., and Francis, C. M. (2002). Do feeder counts reliably indicate bird population changes? 21 years of winter bird counts in Ontario, Canada. Condor 104, 255–270. doi: 10.1093/condor/104.2.255
Link, W. A., and Sauer, J. R. (1998). Estimating population change from count data: application to the North American breeding bird survey. Ecol. Appl. 8, 258–268. doi: 10.1890/1051-0761(1998)0080258:EPCFCD2.0.CO;2
Loss, S. R., Loss, S. S., Will, T., and Marra, P. P. (2015). Linking place-based citizen science with large-scale conservation research: a case study of bird-building collisions and the role of professional scientists. Biol. Conserv. 184, 439–445. doi: 10.1016/j.biocon.2015.02.023
McCabe, J. D., Yin, H., Cruz, J., Radeloff, V., Pidgeon, A., Bonter, D. N., et al. (2018). Prey abundance and urbanization influence the establishment of avian predators in a metropolitan landscape. Proc. R. Soc. B 285:20182120. doi: 10.1098/rspb.2018.2120
Miller, E. T., Bonter, D. N., Eldermire, C., Freeman, B. G., Greig, E. I., Harmon, L. J., et al. (2017). Fighting over food unites the birds of North America in a continental dominance hierarchy. Behav. Ecol. 28, 1454–1463. doi: 10.1093/beheco/arx108
Prince, K., and Zuckerberg, B. (2014). Climate change in our backyards: the reshuffling of North America's winter bird communities. Glob. Chang. Biol. 21, 572–585. doi: 10.1111/gcb.12740
Wells, J. V., Rosenberg, K. V., Dunn, E. H., Tessaglia-Hymes, D. L., and Dhondt, A. A. (1998). Feeder counts as indicators of spatial and temporal variation in winter abundance of resident birds. J. Field Ornithol. 69, 577–586.
Keywords: birds, citizen science, occupancy modeling, place-based dataset, Project FeederWatch, supplementary feeding
Citation: Bonter DN and Greig EI (2021) Over 30 Years of Standardized Bird Counts at Supplementary Feeding Stations in North America: A Citizen Science Data Report for Project FeederWatch. Front. Ecol. Evol. 9:619682. doi: 10.3389/fevo.2021.619682
Received: 20 October 2020; Accepted: 02 March 2021;
Published: 31 March 2021.
Edited by:
Sven Schade, Joint Research Centre (JRC), ItalyReviewed by:
Lucy Bastin, Aston University, United KingdomEduardo Roberto Alexandrino, National Institute of Atlantic Forest (INMA), Brazil
Copyright © 2021 Bonter and Greig. This is an open-access article distributed under the terms of the Creative Commons Attribution License (CC BY). The use, distribution or reproduction in other forums is permitted, provided the original author(s) and the copyright owner(s) are credited and that the original publication in this journal is cited, in accordance with accepted academic practice. No use, distribution or reproduction is permitted which does not comply with these terms.
*Correspondence: Emma I. Greig, ZWlnOUBjb3JuZWxsLmVkdQ==
†These authors have contributed equally to this work