- 1Department of Computer Science, University of Vermont, Burlington, VT, United States
- 2Allen Discovery Center, Tufts University, Medford, MA, United States
- 3Wyss Institute for Biologically Inspired Engineering, Harvard University, Boston, MA, United States
One of the most useful metaphors for driving scientific and engineering progress has been that of the “machine.” Much controversy exists about the applicability of this concept in the life sciences. Advances in molecular biology have revealed numerous design principles that can be harnessed to understand cells from an engineering perspective, and build novel devices to rationally exploit the laws of chemistry, physics, and computation. At the same time, organicists point to the many unique features of life, especially at larger scales of organization, which have resisted decomposition analysis and artificial implementation. Here, we argue that much of this debate has focused on inessential aspects of machines – classical properties which have been surpassed by advances in modern Machine Behavior and no longer apply. This emerging multidisciplinary field, at the interface of artificial life, machine learning, and synthetic bioengineering, is highlighting the inadequacy of existing definitions. Key terms such as machine, robot, program, software, evolved, designed, etc., need to be revised in light of technological and theoretical advances that have moved past the dated philosophical conceptions that have limited our understanding of both evolved and designed systems. Moving beyond contingent aspects of historical and current machines will enable conceptual tools that embrace inevitable advances in synthetic and hybrid bioengineering and computer science, toward a framework that identifies essential distinctions between fundamental concepts of devices and living agents. Progress in both theory and practical applications requires the establishment of a novel conception of “machines as they could be,” based on the profound lessons of biology at all scales. We sketch a perspective that acknowledges the remarkable, unique aspects of life to help re-define key terms, and identify deep, essential features of concepts for a future in which sharp boundaries between evolved and designed systems will not exist.
“Can machines think?” This should begin with definitions of the meaning of the terms “machine” and “think.”
– Alan Turing, 1950
Introduction
Living things are amazing – they show resilience, purposeful action, unexpected complexity. They have true “skin in the game” – they actively care about what happens, and can be rewarded or punished by experience. They surprise us at every turn with their ingenuity, their wholism, and their resistance to naïve reductionist approaches to analysis and control. For these reasons, some (Varela and Maturana, 1972; Varela et al., 1974; Rosen, 1985; Nicholson, 2012, 2013, 2014, 2019) have argued against modern cell biology and bioengineering’s conceptions of cells as machines (Diaspro, 2004; Davidson, 2012; Kamm and Bashir, 2014). Are living things machines? Defining “life” has proven to be notoriously difficult, and important changes in how we view this basic term have been suggested as a means of spurring progress in the field (Fields and Levin, 2018, 2020; Mariscal and Doolittle, 2020). What is an appropriate definition of “machine,” and does it apply to all, some, or no living forms across the tree of life?
Although not unanimously accepted, a powerful view is that all scientific frameworks are metaphors (Honeck and Hoffman, 1980) and the question should be not one of philosophy but of empirical research: does a suitable machine metaphor apply sufficiently to biology to facilitate experimental and conceptual progress? Here we focus attention on common assumptions that have strongly divided organicist and mechanist thinkers with respect to the machine metaphor, and argue that stark classical linguistic and conceptual distinctions are no longer viable or productive. At the risk of making both sides of this debate unhappy, we put our cards on the table as follows. We see life from the organicist perspective (Gurwitsch, 1944; Goodwin, 1977, 1978, 2000; Ho and Fox, 1988; Gilbert and Sarkar, 2000; Solé and Goodwin, 2000; Belousov, 2008). We do not hold reductionist views of the control of life, and one of us (ML) has long argued against the exclusive focus on molecular biology as the only source of order in life (Pezzulo and Levin, 2015, 2016) and the importance of multiple lenses, including a cognitive one, on the problem of biological origins, causation, and biomedical interventions (Manicka and Levin, 2019; Levin, 2020b). However, as often happens, advances in engineering have overtaken philosophical positions, and it is important to re-examine the life-as-machine metaphor with a fair, up-to-date definition of “machine”. Our goal here is not to denigrate the remarkable properties of life by equating them with 18th and 19th century notions of machines. Rather than reduce the conception of life to something lesser, we seek to update and elevate the understanding of “machines,” given recent advances in artificial life, AI, cybernetics, and evolutionary computation. We believe this will facilitate a better understanding of both – living forms and machines, and is an essential step toward a near future in which functional hybridization will surely erase comfortable, classical boundaries between evolved and engineered complex systems.
Here, we make three basic claims. First, that the notion of “machine” often used to claim that living things are not machines tends to refer to an outdated definition of the term which simply no longer fits. Thus, we have the opportunity (and need) to update the definition of “machine” based on insights from the information, engineering, and life sciences toward a better understanding of the space of possible machines (Table 1). We challenge relevant communities to collaborate on a better, more profound definition that makes it clear which aspects fruitfully apply to biological research. Indeed, many other terms such as robot, program, etc. need to be updated in light of recent research trends: these existing concepts simply do not “carve nature at its joints” in the way that seemed obvious in the last century. Second, that progress in the science of machine behavior and in the bioengineering of tightly integrated hybrids between living things and machines breaks down the simplistic dualism of life vs. machine. Instead, we see a continuum of emergence, rational control, and agency that can be instantiated in a myriad of novel implementations, not segregating neatly into categories based on composition (protoplasm vs. silicon) or origin story (evolved vs. designed). Finally, we stress an emerging breakdown not only of distinctions in terminology but of disciplines, suggesting the merging of aspects of information sciences, physics, and biology into a new field whose subject is embodied computation in a very wide range of evolved, designed, and composite media at multiple scales.
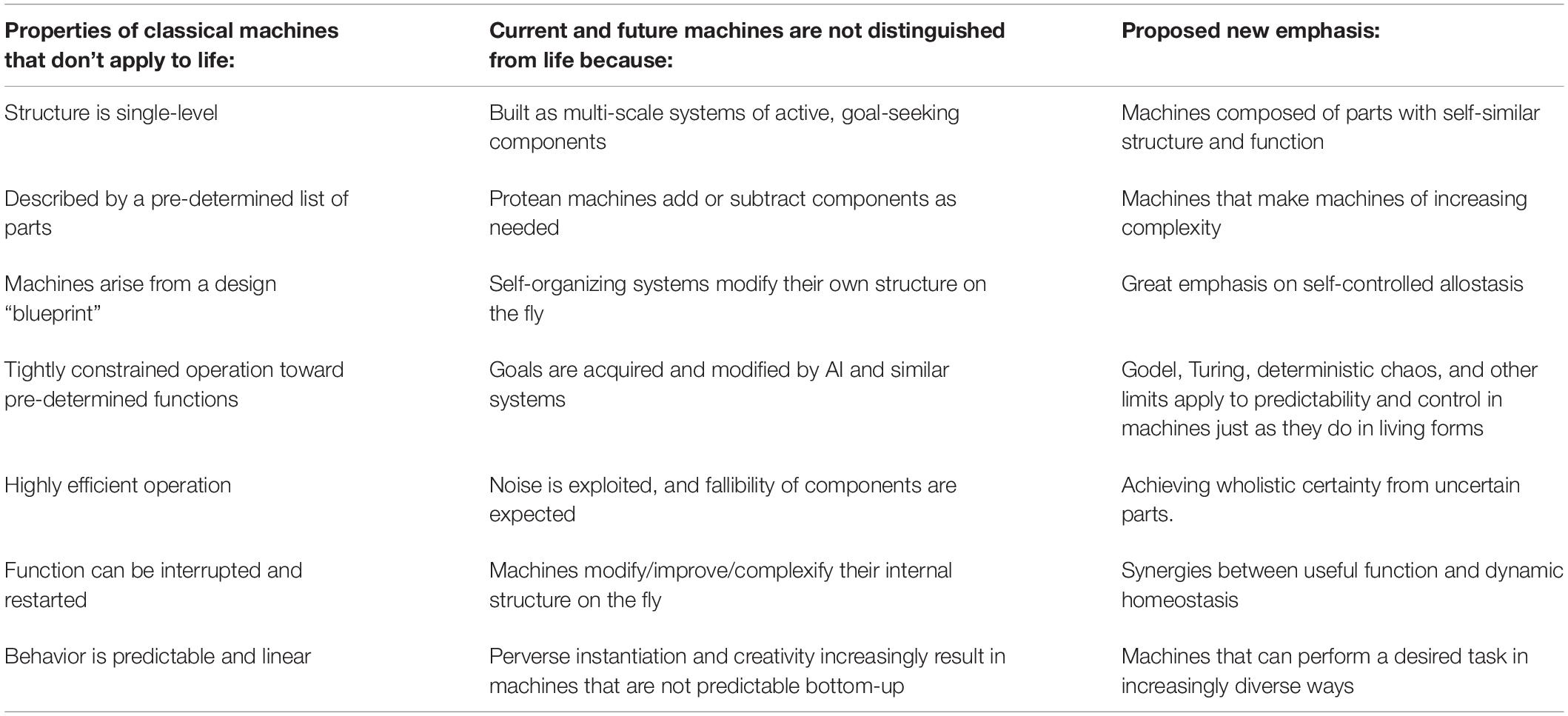
Table 1. A summary of past differences between machines and living systems and proposed updates that blur the boundaries.
What is Meant by “Machine”?
To claim that living things are not, or are, machines, it is first necessary to specify what is meant by a “machine” (Turing, 1950; Arbib, 1961; Lucas, 1961; Conrad, 1989; Davidson, 2012; Nicholson, 2012, 2013, 2014, 2019). We view the main aspects of a machine to refer to a device, constructed according to rational principles that enable prediction (to some threshold of accuracy) of their behavior at chosen scales. Machines constrain known laws of physics and computation to achieve specifiable functionality. In addition to this basic description, numerous properties are often assumed and then used to highlight differences from living forms. Let us consider some of these, to understand to what extent they are based on fundamental aspects of what is essential about the concept of machine, not merely contingent aspects due to historical limitations of technological capability. Each of the sections below focuses on one commonly voiced claim regarding the definitions of “machine,” which we think is in need of revision in light of advances in the science of machine behavior.
Machines Are Independent: Life Is Interdependent
The Turing machine, a theoretical construct of which all computers are physical instantiations, demonstrated that a clear demarcation exists between a machine and its environment: input and output channels mediate between them. This conception of machines also reaches back further, into the industrial revolution, when mechanical devices formed a new class of matter alongside those of inanimate, animate, and divine phenomena: from the outset, machines were considered as something apart, both from the natural world and from each other. In contrast, living systems are deeply interdependent with one another, simultaneously made and maker. Similarly, the Internet, and now the Internet of Things, is demonstrating that more useful machines can often be built by composing simpler machines into ever-more complex interdependencies. Modern physical machines are composed of vast numbers of parts manufactured by increasingly interconnected industrial ecologies, and most of the more complex parts have this same property.
Likewise, software systems have very long dependency trees: the hierarchy of support software that must be installed in order for the system in question to run. Software systems are often not considered machines, but rather something that can be executed by a particular class of machine: the Turing machine. However, modern computer science concepts have blurred this distinction between software and machine. A simple example is that of a virtual machine, which is software that simulates hardware different from that running the virtual machine software. This in turn raises the question of whether there is a distinction between simulating and instantiating a machine, but this deep question will be dealt with in forthcoming work.
Moreover, some machines are now becoming part of highly integrated novel systems with living organisms, for sensory augmentation (Sampaio et al., 2001), brain-machine interfaces (Danilov and Tyler, 2005; Shanechi et al., 2014), brain implants to manage epilepsy, paralysis, and other brain states (Shanechi et al., 2014; Alcala-Zermeno et al., 2020), performance augmentation (Suthana et al., 2012; Salvi et al., 2020), and internal physiological homeostatic devices [e.g., increasingly more intelligent devices to manage context-specific, homeostatic delivery of insulin, neurotransmitters, etc (Lee et al., 2019)]. Machines (such as optogenetics interfaces with machine learning components) can even be used to read memories or incept them directly into biological minds (Shen et al., 2019a,b; Vetere et al., 2019), bypassing traditional mechanisms of perception, memory formation, and communication, to access the core of what it means to be a sentient agent. These biohybrid machines require a constellation of particularly dense software and hardware support, maintenance and monitoring, since any cessation of function could injure or mortally endanger a human wearer. In their more exotic implementations, hybridized biological tissue (including brains) with electronics provide a plethora of possible constructs in which obviously alive components are tightly interweaved, in both structure and function, with machine components (Green and Kalaska, 2011; Wilson et al., 2013; Pais-Vieira et al., 2015). The function, cognition, and status of these hybrid systems make clear that no simple dichotomy can be drawn between life and machine.
Machines Are Predictable: Life Is Unpredictable
Intuitively, a useful machine is a reliable one. In contrast, living systems must be noisy and unpredictable: a reliable organism can be easily predated upon; a stationary species can be out-evolved. But, emerging technologies increasingly achieve reliable function by combining uncertain events in novel ways. Examples include quantum computers and machine learning algorithms peppered with stochastic events to ensure learning does not become trapped in partial solutions (Kingma and Ba, 2014). We are also now learning that unpredictability in the long run is often the signature of particularly powerful technologies. Indeed, the inability to predict the “killer app” for a new technology such as a quantum computer or driverless cars is often a signature of particularly disruptive technologies. The utility of surprising machines has historical roots: Gray Walter’s physical machines (Walter, 1950) and Braitenberg’s hypothetical machines were capable of startlingly complex behavior despite their extreme simplicity (Braitenberg, 1984). Today, robot swarms are often trained to exhibit useful “emergent behavior,” although the global behavior of the swarm may not be surprising, the irreducibility of swarm behavior to individual robot actions is a new concept to many roboticists (McLennan-Smith et al., 2020). Finally, the ubiquity of perverse instantiation – automatically trained or evolved robots often instantiate the requested, desired behavior in unexpected ways - in AI has been cited as a potentially useful way of designing machines (Lehman et al., 2020).
Nicholson (2019) defined a machine as having four clear specifications. For 21st century machines, it is becoming increasingly difficult to write down a clear set of specifications for them which spans all the possible ways in which they may change, and be changed by their increasingly complex environments. Instead, it is more useful to think about specifications for the algorithms that then build machines much more complex than the algorithms: canonical examples include the “specification” of the backpropagation of error algorithm that trains deep networks, and the traversal of a search space by genetic algorithms.
Machines Are Designed by Humans: Life Is Evolved
Almost all machines have a human provenance; whereas the very definition of a living system is that it arose from an evolutionary process. Somewhat surprisingly, economic theory provided one of the first intuition pumps for considering the non-human generators of machines: machines arise from the literal hands of human engineers but also the “invisible hand” of a free market; the latter set of pressures in effect “select,” without human design or forethought, which technologies proliferate (Beinhocker, 2020). More recently, evolutionary algorithms, a type of machine learning algorithm, have demonstrated that, among other things, jet engines (Yu et al., 2019), metamaterials (Zhang et al., 2020), consumer products (Zhou et al., 2020), robots (Brodbeck et al., 2018; Shah et al., 2020), and synthetic organisms (Kriegman et al., 2020) can be evolved rather than designed: an evolutionary algorithm generates a population of random artifacts, scores them against human-formulated desiderata, and replaces low-scoring individuals with randomly modified copies of the survivors. Indeed, the “middle man” has even been removed in some evolutionary algorithms by searching for novelty rather than selecting for a desired behavior (Lehman and Stanley, 2011). Thus, future agents are likely to have origin stories ranging across a very rich option space of combinations of evolutionary processes and intelligent design by humans and other machines.
The cost of evolving useful machines, rather than designing them by hand, is that that they are often inefficient. Like organisms, evolved machines inherently include many sub-functions exapted from sub-functions in their ancestral machines, or mutations that copy and differentiate sub-functions leads to several modules with overlapping functions. Nicholson (2019)’s third necessary feature of machines is that they are efficient: again, 21st century machines increasingly lack this property. The increased use of evolutionary dynamics by engineers, and the ability of both kinds of processes to give rise to highly adapted, complex systems makes it impossible to use evolved vs. designed as a clear demarcation between two classes of beings.
An especially powerful blow to the conceit that machines are the direct result of human ingenuity are machines that make machines. Mass production provided the first example of a machine — a factory – that could produce other machines. John von Neumann postulated theoretical machines that could make perfect copies of themselves, which in turn make copies of themselves, indefinitely, assuming a constant supply of raw building materials (von Neumann and Burks, 1966). Theory has been partly grounded in practice by rapid prototyping machines that print and assemble almost all of their own parts (Jones et al., 2011). Similarly, many are comfortable with the idea that the Internet, a type of machine, helps “birth” new social network applications. Those applications in turn connect experts together in new ways such that they midwife the arrival of brand new kinds of hardware and software. Indeed, most new technologies result from complex admixtures of human and machine effort in which economic and algorithmic evolutionary pressures are brought to bear. Indeed, one defensible metric of technological progress is the growing number of intermediate machine design/optimization layers sandwiched between human ingenuity and deployment of a new technology.
Life Is Hierarchical and Self-Similar: Machines Are Linearly Modular
Living systems exhibit similar structure and function at many different levels of organization. As one example of self-similar structure, at small scales, branching structures are not just self-similar but even fractal. Another example is the interdependence between hierarchical structure and function in the brain (Sporns et al., 2000). Even more important is self-similar function, in the sense of multi-scale competency, allostasis, or homeostasis (Vernon et al., 2015; Schulkin and Sterling, 2019): organelles, cells, organisms, and possibly species evolved adaptive mechanisms to recover when drawn away from agreeable environmental conditions or even placed in novel circumstances. Machines are typically assumed to be hierarchical and modular for sound engineering reasons, but self-similarity in machines is less obvious. Although fractality is currently under investigation in software (Semenov, 2020), circuit design (Chen et al., 2017), and metamaterials (De Nicola et al., 2020), it is conspicuously absent from other classes of machines. Thus, unlike the other features considered in this section, self-similarity remains a feature that, for now, does tend to distinguish living systems from machines. It is important to note, however, that this is not fundamental – there is no deep reason that prevents engineered artifacts from exploiting the deep, multi-scale organization of living organisms to improve problem-solving and robustness. Although many current machines are highly modular and efficient by design, machines produced by other machines increasingly exhibit differing amounts and types of modularity. Indeed artificially evolved neural networks (Clune et al., 2013) and robots (Bernatskiy and Bongard, 2017) often lack modularity unless it is directly selected for, and many exhibit inefficiencies caused by evolutionarily duplicated and differentiated sub-structures and sub-functions (Calabretta et al., 2000).
Autonomy at many scales is especially important with respect to function, not only structure (Pezzulo and Levin, 2016; Fields and Levin, 2017, 2020). Biological systems are holarchies in which each subsystem is competent in achieving specific goals (in the cybernetic, allostatic sense) despite changing local circumstances (Pezzulo and Levin, 2016). For example, a swarm of tadpoles organizes its swimming in a circular pattern to ensure efficient flow of nutrients past their gills. At the same time, individual tadpoles perform goal-directed behaviors and compete with each other, while their craniofacial organs re-arrange toward a specific target morphology of a frog [able to pursue this anatomical goal regardless of their starting configuration (Vandenberg et al., 2012)], their tissues compete for informational and nutritional resources (Gawne et al., 2020), and their individual cells maintain metabolic and homeostatic and transcriptional goal states. Such nested architecture of competing and cooperating units achieves unprecedented levels of robustness, plasticity, and problem-solving in novel circumstances (Levin, 2019; Levin, 2020a). It is also likely responsible for the remarkable evolvability of living forms, because such multi-scale competency flattens the fitness landscape: mutations have fewer deleterious effects if some of the changes they induce can be compensated by various subsystems, allowing their negative effects to be buffered while the positive effects accumulate. At present, this is a real difference between how we engineer machines and how living things are constructed; for now, defections of parts from the goals of the whole system (robot “cancer”) are rare, but this will not be the case for long. We expect near-future work to give rise to machines built on the principles of multi-scale competency in a fluid “society” of components that communicate, trade, cooperate, compete, and barter information and energy resources as do living components of an organism (Gawne et al., 2020).
Life Is Capable of Intelligence (And Free Will, Subjectivity, Consciousness, Agency, and Metacognition): Machines Are Not; Indeed, They Never Will
Nowhere does the specter of Cartesian dualism loom more prominently than in the debates about whether current machines possess any of the cognitive and affective features usually associated with higher animals, such as intelligence, agency, self-awareness, consciousness, metacognition, subjectivity, and so on (Cruse and Schilling, 2013). Indeed, the most intense debates focus on whether machines will ever be able to attain one or more of these internal states. As many have pointed out, the stronger the claim that higher cognition and subjectivity is only accessible to living systems, the stronger the evidence required to prove that living systems possess them. It is still strongly debated what aspects of the body organization are required for these capacities, or even whether such phenomena exist at all (Lyon, 2006; Bronfman et al., 2016; Dennett, 2017). Until such time as firm definitions of these terms is arrived at, claiming them as a point of demarcation between machines and life is an ill-defined exercise. Moreover, it is now clear that composite, hybrid creatures can be bioengineered with any desired combination of living cells (or whole brains) and real-time optical-electrical interfaces to machine-learning architectures (Grosenick et al., 2015; Newman et al., 2015; Pashaie et al., 2015; Roy et al., 2017). Because the living tissue (which houses the symbol grounding and true “understanding”) closely interacts with the machine learning components, forming a single integrated system, such chimeras reveal that there is no principled way to draw a crisp line between systems that have true subjectivity and those that are mere engineered systems.
Machines are increasingly occupying new spaces on the scale of persuadability, which ranges from low-level, physical control that has to be applied to change the function of a mechanical clock, to the use of experiences (positive or negative reinforcement), signals, messages, and arguments that one can use with agents of increasing cognitive sophistication. One way to formalize this distinction is through the relative amount of energy or effort used in an intervention compared to the change in the system’s behavior. Messages, unlike physical pushes, require relatively low energy input because they count on the receiving system to do a lot of the hard work. If one wants a 200 kg block of aluminum to move from point A to point B, one has to push it. If one wants a 200 kg robot to make the same journey, it may be sufficient to provide only a simple signal; and if one is dealing with a human or complex AI, one could even implement the move to occur in the future, in some specific context, by providing a rational reason to do it (via a low-energy message channel (Hoffmeyer, 2000; Pattee, 2001). Modern autonomous machines require increasingly low-energy interventions to produce useful work – a trend begun decades ago by developments in cybernetics. Indeed, in their increasing large-scale lability in the face of very subtle signals, they may get closer to the edge of chaos that is so prevalent in biology (Hiett, 1999; Kauffman and Johnsen, 1991; Mora and Bialek, 2011).
Machines Can Be Studied in a Reductionist Framework: Life Cannot
Until very recently, the very fact that machines could be rapidly disassembled into their component parts, repaired or improved, and then reassembled, was one of their primary advantages over living machines such as domesticated animals or human slaves. This modularity and hierarchy continues today in our most complex technologies, like state-of-the-art computer chips, which contain billions of transistors. Progress in circuit design now requires reaching into the quantum realm (Preskill, 2018), or enlisting DNA to store and transmit information (Chatterjee et al., 2017). Reductionist approaches in Artificial Intelligence are rapidly losing explanatory power as AI systems assume greater complexity. Considering the weight of a particular synaptic connection or a local neural cluster in a deep neural network provides little understanding of the machine’s behavior as a whole. Making progress in these domains may incur a cost of not being able to guarantee how local behavior will resolve into global behavior, like computation speed (a feature related to the predictability issues discussed above). Instead, AI methods may have to be enlisted to design such circuits. Ironically, the AI methods and their products, like neural networks, are both extremely resistant to reductionist analysis. As just one example, although the most common form of training neural networks, the backpropagation of error, is a simple mathematical technique, one of the co-founders of this method and other AI “insiders” have admitted to being baffled at its surprising effectiveness (Sejnowski, 2020). As for AI’s objects – neural networks – the very nature of their immense interconnectivity frustrates most attempts to summarize their global behavior by only referring to the individual behavior of their edge weights. Indeed, the fact that neural networks are modeled on biological nervous system principles makes it unsurprising that they would exhibit many biological features, including that of resistance to reductionist analysis. Many machines, especially swarms, exhibit behavior that requires the same techniques used to study cognition in biological systems (Beer, 2004, 2014, 2015; Swain et al., 2012; Pavlic and Pratt, 2013; Nitsch and Popp, 2014; Beer and Williams, 2015; Slavkov et al., 2018; Valentini et al., 2018), and even relatively straightforward machines are surprisingly resistant to analysis using today’s analytical tools (Jonas and Kording, 2016).
Today’s and future autonomous machines, like living things, will be subject to deterministic chaos (amplification of very small differences in initial conditions), inputs from their environment that radically affect downstream responses, highly complex interactions of a myriad diverse internal parts, and perhaps even quantum uncertainty (Thubagere et al., 2017). For the most sophisticated agents, a high level of analysis (in terms of motivations, beliefs, memories, valences, and goals) may be far more effective than bottom-up prediction approach – much as occurs in biology (Marr, 1982; Pezzulo and Levin, 2015, 2016).
If reductionist analysis is impossible for current and future machines, what remains? A consortium of social scientists, computer scientists and ethologists recently called for the creation of a new field, “machine behavior,” in which the best explanations of machines, and predictions of their likely behavior, are a combination of wholistic methods drawn from ethology, the social sciences, and cognitive science (Rahwan et al., 2019). As just one example, most modern deep learning analytic methods attempt to discover pathological holistic behavior in neural networks, such as bias. Then, these methods attempt to discover the likely root cause of that behavior and rectify it, such as de-biasing biased training data sets (Bolukbasi et al., 2016). Indeed in many cases, the most effective explanations of animal and human behavior stop far short of detailed neurological, chemical or small-scale physical phenomena (Noble, 2012). This call for wholistic thinking is partly intellectual and partly pragmatic: we require compact, falsifiable and predictive claims about how autonomous machines will act in the world, in close proximity to humans. Such claims provide a firm foundation for new knowledge, but also for new legislation, regulation, and social norms. Finally, the deeply social and, increasingly, biological components of modern machines further complicate reductionist thinking: extrapolating what a million people will do with a million plows, given knowledge of a plow, is tractable. Predicting what 3.8 billion people will do with 3.8 billion social media accounts1, or an equivalent number of brain-computer interactive devices, is not.
Nicholson (Nicholson, 2019) concluded his list of three necessary features for machines – specificity, constraint, and efficiency – with a fourth and final feature: non-continuity. By this, he meant that machines could be halted, disassembled, understood, repaired, and reassembled. As with the first three features, 21st century machines are increasingly resistant to reductionist manipulations as well as reductionist explanations (Guidotti et al., 2019; Rudin, 2019). Put differently, modern technologies only achieve utility when they are emplaced appropriately into the technosphere; it is difficult or impossible to describe their function independently of it.
Life Is Embodied: AIs Are Not
Above, we have considered increasingly untenable distinctions between machines and living systems. Another commonly voiced distinction inherited from Cartesian dualism, but one which is also rapidly deteriorating in the face of advances in technology, is that between embodied creatures and pure (software-based) AI. The staying power of this distinction is mostly due to its seeming intuitive nature: a living being (or robot) acts directly on the world, and is affected by it; “AI” are programs that run inside a computer and thus only impact the world indirectly. The sharp separation between AIs, whose essential nature is an algorithm (which can be run on many different kinds of hardware) seems categorically different than a living being which is defined by its particulars, in both mind and body. It is curious that a discipline only 70 years old should be so deeply cleaved along fault lines established at the outset of Western thought, millennia ago. Much ink has been spilled on this subject that we will not attempt to summarize here; instead, we will highlight a few thrusts within both disciplines that unintentionally or intentionally attempt to close this gap.
“Embodied AI” has come to be associated with efforts to run deep learning algorithms on autonomous robots (Savva et al., 2019). However, these methods can be seen as deepening rather than narrowing the brain/body distinction: In these approaches, the robot’s form is usually a fixed shell, previously designed by human engineers, controlled by the machine learning algorithm. In contrast, there is a small but growing literature on embodying intelligence directly into the body of the robot (Nakajima et al., 2015), and in machine learning methods that evolve robot bodies to enhance this and other forms of intelligence (Powers et al., 2020). A small but growing literature on robots capable of self-modeling also blurs the distinction between embodied robots and non-embodied AI methods. Attempts here focus on enabling a robot to model its own body (Bongard et al., 2006; Kwiatkowski and Lipson, 2019), and model unexpected changes to that body such as damage, using AI methods. In such systems, morphological change is occurring alongside mental changes, such as improved understanding of the robot’s current internal and external states (Kwiatkowski and Lipson, 2019). Likewise, an important distinction for the biosciences is between disciplines like zoology, which focus on very specific examples of life, and the study of deep principles of biological regulation [“life as it could be,” (Langton, 1995; Walker and Davies, 2013)] which, like AI software, can be implemented in a wide range of media.
Machines Have Clear Hardware/Software Distinctions: Life Does Not
One of the most enduring technological metaphors applied to organisms is that of DNA as software and cells as hardware. The metaphor sometimes considers transcription and translation as the interface between the two. In this guise, transcription and translation serve as the biological equivalent of finite automata, which translate code into physical changes imposed on the world. Biological nervous systems acquire a similar metaphor by extension, but here software is often considered to be electrical activity in the brain. Software, as the name implies, is usually restricted to “fluid” systems: chemical, electrical, or sub-atomic dynamics. Hardware is instead usually applied to macroscale, Newtonian, mechanical objects such as switches and relays in artificial systems, and physiology in living systems. Several advances in neuroscience and regenerative biology challenge the claim that biology never exploits the software/hardware distinction. For example, it has been argued that changes in blood flow in the brain can convey information (Moore and Cao, 2008), as does the function of astrocytes (Santello et al., 2019) and neurotransmitters (Ma et al., 2016). The non-electrical components of these structures and mechanisms complicate extending the software metaphor to encompass them. The hardware/software distinction is also blurring in technological systems: increasingly specialized hardware is being developed to support deep learning-specific algorithms (Haensch et al., 2018), and the physics of robot movement can be considered to be performing computation (Nakajima et al., 2015). DNA computing further complicates the hardware/software distinction: In one recent application (Chatterjee et al., 2017), DNA fragments simultaneously house the “software” of a given species yet also serve as logic gates and signal transmission lines, the atomic building blocks of computer hardware. Robots built from DNA (Thubagere et al., 2017) reduce the distinction yet further (Thubagere et al., 2017). Moreover, recent work on bioelectric control of regenerative setpoints showed that planarian flatworms contain voltage patterns (in non-neural cells) that are not a readout of current anatomy, but are a re-writable, latent pattern memory that will guide regenerative anatomy if the animal gets injured in the future (Levin et al., 2018). These patterns can now be re-written, analogous to false memory inception in the brain (Ramirez et al., 2013; Liu et al., 2014), resulting in worms that permanently generate 2-headed forms despite their completely wild-type genetic sequence (Durant et al., 2017). This demonstrates a sharp distinction between the machine that builds the body (cellular networks) and the data (stable patterns of bioelectric state) that these collective agents use to decide what to build. The data can be edited in real time, without touching the genome (hardware specification).
Most recently, the authors’ work on computer-designed organisms (Kriegman et al., 2020) calls this distinction into question from another direction. An evolutionary algorithm was tasked with finding an appropriate shape and tissue distribution for simulated cell clusters that yielded the fastest self-motile clusters in a virtual environment. A cell-based construction kit was made available to the algorithm, but it was composed of just two building units: Xenopus laevis epithelial and cardiac muscle cells. The fastest-moving designs were built by microsurgery using physical cells harvested from X. laevis blastulae. The resulting organism’s fast movement, with anatomical structure and behavior entirely different from that of normal frog larvae, was thus purely a function of its evolved, novel shape and tissue distribution, not neural control or genomic information. Such an intervention “reprograms” the wild type organism by forcing it into a novel, stable, bioelectric/morphological/behavioral state, all without altering the DNA “software.” This inverts the normal conception of programming a machine by altering its software but not its hardware.
Improving Definitions
Given the increasingly unsupportable distinctions between machines and life discussed above, we suggest that updated definitions of machine, robot, program, software, and hardware are in order. The very fact that many of these systems are converging makes delineating them from one another an almost paradoxical enterprise. Our goal is not to etch in stone precise new definitions, but rather to provide an update and starting point for discussion of terms that often are used without examination of their limitations. We emphasize aspects that we hope summarize important emerging structure and commonalities across these concepts. Wrestling with these concepts helps identify previously unasked research questions and unify research programs that previously were treated as distinct with respect to funding bodies, educational programs, and academic and industrial research environments.
Machine
Any system that magnifies and partly or completely automates an agent’s ability to effect change on the world. The system should be composed of parts several steps removed from raw materials and should be the result of a rational, or evolutionary (or both), design process. Importantly, a machine uses rationally discoverable principles of physics and computation, at whatever level (from molecular to cognitive), to achieve specific functions and is controllable by interventions either at the physical level or at the level of inputs, stimuli, or persuasion via messages that take advantage of its computational structure. The definition would include domesticated plants and animals (systems with rationally modified structure and behavior), and synthetic organisms. Machines often have exhibit information dynamics that enhance an agent’s ability to effect change on the world. The agent may be the entity who constructed the machine, or a third party. Similarly, the agent need not be self-aware or even sentient.
We propose that physicality is not a requirement. Physicality too easily becomes a seemingly obvious, Cartesian border between one class of phenomena and another. Of more interest are machines in which small-scale physical phenomena, such as quantum and electrodynamic forces in biological cells or microscale robots, influence macroscale behavior, such as whole-body motion or swarm intelligence. By removing the physicality requirement, a machine may be a machine learning algorithm that generates better machine learning algorithms or designs robots or synthetic organisms.
Robot
A machine capable of physical actions which have direct impacts on the world, and which can sense the repercussions of those actions, and is partly or completely independent of human action and intent. This definition is related to embodiment and situatedness, two previous pillars supporting the definition of robot (Pfeifer and Bongard, 2006). Crucially, the property of being a robot is not a binary one, but rather a spectrum – a continuum (independent of origin story or material implementation). The determinant of where a given system lands on the continuum is the degree of autonomous control evidenced by the system (Rosen, 1985; Bertschinger et al., 2008). A closely related continuum reflects the degree of persuadability of the system (Dennett, 1987). On one end of the continuum are highly mechanical systems that can only be controlled by direct physical intervention – micromanagement of outcome by “rewiring”. In the middle are systems that can be stimulated to change their activity – they can be sent signals, or motivated via reward or punishment experiences based on which they can make immediate decisions. At the far end are systems in which an effective means of communication and control is to alter the goals that drive their longer-term behavioral policies – they can be persuaded by informational messages encoding reasons, based on which they will change their goals. The important variables here are the causal closure of the system in its behavior (Rosen, 1974; Montevil and Mossio, 2015), and the amount of energy and intervention effort that need to be applied to get the system to make large changes in its function (the smaller the force needed to affect the system, the more sophisticated the robot). A continuous measure of the level of roboticism is required, to handle the growing class of hybrids of biological and mechano-electronic devices. For example, smart prosthetics, which are mostly under human control via muscle activation or thought processes, are less robotic than an autonomous car.
Program
A program is typically conceived as an abstract procedure that is multiply realizable: different physical systems can be found or constructed that execute the program. We see no need to alter this definition, except to state that execution need not be restricted to electrical activity in a computer chip or nervous tissue; chemical (Gromski et al., 2020) and mechanical (Silva et al., 2014) processes may support computation as well. However, a couple of aspects are important for discussing programs in biology. First, that programs do not need to be written by humans, or be a linear one-step-at-a-time procedure – the kinds of programs that (rightly) cause many to say that living things do not follow programs. The set of possible programs is much broader than that, and subsumes distributed, stochastic, evolved strategies such as carried out by nervous systems and non-neural cellular collectives. Indeed the question of whether something is a program or not is relative to a scale of biological organization. For example, genetic sequence is absolutely not a program with respect to anatomical shape, but it is a program with respect to protein sequence.
Software/Hardware
The common names for this technological pairing hint that material properties are what distinguish software from hardware; one can contrast the fluid flow of electrons through circuitry or photons through photonic circuits (Thomson et al., 2016) against the rigidity of metal boxes, vacuum tubes, and transistors. However, another, operational interpretation of their etymology is possible: it is harder to change hardware than software, but examples abound of both, radical structural change (Birnbaum and Alvarado, 2008; Levin, 2020a) and learning/plasticity at the dynamical system level that does not require rewiring (Biswas et al., 2021). Programmable matter (Hawkes et al., 2010) and shape changing soft robots (Shah et al., 2020) are but two technological disciplines investigating physically fluid technologies. In one study the assumption that changing hardware is hard was fully inverted: it was shown that a soft robot may recover from unexpected physical injury faster if it contorts its body into a new shape (a hardware change) rather than learning a compensating gait [a software change; (Kriegman et al., 2019)]. These distortions and inversions of the hardware/software distinction suggest that a binary distinction may not be useful at all when investigating biological adaptation or creating intelligent machines. However, a continuous variant may be useful in the biosciences as follows: a living system is software reprogrammable to the extent that stimuli (signals, experiences) can be used to alter its behavior and functionality, as opposed to needing physical rewiring (e.g., genome-editing, cellular transplantation, surgical interventions, etc.).
We suggest that the low-hanging fruit of specifiable, constrained, efficient, and fully predictable 20th century machines have now been picked. We as a society, and researchers in several fields, can (and must) now erase artificial boundaries to create machines that are more like the structures and processes that life exploits so successfully.
An Emerging Field: Re-Drawing the Boundaries
“Computer science is no more about computers than astronomy is about telescopes”
– Edsger Dijkstra
The differences that have been cited between living beings and machines are generally ones that can (and will) be overcome by incremental progress. And even if one holds out for some essential ingredient that, in principle, technology cannot copy, there is the issue of hybridization. Biological brains readily incorporate novel sensory-motor (Bach-y-Rita, 1967; Sampaio et al., 2001; Ptito et al., 2005; Froese et al., 2012; Chamola et al., 2020) and information-processing (Clark and Chalmers, 1998) functions provided by embedded electronic interfaces or machine-learning components that provide smart, closed-loop reward neurotransmitter levels (Bozorgzadeh et al., 2016) or electrical activity which can modulate cognition. Even if “true” preferences, motivations, goal-directedness, symbol grounding, and understanding are somehow only possible in biological media, we now know that hybrid functional systems can be constructed that are part living tissue and part (perhaps smart) electronics (Reger et al., 2000; DeMarse and Dockendorf, 2005; Hamann et al., 2015; von Mammen et al., 2016; Ando and Kanzaki, 2020), presumably conferring all of those features onto the system. No principled limits to functionalization between living systems (at any level of organization) and inorganic machinery are known; even if such limits exist, these ineffable components of living things will still tightly interact with engineered components through the interface of other biological aspects of cells and tissues that are already known to be closely interoperable with inorganic machine parts. Thus, we visualize a smooth, multi-axis continuum of beings being made of some percentage of parts that are uncontroversially biological and the remaining percentage of parts that are obviously machines (Figure 1). They are tightly integrated in a way that makes the whole system difficult to categorize, in the same way that molecular machines (e.g., ATPase motors or folding-programmed DNA strands) work together to make living beings that implement much more flexible, high-order behavior.
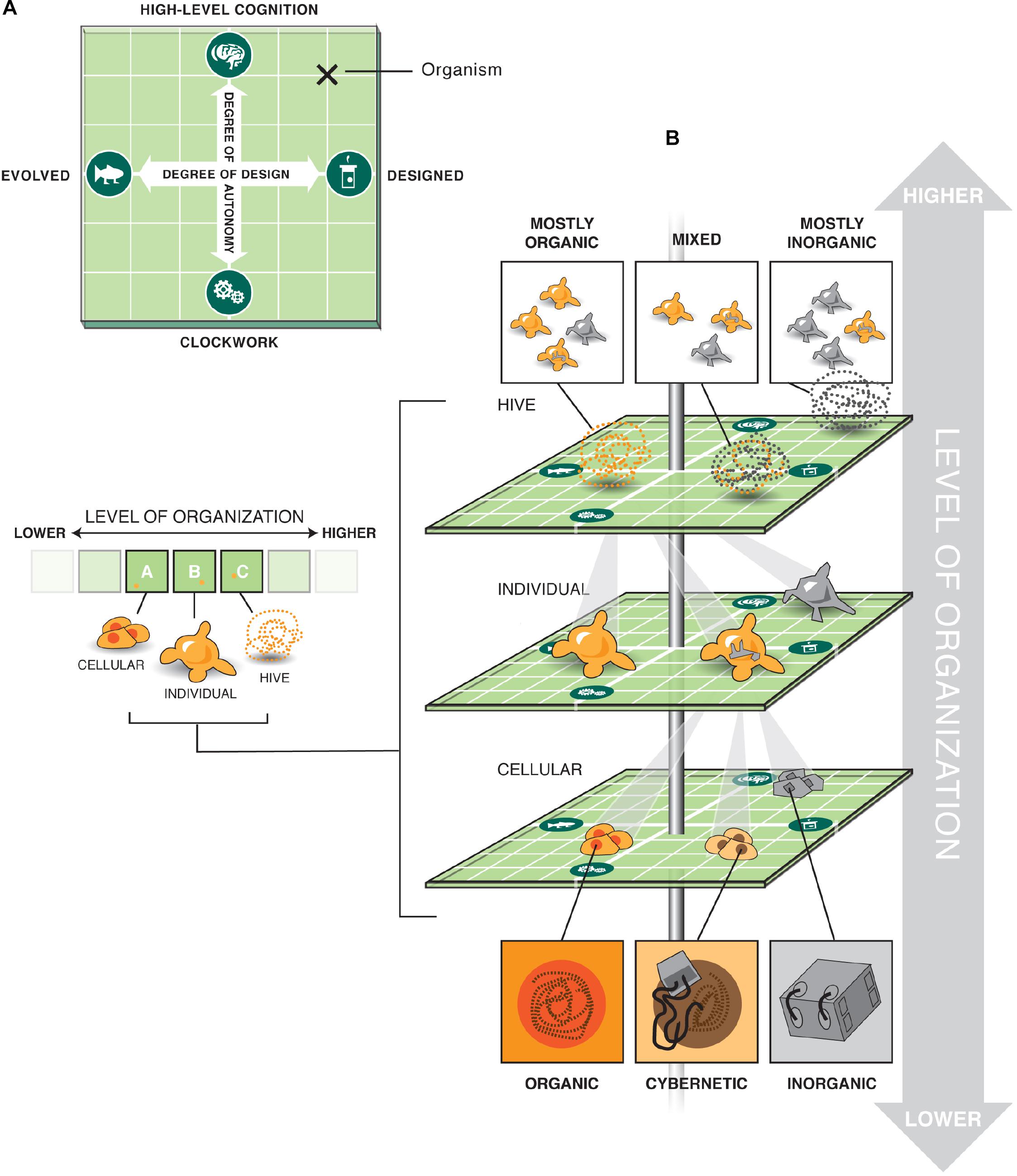
Figure 1. Multi-scale option space for possible living machines. (A) Two orthogonal axes define important aspects of any complex system: the degree of design vs. evolution that created it, and the degree of amount of autonomy it is able to implement. We suggest that both of these principal components are not binary categories (such as evolved vs. designed, mechanical vs. autonomous/cognitive) but rather continuous. Together, they form a 2-dimensional option space within which a great variety of possible agents can be placed. (B) Importantly, such an option space exists at each level of organization (for example, the familiar biological nested scales of cells, individual organisms, and hives/swarms), and each level comprising a complex agent could occupy a different position in the option space – the levels can be independent with respect to how much evolution, design, and cognition they involve. For example, a given system could be in one corner of the option space at the lowest level (e.g., contain cells that include highly predictable synthetic circuits), but be evolved and intelligent at the level of the individual, and at the same time be part of a swarm containing a mix of designed and evolved agents made up of different elements elsewhere on the option space in (A).
The near future will also surely contain systems in which biological and artificial parts and processes are intermixed across many levels of organization, and many orders of spatial and temporal scales. This could include a swarm composed of robots and organisms, and in which this admixture gradually changes over time to respond to slow time scale evolutionary pressures: the biological units reproduce and evolve, and the mechanical units self-replicate and evolve. Each individual in the swarm may itself be a cyborg capable of dynamically reconfiguring its biological and artificial components, while each of its cells may include more or less genetic manipulation. Where in such a system could a binary dividing line between life and artifice be placed?
Working as coherent wholes, such constructs make highly implausible a view of strict life/machine dualism, in the same way that the problem of explaining interaction vexed Descartes’ dualism between body and mind. Thus, the hard work of the coming decades will be to identify what, if any, are essential differences – are there fundamentally different natural kinds, or major transitions, in the continuum of fused biological and technological systems? At stake is a conceptual framework to guide basic research and applied engineering in the coming decades, which is essential given the exponential rate of progress in capabilities of altering and hybridizing the products of biology and computer engineering.
Familiar boundaries between disciplines may be more a relic of the history of science than optimal ways to organize our knowledge of reality. One possibility is that biology and computer science are both studying the same remarkable processes, just operating in different media. We suggest that the material implementation and the back-story of a given system are not sufficient information to reliably place it into a category of machine vs. living being, and indeed that those categories may not be discrete bins but rather positions in a multidimensional but continuous space. By asking hard questions about the utility of terminology whose distinct boundaries were calcified centuries ago, a number of advantages will be gained. The obvious trajectory of today’s technology will result in the presence of novel, composite creatures that in prior ages could be safely treated as fun sci-fi that didn’t have to be dealt with seriously. Updating our definitions and clearly articulating the essential differences between diverse types of systems is especially essential given the aspects of bioengineering and machine learning advances that cannot yet be foreseen.
The Interdisciplinary Benefits of a New Science of Machines
The biosciences have much to gain from a more nuanced, non-binary division between life and machine, and the emergence of the field of machine behavior. First, the fact that modern machines are multi-scale, surprising systems that are often as hard to predict and control as living systems (Man and Damasio, 2019; Rahwan et al., 2019) drives improvement in strategies for reverse-engineering, modeling, and multi-level analysis. This is exactly what is needed to break through complexity barriers facing regenerative medicine and developmental biology (Levin, 2020a). For example, solving the inverse problem in biomedical settings (what molecular-level features can be tweaked in order to achieve large-scale outcomes, such as forming an entire human hand via manipulation of gene and pathway activity in single cells) (Lobo et al., 2014; Pezzulo and Levin, 2016) will likely be advanced by the development of engineering approaches to harness noise, unpredictability, and top-down programming of goal-directed multiscale systems.
Second, grappling with issues of control, programmability, agency, and autonomy helps biologists identify and refine essential features of these concepts, freed from the frozen accidents of evolution and the history of biology, where contingent categories (e.g., “consisting of protoplasm”) offered distinctions that were easy to use in every-day life but misleading for a deeper scientific understanding. Asking how one can implement intrinsic motivation (Oudeyer and Kaplan, 2007), optimal control (Klyubin et al., 2005), and the ability to pursue and set goals in synthetic constructs (Kamm et al., 2018) will help reveal which aspects of living forms are the wellspring of these capacities and which are contingent details that do not matter.
Third, the engineering and information sciences offer many conceptual tools that should be tested empirically for their utility in driving novel work in basic biology, biomedicine, and synthetic bioengineering. Modular decomposition, software-level reprogrammability, embodied and collective intelligence (Sole et al., 2016), morphological computation (Fuchslin et al., 2013; Corucci et al., 2015), codes and encodings (Barbieri, 1998, 2018, 2019; Levin and Martyniuk, 2018), and much more. Finally, an inclusive, continuous view of life and machines frees the creative capacity of bioengineers, providing a much richer option space for the creation of novel biological systems via guided self-assembly (Kamm and Bashir, 2014; Kamm et al., 2018). Advances in this field even help address controversies within the biological sciences, such as whether behavior and intelligence are terms that can apply to plants (Applewhite, 1975; Trewavas, 2009; Garzon and Keijzer, 2011; Cvrckova et al., 2016; Calvo et al., 2017).
Likewise, the breaking down of artificial boundaries between the life and engineering sciences has many advantages for computer science and robotics. The first is bioinspiration. Since its cybernetic beginnings, researchers in Artificial Intelligence and robotics have always looked to biological forms and functions for how best to build adaptive and/or intelligent machines. Notable recent successes include convolutional neural networks, the primary engine of the AI revolution, which are inspired by the hierarchical arrangement of receptive fields in the primary visual cortex (Krizhevsky et al., 2017); deep reinforcement learning, the primary method of training autonomous cars and drones, inspired by behaviorism writ large (Mnih et al., 2015); and evolutionary algorithms, capable of producing a diverse set of robots (Bongard, 2013) or algorithms (Schmidt and Lipson, 2009) for a given problem. However, bioinspiration in technology fields is often ad hoc and thus successes are intermittent. What is lacking is a systematic method for distilling the wealth of biological knowledge down into useful machine blueprints and algorithm recipes, while filtering out proximate mechanisms that are overly reliant on the natural materials that nature had at hand. The products of research in biology (e.g., scientific papers and models) are often brimming with molecular detail such as specific gene names, and it is an important task for biologists to be able to abstract from inessential details of one specific organism and export the fundamental principles of each capability in such a way that human (or AI-based) engineers can exploit those principles in other media (Slusarczyk et al., 2012; Bacchus et al., 2013; Garcia and Trinh, 2019). Design of resilient, adaptive, autonomous robotics will benefit greatly from importing deep ideas discovered in the principles at work in the biological software that exploits noise, competition, cooperation, goal-directedness, and multi-scale competency.
Second, there is much opportunity for better integration across these fields, both in terms of the technology and the relevant ethics (Levin et al., 2020; Lewis, 2020). Consider the creative collective intelligence that will be embodied by the forthcoming integrated combination of human scientists, in silico evolution in virtual worlds, and automated construction of living bodies (Kamm and Bashir, 2014; Kamm et al., 2018; Kriegman et al., 2020; Levin et al., 2020), working together in a closed loop system as a discovery engine for the laws of emergent form and function. All biological and artificial materials and machines strike careful but different balances between many competing performance requirements. By drawing on advances in chemistry, materials science, and synthetic biology, a wider range of material, chemical and biotic building blocks are emerging, such as metamaterials and active matter (Silva et al., 2014; Bernheim-Groswasser et al., 2018; McGivern, 2019; De Nicola et al., 2020; Pishvar and Harne, 2020; Zhang et al., 2020), novel chemical compounds (Gromski et al., 2020), and computer-designed organisms (Kriegman et al., 2020). These new building blocks may in turn allow artificial or natural evolutionary pressures to design hybrid systems that set new performance records for speed, dexterity, metabolic efficiency, or intelligence, while easing unsatisfying metabolic, biomechanical and adaptive tradeoffs. Machine interfaces are also being used to connect brains into novel compound entities, enhancing performance and collaboration (Jiang et al., 2019). If the net is cast wider, and virtual reality, the Internet of Things, and human societies are combined such that they create and co-create one another, it may be possible to obtain the best of both, of all worlds. This would not be the purely mechanistic World-Machine that Newton originally envisioned, but closer to the transhumanist ideal of a more perfect union of technology, biology, and society.
Conclusion
Living cells and tissues are not really machines; but then again, nothing is really anything – all metaphors are wrong, but some are more useful than others. If we update the machine metaphor in biology in accordance with modern research in the science of machine behavior, it can help deepen conceptual understanding and drive empirical research in ways that siloed efforts based on prior centuries’ facile distinctions cannot. If we do not take this journey, we will not only be left mute in the face of numerous hybrid creatures in which these two supposedly different world interact tightly but will also have greatly limited our ability to design and control complex systems that could address many needs of individuals and society as a whole.
Are living things a computer (Wang and Gribskov, 2005; Bray, 2009)? It is a popular trope that humans naively seek to understand mind and life via the common engineering metaphors of the age - hydraulics, gears, electric circuits. However, this easy criticism, suggesting myopia and hyperfocus on each era’s shiny new technology, is mistaken. The reason such technologies are compelling is that they are showing us the space of what is possible, by exploring newly discovered laws of nature in novel configurations. Are cells like steam engines? Not overtly, but the laws of thermodynamics that steam engines helped us to uncover and exploit are as important for biology as they are for physics. Cells and tissues are certainly not like the computers many of us use today, but that critique misses the point. Today’s familiar computers are but a tiny portion of the huge space of systems that compute, and in this deeper, more important sense, living things are profitably studied with the deep concepts of computer science. Computer science offers many tools to help make more profound our understanding of the relationship between “minds and bodies” – physical structures that facilitate and constrain robustness, plasticity, memory, planning, intelligence, and all of the other key features of life.
It is now essential to re-draw (or perhaps erase) artificial boundaries between biology and engineering; the tight separation of disciplines is a hold-over from a past age, and is not the right way to carve nature by its joints. We live in a universe containing a rich, continuous option space of agents with which we can interact by re-wiring, training, motivating, signaling, communicating, and persuading. A better synergy between life sciences and engineering helps us to understand graded agency and nano-cognition across levels in biology, and create new instances (Pattee, 1979, 1982, 1989, 2001; Baluška and Levin, 2016). Indeed, biology and computer science are not two different fields; they are both branches of information science, working in distinct media with much in common. The science of behavior, applied to embodied computation in physical media that can be evolved or designed or both, is a new emerging field that will help us map and explore the enormous and fascinating space of possible machines across many scales of autonomy and composition. At stake is a most exciting future: where deep understanding of the origins and possible embodiments of autonomy help natural and synthetic systems reach their full potential.
Data Availability Statement
The original contributions presented in the study are included in the article/supplementary material, further inquiries can be directed to the corresponding author/s.
Author Contributions
Both authors listed have made a substantial, direct and intellectual contribution to the work, and approved it for publication.
Conflict of Interest
The authors declare that the research was conducted in the absence of any commercial or financial relationships that could be construed as a potential conflict of interest.
Acknowledgments
We thank the members of our labs and helpful members of the Twitterverse for many useful discussions and pointers to literature. We also thank Santosh Manicka and Vaibhav Pai for comments on early versions of this manuscript. ML gratefully acknowledges support of the Barton Family Foundation, the Elisabeth Giauque Trust, and the Templeton World Charity Foundation. JB wishes to thank the family of Cyril G. Veinott for their financial support, as well as the University of Vermont’s Office of the Vice President for Research.
Footnotes
- ^ The current estimate of people with social media accounts as of January 2020 (statista.com; bit.ly/2KSdA9U).
References
Alcala-Zermeno, J. J., Gregg, N. M., Van Gompel, J. J., Stead, M., Worrell, G. A., and Lundstrom, B. N. (2020). Cortical and thalamic electrode implant followed by temporary continuous subthreshold stimulation yields long-term seizure freedom: a case report. Epilepsy Behav. Rep. 14:100390. doi: 10.1016/j.ebr.2020.100390
Ando, N., and Kanzaki, R. (2020). Insect-machine hybrid robot. Curr. Opin Insect. Sci. 42, 61–69. doi: 10.1016/j.cois.2020.09.006
Applewhite, P. B. (1975). “Plant and animal behavior: an introductory comparison,” in Aneural Organisms in Neurobiology, ed. E. M. Eisenstein (New York: Plenum Press), 131–139. doi: 10.1007/978-1-4613-4473-5_9
Bacchus, W., Aubel, D., and Fussenegger, M. (2013). Biomedically relevant circuit-design strategies in mammalian synthetic biology. Mol. Syst. Biol. 9:691. doi: 10.1038/msb.2013.48
Bach-y-Rita, P. (1967). Sensory plasticity. applications to a vision substitution system. Acta Neurol. Scand. 43, 417–426.
Baluška, F., and Levin, M. (2016). On having no head: cognition throughout biological systems. Front. Psychol. 7:902.
Barbieri, M. (1998). The organic codes. the basic mechanism of macroevolution. Riv. Biol. 91, 481–513.
Barbieri, M. (2018). What is code biology? Biosystems 164, 1–10. doi: 10.1016/j.biosystems.2017.10.005
Barbieri, M. (2019). A general model on the origin of biological codes. Biosystems 181, 11–19. doi: 10.1016/j.biosystems.2019.04.010
Beer, R. D. (2004). Autopoiesis and cognition in the game of life. Artif. Life 10, 309–326. doi: 10.1162/1064546041255539
Beer, R. D. (2014). The cognitive domain of a glider in the game of life. Artif. Life 20, 183–206. doi: 10.1162/artl_a_00125
Beer, R. D. (2015). Characterizing autopoiesis in the game of life. Artif. Life 21, 1–19. doi: 10.1162/artl_a_00143
Beer, R. D., and Williams, P. L. (2015). Information processing and dynamics in minimally cognitive agents. Cogn. Sci. 39, 1–38. doi: 10.1111/cogs.12142
Beinhocker, E. D. (2020). The Origin of Wealth: Evolution, Complexity, and the Radical Remaking of Economics. Boston, MA: Harvard Business Press.
Belousov, L. V. (2008). “Our standpoint different from common.” (scientific heritage of alexander gurwitsch) [English]. Russ. J. Dev. Biol. 39, 307–315. doi: 10.1134/s1062360408050081
Bernatskiy, A., and Bongard, J. (2017). Choice of robot morphology can prohibit modular control and disrupt evolution. in Proceedings of the Fourteenth European Conference on Artificial Life. Switzerland: ECAL.
Bernheim-Groswasser, A., Gov, N. S., Safran, S. A., and Tzlil, S. (2018). Living matter: mesoscopic active materials. Adv. Mater. 30:e1707028.
Bertschinger, N., Olbrich, E., Ay, N., and Jost, J. (2008). Autonomy: an information theoretic perspective. Bio. Syst. 91, 331–345. doi: 10.1016/j.biosystems.2007.05.018
Birnbaum, K. D., and Alvarado, A. S. (2008). Slicing across kingdoms: regeneration in plants and animals. Cell 132, 697–710. doi: 10.1016/j.cell.2008.01.040
Biswas, S., Manicka, S., Hoel, E., and Levin, M. (2021). Gene regulatory networks exhibit several kinds of memory: quantification of memory in biological and random transcriptional networks. iScience 102131. doi: 10.1016/j.isci.2021.102131
Bolukbasi, T., Chang, K. W., Zou, J. Y., Saligrama, V., and Kalai, A. T. (2016). “Man is to computer programmer as woman is to homemaker? debiasing word embeddings,” in Proceedings of the 30th Annual Conference on Neural Information Processing Systems, (Barcelona: Spain), 4349–4357.
Bongard, J., Zykov, V., and Lipson, H. (2006). Resilient machines through continuous self-modeling. Science 314, 1118–1121. doi: 10.1126/science.1133687
Bozorgzadeh, B., Schuweiler, D. R., Bobak, M. J., Garris, P. A., and Mohseni, P. (2016). Neurochemostat: a neural interface soc with integrated chemometrics for closed-loop regulation of brain dopamine. IEEE Trans. Biomed. Circ. Syst. 10, 654–667. doi: 10.1109/tbcas.2015.2453791
Brodbeck, L., Hauser, S., and Iida, F. (2018). “Robotic invention: challenges and perspectives for model-free design optimization of dynamic locomotion robots,” in Robotics Research, eds A. Bicchi and W. Burgard (Cham: Springer), 581–596. doi: 10.1007/978-3-319-60916-4_33
Bronfman, Z. Z., Ginsburg, S., and Jablonka, E. (2016). The transition to minimal consciousness through the evolution of associative learning. Front. Psychol. 7:1954.
Calabretta, R., Nolfi, S., Parisi, D., and Wagner, G. P. (2000). Duplication of modules facilitates the evolution of functional specialization. Artif. Life 6, 69–84. doi: 10.1162/106454600568320
Calvo, P., Sahi, V. P., and Trewavas, A. (2017). Are plants sentient? Plant Cell Environ. 40, 2858–2869.
Chamola, V., Vineet, A., Nayyar, A., and Hossain, E. (2020). Brain-computer interface-based humanoid control: a review. Sensors 20:3620. doi: 10.3390/s20133620
Chatterjee, G., Dalchau, N., Muscat, R. A., Phillips, A., and Seelig, G. (2017). A spatially localized architecture for fast and modular DNA computing. Nat. Nanotechnol. 12, 920–927. doi: 10.1038/nnano.2017.127
Chen, J. P., Rogers, L. G., Anderson, L., Andrews, U., Brzoska, A., and Coffey, A. (2017). Power dissipation in fractal AC circuits. J. Phys. Math. Theor. 50:325205. doi: 10.1088/1751-8121/aa7a66
Clune, J., Mouret, J. B., and Lipson, H. (2013). The evolutionary origins of modularity. Proc. Biol. Sci. 280:20122863. doi: 10.1098/rspb.2012.2863
Conrad, M. (1989). The brain-machine disanalogy. Biosystems 22, 197–213. doi: 10.1016/0303-2647(89)90061-0
Corucci, F., Cheney, N., Lipson, H., Laschi, C., and Bongard, J. C. (2015). “Material properties affect evolution’s ability to exploit morphological computation in growing soft-bodied creatures,” in Proceedings of The Fifteenth International Conference on the Synthesis and Simulation of Living Systems, (Cambridge: MIT press).
Cruse, H., and Schilling, M. (2013). How and to what end may consciousness contribute to action? attributing properties of consciousness to an embodied, minimally cognitive artificial neural network. Front. Psychol. 4:324.
Cvrckova, F., Zarsky, V., and Markos, A. (2016). Plant studies may lead us to rethink the concept of behavior. Front. Psychol. 7:622.
Danilov, Y., and Tyler, M. (2005). Brainport: an alternative input to the brain. J. Integr. Neurosci. 4, 537–550.
Davidson, L. A. (2012). Epithelial machines that shape the embryo. Trends Cell Biol. 22, 82–87. doi: 10.1016/j.tcb.2011.10.005
De Nicola, F., Puthiya Purayil, N. S., Miseikis, V., Spirito, D., Tomadin, A., and Coletti, C. (2020). Graphene plasmonic fractal metamaterials for broadband photodetectors. Sci. Rep. 10:6882.
DeMarse, T. B., and Dockendorf, K. P. (2005). Adaptive Flight Control with Living Neuronal Networks on Microelectrode Arrays. Piscataway, NJ: IEEE.
Dennett, D. C. (2017). From Bacteria to Bach and Back : the Evolution of Minds. New York: W.W. Norton & Company.
Diaspro, A. (2004). Introduction: a nanoworld under the microscope–from cell trafficking to molecular machines. Microsc. Res. Tech. 65, 167–168. doi: 10.1002/jemt.20137
Durant, F., Morokuma, J., Fields, C., Williams, K., Adams, D. S., and Levin, M. (2017). Long-term, stochastic editing of regenerative anatomy via targeting endogenous bioelectric gradients. Biophys. J. 112, 2231–2243. doi: 10.1016/j.bpj.2017.04.011
Fields, C., and Levin, M. (2017). Multiscale memory and bioelectric error correction in the cytoplasm–cytoskeleton-membrane system. Wiley Interdiscipl. Rev. Syst. Biol. Med. 10:e1410–n/a. doi: 10.1002/wsbm.1410
Fields, C., and Levin, M. (2018). Are planaria individuals? what regenerative biology is telling us about the nature of multicellularity. Evol. Biol. 45, 237–247. doi: 10.1007/s11692-018-9448-9
Fields, C., and Levin, M. (2020). Scale-free biology: integrating evolutionary and developmental thinking. BioEssays 42:e1900228. doi: 10.1002/bies.201900228
Froese, T., McGann, M., Bigge, W., Spiers, A., and Seth, A. K. (2012). The enactive torch: a new tool for the science of perception. Ieee T Haptics 5, 365–375. doi: 10.1109/TOH.2011.57
Fuchslin, R. M., Dzyakanchuk, A., Flumini, D., Hauser, H., Hunt, K. J., and Luchsinger, R. (2013). Morphological computation and morphological control: steps toward a formal theory and applications. Artif. Life 19, 9–34.
Garcia, S., and Trinh, C. T. (2019). Modular design: implementing proven engineering principles in biotechnology. Biotechnol. Adv. 37:107403. doi: 10.1016/j.biotechadv.2019.06.002
Garzon, P. C., and Keijzer, F. (2011). Plants: adaptive behavior, root-brains, and minimal cognition. Adapt. Behav. 19, 155–171. doi: 10.1177/1059712311409446
Gawne, R., McKenna, K. Z., and Levin, M. (2020). Competitive and coordinative interactions between body parts produce adaptive developmental outcomes. BioEssays 42:e1900245. doi: 10.1002/bies.201900245
Gilbert, S. F., and Sarkar, S. (2000). Embracing complexity: organicism for the 21st century. Dev. Dyn. 219, 1–9. doi: 10.1002/1097-0177(2000)9999:9999<::AID-DVDY1036>3.0.CO;2-A
Goodwin, B. C. (1978). A cognitive view of biological process. J. Soc. Biol. Struct. 1, 117–125. doi: 10.1016/S0140-1750(78)80001-3
Goodwin, B. C. (2000). The life of form. emergent patterns of morphological transformation. comptes rendus de l’Academie des sciences. Serie III Sci. de la vie 323, 15–21. doi: 10.1016/S0764-4469(00)00107-4
Green, A. M., and Kalaska, J. F. (2011). Learning to move machines with the mind. Trends Neurosci. 34, 61–75.
Gromski, P. S., Granda, J. M., and Cronin, L. (2020). Universal chemical synthesis and discovery with ‘the chemputer’. Trends Chem. 2, 4–12. doi: 10.1016/j.trechm.2019.07.004
Grosenick, L., Marshel, J. H., and Deisseroth, K. (2015). Closed-loop and activity-guided optogenetic control. Neuron 86, 106–139. doi: 10.1016/j.neuron.2015.03.034
Guidotti, R., Monreale, A., Ruggieri, S., Turin, F., Giannotti, F., and Pedreschi, D. (2019). A survey of methods for explaining black box models. ACM Comput. Surv. 51:93.
Haensch, W., Gokmen, T., and Puri, R. (2018). The Next Generation of Deep Learning Hardware: Analog Computing. Piscataway, NJ: IEEE.
Hamann, H., Wahby, M., Schmickl, T., Zahadat, P., Hofstadler, D., and Stoy, K. (2015). “flora robotica - mixed societies of symbiotic robot-plant bio-hybrids,” in Proceedings of the 2015 IEEE Symposium Series on Computational Intelligence, (Piscataway: IEEE), 1102–1109.
Hawkes, E., An, B., Benbernou, N. M., Tanaka, H., Kim, S., and Demaine, E. D. (2010). Programmable matter by folding. Proc. Natl. Acad. Sci. U.S.A. 107, 12441–12445.
Hiett, P. J. (1999). Characterizing critical rules at the ‘edge of chaos’. Bio. Syst. 49, 127–142. doi: 10.1016/S0303-2647(98)00039-2
Hoffmeyer, J. (2000). Code-duality and the epistemic cut. Ann. N. Y. Acad. Sci. 901, 175–186. doi: 10.1111/j.1749-6632.2000.tb06277.x
Honeck, R. P., and Hoffman, R. R. (1980). Cognition and Figurative Language. Hillsdale, N.J: L. Erlbaum Associates.
Jiang, L., Stocco, A., Losey, D. M., Abernethy, J. A., Prat, C. S., and Rao, P. N. (2019). BrainNet: a multi-person brain-to-brain interface for direct collaboration between brains. Sci. Rep. 9:6115. doi: 10.1038/s41598-019-41895-7
Jonas, E., and Kording, K. (2016). Could a neuroscientist understand a microprocessor? biooRxiv [preprint] doi: 10.1371/journal.pcbi.1005268
Jones, R., Haufe, P., Sells, E., Iravani, P., Olliver, V., Palmer, C., et al. (2011). RepRap - the replicating rapid prototyper. Robotica 29, 177–191. doi: 10.1017/S026357471000069X
Kamm, R. D., and Bashir, R. (2014). Creating living cellular machines. Ann. Biomed. Eng. 42, 445–459. doi: 10.1007/s10439-013-0902-7
Kamm, R. D., Bashir, R., Arora, N., Dar, R. D., Gillette, M. U., and Griffith, L. G. (2018). Perspective: the promise of multi-cellular engineered living systems. APL Bioeng. 2:040901.
Kauffman, S. A., and Johnsen, S. (1991). Coevolution to the edge of chaos: coupled fitness landscapes, poised states, and coevolutionary avalanches. J. Theor. Biol. 149, 467–505. doi: 10.1016/S0022-5193(05)80094-3
Klyubin, A. S., Polani, D., and Nehaniv, C. L. (2005). Empowerment: a Universal Agent-Centric Measure of Control. Piscataway, NJ: IEEE.
Kriegman, S., Blackiston, D., Levin, M., and Bongard, J. (2020). A scalable pipeline for designing reconfigurable organisms. Proc. Natl. Acad. Sci. U.S.A. 117, 1853–1859. doi: 10.1073/pnas.1910837117
Kriegman, S., Walker, S., Shah, D. S., Levin, M., Kramer-Bottiglio, R., and Bongard, J. (2019). Automated Shapeshifting for Function Recovery in Damaged Robots. Freiburg im Breisgau: Robotics: Science and Systems XV, 28.
Krizhevsky, A., Sutskever, I., and Hinton, G. E. (2017). ImageNet classification with deep convolutional neural networks. Commun. ACM 60, 84–90. doi: 10.1145/3065386
Kwiatkowski, R., and Lipson, H. (2019). Task-agnostic self-modeling machines. Sci. Robot. 4:eaau9354. doi: 10.1126/scirobotics.aau9354
Langton, C. G. (1995). Artificial life : an Overview. Cambridge, Mass: MIT Press. doi: 10.7551/mitpress/1427.001.0001
Lee, S. H., Piao, H., Cho, Y. C., Kim, S. N., Choi, G., and Kim, C. R. (2019). Implantable multireservoir device with stimulus-responsive membrane for on-demand and pulsatile delivery of growth hormone. Proc. Natl. Acad. Sci. U.S.A. 116, 11664–11672.
Lehman, J., and Stanley, K. O. (2011). “Novelty search and the problem with objectives,” in Genetic Programming Theory and Practice, eds R. Riola, E. Vladislavleva, and J. H. Moore (Berlin: Springer), 37–56.
Lehman, J., Clune, J., Misevic, D., Adami, C., Altenberg, L., and Beaulieu, J. (2020). The surprising creativity of digital evolution: a collection of anecdotes from the evolutionary computation and artificial life research communities. Artif. Life 26, 274–306.
Levin, M. (2019). The computational boundary of a “self”: developmental bioelectricity drives multicellularity and scale-free cognition. Front. Psychol. 10:2688. doi: 10.3389/fpsyg.2019.02688
Levin, M. (2020a). Life, death, and self: fundamental questions of primitive cognition viewed through the lens of body plasticity and synthetic organisms. Biochem. Biophys. Res. Commun. doi: 10.1016/j.bbrc.2020.10.077 Online ahead of print.
Levin, M. (2020b). The biophysics of regenerative repair suggests new perspectives on biological causation. BioEssays 42:e1900146. doi: 10.1002/bies.201900146
Levin, M., and Martyniuk, C. J. (2018). The bioelectric code: an ancient computational medium for dynamic control of growth and form. Biosystems 164, 76–93. doi: 10.1016/j.biosystems.2017.08.009
Levin, M., Bongard, J., and Lunshof, J. E. (2020). Applications and ethics of computer-designed organisms. Nat. Rev. Mol. Cell Biol. 21, 655–656. doi: 10.1038/s41580-020-00284-z
Levin, M., Pietak, A. M., and Bischof, J. (2018). Planarian regeneration as a model of anatomical homeostasis: recent progress in biophysical and computational approaches. Semin. Cell Dev. Biol. 87, 125–144. doi: 10.1016/j.semcdb.2018.04.003
Lewis, A. C. F. (2020). Where bioethics meets machine ethics. Am. J. Bioeth. 20, 22–24. doi: 10.1080/15265161.2020.1819471
Liu, X., Ramirez, S., and Tonegawa, S. (2014). Inception of a false memory by optogenetic manipulation of a hippocampal memory engram. Philos. Trans. R. Soc. Lond. B Biol. Sci. 369:20130142. doi: 10.1098/rstb.2013.0142
Lobo, D., Solano, M., Bubenik, G. A., and Levin, M. (2014). A linear-encoding model explains the variability of the target morphology in regeneration. J. R. Soc. 11:20130918.
Lucas, J. R. (1961). Minds, machines, and godel. Philosophy 36, 112–127. doi: 10.1017/S0031819100057983
Lyon, P. (2006). The biogenic approach to cognition. Cogn. Process. 7, 11–29. doi: 10.1007/s10339-005-0016-8
Ma, Z., Stork, T., Bergles, D. E., and Freeman, M. R. (2016). Neuromodulators signal through astrocytes to alter neural circuit activity and behaviour. Nature 539, 428–432.
Man, K., and Damasio, A. (2019). Homeostasis and soft robotics in the design of feeling machines. Nat. Mach. Intell. 1, 446–452. doi: 10.1038/s42256-019-0103-7
Manicka, S., and Levin, M. (2019). The cognitive lens: a primer on conceptual tools for analysing information processing in developmental and regenerative morphogenesis. Philos. Trans. R. Soc. Lond. B Biol. Sci. 374:20180369. doi: 10.1098/rstb.2018.0369
Mariscal, C., and Doolittle, W. F. (2020). Life and life only: a radical alternative to life definitionism. Synthese 197, 2975–2989. doi: 10.1007/s11229-018-1852-2
Marr, D. (1982). Vision : a Computational Investigation into the Human Representation and Processing of Visual Information. San Francisco: Freeman, W. H.
McGivern, P. (2019). Active materials: minimal models of cognition? Adapt. Behav. 28:105971231989174. doi: 10.1177/1059712319891742
McLennan-Smith, T. A., Roberts, D. O., and Sidhu, H. S. (2020). Emergent behavior in an adversarial synchronization and swarming model. Phys Rev. E 102:032607. doi: 10.1103/PhysRevE.102.032607
Mnih, V., Kavukcuoglu, K., Silver, D., Rusu, A. A., Veness, J., and Bellemare, M. G. (2015). Human-level control through deep reinforcement learning. Nature 518, 529–533.
Montevil, M., and Mossio, M. (2015). Biological organisation as closure of constraints. J. Theor. Biol. 372, 179–191. doi: 10.1016/j.jtbi.2015.02.029
Moore, C. I., and Cao, R. (2008). The hemo-neural hypothesis: on the role of blood flow in information processing. J. Neurophysiol. 99, 2035–2047. doi: 10.1152/jn.01366.2006
Mora, T., and Bialek, W. (2011). Are biological systems poised at criticality? J. Stat. Phys. 144, 268–302. doi: 10.1007/s10955-011-0229-4
Nakajima, K., Hauser, H., Li, T., and Pfeifer, R. (2015). Information processing via physical soft body. Sci. Rep. 5:10487.
Newman, J. P., Fong, M. F., Millard, D. C., Whitmire, C. J., Stanley, G. B., and Potter, S. M. (2015). Optogenetic feedback control of neural activity. Elife 4:e07192.
Nicholson, D. J. (2012). The concept of mechanism in biology. Stud. Hist. Philos. Biol. Biomed. Sci. 43, 152–163.
Nicholson, D. J. (2013). Organisms not equal machines. Stud. Hist. Philos. Biol. Biomed. Sci. 44, 669–678.
Nicholson, D. J. (2014). The machine conception of the organism in development and evolution: a critical analysis. Stud. Hist. Philos. Biol. Biomed. Sci. 48, 162–174. doi: 10.1016/j.shpsc.2014.08.003
Nicholson, D. J. (2019). Is the cell really a machine? J. Theor. Biol. 477, 108–126. doi: 10.1016/j.jtbi.2019.06.002
Nitsch, V., and Popp, M. (2014). Emotions in robot psychology. Biol. Cybern. 108, 621–629. doi: 10.1007/s00422-014-0594-6
Noble, D. (2012). A theory of biological relativity: no privileged level of causation. Interface Focus 2, 55–64. doi: 10.1098/rsfs.2011.0067
Oudeyer, P. Y., and Kaplan, F. (2007). What is intrinsic motivation? a typology of computational approaches. Front. Neurorobot. 1:6. doi: 10.3389/neuro.12.006.2007
Pais-Vieira, M., Chiuffa, G., Lebedev, M., Yadav, A., and Nicolelis, M. A. (2015). Building an organic computing device with multiple interconnected brains. Sci. Rep. 5:11869. doi: 10.1038/srep14937
Pashaie, R., Baumgartner, R., Richner, T. J., Brodnick, S. K., Azimipour, M., Eliceiri, K. W., et al. (2015). Closed-loop optogenetic brain interface. IEEE Trans. Biomed. Eng. 62, 2327–2337.
Pattee, H. H. (1979). The complementarity principle and the origin of macromolecular information. Biosystems 11, 217–226. doi: 10.1016/0303-2647(79)90013-3
Pattee, H. H. (1982). Cell psychology: an evolutionary approach to the symbol-matter problem. Cogn. Brain Theory 5, 325–341.
Pattee, H. H. (1989). The measurement problem in artificial world models. Biosystems 23, 281–289. doi: 10.1016/0303-2647(89)90036-1
Pattee, H. H. (2001). The physics of symbols: bridging the epistemic cut. Biosystems 60, 5–21. doi: 10.1016/S0303-2647(01)00104-6
Pavlic, T. P., and Pratt, S. C. (2013). “Superorganismic behavior via human computation,” in Handbook of Human COmputtion, ed. P. Michelucci (Arizona State University: Tempe). doi: 10.1007/978-1-4614-8806-4_74
Pezzulo, G., and Levin, M. (2015). Re-membering the body: applications of computational neuroscience to the top-down control of regeneration of limbs and other complex organs. Integr. Biol. 7, 1487–1517. doi: 10.1039/C5IB00221D
Pezzulo, G., and Levin, M. (2016). Top-down models in biology: explanation and control of complex living systems above the molecular level. J. R. Soc. Interface 13:20160555. doi: 10.1098/rsif.2016.0555
Pfeifer, R., and Bongard, J. (2006). How the Body Shapes the Way We Think: a New View of Intelligence. Cambridge, MA: MIT Press. doi: 10.7551/mitpress/3585.001.0001
Pishvar, M., and Harne, R. L. (2020). Foundations for soft, smart matter by active mechanical metamaterials. Adv. Sci. 7:2001384. doi: 10.1002/advs.202001384
Powers, J., Grindle, R., Kriegman, S., Frati, L., Cheney, N., et al. (2020). “Morphology dictates learnability in neural controllers,” in Proceedings of the ALIFE 2020: The 2020 Conference on Artificial Life, (Cambridge: MIT Press). doi: 10.1162/isal_a_00243
Preskill, J. (2018). Quantum computing in the NISQ era and beyond. Quantum 2:79. doi: 10.22331/q-2018-08-06-79
Ptito, M., Moesgaard, S. M., Gjedde, A., and Kupers, R. (2005). Cross-modal plasticity revealed by electrotactile stimulation of the tongue in the congenitally blind. Brain J. Neurol. 128, 606–614. doi: 10.1093/brain/awh380
Rahwan, I., Cebrian, M., Obradovich, N., Bongard, J., Bonnefon, J. F., and Breazeal, C. (2019). Machine behaviour. Nature 568, 477–486.
Ramirez, S., Liu, X., Lin, P. A., Suh, J., Pignatelli, M., and Redondo, R. L. (2013). Creating a false memory in the hippocampus. Science 341, 387–391.
Reger, B. D., Fleming, K. M., Sanguineti, V., Alford, S., and Mussa-Ivaldi, F. A. (2000). Connecting brains to robots: an artificial body for studying the computational properties of neural tissues. Artif. Life 6, 307–324. doi: 10.1162/106454600300103656
Rosen, R. (1974). Biological-systems as organizational paradigms. Int. J. Gen. Syst. 1, 165–174. doi: 10.1080/03081077408960769
Rosen, R. (1985). Anticipatory Systems : Philosophical, Mathematical, and Methodological Foundations. New York: Pergamon Press.
Roy, D. S., Kitamura, T., Okuyama, T., Ogawa, S. K., Sun, C., Obata, Y., et al. (2017). Distinct neural circuits for the formation and retrieval of episodic memories. Cell 170, 1000–1012 e19.
Rudin, C. (2019). Stop explaining black box machine learning models for high stakes decisions and use interpretable models instead. Nat. Mach. Intell. 1, 206–215. doi: 10.1038/s42256-019-0048-x
Salvi, C., Beeman, M., Bikson, M., McKinley, R., and Grafman, J. (2020). TDCS to the right anterior temporal lobe facilitates insight problem-solving. Sci. Rep. 10:946. doi: 10.1038/s41598-020-57724-1
Sampaio, E., Maris, S., and Bach-y-Rita, P. (2001). Brain plasticity: ‘visual’ acuity of blind persons via the tongue. Brain Res. 908, 204–207. doi: 10.1016/S0006-8993(01)02667-1
Santello, M., Toni, N., and Volterra, A. (2019). Astrocyte function from information processing to cognition and cognitive impairment. Nat. Neurosci. 22, 154–166. doi: 10.1038/s41593-018-0325-8
Savva, M., Kadian, A., Maksymets, O., Zhao, Y., Wijmans, E., and Jain, B. (2019). “Habitat: a platform for embodied ai research,” in Proceedings of the 2019 IEEE/CVF International Conference on Computer Vision (ICCV), (Piscataway, NJ: IEEE), 9339–9347.
Schmidt, M., and Lipson, H. (2009). Distilling free-form natural laws from experimental data. Science 324, 81–85. doi: 10.1126/science.1165893
Schulkin, J., and Sterling, P. (2019). Allostasis: a brain-centered, predictive mode of physiological regulation. Trends Neurosci. 42, 740–752. doi: 10.1016/j.tins.2019.07.010
Sejnowski, T. J. (2020). The unreasonable effectiveness of deep learning in artificial intelligence. Proc. Natl. Acad. Sci. U.S.A. 117, 30033–30038. doi: 10.1073/pnas.1907373117
Semenov, A. S. (2020). Essentials of Fractal Programming, Advances in Theory and Practice of Computational Mechanics. Singapore: Springer, 373–386. doi: 10.1007/978-981-15-2600-8_25
Shah, D. S., Powers, J. P., Tilton, L. G., Kriegman, S., Bongard, J., and Kramer-Bottiglio, R. (2020). A soft robot that adapts to environments through shape change. Nat. Mach. Intell. 3, 51–59. doi: 10.1038/s42256-020-00263-1
Shanechi, M. M., Hu, R. C., and Williams, Z. M. (2014). A cortical-spinal prosthesis for targeted limb movement in paralysed primate avatars. Nat. Commun. 5:3237. doi: 10.1038/ncomms4237
Shen, G., Dwivedi, K., Majima, K., Horikawa, T., and Kamitani, Y. (2019a). End-to-end deep image reconstruction from human brain activity. Front. Comput. Neurosci. 13:21.
Shen, G., Horikawa, T., Majima, K., and Kamitani, Y. (2019b). Deep image reconstruction from human brain activity. PLoS Comput. Biol. 15:e1006633. doi: 10.1371/journal.pcbi.1006633
Silva, A., Monticone, F., Castaldi, G., Galdi, V., Alu, A., and Engheta, N. (2014). Performing mathematical operations with metamaterials. Science 343, 160–163.
Slavkov, I., Carrillo-Zapata, D., Carranza, N., Diego, X., Jansson, F., Kaandorp, J., et al. (2018). Morphogenesis in robot swarms. Sci. Robot. 3:eaau9178. doi: 10.1126/scirobotics.aau9178
Slusarczyk, A. L., Lin, A., and Weiss, R. (2012). Foundations for the design and implementation of synthetic genetic circuits. Nat. Rev. Genet. 13, 406–420. doi: 10.1038/nrg3227
Solé, R. V., and Goodwin, B. C. (2000). Signs of Life : How Complexity Pervades Biology. New York: Basic Books.
Sole, R., Amor, D. R., Duran-Nebreda, S., Conde-Pueyo, N., Carbonell-Ballestero, M., and Montanez, R. (2016). Synthetic collective intelligence. Biosystems 148, 47–61. doi: 10.1016/j.biosystems.2016.01.002
Sporns, O., Tononi, G., and Edelman, G. M. (2000). Theoretical neuroanatomy: relating anatomical and functional connectivity in graphs and cortical connection matrices. Cereb Cortex 10, 127–141. doi: 10.1093/cercor/10.2.127
Suthana, N., Haneef, Z., Stern, J., Mukamel, R., Behnke, E., Knowlton, B., et al. (2012). Memory enhancement and deep-brain stimulation of the entorhinal area. N. Engl. J. Med. 366, 502–510.
Swain, D. T., Couzin, I. D., and Leonard, N. E. (2012). Real-time feedback-controlled robotic fish for behavioral experiments with fish schools. Proc. IEEE 100, 150–163. doi: 10.1109/JPROC.2011.2165449
Thomson, D., Zilkie, A., Bowers, J. E., Komljenovic, T., Reed, G. T., and Vivien, L. (2016). Roadmap on silicon photonics. J. Optics 18:073003.
Thubagere, A. J., Li, W., Johnson, R. F., Chen, Z., Doroudi, S., and Lee, Y. L. (2017). A cargo-sorting DNA robot. Science 357:eaan6558.
Trewavas, A. (2009). What is plant behaviour? Plant Cell Environ. 32, 606–616. doi: 10.1111/j.1365-3040.2009.01929.x
Turing, A. M. (1950). Computing machinery and intelligence. Mind 59, 433–460. doi: 10.1093/mind/LIX.236.433
Valentini, G., Moore, D. G., Hanson, J. R., Pavlic, T. P., Pratt, S. C., et al. (2018). “Transfer of information in collective decisions by artificial agents,” in Proceedings of the 2018 Conference on Artificial Life, (Cambridge, MA: MIT press), 641–648.
Vandenberg, L. N., Adams, D. S., and Levin, M. (2012). Normalized shape and location of perturbed craniofacial structures in the Xenopus tadpole reveal an innate ability to achieve correct morphology. Dev. Dyn. 241, 863–878. doi: 10.1002/dvdy.23770
Varela, F. G., Maturana, H. R., and Uribe, R. (1974). Autopoiesis: the organization of living systems, its characterization and a model. Biosystem 5, 187–196.
Varela, F., and Maturana, H. (1972). Mechanism and biological explanation. Philos. Sci. 39, 378–382. doi: 10.1086/288458
Vernon, D., Lowe, R., Thill, S., and Ziemke, T. (2015). Embodied cognition and circular causality: on the role of constitutive autonomy in the reciprocal coupling of perception and action. Front. Psychol. 6:1660. doi: 10.3389/fpsyg.2015.01660
Vetere, G., Tran, L. M., Moberg, S., Steadman, P. E., Restivo, L., and Morrison, F. G. (2019). Memory formation in the absence of experience. Nat. Neurosci. 22, 933–940.
von Mammen, S., Hamann, H., and Heider, M. (2016). “Robot gardens: an augmented reality prototype for plant-robot biohybrid systems,” in Proceedings of the 22nd ACM Conference on Virtual Reality Software and Technology, ed. E. Kruijff (New York, NY: Association for Computing Machinery), 139–142. doi: 10.1145/2993369.2993400
von Neumann, V., and Burks, A. W. (1966). Theory of Self-Reproducing Automata. Champaign, IL: University of Illinois Press.
Walker, S. I., and Davies, P. C. (2013). The algorithmic origins of life. J. R. Soc. Interface 10:20120869.
Wang, D., and Gribskov, M. (2005). Examining the architecture of cellular computing through a comparative study with a computer. J. R. Soc. Interface 2, 187–195. doi: 10.1098/rsif.2005.0038
Wilson, N. R., Schummers, J., Runyan, C. A., Yan, S. X., Chen, R. E., Deng, Y., et al. (2013). Two-way communication with neural networks in vivo using focused light. Nat. Protoc. 8, 1184–1203.
Yu, X., Wang, C., and Yu, D. (2019). Configuration optimization of the tandem cooling-compression system for a novel precooled hypersonic airbreathing engine. Energy Convers. Manag. 197:111827. doi: 10.1016/j.enconman.2019.111827
Zhang, T., Liu, Q., Dan, Y., Yu, S., Han, X., Dai, J., et al. (2020). Machine learning and evolutionary algorithm studies of graphene metamaterials for optimized plasmon-induced transparency. Opt Express 28, 18899–18916.
Keywords: biology, computer science, robot, artificial life, machine learning
Citation: Bongard J and Levin M (2021) Living Things Are Not (20th Century) Machines: Updating Mechanism Metaphors in Light of the Modern Science of Machine Behavior. Front. Ecol. Evol. 9:650726. doi: 10.3389/fevo.2021.650726
Received: 07 January 2021; Accepted: 22 February 2021;
Published: 16 March 2021.
Edited by:
Pedro Martinez, University of Barcelona, SpainCopyright © 2021 Bongard and Levin. This is an open-access article distributed under the terms of the Creative Commons Attribution License (CC BY). The use, distribution or reproduction in other forums is permitted, provided the original author(s) and the copyright owner(s) are credited and that the original publication in this journal is cited, in accordance with accepted academic practice. No use, distribution or reproduction is permitted which does not comply with these terms.
*Correspondence: Michael Levin, bWljaGFlbC5sZXZpbkB0dWZ0cy5lZHU=
†These authors have contributed equally to this work