- 1Centre for Integrative Ecology, School of Life and Environmental Sciences, Deakin University, Burwood, VIC, Australia
- 2Bio-Inspired Digital Sensing Lab, School of Media and Communication, RMIT University, Melbourne, VIC, Australia
- 3Department of Physiology and Neuroscience Program, Biomedicine Discovery Institute, Monash University, Clayton, VIC, Australia
- 4Centre de Recherches sur la Cognition Animale, Centre de Biologie Intégrative, Université de Toulouse, CNRS, UPS, Toulouse, France
- 5College of Animal Science (College of Bee Science), Fujian Agriculture and Forestry University, Fuzhou, China
- 6Institut Universitaire de France, Paris, France
- 7Monash Rural Health Churchill, Churchill, VIC, Australia
Angiosperms have evolved to attract and/or deter specific pollinators. Flowers provide signals and cues such as scent, colour, size, pattern, and shape, which allow certain pollinators to more easily find and visit the same type of flower. Over evolutionary time, bees and angiosperms have co-evolved resulting in flowers being more attractive to bee vision and preferences, and allowing bees to recognise specific flower traits to make decisions on where to forage. Here we tested whether bees are instinctively tuned to process flower shape by training both flower-experienced and flower-naïve honeybee foragers to discriminate between pictures of two different flower species when images were either normally configured flowers or flowers which were scrambled in terms of spatial configuration. We also tested whether increasing picture contrast, to make flower features more salient, would improve or impair performance. We used four flower conditions: (i) normally configured greyscale flower pictures, (ii) scrambled flower configurations, (iii) high contrast normally configured flowers, and (iv) asymmetrically scrambled flowers. While all flower pictures contained very similar spatial information, both experienced and naïve bees were better able to learn to discriminate between normally configured flowers than between any of the modified versions. Our results suggest that a specialisation in flower recognition in bees is due to a combination of hard-wired neural circuitry and experience-dependent factors.
Introduction
Angiosperms display a combination of olfactory and visual cues to attract or deter pollinators. For example, in terms of visual spatial cues, bee-flies prefer large, and dissected flower models (Johnson and Dafni, 1998), while beetles have a preference for large and circular “bowl-shaped” flowers (Dafni and Kevan, 1997). Different species of small bees prefer to visit small flowers presenting broken outlines while larger bee species preferentially visit large circular flowers (Dafni and Kevan, 1997). The specific spatial preferences of pollinators may thus drive the evolution of angiosperms and the phenotypes of their flowers and in turn, the coevolution of plants and pollinators (Giurfa et al., 1999a; Fenster et al., 2004, 2006; Lázaro and Totland, 2014; Gómez et al., 2016).
Flowers pollinated by bees often share a similar, centrally symmetric, star-like configuration (Dafni et al., 1997). Howard et al. (2019e, 2021) suggested that shape may be a cue used by bees when attracted to images of unknown insect-pollinated flowers, as opposed to bird-pollinated flowers when bees are confronted with a binary choice between these options. A preference for star-like flower configurations has been demonstrated in both eusocial (Lehrer et al., 1995; Dafni et al., 1997; Howard et al., 2019e) and non-eusocial bees (Howard et al., 2021).
Honeybees can rapidly learn to discriminate between flower signals and/or cues including scent, colour, shape, size, and symmetry (scent: Deisig et al., 2001; Vergoz et al., 2007; Giurfa and Sandoz, 2012; colour: Dyer and Chittka, 2004; Giurfa, 2004; Dyer and Neumeyer, 2005; Dyer et al., 2008; Dyer and Murphy, 2009; Dyer, 2012b; Avarguès-Weber and Giurfa, 2014; Sommerlandt et al., 2016; shape: Lehrer et al., 1995; de Ibarra and Giurfa, 2003; Morawetz et al., 2013; size: Avarguès-Weber et al., 2014; Howard et al., 2017a,b; symmetry: Giurfa et al., 1996). This learning fits their foraging lifestyle when considering that foraging bees must discriminate between flowers to make a choice on where to collect nectar and/or pollen. Furthermore, efficient decision making promotes optimal nutrition collection to help enable colony survival (Burns and Dyer, 2008).
Previously, we have reported initial evidence that experienced honeybees show significantly improved learning of configured flowers over scrambled versions (Dyer et al., 2013). Bees are highly sensitive to spatial configuration, including prioritising global configurations over local features when memorising stimuli (Avarguès-Weber et al., 2015). Bees are also able to use spatial configurations to categorise stimuli (Avarguès-Weber et al., 2010), and can use holistic processing, an advanced form of configural processing where the predictive power of certain features is enhanced by the way such features are arranged (Maurer et al., 2002; Avarguès-Weber et al., 2018). For example, considering human vision and face processing, if features like noses, eyes, and mouths are scrambled to different positions within a stimulus, then we are less accurate at processing the face, even though the same basic featural information is available (Tanaka and Farah, 1993; Collishaw and Hole, 2000; Maurer et al., 2002). Importantly, relying on spatial configurations to identify visual objects is thought to be advantageous, as spatial arrangements of features are robust to changes in luminosity, viewpoint and partial occlusion, and holistic processing in humans facilitates subtle discrimination between highly similar objects such as human faces (Tanaka and Farah, 1993; Maurer et al., 2002).
Interestingly, bees learn normal contrast flower pictures more quickly compared to highly contrasted pictures (black and white) (Dyer et al., 2013). This high contrast picture modification was consistent with frequent forms of parameterised stimulus presentation in experiments involving honey bee vision (Lehrer et al., 1995; Dafni and Kevan, 1997; Dafni et al., 1997; Horridge, 1997; Avarguès-Weber et al., 2011). However, recordings of contrast sensitivity functions for orientation sensitive neurons in honeybees show that neural responses increase for a low-contrast range, but saturate beyond intermediate contrast values. This result suggests that lower and intermediate contrast images may already induce efficient discrimination due to maximal activation of feature detectors in the visual brain (Yang and Maddess, 1997). The question of how completely flower-naïve bees may learn and process flower configurations is crucial to start unravelling the mechanisms of flower learning and discrimination by experienced foragers.
High-level performance in bees when processing and discriminating between flower stimuli could be explained by top-down processing due to intensive experience. Top-down processing is a form of information processing where prior learnt knowledge or experience is used to inform behaviour (Sarter et al., 2001). Interestingly, honeybees have demonstrated evidence of using top-down processing (Zhang and Srinivasan, 1994; Chittka and Niven, 2009). In a classic experiment by Zhang and Srinivasan (1994), honeybees initially failed to learn to discriminate three-dimensional camouflaged shapes against a similar patterned background; but when bees were provided with prior experience with salient three-dimensional shapes they could subsequently solve the more complex camouflaged problem. Thus, bees demonstrated a capacity to use acquired information in the brain to improve subsequent discrimination performance. This is opposed to automatic and unidirectional reactions to stimuli inputs without using other knowledge acquired by the brain, which is described as a bottom-up way of processing. Bottom-up processing could potentially explain flower discrimination if bees possess specific cue detectors or filters in the early stage of neuronal visual processing that respond preferentially to key flower cues using a matched filtering type process (Wehner, 1987). In this case, bees should also be efficient in discriminating normally configured flowers within a short number of learning events. For example, honeybees perform well when learning salient colours within 3–7 visits to only a target colour (Menzel, 1967). Matched filter mechanisms can be easily exploited by deceptive species (Warrant, 2016), and thus animals may also require a capacity to learn to be flexible. In this scenario, a choice strategy driven by matched filters, which may underlie initial preferences by naïve animals, could easily been overridden by experience, which may lead to different choices. Considering colour processing in honeybees, fine colour differences require differential conditioning to both target and distractor stimuli for greater than 15 choices (Giurfa, 2004; Reser et al., 2012; Sommerlandt et al., 2016) to learn how to avoid deceptive options, and such learning promotes changes in the higher levels of visual processing in a bee brain and the development of long-term memories (Dyer and Chittka, 2004; Dyer and Garcia, 2014; Sommerlandt et al., 2016). Thus, simple bottom-up or more advanced top-down type processes can be approached by testing stimuli that require differential conditioning for multiple trials (Dyer, 2012a). In such circumstances, animals are expected to assign a higher weight to the role of experience than to innate preferences (if any). The latter would manifest in the very first choices, yet they could be rapidly overshadowed by information acquired through individual experience.
Honeybees show evidence of learning spatial stimuli in a complex and dynamic way depending upon factors including stimulus salience; type of conditioning; and length of training. For example, considering simple stimuli composed of a low number of salient elements, honeybees and bumblebees have been observed to scan each element, which appears to be an innate initial learning behaviour (Lehrer et al., 1985; Dafni et al., 1997; MaBouDi et al., 2020) in a fashion somewhat analogous to how human vision moves eyes to initially scan salient elemental features in a scene when learning a new face, for instance (Henderson et al., 2005). This initial scanning behaviour can permit elemental learning based on spatial frequencies which could be explained by a matched filtering type process and subsequently allow a simple stimulus to be discriminated from alternative dissimilar stimuli (Srinivasan and Lehrer, 1988). One form of such learning can be described as absolute conditioning, where with a low number of learning events, sometimes within less than 10 trials, fast but relatively coarse discrimination is possible (Horridge and Zhang, 1995; Giurfa et al., 1999b). An alternative type of conditioning is termed differential conditioning, where rewarded target stimuli are learnt in the presence of non-rewarded and perceptually similar distractor stimuli. Differential conditioning enables finer levels of discrimination (Giurfa et al., 1999b; Stach et al., 2004). Learning with differential conditioning is dependent on the length of training. For instance, Stach and Giurfa (2005) reported that long training of 42 learning events (trials) led to significantly improved learning outcomes, and a fundamentally different type of visual processing compared to short training of 21 learning events (trials). This difference based upon experience explains why honeybees show evidence of moving from a simple scanning behaviour to a more complex, “cortical-like” way of processing spatial information (Srinivasan et al., 1993). Subsequent work has confirmed the dynamic nature of honeybee vision, and the dependence of training time required to enable different types of visual processing on task complexity (Avarguès-Weber et al., 2020). Interestingly, the human visual system also shows some similar patterns. For instance, in early stages of learning, eye movements are required to initiate learning (Henderson et al., 2005). Yet, later recognition of complex stimuli, such as faces, develops into robust holistic-type processing (Tanaka and Farah, 1993; Maurer et al., 2002) and can occur in the absence of scanning (Thorpe et al., 1996). Thus, visual learning and recognition appear to be enabled by dynamic processes in brains, although our understanding of these processes in insects is still emerging.
Here, we tested the performance of both flower-naïve and experienced bees learning to discriminate between pictures of either normally configured or scrambled flowers. In parallel, we tested the learning abilities of bees with high contrast pictures of normally configured flowers. We decided to employ a differential conditioning procedure with enough stimuli exposure to allow the bees to develop a complete representation of the stimuli.
We aimed to determine if the bee brain is inherently tuned to process flower cues, or if this type of specialisation emerges from foraging experience. To explore this question, we tested both flower-experienced and flower-naïve honeybee foragers with respect to their ability to learn rewarding vs. non-rewarding images depending on how the flower information was presented. We trained bees to discriminate between two flowers when flower spatial information was normally configured, symmetrically scrambled (experienced foragers only), asymmetrically scrambled, or high-contrasted. Experienced foragers were tested on all four stimulus comparisons, while naïve bees were tested on three of the comparisons. Our results suggest that flower shape recognition and memorisation is influenced by both hard-wired neural circuitry and experience-dependent factors.
Materials and Methods
Study Species and Groups
Two groups of bees were trained to differentiate between two flower stimuli. One group consisted of experienced foragers (free-flying honeybee foragers; n = 137), while a second group consisted of flower-naïve foragers (bees living within a greenhouse which had never been exposed to flowers, hereafter referred to as naïve bees/naïve foragers; n = 30). Experienced foragers were allowed to freely forage and visit flowers from hives placed outside at Johannes Gutenberg University of Mainz, which has extensive biological gardens (see below). The experienced bees had previously visited flowers, and were collected while foraging at gravity feeders. These bees were typically 3–4-week-old individuals engaged in intensive foraging activities during the summer when the experiments were performed. Naïve foragers had not previously been exposed to either flowers nor any images of flowers. They were reared inside a greenhouse with only a clear glass von Frisch type gravity feeder to collect sucrose from and access to a water bowl (see below for more information). These bees were typically 3–4-week-old individuals trained and tested after exiting the hive in the greenhouse. While we had no control over the foraging experience of experienced bees prior to our experiments, naïve bees in the greenhouse never experienced appetitive rewards on flower-like visual stimuli. This approach has already been used to study innate colour preferences of bees raising within a greenhouse upon their first foraging flight (Giurfa et al., 1995).
Bees from both groups were divided into several subgroups to test for their ability to discriminate between the two stimuli, which consisted of (a) normal flower images (experienced foragers: n = 36; naïve foragers: n = 10), (b) scrambled flower images (experienced foragers: n = 36), (c) high contrast flower images (experienced foragers: n = 35; naïve foragers: n = 10), and (d) asymmetric scrambled flower images (experienced foragers: n = 30; naïve foragers: n = 10; Figure 1). The difference between the number of groups was due to the challenges and limitations of training and testing honeybees in a greenhouse. Each bee only experienced one set of four images for the training and testing phases. Each set included two identical rewarded images and two identical non-rewarded images, different from the rewarded ones. Bees in all groups then underwent an unconditioned learning test to determine their ability to discriminate between the two training stimuli in the absence of reinforcement. Images of scrambled flowers follows the protocols of Collishaw and Hole (2000), who showed that image scrambling provides experimental access to understand featural or configural mechanisms of visual perception.
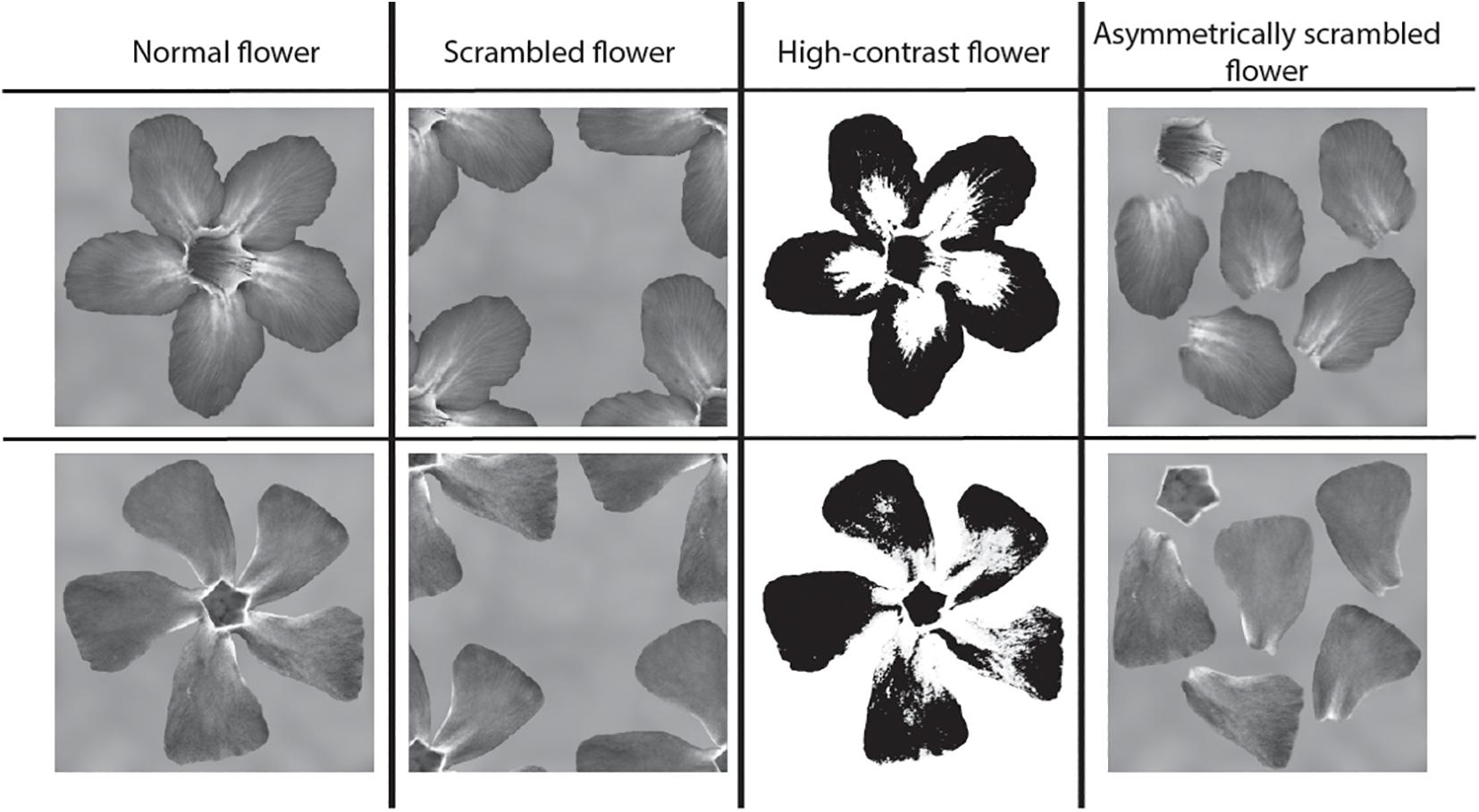
Figure 1. The pairs of stimuli presented to bees learning to differentiate between two normal flower stimuli, two scrambled flower stimuli, two high contrast flower stimuli, and two asymmetrically scrambled flower stimuli. All stimuli contained the same image information compared to the normal flower images but differed in either contrast (black and white images compared to greyscale) or configuration of flower information (e.g., scrambled petals in image).
Group and sample sizes differed between the experienced and naïve groups, as naïve bees living within the greenhouse were harder to maintain and test than the free-flying experienced foragers. It is generally accepted that honeybees are difficult to keep, train, and test when constrained to small spaces, such as greenhouses, resulting in the experiments with naïve bees having lower group sizes than the groups of experienced bees.
All groups were counterbalanced for the image that was rewarding during training with the exception of the group consisting of n = 35 bees.
Experienced Bee Recruitment
Gravity feeders containing approximately 5–10% sucrose solution (by volume) were placed among hives at the Johannes Gutenberg University of Mainz. Honeybees landing on the feeders were recruited to the experiment on a plexiglass spoon containing 25% sucrose. When a bee volitionary returned to the testing site it was marked with a unique identifying colour code and or queen marking number following the standard procedure established by von Frisch (1965). Marked bees were collected onto a spoon containing 25% sucrose solution and taken to the rotating screen apparatus for training. They were placed on the platforms with no stimulus present and allowed to drink until satiated after which they returned to the hive. When the bee next returned to the rotating screen, stimuli were displayed and the experiment commenced.
Naïve Bee Maintenance
To ensure we could use naïve honeybee foragers, which had not experienced flowers throughout their lifespan, a greenhouse was constructed (Giurfa et al., 1995). The greenhouse was made of a transparent plastic sheet for the roof and walls, a grey plastic sheet for the floor, and plastic poles to hold it up. It was approximately 5 m × 2.5 m × 2.5 m (L × W × H; Figure 2). The area under and around the greenhouse was mowed and vegetation was removed to ensure there were no flowers close to the hive. A hive was placed in the wall of the greenhouse, with a mesh divider through the hive to ensure half of the bees could still fly out to collect nectar and pollen. We ensured the queen was in the half of the hive that only had access to the greenhouse. The greenhouse contained a grey gravity feeder, which provided 30% sucrose solution to foraging bees, and access to a water dish. After a few days of acclimatisation of the hive to the new position inside the greenhouse, we began to place colour marks on bees which were newly emerging from cells each morning on the greenhouse side of the hive. This was done for approximately 3 weeks before the marked bees emerged as foragers. Once the marked bees began exiting the hive to forage in the greenhouse, we could test the bees, which were marked and visiting the feeder, thereby ensuring that only naïve honeybee foragers which had lived their whole lives within the greenhouse were tested. Bees exiting the hive were placed on the feeder to allow them to find it within the greenhouse, or they found it themselves. Recruitment of bees to the experiment was performed as above with experienced bees. All marking, training, and testing of naïve bees was conducted within the greenhouse. We trained and tested 30 naïve foragers, with n = 10 per experiment.
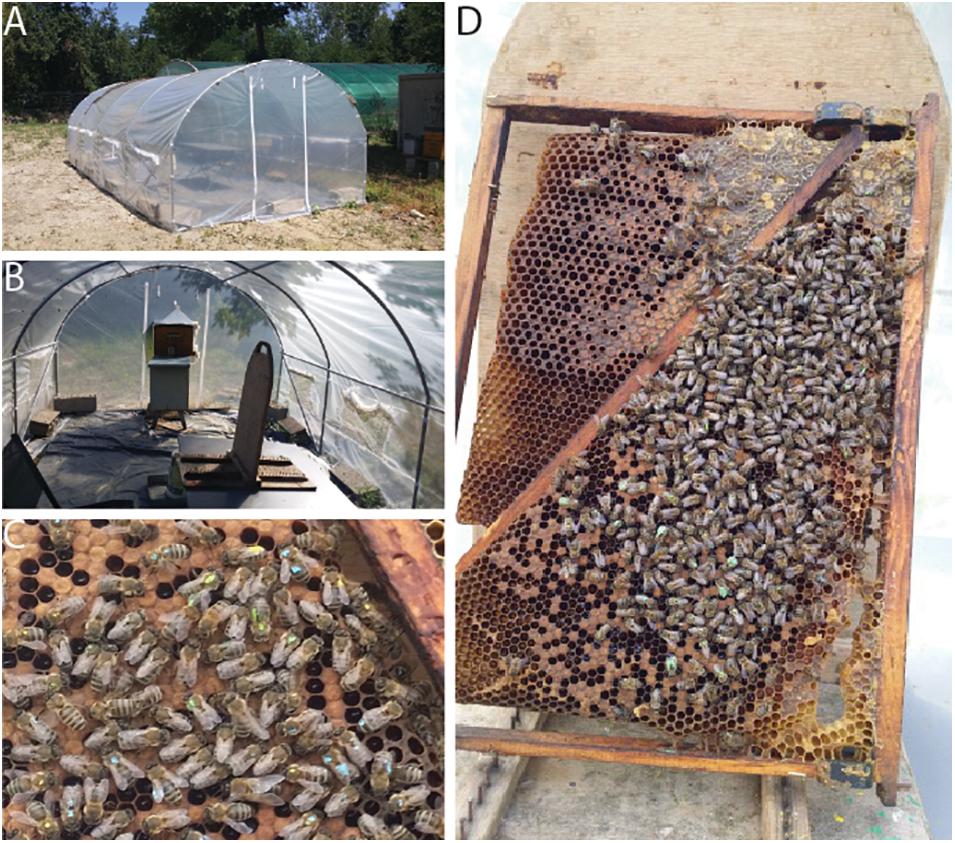
Figure 2. The greenhouse which was constructed to house the naïve honeybees (A,B) and the marked bees inside of the hive on a frame (C,D).
Training
Both groups (naïve and experienced foragers) were presented with four flowers (two identically correct flower images and two identically incorrect flower images, pseudo-randomised per bee; see Figure 3) on a rotating screen for 30 choices. The rotating screen (Figure 3) was 50 cm in diameter and was made of a grey plexiglass material (Dyer et al., 2005; Avarguès-Weber et al., 2010). It contained hangers made of the same material used to present stimuli to bees. The hangers contained a landing platform on which sucrose solution could be placed, and bees could land to drink the solution directly below the stimuli. Bees were trained one at a time. Each time a bee landed on the correct flower type, it received a 10 μL drop of 50% sucrose solution as a reward for choosing the correct flower. If it landed on the incorrect flower option, it received a drop of water, a neutral outcome. Once a bee made a correct decision, it was removed from the apparatus using a transparent plexiglass spoon containing a 10 μL drop of 50% sucrose solution. The bee was placed behind an opaque screen while the apparatus was cleaned with ethanol, dried, stimulus positions were changed, and the screen was rotated to randomise the position of correct and incorrect stimuli. Once the bee finished imbibing the sucrose from the spoon, it could choose to either return to the hive or make another choice. Once a bee completed 30 choices, it was given sucrose on a spoon until it was satiated and returned to the hive. While the bee was in the hive, the apparatus was cleaned and set-up for the non-rewarded test. The training phase lasted approximately 2–3 h.
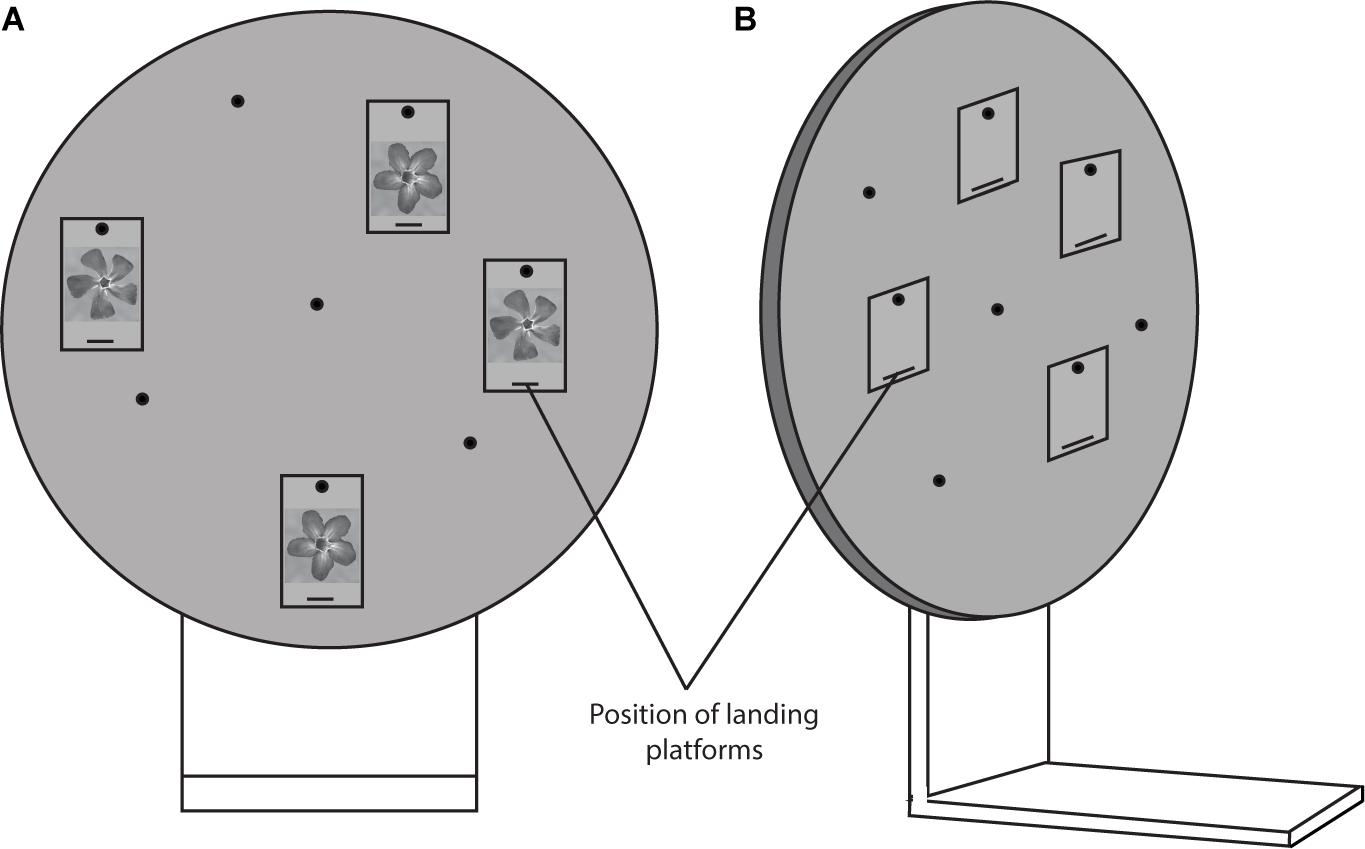
Figure 3. The rotating screen apparatus (50 cm diameter) which was used in all experiments. In this diagram, the apparatus is shown with two identical normal flower stimuli which would be the correct option and two different but identical normal flower stimuli which would be the incorrect option (A). The flower stimulus which was correct was pseudo-randomised between bees. The reward (drop of sucrose solution) was provided on the landing platforms. (B) Shows the apparatus from the side.
Testing
Both groups of bees were given a non-rewarded learning test of 20 choices to determine if they had learnt to choose the correct flower image during training. Each bee was only tested on the stimuli they had been trained on. For the test each bee was presented with the same four flowers from the training phase (two identically correct flower images and two identically incorrect flower images; see Figure 3) on a rotating screen. The number of test choices to be conducted was determined in a pilot study. As tests should occur in the absence of sucrose reward, we replaced it by water which was placed on the platforms to induce landings. Bees were tested using the same stimuli that they had been trained on: (i) normally configured flowers, (ii) scrambled flowers (experienced foragers only), (iii) high contrast flowers, or (iv) asymmetrically scrambled flowers. Prior to the unrewarded test, all stimuli and platforms were cleaned with ethanol and dried to avoid scent marks. Generally, bees made all test choices within 5 min.
Statistical Analysis
Training Phase
Data from naïve and experienced bees were analysed separately as the two groups of bees were not exposed to the same number of flower types nor tested in parallel and originated from different colonies. We tested the null hypothesis that training would have no effect [slope (m) = 0], and that there would be no interaction between flower type and number of trials by fitting an ANCOVA generalised linear mixed model (ANCOVA glmm) to the choice data for each bee group. The linear model included, in addition to the intercept and error term (ε), two predictors representing the number of trial blocks as a continuous variable (five blocks of six choices each) and the flower type group as a categorical predictor with four levels for the experienced bees and three levels for the naïve bees, and an interaction term between the two predictor variables (Equation 1).
We used the proportion of correct choices per block as the response variable [p(C)] and assumed that the observations followed a binomial distribution. A logit function was used to link the proportion of correct choices to the linear predictor function. We included the ID number of each bee as a random term (αi) in the model to account for the repeated measurements collected from each bee participating in the experiment.
Testing Phase
We initially fitted a generalised linear model (GLM) using the proportion of correct choices per bee as the response variable and the flower treatment as a predictor to test for a potential effect of flower treatment on the number of correct choices. We assumed that the proportion of correct choices is described by a binomial distribution and used a logit function to link the response variable with the linear predictor. As each bee contributed a single pair of correct and incorrect responses, we did not include a random term into this model. Flower treatment had the same four levels for the experienced bees or three levels for the naïve bees used during the learning phase. The model is analogous to a one-way ANOVA design described by Equation 2:
In addition to the omnibus test, we tested whether the mean number of correct choices observed for each flower treatment differed significantly from chance. This was done by fitting individual GLM models to response data for each treatment including only the intercept term as a predictor.
All analyses were performed within the R environment for statistical analysis, Version 1.1.456 (R Core Team, 2017).
Results
Training Phase
A graphical representation of the models fitting the choice data for the experienced and naïve bees is given in Figure 4.
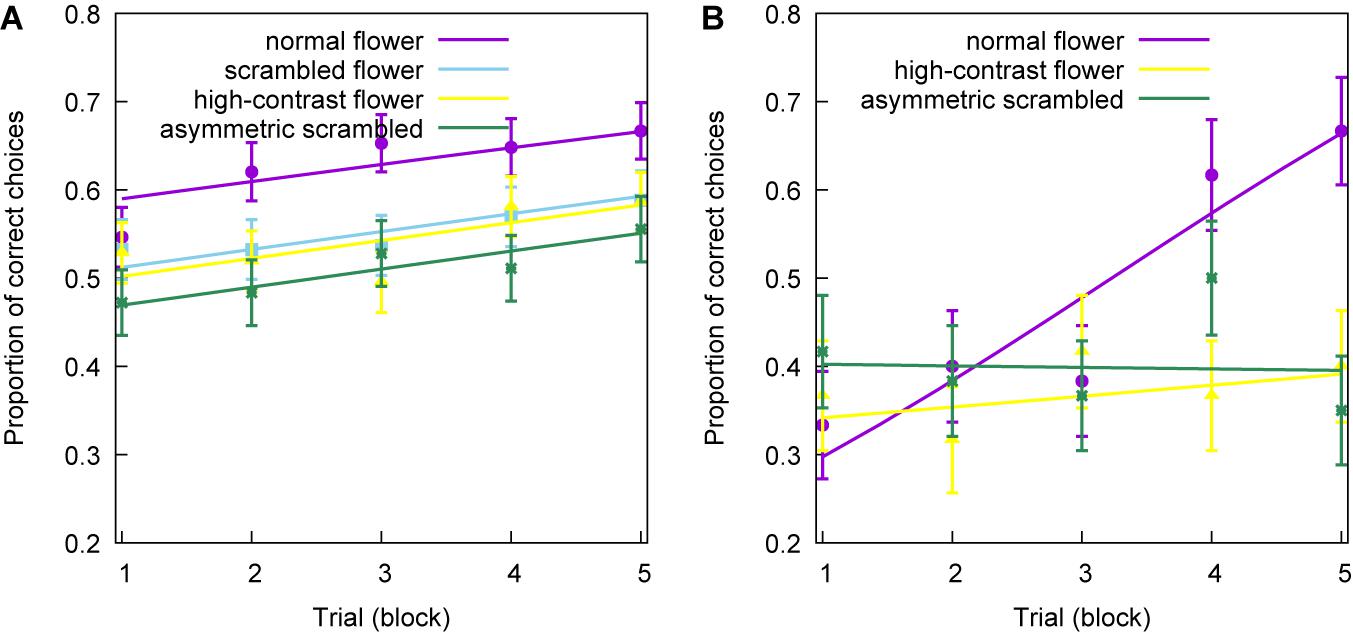
Figure 4. Linear model (solid lines) and mean proportion of correct choices (markers) observed from a group of experienced (A) and naïve bees (B). Different groups of bees were trained with differential conditioning to discriminate between two flower images in different configurations: normal flowers (purple line, circle markers), scrambled flowers (blue line, square markers), high contrast flowers (yellow line, triangle markers), and asymmetric scrambled flowers (green line, asterisk markers). Each training block represents the pooling of six choices following standard methods (Giurfa et al., 2001). Error lines represent the standard error of the mean proportion of correct choices for each trial block. The solid lines represent the fixed effects of the two models.
Experienced Bees
The initial model fitted to the data corresponding to the training phase of the experienced bees showed no interaction between trial number and the type of image [Deviance (G) = 0.883, P = 0.830]. Therefore, we fitted a reduced model to the data excluding the interaction term. The reduced model yielded a significant effect of both trial (G = 13.2, P < 0.001) and image type (G = 21.2, P < 0.001) on the proportion of correct choices performed by the experienced bees.
The reduced model suggests that trial had a significant effect on the performance for all image types, as all four groups of bees improved choice performance at the same rate (Figure 4A). There was a significant effect of image type, indicating that the mean number of correct choices observed for each flower type during training was different. Contrast analyses performed on the image type variable revealed that the mean proportion of correct choices for the scrambled (z = 3.05, P = 0.002), high contrast (z = 3.43, P = 0.001), and asymmetrically scrambled flowers (z = 4.51, P < 0.001) were significantly lower than the mean proportion of choices observed for the normal flowers. This showed that experienced bees learnt the normally configured flowers better than the other images.
Interestingly, when considering the proportion of correct choices for the different image types between the first and second trial blocks, the normal flowers appeared to be learnt faster than the other alternatives (Figure 5) as no bees had learnt the task significantly better than chance level in the first trial block, but bees had learnt the normally configured flower by the end of the second block of trials. Even though the mean proportion of correct choices at the end of the first trial block (Figure 5A) was similar for the normal images and the three flower image variations (zscrambled = −0.290, P = 0.772; zhigh–contrast = −0.367, P = 0.714; zasymmetric = −1.47, P = 0.142), this trend changed by the end of the second block of trials, suggesting that bees learnt the normally configured flower images more quickly in the initial training trials. After 12 trials (Figure 5B), the number of correct choices differed significantly between the normal and high contrast flowers (zhigh–contrast = −2.11, P = 0.035); and between the normal and asymmetric flowers (zasymmetric = −2.73, P = 0.006). No significant difference was observed between the normal and scrambled flowers (zscrambled = −1.85, P = 0.065).
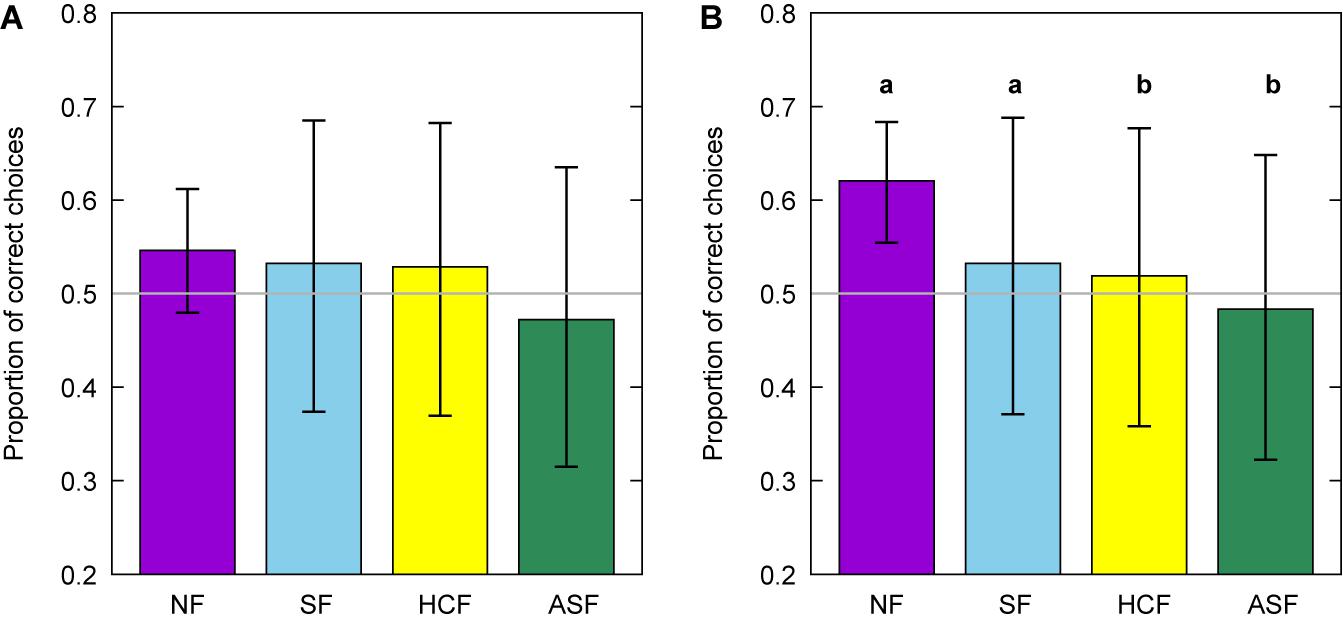
Figure 5. Mean proportion of correct choices by experienced bees for normal (NF), scrambled (SF), high contrast (HCF), and asymmetric scrambled (ASF) flowers for trial blocks 1 (A) and 2 (B). Error bars represent the 95% confidence intervals for the mean number of proportions and letters indicate statistically significant difference between normal flowers (NF) and the three experimental conditions. Refer to Table 2 for the level of significance of each comparison. Grey line indicates chance level performance [p(C) = 50%]. Letters in panel (B) indicate significant differences between the manipulated flower groups and the normal flower group, which was used as a baseline for the analyses.
Pairwise comparisons
The initial omnibus test was followed by pairwise comparisons of the means for the different levels of the fixed treatment factor using estimated marginal means. Results indicate that the mean number of correct choices for the images representing normal flowers was always significantly higher than for each of the manipulated images (Table 1). Interestingly, we could not reject the hypothesis of equality of means when comparing the different pairs representing the three experimental conditions (Table 1). This means that although there is a significant difference between the normal flowers and each of the treatments, there is no difference between the different treatment pairs.
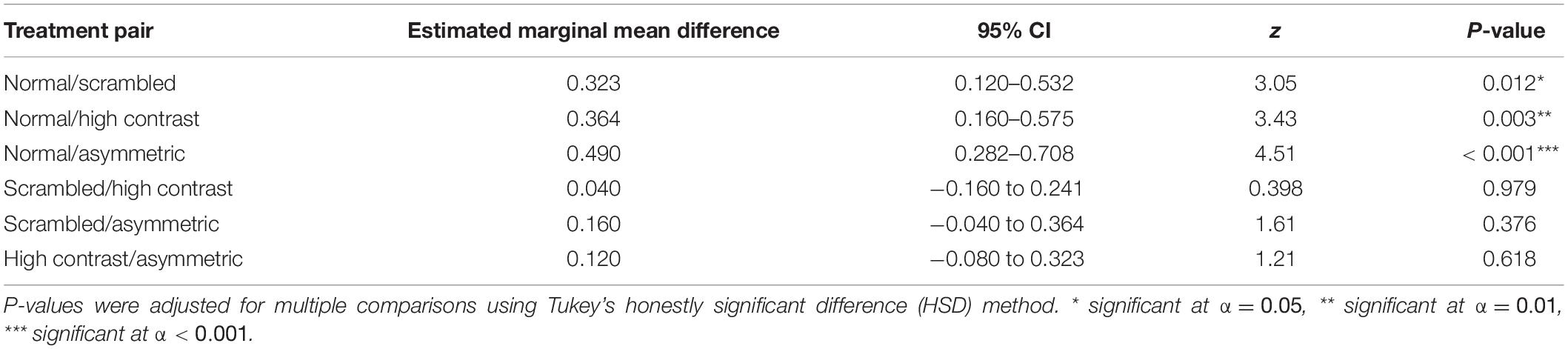
Table 1. Pairwise comparisons of the marginal mean of proportions observed by a group of experienced bees for each treatment level during the training phase.
Naïve Bees
The initial, full model revealed a significant interaction effect (G = 12.0, P = 0.003) between trial and flower type for naïve bees (Figure 4B), indicating a difference in learning rate at least between two of the three flower types considered. A contrast analysis of the interaction term revealed that there were significant differences in the learning rates of naïve bees when learning normal vs. high contrast flowers (z = −2.67, P = 0.008), and when learning normal vs. symmetric, scrambled flowers (z = −3.20, P = 0.001).
Pairwise comparisons
To better understand the nature of the interaction term, we compared the slopes for each pair of levels in the fixed experimental treatment factor. Results indicate that the learning rate of normal flowers by naïve bees is significantly higher than the learning rate for the two different experimental treatments, whilst the different experimental treatments were learnt at the same rate (Table 2). These relationships explain the crossing-over of the learning curves for the different treatments observed for the naïve bees (Figure 1).

Table 2. Pairwise comparisons of the slopes of the learning curves observed by a group of naïve bees for each treatment level during the training phase.
Testing Phase
Experienced Bees
Experienced foragers successfully chose the correct flower option in the unreinforced learning test, when trained and tested with the normally configured flowers (z = 6.85, P < 0.001, n = 36), the scrambled flowers (z = 3.27, P = 0.001, n = 35), and the high contrast flowers (z = 2.57, P = 0.010, n = 36). However, experienced foragers did not choose the correct flower option during the learning test in the asymmetrically scrambled flower condition (z = 0.000, P = 1.000, n = 30) (see Figure 6).
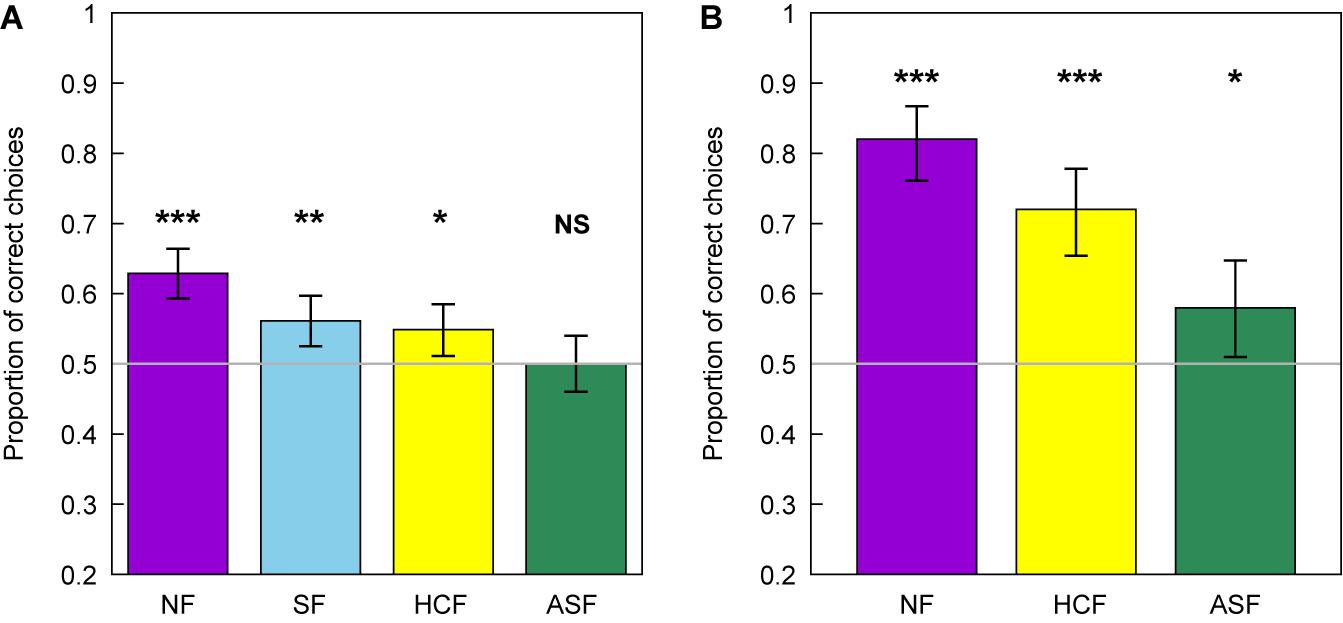
Figure 6. Mean number of correct choices for images representing normal (NF), scrambled (SF), high contrast (HCF), and asymmetric scrambled (ASF) images of flowers by experienced (A) and naïve bees (B) observed during the learning tests. Error bars represent the 95% confidence intervals for the mean in all cases. Asterisks indicate a mean number of correct choices significantly different from chance level expectation of 50% (grey line) at *P < 0.05, **P < 0.001, and ***P < 0.001. NS indicates a mean proportion of choices not significantly different from chance.
Pairwise comparisons
The initial omnibus analysis suggests a significant difference between the mean proportion of correct choices for at least one pair of flower treatments (G = 23.2, P < 0.001). Pairwise comparisons following the initial analysis revealed that the proportion of correct choices observed in bees trained on normal flowers were higher than in the other groups. There was no significant difference in the performance of bees trained and tested with any of the modified flower images (Table 3).
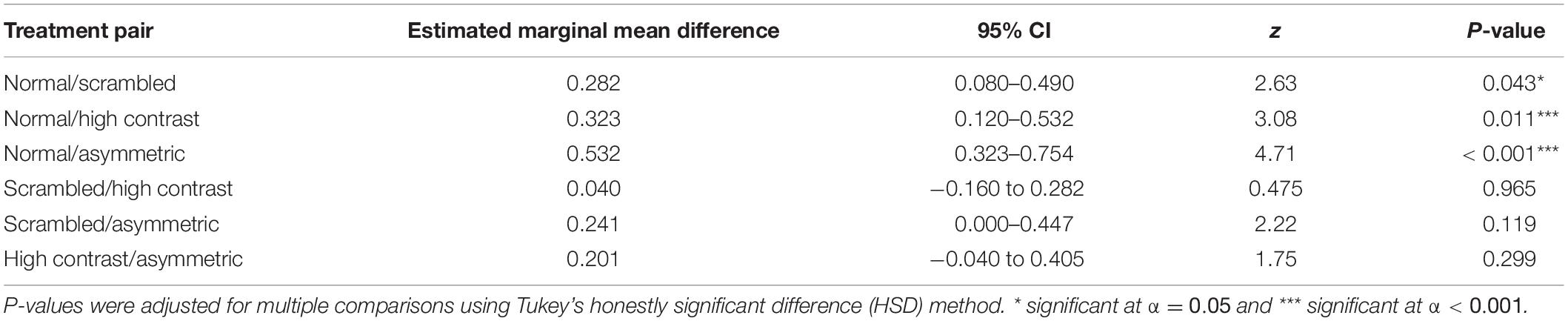
Table 3. Pairwise comparisons of the marginal mean of proportions observed by a group of experienced bees for each treatment level during the learning tests.
Naïve Bees
In contrast to the results of the learning test obtained for the experienced bees, naïve foragers chose all three flower treatments with a frequency significantly higher than chance expectation (normal flower stimuli: z = 8.24, P < 0.001, n = 10, high contrast flower: z = 6.00, P < 0.001, n = 10, and asymmetrically scrambled flower: z = 2.25, P = 0.024, n = 10; Figure 6).
Pairwise comparisons
As for the experienced bees, the initial analysis suggested a significant difference in the mean proportion of correct choices between the different flower treatments (G = 2.83, P < 0.001). Pairwise analyses following the omnibus test indicated a significant difference in the proportion of correct choices between the images representing normal flowers and the two other treatments, and between the high-contrast and asymmetric treatments, with bees showing higher performance for learning normally configured flowers (Table 4).

Table 4. Pairwise comparisons of the marginal mean of proportions observed by a group of naïve bees for each treatment level during the learning tests.
Discussion
Honeybees learnt to discriminate between normally configured pictures of flowers more efficiently than scrambled configurations or high-contrasted versions of the unscrambled pictures, even though these picture manipulations contained a similar level of featural information. Such superior performance for processing normal flower pictures was found both with experienced foragers and flower-naïve bees, providing evidence of an innate bias toward stimuli displaying flower-like configurations. Interestingly, bees which were completely flower naïve showed a higher rate of success for the modified pictures than experienced bees, which we discuss below.
Having an innate template and ability to process flowers would be beneficial to honeybees in a complex natural environment. Such a template would enable naïve foragers to efficiently detect and recognise rewarding flowers whilst avoiding deceptive alternatives like mimics that are known to exist in nature (Dyer and Chittka, 2004; Garcia et al., 2020). Our results (Figures 4–6) suggest that there is a component of innate preference for configured flowers – much like a flower template which is most likely “hard-wired” in the synaptic structure of the brain. Our findings also indicate that with experience, bees may improve their flower recognition and discrimination. When emerging from the hive, naïve honeybees may consequently employ a flower template, as previously shown by Lehrer et al. (1995) for star shaped stimuli, that permits efficient learning of similar star shaped flowers. The neural basis for such a template could be the presence of “matched filters” in the nervous system (Wehner, 1987), that is, visual detectors that specialise in extracting and responding to specific relevant features or configurations in the external world. Whilst initially thought to operate at an early stage of visual processing, such filters show some evidence of operating at the more central levels of sensory processing (Warrant, 2016). The presence of such filters predicts rapid responding to biologically relevant stimuli (Warrant, 2016), although this is not fully consistent with our accumulated evidence that both naïve and experienced foragers take about 30 learning trials with differential conditioning to reliably discriminate between the similar flower images. Detectors in early stages of visual processing could thus only contribute to enhancing discrimination learning abilities by increasing stimuli saliency and by mobilising attentional processes. Indeed, extended learning, which involves some form of top-down use of information, is essential to how bees acquire a capacity to identify target or deceptive flower species. In mammalian systems it is now well appreciated that learning of fine perceptual tasks may involve both higher level neural representations, and also feedback mechanisms that potentially tune responses of feature detectors in the earlier stages of visual processing (Ahissar and Hochstein, 2004; Ahissar et al., 2009). Whilst such phenomenon have proved difficult to access in bees because learning of more complex tasks requires free flying test conditions (Dyer, 2012a; Dyer and Griffiths, 2012), this possibility for information processing remains an interesting area for future work to understand how a miniature brain can enable fine discrimination tasks. Neural elements specialised and tuned via experience in responding to radially organised stimuli could thus promote efficiency to enable avoidance of deceptive stimuli, maximising nutrition collection and promoting colony survival.
There are two potential evolutionary processes by which bees may have acquired specialisation for configured, star-shaped flowers (Howard et al., 2019e, 2021). The first process suggests that the flowers of angiosperms would have evolved to exploit the preferences of insects, such as honeybees, for certain flower morphologies (Lehrer et al., 1995; Gegear et al., 2017). This theory is supported by the knowledge that Hymenoptera, including bees, arose during the Permian time period, millions of years before angiosperms arose during the Late Triassic (van der Kooi and Ollerton, 2020). In the other process, honeybees have evolved a preference for the star-like shapes of flowers pollinated by insects due to the nectar and pollen being generally more accessible in this morphology than in other morphological types (Brown et al., 2011).
The flower naïve test group very efficiently learnt to discriminate between the rewarding and non-rewarding real flower images that we used as stimuli, and this learning was significantly better than for the asymmetrically scrambled flowers that would disrupt an innate flower shape template (Figure 6). Interestingly, whilst not a specifically designed research question of the current study, the naïve bees appeared to learn to discriminate between the holistic flower stimuli to a higher level than the experienced bee group (Figure 6), although both groups demonstrated significant learning after 30 trials. One possible explanation for the potentially better learning in flower naïve honeybees is that the experienced bees had very likely visited a range of different flowers in the university garden prior to participating in the experiment. Previous research on naïve honeybee innate preferences for colour stimuli shows that an initial preference (Menzel, 1967) is overwritten by experience with other rewarding colours (Giurfa et al., 1995). A similar phenomenon has also been observed in free-flying bumblebees (Gumbert, 2000) where bees revert to innate preferences if conditions require. Thus, experience with the shapes of real flowers may lead to overwriting any initial innate preferences to some extent. However, a confound to this conclusion is that the naïve bees also showed the capacity to learn to recognise features in the asymmetric flower stimuli, whilst the experienced bees did not (Figures 5, 6). Therefore, the naïve bees could potentially just have been better learners by chance, and/or the experienced bees had developed a more holistic processing strategy. The latter has been observed to emerge in honeybees through experience (Avarguès-Weber et al., 2020). In humans, holistic processing is known to reduce some featural processing capacity (Maurer et al., 2002). Dissecting how experience may influence innate preferences for flowers in bees appears to be a fruitful avenue for future research.
Our current study complements previous research establishing honeybee’s preference for specific flower-like geometric patterns (star-shaped stimuli and stimuli with elements which radiate outward) (Lehrer et al., 1995; Dafni et al., 1997). The importance of flower configuration for bees may explain their tendency to preferentially approach unfamiliar insect-pollinated flowers, originating from a different continent, since insect-pollinated flowers tend to share star-like shapes and radiating elements by opposition to other flowers. The flower configuration not only attracts bees but also induces improved discrimination and learning performance probably due to a combination of bottom-up (feature detectors) and top-down (attentional bias and refinement of the template with experience) processing.
Brains have evolved to be tuned to important stimuli which are species specific. For example, humans and other primates are tuned to detect, process, recognise, and discriminate between the faces of conspecifics (Kanwisher et al., 1997; Pascalis et al., 2002; Wilmer et al., 2010; Young and Burton, 2018). Non-human primates have a network of cortical face-selective “patches” distributed across the inferior temporal and frontal cortex (Hung et al., 2015; Schaeffer et al., 2020), in which neuronal tuning to faces develops from a scaffolding that is already present at birth (Livingstone et al., 2017). Moreover, human infants prefer human face-like stimuli to other non-face-like stimuli (Goren et al., 1975; Valenza et al., 1996; Mondloch et al., 1999; Pascalis et al., 2002), although experience also plays a decisive role in tuning our capacity to discriminate and memorise faces (Pascalis et al., 2002; Feng et al., 2011). Within insects, paper wasps, capable of individual recognition in a hierarchical context, also possess enhanced capacities to learn conspecific faces by opposition to scrambled faces, prey or high-contrast geometric shapes (Sheehan and Tibbetts, 2011). The paper wasp’s specialisation for processing conspecific faces is composed of an innate and acquired component (Tibbetts et al., 2019a,b).
Previous research shows that paper wasps (Sheehan and Tibbetts, 2011) learn to differentiate natural stimuli more efficiently than simple high contrast patterns. Our observations with naïve and experienced honeybee foragers are consistent with the previous observations for wasps, suggesting insects have visual systems tuned to solve the most biologically relevant tasks in their environment. Such a result might not be expected, as the majority of research on honeybees has used simple high contrast stimuli (e.g., Horridge, 1997), however, measurement of neural responses from feature detectors present in the primary visual centres of honeybees are saturated beyond a contrast of 35–40% (Yang and Maddess, 1997). This result then is not surprising when considering that the brain should likely have evolved to process natural rather than parameterised stimuli (Field, 1987). While high-contrast elemental stimuli may help in certain categorisation tasks, natural stimuli providing more complex information may be favoured to allow fine discrimination between similar stimuli. The current study suggests that this is the case at least for processing flowers, which are ecologically relevant stimuli for bees. Neural recordings in other insects like flies and hoverflies suggest optimal processing may indeed be tuned for more natural type scenes (Maddess and Laughlin, 1985; Straw et al., 2008). Future work should explore whether such a bias in processing natural stimuli presenting realistic contrast levels and coherent spatial arrangement of features stands also for any kind of visual object, thus suggesting a general property of the insect visual system.
Honeybees are a model invertebrate species for studying visual learning and rule acquisition such as size rules (Avarguès-Weber et al., 2014; Howard et al., 2017a,b), oddity rules (Giurfa et al., 2001), above vs. below rules (Avarguès-Weber et al., 2011), numerical rules (Gross et al., 2009; Howard et al., 2018, 2019b,c,d), and maze navigation (Collett et al., 1993; Zhang et al., 1996, 2000), among other tasks. Furthermore, honeybees are also excellent learners of patterns and complex images. In the current study, bees were able to learn the configuration of certain flower types within 30 trials, a timeframe which is longer than might be expected if bees were only using a simple matched filtering type process, but is within the timeframe that bees start to develop more complex configural type representations of spatial stimuli (Stach and Giurfa, 2005). Previous studies have demonstrated similar results, showing that bees can learn to recognise and discriminate complex stimuli which may or may not be biologically relevant. For example, honeybees can learn to recognise images of human faces and discriminate between these faces (Dyer et al., 2005; Avarguès-Weber et al., 2010, 2018; Chittka and Dyer, 2012), learn complex pattern discrimination (Srinivasan et al., 1993; Zhang and Srinivasan, 1994; Giger and Srinivasan, 1996; Horridge, 1997; Giurfa et al., 1999b; Efler and Ronacher, 2000; Deisig et al., 2001; Stach et al., 2004; Dyer and Griffiths, 2012), associate abstract characters or colours with concepts (Howard et al., 2019a,b,d), and categorise complex images (Giurfa et al., 1996; Zhang et al., 2004; Benard et al., 2006; Avarguès-Weber et al., 2010). These studies suggest that bees have adapted to processing patterns and images, like those of flowers, and that plasticity is important to survival for a generalist forager. Our study further demonstrates that bees are efficient visual learners and may be innately primed for specific pattern learning. Performance improvement beyond the initial bias toward preferred flower configurations as a result of experience is a potential mechanism for maximising resource acquisition in the specific environments visited by foraging honeybees.
Data Availability Statement
The datasets supporting this article have been uploaded as part of the Supplementary Material.
Author Contributions
SRH, AGD, MG, DR, MGPR, and AA-W developed the original conceptual framework of the study. SRH and AGD collected the behavioural data. SRH and JEG analysed and modelled the data. SRH, AGD, JEG, and AA-W wrote the initial draft. All authors contributed to reviewing the manuscript and checking domain specific facts.
Conflict of Interest
The authors declare that the research was conducted in the absence of any commercial or financial relationships that could be construed as a potential conflict of interest.
Publisher’s Note
All claims expressed in this article are solely those of the authors and do not necessarily represent those of their affiliated organizations, or those of the publisher, the editors and the reviewers. Any product that may be evaluated in this article, or claim that may be made by its manufacturer, is not guaranteed or endorsed by the publisher.
Acknowledgments
We thank Dr. Patrick Schultheiss for his help constructing the greenhouse and Lucie Hotier for the construction and management of the greenhouse beehive. We also thank Dr. Jürgen Schramme and the students from Johannes Gutenberg University of Mainz for assistance in conducting the experiments with experienced foragers. SRH acknowledges the Alfred Deakin Postdoctoral Research Fellowship, Australian Government Research Training Program (RTP) Scholarship, and the Fyssen Foundation. AGD and MGPR were supported by Australian Research Council DP0878968. AGD acknowledges the Alexander von Humboldt Foundation and the Australian Research Council 160100161. MG thanks the European Research Council (ERC) for funding support (Research Grant “Cognibrains”). AA-W acknowledges the CNRS, Toulouse 3 University and the Fyssen Foundation.
Supplementary Material
The Supplementary Material for this article can be found online at: https://www.frontiersin.org/articles/10.3389/fevo.2021.662336/full#supplementary-material
References
Ahissar, M., and Hochstein, S. (2004). The reverse hierarchy theory of visual perceptual learning. Trends Cogn. Sci. 8, 457–464. doi: 10.1016/j.tics.2004.08.011
Ahissar, M., Nahum, M., Nelken, I., and Hochstein, S. (2009). Reverse hierarchies and sensory learning. Philos. Trans. R. Soc. B Biol. Sci. 364, 285–299.
Avarguès-Weber, A., and Giurfa, M. (2014). Cognitive components of color vision in honey bees: how conditioning variables modulate color learning and discrimination. J. Comp. Physiol. A Neuroethol. Sens. Neural Behav. Physiol. 200, 449–461. doi: 10.1007/s00359-014-0909-z
Avarguès-Weber, A., D’amaro, D., Metzler, M., and Dyer, A. G. (2014). Conceptualization of relative size by honeybees. Front. Behav. Neurosci. 8:80. doi: 10.3389/fnbeh.2014.00080
Avarguès-Weber, A., D’amaro, D., Metzler, M., Finke, V., Baracchi, D., and Dyer, A. G. (2018). Does holistic processing require a large brain? Insights from honeybees and wasps in fine visual recognition tasks. Front. Psychol. 9:1313. doi: 10.3389/fpsyg.2018.01313
Avarguès-Weber, A., Dyer, A. G., and Giurfa, M. (2011). Conceptualization of above and below relationships by an insect. Proc. Biol. Sci. 278, 898–905. doi: 10.1098/rspb.2010.1891
Avarguès-Weber, A., Dyer, A. G., Ferrah, N., and Giurfa, M. (2015). The forest or the trees: preference for global over local image processing is reversed by prior experience in honeybees. Proc. Biol. Sci. 282:20142384.
Avarguès-Weber, A., Finke, V., Nagy, M., Szabó, T., d’Amaro, D., Dyer, A. G., et al. (2020). Different mechanisms underlie implicit visual statistical learning in honey bees and humans. Proc. Natl. Acad. Sci. U.S.A. 117, 25923–25934. doi: 10.1073/pnas.1919387117
Avarguès-Weber, A., Portelli, G., Benard, J., Dyer, A., and Giurfa, M. (2010). Configural processing enables discrimination and categorization of face-like stimuli in honeybees. J. Exp. Biol. 213, 593–601. doi: 10.1242/jeb.039263
Benard, J., Stach, S., and Giurfa, M. (2006). Categorization of visual stimuli in the honeybee Apis mellifera. Anim. Cogn. 9, 257–270. doi: 10.1007/s10071-006-0032-9
Brown, M., Downs, C. T., and Johnson, S. D. (2011). Covariation of flower traits and bird pollinator assemblages among populations of Kniphofia linearifolia (Asphodelaceae). Plant Syst. Evol. 294, 199–206.
Burns, J. G., and Dyer, A. G. (2008). Diversity of speed-accuracy strategies benefits social insects. Curr. Bol. 18, R953–R954. doi: 10.1016/j.cub.2008.08.028
Chittka, L., and Dyer, A. G. (2012). Your face looks familiar. Nature 481, 154–155. doi: 10.1038/481154a
Chittka, L., and Niven, J. (2009). Are bigger brains better? Curr. Biol. 19, R995–R1008. doi: 10.1016/j.cub.2009.08.023
Collett, T., Fry, S., and Wehner, R. (1993). Sequence learning by honeybees. J. Comp. Physiol. A Neuroethol. Sens. Neural Behav. Physiol. 172, 693–706. doi: 10.1007/BF00195395
Collishaw, S. M., and Hole, G. J. (2000). Featural and configural processes in the recognition of faces of different familiarity. Perception 29, 893–909. doi: 10.1068/p2949
Dafni, A., and Kevan, P. G. (1997). Flower size and shape: implications in pollination. Isr. J. Plant Sci. 45, 201–211. doi: 10.1080/07929978.1997.10676684
Dafni, A., Lehrer, M., and Kevan, P. (1997). Spatial flower parameters and insect spatial vision. Biol. Rev. 72, 239–282. doi: 10.1017/S0006323196005002
de Ibarra, N. H., and Giurfa, M. (2003). Discrimination of closed coloured shapes by honeybees requires only contrast to the long wavelength receptor type. Anim. Behav. 66, 903–910. doi: 10.1006/anbe.2003.2269
Deisig, N., Lachnit, H., Giurfa, M., and Hellstern, F. (2001). Configural olfactory learning in honeybees: negative and positive patterning discrimination. Learn. Mem. 8, 70–78. doi: 10.1101/lm.8.2.70
Dyer, A. G. (2012b). “Psychophysics of honey bee color processing in complex environments,” in Honeybee Neurobiology and Behavior, eds C. Galizia, D. Eisenhardt, and M. Giurfa (Berlin: Springer), 303–314. doi: 10.1007/978-94-007-2099-2_23
Dyer, A. G. (2012a). The mysterious cognitive abilities of bees: why models of visual processing need to consider experience and individual differences in animal performance. J. Exp. Biol. 215, 387–395. doi: 10.1242/jeb.038190
Dyer, A. G., and Chittka, L. (2004). Fine colour discrimination requires differential conditioning in bumblebees. Naturwissenschaften 91, 224–227. doi: 10.1007/s00114-004-0508-x
Dyer, A. G., and Garcia, J. E. (2014). Color difference and memory recall in free-flying honeybees: forget the hard problem. Insects 5, 629–638. doi: 10.3390/insects5030629
Dyer, A. G., and Griffiths, D. W. (2012). Seeing near and seeing far; behavioural evidence for dual mechanisms of pattern vision in the honeybee (Apis mellifera). J. Exp. Biol. 215, 397–404. doi: 10.1242/jeb.060954
Dyer, A. G., and Murphy, A. H. (2009). Honeybees choose “incorrect” colors that are similar to target flowers in preference to novel colors. Isr. J. Plant Sci. 57, 203–210. doi: 10.1560/IJPS.57.3.203
Dyer, A. G., and Neumeyer, C. (2005). Simultaneous and successive colour discrimination in the honeybee (Apis mellifera). J. Comp. Physiol. A Neuroethol. Sens. Neural Behav. Physiol. 191, 547–557. doi: 10.1007/s00359-005-0622-z
Dyer, A. G., Neumeyer, C., and Chittka, L. (2005). Honeybee (Apis mellifera) vision can discriminate between and recognise images of human faces. J. Exp. Biol. 208, 4709–4714. doi: 10.1242/jeb.01929
Dyer, A. G., Spaethe, J., and Prack, S. (2008). Comparative psychophysics of bumblebee and honeybee colour discrimination and object detection. J. Comp. Physiol. A Neuroethol. Sens. Neural Behav. Physiol. 194, 617–627. doi: 10.1007/s00359-008-0335-1
Dyer, A., Avargues-Weber, A., Reser, D., Rosa, M., and Giurfa, M. (2013). Hierarchical visual representations enhance complex pattern learning in honeybees (Apis mellifera). Front. Physiol. 4. doi: 10.3389/conf.fphys.2013.25.00042
Efler, D., and Ronacher, B. (2000). Evidence against a retinotopic-template matching in honeybees’ pattern recognition. Vis. Res. 40, 3391–3403. doi: 10.1016/S0042-6989(00)00189-9
Feng, L., Liu, J., Wang, Z., Li, J., Li, L., Ge, L., et al. (2011). The other face of the other-race effect: an fMRI investigation of the other-race face categorization advantage. Neuropsychologia 49, 3739–3749. doi: 10.1016/j.neuropsychologia.2011.09.031
Fenster, C. B., Armbruster, W. S., Wilson, P., Dudash, M. R., and Thomson, J. D. (2004). Pollination syndromes and floral specialization. Annu. Rev. Ecol. Evol. Syst. 35, 375–403. doi: 10.1146/annurev.ecolsys.34.011802.132347
Fenster, C. B., Cheely, G., Dudash, M. R., and Reynolds, R. J. (2006). Nectar reward and advertisement in hummingbird-pollinated Silene virginica (Caryophyllaceae). Am. J. Bot. 93, 1800–1807. doi: 10.3732/ajb.93.12.1800
Field, D. J. (1987). Relations between the statistics of natural images and the response properties of cortical cells. J. Opt. Soc. Am. A 4, 2379–2394. doi: 10.1364/JOSAA.4.002379
Garcia, J. E., Phillips, R. D., Peter, C. I., and Dyer, A. G. (2020). Changing how biologists view flowers—color as a perception not a trait. Front. Plant Sci. 11:601700. doi: 10.3389/fpls.2020.601700
Gegear, R. J., Burns, R., and Swoboda−Bhattarai, K. A. (2017). “Hummingbird” floral traits interact synergistically to discourage visitation by bumble bee foragers. Ecology 98, 489–499. doi: 10.1002/ecy.1661
Giger, A., and Srinivasan, M. (1996). Pattern recognition in honeybees: chromatic properties of orientation analysis. J. Comp. Physiol. A Neuroethol. Sens. Neural Behav. Physiol. 178, 763–769. doi: 10.1007/BF00225824
Giurfa, M. (2004). Conditioning procedure and color discrimination in the honeybee Apis mellifera. Naturwissenschaften 91, 228–231. doi: 10.1007/s00114-004-0530-z
Giurfa, M., and Sandoz, J.-C. (2012). Invertebrate learning and memory: fifty years of olfactory conditioning of the proboscis extension response in honeybees. Learn. Mem. 19, 54–66. doi: 10.1101/lm.024711.111
Giurfa, M., Dafni, A., and Neal, P. R. (1999a). Floral symmetry and its role in plant-pollinator systems. Int. J. Plant Sci. 160, S41–S50. doi: 10.1086/314214
Giurfa, M., Hammer, M., Stach, S., Stollhoff, N., Müller-Deisig, N., and Mizyrycki, C. (1999b). Pattern learning by honeybees: conditioning procedure and recognition strategy. Anim. Behav. 57, 315–324. doi: 10.1006/anbe.1998.0957
Giurfa, M., Eichmann, B., and Menzel, R. (1996). Symmetry perception in an insect. Nature 382, 458–461. doi: 10.1038/382458a0
Giurfa, M., Nunez, J., Chittka, L., and Menzel, R. (1995). Colour preferences of flower-naive honeybees. J. Comp. Physiol. A Neuroethol. Sens. Neural Behav. Physiol. 177, 247–259. doi: 10.1007/BF00192415
Giurfa, M., Zhang, S., Jenett, A., Menzel, R., and Srinivasan, M. V. (2001). The concepts of ‘sameness’ and ‘difference’ in an insect. Nature 410, 930–933. doi: 10.1038/35073582
Gómez, J. M., Torices, R., Lorite, J., Klingenberg, C. P., and Perfectti, F. (2016). The role of pollinators in the evolution of corolla shape variation, disparity and integration in a highly diversified plant family with a conserved floral bauplan. Ann. Bot. 117, 889–904. doi: 10.1093/aob/mcv194
Goren, C. C., Sarty, M., and Wu, P. Y. (1975). Visual following and pattern discrimination of face-like stimuli by newborn infants. Pediatrics 56, 544–549.
Gross, H. J., Pahl, M., Si, A., Zhu, H., Tautz, J., and Zhang, S. (2009). Number-based visual generalisation in the honeybee. PLoS One 4:e4263. doi: 10.1371/journal.pone.0004263
Gumbert, A. (2000). Color choices by bumble bees (Bombus terrestris): innate preferences and generalization after learning. Behav. Ecol. Sociobiol. 48, 36–43. doi: 10.1007/s002650000213
Henderson, J. M., Williams, C. C., and Falk, R. J. (2005). Eye movements are functional during face learning. Mem. Cogn. 33, 98–106. doi: 10.3758/BF03195300
Horridge, G. A. (1997). Pattern discrimination by the honeybee: disruption as a cue. J. Comp. Physiol. A Neuroethol. Sens. Neural Behav. Physiol. 181, 267–277. doi: 10.1007/s003590050113
Horridge, G. A., and Zhang, S. W. (1995). Pattern vision in honeybees (Apis mellifera): flower-like patterns with no predominant orientation. J. Insect Physiol. 41, 681–688. doi: 10.1016/0022-1910(95)00021-L
Howard, S. R., Shrestha, M., Schramme, J., Garcia, J. E., Avarguès-Weber, A., Greentree, A. D., et al. (2019e). Honeybees prefer novel insect-pollinated flower shapes over bird-pollinated flower shapes. Curr. Zool. 65, 457–465.
Howard, S. R., Avarguès-Weber, A., Garcia, J. E., Greentree, A. D., and Dyer, A. G. (2019b). Numerical cognition in honeybees enables addition and subtraction. Sci. Adv. 5:easv0961. doi: 10.1126/sciadv.aav0961
Howard, S. R., Avarguès-Weber, A., Garcia, J. E., Greentree, A. D., and Dyer, A. G. (2019c). Surpassing the subitizing threshold: appetitive–aversive conditioning improves discrimination of numerosities in honeybees. J. Exp. Biol. 222:jeb205658. doi: 10.1242/jeb.205658
Howard, S. R., Avarguès-Weber, A., Garcia, J. E., Greentree, A. D., and Dyer, A. G. (2019d). Symbolic representation of numerosity by honeybees (Apis mellifera): matching characters to small quantities. Proc. R. Soc. B Biol. Sci. 286: 20190238.
Howard, S. R., Avarguès-Weber, A., Garcia, J. E., Greentree, A. D., and Dyer, A. G. (2019a). Achieving arithmetic learning in honeybees and examining how individuals learn. Commun. Integr. Biol. 12, 166–170. doi: 10.1080/19420889.2019.1678452
Howard, S. R., Avarguès-Weber, A., Garcia, J. E., Greentree, A. D., and Dyer, A. G. (2018). Numerical ordering of zero in honey bees. Science 360, 1124–1126. doi: 10.1126/science.aar4975
Howard, S. R., Avarguès-Weber, A., Garcia, J., and Dyer, A. G. (2017a). Free-flying honeybees extrapolate relational size rules to sort successively visited artificial flowers in a realistic foraging situation. Anim. Cogn. 20, 627–638. doi: 10.1007/s10071-017-1086-6
Howard, S. R., Avarguès-Weber, A., Garcia, J. E., Stuart-Fox, D., and Dyer, A. G. (2017b). Perception of contextual size illusions by honeybees in restricted and unrestricted viewing conditions. Proc. Biol. Sci. 284:20172278. doi: 10.1098/rspb.2017.2278
Howard, S. R., Prendergast, K., Symonds, M. R. E., Shrestha, M., and Dyer, A. G. (2021). Spontaneous choices for insect-pollinated flower shapes by wild non-eusocial halictid bees. J. Exp. Biol. 224:jeb242457. doi: 10.1242/jeb.242457
Hung, C.-C., Yen, C. C., Ciuchta, J. L., Papoti, D., Bock, N. A., Leopold, D. A., et al. (2015). Functional mapping of face-selective regions in the extrastriate visual cortex of the marmoset. J. Neurosci. 35, 1160–1172. doi: 10.1523/JNEUROSCI.2659-14.2015
Johnson, S., and Dafni, A. (1998). Response of bee−flies to the shape and pattern of model flowers: implications for floral evolution in a Mediterranean herb. Funct. Ecol. 12, 289–297. doi: 10.1046/j.1365-2435.1998.00175.x
Kanwisher, N., Mcdermott, J., and Chun, M. M. (1997). The fusiform face area: a module in human extrastriate cortex specialized for face perception. J. Neurosci. 17, 4302–4311. doi: 10.1523/JNEUROSCI.17-11-04302.1997
Lázaro, A., and Totland, Ø (2014). The influence of floral symmetry, dependence on pollinators and pollination generalization on flower size variation. Ann. Bot. 114, 157–165. doi: 10.1093/aob/mcu083
Lehrer, M., Horridge, G., Zhang, S., and Gadagkar, R. (1995). Shape vision in bees: innate preference for flower-like patterns. Philos. Trans. R. Soc. London B Biol. Sci. 347, 123–137. doi: 10.1098/rstb.1995.0017
Lehrer, M., Wehner, R., and Srinivasan, M. (1985). Visual scanning behaviour in honeybees. J. Comp. Physiol. A Neuroethol. Sens. Neural Behav. Physiol. 157, 405–415. doi: 10.1007/BF00615140
Livingstone, M. S., Vincent, J. L., Arcaro, M. J., Srihasam, K., Schade, P. F., and Savage, T. (2017). Development of the macaque face-patch system. Nat. Commun. 8:14897. doi: 10.1038/ncomms14897
MaBouDi, H., Dona, H., Gatto, E., Loukola, O. J., Buckley, E., Onoufriou, P. D., et al. (2020). Bumblebees use sequential scanning of countable items in visual patterns to solve numerosity tasks. Integr. Comp. Biol. 60, 929–942. doi: 10.1093/icb/icaa025
Maddess, T., and Laughlin, S. B. (1985). Adaptation of the motion-sensitive neuron H1 is generated locally and governed by contrast frequency. Proc. R. Soc. London B Biol. Sci. 225, 251–275. doi: 10.1098/rspb.1985.0061
Maurer, D., Le Grand, R., and Mondloch, C. J. (2002). The many faces of configural processing. Trends Cogn. Sci. 6, 255–260. doi: 10.1016/S1364-6613(02)01903-4
Menzel, R. (1967). Untersuchungen zum erlernen von spektralfarben durch die honigbiene (Apis mellifica). Z. Vgl. Physiol. 56, 22–62. doi: 10.1007/BF00333562
Mondloch, C. J., Lewis, T. L., Budreau, D. R., Maurer, D., Dannemiller, J. L., Stephens, B. R., et al. (1999). Face perception during early infancy. Psychol. Sci. 10, 419–422. doi: 10.1111/1467-9280.00179
Morawetz, L., Svoboda, A., Spaethe, J., and Dyer, A. G. (2013). Blue colour preference in honeybees distracts visual attention for learning closed shapes. J. Comp. Physiol. A Neuroethol. Sens. Neural Behav. Physiol. 199, 817–827. doi: 10.1007/s00359-013-0843-5
Pascalis, O., De Haan, M., and Nelson, C. A. (2002). Is face processing species-specific during the first year of life? Science 296, 1321–1323. doi: 10.1126/science.1070223
R Core Team (2017). R: A Language and Environment for Statistical Computing (Version 3.4. 2) [Computer software]. Vienna: R Foundation for Statistical Computing.
Reser, D. H., Wijesekara Witharanage, R., Rosa, M. G., and Dyer, A. G. (2012). Honeybees (Apis mellifera) learn color discriminations via differential conditioning independent of long wavelength (green) photoreceptor modulation. PLoS One 7:e48577. doi: 10.1371/journal.pone.0048577
Sarter, M., Givens, B., and Bruno, J. P. (2001). The cognitive neuroscience of sustained attention: where top-down meets bottom-up. Brain Res. Rev. 35, 146–160. doi: 10.1016/S0165-0173(01)00044-3
Schaeffer, D. J., Selvanayagam, J., Johnston, K. D., Menon, R. S., Freiwald, W. A., and Everling, S. (2020). Face selective patches in marmoset frontal cortex. Nat. Commun. 11:4856. doi: 10.1038/s41467-020-18692-2
Sheehan, M. J., and Tibbetts, E. A. (2011). Specialized face learning is associated with individual recognition in paper wasps. Science 334, 1272–1275. doi: 10.1126/science.1211334
Sommerlandt, F. M., Spaethe, J., Rössler, W., and Dyer, A. G. (2016). Does fine color discrimination learning in free-flying honeybees change mushroom-body calyx neuroarchitecture? PLoS One 11:e0164386. doi: 10.1371/journal.pone.0164386
Srinivasan, M. V., and Lehrer, M. (1988). Spatial acuity of honeybee vision and its spectral properties. J. Comp. Physiol. A Neuroethol. Sens. Neural Behav. Physiol. 162, 159–172. doi: 10.1007/BF00606081
Srinivasan, M. V., Zhang, S., and Rolfe, B. (1993). Is pattern vision in insects mediated by ‘cortical’ processing? Nature 362, 539–540. doi: 10.1038/362539a0
Stach, S., and Giurfa, M. (2005). The influence of training length on generalization of visual feature assemblies in honeybees. Behav. Brain Res. 161, 8–17. doi: 10.1016/j.bbr.2005.02.008
Stach, S., Benard, J., and Giurfa, M. (2004). Local-feature assembling in visual pattern recognition and generalization in honeybees. Nature 429, 758–761. doi: 10.1038/nature02594
Straw, A. D., Rainsford, T., and O’carroll, D. C. (2008). Contrast sensitivity of insect motion detectors to natural images. J. Vis. 8, 32–32. doi: 10.1167/8.3.32
Tanaka, J. W., and Farah, M. J. (1993). Parts and wholes in face recognition. Q. J. Exp. Psychol. 46, 225–245. doi: 10.1080/14640749308401045
Thorpe, S., Fize, D., and Marlot, C. (1996). Speed of processing in the human visual system. Nature 381, 520–522. doi: 10.1038/381520a0
Tibbetts, E. A., Den Uyl, J., Dwortz, M., and Mclean, C. (2019a). The development and evolution of specialized face learning in paper wasps. Anim. Behav. 147, 1–7. doi: 10.1016/j.anbehav.2018.10.016
Tibbetts, E. A., Desjardins, E., Kou, N., and Wellman, L. (2019b). Social isolation prevents the development of individual face recognition in paper wasps. Anim. Behav. 152, 71–77. doi: 10.1016/j.anbehav.2019.04.009
Valenza, E., Simion, F., Cassia, V. M., and Umiltà, C. (1996). Face preference at birth. J. Exp. Psychol. 22:892. doi: 10.1037/0096-1523.22.4.892
van der Kooi, C. J., and Ollerton, J. (2020). The origins of flowering plants and pollinators. Science 368, 1306–1308. doi: 10.1126/science.aay3662
Vergoz, V., Roussel, E., Sandoz, J.-C., and Giurfa, M. (2007). Aversive learning in honeybees revealed by the olfactory conditioning of the sting extension reflex. PloS One 2:e288. doi: 10.1371/journal.pone.0000288
von Frisch, K. (1965). “Die tänze der bienen,” in Tanzsprache und Orientierung der Bienen, (Berlin: Springer), 3–330. doi: 10.1007/978-3-642-94916-6_2
Warrant, E. J. (2016). Sensory matched filters. Curr. Biol. 26, R976–R980. doi: 10.1016/j.cub.2016.05.042
Wehner, R. (1987). ‘Matched filters’—neural models of the external world. J. Comp. Physiol. A Neuroethol. Sens. Neural Behav. Physiol. 161, 511–531. doi: 10.1007/BF00603659
Wilmer, J. B., Germine, L., Chabris, C. F., Chatterjee, G., Williams, M., Loken, E., et al. (2010). Human face recognition ability is specific and highly heritable. Proc. Natl. Acad. Sci. U.S.A. 107, 5238–5241. doi: 10.1073/pnas.0913053107
Yang, E.-C., and Maddess, T. (1997). Orientation-sensitive neurons in the brain of the honey bee (Apis mellifera). J. Insect Physiol. 43, 329–336. doi: 10.1016/S0022-1910(96)00111-4
Young, A. W., and Burton, A. M. (2018). Are we face experts? Trends Cogn. Sci. 22, 100–110. doi: 10.1016/j.tics.2017.11.007
Zhang, S., and Srinivasan, M. (1994). Prior experience enhances pattern discrimination in insect vision. Nature 368, 330–332. doi: 10.1038/368330a0
Zhang, S., Bartsch, K., and Srinivasan, M. (1996). Maze learning by honeybees. Neurobiol. Learn. Mem. 66, 267–282. doi: 10.1006/nlme.1996.0069
Zhang, S., Mizutani, A., and Srinivasan, M. V. (2000). Maze navigation by honeybees: learning path regularity. Learn. Mem. 7, 363–374. doi: 10.1101/lm.32900
Keywords: bottom-up processing, configural processing, pollinator, spatial configuration, top-down processing, visual learning
Citation: Howard SR, Dyer AG, Garcia JE, Giurfa M, Reser DH, Rosa MGP and Avarguès-Weber A (2021) Naïve and Experienced Honeybee Foragers Learn Normally Configured Flowers More Easily Than Non-configured or Highly Contrasted Flowers. Front. Ecol. Evol. 9:662336. doi: 10.3389/fevo.2021.662336
Received: 01 February 2021; Accepted: 04 October 2021;
Published: 11 November 2021.
Edited by:
Christopher Irwin Smith, Willamette University, United StatesReviewed by:
Kathryn Theiss, California State University, Dominguez Hills, United StatesDarren Incorvaia, McCann Health, United States
Daniel Papaj, University of Arizona, United States
Copyright © 2021 Howard, Dyer, Garcia, Giurfa, Reser, Rosa and Avarguès-Weber. This is an open-access article distributed under the terms of the Creative Commons Attribution License (CC BY). The use, distribution or reproduction in other forums is permitted, provided the original author(s) and the copyright owner(s) are credited and that the original publication in this journal is cited, in accordance with accepted academic practice. No use, distribution or reproduction is permitted which does not comply with these terms.
*Correspondence: Scarlett R. Howard, s.howard@deakin.edu.au; Adrian G. Dyer, adrian.dyer@rmit.edu.au