- 1Department for Soil Health and Plant Nutrition, Austrian Agency for Health and Food Safety (AGES), Vienna, Austria
- 2Department for Seed Testing, Austrian Agency for Health and Food Safety (AGES), Vienna, Austria
- 3InfoXgen Inputs Evaluation, EASY-CERT Services GmbH, Enzersfeld, Austria
- 4Open Science–Life Sciences in Dialogue, Vienna BioCenter, Vienna, Austria
- 5Department of Knowledge Technologies, Jozef Stefan Institute, Ljubljana, Slovenia
- 6Jozef Stefan International Postgraduate School, Ljubljana, Slovenia
Litter decomposition plays a pivotal role in the global carbon cycle, but is difficult to measure on a global scale, especially by citizen scientists. Here, citizen scientists, i.e., school students with their teachers, used the globally applied and standardized Tea Bag Index (TBI) method to collect data on litter decomposition in urban areas in Austria. They also sampled soils to investigate the linkages between litter decomposition and soil attributes. For this study, 54 sites were selected from the school experiments and assembled into a TBI dataset comprising litter decomposition rates (k), stabilization factors (S), as well as soil and environmental attributes. An extensive pre-processing procedure was applied to the dataset, including attribute selection and discretization of the decomposition rates and stabilization factors into three categories each. Data mining analyses of the TBI data helped reveal trends in litter decomposition. We generated predictive models (classification trees) that identified the soil attributes governing litter decomposition. Classification trees were developed for both of the litter decomposition parameters: decomposition rate (k) and stabilization factor (S). The main governing factor for both decomposition rate (k) and stabilization factor (S) was the sand content of the soils. The data mining models achieved an accuracy of 54.0 and 66.7% for decomposition rates and stabilization factors, respectively. The data mining results enhance our knowledge about the driving forces of litter decomposition in urban soils, which are underrepresented in soil monitoring schemes. The models are very informative for understanding and describing litter decomposition in urban settings in general. This approach may also further encourage participatory researcher-teacher-student interactions and thus help create an enabling environment for cooperation for further citizen science research in urban school settings.
Introduction
More than 50% of the world’s citizens are city dwellers inhabiting urban environments (Seto et al., 2014). These environments may triple in size between 2000 and 2030 if current predictions of urbanization continue (Seto et al., 2012). Accordingly, the closest connection to soils of billions of city dwellers is urban soils, which also represent one of the potential key factors for their wellbeing. Urban soils undergo complex interactions between human populations and the environment while delivering essential functions for society, including climate regulation and habitat for biodiversity (Schulte et al., 2014). At the same time, urban soils face several major challenges due to human influences, i.e., anthropization, including soil sealing, compaction, pollution, heat island effects and loss of biodiversity (Lorenz and Lal, 2009; Guilland et al., 2018). The cycling of carbon in the soil ecosystem, e.g., via decomposition, can be altered when humans alter the vegetation structure and composition, leading to changes in above-and below-ground plant litter dynamics (Kaye et al., 2006; Byrne, 2007). Earthworm abundance and biomass also play a role in the decomposer food web (McDonnell et al., 1997). Despite these challenges and the importance of soils for society, for example in terms of carbon sequestration and storage (Edmondson et al., 2012; Churkina, 2016), urban soils have not been extensively studied. A review by Guilland et al. (2018) showed that only 1% of articles on soils between 1960 and 2016 investigated such soils. The most common urban settings for soils are grasslands, street trees and urban forests, urban wastelands, sports grounds, urban gardens of residents or communities and green roofs (Guilland et al., 2018). Human activities on urban soils such as replacement, disturbance, construction of artificial soils, fertilization and pest management make it difficult to monitor as well as assess how they respond to management and land use changes (Lorenz and Lal, 2009, 2017). The urban landscape has been described as a whole new geoscientific sphere, the astysphere (Norra, 2009). Urban soils are often classified as Technosols (IUSS Working Group WRB, 2014), which are characterized by significant amounts of artifacts, i.e., human-made material such as concrete, bricks, sewage sludge and ash. Technosols are often constructed by humans with sometimes careless decisions about what kind of material to put where in the landscape. The global trend of urbanization and the steep increase in city dwellers calls for more research on urban soils to underline their ecological and societal importance in delivering soil functions.
Urban soils and landscapes are ideal for school citizen science, i.e., the participatory generation of new knowledge with school teachers and students (Ryan et al., 2018), based on their close proximity to a large number of schools and teachers. Focusing on the urban environment enables the teachers to take the classes outdoors nearby and to highlight the important functions urban soils deliver to society, including primary productivity. Agriculture and soil science are rarely topics for citizen science projects (Ryan et al., 2018) even though several historical monitoring examples exist (Wildschut, 2017). The importance of agricultural citizen science is increasing in urban settings, where the connections between food systems and consumption are more fragmented than in the countryside. Citizen science fosters bidirectional exchange of information between schools, farmers and researchers, gets students engaged in science through hands-on activities, and enables the students to become active in the democratic processes in their local communities (Ryan et al., 2018). Research partnerships can also have positive impacts on students’ science and social skills (Harnik and Ross, 2003), including problem solving, setting working standards and being creative. Using citizen science mobile applications embraces the possibilities of online community building around the respective research theme. This helps foster future collaborations and social networking between citizen scientists themselves or between citizen scientists and researchers in a form that would otherwise not take place (Wildschut, 2017). However, attractive the benefits may sound, teachers still face challenges with outdoor teaching. These include the fact that outdoor teaching has no formal status in the curriculum, the difficulty of getting started and gaining confidence to teach new topics, as well as physical constraints (van Dijk-Wesselius et al., 2020). These challenges can be overcome with the appropriate mindset, determination and curiosity (van Dijk-Wesselius et al., 2020), which should be backed up by superiors. Measuring litter decomposition with the Tea Bag Index (Keuskamp et al., 2013) as part of the teaching curriculum has successfully been used in school settings in Sweden and Austria, with the involvement of motivated teachers (Sandén et al., 2020). Showing teachers that their intelligence, knowledge and creativity are appreciated by and included in the project promotes beneficial participatory cooperation that can be sustained long-term (Wildschut, 2017).
Data mining has been used in all scientific fields to discover new patterns and knowledge from large amounts of data (Trajanov et al., 2018). Its use in citizen science is not new: enormous amounts of data that have been generated through citizen scientist experiments remain to be analyzed and understood (Ceccaroni et al., 2019). Data mining can be used in citizen science in two stages, namely data collection or in analyzing the collected data. When applied during data collection it offers guidance for the planned analyses (e.g., classification tasks), minimizing errors and maximizing data quality (Lukyanenko et al., 2020). When applied to citizen scientist data, it can be used to find causal relationships or patterns in the observations, or to detect biases in the data (Chen and Gomes, 2019). The numerous examples of using data mining in citizen science projects include but are not limited to astronomy, life sciences, environmental sciences and oceanography (Franzen et al., 2021). While citizen science offers enormous opportunities, for example in training classification algorithms, there is also a need for rigorous procedures to ensure data quality (Balázs et al., 2021), as in any scientific research.
This study was designed to predict litter decomposition, i.e., stabilization factors and decomposition rates, and to identify the soil attributes that govern litter decomposition by means of data mining. To this end, we addressed the following questions within the framework of the urban sites investigated by the citizen scientists:
(i) Do the selected soil attributes influence litter decomposition?
(ii) What are the driving factors of litter decomposition in the urban sites investigated by citizen scientists?
(iii) Can data mining help develop reliable predictive models of litter decomposition based on citizen scientists’ data?
We hypothesized that soil attributes have a significant influence on litter decomposition and that e.g., soil texture and soil organic carbon are driving the decomposition process. The data mining was expected to give robust predictive models that can be further utilized when studying urban soils, with and without public participation.
Materials and Methods
TeaTime4Schools—Citizen Scientist Experiments
An initial call was disseminated in August 2016 to scout interested teachers, followed by a second call in September 2017 to encourage Austrian schools to participate in the TeaTime4Schools1 activities, i.e., to measure litter decomposition in soils with the help of tea bags. In total, 150 school classes signed up. In April 2018, an interactive workshop was organized for the participating teachers, in which the project tasks including the Tea Bag Index methodology (Keuskamp et al., 2013) were explained and additional information and ideas provided on how to include soils in their curriculum. In May 2018, the teachers received the required materials (a total of 450 pairs of tea bags) by post, along with links to additional video guidance2 on how to study litter decomposition over a 3-month period (June-September 2018) with their students. The school classes weighed and buried commercially available tea bags (green tea (EAN 87 22700 05552 5) and rooibos (EAN 87 22700 18843 8) produced by Lipton (Unilever) in non-woven polypropylene mesh bags) as miniature litter bags. They were buried pairwise at a depth of 8 cm in the school surroundings, following the standardized Tea Bag Index methodology (Keuskamp et al., 2013). After the tea bags were retrieved in September 2018, they were dried for at least 3 days at a warm, dry location, cleaned of adhering soil particles and re-weighed. Thereafter, the schools classes reported their data directly to the global Tea Bag Index database3. Using the mass losses of green tea and rooibos, the TeaTime4Schools researcher team calculated the decomposition rates (k) and the litter stabilization factors (S) according to Keuskamp et al. (2013). Each school class also took one composite soil sample of 10–12 individual soil samples with a spade from 0–10 cm depth at the experimental site in September 2018 and sent it to the researchers for soil analyses (see section “Soil and Environmental Characteristics”). The preliminary results were reported back to the school classes in a final workshop (in presence and online) in March 2019.
Soil and Environmental Characteristics
After arriving in the laboratory, the soil samples were sieved through a 2 mm stainless sieve and air-dried prior to further analyses. Soil pH was measured electrochemically (pH/mV Pocket Meter pH 340i, WTW, Weilheim, Germany) in 0.01 M CaCl2 at a soil-to-solution ratio of 1:5 (ÖNORM L1083). Plant available phosphorous (P) and potassium (K) were determined by calcium-acetate-lactate (CAL) extraction (ÖNORM L1087). Total soil organic C (TOC) concentrations were analyzed by dry combustion in a LECO RC-612 TruMac CN (LECO Corp., St. Joseph, MI, United States) at 650°C (ÖNORM L1080). Total N (Ntot) was determined according to ÖNORM L1095 with elemental analysis using a CNS (carbon, nitrogen, sulfur) 2000 SGA-410–06 at 1250°C. KMnO4 determination of labile carbon was analyzed according to Tatzber et al. (2015). Potential nitrogen mineralization was measured by the anaerobic incubation method (Keeney, 1982), as modified according to Kandeler (1993). Texture was determined according to ÖNORM L1061-1 and L1062-2. The environmental characteristics—annual mean air temperature and sum of precipitation for 2018—were taken from the Central Institution for Meteorology and Geodynamics (ZAMG) webpage4, specifically from their weather stations that were closest to the sampling sites.
Statistical Analyses and Data Mining Methods
As a first step, the statistical analyses of k, S, soil and environmental characteristics were performed using the IBM SPSS Statistics 26 software package. The normality of data was checked with Shapiro-Wilk’s test and their descriptive statistics were calculated. Correlations between variables were presented as Pearson correlation coefficients.
As a second step, we used data mining algorithms to model the decomposition rate and stabilization factor from the data obtained from the citizen scientist experiments. In particular, we used algorithms for decision tree induction (Breiman et al., 1984; Witten et al., 2011). Decision trees are predictive models that predict the value of a dependent variable [also called target attribute, in our case decomposition rate (k) and stabilization factor (S)] from a set of independent (descriptive) attributes. They represent a hierarchical structure with a root node, branches and leaf (terminal) nodes. Each internal node contains a test on an attribute in which the value of that attribute is compared to a constant value. The branches coming out of the node represent the outputs of the test. The leaf (terminal) nodes contain the predictions of the target attribute that apply to all samples that fall into that leaf. To predict the value of the target attribute of a new sample, it is routed down the tree according to the values of the outcomes of the tests in each internal node. When the sample reaches a leaf, it is given the prediction assigned to that leaf. Decision trees are generated automatically from data, and the modeler’s influence on the structure of the generated model is solely through the settings of the algorithm parameters and is therefore minimal. The attributes that appear in the tree are the ones that carry the most information for predicting the target attribute.
When the values of the target attribute are numeric, the leaves of the decision tree can either contain piece-wise linear regression equations, or constant values that represent the average value of the target attribute of the samples that reach that leaf. In the first case, the decision trees are termed model trees, in the latter case, regression trees. When the values of the target attribute are categorical, the decision trees are termed classification trees.
In this study, the decision trees were obtained using the data mining package WEKA (Witten et al., 2011), which implements a large number of data mining algorithms for different data mining tasks. In particular, we used J48 for induction of classification trees.
Data
The data from the citizen scientist experiments were preprocessed prior to statistical analyses and data mining. Out of the 150 school classes (450 pairs of tea bags) that signed up, 130 (390 pairs of tea bags) partly or fully carried out the project tasks. Out of those, 83 school classes sent soil samples to the TeaTime4School researcher team, and 69 school classes successfully sent soil samples and submitted their results into the global Tea Bag Index database5. Here, we focus on data that was sent from urban areas, i.e., artificial surfaces, across Austria (Figure 1), altogether from 54 sites (15 sites were filtered out). From each site, data from 1–3 pairs of tea bags (1 green tea, 1 rooibos) were calculated into an average to be used in the data analyses. The schools described their sites as cropland, grassland or forest, but for simplicity, we used the CORINE Land Cover artificial surfaces to extract these sites from our data set. From some of the sites, only data on litter decomposition and environmental characteristics were available due to insufficient amounts of soil sent for analyses. The dataset comprised information about the soil and environmental characteristics described in Section “Soil and Environmental Characteristics” and “Tea Bag Index, Soil and Environmental Characteristics”. The decomposition rate (k) and stabilization factor (S) were our dependent variables for which predictive models (model, regression and classification trees) were developed.
In order to strengthen the explanatory power of the data mining results, the values of k and S were discretized into three categories (1, 2 and 3) for each attribute. The discretization was carried out automatically using the discretization filtering method Discretize in the WEKA data mining package, which discretizes a selected attribute into a predefined number of classes. The decomposition rate was discretized into three classes according to the following thresholds: low (≤0.0127 g d–1), medium (0.0127–0.0210 g d–1), high (>0.0210 g d–1). The stabilization factor was discretized into three classes according to the following thresholds: low (≤0.15, medium (0.15–0.29), high (>0.29). The discretization thresholds for both attributes are in accordance with Keuskamp et al. (2013).
In the subsequent step, we recognized annual precipitation and annual temperature and land use that the citizen scientists defined as not soil-related attributes and excluded them from the analyses. In addition, we created two scenarios for the data mining analyses in which we used different combinations of attributes. In one scenario, we used detailed information about the soil texture (coarse sand, medium sand, fine sand, coarse silt, medium silt, fine silt), whereas in the second scenario we used aggregated information about the soil texture (sand and silt).
Results
TeaTime4Schools Participation
In total, 130 school classes and at least 2,376 students participated, of which at least 868 were female and 764 male: some school classes did not report the number of students or the number of female and male students. Typical challenges for school classes that failed to complete their tasks were that: (i) the tea bags had disappeared over the course of 3 months, (ii) the tea bags had holes or were otherwise damaged during the experiments, (iii) weighing of the tea bags was unsuccessful due to problems with using the pocket scales (e.g., incorrect unit was used or the final weight exceeded the starting weight), or (iv) the teacher originally involved in the project had changed school class or job and the new teacher could not include the planned activities in her/his curriculum.
Tea Bag Index, Soil and Environmental Characteristics
The decomposition rate (k) ranged between 0.004 and 0.029 g d–1, and the stabilization (S) of the labile fraction of green tea ranged between 0.01 and 0.43 (Table 1). The decomposition rate was positively correlated with total annual precipitation (r2 = 0.283, p < 0.05) and negatively correlated with mean annual temperature (r2 = −0.322, p < 0.05). In addition, positive correlations were found with coarse and middle sand fractions (r2 = 0.494, p < 0.01; r2 = 0.325, p < 0.05) and negative correlation with the latitude (r2 = 0.279, p < 0.05). For stabilization factor, no correlations were found with either decomposition rates, soil attributes or environmental characteristics. Soil pH ranged from acidic 5.16 to alkaline 7.61. A wide range of plant available phosphorous (P) and potassium (K) were observed across the investigated sites, 5.00–763 and 61.0–778 mg kg–1, respectively. TOC ranged between 1.22 and 12.5%, whereas Ntot ranged between 0.13 and 1.11%. Moreover, labile carbon and potentially mineralisable N showed large variation between the sites, as did texture (Table 1). Mean annual temperature ranged between 9.1 and 13.5°C and total annual precipitation between 552 and 1,345 mm across the investigated sites (Table 1).
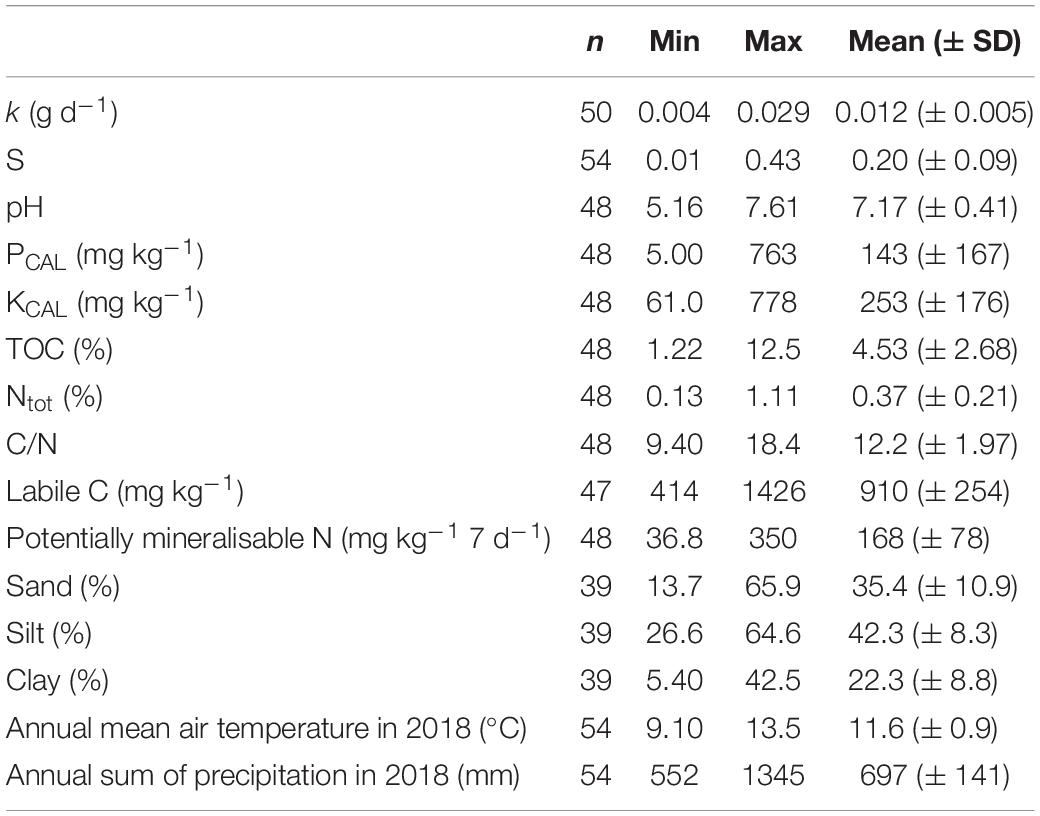
Table 1. Summary of results for the Tea Bag Index, i.e., decomposition rate (k) and stabilization factor (S), soil and environmental characteristics for the sites investigated in TeaTime4Schools, presented as minimum, maximum and mean (±standard deviation).
Data Mining Models for Litter Decomposition
To model the decomposition rate and stabilization factor, we generated four classification trees: one for each dependent variable, k and S, and for each scenario (detailed vs. aggregated soil texture). The accuracy and the root mean squared error of each tree are given in Table 2 and show a moderate predictive performance. The best classification trees for predicting decomposition rate (k) and stabilization factor (S) were obtained using the aggregated soil texture attributes, which resulted in 54.0 and 66.7% accuracy, respectively. Figure 2 presents the classification tree for decomposition rate (k) using aggregated soil texture attributes. It indicates the pivotal role of sand content and total nitrogen for decomposition rates. The classification tree for predicting the stabilization factor using aggregated soil texture attributes (Figure 3) shows that sand contents as well as plant available potassium and phosphorous played a significant role in stabilizing the labile material.
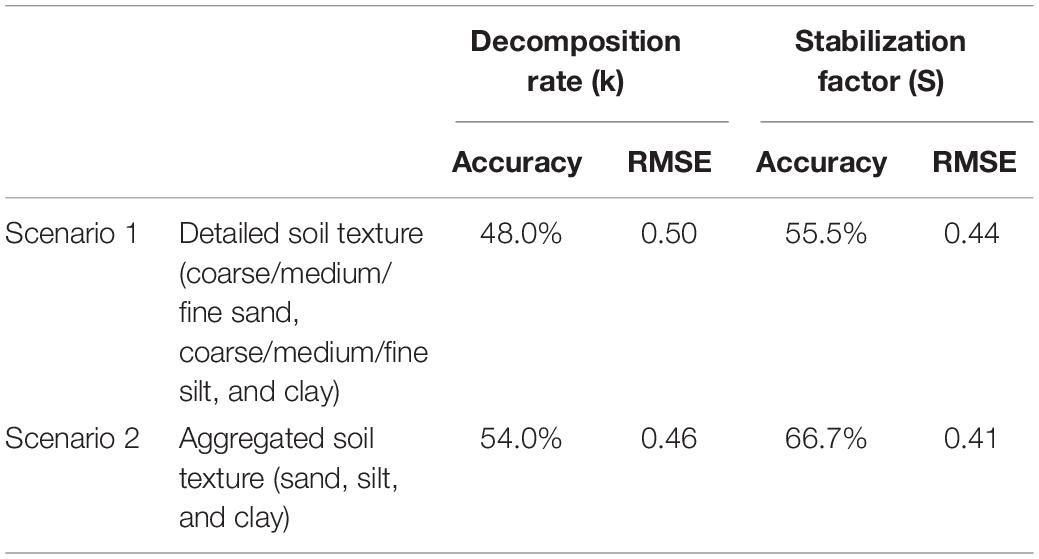
Table 2. Predictive performance (accuracy and RMSE) of the four classification trees generated for modeling decomposition rate and stabilization factor.
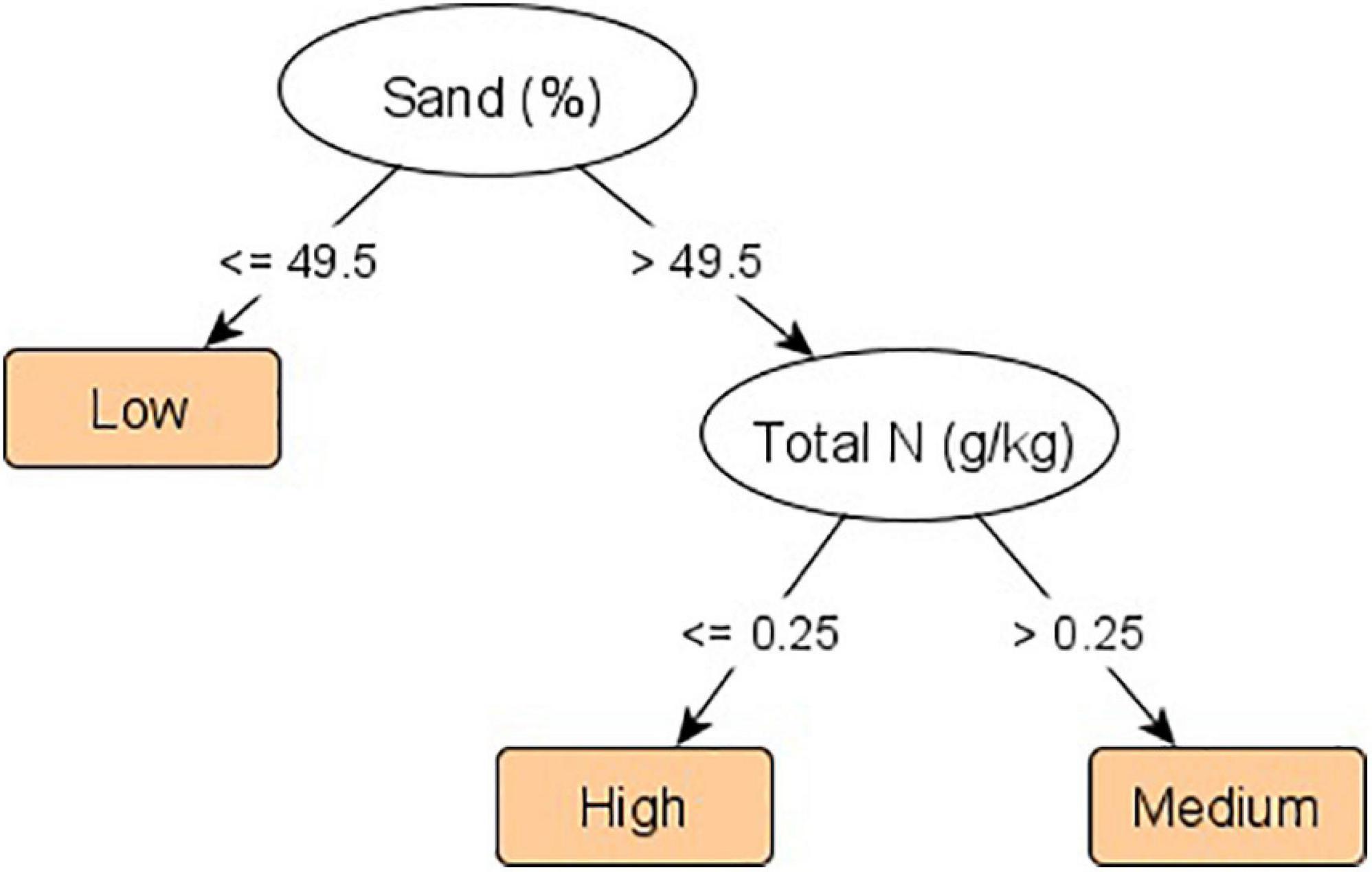
Figure 2. Classification tree for predicting decomposition rate (k). Low represent values (≤0.0127 g d–1), medium values (0.0127–0.0210 g d–1), and high values (>0.0210 g d–1).
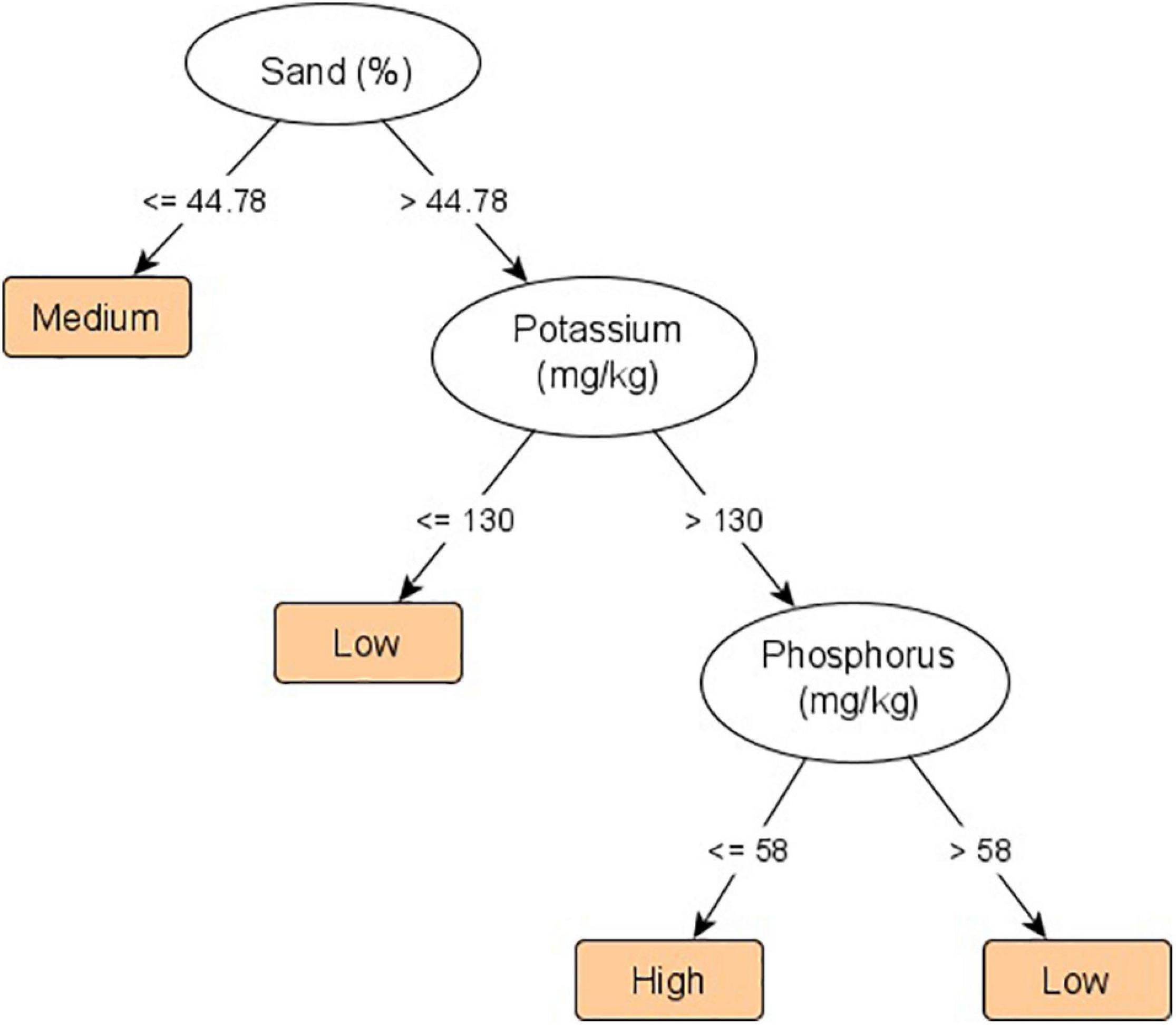
Figure 3. Classification tree for predicting stabilization factor (S). Low represent values (≤0.15), medium values (0.15–0.29), and high values (>0.29).
Discussion
Litter Decomposition
Our results support both previous global (Keuskamp et al., 2013) and local (Sandén et al., 2020) studies on litter decomposition based on the Tea Bag Index methodology. The decomposition rates we calculated were similar to those reported for the Pannonian environmental zone by Sandén et al. (2020), which reflects the fact that most of our present sites are in the Pannonian environmental zone. The stabilization factors were somewhat higher, 0.20 compared to 0.14, than for the Pannonian environmental zone in Sandén et al. (2020), possibly due to the nature of urban soils in our study compared to croplands, grasslands and forests in the other study. Interestingly, our results on stabilization factors agree well with Duddigan et al. (2020) who studied Tea Bag Index in domestic gardens in the United Kingdom, even though the climatic factors were quite different to our sites. Fung et al. (2021) recently reported on litter decomposition using Tea Bag Index in urban Singapore soils and Tresch et al. (2018) from urban gardens in Switzerland, however, they did not calculate the decomposition rates and stabilization factors, but only presented the total mass losses over the burial time that makes comparison of results more difficult. Litter decomposition dynamics in urban soils may be hampered or accelerated because the decomposer community may be disrupted or their microhabitat destroyed (Byrne, 2007). Moreover, the burial of topsoil decomposers or exposure of subsoil decomposers to topsoil conditions (Craul, 1999) due to mechanical soil mixing might explain the different decomposition dynamics. Soil pH changes due to the human influence on urban soils may also affect decomposer communities (Beyer et al., 1995). showed that urbanization may affect decomposition by altering leaf litter quality. They investigated decomposition of five different litters and found that the local litter was decomposing fastest in urban areas (Dorendorf et al., 2015). This can be explained by the home-field advantage (HFA) hypothesis that has been shown in many environments, and estimated to contribute to about 7.5% faster decomposition at the site where the litter originates compared to other litters on a global scale (Veen et al., 2015). In our case, the litter, i.e., green tea and rooibos, are the same in each study site and therefore comparisons between urban and rural areas would be easy to conduct. To get a better overview of differences between Tea Bag Index in urban and rural soils, further data collection from both urban and rural areas should be undertaken. A possible example for how to collect further data in rural areas could be to utilize the Long Term Socio-Ecological Research platforms (LTSER), as has been done in France (Bretagnolle et al., 2018).
The main governing factor for both decomposition rate and stabilization factor was the sand content of the soils according to our data mining models (Figures 2, 3). Soil texture is known to influence decomposition in many ways, including its effects on soil water dynamics, nutrient availability and pore size distribution. Note, however, that its influence on decomposition may sometimes be shown only in combination with its effects on soil water pressure, as in Scott et al. (1996), or not be evident at all as in McLauchlan (2006). Many modeling approaches of soil organic matter dynamics such as century (Parton et al., 1987) or RothC (Jenkinson et al., 1990) focus on silt and clay contents instead of the sand content of the soil. In our case, higher decomposition rates at increased sand contents (>49.5%) may be attributable to suitable pore size distribution for soil microbes to move and to distribute microbial substrates (Scott et al., 1996). One reason why clay content did not appear as an important factor for stabilization in our decision trees could be the time aspect; the tea bags were buried in the soil for only 3 months, which is an ideal timeframe for studying the initial decomposition dynamics. These may likely be more influenced by the sand than by the clay content.
Another important driver for decomposition rates was total nitrogen (Figure 2). The importance of plant available potassium and phosphorous became evident in the model created for the stabilization factor (Figure 3); that model shows that, even in urban areas, fertilization affects soil biochemical cycles (Lorenz and Lal, 2009). The stabilization factor was higher at low to medium phosphorous contents up to 58 mg kg–1, whereas for potassium at least 130 mg kg–1 was needed for higher stabilization factors. Both values of plant available nutrients represent sufficient levels for productivity for grasslands and croplands in Austria (BMLFUW, 2017).
Future Potentials and Trends
The barriers between science and the public (Wildschut, 2017) can be opened by showing that citizen scientists’ data are a very valuable contribution to science. This is especially true when important patterns are revealed by data mining. Ryan et al. (2018) described the benefits of technology for citizen science as a way to convert data into usable information. This is precisely what data mining can do for data collected by citizen scientists. Such data mining poses both challenges and opportunities (Franzen et al., 2021). In this era of digitalization and an increasingly data-oriented society, the use of data mining to understand, analyze and obtain new knowledge is inevitable. Importantly, applying data mining methods to citizen science data requires caution to avoid potential bias and counter the varying quality of the data. The benefits and opportunities of this approach clearly outweigh the challenges. Our study, by applying data mining and generating predictive models from the Tea Bag Index data, has set the basis for creating educational materials for schools about the importance of analyzing urban soils. This sets the framework for planning experiments in order to collect more data and improving the experimental setup in order to obtain wider coverage on regional or national scales. Despite the modest predictive performance of the created models, they are informative for understanding and describing litter decomposition in urban settings where very little data exist (Guilland et al., 2018). To improve the models, the most important task is to collect more data on the Tea Bag Index and on the soil attributes governing litter decomposition. This includes at least soil texture (sand, silt, clay contents), total nitrogen and plant-available phosphorous and potassium.
Showing the results of the analyses to and discussing them with the citizen scientists, i.e., the participating teachers and students, showcases the importance of their involvement in scientific knowledge creation. This is a major motivating factor for their future involvement in similar citizen science projects and may even help inspire careers in science. By sharing our results in social media and through our school network connections, we expect our results to encourage citizen scientists to question the natural phenomena of litter decomposition and its connection to the global carbon cycle. We hope this motivates them to continue their discoveries on their own or in cooperation with us. Previous research has shown that a brief training of citizen scientists may not increase science literacy or overall attitudes (Crall et al., 2013). We therefore aim at several interaction points with the teachers and students to foster future engagement in environmental issues. If a teacher or student is seen as an opinion leader, that person may be able to motivate others to change their personal behavior toward a more environmentally friendly lifestyle (Nisbet and Kotcher, 2009). This multiplies the knowledge gained through their participation. We aim to help citizen scientists understand that the predictive performance of the created models—and of any models—can only be as good as the data behind them, and that better models may require more data, more citizen scientists and/or even more precise data.
Through litter decomposition experiments we aim to generate new knowledge, create learning opportunities for both scientists and citizen scientists, and enable civic participation in science—three aspects that were highlighted as innovation potentials of citizen science by Turrini et al. (2018). In order to make it simpler for new citizen scientists to participate and to enable previous participants to continue their discoveries with new categories of soil characterizations, the Tea Bag Index App was created and launched in 2019, in cooperation with agricultural high school students and their teacher6. This approach promotes long-term engagement of citizen scientists, motivating them to remain active and communicate with other citizen scientists on the Tea Bag Index App or other citizen science Apps running on the same Spotteron Platform7. We envision that this will increase the value of the precious time that the citizen scientists devote and that we essentially borrow for scientific purposes (Ryan et al., 2018). Such cooperation may lead to long-lasting collaborations in which important knowledge networks, as are becoming increasingly common in agriculture (Baumgart-Getz et al., 2012), are being created and science is being done for the people, by the people.
Conclusion
Our study successfully created new scientific knowledge on litter decomposition, i.e., decomposition rates (k) and stabilization factors (S), in cooperation with school students. This approach created new soil science learning opportunities for students in cooperation with their teachers. The most important factor for both k and S was the sand content of the soils. The decomposition rates were also affected by total nitrogen contents of the soils, whereas the stabilization factor was additionally governed by plant-available potassium and phosphorous. We generated data mining models for decomposition rate (k) and stabilization factor (S) from citizen-scientist-collected Tea Bag Index (TBI) data that are representative for poorly studied urban soils. The predictive performance of the generated models, 54.0 and 66.7% for k and S, respectively, could further be improved by additional data collection and subsequent data mining, as is planned with the newly launched Tea Bag Index App. Future discussions about the generated models in the framework of school workshops will be a next major step in connecting citizen scientists with the results and promoting conversations about the driving factors of decomposition dynamics. This is aimed at establishing a long-term participatory cooperation in which citizen scientists and researchers learn from one another and new ground will be broken in participatory citizen soil science.
Data Availability Statement
The raw data supporting the conclusions of this article will be made available by the authors, without undue reservation. The data will be included in the TBI database that will be published online on www.teatime4science.org after publication of global results. Until publication on this platform, the data can be obtained by emailing TS, taru.sanden@ages.at.
Author Contributions
TS, AW, HB, JM, AS, BG, and HS were mainly responsible for the generation of the dataset with the help of citizen scientists. AT and MD were mainly responsible for the data mining and the analyses of those results. TS and AT did most of the writing. All authors contributed to the manuscript revision, read, and approved the submitted version.
Funding
This study was conducted as part of the TeaTime4Schools project that received funding from the Austrian Ministry of Education, Science and Research (BMBWF) through a Sparkling Science Grant (SPA 06/044).
Conflict of Interest
AS was employed by the company EASY-CERT Services GmbH.
The remaining authors declare that the research was conducted in the absence of any commercial or financial relationships that could be construed as a potential conflict of interest.
Acknowledgments
We acknowledge the 130 school classes and more than 2,376 school students across Austria that made this research possible. Jasmin Janisch, Philipp de Jong, Samira Puthuparambil, and Julia Hekerle are acknowledged for their help in contacting the teachers, data check-up and material preparation. Michael Schwarz (AGES, Austria) created Figure 1. Michael Stachowitsch is acknowledged for English proofreading.
Footnotes
- ^ https://teatime4schools.at/
- ^ https://www.youtube.com/playlist?list=PLR7SjaVR2HuOzcyVGYch5s0ECGMtgPUHc
- ^ http://www.teatime4science.org/data/submit-one-data-point/
- ^ https://www.zamg.ac.at/cms/de/klima/klimauebersichten/jahrbuch
- ^ http://www.teatime4science.org/
- ^ https://teatime4schools.at/en/teatime4app/
- ^ https://www.spotteron.net/de/citizen-science-apps
References
Balázs, B., Mooney, P., Nováková, E., Bastin, L., and Jokar Arsanjani, J. (2021). “Data Quality in Citizen Science,” in The Science of Citizen Science, eds K. Vohland, A. Land-Zandstra, L. Ceccaroni, R. Lemmens, J. Perelló, M. Ponti, et al. (Cham: Springer International Publishing), 139–157. doi: 10.1007/978-3-030-58278-4_8
Baumgart-Getz, A., Prokopy, L. S., and Floress, K. (2012). Why farmers adopt best management practice in the United States: a meta-analysis of the adoption literature. J. Environ. Manag. 96, 17–25. doi: 10.1016/j.jenvman.2011.10.006
Beyer, L., Blume, H.-P., Elsner, D.-C., and Willnow, A. (1995). Soil organic matter composition and microbial activity in urban soils. Sci. Total Environ. 168, 267–278. doi: 10.1016/0048-9697(95)04704-5
BMLFUW (2017). Richtlinien für die sachgerechte Düngung, 7th Edn. Vienna: Bundesministerium für Land- und Forstwirtschaft, Umwelt und Wasserwirtschaft.
Breiman, L., Friedman, J., Stone, C. J., and Olshen, R. A. (1984). Classification and Regression Trees. U.K.: Taylor & Francis.
Bretagnolle, V., Berthet, E., Gross, N., Gauffre, B., Plumejeaud, C., Houte, S., et al. (2018). Towards sustainable and multifunctional agriculture in farmland landscapes: lessons from the integrative approach of a French LTSER platform. Sci. Total Environ. 627, 822–834. doi: 10.1016/j.scitotenv.2018.01.142
Byrne, L. B. (2007). Habitat structure: a fundamental concept and framework for urban soil ecology. Urban Ecosyst. 10, 255–274. doi: 10.1007/s11252-007-0027-6
Ceccaroni, L., Bibby, J., Roger, E., Flemons, P., Michael, K., Fagan, L., et al. (2019). Opportunities and Risks for Citizen Science in the Age of Artificial Intelligence. Citiz. Sci. 4, 1–14. doi: 10.5334/cstp.241
Chen, D., and Gomes, C. P. (2019). “Bias Reduction via End-to-End Shift Learning: application to Citizen Science,” in The Thirty-Third AAAI Conference on Artificial Intelligence (AAAI-19) (California: Association for the Advancement of Artificial Intelligence), 493–500. doi: 10.1609/aaai.v33i01.3301493
Churkina, G. (2016). The Role of Urbanization in the Global Carbon Cycle. Front. Ecol. Evol. 3:144. doi: 10.3389/fevo.2015.00144
Crall, A. W., Jordan, R., Holfelder, K., Newman, G. J., Graham, J., and Waller, D. M. (2013). The impacts of an invasive species citizen science training program on participant attitudes, behavior, and science literacy. Pub. Underst. Sci. 22, 745–764. doi: 10.1177/0963662511434894
Dorendorf, J., Wilken, A., Eschenbach, A., and Jensen, K. (2015). Urban-induced changes in tree leaf litter accelerate decomposition. Ecol. Process. 4:1. doi: 10.1186/s13717-014-0026-5
Duddigan, S., Alexander, P. D., Shaw, L. J., Sandén, T., and Collins, C. D. (2020). The Tea Bag Index—UK: using Citizen/Community Science to Investigate Organic Matter Decomposition Rates in Domestic Gardens. Sustainability 12:6895. doi: 10.3390/su12176895
Edmondson, J. L., Davies, Z. G., McHugh, N., Gaston, K. J., and Leake, J. R. (2012). Organic carbon hidden in urban ecosystems. Sci. Rep. 2:963. doi: 10.1038/srep00963
Franzen, M., Kloetzer, L., Ponti, M., Trojan, J., and Vicens, J. (2021). “Machine Learning in Citizen Science: promises and Implications,” in The Science of Citizen Science, eds K. Vohland, A. Land-Zandstra, L. Ceccaroni, R. Lemmens, J. Perelló, M. Ponti, et al. (Cham: Springer International Publishing), 183–198. doi: 10.1007/978-3-030-58278-4_10
Fung, T. K., Richards, D. R., Leong, R. A. T., Ghosh, S., Tan, C. W. J., Drillet, Z., et al. (2021). Litter decomposition and infiltration capacities in soils of different tropical urban land covers. Urban Ecosyst. doi: 10.1007/s11252-021-01126-2 [Epub ahead of print].
Guilland, C., Maron, P. A., Damas, O., and Ranjard, L. (2018). Biodiversity of urban soils for sustainable cities. Environ. Chem. Lett. 16, 1267–1282. doi: 10.1007/s10311-018-0751-6
Harnik, P. G., and Ross, R. M. (2003). Developing Effective K-16 Geoscience Research Partnerships. J. Geosci. Educ. 51, 5–8. doi: 10.5408/1089-9995-51.1.5
Jenkinson, D. S., Andrew, S. P. S., Lynch, J. M., Goss, M. J., Tinker, P. B., Greenwood, D. J., et al. (1990). The turnover of organic carbon and nitrogen in soil. Philos. Trans. R. Soc. Lond. B Biol. Sci. 329, 361–368. doi: 10.1098/rstb.1990.0177
Kandeler, E. (1993). “Bestimmung der N-Mineralisation im anaeroben Brutversuch,” in Bodenbiologische Arbeitsmethoden, eds. F. Schinner, R. Öhlinger, E. Kandeler, and R. Margesin 2. Auflage ed (Berling: Springer Verlag), 160–161.
Kaye, J. P., Groffman, P. M., Grimm, N. B., Baker, L. A., and Pouyat, R. V. (2006). A distinct urban biogeochemistry? Trends Ecol. Evol. 21, 192–199. doi: 10.1016/j.tree.2005.12.006
Keeney, D. R. (1982). “Nitrogen availability indices,” in Methods of Soil Analysis. Part II., 2nd Edn, eds. A. L. Page, R. H. Millet, and D. R. Keeney (Madison, WI: American Society of Agronomy), 711–730.
Keuskamp, J. A., Dingemans, B. J. J., Lehtinen, T., Sarneel, J. M., and Hefting, M. M. (2013). Tea Bag Index: a novel approach to collect uniform decomposition data across ecosystems. Methods Ecol. Evol. 4, 1070–1075. doi: 10.1111/2041-210X.12097
Lorenz, K., and Lal, R. (2009). Biogeochemical C and N cycles in urban soils. Environ. Int. 35, 1–8. doi: 10.1016/j.envint.2008.05.006
Lorenz, K., and Lal, R. (2017). “Impacts of land take and soil sealing on soil carbon,” in Urban Expansion, Land Cover and Soil Ecosystem Services, 1st Edn, ed. C. Gardi (London: Routledge), 332.
Lukyanenko, R., Wiggins, A., and Rosser, H. K. (2020). Citizen Science: an Information Quality Research Frontier. Inform. Syst. Front. 22, 961–983. doi: 10.1007/s10796-019-09915-z
McDonnell, M. J., Pickett, S. T. A., Groffman, P., Bohlen, P., Pouyat, R. V., Zipperer, W. C., et al. (1997). Ecosystem processes along an urban-to-rural gradient. Urban Ecosyst. 1, 21–36. doi: 10.1023/A:1014359024275
McLauchlan, K. K. (2006). Effects of soil texture on soil carbon and nitrogen dynamics after cessation of agriculture. Geoderma 136, 289–299. doi: 10.1016/j.geoderma.2006.03.053
Nisbet, M. C., and Kotcher, J. E. (2009). A Two-Step Flow of Influence?:Opinion-Leader Campaigns on Climate Change. Sci. Commun. 30, 328–354. doi: 10.1177/1075547008328797
Norra, S. (2009). The astysphere and urban geochemistry—a new approach to integrate urban systems into the geoscientific concept of spheres and a challenging concept of modern geochemistry supporting the sustainable development of planet earth. Environ. Sci. Pollut. Res. 16, 539–545. doi: 10.1007/s11356-009-0183-8
Parton, W. J., Schimel, D. S., Cole, C. V., and Ojima, D. S. (1987). Analysis of Factors Controlling Soil Organic Matter Levels in Great Plains Grasslands. Soil Sci. Soc. Am. J. 51, 1173–1179. doi: 10.2136/sssaj1987.03615995005100050015x
Ryan, S. F., Adamson, N. L., Aktipis, A., Andersen, L. K., Austin, R., Barnes, L., et al. (2018). The role of citizen science in addressing grand challenges in food and agriculture research. Proc. Biol. Sci. 285:20181977. doi: 10.1098/rspb.2018.1977
Sandén, T., Spiegel, H., Wenng, H., Schwarz, M., and Sarneel, J. M. (2020). Learning Science during Teatime: using a Citizen Science Approach to Collect Data on Litter Decomposition in Sweden and Austria. Sustainability 12:7745. doi: 10.3390/su12187745
Schulte, R. P. O., Creamer, R. E., Donnellan, T., Farrelly, N., Fealy, R., O’Donoghue, C., et al. (2014). Functional land management: a framework for managing soil-based ecosystem services for the sustainable intensification of agriculture. Environ. Sci. Policy 38, 45–58. doi: 10.1016/j.envsci.2013.10.002
Scott, N. A., Cole, C. V., Elliott, E. T., and Huffman, S. A. (1996). Soil Textural Control on Decomposition and Soil Organic Matter Dynamics. Soil Sci. Soc. Am. J. 60, 1102–1109. doi: 10.2136/sssaj1996.03615995006000040020x
Seto, K. C., Dhakal, S., Bigio, A., Blanco, H., Delgado, G. C., Dewar, D., et al. (2014). “Human Settlements, Infrastructure and Spatial Planning,” in Climate Change 2014: Mitigation of Climate Change. Contribution of Working Group III to the Fifth Assessment Report of the Intergovernmental Panel on Climate Change, eds O. Edenhofer, R. Pichs-Madruga, Y. Sokona, E. Farahani, S. Kadner, K. Seyboth, et al. (Cambridge: Cambridge University Press), 923–1000. doi: 10.1017/cbo9781107415416.018
Seto, K. C., Güneralp, B., and Hutyra, L. R. (2012). Global forecasts of urban expansion to 2030 and direct impacts on biodiversity and carbon pools. Proc. Natl. Acad. Sci. U. S. A. 109, 16083–16088. doi: 10.1073/pnas.1211658109
Tatzber, M., Schlatter, N., Baumgarten, A., Dersch, G., Körner, R., Lehtinen, T., et al. (2015). KMnO4 determination of active carbon for laboratory routines: three long-term field experiments in Austria. Soil Res. 53, 190–204. doi: 10.1071/SR14200
Trajanov, A., Spiegel, H., Debeljak, M., and Sandén, T. (2018). Using data mining techniques to model primary productivity from international long-term ecological research (ILTER) agricultural experiments in Austria. Reg. Environ. Change 19, 1–13. doi: 10.1007/s10113-018-1361-3
Tresch, S., Moretti, M., Le Bayon, R.-C., Mäder, P., Zanetta, A., Frey, D., et al. (2018). A Gardener’s Influence on Urban Soil Quality. Front. Environ. Sci. 6:25. doi: 10.3389/fenvs.2018.00025
Turrini, T., Dörler, D., Richter, A., Heigl, F., and Bonn, A. (2018). The threefold potential of environmental citizen science - Generating knowledge, creating learning opportunities and enabling civic participation. Biol. Conserv. 225, 176–186. doi: 10.1016/j.biocon.2018.03.024
van Dijk-Wesselius, J. E., van den Berg, A. E., Maas, J., and Hovinga, D. (2020). Green Schoolyards as Outdoor Learning Environments: barriers and Solutions as Experienced by Primary School Teachers. Front. Psychol. 10:2919. doi: 10.3389/fpsyg.2019.02919
Veen, G. F., Freschet, G. T., Ordonez, A., and Wardle, D. A. (2015). Litter quality and environmental controls of home-field advantage effects on litter decomposition. Oikos 124, 187–195. doi: 10.1111/oik.01374
Wildschut, D. (2017). The need for citizen science in the transition to a sustainable peer-to-peer-society. Futures 91, 46–52. doi: 10.1016/j.futures.2016.11.010
Witten, I. H., Frank, E., and Hall, M. A. (2011). Data Mining : Practical Machine Learning Tools and Techniques, 3rd Edn. Burlington: Morgan Kaufmann.
Keywords: Tea Bag Index (TBI), decomposition rate (k), stabilization factor (S), citizen science, knowledge discovery, machine learning, classification trees
Citation: Sandén T, Wawra A, Berthold H, Miloczki J, Schweinzer A, Gschmeidler B, Spiegel H, Debeljak M and Trajanov A (2021) TeaTime4Schools: Using Data Mining Techniques to Model Litter Decomposition in Austrian Urban School Soils. Front. Ecol. Evol. 9:703794. doi: 10.3389/fevo.2021.703794
Received: 30 April 2021; Accepted: 09 June 2021;
Published: 28 June 2021.
Edited by:
Piotr Tryjanowski, Poznań University of Life Sciences, PolandReviewed by:
Andrzej M. Jagodziński, Institute of Dendrology, Polish Academy of Sciences (PAN), PolandReuven Yosef, Ben-Gurion University of the Negev, Israel
Copyright © 2021 Sandén, Wawra, Berthold, Miloczki, Schweinzer, Gschmeidler, Spiegel, Debeljak and Trajanov. This is an open-access article distributed under the terms of the Creative Commons Attribution License (CC BY). The use, distribution or reproduction in other forums is permitted, provided the original author(s) and the copyright owner(s) are credited and that the original publication in this journal is cited, in accordance with accepted academic practice. No use, distribution or reproduction is permitted which does not comply with these terms.
*Correspondence: Taru Sandén, taru.sanden@ages.at