- Department of Biology, Indian Institute of Science Education and Research (IISER), Pune, India
The diversity of animal acoustic signals has evolved due to multiple ecological processes, both biotic and abiotic. At the level of communities of signaling animals, these processes may lead to diverse outcomes, including partitioning of acoustic signals along multiple axes (divergent signal parameters, signaling locations, and timing). Acoustic data provides information on the organization, diversity and dynamics of an acoustic community, and thus enables study of ecological change and turnover in a non-intrusive way. In this review, we lay out how community bioacoustics (the study of acoustic community structure and dynamics), has value in ecological monitoring and conservation of diverse landscapes and taxa. First, we review the concepts of signal space, signal partitioning and their effects on the structure of acoustic communities. Next, we highlight how spatiotemporal ecological change is reflected in acoustic community structure, and the potential this presents in monitoring and conservation. As passive acoustic monitoring gains popularity worldwide, we propose that the analytical framework of community bioacoustics has promise in studying the response of entire suites of species (from insects to large whales) to rapid anthropogenic change.
Introduction
With the ongoing global crisis of biodiversity loss, passive acoustic monitoring has gained popularity in both aquatic and terrestrial environments (Magurran et al., 2010; Blumstein et al., 2011; Parks et al., 2014; Linke et al., 2018; Sugai et al., 2019b), employing sound to monitor wildlife. Animal sounds are diverse and varied, and play a critical role in advertising territories and attracting mates (Marler and Slabbekoorn, 2004; Bradbury and Vehrencamp, 2011), as well as echolocation in some species (Griffin, 1958). Acoustic signal parameters and signaling strategies are influenced by noise from biotic and abiotic sources, structural features of the environment (Morton, 1975; Marten and Marler, 1977; Wiley and Richards, 1978; Wiley, 1991; Aubin and Jouventin, 1998; Nemeth et al., 2002; Blumenrath and Dabelsteen, 2004; Brumm and Slabbekoorn, 2005; Bee and Micheyl, 2008; Brumm and Naguib, 2009; Ruppé et al., 2015; Templeton et al., 2016), and the morphological constraints imposed by emitter geometry (Podos, 2001; Riede et al., 2006; Kounitsky et al., 2015). In order to compare signal parameters of different species, Nelson and Marler (1990) articulated the concept of “signal space,” where signal parameters could be quantitatively represented. Multiple factors, both evolutionary and contemporary, may influence how signals are distributed in signal space. For example, acoustic competition (i.e., competition between signalers to communicate without interference) is predicted to lead to divergence in signal parameters of coexisting species, particularly those that are closely related, such that each species in an assemblage occupies a unique region in signal space [birds: (Planqué and Slabbekoorn, 2008; Luther, 2009; Krishnan and Tamma, 2016; Krishnan, 2019a; Chitnis et al., 2020), anurans: (Littlejohn, 1959; Duellman and Pyles, 1983; Chek et al., 2003), insects: (Schmidt et al., 2013; Jain et al., 2014)]. In a scenario where competition drives signal evolution, sympatric species may also signal at different times [birds:(Ficken et al., 1974; Fleischer et al., 1985; Popp et al., 1985; Brumm, 2006; Luther, 2008; Planqué and Slabbekoorn, 2008), anurans: (Sugai et al., 2021b), fish: (Ruppé et al., 2015)] or locations in space [birds: (Nemeth et al., 2002; Chitnis et al., 2020), anurans: (Hodl, 1977), insects: (Diwakar and Balakrishnan, 2007; Jain and Balakrishnan, 2012), bats: (Kennedy et al., 2014)]. The distributions of species in signal space represent a “signature” of each community, a definitive pattern that can be monitored to track ecological changes. These “acoustic communities” represent the sum of all sound-producing species in an aquatic or terrestrial landscape (Drewry and Rand, 1983; Duellman and Pyles, 1983; Luther, 2009; Sueur and Farina, 2015; Farina and James, 2016). Community bioacoustics integrates the signal space of an acoustic community with information on species composition, diversity and signaling activity to study spatiotemporal community dynamics (i.e., seasonal and spatial variation in species composition or phylogenetic diversity) (Luther, 2008, 2009; Krishnan, 2019a; Lahiri et al., 2021). The term “acoustic community structure” (Table 1) adapts the concept of ecological community structure, employing acoustic parameters and signaling activity as measurable traits to examine the distribution of sound-producing species in signal space, together with measures of species composition and phylogenetic diversity. The wealth of data generated by passive acoustic monitoring efforts promises valuable ecological insight, both into the distributions and movements of individual species as well as community-level processes such as seasonal turnover.
Here, we discuss how ecological processes drive acoustic community structure, and propose using acoustic communities to track ecological change. This serves to facilitate non-invasive biodiversity monitoring and conservation informed by behavior, ecology and natural history. The ubiquity and diversity of animal acoustic signals renders them promising indicators of ecosystem health (Sueur and Farina, 2015; Sugai et al., 2019b). Most conservation-oriented studies using passive acoustics typically use either single-species monitoring, or index-driven approaches. We suggest that biodiversity monitoring programs incorporate information on acoustic community composition and spatiotemporal dynamics (Desjonquères et al., 2018; Gasc et al., 2018; Krishnan, 2019a) to effectively and rapidly study species distributions and community turnover. This serves both as an additional use of passive acoustic data, as well as an alternative to other approaches in the field, depending on the needs of conservation. Community bioacoustics is thus potentially valuable to ecologists and conservation biologists working across scales from individual species to entire ecosystems.
Conceptual Foundations
Before examining how acoustic communities inform ecological studies, it is important to understand how ecological processes drive patterns in acoustic community structure (Krishnan and Tamma, 2016; Sugai et al., 2021a). To illustrate this concept, we use some examples from some of the main hypotheses in the field. For effective communication, a high signal-to-noise ratio ensures improved detection by the receiver, thus benefiting the signaler (Endler, 1992; Wollerman and Wiley, 2002; Hart et al., 2015; Vélez et al., 2017). However, signal efficacy is reduced by masking interference from competing sound sources, such as other nearby signalers (Schwartz and Wells, 1983; Narins, 1992; Greenfield, 1994; Aubin and Jouventin, 1998; Bee and Micheyl, 2008; Balakrishnan et al., 2014). Divergence of signal properties reduces masking, enables segregation of competing sound streams (MacDougall-Shackleton et al., 1998; Krishnan, 2019b), and thus reinforces species recognition and premating isolation between close relatives (Nelson, 1988, 1989; Grant and Grant, 1996; Qvarnström et al., 2006). In diverse animals [crickets: (Schmidt et al., 2013), cicadas: (Shieh et al., 2015), aquatic insects: (Gottesman et al., 2020), fish: (Ruppé et al., 2015; Bertucci et al., 2020), anurans: (Drewry and Rand, 1983; Duellman and Pyles, 1983; Narins, 1995; Chek et al., 2003), birds: (Kirschel et al., 2009b, 2020; Krishnan and Tamma, 2016; Chitnis et al., 2020), bats: (Heller and von Helversen, 1989; Kingston et al., 2000; Luo et al., 2019), primates: (Braune et al., 2008)], closely related sympatric species exhibit divergent signals, partitioning the acoustic resource to minimize acoustic competition. Each species is therefore predicted to occupy a unique region or “niche” in the acoustic resource, a hypothesis extrapolated from ecological niche theory (Hutchinson, 1957; Holt, 2009). This realized “acoustic niche” (Farina et al., 2011; Pijanowski et al., 2011b), in the truest sense also includes partitioning at the level of receiver perception (Luther, 2008; Lemmon, 2009; Seddon and Tobias, 2010; Amezquita et al., 2011) and measurement of signal propagation distance (Marten and Marler, 1977; Nemeth et al., 2002). The presence of unintended recipients may alter signaling behavior (Ryan et al., 1982; Zuk et al., 2006). All of these factors influence the volume over which the signal elicits a response, or its “active space” (Brenowitz, 1982; Lohr et al., 2003; Bradbury and Vehrencamp, 2011), and are thus important in measuring overall signal discriminability. However, it is not always possible to investigate receiver partitioning in the field, and most studies of signal partitioning are, therefore, concerned with divergence at the level of signal parameters and the spatiotemporal distribution of signalers.
Competition, however, is not the only factor influencing signal evolution, and thus acoustic traits within communities of species. For example, habitat constraints on sound transmission are predicted to drive signal convergence toward parameters optimal for transmission in a certain habitat (the acoustic adaptation hypothesis, where acoustic signals adapt toward maximized transmission in a particular habitat) (Morton, 1975; Marten and Marler, 1977; Wiley, 1991; Snell-Rood, 2012). In bats, where time-frequency parameters of echolocation pulses have a significant effect on perception and navigation, species occupying the same habitat may possess convergent signals (Parsons and Jones, 2000; Jones and Holderied, 2007). Other vocalizations may also converge owing to conserved function, including alarm calls that are critical for survival (Marler and Slabbekoorn, 2004; Braune et al., 2008). Acoustic adaptation remains debated in the field (Ey and Fischer, 2009), and more studies are necessary to elucidate how ecological processes drive acoustic signal evolution. Patterns of divergence or convergence of signals may also be driven by other factors. For example, sexual selection may drive signal divergence by female choice (Seddon et al., 2013), whereas communication between competing species (extended communication networks) may drive signal convergence (Tobias et al., 2014). Alternatively, neutral processes may drive entirely different patterns in signal evolution, such as signals resembling each other on account of phylogenetic relatedness (Wilkins et al., 2013). Thus, although we discuss patterns of divergence or convergence as examples to illustrate our analytical framework, we note that the drivers of these patterns are diverse. Regardless of this, the patterns in acoustic community structure may themselves provide valuable information, and we use the examples discussed above to illustrate the utility of this approach.
A Framework to Quantify Acoustic Community Structure
The first step in understanding acoustic community structure is to quantify the acoustic traits of all species in a community. The concept of signal space provides a means to quantitatively interrogate whether the signals of coexisting species overlap. Broadly, signal space encompasses spectral and temporal properties of a sound (Figure 1), by quantifying parameters of a vocal unit, for example, a note (parameters include peak frequency and note duration, to name a few) and representing them as a point in multidimensional space. The entire repertoire forms a cloud of such points, the volume of which represents each species’ signal space (Nelson and Marler, 1990), which is constrained both by physical characteristics and the transmissibility of sound (Wilkins et al., 2013). Signal space of diverse sensory signals can be examined at scales ranging from individual species to entire communities or lineages, analogous to a functional trait space in community ecology (Endler, 1992; Stoddard and Prum, 2008; Luther, 2009; Krishnan, 2019a; Chitnis et al., 2020; Krishnan et al., 2020; Lahiri et al., 2021). As an outcome of diverse ecological processes, an acoustic community exhibits organization within signal space. Using the tools of community ecology and community phylogenetics, we may obtain a series of metrics that quantitatively represent the acoustic community, and we lay these out below.
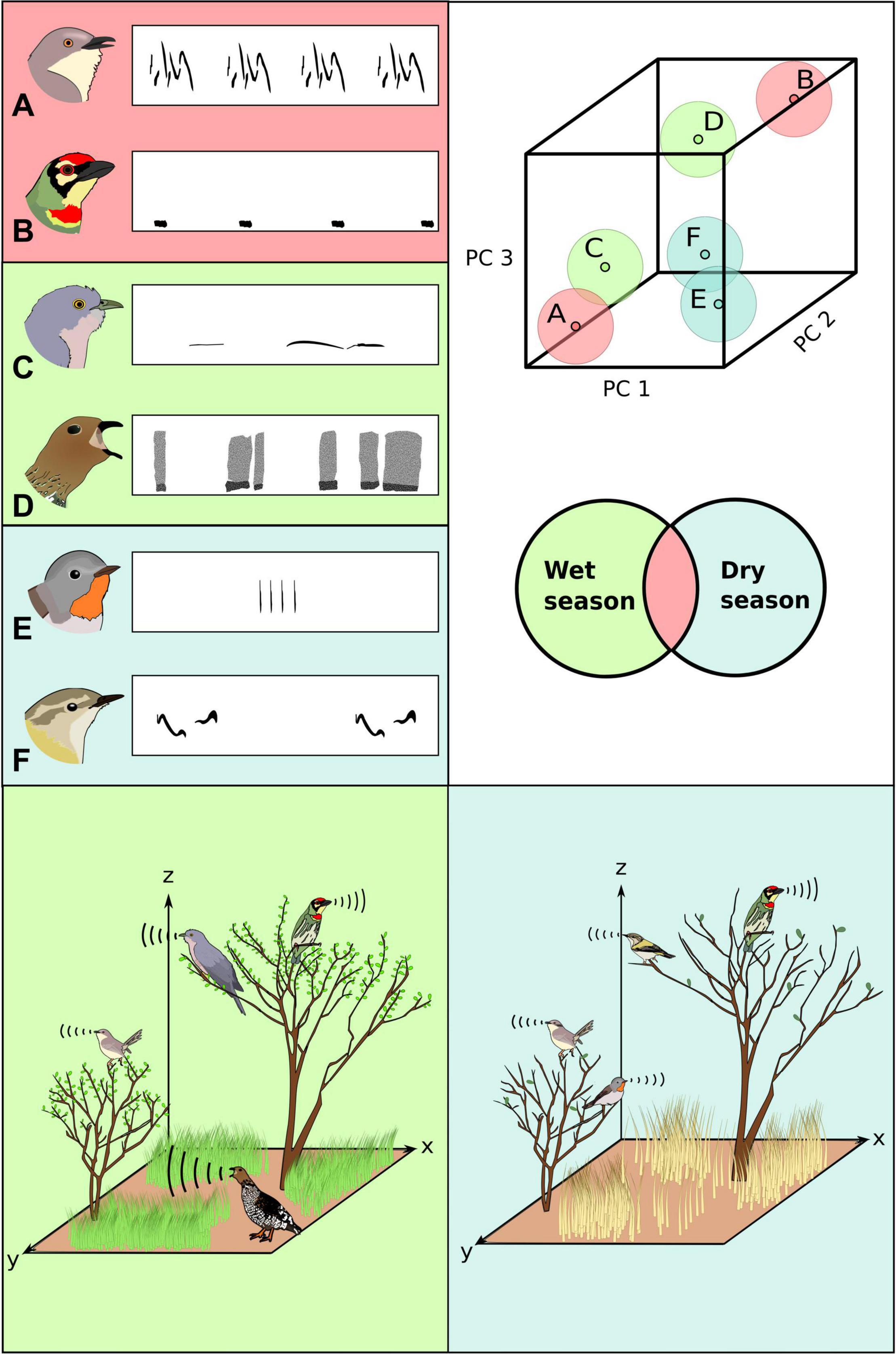
Figure 1. Signal space (top right), derived using principal components analysis on acoustic signal parameters of diverse species (A–F, top left) enables study of acoustic communities across spatiotemporal scales, including different seasons (color codes). Signal space for each species is represented as the volume occupied by the entire repertoire (larger circles, with the smaller point representing the centroid). Bottom panels: Acoustic community structure varies along both spatial and temporal axes (color codes represent different seasons). This figure loosely depicts some bird species from an acoustic community in western India for illustrative purposes, demonstrating seasonal turnover, with species replacing each other, and spatial segregation, with each species singing from a preferred location in space (Krishnan, 2019a).
At the outset, it is important to quantify species diversity and signal space of any acoustic community. For the first, a census of species detected in an acoustic dataset, together with measures of how frequently their vocalizations are detected, provides an estimate of community diversity. In order to quantify community signal space, a call library consisting of the signals of each species is digitized to extract signal parameters (Luther, 2009; Krishnan, 2019a; Lahiri et al., 2021), which are then ordinated using multivariate methods such as principal components or linear discriminant analysis. Thus, all sounds in a community are represented as points in signal space. Ideally, the digitized signals should represent intra- and interspecific variability in signals within the population under consideration, and may be obtained either directly from passive recordings or by making focal recordings to build a call library before passive sampling. The latter is more reliable in poorly studied areas, as it enables researchers to match vocalizations to species before passive sampling.
Signal partitioning is an example of a process which is predicted to lead to overdispersion, where points representing the different acoustic signals are further apart than expected by chance (Chek et al., 2003). The converse is true in case of signal convergence, where clustering around a central tendency is predicted. Clustering may also arise due to phylogenetic relatedness of coexisting species, as the signals of close relatives are likely to resemble each other (i.e., they are phylogenetically conserved) (Figure 2), and quantifying the phylogenetic signal in acoustic traits provides a way to test these hypotheses. Studies have uncovered significant overdispersion in some bird acoustic communities (Luther, 2009; Krishnan, 2019a; Chitnis et al., 2020). These studies hypothesize that acoustic competition may drive the structure of the acoustic community by divergence of time-frequency parameters. Other studies, however, suggest that environmental filtering (for example, acoustic adaptation to habitat features) may result in community convergence instead (Planqué and Slabbekoorn, 2008; Cardoso and Price, 2010). These examples illustrate how the various drivers of signal evolution may lead to distinct patterns in the signal space of a community.
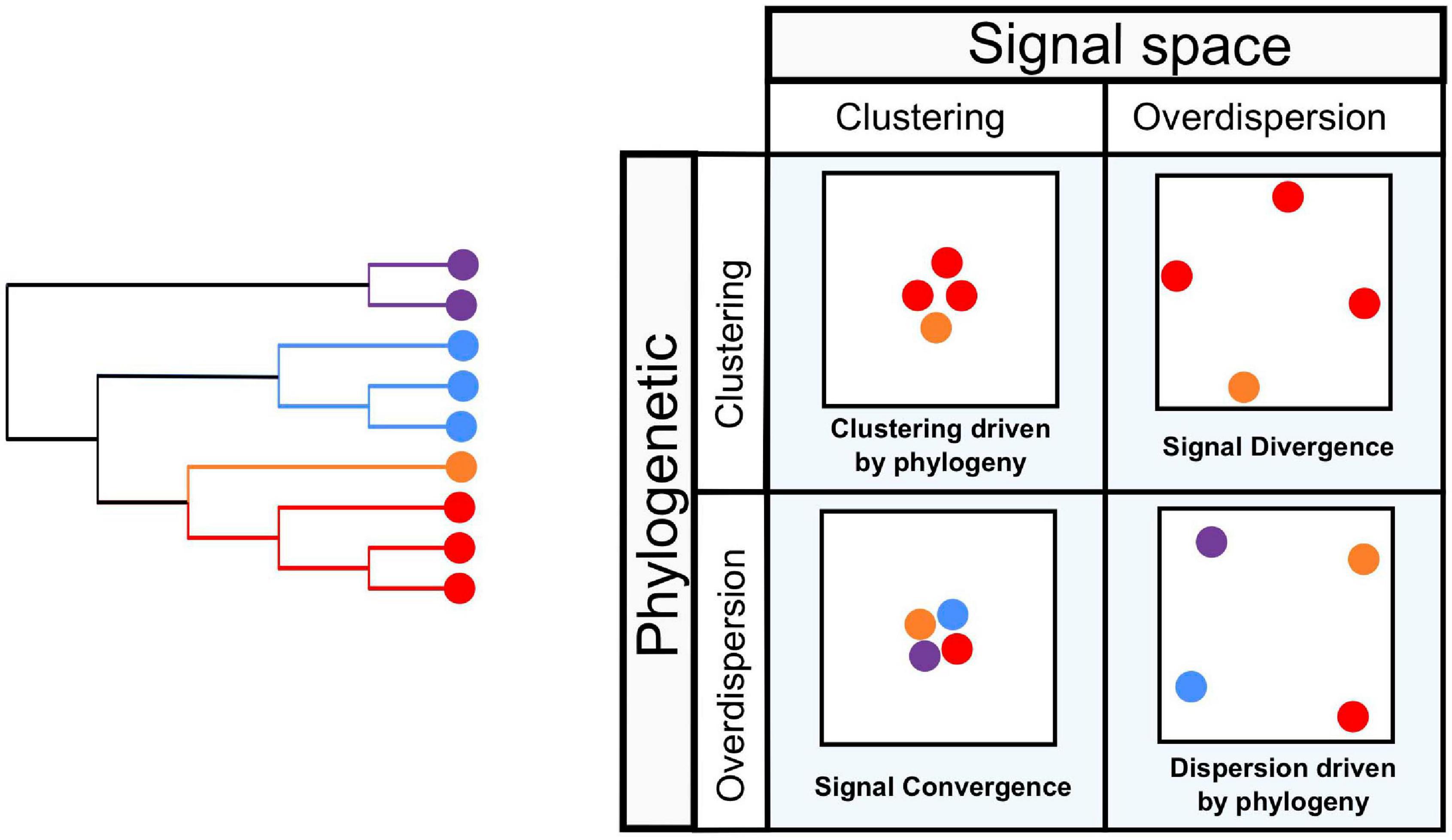
Figure 2. Community phylogenetic analyses and signal space. Phylogenetic information is an important component in our understanding of acoustic community assembly and structure. In a hypothetical, simplified series of communities drawn from the phylogeny on the left [inspired by the principles of community phylogenetics (Webb, 2000; Cavender-Bares et al., 2004)], phylogenetic overdispersion or clustering combined with similar patterns in signal space suggest that phylogenetic relationships drive acoustic community structure (top left and bottom right squares), whereas other patterns may be driven by signal partitioning or convergence, among other processes (top right and bottom left).
In order to statistically examine overdispersion versus convergence in signal space, studies typically employ null model tests (Chek et al., 2003; Chitnis et al., 2020; Sugai et al., 2021a). Null model tests in community ecology compare observed interspecific trait differences or co-occurrence patterns to those in randomly distributed “null” communities (Harvey et al., 1983; Gotelli, 2000; Gotelli and Entsminger, 2001; Gotelli and McCabe, 2002). These tests thus inform us whether species are more likely to co-occur, or certain community trait values are more likely to exist than expected by chance. When studying acoustic communities, null model tests are employed to detect whether species are further apart or closer in signal space than expected by chance (Chek et al., 2003). Interspecific distances greater than those in the null communities are consistent with overdispersion, or signal divergence, whereas clustering is consistent with signal convergence or phylogenetic conservatism (Planqué and Slabbekoorn, 2008; Cardoso and Price, 2010; Schmidt et al., 2013; Tobias et al., 2014).
With detailed phylogenetic information now available for many taxa that produce acoustic signals (Jetz et al., 2012; Shi and Rabosky, 2015), the tools of community phylogenetic analysis (Webb, 2000; Cavender-Bares et al., 2004; Kembel et al., 2010) help us test hypotheses about the role of processes such as competition or environmental filtering in driving the distribution of points in signal space (Figure 2). These methods combine quantification of community phylogenetic structure and diversity with trait space patterns to understand community assembly and organization. For example, overdispersion in signal space coupled with phylogenetic clustering is a pattern consistent with signals of close relatives within a community diverging, potentially to minimize competitive overlap. Conversely, phylogenetic overdispersion coupled with clustering in signal space is consistent with the hypothesis of community convergence. Measures of community phylogenetic dissimilarity and beta-diversity (Ives and Helmus, 2010) may also be used to quantify species turnover in acoustic communities. Although care should be applied when interpreting patterns in community phylogenetics (Gerhold et al., 2015), these analyses provide a preliminary understanding of the relationship between phylogenetic structure and signal space (Lahiri et al., 2021). Additionally, by comparing phylogenetic community structure to different trait spaces, we may investigate the contribution of indirect effects (such as morphological divergence) to acoustic community structure (Krishnan and Tamma, 2016). These indirect effects may be important, particularly in light of the relationship between morphological characters and acoustic traits. Phylogenetic analysis thus opens up important avenues in community bioacoustics, and when combined with trait data, provides insight into the organization and turnover of acoustic communities.
Spatial and Temporal Dimensions of the Acoustic Community
Divergent signal parameters represent only one aspect of acoustic signal partitioning. In addition, many organisms also signal at different times (temporal partitioning, distinct from the temporal properties of the notes themselves, which are used to calculate signal space) or locations in space (spatial partitioning). Temporal partitioning may involve fine-scale adjustments to signal timing and repetition rate, resulting in interdigitation of the signals of different individuals [birds: (Cody and Brown, 1969; Ficken et al., 1974; Fleischer et al., 1985; Popp et al., 1985; Brumm, 2006)]. Alternatively, both terrestrial and aquatic species may simply signal at different times of the day from each other, or even in different seasons [birds: (Luther, 2008; Krishnan, 2019a), bats: (Adams and Thibault, 2006; Mancina et al., 2012), fish: (Ruppé et al., 2015; Bertucci et al., 2020), marine mammals: (De Vreese et al., 2018)]. Further research is required to understand if diel-scale temporal partitioning results indirectly from ecological separation in foraging time, or by adjustments to the presence of competing signalers. Spatial partitioning, on the other hand, involves divergence in the locations from which the signal is emitted (Hodl, 1977; Bee, 2008). Segregation in space or stratification of singing heights have been documented in diverse taxa [insects: (Diwakar and Balakrishnan, 2007; Jain and Balakrishnan, 2012), birds: (Nemeth et al., 2002; Chitnis et al., 2020), bats: (Kalko and Handley, 2001; Kennedy et al., 2014)]. This pattern is putatively linked to maximizing sound transmission at a particular location (Miller and Degn, 1981; Dabelsteen et al., 1993; Nemeth et al., 2002; Marler and Slabbekoorn, 2004; Mathevon et al., 2005; Barker and Mennill, 2009; Kirschel et al., 2009a; Sprau et al., 2012; Núñez et al., 2019), or to indirect partitioning as a result of other ecological processes (Jain and Balakrishnan, 2012; Kennedy et al., 2014; Chitnis et al., 2020). For example, competition for resources may also drive signal partitioning as a by-product (Aldridge and Rautenbach, 1987; Norberg and Rayner, 1987; Kingston et al., 2000; Kingston and Rossiter, 2004; Siemers and Schnitzler, 2004; Kirschel et al., 2009b; Mancina et al., 2012; Krishnan and Tamma, 2016; Roemer et al., 2019). On the other hand, acoustic adaptation is hypothesized to lead to convergence, where species with similar signals occupy similar regions in physical space to maximize sound transmission (Boncoraglio and Saino, 2007). Patterns such as these highlight how distinct ecological processes may both directly and indirectly (Wilkins et al., 2013) drive signal partitioning, and thus acoustic community structure, along multiple axes. These axes range from signal parameters (and thus distributions of species in signal space) to the spatial and temporal distribution of signaling species.
Finally, the radiation and propagation patterns of emitted sound are an oft-ignored aspect of signal partitioning. Radiation patterns are shaped dynamically by the morphology of the sender aperture (Feng et al., 2012; Kounitsky et al., 2015), and emitted sounds are directed by the aim of the head (Ghose and Moss, 2003). Directionality of the signal impacts the perceived amplitude (Naguib, 1995), and aiming competing signals away from each other may further reduce interference, enabling signalers to avoid overlap in their active spaces. Signal amplitude is highly consequential for signal perception and attention (Dantzker et al., 1999; Greenfield and Rand, 2000; Brumm and Todt, 2003; Patricelli et al., 2007; Yorzinski and Patricelli, 2010), and for targeting biosonar in bats (Surlykke et al., 2012; Jakobsen et al., 2013). However, relatively few studies have quantified signal directionality and aiming in the field. This is in part because one requires an array of calibrated microphones, as well as estimates of distance from the source, head aim, horizontal and vertical angles to measure sound directionality accurately in the field (Blumstein et al., 2011). By quantifying signal directionality, the principles of engineering acoustics are directly applied in ecological studies to, for example, understand whether behavioral changes (such as aiming of signals in different directions) reduce the overlap of competing signals in certain environments. In addition, the use of multiple microphones to study these patterns may also prove useful in estimating the relative numbers of different signaling species using triangulation (Blumstein et al., 2011; Mennill et al., 2012). Although sound propagation patterns may differ in aquatic environments, similar general principles and techniques may be used to quantify both signal partitioning (Ruppé et al., 2015; Bertucci et al., 2020) and acoustic community structure (Hannay et al., 2013; De Vreese et al., 2018; Desiderà et al., 2019; Mooney et al., 2020). Using community structure and composition, including the distributions of species in signal space, we may obtain valuable ecological and conservation insight from non-invasive acoustic data, and we explore these possibilities in the sections below.
Acoustic Community Structure as a Barometer of Ecological Change
The patterns and processes discussed above imply that each acoustic community is likely to possess a distinct structure, driven both by the diversity of signals (and their distributions in signal space) as well as the spatial and temporal distributions of signaling species. Because communities are not static, ecological changes (spatial and temporal) are likely to be reflected in acoustic community structure, and we may thus use this framework to quantify and detect these changes. For example, seasonal changes such as hibernation or migration may alter the composition and phylogenetic diversity of both terrestrial and marine acoustic communities (Hannay et al., 2013; Putland et al., 2017; De Vreese et al., 2018; Krishnan, 2019a). Additionally, acoustic communities may exhibit small and large-scale spatial heterogeneity, because habitat preferences of signaling species drive differences in distribution (Van Parijs et al., 2001; Figure 3). Quantifying signal space helps us understand seasonal dynamics and habitat occupancy of acoustic communities, providing real-time information on species distributions and movements. This data potentially enables us to detect arrivals and departures both along migration routes and wintering grounds by measuring turnover in the acoustic community. Further studies could then use multi-year acoustic data to quantify the effects of extensive urbanization or shipping traffic on the migration and community structure of birds, bats and marine mammals. Human impacts are predicted to profoundly affect migrant numbers and timing of arrival, and community bioacoustics provides an early warning of these changes for terrestrial and aquatic ecosystems (Sanders and Mennill, 2014). Studies have quantified phenology (Buxton et al., 2016), or seasonal ecological patterns in bird communities over large geographical areas. Many studies of this variety utilize ecoacoustic or soundscape indices to quantify broad changes in sound profiles (Villanueva-Rivera et al., 2011; Sueur and Farina, 2015). Indices typically either quantify attributes of a recording, or compare recordings to each other in a manner similar to quantifying beta-diversity (Villanueva-Rivera et al., 2011; Desjonquères et al., 2020). Although useful for rapid assessments, they do not provide species-level information, and may sometimes provide conflicting results with census methodologies (Lellouch et al., 2014; Krishnan, 2019a). Thus, studies operating at multiple scales should combine the use of ecoacoustic indices with more detailed analyses of the kind we propose here. The quantification and utility of soundscape indices has been covered in multiple reviews and publications (Farina et al., 2011; Pijanowski et al., 2011a, b; Sueur and Farina, 2015; Gasc et al., 2017; Buxton et al., 2018a, b; Gibb et al., 2019), and the two approaches are compared and contrasted in Table 2. Thus, although our review focuses more on the use of acoustics to study community ecology and change, using the acoustic community as a unit, a combination of different approaches may prove fruitful for future studies.
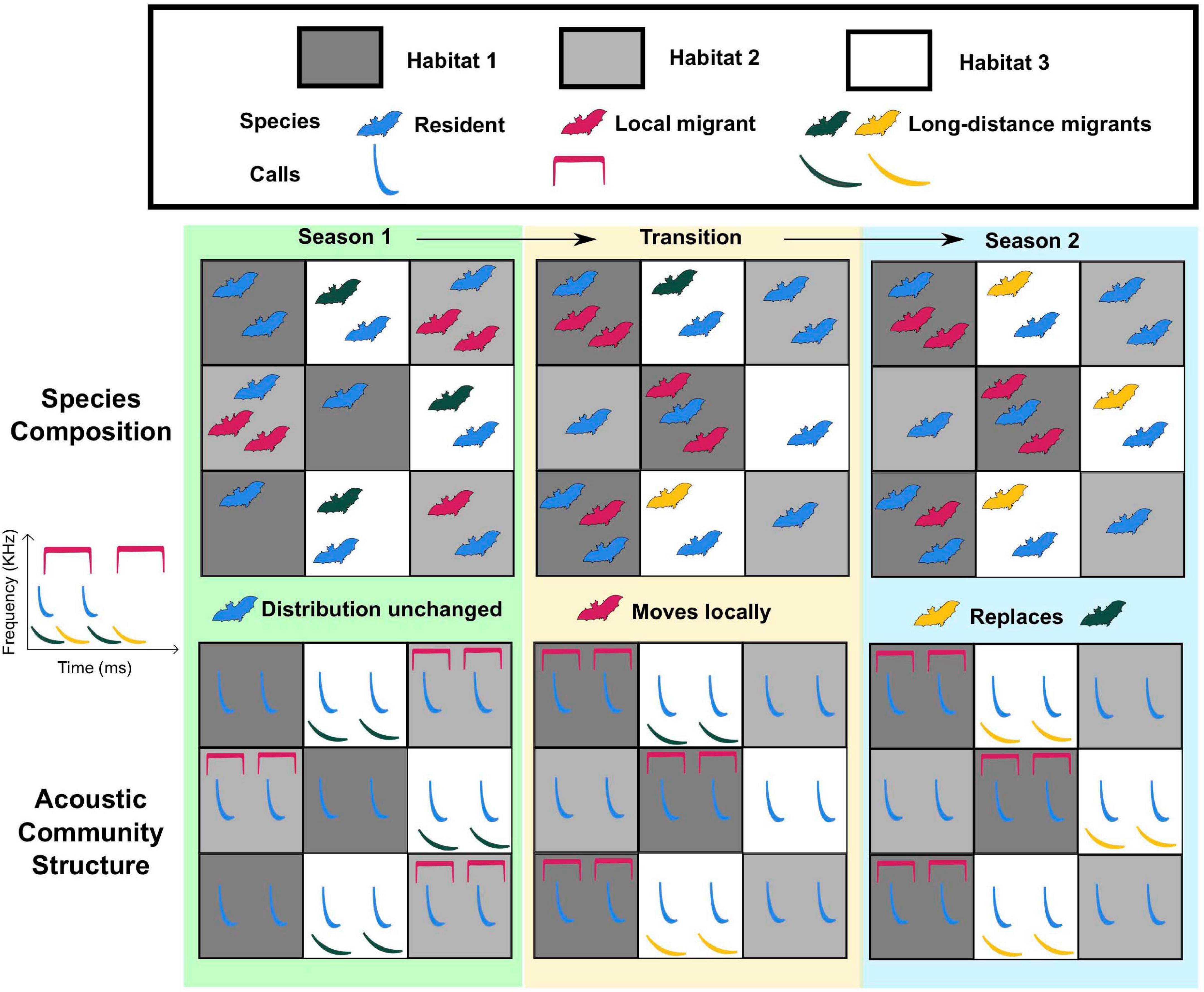
Figure 3. Ecological changes are reflected in the acoustic community. Spatiotemporal change in a hypothetical bat acoustic community (consisting of resident and migrant species). Each habitat within this landscape is represented by a square, with colors representing different habitat types. Each bat species calls at a distinct frequency (see key on the left), and species composition in each cell changes with seasonal transitions (top row). Recorders placed in each square are predicted to detect spatiotemporal changes in acoustic community structure (bottom row), serving as a framework for long-term monitoring. Similar changes may also occur due to hibernation or seasonal differences in signaling activity.
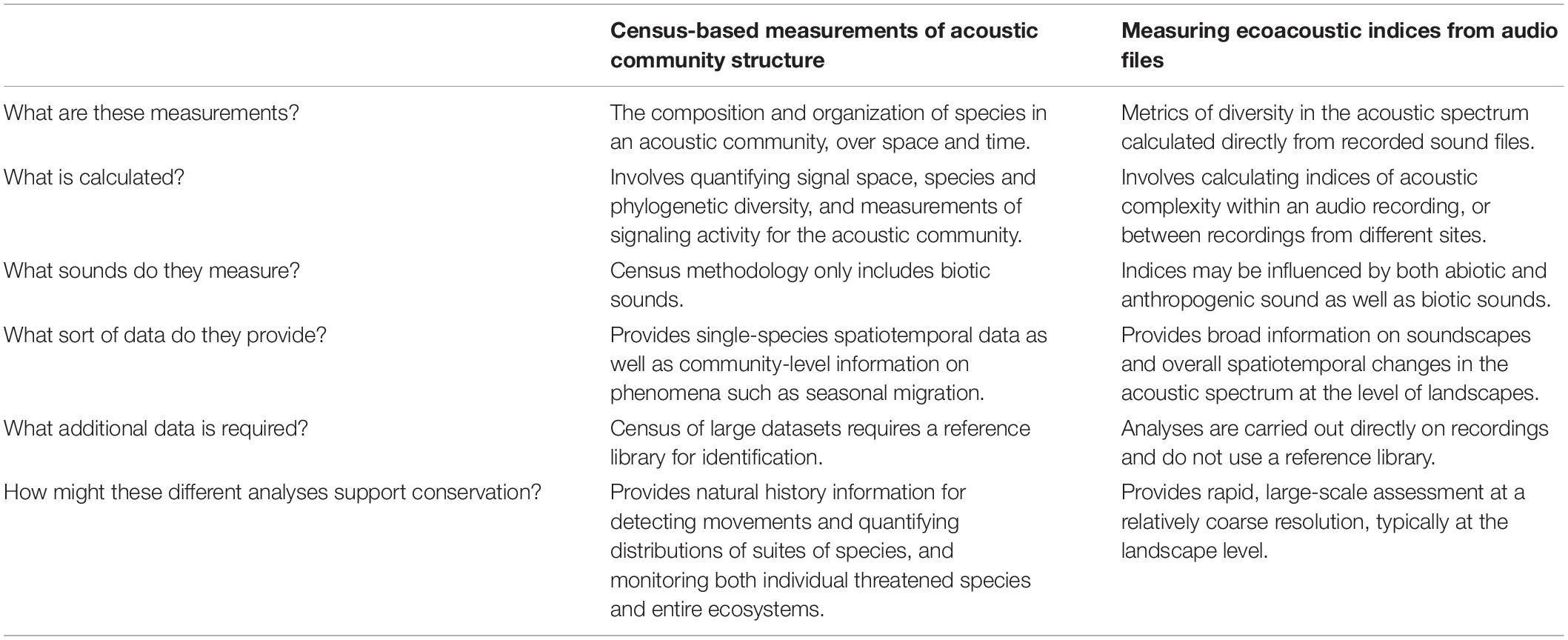
Table 2. Comparison of the information provided by quantifying acoustic community structure using census methodology versus quantifying acoustic diversity using ecoacoustic indices.
For instance, by combining indices with census of changes in species composition, together with change in the community signal space, we can detect the effects of migration on community structure (Krishnan, 2019a). The strength of community bioacoustics lies in providing detailed single-species information as well as data on overall community diversity (Table 2). Gathering these data across seasons or multiple years provides a non-invasive way to track temporal community change. In addition to migration, many bat species enter hibernation in inclement weather, resulting in their absence from the acoustic community. Hibernating bats are very sensitive to environmental changes (Fenton, 2012; Nocera et al., 2019). Long-term community acoustic data potentially provides information on whether individual species are declining, on changes to hibernation timings, and changes in overall community diversity over time.
Heterogeneity in habitat and species distributions across a landscape is also putatively reflected in a corresponding heterogeneity of acoustic communities (Luther, 2009). Acoustic monitoring has proved very useful in understanding occupancy of species which are hard to detect using traditional survey methods (Weller and Baldwin, 2012; Kalan et al., 2015; Campos-Cerqueira and Aide, 2016; Law et al., 2018; Gibb et al., 2019; Abrahams and Geary, 2020), and this provides a starting point to quantify spatial structure in the acoustic community. Occupancy, or the proportion of sites occupied by a species of interest, provides a quantitative estimate of the distribution and spread of a species. Dynamic multispecies occupancy models enable quantitative description of spatial heterogeneity in the acoustic community, as well as detection of the effects of environmental change on the relative distributions of species (Dorazio et al., 2010; Iknayan and Beissinger, 2018). There is a need for more large-scale multispecies occupancy studies using acoustics, in order to comprehensively quantify spatial community structure. This, together with metrics of community beta-diversity (Avolio et al., 2019), enables site-by-site spatial diversity comparisons. We may then test two contrasting hypotheses: does signal similarity drive spatial segregation in species distributions, or does habitat structure indirectly structure the acoustic community (Sugai et al., 2021a)? Similar analyses have also proved useful in understanding how isolation on islands influences the acoustic community, and thus signal partitioning in birds (Robert et al., 2019). This study framework therefore has great value in understanding the relationship between biogeography and acoustic community structure. Combining data from multiple recorders with triangulation algorithms will enable estimates of density and relative numbers of each signaling species in the community, and even open up the possibility of tracking local movements of taxa such as marine mammals (Blumstein et al., 2011).
Current Limitations in Community Bioacoustics, and Possible Solutions
In spite of the usefulness of community bioacoustics, certain limitations must be considered as areas for future streamlining and improvement. Firstly, scientific studies undertaking biodiversity inventories including passive acoustics should be mindful of taxa that are silent or infrequently vocal, and use a combination of survey methods to detect all species (Darras et al., 2018; Wheeldon et al., 2019). For example, relatively silent birds such as storks, or fruit bats that do not produce echolocation calls, may be more reliably detected by visual surveys. Thus, the acoustic community is best treated as a surrogate of the ecological community, and as an indicator of its diversity rather than an exact inventory. Secondly, censusing massive acoustic data to quantify community structure can be labor-intensive. Recent advances in automated species recognition provide hope of a streamlined analytical pipeline (Mac Aodha et al., 2018; Ulloa et al., 2018; Stowell et al., 2019). However, in understudied tropical regions, comprehensive call libraries are a relatively recent phenomenon, and there is a global paucity of information on many taxa (Chakravarty et al., 2020; Desjonquères et al., 2020). More data is needed to improve automated recognition, particularly in high-noise environments where the sounds from multiple vocal species may result in masking that interferes with many recognition algorithms. In the meantime, researchers in these regions should consider randomized subsampling of large datasets, (Krishnan, 2019a) which may provide a more tractable method to census the acoustic community. This approach will also reduce the computational power required.
Another issue generally faced by passive acoustic studies is correcting for detectability in estimates of occupancy and community composition (Darras et al., 2016). This is particularly relevant for bats and dolphins, whose ultrasonic signals attenuate quickly over distance in a frequency-dependent manner. Low signal-to-noise ratios further accentuate this problem, reducing detectability of calls (Sugai et al., 2019a). Thus, false absences of higher-frequency species must be accounted for in any study, potentially by correcting the data to account for differences in detectability (Meyer et al., 2011) or by matching visual and acoustic survey data (Richman et al., 2014). Playback experiments using speakers may provide a detection space for each species in an environment, which can then be implemented in an occupancy-based model. Combining this method with triangulation, as described earlier, may help refine estimates and even provide relative abundance data for each species in a community (Mennill et al., 2012). This is an important area of future research into expanding the possibilities of acoustic monitoring.
Synthesis: Community Bioacoustics in Long-Term Biodiversity Monitoring and Conservation
As the Anthropocene brings with it rapid changes in the earth’s biodiversity, long-term biodiversity monitoring is the need of the hour to inform policy and conservation (Magurran et al., 2010). Non-invasive monitoring methods, in particular, are powerful sources of continuous ecological data. For this reason, passive acoustic monitoring has increased in popularity both in marine and terrestrial environments. Human activities such as land-use change could fundamentally alter spatiotemporal acoustic community structure (Zuk et al., 2006; Gasc et al., 2018; Torrent et al., 2018; Pillay et al., 2019), at the extreme resulting in the loss of natural sounds (Pijanowski et al., 2011a; Sueur et al., 2019). Quantifying these effects is very important in developing conservation policy for diverse ecosystems, from forests and deserts to the ocean depths. Studying how anthropogenic change influences spatiotemporal community composition could inform conservation planning and management, by serving both as an early warning system and as a barometer of the extent of ecological change.
As recorders become increasingly cost-effective (Hill et al., 2019), the potential for acoustic monitoring, particularly in tropical countries that harbor most of the world’s biodiversity, is tremendous (Wrege et al., 2017). With these developments comes a need for capacity-building not just in the collection of data, but also in building robust analytical frameworks that support science and conservation planning. As a highly biodiverse tropical country, the example of India serves to illustrate the global promise of community bioacoustics, and the need for more studies utilizing these methods. There are still relatively few passive acoustic studies in India (Diwakar et al., 2007; Buxton et al., 2018a; Krishnan, 2019a; Lahiri et al., 2021), and most acoustic sampling relies on call counts or focal recordings (Khaling et al., 1998; Ghose et al., 2003; Agnihotri et al., 2014; Purushotham and Robin, 2016). For lesser-known Indian taxa such as bats, comprehensive call libraries are few (Raghuram et al., 2014; Wordley et al., 2018; Chakravarty et al., 2020). Expanding the study of acoustic community structure will thus be extremely valuable in understanding and conserving global biodiversity. The ability to rapidly collect large amounts of data in the absence of observers sets passive acoustics apart from focal community sampling (Haselmayer and Quinn, 2000; Abrahams, 2019). Even when deploying passive recorders for single-species surveys, a wealth of community-level information may be obtained. Most studies we have illustrated in this review, however, focus largely either on single species, or use indices for broader comparisons. By studying acoustic community dynamics, deriving a community signal space based on acoustic parameters, and quantifying the diversity of communities in different habitats, we can build a comprehensive tapestry of signaling species across landscapes. This enables us to obtain information both on individual species, as well as entire communities, using the same datasets as other passive acoustic studies. For example, by recording the acoustic community, we may identify the habitat preferences of each individual species (as detailed above), compare different sites to measure differences in community composition and diversity across different habitat types, and also track change in the overall community (and individual species) over time. Therefore, if anthropogenic activity alters the distribution of a certain species, this will be detected by passive recorders, and will also change community composition over time. By measuring the effect of anthropogenic changes on community turnover (for example, beta-diversity as a response variable), we can obtain valuable ecological information across scales.
For threatened species, acoustic community structure, diversity and dynamics at known sites is a useful barometer of ecosystem health. Studies using acoustics to date primarily focus on presence-absence of specific species, or on occupancy patterns, as detailed earlier. However, community-level data provides considerably more in the way of potential opportunities for conservation insight. For example, we can identify what species typically co-occur with a certain threatened species in the acoustic community, and the patterns of community structure in its preferred habitat. This could potentially be used to identify new priority sites for survey, reintroduction or conservation of these species, and to evaluate the success of reintroduction and restoration efforts (Lewis et al., 2021). Sites that possess the requisite suite of co-occurring species may be suitable for reintroduction of a threatened species, and community structure may be monitored after reintroduction, to measure whether it returns to a pattern similar to the source site. Such efforts are particularly relevant in insular ecosystems, where eradication of invasive species and translocation of native species is being undertaken to restore ecological communities (Borker et al., 2020). Acoustic community structure could provide a well-defined, easily quantified metric of the success of these efforts in restoring native insular communities of birds, frogs, insects, and other signaling animals.
Community bioacoustics will further enable quantification of long-term change in global ecosystems. This, in turn, will help understand how human-induced environmental changes impact global biodiversity, from densely populated cities to the ocean depths. Although approaches driven by indices are gaining popularity in passive acoustic studies, there is also great benefit in approaches that provide species-level, natural history-driven information that is of direct use in informing conservation policy. Community bioacoustics, and specifically acoustic community structure, is an approach that provides this information, from the entire community down to individual species (Krishnan, 2019a; Pillay et al., 2019). By quantifying the distributions and volume occupied by species in community signal space, together with phylogenetic diversity (Lahiri et al., 2021; Figure 4), field researchers may compare them across environmental conditions or grades of anthropogenic impact. For instance, we can compare signal spaces across different land-use types, to examine how the distributions of species within signal space change. This enables a non-invasive estimate of how land-use change impacts community diversity, phylogenetic diversity and the composition of communities of sound-producing species. The same data can also be used to track how detections of individual species change across land-use types. These comparisons thus provide critical data on acoustic community structure, which reflects the ecological impacts of anthropogenic change. By broadening the use of community bioacoustics, we can thus apply an interdisciplinary understanding of acoustic signaling to inform effective global biodiversity monitoring and conservation.
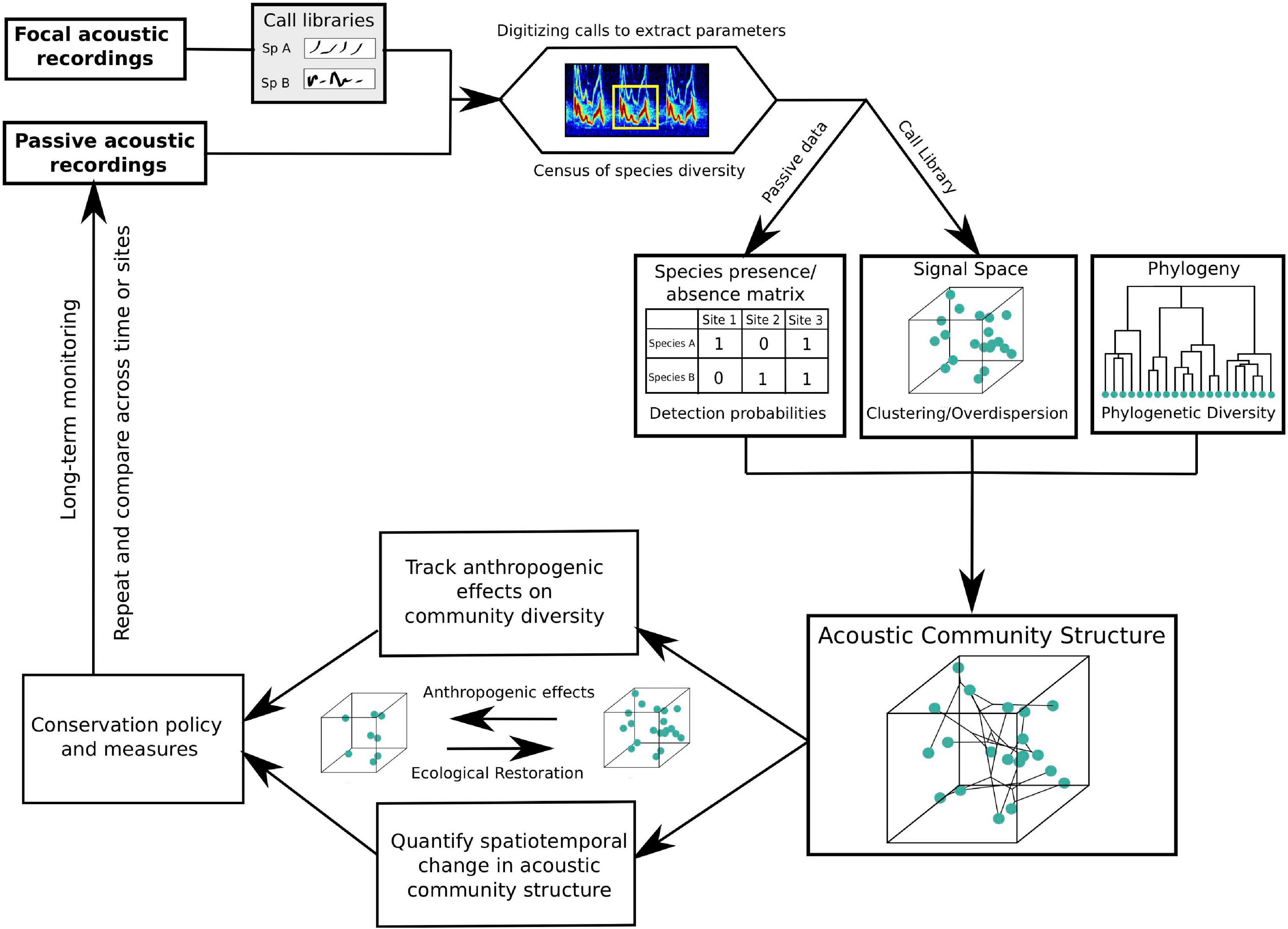
Figure 4. A generalized work flow highlighting the utility of community bioacoustics in long-term monitoring and conservation. By combining a quantification of community signal space with phylogenetic analyses and passive acoustic census of spatiotemporal community diversity, we may track ecological changes, inform conservation measures and also gauge their success over time.
Author Contributions
VC, SL, MJ, and AK contributed to figure conception and design. All authors listed made substantial, direct and intellectual contributions to the text, and approved this manuscript for publication.
Funding
AK is funded by an INSPIRE Faculty Award from the Department of Science and Technology and an Early Career Research Award (ECR/2017/001527) from the Science and Engineering Research Board (SERB), Government of India, and is a recipient of a Wildlife Acoustics Scientific Product Grant. SL is a recipient of an Oriental Bird Club Conservation Grant and equipment support from Idea Wild. RM is a recipient of a Rufford Foundation Small Grant. VC and MJ are both recipients of KVPY Fellowships from the Government of India.
Conflict of Interest
The authors declare that the research was conducted in the absence of any commercial or financial relationships that could be construed as a potential conflict of interest.
Publisher’s Note
All claims expressed in this article are solely those of the authors and do not necessarily represent those of their affiliated organizations, or those of the publisher, the editors and the reviewers. Any product that may be evaluated in this article, or claim that may be made by its manufacturer, is not guaranteed or endorsed by the publisher.
Acknowledgments
We thank Shivam Chitnis and Rohit Chakravarty for comments, Ritesh Dighe and Vivek Kannadi for photographic references, and the reviewers for constructive feedback.
References
Abrahams, C. (2019). Comparison between lek counts and bioacoustic recording for monitoring Western Capercaillie (Tetrao urogallus L.). J. Ornithol. 160, 685–697. doi: 10.1007/s10336-019-01649-8
Abrahams, C., and Geary, M. (2020). Combining bioacoustics and occupancy modelling for improved monitoring of rare breeding bird populations. Ecol. Indic. 112:106131. doi: 10.1016/j.ecolind.2020.106131
Adams, R. A., and Thibault, K. M. (2006). Temporal resource partitioning by bats at water holes. J. Zool. 270, 466–472. doi: 10.1111/j.1469-7998.2006.00152.x
Agnihotri, S., Sundeep, P. V. D. S., Seelamantula, C. S., and Balakrishnan, R. (2014). Quantifying vocal mimicry in the greater racket-tailed drongo: A comparison of automated methods and human assessment. PLoS One 9:e0089540. doi: 10.1371/journal.pone.0089540
Aldridge, H. D. J., and Rautenbach, I. L. (1987). Morphology, echolocation and resource partitioning in insectivorous bats. J. Anim. Ecol. 56, 763–778. doi: 10.1644/11-MAMM-A-331.1
Amezquita, A., Flechas, S. V., Lima, A. P., Gasser, H., and Hodl, W. (2011). Acoustic interference and recognition space within a complex assemblage of dendrobatid frogs. Proc. Natl. Acad. Sci. 108, 17058–17063. doi: 10.1073/pnas.1104773108
Aubin, T., and Jouventin, P. (1998). Cocktail-party effect in king penguin colonies. Proc. R. Soc. B Biol. Sci. 265, 1665–1673.
Avolio, M. L., Carroll, I. T., Collins, S. L., Houseman, G. R., Hallett, L. M., Isbell, F., et al. (2019). A comprehensive approach to analyzing community dynamics using rank abundance curves. Ecosphere 10:e02881. doi: 10.1002/ecs2.2881
Balakrishnan, R., Bahuleyan, J., Nandi, D., and Jain, M. (2014). Modelling the effects of chorus species composition and caller density on acoustic masking interference in multispecies choruses of crickets and katydids. Ecol. Inform. 21, 50–58. doi: 10.1016/j.ecoinf.2013.11.006
Barker, N. K. S., and Mennill, D. J. (2009). Song perch height in rufous-and-white wrens: Does behaviour enhance effective communication in a tropical forest? Ethology 115, 897–904. doi: 10.1111/j.1439-0310.2009.01674.x
Bee, M. A. (2008). Finding a mate at a cocktail party: spatial release from masking improves acoustic mate recognition in grey treefrogs. Anim. Behav. 75, 1781–1791. doi: 10.1016/j.anbehav.2007.10.032
Bee, M. A., and Micheyl, C. (2008). The “Cocktail Party Problem”: What is it? How can it be solved? And why should animal behaviorists study it? J. Comp. Psychol. 122, 235–251.
Bertucci, F., Maratrat, K., Berthe, C., Besson, M., Guerra, A. S., Raick, X., et al. (2020). Local sonic activity reveals potential partitioning in a coral reef fish community. Oecologia 193, 125–134. doi: 10.1007/s00442-020-04647-3
Blumenrath, S. H., and Dabelsteen, T. (2004). Degradation of great tit (Parus major) song before and after foliation: Implications for vocal communication in a deciduous forest. Behaviour 141, 935–958. doi: 10.1163/1568539042360152
Blumstein, D. T., Mennill, D. J., Clemins, P., Girod, L., Yao, K., Patricelli, G., et al. (2011). Acoustic monitoring in terrestrial environments using microphone arrays: Applications, technological considerations and prospectus. J. Appl. Ecol. 48, 758–767. doi: 10.1111/j.1365-2664.2011.01993.x
Boncoraglio, G., and Saino, N. (2007). Habitat structure and the evolution of bird song: A meta-analysis of the evidence for the acoustic adaptation hypothesis. Funct. Ecol. 21, 134–142. doi: 10.1111/j.1365-2435.2006.01207.x
Borker, A. L., Buxton, R. T., Jones, I. L., Major, H. L., Williams, J. C., Tershy, B. R., et al. (2020). Do soundscape indices predict landscape-scale restoration outcomes? A comparative study of restored seabird island soundscapes. Restor. Ecol. 28, 252–260. doi: 10.1111/rec.13038
Bradbury, J. W., and Vehrencamp, S. L. (2011). Principles of Animal Communication, 2nd Edn. Sunderland, MA: Sinauer Associates.
Braune, P., Schmidt, S., and Zimmermann, E. (2008). Acoustic divergence in the communication of cryptic species of nocturnal primates (Microcebus ssp.). BMC Biol. 6:19. doi: 10.1186/1741-7007-6-19
Brenowitz, E. A. (1982). The active space of red-winged blackbird song. J. Comp. Physiol. 147, 511–522. doi: 10.1007/BF00612017
Brumm, H. (2006). Signalling through acoustic windows: Nightingales avoid interspecific competition by short-term adjustment of song timing. J. Comp. Physiol. A Neuroethol. Sens. Neural. Behav. Physiol. 192, 1279–1285. doi: 10.1007/s00359-006-0158-x
Brumm, H., and Naguib, M. (2009). Environmental acoustics and the evolution of bird song. Adv. Study Behav. 40, 1–33. doi: 10.1016/S0065-3454(09)40001-9
Brumm, H., and Slabbekoorn, H. (2005). Acoustic communication in noise. Adv. Study Behav. 35, 151–209. doi: 10.1016/S0065-3454(05)35004-2
Brumm, H., and Todt, D. (2003). Facing the rival: directional singing behaviour in nightingales. Behaviour 140, 43–53.
Buxton, R. T., Agnihotri, S., Robin, V. V., Goel, A., and Balakrishnan, R. (2018a). Acoustic indices as rapid indicators of avian diversity in different land-use types in an Indian biodiversity hotspot. J. Ecoacoustics 2, 1–17. doi: 10.22261/JEA.GWPZVD
Buxton, R. T., McKenna, M. F., Clapp, M., Meyer, E., Stabenau, E., Angeloni, L. M., et al. (2018b). Efficacy of extracting indices from large-scale acoustic recordings to monitor biodiversity. Conserv. Biol. 32, 1174–1184. doi: 10.1111/cobi.13119
Buxton, R. T., Brown, E., Sharman, L., Gabriele, C. M., and McKenna, M. F. (2016). Using bioacoustics to examine shifts in songbird phenology. Ecol. Evol. 6, 4697–4710. doi: 10.1002/ece3.2242
Campos-Cerqueira, M., and Aide, T. M. (2016). Improving distribution data of threatened species by combining acoustic monitoring and occupancy modelling. Methods Ecol. Evol. 7, 1340–1348. doi: 10.1111/2041-210X.12599
Cardoso, G. C., and Price, T. D. (2010). Community convergence in bird song. Evol. Ecol. 24, 447–461. doi: 10.1007/s10682-009-9317-1
Cavender-Bares, J., Ackerly, D. D., Baum, D. A., and Bazzaz, F. A. (2004). Phylogenetic overdispersion in Floridian oak communities. Am. Nat. 163, 823–843. doi: 10.1086/386375
Chakravarty, R., Ruedi, M., and Ishtiaq, F. (2020). A recent survey of bats with descriptions of echolocation calls and new records from the Western Himalayan Region of Uttarakhand, India. Acta Chiropterologica 22:197. doi: 10.3161/15081109acc2020.22.1.019
Chek, A. A., Bogart, J. P., and Lougheed, S. C. (2003). Mating signal partitioning in multi-species assemblages: A null model test using frogs. Ecol. Lett. 6, 235–247. doi: 10.1046/j.1461-0248.2003.00420.x
Chitnis, S. S., Rajan, S., and Krishnan, A. (2020). Sympatric wren-warblers partition acoustic signal space and song perch height. Behav. Ecol. 31, 559–567. doi: 10.1093/beheco/arz216
Cody, M. L., and Brown, J. H. (1969). Song asynchrony in neighbouring bird species. Nature 222, 778–780.
Dabelsteen, T., Larsen, O. N., and Pedersen, S. B. (1993). Habitat-induced degradation of sound signals: Quantifying the effects of communication sounds and bird location on blur ratio, excess attenuation, and signal-to-noise ratio in blackbird song. J. Acoust. Soc. Am. 93, 2206–2220. doi: 10.1121/1.406682
Dantzker, M. S., Deane, G. B., and Bradbury, J. W. (1999). Directional acoustic radiation in the strut display of male sage grouse Centrocercus urophasianus. J. Exp. Biol. 202, 2893–2909.
Darras, K., Batáry, P., Furnas, B., Celis-Murillo, A., Van Wilgenburg, S. L., Mulyani, Y. A., et al. (2018). Comparing the sampling performance of sound recorders versus point counts in bird surveys: A meta-analysis. J. Appl. Ecol. 55, 2575–2586. doi: 10.1111/1365-2664.13229
Darras, K., Pütz, P., Fahrurrozi, Rembold, K., and Tscharntke, T. (2016). Measuring sound detection spaces for acoustic animal sampling and monitoring. Biol. Conserv. 201, 29–37. doi: 10.1016/j.biocon.2016.06.021
De Vreese, S., van der Schaar, M., Weissenberger, J., Erbs, F., Kosecka, M., Solé, M., et al. (2018). Marine mammal acoustic detections in the Greenland and Barents Sea, 2013 – 2014 seasons. Sci. Rep. 8:16882. doi: 10.1038/s41598-018-34624-z
Desiderà, E., Guidetti, P., Panzalis, P., Navone, A., Valentini-Poirrier, C. A., Boissery, P., et al. (2019). Acoustic fish communities: Sound diversity of rocky habitats reflects fish species diversity. Mar. Ecol. Prog. Ser. 608, 183–197. doi: 10.3354/meps12812
Desjonquères, C., Gifford, T., and Linke, S. (2020). Passive acoustic monitoring as a potential tool to survey animal and ecosystem processes in freshwater environments. Freshw. Biol. 65, 7–19. doi: 10.1111/fwb.13356
Desjonquères, C., Rybak, F., Castella, E., Llusia, D., and Sueur, J. (2018). Acoustic communities reflects lateral hydrological connectivity in riverine floodplain similarly to macroinvertebrate communities. Sci. Rep. 8, 1–11. doi: 10.1038/s41598-018-31798-4
Diwakar, S., and Balakrishnan, R. (2007). Vertical stratification in an acoustically communicating ensiferan assemblage of a tropical evergreen forest in southern India. J. Trop. Ecol. 23:479. doi: 10.1017/S0266467407004208
Diwakar, S., Jain, M., and Balakrishnan, R. (2007). Psychoacoustic sampling as a reliable, non-invasive method to monitor orthopteran species diversity in tropical forests. Biodivers. Conserv. 16, 4081–4093. doi: 10.1007/s10531-007-9208-0
Dorazio, R. M., Kéry, M., Royle, J. A., and Plattner, M. (2010). Models for inference in dynamic metacommunity systems. Ecology 91, 2466–2475. doi: 10.1890/09-1033.1
Drewry, G. E., and Rand, A. S. (1983). Characteristics of an acoustic community: puerto rican frogs of the genus Eleutherodactylus. Copeia 4, 941–953.
Duellman, W. E., and Pyles, R. A. (1983). Acoustic resource partitioning in anuran communities. Copeia 1983, 639–649. doi: 10.2307/1444328
Endler, J. A. (1992). Signals, signal conditions, and the direction of evolution. Am. Nat. 139, S125–S153.
Ey, E., and Fischer, J. (2009). The “acoustic adaptation hypothesis”—a review of the evidence from birds, anurans and mammals. Bioacoustics 19, 21–48. doi: 10.1080/09524622.2009.9753613
Farina, A., and James, P. (2016). The acoustic communities: Definition, description and ecological role. Biosystems 147, 11–20.
Farina, A., Lattanzi, E., Malavasi, R., Pieretti, N., and Piccioli, L. (2011). Avian soundscapes and cognitive landscapes: Theory, application and ecological perspectives. Landsc. Ecol. 26, 1257–1267. doi: 10.1007/s10980-011-9617-z
Feng, L., Gao, L., Lu, H., and Muller, R. (2012). Noseleaf dynamics during pulse emission in horseshoe bats. PLoS One 7:e34685. doi: 10.1371/journal.pone.0034685
Fenton, M. B. (2012). Bats and white-nose syndrome. Proc. Natl. Acad. Sci. U. S. A. 109, 6794–6795. doi: 10.1073/pnas.1204793109
Ficken, R. W., Ficken, M. S., and Hailman, J. P. (1974). Temporal pattern shifts to avoid acoustic interference in singing birds. Science 183, 762–763.
Fleischer, R. C., Boarman, W. I., and Cody, M. L. (1985). Asynchrony of song series in the Bewick’s wren and wrentit. Anim. Behav. 33, 674–676. doi: 10.1016/S0003-3472(85)80095-6
Gasc, A., Anso, J., Sueur, J., Jourdan, H., and Desutter-Grandcolas, L. (2018). Cricket calling communities as an indicator of the invasive ant Wasmannia auropunctata in an insular biodiversity hotspot. Biol. Invasions 20, 1099–1111. doi: 10.1007/s10530-017-1612-0
Gasc, A., Francomano, D., Dunning, J. B., and Pijanowski, B. C. (2017). Future directions for soundscape ecology: The importance of ornithological contributions. Auk 134, 215–228. doi: 10.1642/AUK-16-124.1
Gerhold, P., Cahill, J. F., Winter, M., Bartish, I. V., and Prinzing, A. (2015). Phylogenetic patterns are not proxies of community assembly mechanisms (they are far better). Funct. Ecol. 29, 600–614. doi: 10.1111/1365-2435.12425
Ghose, D., Kaul, R., and Saha, G. K. (2003). Status survey of the Blyth’s tragopan in Blue Mountain National Park, Mizoram, India using call-count technique. Curr. Sci. 84, 95–97.
Ghose, K., and Moss, C. F. (2003). The sonar beam pattern of a flying bat as it tracks tethered insects. J. Acoust. Soc. Am. 114, 1120–1131.
Gibb, R., Browning, E., Glover-Kapfer, P., and Jones, K. E. (2019). Emerging opportunities and challenges for passive acoustics in ecological assessment and monitoring. Methods Ecol. Evol. 10, 169–185. doi: 10.1111/2041-210X.13101
Gotelli, N., and Entsminger, G. (2001). Swap and fill algorithms in null model analysis: rethinking the knight’s tour. Oecologia 129, 281–291. doi: 10.1007/s004420100717
Gotelli, N. J. (2000). Null model analysis of species co-occurrence patterns. Ecology 81, 2606–2621.
Gotelli, N. J., and McCabe, D. J. (2002). Species co-occurrence: A meta-analysis of J. M. Diamond’s assembly rules model. Ecology 83, 2091–2096.
Gottesman, B. L., Francomano, D., Zhao, Z., Bellisario, K., Ghadiri, M., Broadhead, T., et al. (2020). Acoustic monitoring reveals diversity and surprising dynamics in tropical freshwater soundscapes. Freshw. Biol. 65, 117–132. doi: 10.1111/fwb.13096
Grant, B. R., and Grant, P. R. (1996). Cultural inheritance of song and its role in the evolution of Darwin’s Finches. Evolution (N. Y). 50:2471. doi: 10.2307/2410714
Greenfield, M. D. (1994). Synchronous and alternating choruses in insects and anurans: Common mechanisms and diverse functions. Integr. Comp. Biol. 34, 605–615. doi: 10.1093/icb/34.6.605
Greenfield, M. D., and Rand, A. S. (2000). Frogs have rules: Selective attention algorithms regulate chorusing in Physalaemus pustulosus (Leptodactylidae). Ethology 106, 331–347. doi: 10.1046/j.1439-0310.2000.00525.x
Griffin, D. (1958). Listening in the Dark: the Acoustic Orientation of Bats and Men. New Haven, CT: Yale University Press.
Hannay, D. E., Delarue, J., Mouy, X., Martin, B. S., Leary, D., Oswald, J. N., et al. (2013). Marine mammal acoustic detections in the northeastern Chukchi Sea, September 2007-July 2011. Cont. Shelf Res. 67, 127–146. doi: 10.1016/j.csr.2013.07.009
Hart, P. J., Hall, R., Ray, W., Beck, A., and Zook, J. (2015). Cicadas impact bird communication in a noisy tropical rainforest. Behav. Ecol. 26, 839–842. doi: 10.1093/beheco/arv018
Harvey, P. H., Colwell, R. K., Silvertown, J. W., and May, R. M. (1983). Null models in ecology. Annu. Rev. Ecol. Syst. 14, 189–211. doi: 10.1146/annurev.es.14.110183.001201
Haselmayer, J., and Quinn, J. S. (2000). A comparison of point counts and sound recording as bird survey methods in Amazonian Southeast Peru. Condor 102, 887–893.
Heller, K.-G., and von Helversen, O. (1989). Resource partitioning of sonar frequency bands in rhinolophoid bats. Oecologia 80, 178–186.
Hill, A. P., Prince, P., Snaddon, J. L., Doncaster, C. P., and Rogers, A. (2019). AudioMoth: A low-cost acoustic device for monitoring biodiversity and the environment. HardwareX 6:e00073. doi: 10.1016/j.ohx.2019.e00073
Hodl, W. (1977). Call differences and calling site segregation in anuran species from Central Amazonian Floating meadows. Oecologia 363, 351–363.
Holt, R. D. (2009). Bringing the Hutchinsonian niche into the 21st century: Ecological and evolutionary perspectives. Proc. Natl. Acad. Sci. U. S. A. 106, 19659–19665. doi: 10.1073/pnas.0905137106
Iknayan, K. J., and Beissinger, S. R. (2018). Collapse of a desert bird community over the past century driven by climate change. Proc. Natl. Acad. Sci. U. S. A. 115, 8597–8602. doi: 10.1073/pnas.1805123115
Ives, A. R., and Helmus, M. R. (2010). Phylogenetic metrics of community similarity. Am. Nat. 176, E128–E142. doi: 10.1086/656486
Jain, M., and Balakrishnan, R. (2012). Does acoustic adaptation drive vertical stratification? A test in a tropical cricket assemblage. Behav. Ecol. 23, 343–354. doi: 10.1093/beheco/arr191
Jain, M., Diwakar, S., Bahuleyan, J., Deb, R., and Balakrishnan, R. (2014). A rain forest dusk chorus: Cacophony or sounds of silence? Evol. Ecol. 28, 1–22. doi: 10.1007/s10682-013-9658-7
Jakobsen, L., Brinkløv, S., and Surlykke, A. (2013). Intensity and directionality of bat echolocation signals. Front. Physiol. 4:89. doi: 10.3389/fphys.2013.00089
Jetz, W., Thomas, G. H., Joy, J. B., Hartmann, K., and Mooers, A. O. (2012). The global diversity of birds in space and time. Nature 491, 444–448. doi: 10.1038/nature11631
Jones, G., and Holderied, M. W. (2007). Bat echolocation calls: Adaptation and convergent evolution. Proc. R. Soc. B Biol. Sci. 274, 905–912. doi: 10.1098/rspb.2006.0200
Kalan, A. K., Mundry, R., Wagner, O. J. J., Heinicke, S., Boesch, C., and Kühl, H. S. (2015). Towards the automated detection and occupancy estimation of primates using passive acoustic monitoring. Ecol. Indic. 54, 217–226. doi: 10.1016/j.ecolind.2015.02.023
Kalko, E. K. V., and Handley, C. O. (2001). Neotropical bats in the canopy: diversity, community structure, and implications for conservation. Plant Ecol. 153, 319–333. doi: 10.1007/978-94-017-3606-0_26
Kembel, S. W., Cowan, P. D., Helmus, M. R., Cornwell, W. K., Morlon, H., Ackerly, D. D., et al. (2010). Picante: R tools for integrating phylogenies and ecology. Bioinformatics 26, 1463–1464.
Kennedy, J.-P., Sillett, S. C., and Szewczak, J. M. (2014). Bat activity across the vertical gradient of an old-growth sequoia sempervirens forest. Acta Chiropterologica 16, 53–63. doi: 10.3161/150811014x683264
Khaling, S., Kaul, R., and Saha, G. K. (1998). Surveys of the Satyr tragopan Tragopan satyra in the Singhalila national park, Darjeeling, India using spring call counts. Bird Conserv. Int. 8, 361–371. doi: 10.1017/S0959270900002124
Kingston, T., Jones, G., Zubaid, A., and Kunz, T. H. (2000). Resource partitioning in rhinolophoid bats revisited. Oecologia 124, 332–342. doi: 10.1007/PL00008866
Kingston, T., and Rossiter, S. J. (2004). Harmonic-hopping in Wallacea’s bats. Nature 429, 9–12. doi: 10.1038/nature02561.1
Kirschel, A. N. G., Blumstein, D. T., Cohen, R. E., Buermann, W., Smith, T. B., and Slabbekoorn, H. (2009a). Birdsong tuned to the environment: Green hylia song varies with elevation, tree cover, and noise. Behav. Ecol. 20, 1089–1095. doi: 10.1093/beheco/arp101
Kirschel, A. N. G., Blumstein, D. T., and Smith, T. B. (2009b). Character displacement of song and morphology in African tinkerbirds. Proc. Natl. Acad. Sci. U. S. A. 106, 8256–8261.
Kirschel, A. N. G., Nwankwo, E. C., Seal, N., and Grether, G. F. (2020). Time spent together and time spent apart affect song, feather colour and range overlap in tinkerbirds. Biol. J. Linn. Soc. 129, 439–458. doi: 10.1093/biolinnean/blz191
Kounitsky, P., Rydell, J., Amichai, E., Boonman, A., Eitan, O., Weiss, A. J., et al. (2015). Bats adjust their mouth gape to zoom their biosonar field of view. Proc. Natl. Acad. Sci. U. S. A. 112, 6724–6729. doi: 10.1073/pnas.1422843112
Krishnan, A. (2019a). Acoustic community structure and seasonal turnover in tropical South Asian birds. Behav. Ecol. 30, 1364–1374. doi: 10.1093/beheco/arz087
Krishnan, A. (2019b). Simultaneously vocalizing Asian barbets adopt different frequencies without coordinating temporal rhythms. bioRxiv 2019:754580. doi: 10.1101/754580
Krishnan, A., Singh, A., and Tamma, K. (2020). Visual signal evolution along complementary color axes in four bird lineages. Biol. Open 9:bio052316. doi: 10.1242/bio.052316
Krishnan, A., and Tamma, K. (2016). Divergent morphological and acoustic traits in sympatric communities of Asian barbets. R. Soc. Open Sci. 3:160117. doi: 10.1098/rsos.160117
Lahiri, S., Pathaw, N. A., and Krishnan, A. (2021). Convergent acoustic community structure in South Asian dry and wet grassland birds. Biol. Open 10:bio058612. doi: 10.1242/bio.058612
Law, B. S., Brassil, T., Gonsalves, L., Roe, P., Truskinger, A., and McConville, A. (2018). Passive acoustics and sound recognition provide new insights on status and resilience of an iconic endangered marsupial (koala Phascolarctos cinereus) to timber harvesting. PLoS One 13:e0205075. doi: 10.1371/journal.pone.0205075
Lellouch, L., Pavoine, S., Jiguet, F., Glotin, H., and Sueur, J. (2014). Monitoring temporal change of bird communities with dissimilarity acoustic indices. Methods Ecol. Evol. 5, 495–505. doi: 10.1111/2041-210X.12178
Lemmon, E. M. (2009). Diversification of conspecific signals in sympatry: Geographic overlap drives multidimensional reproductive character displacement in frogs. Evolution (N. Y). 63, 1155–1170. doi: 10.1111/j.1558-5646.2009.00650.x
Lewis, R. N., Williams, L. J., and Gilman, R. T. (2021). The uses and implications of avian vocalizations for conservation planning. Conserv. Biol. 35, 50–63. doi: 10.1111/cobi.13465
Linke, S., Gifford, T., Desjonquères, C., Tonolla, D., Aubin, T., Barclay, L., et al. (2018). Freshwater ecoacoustics as a tool for continuous ecosystem monitoring. Front. Ecol. Environ. 16, 231–238. doi: 10.1002/fee.1779
Littlejohn, M. J. (1959). Call differentiation in a complex of seven species of Crinia (Anura, Leptodactylidae). Evolution (N. Y). 13, 452–468. doi: 10.2307/2406128
Lohr, B., Wright, T. F., and Dooling, R. J. (2003). Detection and discrimination of natural calls in masking noise by birds: estimating the active space of a signal. Anim. Behav. 65, 763–777. doi: 10.1006/anbe.2003.2093
Luo, B., Leiser-Miller, L., Santana, S. E., Zhang, L., Liu, T., Xiao, Y., et al. (2019). Echolocation call divergence in bats: a comparative analysis. Behav. Ecol. Sociobiol. 73:154. doi: 10.1007/s00265-019-2766-9
Luther, D. (2009). The influence of the acoustic community on songs of birds in a neotropical rain forest. Behav. Ecol. 20, 864–871. doi: 10.1093/beheco/arp074
Luther, D. A. (2008). Signaller: receiver coordination and the timing of communication in Amazonian birds. Biol. Lett. 4, 651–654. doi: 10.1098/rsbl.2008.0406
Mac Aodha, O., Gibb, R., Barlow, K. E., Browning, E., Firman, M., Freeman, R., et al. (2018). Bat detective—Deep learning tools for bat acoustic signal detection. PLoS Comput. Biol. 14:e1005995. doi: 10.1371/journal.pcbi.1005995
MacDougall-Shackleton, S. A., Hulse, S. H., Gentner, T. Q., and White, W. (1998). Auditory scene analysis by European starlings (Sturnus vulgaris): perceptual segregation of tone sequences. J. Acoust. Soc. Am. 103, 3581–3587.
Magurran, A. E., Baillie, S. R., Buckland, S. T., Dick, J. M. P., Elston, D. A., Scott, E. M., et al. (2010). Long-term datasets in biodiversity research and monitoring: Assessing change in ecological communities through time. Trends Ecol. Evol. 25, 574–582. doi: 10.1016/j.tree.2010.06.016
Mancina, C. A., García-Rivera, L., and Miller, B. W. (2012). Wing morphology, echolocation, and resource partitioning in syntopic Cuban mormoopid bats. J. Mamm. 93, 1308–1317. doi: 10.1644/11-mamm-a-331.1
Marler, P., and Slabbekoorn, H. (2004). Nature’s Music: the Science of Birdsong. London, UK: Elsevier Academic Press.
Marten, K., and Marler, P. (1977). Sound transmission and its significance for animal vocalization - I. Temperate habitats. Behav. Ecol. Sociobiol. 2, 271–290. doi: 10.1007/BF00299740
Mathevon, N., Dabelsteen, T., and Blumenrath, S. H. (2005). Are high perches in the blackcap Sylvia atricapilla song or listening posts? A sound transmission study. J. Acoust. Soc. Am. 117, 442–449. doi: 10.1121/1.1828805
Mennill, D. J., Battiston, M., Wilson, D. R., Foote, J. R., and Doucet, S. M. (2012). Field test of an affordable, portable, wireless microphone array for spatial monitoring of animal ecology and behaviour. Methods Ecol. Evol. 3, 704–712. doi: 10.1111/j.2041-210X.2012.00209.x
Meyer, C. F. J., Aguiar, L. M. S., Aguirre, L. F., Baumgarten, J., Clarke, F. M., Cosson, J. F., et al. (2011). Accounting for detectability improves estimates of species richness in tropical bat surveys. J. Appl. Ecol. 48, 777–787. doi: 10.1111/j.1365-2664.2011.01976.x
Miller, L. A., and Degn, H. J. (1981). The acoustic behavior of four species of vespertilionid bats studied in the field. J. Comp. Physiol. A 142, 67–74. doi: 10.1007/BF00605477
Mooney, T. A., Di Iorio, L., Lammers, M., Lin, T. H., Nedelec, S. L., Parsons, M., et al. (2020). Listening forward: Approaching marine biodiversity assessments using acoustic methods. R. Soc. Open Sci. 7:201287. doi: 10.1098/rsos.201287
Morton, E. S. (1975). Ecological sources of selection on avian sounds. Am. Nat. 109, 17–34. doi: 10.1086/282971
Naguib, M. (1995). Auditory distance assessment of singing conspecifies in Carolina wrens: The role of reverberation and frequency-dependent attenuation. Anim. Behav. 50, 1297–1307. doi: 10.1016/0003-3472(95)80045-X
Narins, P. M. (1992). Evolution of anuran chorus behavior: neural and behavioral constraints. Am. Nat. 139:S90. doi: 10.1086/285306
Nelson, D. A. (1988). Feature weighting in species song recognition by the field sparrow (Spizella Pusilla). Behaviour 106, 158–181. doi: 10.1163/156853988X00142
Nelson, D. A. (1989). Song frequency as a cue for recognition of species and individuals in the field sparrow (Spizella pusilla). J. Comp. Psychol. 103, 171–176. doi: 10.1037/0735-7036.103.2.171
Nelson, D. A., and Marler, P. (1990). “The perception of birdsong and an ecological concept of signal space,” in Comparative Perception: Complex Signals, Vol. 2, eds W. C. Stebbins and M. A. Berkley (New York: Wiley), 443–478.
Nemeth, E., Winkler, H., and Dabelsteen, T. (2002). Differential degradation of antbird songs in a Neotropical rainforest: Adaptation to perch height? J. Acoust. Soc. Am. 110, 3263–3274. doi: 10.1121/1.1420385
Nocera, T., Ford, W. M., Silvis, A., and Dobony, C. A. (2019). Patterns of acoustical activity of bats prior to and 10 years after WNS on fort drum Army installation, New York. Glob. Ecol. Conserv. 18:e00633. doi: 10.1016/j.gecco.2019.e00633
Norberg, U. M., and Rayner, J. M. V. (1987). Ecological morphology and flight in bats (Mammalia; Chiroptera): wing adaptations, flight performance, foraging strategy and echolocation. Philos. Trans. R. Soc. B Biol. Sci. 316, 335–427.
Núñez, S. F., López-Baucells, A., Rocha, R., Farneda, F. Z., Bobrowiec, P. E. D., Palmeirim, J. M., et al. (2019). Echolocation and stratum preference: key trait correlates of vulnerability of insectivorous bats to tropical forest fragmentation. Front. Ecol. Evol. 7:373. doi: 10.3389/fevo.2019.00373
Parks, S. E., Miksis-Olds, J. L., and Denes, S. L. (2014). Assessing marine ecosystem acoustic diversity across ocean basins. Ecol. Inform. 21, 81–88. doi: 10.1016/j.ecoinf.2013.11.003
Parsons, S., and Jones, G. (2000). Acoustic identification of twelve species of echolocating bat by discriminant function analysis and artificial neural networks. J. Exp. Biol. 203, 2641–2656. doi: 10.1080/09524622.2006.9753565
Patricelli, G. L., Dantzker, M. S., and Bradbury, J. W. (2007). Differences in acoustic directionality among vocalizations of the male red-winged blackbird (Agelaius pheoniceus) are related to function in communication. Behav. Ecol. Sociobiol. 61, 1099–1110. doi: 10.1007/s00265-006-0343-5
Pijanowski, B. C., Farina, A., Gage, S. H., Dumyahn, S. L., and Krause, B. L. (2011a). What is soundscape ecology? An introduction and overview of an emerging new science. Landsc. Ecol. 26, 1213–1232. doi: 10.1007/s10980-011-9600-8
Pijanowski, B. C., Villanueva-Rivera, L. J., Dumyahn, S. L., Farina, A., Krause, B. L., Napoletano, B. M., et al. (2011b). Soundscape ecology: the science of sound in the landscape. Bioscience 61, 203–216. doi: 10.1525/bio.2011.61.3.6
Pillay, R., Fletcher, R. J., Sieving, K. E., Udell, B. J., and Bernard, H. (2019). Bioacoustic monitoring reveals shifts in breeding songbird populations and singing behaviour with selective logging in tropical forests. J. Appl. Ecol. 56, 2482–2492. doi: 10.1111/1365-2664.13492
Planqué, R., and Slabbekoorn, H. (2008). Spectral overlap in songs and temporal avoidance in a Peruvian bird assemblage. Ethology 114, 262–271. doi: 10.1111/j.1439-0310.2007.01461.x
Podos, J. (2001). Correlated evolution of morphology and vocal signal structure in Darwin’s finches. Nature 409, 185–188.
Popp, J., Ficken, R., and Reinartz, J. (1985). Short-term temporal avoidance of interspecific acoustic interference among forest birds. Auk 102, 744–748.
Purushotham, C. B., and Robin, V. V. (2016). Sky island bird populations isolated by ancient genetic barriers are characterized by different song traits than those isolated by recent deforestation. Ecol. Evol. 6, 7334–7343. doi: 10.1002/ece3.2475
Putland, R. L., Constantine, R., and Radford, C. A. (2017). Exploring spatial and temporal trends in the soundscape of an ecologically significant embayment. Sci. Rep. 7, 1–12. doi: 10.1038/s41598-017-06347-0
Qvarnström, A., Haavie, J., Sæther, S. A., Eriksson, D., and Pärt, T. (2006). Song similarity predicts hybridization in flycatchers. J. Evol. Biol. 19, 1202–1209. doi: 10.1111/j.1420-9101.2006.01140.x
Raghuram, H., Jain, M., and Balakrishnan, R. (2014). Species and acoustic diversity of bats in a palaeotropical wet evergreen forest in southern India. Curr. Sci. 107, 631–641. doi: 10.18520/cs/v107/i4/631-641
Richman, N. I., Gibbons, J. M., Turvey, S. T., Akamatsu, T., Ahmed, B., Mahabub, E., et al. (2014). To see or not to see: Investigating detectability of Ganges river dolphins using a combined visual-acoustic survey. PLoS One 9:e096811. doi: 10.1371/journal.pone.0096811
Riede, T., Suthers, R. A., Fletcher, N. H., and Blevins, W. E. (2006). Songbirds tune their vocal tract to the fundamental frequency of their song. Proc. Natl. Acad. Sci. U. S. A. 103, 5543–5548. doi: 10.1073/pnas.0601262103
Robert, A., Lengagne, T., Melo, M., Gardette, V., Julien, S., Covas, R., et al. (2019). The theory of island biogeography and soundscapes: Species diversity and the organization of acoustic communities. J. Biogeogr. 46, 1901–1911. doi: 10.1111/jbi.13611
Roemer, C., Coulon, A., Disca, T., and Bas, Y. (2019). Bat sonar and wing morphology predict species vertical niche. J. Acoust. Soc. Am. 145, 3242–3251. doi: 10.1121/1.5102166
Ruppé, L., Clément, G., Herrel, A., Ballesta, L., Décamps, T., Kéver, L., et al. (2015). Environmental constraints drive the partitioning of the soundscape in fishes. Proc. Natl. Acad. Sci. U. S. A. 112, 6092–6097. doi: 10.1073/pnas.1424667112
Ryan, M. J., Tuttle, M. D., and Rand, A. S. (1982). Bat predation and sexual advertisement in a neotropical anuran. Am. Nat. 119, 136–139.
Sanders, C. E., and Mennill, D. J. (2014). Acoustic monitoring of nocturnally migrating birds accurately assesses the timing and magnitude of migration through the Great Lakes. Condor 116, 371–383. doi: 10.1650/CONDOR-13-098.1
Schmidt, A. K. D., Römer, H., and Riede, K. (2013). Spectral niche segregation and community organization in a tropical cricket assemblage. Behav. Ecol. 24, 470–480. doi: 10.1093/beheco/ars187
Schwartz, J. J., and Wells, K. D. (1983). An experimental study of acoustic interference between two species of neotropical treefrogs. Anim. Behav. 31, 181–190. doi: 10.1016/S0003-3472(83)80187-0
Seddon, N., Botero, C. A., Tobias, J. A., Dunn, P. O., Macgregor, H. E. A., Rubenstein, D. R., et al. (2013). Sexual selection accelerates signal evolution during speciation in birds. Proc. R. Soc. B Biol. Sci. 280:20131065.
Seddon, N., and Tobias, J. A. (2010). Character displacement from the receiver’s perspective: species and mate recognition despite convergent signals in suboscine birds. Proc. R. Soc. B Biol. Sci. 277, 2475–2483. doi: 10.1098/rspb.2010.0210
Shi, J. J., and Rabosky, D. L. (2015). Speciation dynamics during the global radiation of extant bats. Evolution (N. Y). 69, 1528–1545. doi: 10.1111/evo.12681
Shieh, B. S., Liang, S. H., and Chiu, Y. W. (2015). Acoustic and temporal partitioning of cicada assemblages in city and mountain environments. PLoS One 10:e0116794. doi: 10.1371/journal.pone.0116794
Siemers, B. M., and Schnitzler, H. U. (2004). Echolocation signals reflect niche differentiation in five sympatric congeneric bat species. Nature 429, 657–661. doi: 10.1038/nature02547
Snell-Rood, E. C. (2012). The effect of climate on acoustic signals: Does atmospheric sound absorption matter for bird song and bat echolocation? J. Acoust. Soc. Am. 131, 1650–1658. doi: 10.1121/1.3672695
Sprau, P., Roth, T., Naguib, M., and Amrhein, V. (2012). Communication in the third dimension: Song perch height of rivals affects singing response in nightingales. PLoS One 7:e032194. doi: 10.1371/journal.pone.0032194
Stoddard, M. C., and Prum, R. O. (2008). Evolution of avian plumage color in a tetrahedral color space: a phylogenetic analysis of new world buntings. Am. Nat. 171, 755–776.
Stowell, D., Petrusková, T., Šálek, M., and Linhart, P. (2019). Automatic acoustic identification of individuals in multiple species: Improving identification across recording conditions. J. R. Soc. Interface 16:20180940. doi: 10.1098/rsif.2018.0940
Sueur, J., and Farina, A. (2015). Ecoacoustics: The ecological investigation and interpretation of environmental sound. Biosemiotics 8, 493–502. doi: 10.1007/s12304-015-9248-x
Sueur, J., Krause, B., and Farina, A. (2019). Climate change is breaking Earth’s beat. Trends Ecol. Evol. 34, 971–973. doi: 10.1016/j.tree.2019.07.014
Sugai, L. S. M., Desjonquères, C., Silva, T. S. F., and Llusia, D. (2019a). A roadmap for survey designs in terrestrial acoustic monitoring. Remote Sens. Ecol. Conserv. 6, 220–235. doi: 10.1002/rse2.131
Sugai, L. S. M., Silva, T. S. F., Ribeiro, J. W., and Llusia, D. (2019b). Terrestrial passive acoustic monitoring: review and perspectives. Bioscience 69, 5–11. doi: 10.1093/biosci/biy147
Sugai, L. S. M., Llusia, D., Siqueira, T., and Silva, T. S. F. (2021a). Revisiting the drivers of acoustic similarities in tropical anuran assemblages. Ecology 102:e03380. doi: 10.1002/ecy.3380
Sugai, L. S. M., Silva, T. S. F., Llusia, D., and Siqueira, T. (2021b). Drivers of assemblage-wide calling activity in tropical anurans and the role of temporal resolution. J. Anim. Ecol. 90, 673–684. doi: 10.1111/1365-2656.13399
Surlykke, A., Pedersen, S. B., and Jakobsen, L. (2012). Echolocating bats emit a highly directional sonar sound beam in the field. Proc. R. Soc. B Biol. Sci. 276, 853–860. doi: 10.1098/rspb.2008.1505
Templeton, C. N., Zollinger, S. A., and Brumm, H. (2016). Traffic noise drowns out great tit alarm calls. Curr. Biol. 26, R1173–R1174. doi: 10.1016/j.cub.2016.09.058
Tobias, J. A., Planque, R., Cram, D. L., and Seddon, N. (2014). Species interactions and the structure of complex communication networks. Proc. Natl. Acad. Sci. 111, 1020–1025. doi: 10.1073/pnas.1314337111
Torrent, L., López-Baucells, A., Rocha, R., Bobrowiec, P. E. D., and Meyer, C. F. J. (2018). The importance of lakes for bat conservation in Amazonian rainforests: an assessment using autonomous recorders. Remote Sens. Ecol. Conserv. 4, 339–351. doi: 10.1002/rse2.83
Ulloa, J. S., Aubin, T., Llusia, D., Bouveyron, C., and Sueur, J. (2018). Estimating animal acoustic diversity in tropical environments using unsupervised multiresolution analysis. Ecol. Indic. 90, 346–355. doi: 10.1016/j.ecolind.2018.03.026
Van Parijs, S. M., Kovacs, K. M., and Lydersen, C. (2001). Spatial and temporal distribution of vocalising male bearded seals - Implications for male mating strategies. Behaviour 138, 905–922. doi: 10.1163/156853901753172719
Vélez, A., Gordon, N. M., and Bee, M. A. (2017). The signal in noise: Acoustic information for soundscape orientation in two North American tree frogs. Behav. Ecol. 28, 844–853. doi: 10.1093/beheco/arx044
Villanueva-Rivera, L. J., Pijanowski, B. C., Doucette, J., and Pekin, B. (2011). A primer of acoustic analysis for landscape ecologists. Landsc. Ecol. 26, 1233–1246. doi: 10.1007/s10980-011-9636-9
Webb, C. O. (2000). Exploring the phylogenetic structure of ecological communities: an example for rain forest trees. Am. Nat. 156, 145–155. doi: 10.1086/303378
Weller, T. J., and Baldwin, J. A. (2012). Using echolocation monitoring to model bat occupancy and inform mitigations at wind energy facilities. J. Wildl. Manage. 76, 619–631. doi: 10.1002/jwmg.260
Wheeldon, A., Mossman, H. L., Sullivan, M. J. P., Mathenge, J., and de Kort, S. R. (2019). Comparison of acoustic and traditional point count methods to assess bird diversity and composition in the Aberdare National Park, Kenya. Afr. J. Ecol. 57, 168–176. doi: 10.1111/aje.12596
Wiley, R. H. (1991). Associations of song properties with habitats for territorial oscine birds of eastern North America. Am. Nat. 138, 973–993. doi: 10.1086/285263
Wiley, R. H., and Richards, D. G. (1978). Physical constraints on acoustic communication in the atmosphere: implications for the evolution of animal vocalizations. Behav. Ecol. Sociobiol. 3, 69–94. doi: 10.1080/00986445.2011.534011
Wilkins, M. R., Seddon, N., and Safran, R. J. (2013). Evolutionary divergence in acoustic signals: causes and consequences. Trends Ecol. Evol. 28, 156–166. doi: 10.1016/j.tree.2012.10.002
Wollerman, L., and Wiley, R. H. (2002). Background noise from a natural chorus alters female discrimination of male calls in a neotropical frog. Anim. Behav. 63, 15–22. doi: 10.1006/anbe.2001.1885
Wordley, C. F. R., Sankaran, M., Mudappa, D., and Altringham, J. D. (2018). Heard but not seen: Comparing bat assemblages and study methods in a mosaic landscape in the Western Ghats of India. Ecol. Evol. 8, 3883–3894. doi: 10.1002/ece3.3942
Wrege, P. H., Rowland, E. D., Keen, S., and Shiu, Y. (2017). Acoustic monitoring for conservation in tropical forests: examples from forest elephants. Methods Ecol. Evol. 8, 1292–1301. doi: 10.1111/2041-210X.12730
Yorzinski, J. L., and Patricelli, G. L. (2010). Birds adjust acoustic directionality to beam their antipredator calls to predators and conspecifics. Proc. R. Soc. B Biol. Sci. 277, 923–932. doi: 10.1098/rspb.2009.1519
Keywords: community bioacoustics, signal space, acoustic community, passive acoustic monitoring, conservation
Citation: Chhaya V, Lahiri S, Jagan MA, Mohan R, Pathaw NA and Krishnan A (2021) Community Bioacoustics: Studying Acoustic Community Structure for Ecological and Conservation Insights. Front. Ecol. Evol. 9:706445. doi: 10.3389/fevo.2021.706445
Received: 07 May 2021; Accepted: 05 July 2021;
Published: 30 July 2021.
Edited by:
Daniel Marques Almeida Pessoa, Federal University of Rio Grande do Norte, BrazilReviewed by:
Larissa Sugai, Autonomous University of Madrid, SpainManjari Jain, Indian Institute of Science Education and Research Mohali, India
Copyright © 2021 Chhaya, Lahiri, Jagan, Mohan, Pathaw and Krishnan. This is an open-access article distributed under the terms of the Creative Commons Attribution License (CC BY). The use, distribution or reproduction in other forums is permitted, provided the original author(s) and the copyright owner(s) are credited and that the original publication in this journal is cited, in accordance with accepted academic practice. No use, distribution or reproduction is permitted which does not comply with these terms.
*Correspondence: Anand Krishnan, YW5hbmRrQGlpc2VycHVuZS5hYy5pbg==
†These authors have contributed equally to this work