- 1State Key Laboratory of Resources and Environmental Information System, Institute of Geographic Sciences and Natural Resources Research, Chinese Academy of Sciences, Beijing, China
- 2College of Resources and Environment, University of Chinese Academy of Sciences, Beijing, China
The Sanjiang Plain includes the largest freshwater marsh in China, playing an important role in regional carbon cycle. As an important indicator of carbon cycle, the net primary productivity (NPP) is a crucial index for estimating the carbon storage of marshy wetlands. Investigating the association between climate factors and NPP variation quantitatively is of great significance for estimating carbon sequestration of marsh. Based on NPP data and climatic data from 1954 to 2014, the spatiotemporal change of NPP in marsh area was analyzed and its association with climate factors was investigated in the Sanjiang Plain in this study. The results indicated that the NPP showed an increase trend in the marsh area of the Sanjiang Plain in the past six decades. Temperate growth made the largest contribution to the NPP increase among the main climate factors in the last six decades, followed by CO2 concentration. Solar Radiation had the largest explanatory power on the spatial distribution of NPP among three climate factors before 1985. After 1985, temperature played an important role in leading the NPP distribution. Results also showed that the explanatory power of interactions between climate factors was stronger than that of single factor. Our results highlight the asymmetric effects of interactions between climate factors on marsh vegetation, which should be adequately considered in estimating carbon sequestration in marsh area in the Sanjiang Plain.
Introduction
Wetlands are regions with low-level water, which are usually covered by vegetation during growing season (Vivian et al., 2014; Marthews et al., 2022). As the kidneys of the Earth, wetlands play an important role in biogeochemical cycle (Tercero et al., 2017; Xiao et al., 2019; Deng et al., 2022). Wetlands provide a lot of ecosystem service values for mankind such as water conservation, biodiversity maintenance, coastal protection, water purification and flood mitigation (Yan and Zhang, 2019; Thomaz, 2021; Monge-Salazar et al., 2022; Tong et al., 2022). It is estimated that wetlands store 20–30% of the earth’s carbon soil pool while covering only approximately 5–8% of the world’s land surface (Mitsch and Gosselink, 2000; Roulet, 2000). Wetlands are good natural environments for separating and storing carbon from the atmosphere, due to their anoxic and humid conditions (O’Connor et al., 2010; Dargie et al., 2017). Net primary productivity (NPP) refers to the difference between the total amount of organic matter accumulated by green vegetation in the ecosystem through photosynthesis and the cumulative respiration per unit time. NPP, a significant indicator of carbon cycle, is the basis of the energy and material cycle of the ecosystem, which is related to the carbon fixation capacity of the ecosystem (Brouwers and Coops, 2016; Xiao et al., 2019). Studies have indicated that climate variation can influence vegetation coverage, growth conditions and growing season duration, leading to NPP variation and the subsequent carbon cycle (Brouwers and Coops, 2016; Li et al., 2018; Sun et al., 2022). Therefore, it is of great significance to investigate the effects of climate change on NPP variation in wetland areas quantitatively.
As one of the driving factors of vegetation growth (Schimel et al., 2000; Cao et al., 2022b; Ren et al., 2022), climate is influenced by land use and land cover changes (LUCC), and vice versa. Generally, climate warming enhances vegetation growth globally, with negative impacts in low latitudes (Shestakova et al., 2019; Madani et al., 2021; Cao et al., 2022b; Chen et al., 2022; Qiu et al., 2022). Additionally, increased precipitation would promote vegetation growth in water-limited regions. Accordingly, vegetation greening could produce biophysical feedback to climate, such as increase of evapotranspiration, surface cooling (Davin and de Noblet-Ducoudre, 2010). Climate changes are expected to act critical roles in the spatiotemporal patterns of vegetation growth. Previous studies usually focus on the response of vegetation growth on single climate factor (Brouwers and Coops, 2016; Madani et al., 2021; Chen et al., 2022), ignoring the response of vegetation growth on interactions between the dominate climate factors.
Although the effects of climate changes on vegetation NPP variation have become hot topics in the field of global change, most of which focus on forest, agriculture or grassland ecosystems (Schimel et al., 2000; Zarei et al., 2021; Cao et al., 2022a; Ji et al., 2022). Few studies investigated the response of NPP variation to climate change in wetland ecosystem, which has a unique environmental condition (Mao et al., 2014; Caplan et al., 2015; Wang et al., 2022). Due to the anoxic and humid condition, there are obvious differences in the effect of climate change on vegetation between wetland and other ecosystems (Zhang et al., 2016; Shen et al., 2019; Wang et al., 2022). Marsh is the main type of wetlands, playing an important role in carbon sequestration (Shen et al., 2021). Therefore, clarifying the effect of climate change on marsh NPP is crucial for assessing regional carbon storage and understanding carbon cycle. However, the quantitative association between climate change and NPP variation in marsh area remains poorly understood.
The Sanjiang Plain includes the largest freshwater marsh distribution area in China, which has also experienced intense human activities in the past decades (Wang et al., 2015; Yan, 2020). As a dominant type of wetlands in the Sanjiang Plain, marsh provides important ecosystem services (Wang et al., 2015; Yan and Zhang, 2019). And vegetation is crucial for carbon sequestration in the Sanjiang plain marshy wetland. Liu et al. (2015) estimated the NPP changes of Calamagrostis Angustifolia marsh at Fujin City in the Sanjiang Plain under IPCC SRES emission scenarios and found that increase of CO2 concentration and temperature have a positive impact on the vegetation growth of wetland vegetation (Liu et al., 2015). Additionally, the effect of precipitation on NPP vegetation was more significant than that of temperature. Mao et al. (2014) found that human activities caused a loss of 84 gC m–2 yr–1 in marsh NPP during 2000–2011 in Northeast China (Mao et al., 2014); Wang et al. (2009) suggested that land use and land cover (LUCC) is the main factor affecting vegetation NPP in the Sanjiang Plain from 2000 to 2015 (Wang et al., 2009). Considering that climate factors are also important factors, it is necessary to investigate the response of marshy wetland NPP on climate changes in the Sanjiang Plain for a long-time.
To address these knowledge gaps, we investigated the association between climate change and NPP variation quantitatively in the Sanjing marsh area in the past six decades. Firstly, I analyzed the spatiotemporal changes in NPP and climate factors. Secondly, the association between climate changes and NPP changes was explored quantitatively. Lastly, the response of NPP distribution on climate factors and climate interactions were estimated quantitatively in two stages: stage I (1954–1985) and stage II (1986–2014).
Materials and methods
Study area
The Sanjiang Plain (Figure 1) is located in the northeastern part of Heilongjiang Province, from 45°01′05“N to 48°27′56” N and from 130°13′10“E to 135°05′26”E, with a total area of about 108,900 km2 (Yan and Zhang, 2019). The Sanjiang Plain consists of the alluvial deposits of three rivers (the Amur, Ussuri, and Songhua rivers), and is the largest distribution of freshwater marsh in China. The Sanjiang Plain consists of 23 counties, namely Fuyuan, Tongjiang, Hegang, Luobei, Suibin, Fujin, Raohe, Huachuan, Jixian, Youyi, Tangyuan, Jiamusi, Shuangyashan, Baoqing, Hulin, Yilan, Huainan, Qitaihe, Boli, Mishan, Jixi, Jidong, and Mulin. The study area in this study concentrates on the marsh wetland distribution area and its associated land use/cover changes (based on the marsh distribution in 1954).
Data and methods
The climatic data (1954–2014) in this study include average temperature, precipitation and solar radiation. The meteorological data were obtained from the meteorological station data of China National Meteorological Data Sharing Center. A total of 10 meteorological data were selected, 7 of which are within the Three Rivers Plain and 3 outside the region. Using Kriging interpolation by ArcGIS software, combined with Fortran programming, the meteorological station data was interpolated into raster format with a resolution of 2 km × 2 km. The CO2 concentration data was download from the Carbon Dioxide Information Analysis Center (CDIAC), whose website is: https://cdiac.ess-dive.lbl.gov/.
The NPP data was obtained from previous publication (Yan, 2017), which was produced by Dynamic Land Ecosystem Model. DLEM comprehensively considers the influence of carbon nitrogen coupling on carbon cycle and is well-evaluated in global and regional scale including China, United States and India etc. In the DLEM model, NPP was defined as the difference between gross primary productivity (GPP) and plant autotrophic respiratory consumption (RA).
The simulation of GPP (Unit: g Cm–2day–1) is based on the improved Farquar model (Farquhar et al., 1980), and the canopy is divided into sunlit and shaded layers.
Where GPPsun and GPPshade represent the GPP of sunlit and shaded canopy, respectively. Asun and Ashade are the assimilation rates while plaisun and plaishade are the indices of sunlit and shaded canopy, respectively. dayl represents the daily daytime length.
The plaisun and plaishade are calculated as followed:
projLAI is the leaf carbon content (g C m–2) multiplied by the specific leaf area (SLA) of different vegetation function type. DLEM sets the carbon assimilation rate as the minimum of three limiting rates (wc,wj,we). For the C3 species, they represent the assimilation rates limited by the efficiency of the photosynthetic enzymes system, available light photosynthetically active radiation (PAR) and transport of photosynthetic products, respectively. For the C3 species, we is the assimilation rates limited by PEP carboxylase.
Mr and Gr denote maintenance and growth respiration, respectively. In the DLEM,25% of GPP is considered to be used as Gr.
The Mr of different plants (leaf, sapwood, fine root and coarse foot) is estimated as follows:
Where i is the carbon pool of different plants. Mri is the maintenance respiration of different carbon pools. Rf denotes growing phase. It is defined as 0.5 in non-growing season and 1 in growing season. The growing season and non-growing season are defined according to the vegetation phenology observed by satellite.; Rcoeff_i is the respiration coefficient of different plant function types. Ni(g N m–2)is the nitrogen content of different pools and f(T) is a temperature factor.
T is the daily average temperature of the aboveground carbon pools (i.e., leaf and sapwood), or the soil temperature of the underground carbon pool (i.e., coarse roots and fine roots).
The LUCC data were obtained from remote sensing images. LUCC in 1976 was dominated by Landsat MSS imagery with a spatial resolution of 80 m. LUCC in 1986/2000/2010 were dominated by Landsat TM imagery while LUCC in 2015 was obtained from Landsat OIL imagery, with a spatial resolution of 30 m. Remote sensing imagery for the Landsat series is available on the USGS website.1 LUCC data since 1976 were obtained based on a uniform set of standard processes (Liu et al., 2002, 2010). The land use data in 1954 were reconstructed from the Cellular Automata (CA) model (Yan and Zhang, 2019). To reduce post-classification error, LUCC maps were produced by comparing Landsat imagery between different years (Liu et al., 2003, 2014). The accuracies of the LUCC data interpretation were verified by comparing with a large number of field surveys with historical records, including aerial films, statistical yearbooks, etc. (Liu et al., 2002, 2003, 2014).
The Pearson Correlation Coefficient was used to detect the correlation between NPP increase and four climate factors (solar radiation, average temperature, precipitation and CO2 concentration).
Where r denotes the Pearson Correlation Coefficient. and are the average values of x and y, respectively.
The Geographical Detection Method (GDM) was used to estimate the association between climatic factors and the spatial heterogeneity of NPP quantitatively in this study. GDM is a kind of variance analysis, which can detect spatial heterogeneity and potential factors (Wang et al., 2010). The GDM can identify the factors influence stratified heterogeneity without requiring a linear hypothesis. The GDM method has been widely used in many aspects including natural sciences, social sciences, environmental sciences and human health (Zhang and Zhao, 2018; Bai et al., 2019; Zhao et al., 2020b; Chang et al., 2022a; Guo et al., 2022). GDM includes four parts, namely, factor detector, interaction detector, ecological detector a; 43nd risk detector. The factor detector detects the magnitude of the influence of different environmental factors on the spatial variation of NPP. The interaction detector can reveal the interaction between different factors, that is evaluate the change of explanatory power when different factors interact in pairs. The higher the value of q, the greater the influence, and the expression is as follows (Wang et al., 2010):
where q (∈[0,1]) is the explanatory power of different factors and h is the classification of the impact factors. Nh and N are, respectively, the layer h and the number of samples in the whole region; σh2 and σ2 are the variances of the Y-values for stratum h and the whole region, respectively. SSW and SST are the sum of within Sum of Squares and Total Sum of Squares, respectively. In this study, solar radiation, average temperature and precipitation are selected as influencing factors, and these the numerical variables are divided into 9 types by using the Natural Breaks classification method. Since the atmospheric CO2 concentration was uniform spatially in the same year, it was not considered in the analysis of influencing factors of spatial differentiation.
Results
Net primary productivity changes
Temporal change analysis
The total NPP in the Sanjiang Plain marshy area showed an increasing trend generally, with a decreasing trend in the 1950s. The statistical analysis of the data shows that the total NPP in the study area increased from 29.99 Tg Cyr-1 in the 1950s to 30.94 Tg Cyr-1 in the 2010s (Figure 2), with an increase of 3.2%. The highest value of the total NPP occurred in 2014 while the lowest value was in 1964.
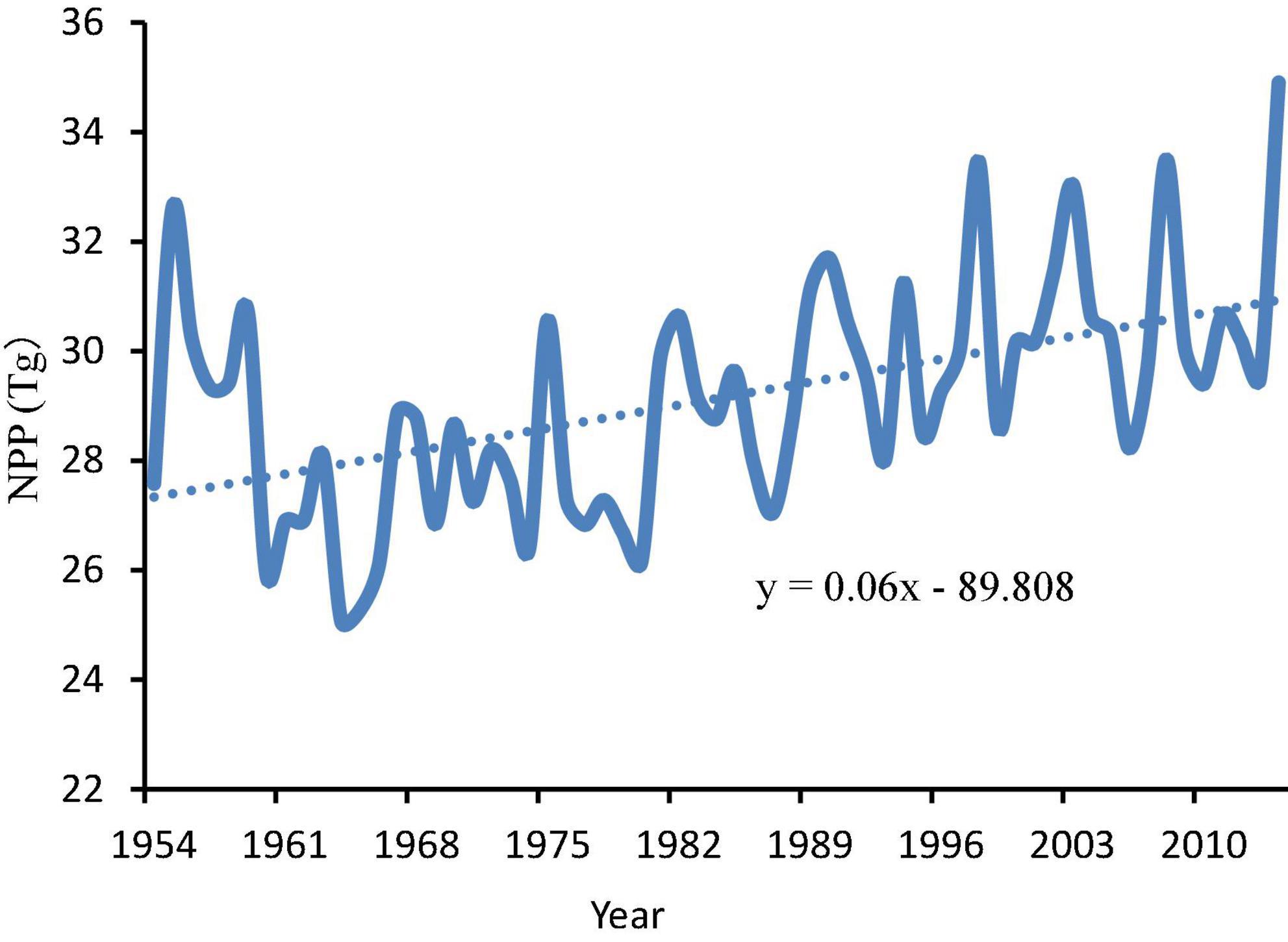
Figure 2. Temporal changes of net primary productivity since 1954 in the marsh area of the Sanjiang Plain.
The NPP changes between different decades were also analyzed (Figure 2). The total NPP showed a decreasing trend between the 1950s and 1960s, with a decrease of approximately 3.12 TgC. During the 1960s–1970s, the total NPP increased by 0.81 Tg C while it increased by 1.21 TgC between 1970s and 1980s. The increase of NPP during the 1980s–1990s was nearly same as that of the 1970s–1980s. The NPP increased by approximately 0.63 TgC and 0.21 TgC during the 1990s–2000s and 2000s–2010s, respectively.
Spatial change analysis
In this study, the spatial distribution of NPP in the marshy areas of the study area during the 1950s and 2010s and its difference in the marshy areas of the study area were analyzed (Figure 3). The results showed that the NPP in the marshy wetlands of the Sanjiang Plain was mostly between 300 and 400 gC/m–2/yr. And the average NPP values in the southern part of the study area are greater than those in the northern part. In areas where marshy wetland was not been disturbed, the mean marsh NPP values tend to decrease. Additionally, NPP tends to decrease in areas where marsh were transformed into dry farmland, bare land and water body while NPP tends to increase in areas where marsh were transformed into woodland and paddy fields.
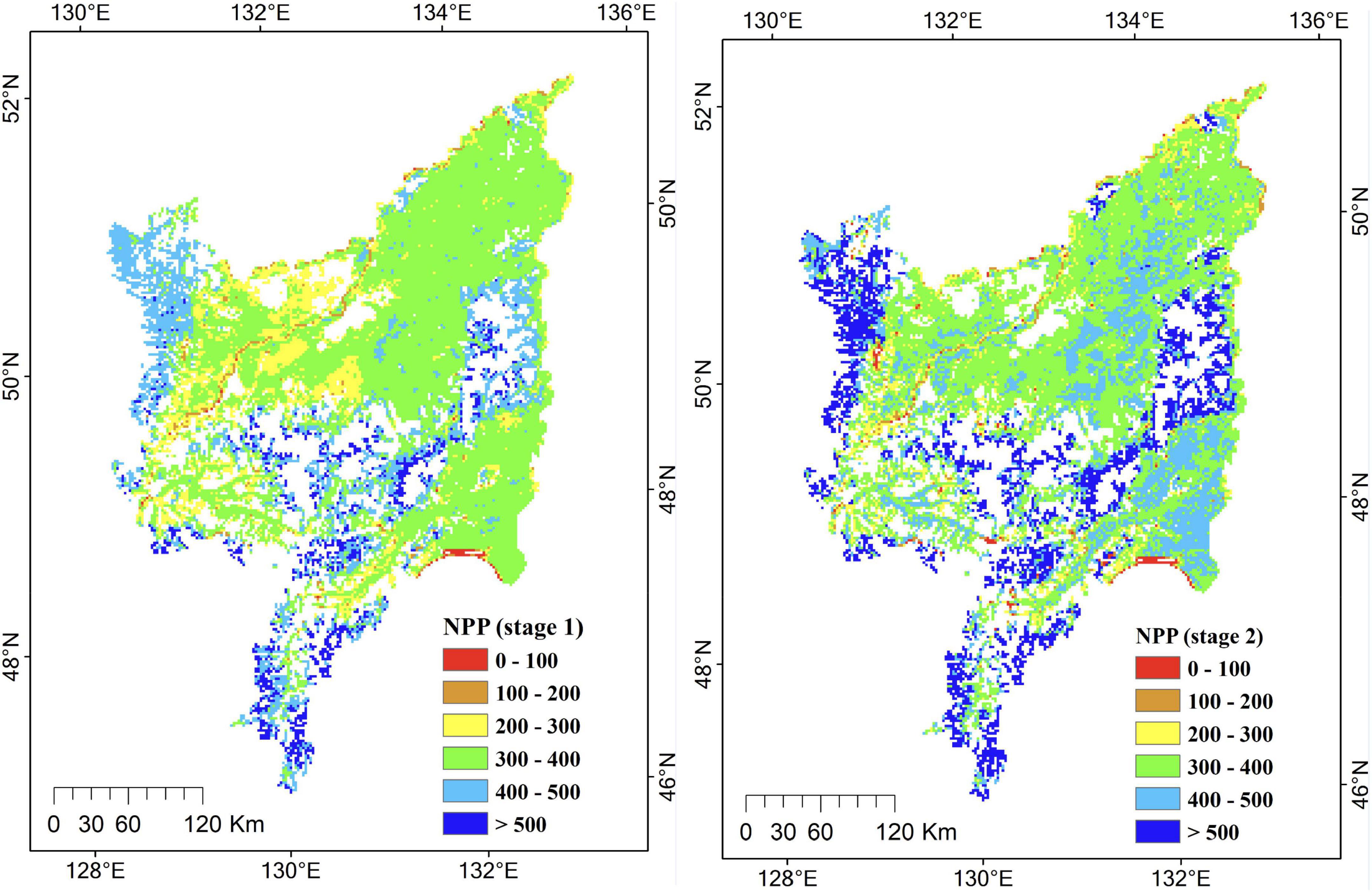
Figure 3. Spatial changes of net primary productivity since 1954 in the marsh area of the Sanjiang Plain (unit: gC/m–2/yr).
Changes of other environmental variables
The average temperature in the Sanjiang Plain has shown an increasing trend over the past 60 years or so (Figure 4). The highest temperature in the study area occurred in 2007 (4.78°C) while the lowest temperature occurred in 1969 (1.03°C), with a mean increase rate of approximately 0.23°C/10a. Between 1954 and 2014, the average temperature in the Sanjiang Plain was 3.3°C, with a relatively high inter-annual variability. The mean temperature between 1954 and 1985 was 2.88°C while that between 1985 and 2014 was 3.75°C.
During the period 1954–2014, the average annual precipitation in the Sanjiang Plain was 577.41 mm/year (Figure 5), with a relatively high inter-annual variability. Statistical data indicates that the maximum annual precipitation can reach to 810.52 mm (1994) while the minimum can reach to 396.94 mm (1976). There was a weak increasing trend in general during the past six decades in the Sanjiang Plain.
This study mapped the interannual variation of solar radiation in the Sanjiang Plain during the period 1954–2014 (as shown in Figure 6). Statistical analysis of the data shows that solar radiation in the Sanjiang Plain has generally shown a weak fluctuating growth trend over the past 60 years. The maximum solar radiation can reach to 168.08 W/m2 (1986) while the minimum solar radiation was 156.93 W/m2 (1971). There is no obvious trend in the annual solar radiation variation in the Sanjiang Plain, with a decreasing trend after an increasing trend.
This study mapped the annual variation of CO2 concentration in the Sanjiang Plain from 1954 to 2014 (as shown in Figure 7). Statistical analysis indicates that CO2 concentration in the Sanjiang showed an obvious growth trend over the past 60 years, with an increase value of 85.3 ppm. It increased from 312.4 ppm in 1954 to 397.7 ppm in 2014, increasing by 21.45% in the last six decades.
Relationship between net primary productivity and climate factors
Correlation analysis
This study detected the correlation between NPP and different climate factors during the past six decades (as shown in Table 1). Results indicate that the average temperature had the largest correlation with NPP (0.6988) among four climate factors, followed by CO2 (0.5442). The correlation coefficient between NPP and other two factors were relatively small, with a value of 0.1703 (precipitation) and 0.1266 (solar radiation), respectively. Results show that temperature made a great contribution to NPP increase in the marsh area in the Sanjiang Plain from 1954 to 2014. The increase of temperature promoted NPP growth.
Influencing factors of spatial heterogeneity
The explanatory power of the main climate factors to the spatial heterogeneity of NPP in two stages was detected by the GDM method. A total of 19,466 sampling points in the study area were chosen as sampling points. The p-values of the explanatory power of each factor on the spatial heterogeneity of NPP were less than 0.001, indicating that the q-values of each climate factor were statistically significant (as shown in Table 2). Result indicated that solar radiation had the largest explanatory power in stage I (1954–1985) while temperature had the largest in stage II (1986–2014). The explanatory power of precipitation was the least both in stage I (1954–1985) and stage II (1986–2014). The explanatory power of each climate factor on spatial heterogeneity of NPP in stage I (1954–1985) was larger than that in stage II (1986–2014).
This study also analyzed the explanatory power of interactions between different climate factors on spatial heterogeneity of NPP (as shown in Table 3). Results indicated that the explanatory power of interactions between climate factors was stronger than that of single factor. In stage I, the explanatory powers of interaction between solar radiation and precipitation reached to 0.3634, which is more than twice that of the single factor. The explanatory power of the interaction between temperature and precipitation was 0.2958 while that of temperature and solar radiation was 0.2705, both of which are much larger than that of single factor. In stage II, the explanatory powers of interaction between solar radiation and precipitation reached to 0.2475, which is more than three times that of the single factor. The explanatory power of the interaction between temperature and precipitation was 0.2050 while that of temperature and solar radiation was 0.1943, both of which are much larger than single factor.
Discussion
The effect of climate changes on net primary productivity variation
The effect of temperature increase on NPP has two aspects: positive effect and negative effect (Brouwers and Coops, 2016; Madani et al., 2021; Ji et al., 2022). On one hand, temperature increase can prolong the growing season and enhance the efficiency of photosynthesis, thereby increasing NPP (Madani et al., 2021; Ji et al., 2022). On the other hand, it can increase water consumption and cause drought, leading to a negative effect on NPP growth (Osborne et al., 2000; Bilgili et al., 2020). Results indicate that the annual average temperature in Sanjiang Plain showed an upward trend. And there was a consistency between the growth trend of NPP and that of temperature, which shows that the increase of temperature has a positive effect on the growth of NPP in Sanjiang plain at middle and high latitudes. Global NPP is increased under the influence of climate warming generally, with NPP decreased in low latitudes and increased in middle and high latitudes (Hou et al., 2013; Tum et al., 2016; Gang et al., 2017; Yin et al., 2020). This study confirms that climate warming promotes the growth of NPP in middle and high latitudes. The influence of climate warming on NPP changes is manifested in two aspects. On the one hand, climate warming can directly affect photosynthesis and change NPP (Madani et al., 2021). On the other hand, climate warming can change the mineralization rate of soil nitrogen and soil water content, thus indirectly affecting NPP (Wan et al., 2005). The promotion of climate warming on NPP in Sanjiang Plain may be due to the promotion of vegetation fixation of more CO2 under warming conditions, and the promotion of soil nitrogen mineralization rate. Although climate warming will reduce soil water content to a certain extent, which will lead to the decline of NPP. However, the study area is located in the humid area, which is less sensitive to the negative effect of water consumption caused by the rise of temperature than the arid/semi-arid area. In general, the increase of NPP caused by the increase of photosynthesis and soil nitrogen mineralization rate in the study area exceeded the decrease of NPP caused by the decrease of soil water content. Additionally, in the middle and high latitudes of the northern hemisphere, climate warming will prolong the vegetation growth season and increase vegetation activities, thus promoting the growth of NPP.
In the past 60 years, the increase of atmospheric CO2 concentration has promoted the growth of NPP in the study area. As an important material of photosynthesis, CO2 directly participates in photosynthesis and thus directly associated with vegetation NPP (Amthor, 1995; Running et al., 2000). The increase of atmospheric CO2 concentration will increase the CO2 flux of roots and the carbon supply of microorganisms, increase the demand for water and nitrogen in the process of plant photosynthesis, and lead to the enhancement of vegetation activities, thus promoting the increase of NPP (Amthor, 1995; Reich et al., 2006). Additionally, the change of CO2 concentration can disturb the earth’s radiation balance and affect the change of climate factors such as temperature, precipitation and relative humidity (Pörtner et al., 2005; Reich et al., 2006). The change of these climate factors will also have an impact on the NPP changes. Many studies have also indicated that the increase of CO2 concentration can promote NPP growth (Amthor, 1995; Cao and Woodward, 1998; Schimel et al., 2000).
In the past 60 years, precipitation changes have promoted the growth of NPP in the study area. Wetlands are often characterized by perennial or seasonal ponding. If the precipitation is low, it will obviously have an important impact on their vegetation growth (Liu et al., 2015). This study finds that the annual average value of NPP in the years with higher precipitation (greater than average precipitation) is 29.59 TG c/yr, while the annual average value of NPP in the years with lower precipitation (greater than average precipitation) is 29.34 tgc/yr, which is lightly less than that in the years with higher precipitation. This is not completely consistent with previous studies. For example, Wang et al. (2011) pointed that the NPP of Qixinghe marshy wetland in Sanjiang Plain was negatively correlated with the annual precipitation (Wang et al., 2011). Because the water resources in this region were relatively sufficient, the better coordination of hydrothermal conditions in years with relatively dry climate led to the increase of vegetation evaporation and photosynthesis, as well as increasing accumulation of dry matter. This may be because the marshy wetland area in this study includes not only the original marsh, but also the areas that have been converted to other land use types. Vegetation growth has a strong association with solar radiation (Moreau et al., 2003; Fang et al., 2019; Zhou et al., 2021), but previous study paid less attention on effects of solar radiation on NPP variation of marsh area. This study indicated that radiation growth enhanced NPP increase in the past six decades, which is consistent with previous studies. And solar radiation also influenced the spatial heterogeneity of NPP, especially in stage I. Although the precipitation had the least explanatory power on the spatial heterogeneity of NPP, its interaction with solar radiation had the largest explanatory power both in two stages. Additionally, this study indicated that explanatory power of interactions between two climate factors was stronger than that of single factor. Therefore, asymmetric effects of interactions between climate factors on vegetation NPP should be given more attention in the future.
The effect of land cover changes on net primary productivity variation
In the past six decades, marsh in the Sanjiang Plain has been cultivated drastically. The marsh area decreased from 3770.12 in 1954 to 778.18 thousand ha in 2015, with the percentage decreased from 34.71% of the total area in 1954 to 7.17% in 2015 (Table 4). From 1954 to 1986, the percentage of marsh in the Sanjiang Plain decreased by 24.40%, with the value much larger than that between 1986 and 2015. The area of marsh decreased by 79.4% since 1954 in the Sanjiang Plain, with a value about 2.99 million ha. Only 14% of the original marshy wetland was preserved in the last six decades. And 46% of the original marsh transformed to paddy filed while 27% of that converted to dry farmland. Large area of marsh has been reclaimed into dry farmland and then paddy field in the past six decades, leading to NPP changes. Landscape index analysis shows that the landscape fragmentation of marsh increased since the 1950s in the Sanjiang Plain (Yan and Zhang, 2019; Yan, 2020), also influencing carbon cycle and NPP changes. Previous studies indicated that LUCC in the Sangjiang Plain decreased NPP changes (Wang et al., 2009; Dong et al., 2015). The study of Sanjiang Plain from 2000 to 2005 showed that LUCC is the main factor affecting vegetation NPP, which directly leads to changes in regional NPP (Wang et al., 2009). Although NPP showed a general increasing trend in the Sanjiang Plain during 2000–2010, LUCC played a negative role in the NPP growth (Dong et al., 2015).
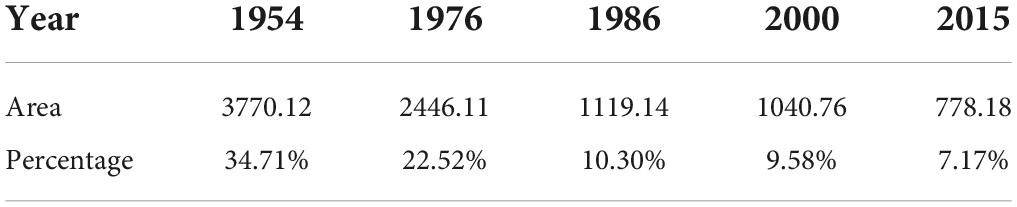
Table 4. The area (thousand ha) and percentage changes of marsh during 1954–2015 in the Sanjiang Plain.
LUCC is an important reason that affects the carbon cycle process of terrestrial ecosystem and causes the change of carbon budget (Li et al., 2014; Chang et al., 2022b). On one hand, LUCC can directly affect the carbon cycle process by changing the vegetation types (Li et al., 2014). On the other hand, LUCC can cause changes in N deposition and CO2 concentration, and then indirectly affect the carbon cycle process (Li et al., 2014; Lu et al., 2016; Zhao et al., 2020a). The research on the change of carbon budget caused by LUCC shows that the transformation between forest loss and urbanization usually reduced the carbon budget and farmland expansion can increase carbon budget in some regions (Hu and Wang, 2008; Fu et al., 2009; Yu et al., 2009; Chang et al., 2022b; Zhuang et al., 2022). For example, Yu et al. (2009) found that urbanization in Shenzhen city during 1999–2005 caused a loss of 321.51 Gg carbon. The increase of dry farmland decreased NPP in the Sanjiang plian from 2000 to 2010 (Dong et al., 2015). Study in Guangzhou indicated that conversion from wood grassland, shrublands/forests to croplands and the urban led to a large loss of NPP (Fu et al., 2013). In the past 60 years, the overall situation of LUCC in the study area is the large area loss of marsh, forest and grassland. LUCC in the marsh area in the past 60 years has a negative effect on NPP growth in the Sanjiang Plain. In the future, samples can be taken in areas where the marsh area that has not been reclaimed to exclude the impact of LUCC. Additionally, LUCC can influence temperature, precipitation, and radiation etc. (Davin and de Noblet-Ducoudre, 2010; Li et al., 2014; Cao et al., 2021). Therefore, the interactions between climate factors and LUCC should be paid more attention in the future.
Conclusion
In our study, NPP increase was observed in the Sanjiang Plain in both stages (1954–1985 and 1986–2014) in the past six decades. Temperature had the largest correlation with NPP trends among four climate factors, followed by CO2. Solar Radiation had the largest explanatory power on the spatial heterogeneity of NPP among three climate factors before 1985. After 1985, temperature played an important role in leading the spatial heterogeneity of NPP. The explanatory power of precipitation changes was relatively smaller than other two factor on NPP changes between two stages. However, the explanatory power of its interactions with solar radiation was much stronger than that of single factor. Future study should pay more attention to the interactions between climate factors as well as the interactions between different factors. Our results suggest the importance of association between climate factors and NPP variations for predicting marsh carbon storage variation.
Data availability statement
The original contributions presented in this study are included in the article/supplementary material, further inquiries can be directed to the corresponding author.
Author contributions
FY contributed to conception and design of the study, organized the database, performed the statistical analysis, wrote the first draft of the manuscript, and wrote sections of the manuscript.
Funding
This research was funded by the National Natural Science Foundation of China (grant no. 41901383).
Conflict of interest
The author declares that the research was conducted in the absence of any commercial or financial relationships that could be construed as a potential conflict of interest.
Publisher’s note
All claims expressed in this article are solely those of the authors and do not necessarily represent those of their affiliated organizations, or those of the publisher, the editors and the reviewers. Any product that may be evaluated in this article, or claim that may be made by its manufacturer, is not guaranteed or endorsed by the publisher.
Footnotes
References
Amthor, J. S. (1995). Terrestrial higher-plant response to increasing atmospheric [CO2] in relation to the global carbon cycle. Glob. Change Biol. 1, 243–274. doi: 10.1111/j.1365-2486.1995.tb00025.x
Bai, L., Jiang, L., Yang, D. Y., and Liu, Y. B. (2019). Quantifying the spatial heterogeneity influences of natural and socioeconomic factors and their interactions on air pollution using the geographical detector method: A case study of the Yangtze River Economic Belt, China. J. Clean. Prod. 232, 692–704. doi: 10.1016/j.jclepro.2019.05.342
Bilgili, B. C., Ersahin, S., Kavakligil, S. S., and Oner, N. (2020). Net primary productivity of a mountain forest ecosystem as affected by climate and topography. Cerne 26, 356–368. doi: 10.1590/01047760202026032730
Brouwers, N. C., and Coops, N. C. (2016). Decreasing net primary production in forest and shrub vegetation across southwest Australia. Ecol. Indic. 66, 10–19. doi: 10.1016/j.ecolind.2016.01.010
Cao, S. P., Zhang, L. F., He, Y., Zhang, Y. L., Chen, Y., Yao, S., et al. (2022b). Effects and contributions of meteorological drought on agricultural drought under different climatic zones and vegetation types in Northwest China. Sci. Total Environ. 821:153270. doi: 10.1016/j.scitotenv.2022.153270
Cao, D., Zhang, J. H., Han, J. Q., Zhang, T., Yang, S. S., Wang, J. W., et al. (2022a). Projected increases in global terrestrial net primary productivity loss caused by drought under climate change. Earths Future 10:e2022EF002681. doi: 10.1029/2022EF002681
Cao, F., Dan, L., Ma, Z., and Gao, T. (2021). The impact of land use and land cover change on regional climate over East Asia during 1980–2010 using a coupled model. Theor. Appl. Climatol. 145, 549–565. doi: 10.1007/s00704-021-03629-6
Cao, M., and Woodward, F. I. (1998). Net primary and ecosystem production and carbon stocks of terrestrial ecosystems and their responses to climate change. Glob. Change Biol. 4, 185–198. doi: 10.1046/j.1365-2486.1998.00125.x
Caplan, J. S., Hager, R. N., Megonigal, J. P., and Mozdzer, T. J. (2015). Global change accelerates carbon assimilation by a wetland ecosystem engineer. Environ. Res. Lett. 10:115006. doi: 10.1088/1748-9326/10/11/115006
Chang, J. J., Gong, L., Zeng, F. J., Xue, J., Mao, D. L., Cao, Y. X., et al. (2022a). Using hydro-climate elasticity estimator and geographical detector method to quantify the individual and interactive impacts on NDVI in oasis-desert ecotone. Stoch. Environ. Res. Risk Assess. 2022, 1–18. doi: 10.1007/s00477-022-02184-4
Chang, X. Q., Xing, Y. Q., Wang, J. Q., Yang, H., and Gong, W. S. (2022b). Effects of land use and cover change (LUCC) on terrestrial carbon stocks in China between 2000 and 2018. Resour. Conserv. Recycl. 182:106333. doi: 10.1016/j.resconrec.2022.106333
Chen, Z. T., Liu, H. Y., Xu, C. Y., Wu, X. C., Liang, B. Y., Cao, J., et al. (2022). Deep learning projects future warming-induced vegetation growth changes under SSP scenarios. Adv. Clim. Change Res. 13, 251–257. doi: 10.1016/j.accre.2022.01.007
Dargie, G. C., Lewis, S. L., Lawson, I. T., Mitchard, E. T. A., Page, S. E., Bocko, Y. E., et al. (2017). Age, extent and carbon storage of the central Congo Basin peatland complex. Nature 542, 86–90. doi: 10.1038/nature21048
Davin, E. L., and de Noblet-Ducoudre, N. (2010). Climatic impact of global-scale deforestation: Radiative versus nonradiative processes. J. Clim. 23, 97–112. doi: 10.1175/2009JCLI3102.1
Deng, J. M., Xiao, T. F., Fan, W. J., Ning, Z. P., and Xiao, E. Z. (2022). Relevance of the microbial community to Sb and as biogeochemical cycling in natural wetlands. Sci. Total Environ. 818:151826. doi: 10.1016/j.scitotenv.2021.151826
Dong, G., Bai, J., Yang, S., Wu, L., Cai, M., Zhang, Y., et al. (2015). The impact of land use and land cover change on net primary productivity on China’s Sanjiang Plain. Environ. Earth Sci. 74, 2907–2917. doi: 10.1007/s12665-015-4318-6
Fang, H. L., Baret, F., Plummer, S., and Schaepman-Strub, G. (2019). An overview of global leaf area index (LAI): methods, products, validation, and applications. Rev. Geophys. 57, 739–799. doi: 10.1029/2018RG000608
Farquhar, G. D., von Caemmerer, S., and Berry, J. A. (1980). A biochemical model of photosynthetic CO2 assimilation in leaves of C3 species. Planta 149, 78–90.
Fu, C., Yu, G. R., Fang, H. J., and Wang, Q. F. (2009). Effects of land use and cover change on terrestrial carbon balance of China. Prog. Geogr. 31, 88–96.
Fu, Y., Lu, X., Zhao, Y., Zeng, X., and Xia, L. (2013). Assessment impacts of weather and land use/land cover (LULC) change on Urban vegetation net primary productivity (NPP): A case study in Guangzhou, China. Remote Sens. 5, 4125–4144. doi: 10.3390/rs5084125
Gang, C. C., Zhang, Y. Z., Wang, Z. Q., Chen, Y. Z., Yang, Y., Li, J. L., et al. (2017). Modeling the dynamics of distribution, extent, and NPP of global terrestrial ecosystems in response to future climate change. Glob. Planet. Change 148, 153–165. doi: 10.1016/j.gloplacha.2016.12.007
Guo, G. H., Li, K., Zhang, D. G., and Lei, M. (2022). Quantitative source apportionment and associated driving factor identification for soil potential toxicity elements via combining receptor models, SOM, and geo-detector method. Sci. Total Environ. 830:154721. doi: 10.1016/j.scitotenv.2022.154721
Hou, S. S., Lei, L. P., and Zeng, Z. C. (2013). The response of global net primary productivity (NPP) to CO2 increasing and climate change: Evaluation of coupled model simulations. J. Food Agric. Environ. 11, 937–944.
Hu, H. F., and Wang, G. G. (2008). Changes in forest biomass carbon storage in the South Carolina Piedmont between 1936 and 2005. Forest Ecol. Manag. 255, 1400–1408. doi: 10.1016/j.foreco.2007.10.064
Ji, Y. H., Zhou, G. S., Wang, S. D., and Zhao, J. (2022). Warm-Wet climate trend enhances net primary production of the main ecosystems in China during 2000-2021. Atmosphere 13:738. doi: 10.3390/atmos13050738
Li, B. B., Fang, X. Q., Ye, Y., and Zhang, X. Z. (2014). Carbon emissions induced by cropland expansion in Northeast China during the past 300 years. Sci. China Earth Sci. 57, 2259–2268. doi: 10.1007/s11430-014-4894-4
Li, Y. N., Shao, L. Y., Yan, Z. M., Hou, H. H., Tang, Y., and Large, D. J. (2018). Net primary productivity and its control of the Middle Jurassic peatlands: An example from the southern Junggar coalfield. Sci. China Earth Sci. 61, 1633–1643. doi: 10.1007/s11430-017-9263-4
Liu, J. Y., Deng, X. Z., Liu, M. L., and Zhang, S. W. (2002). Study on the spatial patterns of land-use change and analyses of driving forces in Northeastern China during 1990-2000. Chin. Geogr. Sci. 12, 299–308. doi: 10.1007/s11769-002-0033-9
Liu, J. Y., Kuang, W. H., Zhang, Z. X., Xu, X. L., Qin, Y. W., Ning, J., et al. (2014). Spatiotemporal characteristics, patterns, and causes of land-use changes in China since the late 1980s. J. Geogr. Sci. 24, 195–210. doi: 10.1007/s11442-014-1082-6
Liu, J. Y., Liu, M. L., Zhuang, D. F., Zhang, Z. X., and Deng, X. Z. (2003). Study on spatial pattern of land-use change in China during 1995-2000. Sci. China Ser. D Earth Sci. 46, 373–384.
Liu, J. Y., Zhang, Z. X., Xu, X. L., Kuang, W. H., Zhou, W. C., Zhang, S. W., et al. (2010). Spatial patterns and driving forces of land use change in China during the early 21st century. J. Geogr. Sci. 20, 483–494. doi: 10.1007/s11442-010-0483-4
Liu, X., Wang, Y. Y., and Fan, Y. Q. (2015). Risk assessment of net primary productivity for wetland under climate change scenario: A case study of the Calamagrostis angustifolia wetland at Fujin in Sanjiang Plain, Northeast China. China Environ. Sci. 35, 3762–3770.
Lu, X. H., Jiang, H., Zhang, X. Y., and Jin, J. X. (2016). Relationship between nitrogen deposition and LUCC and its impact on terrestrial ecosystem carbon budgets in China. Sci. China Earth Sci. 59, 2285–2294. doi: 10.1007/s11430-015-5277-0
Madani, N., Parazoo, N. C., Kimball, J. S., Reichle, R. H., Chatterjee, A., Watts, J. D., et al. (2021). The impacts of climate and wildfire on ecosystem gross primary productivity in Alaska. J. Geophys. Res. Biogeosci. 126:e2020JG006078. doi: 10.1029/2020JG006078
Mao, D. H., Wang, Z. M., Li, L., Song, K. S., and Jia, M. M. (2014). Quantitative assessment of human-induced impacts on marshes in Northeast China from 2000 to 2011. Ecol. Eng. 68, 97–104. doi: 10.1016/j.ecoleng.2014.03.010
Marthews, T. R., Dadson, S. J., Clark, D. B., Blyth, E. M., Hayman, G. D., Yamazaki, D., et al. (2022). Inundation prediction in tropical wetlands from JULES-CaMa-Flood global land surface simulations. Hydrol. Earth Syst. Sci. 26, 3151–3175. doi: 10.5194/hess-26-3151-2022
Mitsch, W. J., and Gosselink, J. G. (2000). The value of wetlands: Importance of scale and landscape setting. Ecol. Econ. 35, 25–33. doi: 10.1016/S0921-8009(00)00165-8
Monge-Salazar, M. J., Tovar, C., Cuadros-Adriazola, J., Baiker, J. R., Montesinos-Tubee, D. B., Bonnesoeur, V., et al. (2022). Ecohydrology and ecosystem services of a natural and an artificial bofedal wetland in the central Andes. Sci. Total Environ. 838:155968. doi: 10.1016/j.scitotenv.2022.155968
Moreau, S., Bosseno, R., Gu, X. F., and Baret, F. (2003). Assessing the biomass dynamics of Andean bofedal and totora high-protein wetland grasses from NOAA/AVHRR. Remote Sens. Environ. 85, 516–529. doi: 10.1016/S0034-4257(03)00053-1
O’Connor, F. M., Boucher, O., Gedney, N., Jones, C. D., Folberth, G. A., Coppell, R., et al. (2010). Possible role of wetlands, permafrost, and methane hydrates in the methane cycle under future climate change: A review. Rev. Geophys. 48:RG4005. doi: 10.1029/2010RG000326
Osborne, C. P., Mitchell, P. L., Sheehy, J. E., and Woodward, F. I. (2000). Modelling the recent historical impacts of atmospheric CO2 and climate change on Mediterranean vegetation. Glob. Change Biol. 6, 445–458. doi: 10.1046/j.1365-2486.2000.00336.x
Pörtner, H. O., Langenbuch, M., and Michaelidis, B. (2005). Synergistic effects of temperature extremes, hypoxia, and increases in CO2 on marine animals: From Earth history to global change. J. Geophys. Res. 110:C09S10. doi: 10.1029/2004JC002561
Qiu, B. W., Ye, Z. Y., Chen, C. C., Tang, Z. H., Chen, Z. Q., Huang, H. Y., et al. (2022). Dense canopies browning overshadowed by global greening dominant in sparse canopies. Sci. Total Environ. 826:154222. doi: 10.1016/j.scitotenv.2022.154222
Reich, P. B., Hungate, B. A., and Luo, Y. (2006). Carbon-nitrogen interactions in terrestrial ecosystems in response to rising atmospheric carbon dioxide. Annu. Rev. Ecol. Evol. Syst. 37, 611–636. doi: 10.1146/annurev.ecolsys.37.091305.110039
Ren, Z. G., Tian, Z. H., Wei, H. T., Liu, Y., and Yu, Y. P. (2022). Spatiotemporal evolution and driving mechanisms of vegetation in the Yellow River Basin, China during 2000-2020. Ecol. Indic. 138:108832. doi: 10.1016/j.ecolind.2022.108832
Roulet, N. T. (2000). Peatlands, carbon storage, greenhouse gases, and the Kyoto Protocol: Prospects and significance for Canada. Wetlands 20, 605–615. doi: 10.1672/0277-5212(2000)020[0605:PCSGGA]2.0.CO;2
Running, S. W., Thornton, P. E., Nemani, R., and Glassy, J. M. (2000). “Global terrestrial gross and net primary productivity from the earth observing system,” in Methods in ecosystem science, eds O. E. Sala, R. B. Jackson, H. A. Mooney, and R. W. Howarth (New York, NY: Springer), 44–57. doi: 10.1007/978-1-4612-1224-9_4
Schimel, D., Melillo, J., Tian, H. Q., McGuire, A. D., Kicklighter, D., Kittel, T., et al. (2000). Contribution of increasing CO2 and climate to carbon storage by ecosystems in the United States. Science 287, 2004–2006. doi: 10.1126/science.287.5460.2004
Shen, X. J., Liu, B. H., Jiang, M., Wang, Y. J., Wang, L., Zhang, J. Q., et al. (2021). Spatiotemporal change of Marsh vegetation and its response to climate change in China From 2000 to 2019. J. Geophys. Res. Biogeosci. 126:e2020JG006154. doi: 10.1029/2020JG006154
Shen, X. J., Liu, B. H., Xue, Z. S., Jiang, M., Lu, X. G., and Zhang, Q. (2019). Spatiotemporal variation in vegetation spring phenology and its response to climate change in freshwater marshes of Northeast China. Sci. Total Environ. 666, 1169–1177. doi: 10.1016/j.scitotenv.2019.02.265
Shestakova, T. A., Gutierrez, E., Valeriano, C., Lapshina, E., and Voltas, J. (2019). Recent loss of sensitivity to summer temperature constrains tree growth synchrony among boreal Eurasian forests. Agric. Forest Meteorol. 268, 318–330. doi: 10.1016/j.agrformet.2019.01.039
Sun, H. Z., Chen, Y. B., Xiong, J. N., Ye, C. H., Yong, Z. W., Wang, Y., et al. (2022). Relationships between climate change, phenology, edaphic factors, and net primary productivity across the Tibetan Plateau. Int. J. Appl. Earth Observ. Geoinform. 107:102708. doi: 10.1016/j.jag.2022.102708
Tercero, M. D., Alvarez-Rogel, J., Conesa, H. M., Parraga-Aguado, I., and Gonzalez-Alcaraz, M. N. (2017). Phosphorus retention and fractionation in an eutrophic wetland: A one-year mesocosms experiment under fluctuating flooding conditions. J. Environ. Manag. 190, 197–207. doi: 10.1016/j.jenvman.2016.12.060
Thomaz, S. M. (2021). Ecosystem services provided by freshwater macrophytes. Hydrobiologia 2021, 1–21. doi: 10.1007/s10750-021-04739-y
Tong, L. L., Mao, X. F., Song, X. H., Wei, X. Y., Tang, W. J., Deng, Y. F., et al. (2022). PSR-BP neural network-based health assessment of the Huangshui Plateau Urban Wetlands in China. Front. Ecol. Evol. 10:866597. doi: 10.3389/fevo.2022.866597
Tum, M., Zeidler, J. N., Gunther, K. P., and Esch, T. (2016). Global NPP and straw bioenergy trends for 2000-2014. Biomass Bioenergy 90, 230–236. doi: 10.1016/j.biombioe.2016.03.040
Vivian, L. M., Godfree, R. C., Colloff, M. J., Mayence, C. E., and Marshall, D. J. (2014). Wetland plant growth under contrasting water regimes associated with river regulation and drought: implications for environmental water management. Plant Ecol. 215, 997–1011. doi: 10.1007/s11258-014-0357-4
Wan, S., Hui, D., Wallace, L., and Luo, Y. (2005). Direct and indirect effects of experimental warming on ecosystem carbon processes in a tallgrass prairie. Glob. Biogeochem. Cycles 19:GB2014. doi: 10.1029/2004GB002315
Wang, F., Gao, Y. G., and Bai, M. Q. (2011). Impact of climate change on natural vegetation net primary productivity in Qixing River Wetland ecosystem from 1961-2008. Chin. Agric. Sci. Bull. 27, 257–262.
Wang, J. F., Li, X. H., Christakos, G., Liao, Y. L., Zhang, T., Gu, X., et al. (2010). Geographical detectors-based health risk assessment and its application in the neural tube defects study of the Heshun Region, China. Int. J. Geogr. Inf. Sci. 24, 107–127. doi: 10.1080/13658810802443457
Wang, Y. J., Shen, X. J., Jiang, M., Tong, S. Z., and Lu, X. G. (2022). Daytime and nighttime temperatures exert different effects on vegetation net primary productivity of marshes in the western Songnen Plain. Ecol. Indic. 137:108789. doi: 10.1016/j.ecolind.2022.108789
Wang, Z. M., Guo, Z. X., Song, K. S., Liu, D. W., Zhang, B., Zhang, S. Q., et al. (2009). Effects of land use/cover change on net primary productivity of Sanjiang Plain, during 2000-2005. J. Nat. Resour. 24, 136–146.
Wang, Z. M., Mao, D. H., Li, L., Jia, M. M., Dong, Z. Y., Miao, Z. H., et al. (2015). Quantifying changes in multiple ecosystem services during 1992-2012 in the Sanjiang Plain of China. Sci. Total Environ. 514, 119–130. doi: 10.1016/j.scitotenv.2015.01.007
Xiao, D. R., Deng, L., Kim, D. G., Huang, C. B., and Tian, K. (2019). Carbon budgets of wetland ecosystems in China. Glob. Change Biol. 25, 2061–2076. doi: 10.1111/gcb.14621
Yan, F. Q. (2017). Spatial and temporal variation of net primary productivity of the Sanjiang plain and its influencing factors doctor. Beijing: University of Chinese Academy of Sciences.
Yan, F. Q. (2020). Large-scale marsh loss reconstructed from satellite data in the small Sanjiang plain since 1965: Process, pattern and driving force. Sensors 20:1036. doi: 10.3390/s20041036
Yan, F. Q., and Zhang, S. W. (2019). Ecosystem service decline in response to wetland loss in the Sanjiang Plain, Northeast China. Ecol. Eng. 130, 117–121. doi: 10.1016/j.ecoleng.2019.02.009
Yin, S. Y., Wu, W. J., Zhao, X. J., Gong, C., Li, X. W., and Zhang, L. (2020). Understanding spatiotemporal patterns of global forest NPP using a data-driven method based on GEE. PLoS One 15:e0230098. doi: 10.1371/journal.pone.0230098
Yu, D., Shao, H., Shi, P., Zhu, W., and Pan, Y. (2009). How does the conversion of land cover to urban use affect net primary productivity? A case study in Shenzhen city, China. Agric. Forest Meteorol. 149, 2054–2060. doi: 10.1016/j.agrformet.2009.07.012
Zarei, A., Chemura, A., Gleixner, S., and Hoff, H. (2021). Evaluating the grassland NPP dynamics in response to climate change in Tanzania. Ecol. Indic. 125:107600. doi: 10.1016/j.ecolind.2021.107600
Zhang, X. L., and Zhao, Y. (2018). Identification of the driving factors’ influences on regional energy-related carbon emissions in China based on geographical detector method. Environ. Sci. Pollut. Res. 25, 9626–9635. doi: 10.1007/s11356-018-1237-6
Zhang, Z. S., Craft, C. B., Xue, Z. S., Tong, S. Z., and Lu, X. G. (2016). Regulating effects of climate, net primary productivity, and nitrogen on carbon sequestration rates in temperate wetlands, Northeast China. Ecol. Indic. 70, 114–124. doi: 10.1016/j.ecolind.2016.05.041
Zhao, Y. J., Deng, Q. Y., Lin, Q., Zeng, C. Y., and Zhong, C. (2020b). Cadmium source identification in soils and high-risk regions predicted by geographical detector method. Environ. Pollut. 263:114338. doi: 10.1016/j.envpol.2020.114338
Zhao, J., Cohen, J. B., Chen, Y. T., Cui, W. H., Cao, Q. Q., Yang, T. F., et al. (2020a). High-resolution spatiotemporal patterns of China’s FFCO2 emissions under the impact of LUCC from 2000 to 2015. Environ. Res. Lett. 15:044007. doi: 10.1088/1748-9326/ab6edc
Zhou, Y. Y., Yue, D. X., Li, C., Mu, X. L., and Guo, J. J. (2021). Identifying the spatial drivers of net primary productivity: A case study in the Bailong River Basin, China. Glob. Ecol. Conserv. 28:e01685. doi: 10.1016/j.gecco.2021.e01685
Keywords: net primary productivity, marsh, the Sanjiang Plain, climate change, geographical detection method
Citation: Yan F (2022) Effects of climate changes on net primary productivity variation in the marsh area of the Sanjiang Plain. Front. Ecol. Evol. 10:1002397. doi: 10.3389/fevo.2022.1002397
Received: 25 July 2022; Accepted: 24 August 2022;
Published: 20 September 2022.
Edited by:
Chuanyu Gao, Northeast Institute of Geography and Agroecology (CAS), ChinaReviewed by:
Rongshuo Cai, Third Institute of Oceanography, State Oceanic Administration, ChinaXiangrui Meng, Changchun Normal University, China
Bing Zhang, Tianjin Normal University, China
Copyright © 2022 Yan. This is an open-access article distributed under the terms of the Creative Commons Attribution License (CC BY). The use, distribution or reproduction in other forums is permitted, provided the original author(s) and the copyright owner(s) are credited and that the original publication in this journal is cited, in accordance with accepted academic practice. No use, distribution or reproduction is permitted which does not comply with these terms.
*Correspondence: Fengqin Yan, eWFuZnFAbHJlaXMuYWMuY24=