- 1UMR "Ecologie et Dynamique des Systèmes Anthropisés" (EDYSAN, UMR 7058 CNRS), Université de Picardie Jules Verne, Amiens, France
- 2Dipartimento di Scienze AgroAlimentari, Ambientali e Animali, University of Udine, Udine, Italy
- 3Departament de Genètica, Microbiologia i Estadística, Universitat de Barcelona, Barcelona, Spain
- 4Institut Català de Recerca I Estudis Avançats (ICREA), Barcelona, Spain
In this review essay, we give a detailed synopsis of the twelve contributions which are collected in a Special Issue in Frontiers Ecology and Evolution, based on the research topic “Current Thoughts on the Brain-Computer Analogy—All Metaphors Are Wrong, But Some Are Useful.” The synopsis is complemented by a graphical summary, a matrix which links articles to selected concepts. As first identified by Turing, all authors in this Special Issue recognize semantics as a crucial concern in the brain-computer analogy debate, and consequently address a number of such issues. What is missing, we believe, is the distinction between metaphor and analogy, which we reevaluate, describe in some detail, and offer a definition for the latter. To enrich the debate, we also deem necessary to develop on the evolutionary theories of the brain, of which we provide an overview. This article closes with thoughts on creativity in Science, for we concur with the stance that metaphors and analogies, and their esthetic impact, are essential to the creative process, be it in Sciences as well as in Arts.
1. Introduction
Drawing comparisons between Brains and Computers has been a long intellectual exercise carried out by Philosophers, Psychologists, Mathematicians, Physicists, Computer Scientists and Neuroscientists. While some authors have suggested that this is a vacuous discussion (they assume that brains are “obviously” computers), others believe that there are instances in the functioning of both systems that do not allow this easy jump to conclusions, meriting further analysis. Some of the confusions come from the fact that, according to some authors many researchers do not understand the fact that behaving intelligently does not mean being just an information processor (because computers seem to behave intelligently using processors it does not mean that “intelligence” and “information processing” are equivalent; opinion articulated by psychologist Robert Epstein assay “The empty brain” 2016). Others, however, think that the assignment of a name such as “computational system” to the brain is limiting and biases the way in which we see brain processes occurring, e.g., consciousness, awareness, or simply “making sense of the world.” In this context, we think that re-visiting the Brain-Computer analogy is still a very valid endeavor. Indeed, based on the Research Topic “Current Thoughts on the Brain-Computer Analogy—All Metaphors Are Wrong, But Some Are Useful” this Special Issue gathers 12 articles that deal with these problematics, all showing that the subject (or the debate) is still very much alive.
1.1. The research topic
From the very beginning, it was clear to us that the project was to be constructed according to the architecture of a network, since the main concepts around which it was conceived were interconnected at various degrees. We reasoned that the structure of such a network would favor “information” to be exchanged among nodes (disciplines, approaches, articles). This useful approach allowed us to view our subject (brain) and the approaches taken to study it as two manifestations of a similar phenomenon, and the reticular interconnection of nodes (ideas, approaches or physical entities) as the graphical expression of these connections.
The topics and concepts that we saw as the “nodes of the network,” and which formed the foundation of the project, were the following: Conceptual points (Philosophy); Network Science; Complex systems (self-organization); Neural Networks and Computational Neuroscience (Artificial neural networks); Computer Science (distributed-centralized architectures; Church-Turing thesis; computational complexity); Information theory (reliability-error checking; efficiency-vs-speed of information; information asymmetry); Game theory (decentralized neural architecture; asymmetric information distribution); Quantum brain—quantum computer; Artificial Intelligence (AI) and Artificial Life; Experimental and theoretical Neuroscience; brain evolution (evo-devo).
The historical development of the field, lead to the “obvious” realization that knowledge derived from Network Science (e.g., work by A-L. Barabasi, MEJ Newman, DJ Watts, and others) could contribute to understand how the brain works, interacts, manages task flexibly, and the underlying involvement of synaptic distribution, density and strength. Moreover, it has become clearer over time that network evolution could shed light on the evolutionary history of neural network architecture (and its governing principles; see for instance, Sterling and Laughlin, 2017). The subject has been treated from diverse points of view, derived from the application of different intellectual approaches (cellular neuroscience, computational modeling, connectomic analysis, philosophy of neurosciences, etc.). These different approaches suggested alternatives views of the roles of networks in the functionality of the brain. Most of them would deal with the general problem of representation, though more recent developments have changed the focus on the flow of information (the routing). In this context, as we will see in one of the SI papers, the contribution by D. Graham identifies the mode(s) of function of the internet network as a new frame of reference to understand (aspects of) brain function.
Another essential issue related to network evolution we wanted to address in this SI was the role of self-organization and complex systems in shaping brain (any brain) architecture and its evolution (e.g., works by I. Prigogine and G. Nicolis, C. G. Langton, S. Kauffman, S. Kelso, P. Bak, S. H. Strogatz, C. Gershenson and F. Heylighen, R. Solé, and many others). Concepts such as “emergent properties” or “organizational levels” come to mind as relevant here.
Finally, being well aware that the relationships between Brain and Computer encompass a vast spectrum of topics from Natural Sciences, Mathematics, Computer Science, Psychology and Philosophy, we needed to narrow our scope: some of the topics we opted not to deal with were Consciousness, Behavior, Language, and Culture.
Before dealing with the articles presented in this SI (see Table 1), and what they contribute to the debate, we revisit some critical, and necessary, concepts/topics: machine(s), metaphor and analogy in science, and brain(s). In the following text, the references belonging to this Special Issue are identified by a (*) as a superscript of the year of publication.
1.2. Semantics: Concepts and definitions
“I PROPOSE to consider the question, ‘Can machines think?’ This should begin with definitions of the meaning of the terms ‘machine’ and ‘think’” (Turing, 1950). No doubt, Alan Turing had a clear understanding of the importance of semantics in this context. Likewise, all Authors and Editors of this Special Issue recognize semantics as a crucial concern in the brain-computer analogy debate.
Indeed, the authors identify a number of terms whose current definitions are “problematic,” and need therefore to be taken with caution. First and foremost, is the definition of “computer” (Brette, 2022*; Richards and Lillicrap, 2022*). And the list continues with “computing” and “recursion” (Danchin and Fenton, 2022*), “algorithm” (Brette, 2022*; Richards and Lillicrap, 2022*; Roli et al., 2022*), “computable function” (Richards and Lillicrap, 2022*), “robot,” “program,” and “software” (Bongard and Levin, 2021*), “information” (Cobb, 2021*; Gershenson, 2021*; Danchin and Fenton, 2022*), “Artificial Intelligence” (Roli et al., 2022*), “intelligence” (Gershenson, 2021*), “cognition” (Fraser et al., 2021*).
In the following, we are focusing on two specific, fundamental, issues: the definition of “machine,” and the distinction between “metaphor” and “analogy.” As for the former, in this Special Issue, Cobb mainly deals with images of the brain in history, and Bongard/Levin express their concern about an “outdated” view of term machine. In this paper, we follow the history of how “different kinds of machines” have best represented the brain. As for the latter, the distinction between metaphor and analogy is only touched on by Brette and Gomez-Marin. Consequently, we deem necessary to have a more detailed description of these concepts, which we deal with in turn, and offer a revised definition for the latter.
1.3. Machine(s)
Among neuroscientists there is a general opinion (sustained over decades of research) that what the brain “is” depends on how you study it. We live in a mechanical age, so we study it as a machine. In this context, we should question ourselves, upfront, what machines are and how our view of them has changed over time. In this SI, Cobb and Bongard/Levin introduce us to the ways we came to understand machines, in the past and nowadays. The underlying rationale for discussing “machines” is that the method of study has determined always what we have learned about them and how we have transferred these methodologies to study the brain.
For a long time, brains have been assimilated to certain kinds of “machines.” The idea can be traced back, for a solid articulation, to the Cartesian view of the World, understanding machines as any physical system capable of performing certain functions. Descartes’ body organs operate in purely mechanical fashion, and in this proposal, Descartes “creatively” adapted previous theories (Aristotle, Galen, etc.) to his own mechanistic program (Hatfield, 2012).
The form the machine analogy has taken over the years has suffered many transformations, adopting at every time the dominant mechanical view of the world (hydraulic, electrical or informational). In this SI, Cobb has revisited some of the historical views, with Bongard/Levin adding a perspective that includes recent developments in Artificial Intelligence. Interestingly, with the 20th century advancements in molecular biology, the machine analogy has been transferred from the whole tissue to the biochemical components that control its different functions. In this sense, the brain is equated to a soup/stew of highly coordinated chemical ingredients (molecular machines) that, ultimately, enable our rich psychological experiences. The whole field of neurochemistry, which foundations were laid in Europe, notably France and Germany, in the late 18th and early 19th centuries, with an important momentum gained in the 60 and 70’s of the 20th century (Boullerne et al., 2020) is based on the assumption that interrelating chemistry and function in the nervous system is a most productive avenue to understand the brain (e.g., Brady et al., 2011).
From a functional perspective, over time our view of the brain has been transformed from a rather passive, fluid conducting device, to a more active, information processing one (a device able to compute; calculate in the original meaning). The computer (originally a person able to “compute” operations) was, and is in good part, understood as a mechanical device with certain properties (ability to store, retrieve, and process data). At this stage, it is relevant to consider that in spite that the English word “computer” is meant to signify (programmable machine that can store, retrieve, and process data; Encyclopedia Britannica) the different Romanic languages retain the original meaning of computers as a person who either organizes or computes datasets (e.g., “ordinateur, ordenador,” in French and Spanish). In any case, we now universally use the term as meaning a device, usually electronic, that processes data according to a set of instructions. In this context, it is worth to remember that a more precise characterization of the computer was given early on by Mahoney in his historical review of computing in which the computer is being specifically defined as a fundamentally tripartite structure, which reflect the contributions of three historical disciplines concerned with the nature of this “machine/device” (electrical engineering; computer science and software engineering). Moreover, Mahoney clearly stated what those contributions were in the summary sentence: “between the mathematics that makes the device theoretically possible and the electronics that makes it practically feasible lies the programming that makes it intellectually, economically, and socially possible” (Mahoney, 1988).
Interestingly, and as a result of the inception of the information age (in the 1940’s), where information content and logical operations were introduced by logicians such as Alonzo Church and Alan Turing, the most salient analogy for the structure and function of the brain has been the computer, an instantiation of the so-called Turing machines (TM). The focus has changed from the instantiation of the machine to the underlying operative. In his seminal 1950 paper, Turing describes “machines” as those artifacts produced by “… every kind of engineering technique,” and suggests to identify them with “electronic computers” or “digital computers,” given the interest in his historical time in those devices (Turing, 1950). He gives a definition of computer as a finite state machine (a mathematical model of computation). An extended quote from Turing seems appropriate here.
"A digital computer can usually be regarded as consisting of three parts: (i) Store [of information] … corresponding to the paper used by a human computer … [and] … the book of rules ", (ii) Executive unit [carries out calculations], (iii) Control [handles the correct use of instructions]. … digital computers … fall within the class of discrete state machines. … This machine could be described abstractly as follows. The internal state of the machine (which is described by the position of the wheel) may be q1, q2 or q3. There is an input signal i0 or i1 (position of lever). The internal state at any moment is determined by the last state and input signal according to the table [of instructions]. … These are the machines which move by sudden jumps or clicks from one quite definite state to another. … the digital computer … must be programmed afresh for each new machine which it is desired to mimic. This special property of digital computers … is described by saying that they are universal machines."Needless to say, not all brain-computer metaphors require traditional TM or von Neumann architectures. We now have parallel or quantum computing, for instance, and these modalities have enriched our view of what computers can do (see Kerskens and Lopez-Perez, 2022, suggesting that our brains use quantum computation). However, one particularly persistent (and relevant here) view of computing and brain functions emphasizes the parallel architectures that both utilize, breaking up problems into smaller units that are executed by different components, all communicating through a shared memory. Thus, when comparing computers and brains, a common inference is that both systems, essentially, rely on parallel processors. This is not an accurate representation of the similarities, and for a number of reasons. (i) Brains and computers use different orders of magnitude (6 or 7) of independent (computing) units. (ii) While processors in a computer are “all purpose,” the human brain has specific areas specialized in processing different kinds of input. (iii) There are big differences in reliability and adaptability between brains and computers (a concept linked to that of “reprogrammability” in both systems), where brains information-processing systems are intrinsically “noisy” (Faisal et al., 2008) and this explains the differences in reliability and adaptability between them and the computers. (iv) Brains are fast at recognizing patterns from complex data, which (in many cases) are not possible by massive parallel computing systems (Hawkins and Blakeslee, 2004). These factors seem to suggest that parallel processing in the brain is never “truly” parallel and that reprograming in brains and computers rely on different network “reconfiguration” strategies (re-routing in machines versus neural plasticity in living systems). As the needs arise (e.g., “landscape modifications”), the adaptability of biological systems (e.g., brains) is a unique property derived from the plasticity of cell and circuit configurations, and a result of both genetic and epigenetic factors controlling birth, death and connectivity of brain neuronal sets.
In the following, we return to the processing system and provide an “accessible” description of a Turing machine (TM) that should be useful to understand the metaphor used for the brain. Briefly, a TM, or an “automatic machine” as Turing called it (Turing, 1937), is an abstract idealized model of a simple kind of digital computer. The machine input is a string of symbols each one of them carried by a single cell on a linear tape. The machine possesses some sort of read-write scanning head that considers one cell at a time. It is an automatic machine (i.e., at any given moment, its behavior is completely determined by the current state and symbol, the “configuration,” being scanned). It is a machine capable of a finite set of configurations. A finite set of rules (i.e., the program representing the algorithm) instructs the machine what to do in response to each symbol (i.e., erase, write, move left, move right, do not move). In principle, for any function that is computable (i.e., a function whose values may be computed by means of an algorithm), there is a TM capable of computing it. This logically implies the property of imitating another machine, meaning that there is a Universal Turing Machine (UTM) capable of simulating any other TM performing different tasks, by reading the corresponding set of rules from the tape. This is the theoretical model of a programmable computer (for more details on TM-UTM, see Gershenson, 2021*; Danchin and Fenton, 2022*; Richards and Lillicrap, 2022*).
Given the definition of a TM, it soon became clear that the brain (or mind) could be equated to a computational system very similar to a TM, and with many of the mental processes very similar to computations performed by a TM. Some authors consider that this identification of brains with TM is too strong, and thus, an adherence to it is called the “hard position.”
This “hard position” is being criticized by other authors saying that neither the principles, nor the materials or the way they are utilized (or organized) in a brain can be equated to a TM, except, perhaps, in the way both perform arithmetic operations (some authors deem the whole comparison “vacuous”; see Epstein, 2016). We are not going to delve on this problematic here, just want to stress the enormous influence that Turing machines have had, as computational neuroscientists have maintained (not all), for the last 80 years, in the view of brains as computer (working as a TM). This model was vindicated early on when neuroscientists realized that neurons were performing their physiological roles, firing action potentials, in a “all or none” fashion. This view was mostly promoted by Warren McCulloch and Walter Pitts in 1943, who also saw neural circuits in the brain as circuits of logical gates. Modern neuroscience has revealed more complex firing patterns, as well as complex patterns of firing regulation, adding nuances to the original McCulloch-Pitts view.
In the comparison between computers and brains, the semantics issue has often been raised, in one form or another. In particular, in the 80’s John Searle asked the question: “Can a machine ever be truly called intelligent?” (Searle, 1984). The question was encapsulated in the well-known “Chinese room” argument. It suggests that however well one programs a computer, nonetheless the machine does not understand Chinese; it only simulates that knowledge, and therefore this behavior cannot be equated with intelligence. Searle argues that his thought-experiment underscores the fact that computers merely use syntactic rules to manipulate strings of symbols, but have no understanding of their meaning. The issue of “meaning” is not further explored here, though we recognize its enormous interest. Searle’s main conclusion was that passing the “Turing Test” is inadequate as an answer (see also Cole, 2020).
All in all, in spite of the historical fortunes (and misfortunes) of the brain-computer analogy, the use of this “metaphor” is widespread, a testimony of which can be found in the different papers of this special issue. The subject remains fertile and open for further discussions.
1.4. Metaphor and analogy in science
The definitions of metaphor and analogy, at least in English dictionaries and encyclopedias, serve well to illustrate how muddled these concepts still are, in spite of the massive literature, in both Science and Humanities, devoted to them. A telling example comes from the Merriam-Webster in which metaphor is defined as “a figure of speech in which a word or phrase literally denoting one kind of object or idea is used in place of another to suggest a likeness or analogy between them.”
The definition of the two concepts has been “adapted” in different branches of human knowledge. Here we are only concerned with the meaning(s) of Metaphor and Analogy (M&A) in (western) scientific thought. As we will see in the following sections, in Science, and also in this SI, M&A are used as synonyms, yet they are not. Aware of this, in the title of this Research Topic (Current thoughts on the Brain-Computer analogy—All metaphors are wrong but some are useful) we intentionally, and provokingly, used metaphor and analogy as synonyms; and it is precisely in Science that the distinction is more conspicuous. This is the rationale for discussing in detail the issue in this section.
It is important to note, upfront, that some argue that metaphor and analogy have actually no place in science (for a discussion see Haack, 2019; Reynolds, 2022), though others claim that M&A are essential for scientific creativity (a position sustained in this article). According to Ziman “… scientific theories are unavoidably metaphorical” (Ziman, 2000), and it has been suggested that they are “the basis of our ability to extend the boundaries of human knowledge” (Yohan, 2012). Moreover, the aptitude for metaphorical and analogical reasoning is an essential part of human cognition. Undeniably, M&A have been a powerful way to communicate knowledge and consequently a powerful tool in education and learning. Just think of how many times we use metaphorical language to convey concepts to students in our own teaching experience (Kovac, 2003). Scientific M&A can guide scientific discovery, hypothesis and theory, and plays also an important role in adapting scientific language to the world. As Kuhn put it “Metaphors play an essential role in establishing links between scientific language and the world” but what is crucial (see also section 3) is that “… Those links are not, however, given once and for all” (Kuhn, 1993). Then choosing the “right” metaphor may be regarded as part of scientists/teacher work and ultimately becomes a form of art (Haack, 2019). In the following, we discuss M&A in more detail, given the relevance we assign to them in the context of discussing our current images of the brain.
1.5. Metaphor
The literature on metaphor is overwhelming and definitions abound. Robert R. Hoffman reasons that scientific metaphors appear in a variety of different forms and serve a variety of functions, and it makes a rather exhaustive list of them (Hoffman, 1985). One example for all, the “Tree of Knowledge.” In its various flavors over the centuries, it is certainly one of the founding metaphors of human civilization, not only of Science (Lima, 2014). And, to an evolutionary biologist (like the two authors of this paper), there is hardly a more fundamental metaphor than the Tree of Life, which Darwin, and Lamarck (1809) before him, used to illustrate his theory of descent with modification and depicted in his “Diagram of diverging taxa” (Darwin, 1859, pp. 116–117 in 6th edn). Indeed, the tree metaphor has been used in evolutionary biology ever since. However, and most notably, based on the regained awareness of the evolutionary impact of the phenomenon of gene flow between species (a.k.a. Horizontal/Lateral Gene Transfer) less than three decades ago, a new metaphor has emerged to account for the diversity of species: The Network of Life (Doolittle, 1999; Martin, 1999; Ragan, 2009). Incidentally, as an historical note, contrary to common knowledge, the network metaphor predates that of the branching tree. Indeed, it is dated 1750 and credited to Vitaliano Donati, whereas the first use of the tree metaphor is attributed to Pallas in 1776 (cited in Ragan, 2009). The example of the use of Trees and Networks in evolutionary biology is mentioned here specifically to emphasize that the two metaphors are complementary; we consider this position as pivotal in our review essay for both trees and networks have been specifically used in modeling our ideas of the brain and its evolution (see also section 3).
As to the definitions of metaphors in science, for the sake of brevity, we single out two of them (JC Maxwell, and Lakoff and Johnson) adding three illustrative examples for a better understanding.
James Clerk Maxwell wrote “The figure of speech or of thought by which we transfer the language and ideas of a familiar science to one with which we are less acquainted may be called Scientific Metaphor” (Maxwell, 1870). This would refer to a “logical semantics” view of metaphors, very much used in science and everyday life.
However, and in the classical definition by Lakoff and Johnson, the concept of “mapping” is also introduced. This leads them to state that “The essence of metaphor is understanding and experiencing one kind of thing in terms of another” (Lakoff and Johnson, 1980). And also introduce the different idea that the “Metaphor is the main mechanism through which we comprehend abstract concepts and perform abstract reasoning … Metaphors are mappings across conceptual domains” (Lakoff, 1993a). Hence, metaphors “become” conceptual tools (aids in understanding). Therefore, as Humar put it “A metaphor links two domains by mapping attributes from one onto the other. Thus, metaphor is an act of transferring … [where] … the key terms, ‘target’ and ‘source’, were introduced by Lakoff and Johnson … For instance, the biological metaphor ‘genes are text’ links the source ‘text’ and the target ‘genes’” (Humar, 2021). Black (1962) points out, in this context, how similar is the “standardized” Oxford English Dictionary (OED)1 description of metaphor to the one described above: “The figure of speech in which a name or descriptive term is transferred to some object different from, but analogous to, that to which it is properly applicable; an instance of this, a metaphorical expression”.
Interestingly, the etymology of the term “metaphor” originates from the ancient Greek noun “metaphora” (μεταφορά), which is derived from the verb “metapherein” (μεταφέρειν), originally meaning “to transfer,” “to transform.” Or else, derived from μετα (over, beyond) and πηερειν (to carry). It all depends on what we mean by “transfer” or “carry beyond” in the above definitions; more precisely we may ask: What is being transferred?
Before delving into the next relevant concept of analogy, we need consider another rather problematic term which is very often linked or likened to metaphor: the concept of “model.” Often, in the scientific literature there is no clear distinction between model and metaphor. In fact, we think that a distinction needs be made, for clarity. Contrary to a metaphor, a model (a conceptual model) has for us a narrower scope and, being a hypothetical representation of a system, it aims at simulating and understanding reality (e.g., a biological model; see also Ziman, 2000). Moreover, “… a model is, in its etymological and technical sense, a substantive thing which is the best or ideal representative of something else. All other uses of the word “model” are metaphorical extensions of this basic meaning” (Hoffman, 1985). Therefore, we see models as methods or representations aimed to understand, and predict, specific patterns.
To complete this section, we would like to propose a “concept” of metaphor that does not require, but accepts, the use of the mapping concept (but see section 1.6, below). The type of metaphor we have in mind is founded on a visual perception. It is the visual image that is the driver for scientific insight and provides educational power. As an example, we identify three metaphors that best illustrate this idea: Adaptive Landscapes by Wright (1931), Epigenetic Landscapes by Waddington (1957), and the Gene Regulatory Network by Davidson and Peter (2015). For a recent, and more extensive, discussion of the use of metaphors in science, with its dangers and pitfalls, we refer to the excellent new book by Reynolds (2022).
1.6. Analogy
Metaphors may be a source for “analogies” (and “similarities”) and may guide building models. Among the definitions of analogy given in the OED there are the following: (a) A comparison between one thing and another, typically for the purpose of explanation or clarification; (b) Biology: The resemblance of function between organs that have a different evolutionary origin. Our focus here is on the first, more general, definition.
Atran (1990) traces back the concept to Aristotle and his effort to compare structures and functions between man, other animals and plants; “… Aristotelian life-forms are distinguished and related through possession of analogous organs of the same essential functions.” Along the same line, the concept of “analogue” was introduced in comparative anatomy in 1843 and defined as “a part or organ in one animal which has the same function as another part or organ in a different animal” (Owen, 1843, p. 374). Atran brings us to a more generalized version of the analogy concept, also mentions the Newton’s concept of “Analogy of Nature” (ibid p. 232) and points out that this analogy “… combines two older ideas: the theological “Chain of Being” through which Nature seeks Divine Perfection, and the unity of causal pattern in the macrocosm and the microcosm.”
But it is in her classic book that Mary Hesse describes in considerable detail both scientific models and analogies (Hesse, 1970). A dialog is imagined between two men of science: Campbellian, who argues that analogies and “models in some sense are essential to the logic of scientific theories” and Duhemist, who denies it. Campbellian identifies three types of analogies: positive, negative and neutral. Two physical objects or systems have positive analogy based on their shared “properties”: “Take, for example, the earth and the moon. Both are large, solid, opaque, spherical bodies, receiving heat and light from the sun, …” yet, the same objects may differ in a number of respects: “On the other hand, the moon is smaller than the earth, more volcanic, and has no atmosphere and no water … there is negative analogy between them.” Neutral analogies are “properties of the model about which we do not yet know whether they are positive or negative analogies.” Note that Campbellian too is concerned with semantics: “But first let us agree on the sense in which we are using the word model.” Thus Hesse tells us that analogies can have specific “values”: positive, negative or neutral.
Humar has posed a dichotomy between “structural metaphors,” such as those described above and functional ones. In fact, “functional metaphors … draw attention to a similarity in function between a source and a target are also found in ancient scientific literature” (2021). And again, Gentner and Jezioreski (1993) contend that an underlying idea pervades the use of any concept of analogy “The central idea is that an analogy is a mapping of knowledge from one domain (the base) into another (the target) such that a system of relations that holds among the base objects also holds among the target objects. In interpreting an analogy, people seek to put the objects of the base in one-to-one correspondence with the objects of the target so as to obtain the maximal structural match.” More so, broadly speaking, Hoffman sees the distinction between the two concepts as a chicken-and-egg problem, analogy regarded as the “psychological egg” and metaphor the “chicken” (Hoffman, 1985, p. 348).
Finally, we suggest the use of two criteria, the structural and the functional in the very definition of analogy (in science), and indicate the latter as its most characterizing property. In doing so, we do link this definition of analogy with the Lakoff-Johnson definition of metaphor and its associated action of “transfer.” We think this definition of analogy is more pertinent (of practical importance) here because metaphors are not intended to provide a solution to a given problem, they have no explanatory power. In contrast, analogies do have an explanatory power, and enable to make connections to understand the structure/function of a given system based on the knowledge acquired on another system. A telling example for the “explanatory role” of analogy is the transfer of themata [sensu Holton, in Ziman (2000)] between different disciplines—for example, the notion of a “code” from information theory to molecular genetics.
1.7. Brain(s)
A key concept in this Special Issue is obviously that of a Brain, but how to define one? The brain, defined in simple terms, according to the Encyclopedia Britannica is: “the mass of nerve tissue in the anterior end of an organism.” The brain integrates sensory information and directs motor responses. While this mostly represent the vertebrate condition, the substitution of nerves by neurons would be still a valid assertion. Brains as centralized structures are old, dating back to the origin of bilaterian animals in the Ediacaran Period (571 to 539 million years ago; Martinez and Sprecher, 2020). The coalescence of neurons in a pole of the larvae/animal allows a better, centralized, coordination of functions, and in that sense, brains have been also equated to “central processing units” (CPUs). How centralization has happened and the conditions that drove their appearance have been discussed before (see Martinez and Sprecher, 2020) and do not need a further discussion here.
Our ideas of the brain have changed radically over the centuries, mainly due to the lack of proper understanding of their physical constituents and the modes of functioning. Explanations have used the current metaphors that conformed the mechanical world at every age (see Cobb’s historical account in this SI). Most recently, and with the instantiation of computing devices and the rise of the information age, computing and information processing have been our reference mark when thinking about brains and their activities. The current view originated early in the 20th century, when the brain tissue was systematically analyzed under the microscope. The presence of isolated cells organized as neural nets contributed to the view of the brain as a “machine” dedicated to compute and process information.
The intricate nature of brain connections (neurons and substructures) suggested the possibility that the brain is actually a connected set of wires, with complex architectures (see Cobb, 2020). Moreover, the discovery of chemical and electrical connections between neurons reinforced the image of a giant electrical device with multiple, complex, switching mechanisms. It is the emergence of the information age, with the first devices able to “compute” operations, that led to a new model of the brain, which in addition to conducting electrical impulses, was assimilated to a complex computing device.
The integrative model of the neurons, with empirical data and modeling processes, was developed by pioneer cyberneticians/neurophysiologists Warren McCulloch and mathematician Walter Pitts (among others). McCulloch’s brand of cybernetics used logic and mathematics to develop models of neural networks that embodied the functioning of the brain in the workings of the brain (Pitts and McCulloch, 1947). How accurate is this model? The question has been a subject of intense debate, to which some of the papers in this issue refer (e.g., Davis, 2021*; Fraser et al., 2021*). Ideas about how the information is processed, the speed of neuronal communication, the role of the neuron in integrating inputs, the routing of information and the correlation between firing patterns and brain activities (i.e., mental activities), have all contributed to the debate on the validity of using “computer” metaphors for understanding different aspects of neuroscience. The debate is alive today as it ever was.
At the base of our utilization of metaphors for specific organ systems is the consideration that the activities of the organ as properties “define” the realm (domain) and the contents of the metaphors. In this sense, brains are equated to computers because, at least according to some authors, they are actually performing “computing” operations (see Chirimuuta, 2022*). However, there is not a unified agreement on the use of this metaphor (others are explored in this SI by Gomez-Marin and Graham), and this has led to a heated debate on the meaningfulness of using some specific metaphors in neuroscience (see a later discussion in this paper).
One of the key issues discussed by many authors interested in modeling the brain and its functions revolves around the nature of information flow and how input signals are transformed into output behaviors, including the routing problem (see Graham, 2022*; in preparation)2. This is linked to the idea that our brain does not function as a linear processor in which the flowing streams of information, from input data to output realization (behavior) are not unidirectional, a “one-way street.” Instead, many authors consider that the output of the brain’s processing is the result of some “emergent properties” not linearly derived from the original inputs, properties that are not “just” the result of simple operations (addition/subtraction) of inputs. Some of the problems not solved by the different physical models of the brain are linked to the capacities for self-reference in human brains, or more generally the awareness of our own existence (consciousness). These problems are not easily dispatched by models of emergence, and a proof of the complexity that self-reference models have in computer science is shown by Fraser et al. in this SI. Once more, mathematical descriptions and observable reality are not easy to compare.
2. The 12 articles in the special issue
In this section, we present our own summaries of the 12 articles (see Table 1) in this Special Issue (SI), each of which (but one) has been endorsed by the corresponding author(s).
In Figure 1 we propose a graphical picture, a sort of a snapshot of the entire Special Issue, based on 20 concepts (keywords) we have arbitrarily selected. It is a kind of [0,1] matrix in which the presence of those concepts in a given paper is denoted by a dot. The usefulness of such a representation is self-explanatory. In the following, article summaries are listed by Authors’ names (and number in Figure 1).
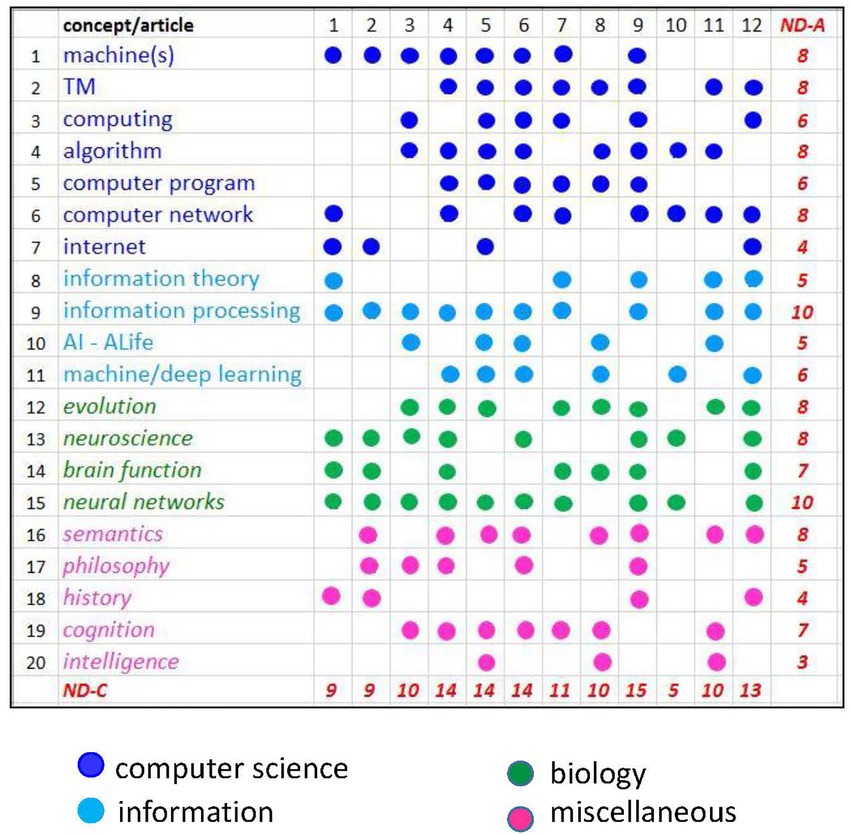
Figure 1. The Special Issue at a glance. The figure is a kind of [0,1] matrix that describes the 12 articles in this Special Issue by means of 20 concepts (keywords) whose presence is denoted by a dot. The external column shows the number of Articles per concept (ND-A), the external row the number of Concepts per articles [ND-C; i.e., the Node Degree (ND), the number of links per node, in the corresponding bipartite graph, not shown]. Articles are as follows: Cobb (1), Gomez-Marin (2), Chirimuuta (3), Brette (4), Bongard-Levin (5), Richards-Lillicrap (6), Fraser (7), Roli-Jaeger-Kauffman (8), Danchin-Fenton (9), Davis (10), Gershenson (11), Graham (12). Abbreviations: TM-Turing machine; AI-Artificial Intelligence; ALife-Artificial Life.
We have chosen as the opening article of this Special Issue Matthew Cobb’s historical account of the metaphors used over the centuries to describe the brain and try to understand its functioning.
2.1. Cobb (1)
In the opening article of this Special Issue, Matthew Cobb, the author of the excellent book "The Idea of the Brain" (2020), provides a detailed and instructive history of the “wiring diagram” metaphor of the brain and explores its role, together with that of its associated metaphors, on the conception of the brain over the last two centuries.
His historical account of the use of metaphors for brain functioning starts in the 18th century stemming from mechanics, and the discovery of electricity (telegraph). Cobb identifies a drastic shift toward the end of 19th century with the appearance of " … the telephone exchange, where messages can be flexibly routed." In the 20th century" … two kinds of wiring diagram – that of the animal body and that of the computer – entered into dialogue" (McCulloch and Pitts, 1943; von Neumann, 1958).
In the 21st century, the connectomic projects, which are aimed at a complete description of the structural connectivity of the central nervous system, became prominent, in many respects. Cobb criticizes these approaches mainly because they produce a static representations of the nervous system. He thinks that we should proceed from small circuits (controlling specific behaviors) to the whole map of neuronal connections, and gives the example of the lobster’s stomach, whose processes are controlled by a few neurons, which has been studied (excruciatingly) for a long time, and for which we still do not have a full understanding. Moreover, quite rightly, Cobb points out that knowing the genome sequence cannot by itself explain the “functioning” of the corresponding organism, likewise " … the wiring diagram itself could not explain the workings of the human mind."
Cobb mentions what is regarded as the most recent metaphor for brain function “cloud computing or the internet.” On the one hand he acknowledges that " … it embodies plasticity and distributed function into our conception of the brain," on the other hand, he points out its limits if the notion of robustness is taken into account" … the internet is designed to function even if key parts it removed, whereas some aspects of brain function can be decisively disrupted if particular areas are damaged." Interestingly, the internet metaphor will be explored in great detail by David Graham, in the last contribution of this Special Issue.
As a cautionary note, Cobb warns us about the limits of the use of these metaphors to study brain function, mainly " … because of the plasticity and distributed function of most nervous systems." The notion of brain plasticity is central to this Special Issue and alludes to the plasticity that nervous systems shown in individuals during their lives and linked to the processes of learning and memory acquisition.
The next three articles deal with conceptual issues, and are written by A. Gomez-Marin, M. Chirimuuta, and R. Brette. They tackle the problem of whether there is any foundation for the comparison between brains and computers. In different ways, they do that by interrogating the interrelated questions of what is a computer, how it can be characterized and the limitations that these characterizations, and their associated metaphors, have in our current understanding of both brains and computers.
2.2. Gomez-Marin (2)
Gomez-Marin introduces us to the well-known book "Metaphors we live by" (1980), authored by the cognitive scientists George P. Lakoff and Mark Johnson. In this seminal work, the authors provide a detailed analysis of the nature of metaphors, suggesting that metaphors, which were once known as mere “linguistic devices” (semantics), are mostly “conceptual constructions” that shape the way we think and act. In a sense, as Gomez Marin points out, the semantic role for metaphors is secondary to their conceptual (cognitive) nature. Following Lakoff (1993b), metaphor mapping (from one conceptual domain to another) would occur independently of their linguistic expressions, so there is a priority status given to their cognitive function, over those expressed in language terms. Or put it another way: the conceptual structure of metaphor is given more weight than the structure of metaphoric language.
In this context, Gomez-Marin revisits the analogy of computers and brains. After a brief historical overview of the most pervasive ways in which brains and computers have been visualized, Gomez-Marin draws our attention to the lesser-known images of the brain such as holograms and radio sets. The latter suggests the intriguing possibility that “brains would not create thoughts but receive and filter them.”
Gomez-Marin summarizes his appraisal of metaphors with the advice that we apply them as pragmatic tools with the proviso that we should be always vigilant to avoid what he calls falling into a “metaphorical monoculture”, which would become “a burden” rather than “a blessing.”
2.3. Chirimuuta (3)
In a suggestive parallel, Chirimuuta comments on the assertion by different authors that the brain and computers (or any other complex artifact) could be made tractable by using multi-level approaches. These approaches use top down, functional characterizations of systems to compliment bottom up reductionist strategies. The important assumption is that the brain decomposes into relatively autonomous levels of organization, similar to the hardware-software distinction in computing.
However, as appealing the simile can be, Chirimuuta contends that several limitations need to be accounted for. (1) Low-level components (neurons in the brain) are not mere “hardware implementors” in brain information processing. In computers, the elements maintaining the physical integrity of the machine and the components performing information processing are different. Whether this separation occurs in the brain is far from clear. (2) Computers and artifacts are assembled differently. While computers are designed to ensure that high level functionality is relatively independent of variations in hardware the functionality of the brain may well depend on low level details often assumed to be irrelevant to cognition. Interestingly, the two alternatives are, again, assumed to be the products of two constructive methods: engineering, in the case of computers/artifacts, and evolution, in the case of brains. Chirimuuta, however, is concerned about oversimplifying the principles that govern the construction and functionality of complex biological systems, such as the brain.
2.4. Brette (4)
The question central to Brette and Richards/Lillicrap (see below) is a semantic one, they ask "What is a computer?" Brette states that both in common and technical usage a “computer” is thought of as a “programmable machine.” Then, while pointing out that in computer science there is no formal definition of computer, he draws our attention on the concept of “program” defined as " … a set of explicit instructions that fully specify the behavior of the system in advance (“pro-”, before; “-gram”, write)."
Moreover, and quite appropriately, Brette discuss the notions of algorithm and computation in the brain. At this point, two deep questions, both from evolutionary and philosophical perspectives: "what is a brain program"? and "who gets to "program" the brain?" The reasoning those entail, seem to lead to a logical consequence " … The brain might not be a computer, because it is not literally programmable." Offering a definition of metaphor and analogy, Brette concludes that the brain-computer metaphor seems to be of little use, if not misleading, for it provides, according to the author, a reductionist view of cognition and behavior. This conclusion contrasts sharply with that of Richards and Lillicrap.
The next contributions deal with different problematics arising from the brain-computer comparisons; whether these are semantic misunderstandings (formal definitions in the field) or with misleading assumptions of what a computer or a brain can do. The papers by Bongard and Levin and Richards and Lillicrap deal with a fundamental problem that affect all definitions. The definitions of concepts bear very much the stamp of the fields in which they are generated (e.g., computer science, engineering or neurobiology). This straightjacket affects the way we conceive the possibilities of what a computer or a brain can do. Revised versions of those concepts should liberate the concepts from the “semantic constrains” that those fields have imposed in them. Here, brain, computer and machines are the three examples analyzed in detail. In the following three papers, authored by Fraser et al., Roli et al., and Danchin and Fenton the subject of software (the running of algorithms) and how brains and computers deal with processing information is clearly put. All authors discuss the idea of to what extent Artificial Intelligence should be able to reproduce behaviors of living organisms. Irrespective of the general optimism in the possibilities of Artificial Intelligence, these authors introduce some cautionary notes; which cast some doubts on the real possibilities of “imitating,” for instance, human behaviors. “Agency” and “self-reference” become clear stumbling blocks. One last paper in this section, authored by Davis, suggests a series of questions posed by the analogy, asking himself (and the people in the field) to what extend they have been answered and what the answers would add to the debate.
2.5. Bongard and Levin (5)
It has been assumed for a long time that life and machines are fundamentally different entities, and that the former can’t be reduced to the latter (see Nicholson, 2013). Bongard and Levin contend that this dichotomy is mostly based on an old conception of machine, a 17th to 19th century vision that doesn’t account for modern development in disciplines such as Artificial Intelligence, Bioengineering, etc. In this context the authors re-visit the problem and ask: “does a suitable machine metaphor apply sufficiently to biology to facilitate experimental and conceptual progress?.” The path toward understanding this goes from a clear definition of what a machine is, and the properties characterize them, to a critical appraisal of what modern science and technology tells us about those properties. Do these properties are clearly demarcated between alive (or evolved) and engineered “things”? In view of modern developments in the above-mentioned sciences it becomes harder and harder to sustain a clear separation between these two “systems”, with borders becoming more fluid as modern engineering progresses. The authors emphasize the fact that the analysis of properties once associated to the living beings in newly developed machines clearly show that the boundaries between those, once considered unmistakably different systems, are nowadays becoming blurred. Several, and very detailed, examples are provided. At the end they try to provide a new working definition of machine that can accommodate all of our newly gained insights.
2.6. Richards and Lillicrap (6)
Richards and Lillicrap emphasize the fact the word "computer" is given different definitions in different disciplines, and specifically they contrast the definition used in computer science with the one used by academics outside computer science. According to this argument, much of the debate about the brain-computer metaphor would be just a matter of semantic disagreement. End of the debate. Is it so?
While the common usage of "computer" is straightforward—"human-made devices (laptops, smartphones, etc) that engage in sequential processing of inputs to produce outputs"—this is certainly not so for the notion in computer science. The authors carry out an in depth analysis of the notion of "computer" in computer science, definition based on two other notions, those of "algorithm" and "computable function." An "algorithm" can be informally defined as a sequence of finite logical steps that mechanically lead to the solving of a problem. A “computable function” is "any function whose values can be determined using an algorithm." The formal definition of algorithm was developed independently by mathematicians Alan Turing and Alonzo Church in 1936–19837, and the authors introduce the Church-Turing Thesis.
The authors provide a formal definition of “computer”: a "physical machinery that can implement algorithms in order to solve computable functions." They stress that this definition "is important because it underpins work in computational neuroscience and AI." The authors also describe the applications of this definition to brains and discuss its limits.
In sum, Richards and Lillicrap invite us to contrast the two definition of "computer," inside and outside computer science. If one adopts the definition from computer science "one can … simply ask, what type of computer is the brain?" However, " … if one adopts the definition from outside of computer science then brains are not computers, and arguably, computers are a very poor metaphor for brains." End of the debate?
2.7. Fraser et al. (7)
One of the properties associated to (human) brains is the capacity of being conscious of its own existence. This seems an obvious difference to any other standard machine, including computers. Fraser and collaborators (see Fraser et al., this issue) make a good case for this, by appealing to self-reference statements, which cannot be resolved by computers. The case is clearly stated: verbal statements like “the sentence presently being uttered is false” are being “understood” by our brains. A computer presented with it, however, enters into an “endless loop,” with no resolution. How do brains deal with the above paradox? Fraser et al. present an elegant dynamical model, in which brains are composed of interacting units (modules) moving through time (the strange loop model). The model suggests a way the brain has for dealing/resolving the paradox, and this is by extending the analysis of the inconsistency over time (deconvoluting it along this axis). Temporalizing the problem, the brain is able to cope with the paradox. This avoids the system (the brain) to enter into the endless loops that would characterize the response of a computer.
2.8. Roli et al. (8)
Roli, Jaeger and Kauffman put the focus on the fields of Artificial Intelligence (AI) and Artificial Life (Alife). The notions of Natural Intelligence and Artificial General Intelligence (AGI) are contrasted, the latter being defined as "the ability of combining 'analytic, creative and practical intelligence'." The ultimate goal of AI and ALife would be to create a computational or mechanical system (an AI-ALife-agent; e.g., a robot) able to autonomously (i.e., without human intervention) identify, appraise and exploit new alternative opportunities (dubbed affordances) so that to evolve and innovate in ways equivalent to a natural organism (autonomous Bio-agent). Affordances are here defined as "opportunities or impediments on [a] path to attain a goal." The authors argue that AI-and ALife-agents cannot "evolve and innovate in ways equivalent to natural evolution" for current AI algorithms do not allow such capability (broadly dubbed “agency”) since they cannot transcend their predefined space of possibilities (determined by the human designer). Moreover, they show that the term “agency” refers to radically different notions in biology and AI research.
As possible objections to their position, the authors mention (i) deep-learning algorithms and (ii) unpredictability of AI systems (e.g., playing chess, composing music); they address and dismiss both.
Finally, citing the work of William Byers and Roger Penrose, the authors distinguish the capabilities of Natural and Artificial intelligence using the notion of creativity in mathematics, creativity "which does not come out of algorithmic thought but via insight," which is not formal and involves shifting frames.
2.9. Danchin and Fenton (9)
Danchin and Fenton's paper correlates notions from neurobiology and computer science. Parallels are also drawn between computing, genomics and species evolution. The focal point of the paper, made explicit in the title, is built around the concept of Turing Machine (TM); an entire section of the paper is devoted to it. Noteworthy, the TM concept is also transposed into biology.
In order to explore the potential analogies between brain and computer, an issue of semantics is addressed first: the definition of computing. The differences between analog and digital computing are contrasted, with emphasis on the fact that analog computation implements of a variety of feedback and feedforward loops, whereas digital algorithms make use of recursive processes. Recursion is a central concept in this essay; it is a characteristic feature of the digital world of a TM. Recursion allows one of the steps of a procedure (e.g., set of rules of the TM machine) to invoke the procedure itself. "A mechanical device is usually both deterministic and predictable, while computation involving recursion is deterministic but not necessarily predictable." The brain does certainly some sort of computation, and "with remarkable efficiency, but this calculation is based on a network organisation made up of cells organised in superimposed layers, which gives particular importance to the surface/volume ratio … This computation belongs to the family of analog computation" (A. Danchin).
In an extremely useful Table, the key features of a Turing Machine, a digital computer, and the human brain are compared. The authors conclude that brains are not digital computers. However, they speculate that the recent (in evolutionary time) invention of language in human history, and writing in particular (maybe around 6,000 years ago), might constitute a step toward the evolution of "the brain into a genuine (slow) Turing machine."
2.10. Davis (10)
Davis' short paper suggest a range of questions that bear into our use of brain computer analogies. His focus is on the programing of brain processes. What kind of algorithms use the brain to navigate the world? Would computers be able to simulate those? Davis suggest that modern use of optimization algorithms (network training) should provide an avenue, improving over the longer (older) numerical computations. He ends up by posing a provocative hypothesis: consciousness could play the role of an “interface to the brain’s operating system.” Definitely, questions that still remain unresolved in the fields of computer and Artificial Intelligence.
The paper by Carlos Gershenson introduces a different perspective to this SI by bringing to the fore the notion of intelligence, and collective (swarm) intelligence in particular and by linking it to the theory of information processing.
2.11. Gershenson (11)
The main take of the MS is stated in its Title: the focus is on Intelligence that is studied in terms of information processing. This approach could be applied to brains (single and collective), and machines.
A major issue arises immediately: There is no agreed definition of intelligence; semantics again. Gershenson defines intelligence in terms of information processing: "An agent a can be described as intelligent if it transforms information … to increase its 'satisfaction' … Examples of goals are sustainability, survival, happiness, power, control, and understanding." In previous work, Gershenson suggested to use measures of information as a tool to study complexity, emergence, self-organization, homeostasis, and autopoiesis (Fernandez et al., 2014); here he aims to extend this approach to cognitive systems, including brains and computers.
Information, a new semantic challenge. Gershenson presents a definition of information quoting the classic work of Shannon. Our attention is drawn on the meaning of the message being transferred; in this context, the failure of Laplace daemon (and Leibniz mill for that matter) is instrumental in identifying a crucial, and much-overlooked notion (not only) in Biology: the existence of different scales, different frames of reference, which (ought to) modify the models and hypothesis for a given phenomenon. "Even with full information of the states of the components of a system, prediction is limited because interactions generate novel information.”
A stimulating comparison is offered between the intelligence of “the single brain” and the collective intelligence of swarms (groups of humans, animals, machines). In the case of insect swarms, which can be described as information processing systems, the processing is distributed. Gershenson compares the cognitive architectures of brains and swarms, and identifies a key feature distinguishing the two: "the speed and scalability of information processing of brains is much superior than that of swarms: neurons can interact in the scale of milliseconds, … insects interact in the scale of seconds, … [in practice,] this limits considerably the information processing capacities of swarms over brains."
Finally, “intelligence as information processing” is used as a metaphor to understand its evolution and ecology. The author's arguments about ecological (selective) pressures with respect to the evolution of intelligence and the complexity of ecosystems may be agreed or not.
In conclusion, while " … the brain as computer metaphor is not appropriate for studying collective intelligence in general, nor swarm intelligence in particular … " nonetheless since " … computation can be understood as the transformation of information (Gershenson, 2012), “computers”, broadly understood as machines that process information can be a useful metaphor … "
Graham extends the comparison of brains and computers by introducing a further element of complexity. It is not a computer that needs to be compared to a brain; in fact, the functioning of the latter is better represented by a collection of interconnected computers (internet). He points to a relevant issue that is not solved by the proponents those that advance the “strong” brain-computer analogy, and this is the problem of information routing (how the information flows within the brain and the computers; how is directed from the input site to the output resolution).
2.12. Graham (12)
Daniel Graham analyzes the appropriateness of the computer–brain metaphor (see also Graham, 2021); instantiated as what he calls the “representational” view of neural components. According to Graham, the analogy is useful but incomplete. Although he agrees that the brain performs some “computations”, he posits that brains themselves can be seen as the result of both representation and communication activities. The emphasis on pure mathematical operations in the brain, along with their translations in neuronal patterns of electrical spikes, does not provide a complete view of what happens inside brains as they perform tasks. One of the reasons for not supporting the strict computer–brain functional (representational) analogy is that it does not deal with the key problem of information flow within the neuronal nets of the brain. The routing of information and the remodeling of circuits transcend the limits of the computer analogy. Graham suggests the internet as a better image of our (or any) brain architecture and functional properties. The internet is constructed with clear routing protocols, with an efficient distribution of information (termed the small-network configuration, Sporns and Honey, 2006; but see also Hilgetag and Goulas, 2016 for a critical view) and the continuous remodeling of their connectivity (plus growth). These properties should remind us of the way our brains seem to be constructed and how they route information, from external/internal inputs to higher integrative circuits and on to motor systems. Integrating views of signal processing and routing strategies should give us a more nuanced view of the activities of brains.
3. Discussion
3.1. The brain-computer analogy: “Cum grano salis”
Much the same way as scientific hypotheses, metaphors and analogies are transitory, always adjusting to technological advances. The Brain-Computer is usually referred to as a metaphor, but it should be thought of as an analogy instead. Indeed, here we are suggesting that metaphor and Analogy are two distinct concepts and must not be used as synonyms (see also above). While we think that a salient feature of a metaphor is a “visual insight” (an evocative visual image), the concept of analogy would be mainly associated to the idea of “function.” In short, metaphors have no explanatory power, whereas analogies do, for the knowledge acquired on the functionality of a system can be transferred to an analogous one, thereby leading to understanding and discovery.
The Brain-Computer analogy has raised a harsh debate in the scientific community; some took it literally whereas the very meaning of analogies implies only a partial overlap of properties. In fact, it is very possible that analogies or metaphors are inescapable (and used regularly as cognitive tools; sensu Lakoff and Johnson, 1980; Gomez-Marin, 2022). Metaphors are rooted in things we know and/or manipulate. In this sense the only way to grasp what many things are is by describing the phenomena in terms we understand. In this process from “the physical phenomenon” to “the understanding of it,” a metaphor/analogy always arise. We hypothesize that we can claim “as original” only those things apprehended by the senses (always assuming that our senses are not tricking us). Metaphors might be the only things that we “comprehend,” and this is because they are rooted in our sensible experiences. Kuhn himself seems to acknowledge the importance of metaphors when he claims: “Metaphors play an essential role in establishing links between scientific language and the world. Those links are not, however, given once and for all. Theory change, in particular, is accompanied by a change in some of the relevant metaphors and in the corresponding parts of the network of similarities through which terms attach to nature” (1993).
In this context, the wrong question to ask is if metaphors and analogies are actually useful or misleading. Quite appropriately, Yohan (2012) points out that “… No one can claim to know how metaphors work … how we form them, and how we decide whether they are successful or not.” Along the same line, we do believe that it is totally irrelevant, to their role in science, whether metaphors and analogies are “right or wrong.” This attitude being best exemplified by the famous Niels Bohr’s horseshoe anecdote (many similar versions are available on the internet): A friend asked if he believed in it. “Absolutely not! Bohr replied, but they say it works even if you do not believe in it.”
Usefulness seems a more appropriate adjective for metaphors; being as successful as they provide clues to the phenomena under analysis.
Moreover, from the mathematician’s standpoint, but easily translatable to any scientific discipline, William Byers maintains that “many important mathematical ideas are metaphoric in nature” and emphasizes “the close relationship between metaphors and ideas. A metaphor, like an idea, arises out of an act of creativity” (Byers, 2010, p. 240). Moreover, Byers points out that “… In general, most sweeping conjectures turn out to be “wrong” in the sense that they need to be modified during the period in which they are being worked on. Nevertheless, they may well be very valuable. The whole of mathematical research often proceeds in this way—the way of inspired mistakes. … Ideas that are “wrong” can still be valuable.”
A number of articles in this SI deal with the use of metaphors in a specific area of science, the interphase between neuroscience and computer science. In this context it is important to emphasize once more that the metaphors are essential, but also transitory, in the sense that more, newer, data to the formulation of others (or a more refined version of previous) that seem more suitable at the moment. In addition, and in the absence of new data piling up, sociological or epistemic changes could also be, at certain moments, fruitful sources of new metaphors. Moreover, Gershenson reminds us that “different metaphors can be useful for different purposes … and in different contexts” within a discipline. Utilizing a unique metaphor (as we explain below) might not be the most productive avenue to explain certain complex structures, for instance the brain.
In the preceding paragraphs, we have proposed two features that may be useful to characterize and differentiate metaphor from analogy. In our view, a metaphor develops from a visual image, a picture that serves as creative force for scientific insight. Again with reference to mathematics, Ivar Ekeland stresses the relationship between mathematical ideas and “certain pictures” and the power of those pictures “… of certain visual representations, in the historical development of science … It is a power, in the early stages, to initiate progress, when the ideas it conveys are still creative and successful, and it becomes, later on, power to obstruct, when the momentum is gone and repetition of the old theories prevents the emergence of new ideas” (Ekeland, 1988, p. 9).
As Denis Nobles put it “… Different, even competing, metaphors can illuminate different aspects of the same situation, each of which may be correct even though the metaphors themselves may be incompatible. … Metaphors compete for insight, and for criteria like simplicity, beauty, creativity … Metaphor invention is an art not a science and, as with other art forms, the artist is not necessarily the best interpreter” (Noble, 2006). To these views we subscribe fully.
As for analogy, we think that the criterion of “function” could be regarded as its most characterizing property, a property endowed with explanatory power. For example, in this SI, Daniel Graham proposes internet as a new metaphor for the Brain. According to our definitions (see above), in the case internet works both as metaphor and analogy.
To end this section, a cautionary note is warranted. Metaphors may be inevitable and necessary to Science, because of psychological factors associated to learning or the search for explanations (Hoffman, 1980). The alternative to using metaphors would be a crude description of facts. In the philosophy of mind (or our discussion here) this would imply a “pure” description of physiological states in the brain. Whether there is any “information content” attached to this description, the authors of this review think there is very little, if any. We do not envision a productive substitute for the use of metaphors in science.
3.2. Theories of the brain
We would like to emphasize here the importance of considering the brain as a structure that can be analyzed at “different scales,” where functions might be distributed in particular domains and with the involvement of different components. In this sense we believe that it is wrong to search for “an ultimate THEORY of the brain,” since a better description would have to accommodate explanations on how these levels of architectural organization (including the varied set of functional domains) are established and integrated. As explained below, it may be more appropriate to explore “different theories of the brain,” perhaps a more suitable name for the exploratory endeavor we propose next.
In this context we would like to bring about a different perspective for analyzing the brain-computer analogy and this is through a systems approach in which the different levels of organization are candidates for specific analogies. We think that a theory that tries to analogize components (or modules, see below) should be more productive that a single theory encompassing such a complex structure as the brain. The underlying assumption here is that brains are the (non-linear) sum of components (modules) that are juxtaposed to perform, or facilitate, certain mental tasks. This is not a gratuitous assumption, since current data in the neurosciences, has proven the modularity of many of the structures in the brain, all products of evolutionary history. From the commonalities of neuronal subtypes to the conservation of specific neuronal circuits or the distribution of cortical areas, the brains of many animals share structures that were selected for specific functions and that are now recognized as homologous across taxa (Schlosser, 2018; Barsotti et al., 2021; Tosches, 2021).
In fact, brains, as any other organ or tissue, are organized at different levels, with modular blocks contributing to the next one; this suggests a parallel discussion between analogizing brain structures and the more classical discussion of biological homologies across scales (proteins, cells, organs, etc.).
In this framework, the fact that brains are organized in a series of hierarchical levels allows us to re-focus our attention on finding analogies that best represent different scales (i.e., a computer at one level, a radio at another, a hologram below, and an internet above, etc.). This does not imply setting aside the problem of the brain as a whole, just that it could be more productive finding good (useful) analogies of those, lower level, modules involved in its construction; and use modules as recognized functional units (e.g., neurons or neuronal circuits). A cautionary note needs to be introduced here: we are not claiming any strong/rigid interpretation of the brain modularity since we understand that there are clear instances of distributed function, and plasticity, plus the shifting localization of representations of stimuli over time. In fact, it is the distributed and flexible organization of modules what allows us for the integration of levels.
In a sense, and as explained by Cobb, our hypotheses on brain development and function have depended, at every historical time, on the current knowledge of the system. Hydraulic or electrical images of the brain were suggested at the time when discoveries were made on these areas; within and without the body. Calculations and algorithms promoted computational ideas of the brain, though, later on, of the neurons themselves (including the more recent idea that single neurons are doing complex computations at the synapsis). Analogies, sometimes, are exported from one level of analysis to another, with the computing image of whole brains or “single” synapsis as an obvious example. Similarly, the network analogy flows from the local connection of a few neurons (the reflex arc) to areas of the brain involved in specific tasks, to the whole brain or swarms of them.
To sum up what is explained above, we would like to suggest a reappraisal of the use of our analogies, so we can better understand how every level is organized and, importantly, how the integration of different modules at a particular level contribute to generate (emergent) properties observed at the next higher level. Moreover, we would encourage the introduction of different analogies that best represent the different levels of construction (avoiding, perhaps, the trap of overarching analogies explaining every single component in a complex system). Surely enough, we should notice here that “parts” in the construction of the living organism can be attributed to chance (drift), to physical–chemical laws (self-organizing), to emergent phenomena or to adaptive processes. All of these, constructional, principles bear no relationship with our purely structural view of the organism. Here we base our suggestion on the analysis of structures per se, at many levels, but not about their developmental assembly. Perhaps this last approximation can be considered in the future, in refined forms of our analogical search.
3.3. Creativity in science
Why are we interested in creativity in the context of this Special Issue? Because if we ask “can computers think,” next we ought to ask “can computers create.” And the very act of creation (be it in sciences or in the arts) stems from the awareness of the esthetic element. Reflection on creativity, and its sources, has a long history. While philosophers are far from a consensus definition of what creativity is and what it entails (see Erden, 2010), there are some tenets that are commonly recognized as pertaining to the creative act (freedom, potential, originality, etc.). Moreover, some philosophers also recognize that creation, in fact, it is a process with some specific requirements (McGilchrist, 2021): (i) a generative faculty (allowing ideas to come about; recognizing patterns, etc.) (ii) a permissive element (generating the conditions for the ideas to develop), and (iii) a translational disposition (the insights carried over for a period up to the moment of the final “creative act”). These are not, per se, components of other (non-necessarily creative) process such as problem solving. In the latter sense, it has been manifested that “there is no algorithm behind creative processes.” What many authors agree upon is the fact that metaphors expand your creative thinking, and in that sense, the analysis of metaphors becomes a key component in understanding creativity in science. But how?
The analysis of metaphors and analogies relies on the understanding of its sources. Creativity is an obvious candidate. But what is the source of “true creativity” … in science? Undeniably, the history of science tells us that chance has played and will play an important role in scientific creativity. Aside from orthodox views, more innovative paths have been explored in recent times. This is a subject that bears an important place in our discussion about the brain-computer metaphor.
But first we need to define what creativity is and what is the suggested relationship between creativity and metaphors?
In formal and natural sciences, the issue of creativity has been thoroughly discussed mainly in Mathematics and Physics. The mathematician William Byers distinguished two types of thought in mathematics: algorithmic (based on logical operations) defined as trivial and profound (deep) thought, defined as creative (Byers, 2010).
Byers asks a number of deep questions “Could a computer be programmed to distinguish between the trivial and the deep?” … “Can a computer do mathematics?” … “Is mathematics algorithmic?.” Therefore, and inevitably, he is confronted with “THE question” first addressed by Turing in 1950: “Can a computer think?,” which, he says “… is equivalent to the question: Is [human] thought algorithmic?” If, following Byers again, “human creativity involves ideas, ambiguity, paradox, depth, and complexity” an act of creativity (a very rare event indeed) might be analogous to a biological evolutionary event, since (as some authors have pointed out), it is impossible to predict, a priori, how ideas will unfold in the future. Ideas seem to unfold over time, through culture (Gabora and Kaufman, 2010).
The mathematician Henri Poincaré, in asking “what is mathematical creation?” proposed the following: “The mathematical facts worthy of being studied are those which, by their analogy with other facts, are capable of leading us to the knowledge … Among chosen combinations the most fertile will often be those formed of elements drawn from domains which are far apart … Invention is discernment, choice” (Poincaré, 1910). The theoretical physicist Paul Dirac, followed an even more unconventional path designating the potent role of esthetics in scientific creativity (see below). We share the opinion of those authors who think metaphors to be invaluable to scientific creativity in that they permit to explore uncharted lands and offer new perspectives for expanding scientific knowledge.
Here we propose to consider another aspect of creativity that we think to be highly relevant, but still not fully appreciated: it is the feeling of emotion, of amazement (émerveillement, in French) the researcher may feel in front of a phenomenon (though Socrates and Plato linked it already to wisdom). This feeling might be necessary and sufficient to awaken the scientist and illuminate his/her thoughts. Moreover, and probably facilitated by “émerveillement,” we would also like to suggest that creativity in science might depend on the integration of views arising from different disciplines (as many scientists have stated when asked about their own work). In this context, we would speculate that “transferring metaphors” from one field to another could be a good source of new ideas, thus propitiatory of a creative act. Metaphors as tools for understanding in one field should be able to illuminate other aspects of reality, in another field. This mental “transferring” could be productive, and, thus lead to “understanding” of unrelated phenomena.
In the context of the Brain-Computer analogy the comparison between human creativity and “AI creativity” arises spontaneously. And, the debate as to whether AI does and will play a role in human creativity is undeniably timely.
Obviously, human creativity is influenced by cultural traditions (context), or through the connections between very different ideas (i.e., from different/distant fields). This might suggest some intrinsic difficulties in imitating (the process of) human creativity with AI.
In a rather trivial way, and following the assertion above, we can state that computers are not creative, unable to produce “acts of creativity,” since creation might not be a pure algorithmic process.
Indeed, supporting the preeminence of human creativity, Byers (2010) writes “… mathematical thought can be simple and it can also be complex but mostly it is nontrivial. Computer thought, on the other hand, even though it may be very lengthy and complicated, is essentially trivial.” Along the same lines, Roli et al. support the notion that creativity in mathematics “… does not come out of algorithmic thought but via insight,” based on the argument that AI algorithms (being human devised) cannot identify, appraise and exploit (adapt to) new “environmental” alternatives (called affordances), to new frames of reference (Roli et al., 2022*).
Advocates of the potential of AI describe its performance, either in autonomous creative processes or with human intervention, mainly in fields such as writing, music, and painting (e.g., Zylinska, 2020; Jukebox, https://openai.com/blog/jukebox/), but also in scientific discovery (e.g., protein fold prediction via machine-and deep-learning techniques; see Gil et al., 2014 and Callaway, 2020).
Other authors sustain a more optimistic view of AI creativity (e.g., Boden, 2003; Forbes, 2020). These authors, following tenets of classical psychology (e.g., Boden, 1992), view creativity as having different sources and, thus, classify it as: combinatorial (combination of familiar ideas; e.g., poetic images), exploratory (arising within cultural traditions; e.g., cooking recipes), and transformational creativity (older rules are broken; e.g., cubism). While combinatorial and exploratory components are imitable by AI, the possibility of transformational creativity in computing seems a bit more problematic. Breaking rules seem to be a capacity specific to humans, since in the final acceptance of those new rules depends on a “value judgement” (only those outcomes that satisfy some new needs are incorporated). Of course, the possibility of having evolving programs (with selective regimes) are now a reality and this allows the possibility of “transformation.” Here again value is an important selective factor, which can hardly be implemented (nowadays) by AI. We should be reminded that critical thinking is still central to the creative process and, while humans are able to scrutinize their ideas/creations, computers cannot. All in all, these authors seem to suggest the possibility of finally exorcizing Cartesian dualism while establishing that the brain is, perhaps, a wonderfully subtle machine.
In spite of these, sometimes, entrenched opinions, what every scientist seems to agree upon is that with the current state of AI, our “machines” capabilities for imitating human creativity is still quite limited nowadays, though the future can change our views quite rapidly. All in all, creativity should be incorporated as a key concept when discussing metaphors such as that relevant to this Special Issue.
To end this review essay, we think it is important to mention the role of beauty (and esthetics in general) in scientific creativity.
Creativity and esthetics go hand in hand. Evaluation of creativity always requires a judgment of beauty. For instance, and according to a Kant’s classical statement: “aesthetics is not the goal of creativity but it is its essential component.”
Its crucial role in science has been recognized by a number of great mathematicians and physicists: G. W. Leibniz, H. Poincaré, A. Einstein, G. H. Hardy, P. A. M. Dirac, M. Gell-Mann, to name just a few. In 1910 Poincare writes “… the feeling of mathematical beauty, of the harmony of numbers and forms, of geometric elegance. This is a true esthetic feeling that all real mathematicians know, and surely it belongs to emotional sensibility. Now, what are the mathematic entities to which we attribute this character of beauty and elegance, and which are capable of developing in us a sort of esthetic emotion?” (Poincaré, 1910). In a famous and often quoted sentence Dirac boldly stated that “… it is more important to have beauty in one’s equations than to have them fit experiment” (Dirac, 1963). And in G. H. Hardy’s words: “The mathematician’s patterns, like the painter’s or the poet’s, must be beautiful.” Finally, the pervasiveness of the esthetics approach to human knowledge is epitomized in two extraordinarily powerful lines by the romantic English poet John Keats: “What the imagination seizes as beauty must be the truth” (1817) and “Beauty is truth, truth beauty. That is all ye know, and all ye need to know” (1884; in Keats, 2015). The relationships linking computer science, creativity and esthetics are explored in a recent review by Yang and Lu (2022), in which the authors also propose a framework that uses computational methods to connect creativity and esthetics.
It is fairly obvious that the meaning of the concept of beauty differs greatly among cultures, and also among individuals; this is trivial. Indeed, the commonly accepted stance is that the notion of esthetic quality is elusive. But here lies the problem, as metaphorically illustrated by the famous Bruegel’s painting “The blind conducts the blind”; everybody is looking for the “absolute” definition of beauty, and exactly this is the mistake. In contrast, the most crucial point is that, as far as science is concerned, all meanings and definitions of beauty are equivalent, and they all fulfill the same goal: to show the way to scientific discovery. Beauty is impossible to define because it lies in the eyes of the observer? Sure, but … “It does not matter!” for the chances for any esthetic criterion to be effective are not negligible at all. Likewise, for metaphors and analogies.
Author contributions
All authors listed have made a substantial, direct, and intellectual contribution to the work and approved it for publication.
Acknowledgments
We thank Matthew Cobb and Alex Gomez-Marin for critical reading of the MS. We also thank R. Brette, M. Cobb, M. Chirimuuta, A. Danchin, C. Gershenson, A. Gomez-Marin, D. Graham, M. Levin, B. Richards, A. Roli, and R. Solé for feedback on resumes of their articles. Thanks are also due to Marino Miculan for clarifying a number of concepts in computer science. GM wishes to thank most warmly Annalisa Pastore and Pierandrea Temussi for valuable advice and constant encouragement.
Conflict of interest
The authors declare that the research was conducted in the absence of any commercial or financial relationships that could be construed as a potential conflict of interest.
Publisher’s note
All claims expressed in this article are solely those of the authors and do not necessarily represent those of their affiliated organizations, or those of the publisher, the editors and the reviewers. Any product that may be evaluated in this article, or claim that may be made by its manufacturer, is not guaranteed or endorsed by the publisher.
Footnotes
1. ^https://www.oed.com/view/Entry/117328?redirectedFrom=metaphor&
2. ^ Graham, D. (2022). Nine insights from internet engineering that help us understand brain network communication. Front. Comput. Sci. (in preparation).
References
Atran, S. (1990). Cognitive Foundations of Natural History, Towards an Anthropology of Science. Cambridge, UK: Cambridge University Press.
Barsotti, E., Correia, A., and Cardona, A. (2021). Neural architectures in the light of comparative connectomics. Curr. Opin. Neurobiol. 71, 139–149. doi: 10.1016/j.conb.2021.10.006
Black, M. (1962). Models and Metaphors, Studies in Language and Philosophy. Ithaca, New York, USA: Cornell University Press.
Boden, M. A. (1992). Understanding creativity. J. Creat. Behav. 26, 213–217. doi: 10.1002/j.2162-6057.1992.tb01178.x
Bongard, J., and Levin, M. (2021). Living things are not (20th century) machines: updating mechanism metaphors in light of the modern science of machine behavior. Front. Ecol. Evol. 9:650726. doi: 10.3389/fevo.2021.650726
Boullerne, A. I., Foley, P., Turner, A. J., Johnston, G. A. R., and Beart, P. M. (2020). The origins and early history of neurochemistry and its societies. J. Neurochem. 152, 8–28. doi: 10.1111/jnc.14839
Brady, S., Siegel, G., and Albers, R. W. and Editors P. D. L. (2011). Basic Neurochemistry: Principles of Molecular, Cellular and Medical Neurobiology. New York, USA: Academic Press.
Brette, R. (2022). Brains as computers: metaphor, analogy, theory or fact? Front. Ecol. Evol. 10:878729. doi: 10.3389/fevo.2022.878729
Callaway, E. (2020). It will change everything': DeepMind's AI makes gigantic leap in solving protein structures. Nature 588, 203–204. doi: 10.1038/d41586-020-03348-4
Chirimuuta, M. (2022). Artifacts and levels of abstraction. Front. Ecol. Evol. 10:952992. doi: 10.3389/fevo.2022.952992
Cobb, M. (2021). A brief history of wires in the brain. Front. Ecol. Evol. 9:760269. doi: 10.3389/fevo.2021.760269
Cole, D. (2020). “The Chinese room argument,” in The Stanford Encyclopedia of Philosophy (Winter 2020 Edition). ed. E. N. Zalta. (Stanford, USA)
Danchin, A., and Fenton, A. A. (2022). From analog to digital computing: is Homo sapiens’ brain on its way to become a Turing machine? Front. Ecol. Evol. 10:796413. doi: 10.3389/fevo.2022.796413
Davidson, E. H., and Peter, I. (2015). Genomic Control Process: Development and Evolution. New York, USA: Academic Press.
Davis, M. (2021). The brain-as-computer metaphor. Front. Comp. Sci. 3:681416. doi: 10.3389/fcomp.2021.681416
Dirac, P. A. M. (1963). The evolution of the physicists picture of nature. Sci. Am. 208, 45–53. doi: 10.1038/scientificamerican0563-45
Doolittle, W. F. (1999). Phylogenetic classification and the universal tree. Science 284, 2124–2128. doi: 10.1126/science.284.5423.2124
Epstein, R. (2016). Your brain does not process information and it is not a computer, Aeon. Available at: https://aeon.co/essays/your-brain-does-not-process-information-and-it-is-not-a-computer
Erden, Y. J. (2010). Could a created being ever be creative? Some philosophical remarks on creativity and AI development. Mind. Mach. 20, 349–362. doi: 10.1007/s11023-010-9202-2
Faisal, A. A., Selen, L. P., and Wolpert, D. M. (2008). Noise in the nervous system. Nat. Rev. Neurosci. 9, 292–303. doi: 10.1038/nrn2258
Fernandez, N., Maldonado, C., and Gershenson, C. (2014). “Information measures of complexity, emergence, self-organization, homeostasis, and autopoiesis,” in Guided Self-Organization: Inception. Vol. 9. ed. M. Prokopenko (Berlin Heidelberg: springer).
Forbes, A. C. (2020). AI: from expressive mimicry to critical inquiry. Artnodes 26, 1–10. doi: 10.7238/a.v0i26.3370
Fraser, P., Solé, R., and De las Cuevas, G. (2021). Why can the brain (and not a computer) make sense of the liar paradox? Front. Ecol. Evol. 9:802300. doi: 10.3389/fevo.2021.802300
Gabora, L., and Kaufman, S. B. (2010). “Evolutionary perspectives on creativity,” in The Cambridge Handbook of Creativity. eds. J. Kaufman and R. Sternberg (Cambridge, UK: Cambridge University Press), 279–300.
Gentner, D., and Jezioreski, M. (1993). “The shift from metaphor to analogy in Western science,” in Metaphor and Thought. 2nd Edn. ed. A. Ortony (Cambridge, UK: Cambridge University Press), 447–480.
Gershenson, C. (2012). “The world as evolving information,” in Unifying Themes in Complex Systems. Vol. VII. eds. A. Minai, D. Braha, and Y. Bar-Yam (Berlin Heidelberg: Springer), 100–115.
Gershenson, C. (2021). Intelligence as information processing: brains, swarms, and computers. Front. Ecol. Evol. 9:755981. doi: 10.3389/fevo.2021.755981
Gil, Y., Greaves, M., Hendler, J., and Hirsh, H. (2014). Amplify scientific discovery with artificial intelligence. Science 346, 171–172. doi: 10.1126/science.1259439
Gomez-Marin, A. (2022). Commentary: metaphors we live by. Front. Comp. Sci. 4:890531. doi: 10.3389/fcomp.2022.890531
Graham, D. (2021). An Internet in Your Head: A New Paradigm for How the Brain Works. New York: Columbia University Press.
Haack, S. (2019). The art of scientific metaphors. Rev. Port. Filos. 75, 2049–2066. doi: 10.17990/RPF/2019_75_4_2049
Hatfield, G. (2012). “Mechanizing the sensitive soul,” in Matter and Form in Early Modern Science and Philosophy. ed. G. Manning (Leiden, Netherlands: Brill).
Hesse, M. B. (1970). Models and Analogies in Science. Notre Dame, USA: University of Norte Dame Press.
Hilgetag, C. C., and Goulas, A. (2016). Is the brain really a small-world network? Brain Struct. Funct. 221, 2361–2366. doi: 10.1007/s00429-015-1035-6
Hoffman, R. R. (1980). “Metaphor in science,” in Cognition and Figurative Language. eds. R. P. Honeck and R. R. Hoffman (New York: Routledge).
Hoffman, R. R. (1985). “Some implications of metaphor for philosophy and psychology of science,” in The Ubiquity of Metaphor: Metaphor in Language and Thought. Vol. 29. eds. W. Paprotté and R. Dirven (Amsterdam, Netherlands: Current Issues in Linguistic Theory; John Benjamins Publishing Company), 327–380.
Humar, M. (2021). Metaphors as models: towards a typology of metaphor in ancient science. HPLS 43:101. doi: 10.1007/s40656-021-00450-2.Jukebox
Kerskens, C. M., and Lopez-Perez, D. (2022). Experimental indications of non-classical brain functions. J. Phys. Commun. 6:105001. doi: 10.1088/2399-6528/ac94be
Kovac, J. (2003). Writing as thinking. Ann. N. Y. Acad. Sci. 988, 233–238. doi: 10.1111/j.1749-6632.2003.tb06103.x
Kuhn, T. S. (1993). “Metaphor in science,” in Metaphor and Thought. 2nd Edn. ed. A. Ortony (Cambridge, UK: Cambridge University Press), 533–542.
Lakoff, G. (1993a). “The contemporary theory of metaphor,” in Metaphor and Thought. 2nd Edn. ed. A. Ortony (Cambridge, UK: Cambridge University Press).
Lakoff, G. (1993b). “The syntax of metaphorical semantic roles,” in Semantics and the Lexicon. Studies in Linguistics and Philosophy. Vol. 49. ed. J. Pustejovsky (Dordrecht: Springer).
Mahoney, M. S. (1988). The history of computing in the history of technology. Ann. Hist. Comput. 10, 113–125. doi: 10.1109/MAHC
Martin, W. (1999). Mosaic bacterial chromosomes: a challenge en route to a tree of genomes. Bio Essays 21, 99–104. doi: 10.1002/(SICI)1521-1878(199902)21:2<99::AID-BIES3>3.0.CO;2-B
Martinez, P., and Sprecher, S. G. (2020). Of circuits and brains: the origin and diversification of neural architectures. Front. Ecol. Evol. 8:82. doi: 10.3389/fevo.2020.00082
Maxwell, J. C. (1870). “Address to the mathematical and physical sections of the British association. British association report, p 227; reprinted,” in The Scientific Papers of James Clerk Maxwell. ed. W. D. Niven (Cambridge, UK: Cambridge University Press), 215–229.
McCulloch, W., and Pitts, W. (1943). A logical calculus of ideas immanent in nervous activity. Bull. Math. Biophys. 5, 115–133. doi: 10.1007/BF02478259
McGilchrist, I. (2021). The Matter With Things: Our Brains, Our Delusions, and the Unmaking of the World. UK: Perspectiva Press.
Nicholson, D. J. (2013). Organisms ≠ Machines. Stud. Hist. Philos. Biol. Biomed. Sci. 44, 669–678. doi: 10.1016/j.shpsc.2013.05.014
Owen, R. (1843). Lectures on the Comparative Anatomy and Physiology of the Invertebrate Animals. London: Longman, Brown, Green, and Longmans.
Pitts, W., and McCulloch, W. S. (1947). How we know universals the perception of auditory and visual forms. Bull. Math. Biophys. 9, 127–147. doi: 10.1007/BF02478291
Ragan, M. A. (2009). Trees and networks before and after Darwin. Philos. Trans. R. Soc. B 364, 2169–2175. doi: 10.1098/rstb.2009.0046
Reynolds, A. S. (2022). Understanding Metaphors in the Life Sciences (Understanding Life). Cambridge, UK: Cambridge University Press.
Richards, B. A., and Lillicrap, T. P. (2022). The brain-computer metaphor debate is useless: a matter of semantics. Front. Comp. Sci. 4:810358. doi: 10.3389/fcomp.2022.810358
Roli, A., Jaeger, J., and Kauffman, S. A. (2022). How organisms come to know the world: fundamental limits on artificial general intelligence. Front. Ecol. Evol. 9:806283. doi: 10.3389/fevo.2021.806283
Schlosser, G. (2018). A short history of nearly every sense-the evolutionary history of vertebrate sensory cell types. Integr. Comp. Biol. 58, 301–316. doi: 10.1093/icb/icy024
Sporns, O., and Honey, C. J. (2006). Small worlds inside big brains. Proc. Natl. Acad. Sci. U. S. A. 103, 19219–19220. doi: 10.1073/pnas.0609523103
Tosches, M. A. (2021). Different origins for similar brain circuits. Science 371, 676–677. doi: 10.1126/science.abf9551
Turing, A. M. (1937). On computable numbers, with an application to the Entscheidungsproblem. Proc. Lond. Math. Soc. s2-42, 230–265. doi: 10.1112/plms/s2-42.1.230
Turing, A. M. (1950). Computing machinery and intelligence. Mind LIX, 433–460. doi: 10.1093/mind/LIX.236.433
Waddington, C. H. (1957). The Strategy of the Genes; A Discussion of Some Aspects of Theoretical Biology. Crows Nest, Australia: George Allen & Unwin Ltd.
Wright, S. (1931). Evolution in Mendelian populations. Genetics 16, 97–159. doi: 10.1093/genetics/16.2.97
Yang, H., and Lu, Z. (2022). “Computerising connections between creativity and aesthetics,” in IEEE International Conference on Service-Oriented System Engineering (SOSE), 185–188.
Yohan, J. J. (2012). Metaphor: the Alchemy of Thought. Available at: https://axispraxis.wordpress.com/2012/09/26/metaphor-the-alchemy-of-thought/
Ziman, J. (2000). Real Science, What It Is and What It Means. Cambridge, UK: Cambridge University Press.
Keywords: neuroscience, computer science, semantics, metaphor, analogy, creativity, philosophy of science
Citation: Matassi G and Martinez P (2023) The brain-computer analogy—“A special issue”. Front. Ecol. Evol. 10:1099253. doi: 10.3389/fevo.2022.1099253
Edited by:
Thilo Gross, Helmholtz Institute for Functional Marine Biodiversity (HIFMB), GermanyReviewed by:
Laura Antonia Barth Martinez, Helmholtz Institute for Functional Marine Biodiversity (HIFMB), GermanyJohn Bickle, Mississippi State University, United States
Soumyajit Mandal, Brookhaven National Laboratory (DOE), United States
Marcos Cramer, Technical University Dresden, Germany
Michael Levin, Tufts University, United States
Copyright © 2023 Matassi and Martinez. This is an open-access article distributed under the terms of the Creative Commons Attribution License (CC BY). The use, distribution or reproduction in other forums is permitted, provided the original author(s) and the copyright owner(s) are credited and that the original publication in this journal is cited, in accordance with accepted academic practice. No use, distribution or reproduction is permitted which does not comply with these terms.
*Correspondence: Giorgio Matassi, ✉ Z2lvcmdpby5tYXRhc3NpQHVuaXVkLml0; Pedro Martinez, ✉ cGVkcm8ubWFydGluZXpAdWIuZWR1