- 1School of Mathematics and Statistics, The University of Melbourne, Melbourne, VIC, Australia
- 2Melbourne Centre for Data Science, The University of Melbourne, Melbourne, VIC, Australia
- 3Centre of Excellence for Biosecurity Risk Analysis, The University of Melbourne, Melbourne, VIC, Australia
- 4Peter Doherty Institute for Infection and Immunity, The Royal Melbourne Hospital and The University of Melbourne, Melbourne, VIC, Australia
- 5Melbourne School of Population and Global Health, University of Melbourne, Parkville, VIC, Australia
- 6Land and Water, Commonwealth Scientific and Industrial Research Organisation (CSIRO), Ecosciences Precinct, Dutton Park, QLD, Australia
- 7School of Agriculture and Food Science, The University of Queensland, St Lucia, QLD, Australia
- 8School of Biology and Environmental Science, Queensland University of Technology, Brisbane, QLD, Australia
- 9University of New England (UNE) Business School, University of New England, Armidale, NSW, Australia
- 10Centre for Applications in Natural Resource Mathematics (CARM), University of Queensland, St Lucia, QLD, Australia
- 11The Royal Children’s Hospital, Murdoch Children’s Research Institute, Melbourne, VIC, Australia
- 12School of Biological Sciences, University of Queensland, St Lucia, QLD, Australia
Scientific knowledge and advances are a cornerstone of modern society. They improve our understanding of the world we live in and help us navigate global challenges including emerging infectious diseases, climate change and the biodiversity crisis. However, there is a perpetual challenge in translating scientific insight into policy. Many articles explain how to better bridge the gap through improved communication and engagement, but we believe that communication and engagement are only one part of the puzzle. There is a fundamental tension between science and policy because scientific endeavors are rightfully grounded in discovery, but policymakers formulate problems in terms of objectives, actions and outcomes. Decision science provides a solution by framing scientific questions in a way that is beneficial to policy development, facilitating scientists’ contribution to public discussion and policy. At its core, decision science is a field that aims to pinpoint evidence-based management strategies by focussing on those objectives, actions, and outcomes defined through the policy process. The importance of scientific discovery here is in linking actions to outcomes, helping decision-makers determine which actions best meet their objectives. In this paper we explain how problems can be formulated through the structured decision-making process. We give our vision for what decision science may grow to be, describing current gaps in methodology and application. By better understanding and engaging with the decision-making processes, scientists can have greater impact and make stronger contributions to important societal problems.
Introduction
Scientific discoveries have fueled enormous change in our society, and many scientists aim to make a positive impact through their work. One way to create impact is to engage with decision-making and policy in the public sphere (Pham, 2016). However, there are barriers to this dialogue and many articles focus on how to improve communication and better engage with policy-makers (Cvitanovic et al., 2015; Berger et al., 2019; Oliver and Cairney, 2019). But we believe that improving communication channels between science and policy is only part of the picture; it is important to incorporate the social, political and organizational context of issues within the scientific work itself. Further, we argue that the decision science approach is an effective approach to incorporate those issues within scientific work.
Decision science provides methods for formulating scientific problems that explicitly link the conduct of science to the aim of informing and improving decisions. The key feature of decision science is that it identifies, first and foremost, a decision problem, with associated objectives and the potential management actions (Blomquist et al., 2010). The goal is then to use evidence to determine which action—or set of actions—best meets the objectives. Within the process, the role of scientists is to provide the evidence that enables decision makers to make an informed choice on which action(s) to take. Hence, a decision science framing of the problem can pinpoint areas of future research that are both fundamental knowledge gaps and that will contribute directly to improved management.
Decision science is not the only method that is useful for supporting evidenced-based decisions, but its contributions are complementary to other approaches, such as decision support tools and participatory modeling. Decision support tools (or systems) are pieces of software designed specifically to aid decision-makers when making decisions or setting policy (McIntosh et al., 2011; Shearer et al., 2020). Participatory modeling is another approach aimed at improving uptake of scientific work through a participatory process, where stakeholders are involved in the design and modeling process (Voinov et al., 2016, 2018). These methods are essentially a way to strengthen the relationship and communication channels between scientists and stakeholders and improve timeliness of information, which is important, and particularly critical in emergency response situations (Albris et al., 2020). But decision science, as we view it, is strengthening scientists’ understanding of how they can incorporate the decision context within their work.
In this paper we describe our vision for decision science, and how scientists can use it to make an impact in the policy sphere. We begin by explaining the fundamentals of decision science, with an overview of structured decision making (Gregory et al., 2012). As decision science methods have been covered in detail elsewhere (e.g., Hemming et al., 2021), we only provide the necessary background to understand our vision. We then describe our vision for decision science and explain why we believe it’s important to include an awareness of the broader context of decisions when framing scientific questions. We identified the timescales involved in decision-making to be a critical aspect of how decision science works in practice, and we discuss how science fits into the fast-paced world of managing the COVID-19 pandemic. Finally, we discuss the importance of relationships between scientists and decision-makers and describe how scientists can increase their impact, using decision science.
Structured Decision Making
At the core of decision science is the “decision problem”, and structured decision making is one method to identify a decision problem and is a good way to understand each aspect of a decision problem. Structured decision making provides a framework for breaking down complex problems and identifying objectives, actions and knowledge gaps. It is a participatory process that draws together objectives around an issue, determines potential actions and attempts to predict their outcomes, before finally determining a recommended pathway forward. In this section we give a brief overview of the methodology, but greater detail is available elsewhere (Martin et al., 2009; Clemen and Reilly, 2013). Throughout this section, we use the Project Prioritization Protocol (PPP) for threatened species funding allocation in New Zealand and New South Wales as an illustration to give a “real world example” for each step (Joseph et al., 2009; Brazill-Boast et al., 2018). Before PPP was deployed, the allocation of funding to threatened species was largely ad hoc, determined by individuals without public transparency, focussed almost entirely on the species most likely to go extinct or of an iconic nature, and with no explicit objective and hence no measurable outcome.
Identifying the Fundamental Objective(s)
Objective-setting is a process whereby stakeholders identify what they wish to achieve. The desired outcomes should be narrowed to a set of measurable fundamental objectives. Importantly, the set of fundamental objectives can include multiple and even competing objectives. For PPP in New Zealand there was a budget constraint and one fundamental objective: to secure the most number of threatened species.
Determine Potential Actions
Actions include any type of intervention that affects the system itself, such as policy changes or on-ground projects. The list of potential actions should be as diverse as possible, and not be constrained by a priori assumptions on pre-conceived views about feasibility and/or effectiveness. For PPP, the actions were a list of potential projects, one for each of the threatened species being considered.
Predict the Outcome of Potential Actions
It is necessary to predict how well each action will help achieve the fundamental objective(s), and scientists can make a critical contribution to this part of the structured decision-making process. Scientists—through the traditional approach of discovery—can provide the fundamental scientific knowledge required to develop models (mathematical or otherwise) of the underlying processes driving observed system behavior. These models may then be used to project the state of the system into the future in response to different possible policy settings. PPP used taxonomic distinctiveness to quantify the uniqueness of each species and used expert elicitation to estimate the benefits, costs and likelihood of success of each of the projects.
Determine a Pathway Forward
The final step is to choose the action, or set of actions, that best meet the fundamental objective, given our understanding of the outcomes. In some situations, competing objectives may arise, where actions help achieve one objective at the expense of achieving another. In these contexts, additional processes such as multi-criteria decision science (Mendoza and Martins, 2006) and/or conflict resolution may be required (Biggs et al., 2017). PPP used a “project efficiency” metric to rank each project, which is related to the cost-effectiveness—top-ranked projects were funded until the budget was exhausted.
Problem Framing With Structured Decision Making
Correctly identifying and specifying the decision problem is central to the decision science process. The decision problem being the set of fundamental objectives and potential actions. One common point of confusion is around the difference between means objectives (often called intermediate objectives) and fundamental objectives. Means objectives are objectives that may indicate progress toward a fundamental objective, but they are not the specific aim (Schwartz et al., 2018). For example, in public health eliminating measles may be a fundamental objective, but a target like vaccinating 95% of the population would be a means objective.
Decision Science as a Pathway for Impact
Our vision of decision science for impact embeds the structured decision-making principles within an overarching understanding of the social, political and organizational context in which decisions are made and implemented (Figure 1). In particular, decision analyses often focus on understanding systems and identifying actions that meet fundamental system-level objectives, while keeping economic costs low. But there are many more factors that matter, including political and logistical constraints and social and ethical accountability.
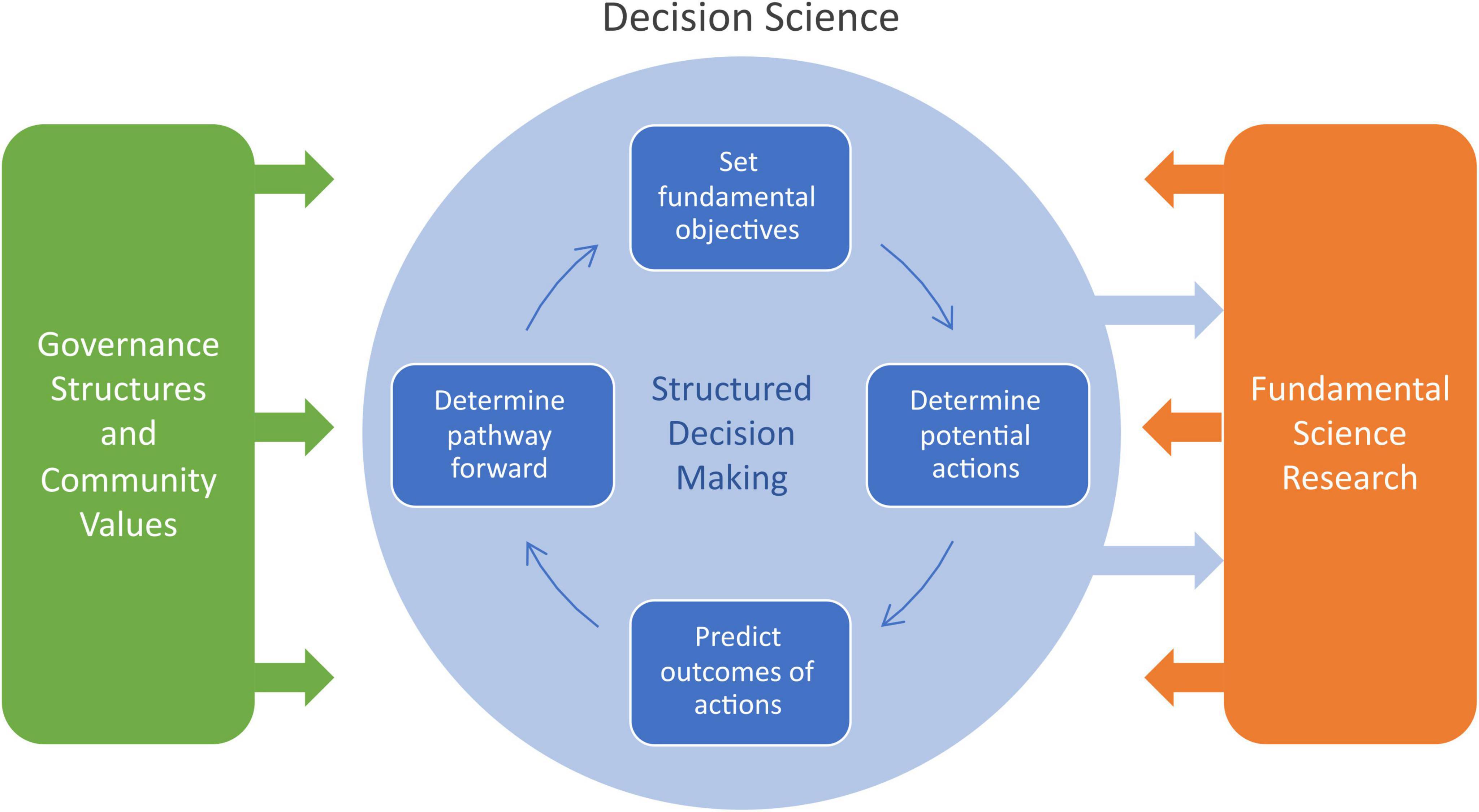
Figure 1. Decision science framework. Our vision of decision science for impact embeds Structured Decision Making as the nexus between the social, political and organizational context in which decisions are made and implemented and the science underlying the problem. Fundamental knowledge is integrated within the decision-making process, while incorporating the overarching governance structures and community values. When approached in a cyclic manner, with objectives and models being revised and refined over time, it becomes “adaptive management” (Canessa et al., 2016). The process both informs and is informed by fundamental science research.
Understanding the overall context of decisions, and how scientific research fits into this puzzle, is important when aiming to inform decisions. People, including scientists, do not evaluate knowledge solely on the basis of objective criteria (such as quality and content) but also according to subjective criteria including attitudes and social relationships (Burcharth and Fosfuri, 2015). Co-production of research, regular contact between research producers and stakeholders, use of “knowledge brokers” and clear communication of results are all ways to build trust and understanding been research producers and users, and thus increase uptake of research (Meyer, 2010; Arndt et al., 2020; Hester and Reed, 2021). Omitting the broader social context when framing scientific projects can therefore lead to downstream issues. Box 1 explores a case study of the “Death-Row Dingoes,” where dingoes were introduced to Pelorus Island to control a goat population. The negative publicity associated with the decision caused serious issues for the managers. This is a situation where we believe societies’ values were not adequately incorporated in the decision science process and a broader framing of the decision problem may have averted the controversy.
BOX 1. An example of science and decision making.
The “Death-Row Dingoes”: feral goat control in Australia
Ignoring societal values and the views of diverse stakeholders and a well-informed public can turn actions that meet scientific objectives into public policy failures. The case of the “death-row dingoes” in Northern Queensland, Australia in 2016 (van Eeden et al., 2017) is a case in point. The Hinchinbrook Shire Council released two dingoes onto Pelorus Island in a bid to cost-effectively reduce the feral goat population—other methods had proved ineffective. The small island is home to rare littoral rainforest that was being destroyed by several hundred feral goats, and the use of dingoes to control this pest had been used successfully several decades earlier, although in that case the dingoes themselves had become a pest (Schwartz, 2016). Due to concerns about potential impacts of the dingoes on Pelorus Island’s local bird populations, the dingoes were implanted with poison tablets that would activate within two years to ensure they would not become a long-term problem if they evaded capture. While some would argue this was a practical and necessary approach to reducing the threat, it caused national outrage and many questioned the ethics of the project (Yanco et al., 2019). In response, the Queensland Government ordered the Council to remove the dingoes, and it reduced public trust in the authorities’ ability to manage environmental issues (van Eeden et al., 2017). While predicting a public outrage may seem difficult, Australia has a history of wildlife population management being strongly influenced by the public (e.g., Drijfhout and Kendal, 2018), and we believe a decision science approach could have helped here. With a fundamental objective of cost-effectively reducing the feral goat population, identifying potential actions that pass the threshold of being socially acceptable from the perspective of diverse stakeholders and predicting outcomes within the overarching governance structures and community values, a decision science approach may have led to decisions that were more acceptable to society.
Using the decision science approach to formulate problems is not limited in utility to those directly involved in decision-making, but we believe it is useful for scientists who want their work to make a difference. The key role of science within a decision-making process is in providing evidence that underpins decisions, by quantifying how different actions relate to the fundamental objectives. Therefore, from a decision-making perspective, the most useful science is the science that helps them discriminate between different actions, and if scientists can gain a good understanding of the decision context, they can produce research that fits into decision-making frameworks and stands a good chance of making a difference.
The Role of Time Scales in Practical Decision Science
Decision science provides a framework to make decisions across a variety of contexts with differing time pressures. Some problems develop gradually over many decades (e.g., climate change), while others are acute and elicit an immediate response (e.g., wildfire or epidemic management). As we reduce uncertainty about the future (negative) impact of climate change, intervention options and actions are already available, and the world is implementing solutions informed by increased understanding (Wong-Parodi et al., 2016). In contrast, for pandemic events and natural disasters, such as earthquakes, tsunamis and wildfires, there is limited time to learn about the event before acting. For such “short time-scale” events, while we can plan and deploy defense strategies beforehand and make systems more resilient, it is not until the event occurs that a disaster management plan can be enacted (Borchers, 2005; Marcot et al., 2012; Glasscoe et al., 2015; Ai et al., 2016; Lourenço et al., 2021). For these short time-scale events, community values can still be considered through a structured decision-making process, but it must happen in advance as part of “preparedness” activities (e.g., Degeling et al., 2021).
Decision Science for Pandemic Preparedness
The World Health Organization, The United States Centers for Disease Control and Prevention, and many national governments, have highlighted the significant threats to society from emerging and re-emerging infectious diseases such as pandemic influenza, Ebola virus disease, SARS-CoV-1 and most recently SARS-CoV-2. Given the unique biological characteristics of each pandemic pathogen, the potential for pandemics to impact on broad societal freedoms and the economy (i.e., beyond health concerns), multiple stakeholders (including national, jurisdictional governments, clinical and public health, and other parts of government) must be brought together to understand fundamental objectives and actions.
For COVID-19, modeling to predict the outcomes of potential actions and guide policy had to occur extremely quickly in the early stages of the pandemic (Shea et al., 2020). Fortuitously, modeling of infectious diseases is a well-established field (Anderson and May, 1979) and there has been a recent focus on incorporating models and decision science into pandemic planning (Alahmadi et al., 2020; Shearer et al., 2020). Since the options available to decision-makers, the scientific knowledge of the disease and the virus are rapidly changing, scientists seeking to contribute to policy arguably needed to already have trusted relationships with decision-makers in place (Burcharth and Fosfuri, 2015). Established relationships enable scientists to provide relevant and timely scientific advice. Despite the shorter timescales inherent in emergency response, the process of decision science and structured decision making remains the same. The key difference compared to the other examples we have discussed is that with less time, there are fewer opportunities to resolve uncertainty and make precise predictions. But this is a typical situation for an adaptive management cycle (Chadès et al., 2016), where decisions are made, data is collected, predictions are improved, and decisions are refined in a cycle.
As the COVID-19 pandemic has progressed, and particularly with the availability of vaccines, the need to move from a short-term decision problem to a medium- to long-term decision problem is becoming clear. In moving away from emergency decision making, we need to carefully consider the competing objectives, uncertainty and changing population behavior. While country lockdowns have been extremely successful in reducing transmission, they come with a raft of other costs—economic, social, and related (non-COVID) health issues. One method for dealing with multiple objectives is multi-criteria decision science (Marttunen et al., 2017). In addition, predictions of possible actions will all likely have large uncertainties, in part due to our still-evolving understanding of the virus. In scenarios with uncertainty of system dynamics, value of information theory is a useful method to pinpoint the most useful data to collect to improve decisions (Canessa et al., 2015). Finally, how members of society respond to policies—when they have been subject to unprecedented and unexpected disruption and trauma—must be incorporated into the modeling and decision-making process, and incentive theory and behavioral sciences can help predict people’s response to policy change (Laffont and Martimort, 2009; Bavel et al., 2020).
Building Relationships for Effective Decision Science
Decision science provides a way to explicitly frame scientific questions within the relevant community and government structures. Community consultation is required to properly understand the values of a population, and clear communication is important to ensure that everyone can understand the fundamentals of the science underlying the prediction of potential outcomes of different management strategies. While these aspects bring together governance, community engagement and science, we must also include social and behavioral science, as it is critical to understand how policy influences community behaviors that may impact on potential outcomes. Underlying these themes is trust, which must exist between scientists, governments and the general population. Losing public trust can undermine progress (No authors listed, 2019; Bavel et al., 2020).
Decision science facilitates effective dialogue and relationships between scientists and decision-makers by providing a framework that requires scientists to elicit decision-maker opinions and values. Firstly, it demands that the scientists understand the decision context. A better understanding of decision-maker needs—type of inputs needed, the local context, the timing of decisions, stakeholder preferences and values, the degree of risk or uncertainty that is acceptable—can enable tailoring of scientific outputs for best application (Patt, 2009; Marcot et al., 2012). Secondly, dialogue is also pivotal for fostering relationships, shared understanding, trust (from the decision-maker) in the scientific integrity of the process, and trust (from the scientist) that cautions any uncertainties in predictions will be considered appropriately (Patt, 2009; von Winterfeldt, 2013; Doyle et al., 2014). Dialogue also allows scientists to gauge how their information is received, and provides them with the opportunity to clarify the scientific process, explain potential caveats or ways to apply findings across different contexts (Patt, 2009).
One of the specific challenges in decision science is how to communicate uncertainty in a way that supports decision making rather than inadvertently eliciting indecision or rejection of scientific information. Effective communication of uncertainty can build trust, and conversely, hiding uncertainty can undermine it (Patt, 2009). Uncertainty can arise from a range of sources, and these sources can include (but are not limited to) incomplete knowledge of all parameters within a complex system, incomplete control of how a response will be implemented, and errors in measurement of estimates (Johnson et al., 2015). The style of communication can also inadvertently contribute to uncertainty. For example, numerical likelihoods (e.g., “75% chance of occurring”) are perceived to be more certain than descriptive likelihoods (e.g., “Likely to occur”) which are often misinterpreted (Doyle et al., 2014). In communicating uncertainty, scientists must reduce uncertainties to their “decision-relevant” elements, describe the source of uncertainty, and incorporate uncertainty into estimates via use of credible intervals or ranges (Fischhoff and Davis, 2014). Ranges and credible intervals may incorporate different sources of uncertainty, including variability in estimates’ applicability to the decision context, and overall strength of the evidence (Fischhoff and Davis, 2014).
Discussion
So how, as scientists, can we reframe our practice to maximize our contribution to public policy? First and foremost, we must understand the context in which the technical work we perform might be used. Particularly for those who are policy-focused, technical work should be embedded within the decision science framework, acknowledging the social and political context in which decisions are made. If as a scientific community more of us can engage and work in this way, science will play a greater and more effective role in public policy. Secondly, all scientists must recognize that resolving uncertainty is not always necessary for decision making. Some unknowns are critical to resolve, while others are irrelevant for decisions (Li et al., 2017). Critical aspects of uncertainty in the context of decision making are the fundamental objectives and the list of possible actions; and changing the actions or objectives alters which system uncertainties are important.
While the decision science toolkit is well-developed, there are still areas where our current approaches are incomplete, which include balancing complexity and interpretability of solutions, and where stakeholders have antithetical aims and values. Complex decision problems often demand complex science and modeling, but increasing complexity makes it hard to understand and interpret results (Ferrer-Mestres et al., 2020). While creating tools that stakeholders can interact with helps understanding and dialogue (von Winterfeldt, 2013), we should aim to develop the simplest possible tools to aid decision-makers, those that still convey the complexity and subtlety of the situation. Decision scientists have developed methods to deal with multiple competing objectives (Mendoza and Martins, 2006; Marttunen et al., 2017), but these methods are designed for situations where there are trade-offs (for example, between biodiversity and economic gain), and are not equipped for situations where stakeholders are unwilling to make any trade-offs. The illegal wildlife trade is a prime example where there are extreme differences in opinion (Biggs et al., 2017) and decision science does not yet provide an effective way forward.
Despite its broad utility, we consider the name “decision science” to be a misnomer. It’s less of a science than a problem-solving approach, and perhaps “decision analysis” would be a better name (Shearer et al., 2020; van Poorten and MacKenzie, 2020). The point of decision science is to view scientific problems from a decision-making perspective, focusing on fundamental objectives and feasible actions to identify critical scientific questions that will inform decisions. As such, the more widely the tenets of decision science are understood, the better science-based decisions will be and the greater the impact of science will be.
Data Availability Statement
The original contributions presented in the study are included in the article/supplementary material, further inquiries can be directed to the corresponding author/s.
Author Contributions
CB wrote the first draft of manuscript. All authors contributed to manuscript revision, read, and approved the submitted version, conception of the work.
Funding
We acknowledge funding from the ARC Centre of Excellence for Environmental Decisions, the NHMRC Centres of Research Excellence Policy Relevant Infectious Disease Simulation and Mathematical Modelling (PRISM2) and Supporting Participatory Evidence use for the Control of Transmissible diseases in our Region Using Modelling (SPECTRUM; Grant No. GNT1170960), the ARC Centre of Excellence for Biodiversity Risk Analysis, the ARC Centre of Excellence for Mathematical and Statistical Frontiers, the Australian Partnership for Preparedness Research on Infectious Disease Emergencies (APPRISE; Grant No. GNT1116530), and the Centre of Excellence for Biosecurity Risk Analysis. JMcV was supported by an NHMRC Principal Research Fellowship.
Conflict of Interest
The authors declare that the research was conducted in the absence of any commercial or financial relationships that could be construed as a potential conflict of interest.
Publisher’s Note
All claims expressed in this article are solely those of the authors and do not necessarily represent those of their affiliated organizations, or those of the publisher, the editors and the reviewers. Any product that may be evaluated in this article, or claim that may be made by its manufacturer, is not guaranteed or endorsed by the publisher.
References
Ai, F., Comfort, L. K., Dong, Y., and Znati, T. (2016). A dynamic decision support system based on geographical information and mobile social networks: a model for tsunami risk mitigation in Padang, Indonesia. Saf. Sci. 90, 62–74. doi: 10.1016/j.ssci.2015.09.022
Alahmadi, A., Belet, S., Black, A., Cromer, D., Flegg, J. A., House, T., et al. (2020). Influencing public health policy with data-informed mathematical models of infectious diseases: recent developments and new challenges. Epidemics 32:100393. doi: 10.1016/j.epidem.2020.100393
Albris, K., Lauta, K. C., and Raju, E. (2020). Disaster knowledge gaps: exploring the interface between science and policy for disaster risk reduction in Europe. Int. J. Disaster Risk Sci. 11, 1–12. doi: 10.1007/s13753-020-00250-5
Anderson, R. M., and May, R. M. (1979). Population biology of infectious diseases: part I. Nature 280, 361–367. doi: 10.1038/280361a0
Arndt, E., Burgman, M., Schneider, K., and Robinson, A. (2020). “Working with government – innovative approaches to evidence-based policy-making,” in Conservation Research, Policy and Practice, Ecological Reviews, eds J. A. Vickery, N. Ockendon, N. Pettorelli, P. N. M. Brotherton, W. J. Sutherland, and Z. G. Davies (Cambridge: Cambridge University Press), 216–229. doi: 10.1017/9781108638210.013
Bavel, J. J. V., Baicker, K., Boggio, P. S., Capraro, V., Cichocka, A., Cikara, M., et al. (2020). Using social and behavioural science to support COVID-19 pandemic response. Nat. Hum. Behav. 4, 460–471. doi: 10.1038/s41562-020-0884-z
Berger, K. M., Wood, J. L. N., Jenkins, B., Olsen, J., Morse, S. S., Gresham, L., et al. (2019). Policy and science for global health security: shaping the course of international health. Trop. Med. Infect. Dis. 4:60. doi: 10.3390/tropicalmed4020060
Biggs, D., Holden, M. H., Braczkowski, A., Cook, C. N., Milner-Gulland, E. J., Phelps, J., et al. (2017). Breaking the deadlock on ivory. Science 358, 1378–1381. doi: 10.1126/science.aan5215
Blomquist, S. M., Johnson, T. D., Smith, D. R., Call, G. P., Miller, B. N., Thurman, W. M., et al. (2010). Structured decision-making and rapid prototyping to plan a management response to an invasive species. J. Fish Wildl. Manag. 1, 19–32. doi: 10.3996/jfwm-025
Borchers, J. G. (2005). Accepting uncertainty, assessing risk: decision quality in managing wildfire, forest resource values, and new technology. For. Ecol. Manag. 211, 36–46.
Brazill-Boast, J., Williams, M., Rickwood, B., Partridge, T., Bywater, G., Cumbo, B., et al. (2018). A large-scale application of project prioritization to threatened species investment by a government agency. PLoS One 13:e0201413. doi: 10.1371/journal.pone.0201413
Burcharth, A. L. D. A., and Fosfuri, A. (2015). Not invented here: how institutionalized socialization practices affect the formation of negative attitudes toward external knowledge. Ind. Corp. Change 24, 281–305.
Canessa, S., Guillera-Arroita, G., Lahoz-Monfort, J. J., Southwell, D. M., Armstrong, D. P., Chadès, I., et al. (2015). When do we need more data? A primer on calculating the value of information for applied ecologists. Methods Ecol. Evol. 6, 1219–1228. doi: 10.1111/2041-210x.12423
Canessa, S., Guillera-Arroita, G., Lahoz-Monfort, J. J., Southwell, D. M., Armstrong, D. P., Chadès, I., et al. (2016). Adaptive management for improving species conservation across the captive-wild spectrum. Biol. Conserv. 199, 123–131.
Chadès, I., Nicol, S., Rout, T. M., Péron, M., Dujardin, Y., Pichancourt, J.-B., et al. (2016). Optimization methods to solve adaptive management problems. Theor. Ecol. 10, 1–20.
Clemen, R. T., and Reilly, T. (2013). Making Hard Decisions with DecisionTools. Boston: Cengage Learning.
Cvitanovic, C., Hobday, A. J., van Kerkhoff, L., Wilson, S. K., Dobbs, K., and Marshall, N. A. (2015). Improving knowledge exchange among scientists and decision-makers to facilitate the adaptive governance of marine resources: a review of knowledge and research needs. Ocean Coast. Manag. 112, 25–35.
Degeling, C., Williams, J., Carter, S. M., Moss, R., Massey, P., Gilbert, G. L., et al. (2021). Priority allocation of pandemic influenza vaccines in Australia – Recommendations of 3 community juries. Vaccine 39, 255–262. doi: 10.1016/j.vaccine.2020.12.010
Doyle, E. E. H., McClure, J., Paton, D., and Johnston, D. M. (2014). Uncertainty and decision making: volcanic crisis scenarios. Int. J. Disaster Risk Reduct. 10, 75–101. doi: 10.1111/risa.12904
Ferrer-Mestres, J., Dietterich, T. G., Buffet, O., and Chadès, I. (2020). Solving K-MDPs. Proc. Int. Conf. Autom. Plan. Sched. 30, 110–118.
Fischhoff, B., and Davis, A. L. (2014). Communicating scientific uncertainty. Proc. Natl. Acad. Sci. U. S. A. 111, 13664–13671.
Glasscoe, M. T., Wang, J., Pierce, M. E., Yoder, M. R., Parker, J. W., Burl, M. C., et al. (2015). E-DECIDER: using earth science data and modeling tools to develop decision support for earthquake disaster response. Pure Appl. Geophys. 172, 2305–2324. doi: 10.1007/s00024-014-0824-9
Gregory, R., Failing, L., Harstone, M., Long, G., McDaniels, T., and Ohlson, D. (2012). Structured Decision Making: A Practical Guide to Environmental Management Choices. Hoboken: John Wiley & Sons.
Hemming, V., Camaclang, A. E., Adams, M., Burgman, M., Carbeck, K., Carwardine, J., et al. (2021). An introduction to decision science for conservation. Conserv. Biol. [Epub Online ahead of print]. doi: 10.1111/cobi.13868
Hester, S., and Reed, C. (2021). CEBRA Research: Harnessing Past and New Work to Improve Uptake and Impact of Best Practise Risk Analysis Approaches in MPI Analysis. Melbourne: Centre of Excellence for Biosecurity Risk Analysis (CEBRA).
Johnson, F. A., Eaton, M. J., Williams, J. H., Jensen, G. H., and Madsen, J. (2015). Training conservation practitioners to be better decision makers. Sustainability 7, 8354–8373. doi: 10.3390/su7078354
Joseph, L. N., Maloney, R. F., and Possingham, H. P. (2009). Optimal allocation of resources among threatened species: a project prioritization protocol. Conserv. Biol. 23, 328–338. doi: 10.1111/j.1523-1739.2008.01124.x
Laffont, J.-J., and Martimort, D. (2009). The Theory of Incentives: The Principal-Agent Model. Princeton: Princeton University Press.
Li, S.-L., Bjørnstad, O. N., Ferrari, M. J., Mummah, R., Runge, M. C., Fonnesbeck, C. J., et al. (2017). Essential information: uncertainty and optimal control of Ebola outbreaks. Proc. Natl. Acad. Sci. U. S. A. 114, 5659–5664. doi: 10.1073/pnas.1617482114
Lourenço, M., Oliveira, L. B., Oliveira, J. P., Mora, A., Oliveira, H., and Santos, R. (2021). An integrated decision support system for improving wildfire suppression management. ISPRS Int. J. Geoinf. 10:497. doi: 10.3390/ijgi10080497
Marcot, B. G., Thompson, M. P., Runge, M. C., Thompson, F. R., McNulty, S., Cleaves, D., et al. (2012). Recent advances in applying decision science to managing national forests. For. Ecol. Manag. 285, 123–132. doi: 10.1016/j.jenvman.2020.111676
Martin, J., Runge, M. C., Nichols, J. D., Lubow, B. C., and Kendall, W. L. (2009). Structured decision making as a conceptual framework to identify thresholds for conservation and management. Ecol. Appl. 19, 1079–1090. doi: 10.1890/08-0255.1
Marttunen, M., Lienert, J., and Belton, V. (2017). Structuring problems for Multi-Criteria Decision Analysis in practice: a literature review of method combinations. Eur. J. Oper. Res. 263, 1–17.
McIntosh, B. S., Ascough, J. C., Twery, M., Chew, J., Elmahdi, A., Haase, D., et al. (2011). Environmental decision support systems (EDSS) development – Challenges and best practices. Environ. Model. Softw. 26, 1389–1402. doi: 10.1016/j.envsoft.2011.09.009
Mendoza, G. A., and Martins, H. (2006). Multi-criteria decision analysis in natural resource management: a critical review of methods and new modelling paradigms. For. Ecol. Manag. 230, 1–22. doi: 10.1016/j.foreco.2006.03.023
Meyer, M. (2010). The rise of the knowledge broker. Sci. Commun. 32, 118–127. doi: 10.1177/1075547009359797
No authors listed (2019). Building trust is essential to combat the Ebola outbreak. Nature 567, 433–433. doi: 10.1038/d41586-019-00892-6
Oliver, K., and Cairney, P. (2019). The dos and don’ts of influencing policy: a systematic review of advice to academics. Palgrave Commun. 5:21.
Patt, A. (2009). “Communicating uncertainty to policy makers,” in Uncertainties in Environmental Modelling and Consequences for Policy Making, NATO Science for Peace and Security Series C: Environmental Security, eds P. C. Baveye, M. Laba, and J. Mysiak (Berlin: Springer), 231–251. doi: 10.1007/978-90-481-2636-1_10
Pham, D. (2016). Public engagement is key for the future of science research. NPJ Sci. Learn. 1:16010. doi: 10.1038/npjscilearn.2016.10
Schwartz, D. (2016). Grazier’s Enemy Set to be Pelorus Island’s Environmental Hero. New York: ABC News.
Schwartz, M. W., Cook, C. N., Pressey, R. L., Pullin, A. S., Runge, M. C., Salafsky, N., et al. (2018). Decision support frameworks and tools for conservation. Conserv. Lett. 11:e12385. doi: 10.1111/conl.12385
Shea, K., Runge, M. C., Pannell, D., Probert, W. J. M., Li, S.-L., Tildesley, M., et al. (2020). Harnessing multiple models for outbreak management. Science 368, 577–579. doi: 10.1126/science.abb9934
Shearer, F. M., Moss, R., McVernon, J., Ross, J. V., and McCaw, J. M. (2020). Infectious disease pandemic planning and response: incorporating decision analysis. PLoS Med. 17:e1003018. doi: 10.1371/journal.pmed.1003018
van Eeden, L. M., Dickman, C. R., Ritchie, E. G., and Newsome, T. M. (2017). Shifting public values and what they mean for increasing democracy in wildlife management decisions. Biodivers. Conserv. 26, 2759–2763. doi: 10.1007/s10531-017-1378-9
van Poorten, B. T., and MacKenzie, C. J. A. (2020). Using decision analysis to balance angler utility and conservation in a recreational fishery. North Am. J. Fish. Manag. 40, 29–47.
Voinov, A., Jenni, K., Gray, S., Kolagani, N., Glynn, P. D., Bommel, P., et al. (2018). Tools and methods in participatory modeling: selecting the right tool for the job. Environ. Model. Softw. 109, 232–255.
Voinov, A., Kolagani, N., McCall, M. K., Glynn, P. D., Kragt, M. E., Ostermann, F. O., et al. (2016). Modelling with stakeholders – next generation. Environ. Model. Softw. 77, 196–220.
von Winterfeldt, D. (2013). Bridging the gap between science and decision making. Proc. Natl. Acad. Sci. U. S. A. 110, 14055–14061.
Wong-Parodi, G., Krishnamurti, T., Davis, A., Schwartz, D., and Fischhoff, B. (2016). A decision science approach for integrating social science in climate and energy solutions. Nat. Clim. Change 6, 563–569.
Keywords: decision making, conservation, epidemiology, public policy, modeling
Citation: Baker CM, Campbell PT, Chades I, Dean AJ, Hester SM, Holden MH, McCaw JM, McVernon J, Moss R, Shearer FM and Possingham HP (2022) From Climate Change to Pandemics: Decision Science Can Help Scientists Have Impact. Front. Ecol. Evol. 10:792749. doi: 10.3389/fevo.2022.792749
Received: 11 October 2021; Accepted: 24 January 2022;
Published: 14 February 2022.
Edited by:
Martin Drechsler, Helmholtz Centre for Environmental Research, Helmholtz Association of German Research Centres (HZ), GermanyReviewed by:
Amy Whitehead, National Institute of Water and Atmospheric Research (NIWA), New ZealandJulia M. Touza, University of York, United Kingdom
Copyright © 2022 Baker, Campbell, Chades, Dean, Hester, Holden, McCaw, McVernon, Moss, Shearer and Possingham. This is an open-access article distributed under the terms of the Creative Commons Attribution License (CC BY). The use, distribution or reproduction in other forums is permitted, provided the original author(s) and the copyright owner(s) are credited and that the original publication in this journal is cited, in accordance with accepted academic practice. No use, distribution or reproduction is permitted which does not comply with these terms.
*Correspondence: Christopher M. Baker, Y2Jha2VyMUB1bmltZWxiLmVkdS5hdQ==