- 1Max Planck Institute of Animal Behavior, Konstanz, Germany
- 2Department of Biology, University of Konstanz, Konstanz, Germany
- 3Center for the Advanced Study of Collective Behavior, University of Konstanz, Konstanz, Germany
- 4Data Visualization and Interaction at Cincinnati Laboratory, University of Cincinnati, Cincinnati, OH, United States
- 5Computer Science and Engineering, Ohio State University, Columbus, OH, United States
- 6Electrical and Computer Engineering, Ohio State University, Columbus, OH, United States
- 7Evolution, Ecology, and Organismal Biology, Ohio State University, Columbus, OH, United States
- 8Translational Data Analytics Institute, Ohio State University, Columbus, OH, United States
- 9Department of Anthropology, University of California, Davis, Davis, CA, United States
- 10Animal Behavior Graduate Group, University of California, Davis, Davis, CA, United States
- 11Smithsonian Tropical Research Institute, Panama City, Panama
- 12College of Science and Engineering, James Cook University, Townsville, QLD, Australia
- 13North Carolina Museum of Natural Sciences, Raleigh, NC, United States
- 14Department of Forestry and Environmental Resources, North Carolina State University, Raleigh, NC, United States
- 15Science Communication Lab, Inc., San Francisco, CA, United States
- 16Research and Collections, Natural History Museum of Denmark, University of Copenhagen, Copenhagen, Denmark
The complex, interconnected, and non-contiguous nature of canopy environments present unique cognitive, locomotor, and sensory challenges to their animal inhabitants. Animal movement through forest canopies is constrained; unlike most aquatic or aerial habitats, the three-dimensional space of a forest canopy is not fully realized or available to the animals within it. Determining how the unique constraints of arboreal habitats shape the ecology and evolution of canopy-dwelling animals is key to fully understanding forest ecosystems. With emerging technologies, there is now the opportunity to quantify and map tree connectivity, and to embed the fine-scale horizontal and vertical position of moving animals into these networks of branching pathways. Integrating detailed multi-dimensional habitat structure and animal movement data will enable us to see the world from the perspective of an arboreal animal. This synthesis will shed light on fundamental aspects of arboreal animals’ cognition and ecology, including how they navigate landscapes of risk and reward and weigh energetic trade-offs, as well as how their environment shapes their spatial cognition and their social dynamics.
Introduction
Forests cover over 30% of the world’s land area, and support the vast majority of land-based animal species (FAO and UNEP, 2020). In tropical forests, approximately 50–90% of vertebrates, and up to 75% of mammals, representing hundreds of species, make use of arboreal substrates (Kays and Allison, 2001; Malcolm and Lowman, 2004). Forest canopies are complex, dynamic, three-dimensional networks of intertwined branches and vines connecting at variable heights and angles, and with variable strengths and spacing. Because arboreal animals are generally constrained to moving along these substrates, the full three-dimensional space of the canopy is not available to them; they live their lives in “2.5D.” Canopy-dwelling animals (that do not fly) therefore face cognitive, locomotor, and sensory challenges that are not shared by ground-dwelling animals, nor by most aerial or aquatic animals whose substrates (air and water) are continuous in three-dimensional space. Exactly how the unusual 2.5D properties of forest canopy habitats shape the lives of the animals that inhabit them has been difficult to study, largely due to the inaccessibility of these treetop habitats to bipedal human observers.
Technological advances are poised to provide a new window through which we can observe and study arboreal species in their canopy habitat. Ground, aerial, and satellite-borne scanning technologies can produce digital reconstructions of complex forest habitats, allowing for the quantification and mapping of canopy branch networks. Combining multiple data-logging devices into small, wearable, on-animal sensors allows for the fine-scale tracking of animal movement in three dimensions. Cutting-edge computational modeling and virtual reality technologies now allow us to integrate these data streams—detailed habitat structure and animal movement data on both the horizontal and vertical axes—making it possible for us to see the world from the perspectives of arboreal animals. The development and refinement of these technologies is opening new avenues of study, and allowing us to ask new questions about how the unusual aspects of arboreal habitats shape the decision-making of canopy-dwelling animals.
A Virtual Window Into a 2.5D World
Forest canopies, and especially tropical rainforest canopies, are complex and dynamic networks of diverse trees and lianas. From the ground, we see a dense tangle of branches, vines, and epiphytes; it is nearly impossible to decipher where one plant ends and another begins. While these forests consist of multiple strata (Hallé et al., 2012)—layers that can be used to classify vertical patterns of vegetation and biodiversity—their boundaries are blurry and often indifferentiable from below. From above, we can see the irregular height of the forest canopy, with emergent trees reaching above a lower, denser layer of leafy canopy, but we are blind to the branching structures hidden below. Forests are dynamic, ever-changing, environments; branches break and fall, lianas and epiphytes get dislodged, trees die and eventually topple over—often pulling down, or crushing, others along the way (Cushman et al., 2022). From our boots on the ground, or our seats in the sky, we cannot fully appreciate, let alone accurately quantify or map, the complex structure of these arboreal habitats.
Technological advances allow for increasingly accurate and detailed mapping of complex canopy habitat structure. Light Detection and Ranging (LiDAR) systems can map the three-dimensional architecture of forests (Davies and Asner, 2014; Rodríguez-Ronderos et al., 2016; McClune, 2018; Moorthy et al., 2019), and when mounted on a drone or a plane, offer promising opportunities for balancing fine-scale and landscape-level measurements of tree and forest structure, canopy height, and vegetation density (Asner et al., 2008). Satellite-borne LiDAR systems can also measure vertical canopy structure at larger spatial scales, although with coarser resolution (e.g., Global Ecosystem Dynamics Investigation, GEDI) (Dubayah et al., 2020; Lang et al., 2021). Future advances that allow for higher spatial- and temporal-resolution forest and canopy structure data to be collected from satellites will enable the quantification and mapping of canopy structure over wider and longer scales. Although analytical challenges remain in moving from the point-clouds produced by LiDAR systems to full and robust representations of the three-dimensional networks of branches and lianas that connect the forest canopy (Okura, 2022), it is likely that we will, in the near future, have the ability to recreate detailed maps of these environments.
The parallel challenge is to situate animals and their movements within these detailed canopy maps (McLean et al., 2016; Davies et al., 2017). Unlocking the ability to precisely measure the vertical axis of movement is critical to this endeavor; current tracking of forest animals is largely confined to two dimensions, focusing on animal locations and tracks on the horizontal plane (e.g., Crofoot et al., 2010). There are technological hurdles to obtaining accurate, fine-scale vertical position data, but progress is being made. Vertical positioning signals can be extracted from GPS (Figure 1B), and can be used to assess questions about canopy use at gross scales (Thiel et al., 2021, e.g., niche stratification; Figure 1C), but may be too noisy to look at fine-scale movement decisions (Péron et al., 2020). Because the vertical axis of movement in a forest canopy is relatively limited—mean forest heights rarely exceed 40 m (Lefsky, 2010)—more precision is required to obtain relevant measures of vertical position for forest-dwelling animals than for many aerial and aquatic species, where three-dimensional tracking is already often used (e.g., Wilson et al., 2007; Bestley et al., 2015; Nagy et al., 2018). Barometers, by tracking air pressure, can detect small changes in height above ground, although calibration with nearby ground-station measurements is needed to account for weather-induced changes in air pressure (Sjöberg et al., 2018). Accelerometers, magnetometers, and gyroscopes can be used for dead reckoning, i.e., the fine-scale reconstruction of an animals’ movement paths through three dimensions (Bidder et al., 2015). The fusion of these data streams holds incredible promise for measuring the vertical axis of animal movement at the fine-scale that is needed for canopy-dwelling species. To date, this has not yet been attempted for arboreal species, but it is a promising avenue for future development.
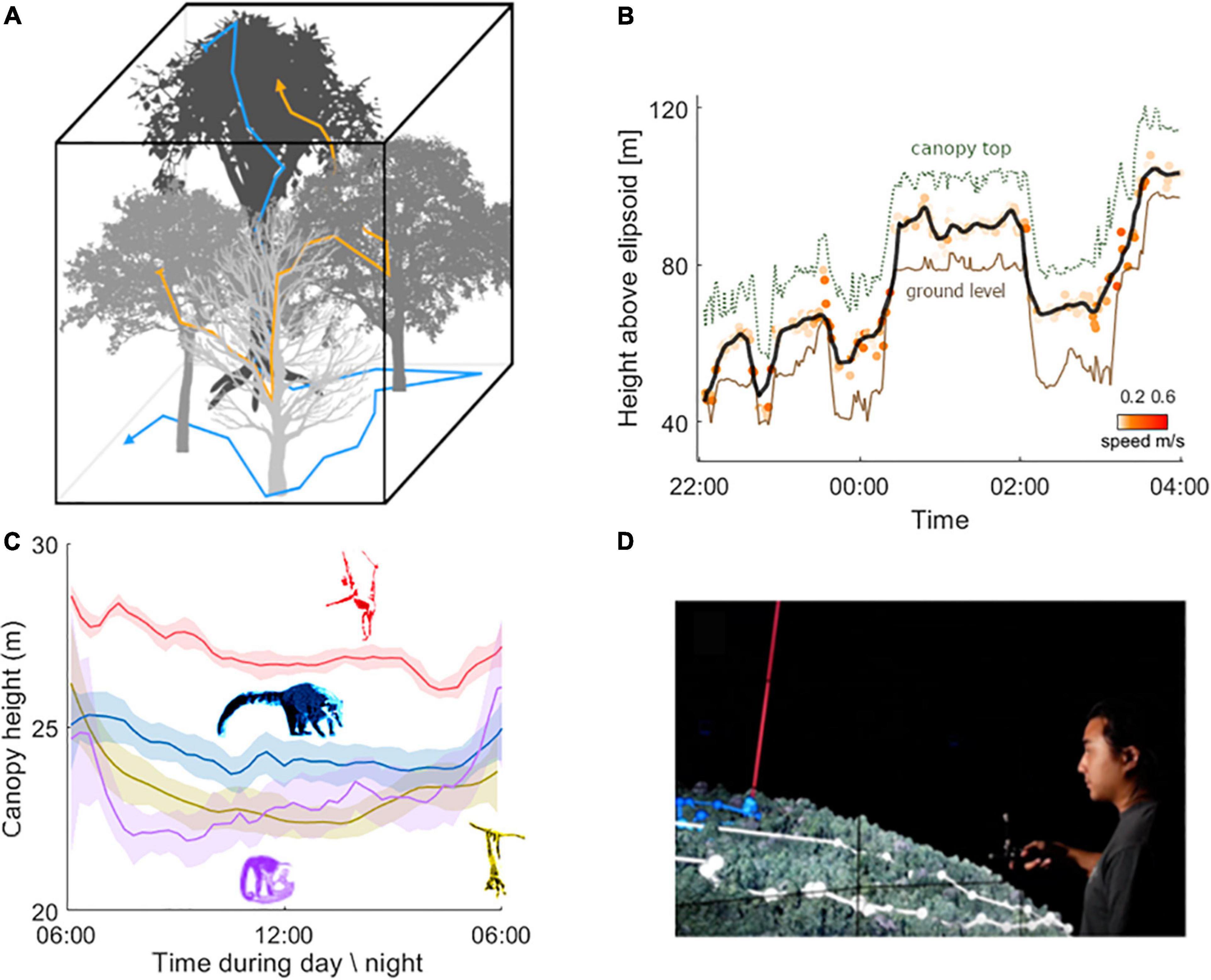
Figure 1. A virtual window into a 2.5D world. (A) Integrating three-dimensional animal movement tracks with the mapping of these complex three-dimensional habitats, will enable us to study how animals navigate the branching structures of their arboreal environments. (B) Vertical movement track over time of an arboreal kinkajou (Potos flavus) in reference to ground (brown solid line) tracked on Barro Colorado Island, Panama, and canopy height (green dashed line). GPS data were sampled at 1 Hz and dots represent 1-min median values. The solid black line represents a running median of the height measurements over a 15-min interval. (C) Based on the animal’s horizontal movements we can extract where in the canopy animals move over the course of the day. Spider monkeys (Ateles geoffroyi, red) tend to stay in higher canopy areas, while coatis (Nasua narica, blue), capuchins (Cebus capucinus, purple), and kinkajous (yellow) move through areas with lower canopy heights. The averages (and 95% CI shading) over the active period for four arboreal mammals species: coati (blue, N = 17 individuals), kinkajou (purple, N = 13 individuals), capuchin (yellow, N = 8 individuals), and spider monkey (red, N = 8 individuals) are presented. Each line represents the cumulative average values smoothed over 15-min of the individuals of a single species during the activity time of the individuals. Sources of DEM (digital elevation model) and canopy height data (Havmøller et al., 2021). Coatis sleep high in trees and descend to the ground in the mornings, forage on the ground during the day to return up into the trees at night (Kaufmann, 1962). Kinkajous and capuchins stay within the canopy and are known to only rarely come to the ground. Spider monkeys spend the majority of their day high in the canopy and have the least overall variation in vertical positioning. (D) Sensei-Panama visualization in CAVE2, visualizes animal movement trajectories within a virtual tropical environment, reconstructed from sensor and image data. Animal movements are shown with points connected by lines.
The question then becomes, “How can we use these data to develop and test meaningful hypotheses about the behavior and ecology of arboreal animals?” The solution may lie in virtual reality (VR), where emerging technologies are offering new avenues through which we can embed animals’ movement trajectories into reconstructions of their canopy habitat’s branching systems, and then not only “see” the choices that animals make but also “observe” counterfactuals, i.e., the options that were available but not chosen. For example, the “Sensei-Panama” application at the CAVE2 facility in the Electronic Visualization Laboratory, University of Illinois Chicago, allows observers to “fly through” a VR visualization of the 1560-ha tropical forest on Barro Colorado Island in Panama, reconstructed from depth-from-motion drone data and high-resolution aerial image data (Aurisano et al., 2019; Figure 1D). Within this virtually-reconstructed landscape, we can visualize the movement trajectories of GPS-tracked animals from the animal’s own point of view by “moving” along animal GPS tracks through the canopy. We can thus qualitatively and quantitatively assess our study animals’ movement decision-making, from their own points of view. Integrative and cutting-edge VR approaches need not be so large-scale and infrastructure-intensive, however. VR headsets are becoming increasingly accessible and powerful, and the democratization of this technology will enable its more widespread implementation.
Understanding Animal Decision-Making in a 2.5D World
The 2.5D nature of the forest canopy shapes many facets of arboreal animals’ lives. Integrating three-dimensional animal movement tracks with the mapping of these complex three-dimensional habitats (Figure 1A), will enable us to explore a whole new dimension of key questions related to animal navigation, cognition, and the mechanisms that underlie movement decisions.
How Do Animals Navigate the Vertical Distribution of Risk and Reward?
The vertical structuring of canopy habitats can have significant bearing on the spatial distribution of nutritional rewards. The nutritional quality of leaves (chlorophyll content) and fruits (sugar and protein content) is often higher where sun exposure is higher (Houle et al., 2014; Rothman et al., 2015), making the top of the canopy and forest edges desirable foraging areas. The potential benefits of accessing particular resources must, however, be weighed against potential costs, including predation risk (Gaynor et al., 2019), which also varies with height in the canopy; animals are more vulnerable to aerial predators when in high exposed areas, and to ground-dwelling predators when descending to the ground (Shultz et al., 2004; Makin et al., 2012). From studies focusing on two spatial dimensions, we know that animals’ movement is heavily influenced by the combined pressures of their landscapes of fear and the spatio-temporal distribution of important resources, such as food and nesting sites (Willems and Hill, 2009; Willems et al., 2009). But how do these competing forces interact with 2.5D environmental considerations—such as the animal’s height above ground, and the surrounding canopy branching structure—as well as energetic and safety considerations, to influence animal movement in three-dimensions? When and where do animals choose to climb higher, into more exposed areas (potentially increasing their risk of aerial predation) in order to access nutritionally higher-quality food sources? Similarly, are animals more likely to descend lower (potentially increasing their risk of ground-based predation) around forest edges or canopy gaps than elsewhere, to access unique resources that are only available—or that are more nutritious—in these boundary areas?
Arboreal animals must also consider the safety risks associated with potentially falling. While some travel route options may be more direct (i.e., energy- and time-efficient) and some foraging locations maybe more valuable (i.e., allow access to more nutrient-dense resources) they may present higher safety risks, such as a higher risk of falling from needing to jump across gaps or from relying on smaller and weaker branches (Pontzer and Wrangham, 2004). Evidence suggest that safety considerations have a strong effect on animal movement decisions: during vertical climbing, primates slow down and attempt to increase stability (Granatosky et al., 2019); and in species where canopy gaps are often navigated by jumping, larger-bodied individuals are more likely to choose an energetically expensive mode of circumventing canopy gaps (e.g., an indirect route) rather than risk jumping (Halsey et al., 2016). Furthermore, humans’ energetic costs while climbing are comparable to those of other animals, suggesting that morphological adaptations to arboreality may be the result of selection for safety, rather than energetic efficiency (Kozma and Pontzer, 2021). When and where do arboreal animals choose “riskier” travel pathways, and how does this vary between individuals, groups, and species? Species and individuals vary in their abilities to move across, over, under, and between different sizes, angles, rigidities, and strengths of arboreal substrates. Thus, the degree of risk incurred, and the energetic and time costs of travel, can be extremely variable—depending on the individuals and species, but also the structure of the habitat through which they are moving (Granatosky, 2018; Wheatley et al., 2021). How does variation in animals’ body characteristics (e.g., body size, limb length) and locomotor styles (e.g., quadrupedalism, brachiation, and leaping), interact with substrate availability and connectivity (e.g., branch characteristics and gap sizes) to shape locomotor choices, such as when to walk, leap, swing, reach or sway (e.g., Emmons and Gentry, 1983; Graham and Socha, 2020)?
Where ground-based predation pressure is comparatively low, such as predator-free islands, animal species that are elsewhere confined to the trees, may spend relatively more time on the ground (Ashbury et al., 2015; Monteza-Moreno et al., 2020)—suggesting that ground-use and ground-travel may have important benefits (e.g., access to particular nutritional resources, and energetically-efficient and safe travel). Indeed, ground-travel may be an energetically cheap option—especially for larger-bodied animals (Hanna and Schmitt, 2011)—that allows for direct navigation without any risk of falling (Janson, 1988). Conversely, in some cases, traveling through the canopy may be significantly less energetically expensive than ground travel, such as when moving through the trees allows an animal to buffer the effects of topography (e.g., when crossing ravines or narrow saddles), or when leveraging the biomechanical energy of compliant trees and branches (e.g., orangutans tree-swaying, Thorpe et al., 2007). Integrating the paradigms of energy landscapes and landscapes of fear into the three-dimensional study of arboreal animals’ movement decisions is a promising approach for better understanding how animals weigh predation risk against energetic benefits (Gallagher et al., 2017; Williams and Safi, 2021).
How Does Incomplete Substrate Connectivity Shape the Way That Animals See and Think About the World?
Arboreal animals face cognitive challenges that are specific to moving through the canopy. Compared to their ground-dwelling counterparts, arboreal animals’ cognitive maps may have to incorporate the vertical dimension—recognizing that the same location in horizontal space may look (and sound, and smell) very different at different heights in the forest. Adding to this potential cognitive challenge, in the trees, dense vegetation limits visual perception range (Janson and Di Bitetti, 1997). While this likely increases the importance of acoustic (Richards and Wiley, 1980), olfactory (Kuijper et al., 2014) cues, vegetation can be noisy enough to disrupt an animal’s ability to hear, and locate the source of, surrounding sounds (Yip et al., 2017), and can disrupt an animal’s ability to follow scent plumes due to high airflow turbulence (Garber and Hannon, 1993), and increase reliance on memory. Compared to animals that swim or fly, arboreal animals’ cognitive maps must account for the discontinuous and dynamic nature of their substrates; their movement decisions are constrained to the discrete pathways offered by trees’ and lianas’ inter-connectedness, and viable pathways may shift, change, emerge, or disappear gradually or suddenly over time (Pretzsch, 2009; Cushman et al., 2022). Furthermore, many horizontal and vertical movement options may not follow linear paths that lead directly or immediately in the intended direction of travel (Juliani et al., 2016). How do these challenges and constraints shape the spatial cognition of arboreal animals? Do arboreal animals have comparatively well-developed attentional filtering, memory, and reasoning to enable flexible and efficient search and navigation (as per Mueller and Fagan, 2008; Matzel and Kolata, 2010; Fagan et al., 2013)?
Animals might cognitively adapt to the constraints of an arboreal habitat by evolving an increased capacity for identifying local landmarks and repeating—through reinforcement learning—sequences of navigational decisions that result in efficient travel paths (Anggraini et al., 2018; Wu et al., 2021). Such adaptations would increase the ability to find and follow known, safe routes in the canopy without needing to keep track of the three-dimensional spatial relationships between those resources. Additional adaptations that aid in mentally modeling three-dimensional spatial relationships would improve an animal’s ability to navigate flexibly in response to changing canopy structure. For example, if arboreal animals are to keep track of their own position relative to out-of-sight locations, doing so in an extra dimension may require a larger working memory capacity and higher attunement to their body’s movement and position in space (proprioception) than that required by ground-dwelling animals (Heinze et al., 2018). Arboreal animals might also acquire spatially explicit information about the relationships between multiple locations by seeking out vantage points and integrating multiple perceptual vistas into a single cognitive map–behavior that would require some degree of meta-cognition and mental rotation of perceived scenes. Investigating the relationships between the connectedness and dynamism of animals’ arboreal habitats, and certain key aspects of their movement behavior—such as their frequency of route-use and the timing of their spatial information-seeking behavior—will shed light on the strategies that arboreal animals use to cope with the cognitive challenges of living in forest canopies.
How Does Canopy Structure Shape Social Dynamics?
For group living species, the vertical component and branching structures of their arboreal environments may play an important role in foraging competition and social dynamics (Boinski, 1989; Williamson et al., 2021). Competition for resources may be shaped by, not only the abundance and distribution of the resources themselves, but also the availability of pathways by which to even access these resources, with more dominant individuals monopolizing the branches that are within reach of the best foraging patches. Vertical stratification of group members may allow for increased within-group resource partitioning, allowing individuals to remain in proximity with each other but avoid direct conflicts over feeding space (Houle and Wrangham, 2021). Vertical stratification of group members may also block line-of-sight and interfere with within-group information transfer (Webster et al., 2013). How does vertical stratification enable or inhibit group cohesion and coordination?
Group cohesion and social interactions, while traveling, foraging and resting, may also be shaped by the structure of the forest canopy. The benefits of maintaining cohesion and engaging in these affiliative interactions with group-mates must be weighed against the safety of congregating in different canopy locations and the ability of all group members—including larger-bodied and heavier individuals, and individuals with reduced locomotor capacities, e.g., immatures—to access and move through these locations (as per del Mar Delgado et al., 2018; Jolles et al., 2020; Harel et al., 2021). How does variation in body size and locomotor capacity between individuals within a group, as well as the number of individuals in the group, interact with factors such as branch connectivity, strength and space, vulnerability to potential predators, access to resources, and the likelihood and potential severity of falling, to determine travel routes and congregation locations that are available to the group? Where a travel route or location is not accessible or safe for all group members, how are conflicts of interest handled?
Conclusion
The key to answering these questions lies in the development and integration of technological advances that enable detailed three-dimensional mapping of canopy habitat structure and arboreal animals’ movements on a very fine spatio-temporal scale (Nathan et al., 2022). No technology can compete with the richness of direct observation, but—particularly in cases where observation, or at least detailed quantification, is not possible—remote sensing technologies have a unique role to play in letting us see worlds that we could not otherwise see. Careful attention needs to be paid to how these technologies—e.g., on-animal collars, or low-flying drones—may influence the very behaviors we seek to study. With careful and ethical implementation, these emerging technologies will enable us to develop and reliably test meaningful hypotheses specific to the ecology, behavior, and cognition of canopy-dwelling animals.
Living in constrained three-dimensional environments is likely not unique to canopy-dwelling animals; animals that depend heavily, for example, on coral reefs and underground tunnels may face many similar challenges associated with complex and constrained three-dimensional habitats. Many aerial and aquatic species may also face movement constraints in their habitats, as the invisible structuring of currents in open air and aquatic environments may heavily influence the actual movement path choices that are available to them. By developing and integrating technologies that allow for the fine-scale reconstruction of complex habitats—be it forests or other—as well as the tracking of animal movement, we can achieve an animal’s-eye view of the challenges and choices associated with living in 2.5D.
Data Availability Statement
Publicly available datasets were analyzed in this study. Data are available upon request at “Movebank” (www.movebank.org).
Ethics Statement
The Institutional Animal Care and Use Committees (IACUC) at the Smithsonian Tropical Research Institute (protocol number 2014-1001-2017, 2017-0605-2020, and 2017-0912-2020) and the University of California, Davis (protocol number 18239) approved all animal handling and collaring procedures.
Author Contributions
MC, RK, BH, KM, and RH formulated the idea. RWH, MC, BH, and RK collected data. RH, AA, and CN prepared the figures. RH, AA, and MC lead the writing. All authors contributed to the text.
Funding
This project received funding from the National Science Foundation (BCS-1440755), a Packard Foundation Fellowship (2016-65130), and the Alexander von Humboldt Foundation in the framework of the Alexander von Humboldt Professorship endowed by the Federal Ministry of Education and Research awarded to MC. Further support for the project was also provided by the Center for the Advanced Study of Collective Behavior at the University of Konstanz, Deutsche Forschungsgemeinschaft Centre of Excellence 2117 (ID: 422037984).
Conflict of Interest
KM was employed by the company Science Communication Lab, Inc.
The remaining authors declare that the research was conducted in the absence of any commercial or financial relationships that could be construed as a potential conflict of interest.
The reviewer MD declared a past collaboration with one of the author SA to the handling editor.
Publisher’s Note
All claims expressed in this article are solely those of the authors and do not necessarily represent those of their affiliated organizations, or those of the publisher, the editors and the reviewers. Any product that may be evaluated in this article, or claim that may be made by its manufacturer, is not guaranteed or endorsed by the publisher.
References
Anggraini, D., Glasauer, S., and Wunderlich, K. (2018). Neural signatures of reinforcement learning correlate with strategy adoption during spatial navigation. Sci. Rep. 8, 1–14. doi: 10.1038/s41598-018-28241-z
Ashbury, A. M., Posa, M. R., Dunkel, L. P., Spillmann, B., Atmoko, S. S., van Schaik, C. P., et al. (2015). Why do orangutans leave the trees? Terrestrial behavior among wild bornean orangutans (pongo pygmaeus wurmbii) at tuanan, central kalimantan. Am. J. Primatol. 77, 1216–1229. doi: 10.1002/ajp.22460
Asner, G. P., Knapp, D. E., Kennedy-Bowdoin, T., Jones, M. O., Martin, R. E., Boardman, J., et al. (2008). Invasive species detection in hawaiian rainforests using airborne imaging spectroscopy and Lidar. Remote Sens. Env. 112, 1942–1955. doi: 10.1016/j.rse.2007.11.016
Aurisano, J., Hwang, J., Johnson, A., Long, L., Crofoot, M., and Berger-Wolf, T. (2019). “Bringing the field into the lab: large-scale visualization of animal movement trajectories within a virtual island,” in 2019 IEEE 9th Symposium on Large Data Analysis and Visualization (LDAV). (Piscataway, NJ: IEEE), 83–84.
Bestley, S., Jonsen, I. D., Hindell, M. A., Harcourt, R. G., and Gales, N. J. (2015). Taking animal tracking to new depths: synthesizing horizontal–vertical movement relationships for four marine predators. Ecology 96, 417–427. doi: 10.1890/14-0469.1
Bidder, O. R., Walker, J. S., Jones, M. W., Holton, M. D., Urge, P., Scantlebury, D. M., et al. (2015). Step by step: reconstruction of terrestrial animal movement paths by dead-reckoning. Mov. Ecol. 3:23. doi: 10.1186/s40462-015-0055-4
Boinski, S. (1989). The positional behavior and substrate use of squirrel monkeys: ecological implications. J. Human Evol. 18, 659–677. doi: 10.1016/0047-2484(89)90099-7
Crofoot, M. C., Lambert, T. D., Kays, R., and Wikelski, M. C. (2010). Does watching a monkey change its behaviour? Quantifying observer effects in habituated wild primates using automated radiotelemetry. Animal Behav. 80, 475–480. doi: 10.1016/j.anbehav.2010.06.006
Cushman, K. C., Detto, M., García, M., and Muller-Landau, H. C. (2022). Soils and Topography Control Natural Disturbance Rates And Thereby Forest Structure In A Lowland Tropical Landscape. Hoboken: Ecology Letters.
Davies, A. B., Ancrenaz, M., Oram, F., and Asner, G. P. (2017). Canopy structure drives orangutan habitat selection in disturbed bornean forests. Proc. Nat. Acad. Sci. 114, 8307–8312. doi: 10.1073/pnas.1706780114
Davies, A. B., and Asner, G. P. (2014). Advances in animal ecology from 3D-LiDAR ecosystem mapping. Trends Ecol. Evol 29, 681–691. doi: 10.1016/j.tree.2014.10.005
Delgado, M., Miranda, M., Alvarez, S. J., Gurarie, E., Fagan, W. F., Penteriani, V., et al. (2018). The importance of individual variation in the dynamics of animal collective movements. Philos. Trans. R. Soc. B Biol. Sci. 373:20170008. doi: 10.1098/rstb.2017.0008
Dubayah, R., Blair, J. B., Goetz, S., Fatoyinbo, L., Hansen, M., Healey, S., et al. (2020). The global ecosystem dynamics investigation: high-resolution laser ranging of the earth’s forests and topography. Sci. Remote Sens. 1:100002. doi: 10.1016/j.srs.2020.100002
Emmons, L. H., and Gentry, A. H. (1983). Tropical forest structure and the distribution of gliding and prehensile-tailed vertebrates. Am. Nat. 121, 513–524. doi: 10.1086/284079
Fagan, W. F., Lewis, M. A., Auger-Méthé, M., Avgar, T., Benhamou, S., Breed, G., et al. (2013). Spatial memory and animal movement. Ecol. Lett. 16, 1316–1329.
Gallagher, A. J., Creel, S., Wilson, R. P., and Cooke, S. J. (2017). Energy landscapes and the landscape of fear. Trends Ecol. Evol. 32, 88–96. doi: 10.1016/j.tree.2016.10.010
Garber, P. A., and Hannon, B. (1993). Modeling monkeys: a comparison of computer-generated and naturally occurring foraging patterns in two species of neotropical primates. Int. J. Primatol. 14, 827–852. doi: 10.1007/bf02220255
Gaynor, K. M., Brown, J. S., Middleton, A. D., Power, M. E., and Brashares, J. S. (2019). Landscapes of fear: spatial patterns of risk perception and response. Trends Ecol. Evol. 34, 355–368. doi: 10.1016/j.tree.2019.01.004
Graham, M., and Socha, J. J. (2020). Going the distance: the biomechanics of gap-crossing behaviors. J. Exp. Zool. Part A Ecol. Int. Physiol. 333, 60–73. doi: 10.1002/jez.2266
Granatosky, M. C. (2018). A review of locomotor diversity in mammals with analyses exploring the influence of substrate use, body mass and intermembral index in primates. J. Zool. 306, 207–216. doi: 10.1111/jzo.12608
Granatosky, M. C., Schmitt, D., and Hanna, J. (2019). Comparison of spatiotemporal gait characteristics between vertical climbing and horizontal walking in primates. J. Exp. Biol. 222:jeb185702. doi: 10.1242/jeb.185702
Hallé, F., Oldeman, R. A., and Tomlinson, P. B. (2012). Tropical Trees And Forests: An Architectural Analysis. Berlin: Springer.
Halsey, L. G., Coward, S. R., and Thorpe, S. K. (2016). Bridging the gap: parkour athletes provide new insights into locomotion energetics of arboreal apes. Biol. Lett. 12:20160608. doi: 10.1098/rsbl.2016.0608
Hanna, J. B., and Schmitt, D. (2011). Locomotor energetics in primates: gait mechanics and their relationship to the energetics of vertical and horizontal locomotion. Am. J. Biol. Anthropol. 145, 43–54. doi: 10.1002/ajpa.21465
Harel, R., Loftus, J. C., and Crofoot, M. C. (2021). Locomotor compromises maintain group cohesion in baboon troops on the move. Proc. Royal Soc. B 288:20210839.
Havmøller, L. W., Loftus, J. C., Havmøller, R. W., Alavi, S. E., Caillaud, D., Grote, M. N., et al. (2021). Arboreal monkeys facilitate foraging of terrestrial frugivores. Biotropica 53, 1685–1897. doi: 10.1111/btp.13017
Heinze, S., Narendra, A., and Cheung, A. (2018). Principles of insect path integration. Curr. Biol. 28, R1043–R1058. doi: 10.1016/j.cub.2018.04.058
Houle, A., and Wrangham, R. W. (2021). Contest competition for fruit and space among wild chimpanzees in relation to the vertical stratification of metabolizable energy. Anim. Behav. 175, 231–246. doi: 10.1016/j.anbehav.2021.03.003
Houle, A., Conklin-Brittain, N. L., and Wrangham, R. W. (2014). Vertical stratification of the nutritional value of fruit: macronutrients and condensed tannins. Am. J. Primatol. 76, 1207–1232. doi: 10.1002/ajp.22305
Janson, C. H. (1988). Food competition in brown capuchin monkeys (Cebus apella): quantitative effects of group size and tree productivity. Behaviour 105, 53–76. doi: 10.1163/156853988x00449
Janson, C. H., and Di Bitetti, M. S. (1997). Experimental analysis of food detection in capuchin monkeys: effects of distance, travel speed, and resource size. Behav. Ecol. Sociobiol. 41, 17–24. doi: 10.1007/s002650050359
Jolles, J. W., King, A. J., and Killen, S. S. (2020). The role of individual heterogeneity in collective animal behaviour. Trends Ecol. Evol. 35, 278–291. doi: 10.1016/j.tree.2019.11.001
Juliani, A. W., Bies, A. J., Boydston, C. R., Taylor, R. P., and Sereno, M. E. (2016). Navigation performance in virtual environments varies with fractal dimension of landscape. J. Env. Psychol. 47, 155–165. doi: 10.1016/j.jenvp.2016.05.011
Kaufmann, J. (1962). Ecology and the Social Behavior Of The Coati, Nasua Narica, On Barro Colorado Island, Panama. Berkeley: University of California Press, 60.
Kays, R., and Allison, A. (2001). Arboreal tropical forest vertebrates: current knowledge and research trends. Trop. Forest Can. Ecol. Manag. 153, 109–120. doi: 10.1007/978-94-017-3606-0_9
Kozma, E. E., and Pontzer, H. (2021). Determinants of climbing energetic costs in humans. J. Exp. Biol. 224:jeb234567. doi: 10.1242/jeb.234567
Kuijper, D. P., Verwijmeren, M., Churski, M., Zbyryt, A., Schmidt, K., Jêdrzejewska, B., et al. (2014). What cues do ungulates use to assess predation risk in dense temperate forests? PLoS One 9:e84607. doi: 10.1371/journal.pone.0084607
Lang, N., Kalischek, N., Armston, J., Schindler, K., Dubayah, R., and Wegner, J. D. (2021). Global canopy height estimation with GEDI LIDAR waveforms and Bayesian deep learning. Arxiv preprint 2103:03975.
Lefsky, M. A. (2010). A global forest canopy height map from the moderate resolution imaging spectroradiometer and the geoscience laser altimeter system. Geophys. Res. Lett. 37:L15401.
Makin, D. F., Payne, H. F., Kerley, G. I., and Shrader, A. M. (2012). Foraging in a 3-D world: how does predation risk affect space use of vervet monkeys? J. Mammal. 93, 422–428. doi: 10.1644/11-mamm-a-115.1
Malcolm, J. R., and Lowman, M. D. (2004). “Ecology and conservation of canopy mammals,” in Forest Canopies, eds Edn, eds M. D. Lowman, N. M. Nadkarni, and H. B. Rinker (New York:NY: Academic Press), 297–331. doi: 10.1016/b978-012457553-0/50021-6
Matzel, L. D., and Kolata, S. (2010). Selective attention, working memory, and animal intelligence. Neurosci. Biobehav. Rev. 34, 23–30. doi: 10.1016/j.neubiorev.2009.07.002
McClune, D. W. (2018). Joining the dots: reconstructing 3D environments and movement paths using animal-borne devices. Anim. Biotelemetry 6, 1–9.
McLean, K. A., Trainor, A. M., Asner, G. P., Crofoot, M. C., Hopkins, M. E., Campbell, C. J., et al. (2016). Movement patterns of three arboreal primates in a neotropical moist forest explained by LiDAR-estimated canopy structure. Landsc. Ecol. 31, 1849–1862. doi: 10.1007/s10980-016-0367-9
Monteza-Moreno, C. M., Crofoot, M. C., Grote, M. N., and Jansen, P. A. (2020). Increased terrestriality in a neotropical primate living on islands with reduced predation risk. J. Human Evol. 143:102768. doi: 10.1016/j.jhevol.2020.102768
Moorthy, S. M. K., Bao, Y., Calders, K., Schnitzer, S. A., and Verbeeck, H. (2019). Semi-automatic extraction of liana stems from terrestrial Lidar point clouds of tropical rainforests. ISPRS J. Photogramm. Remote Sens. 154, 114–126. doi: 10.1016/j.isprsjprs.2019.05.011
Mueller, T., and Fagan, W. F. (2008). Search and navigation in dynamic environments–from individual behaviors to population distributions. Oikos 117, 654–664. doi: 10.1111/j.0030-1299.2008.16291.x
Nagy, M., Couzin, I. D., Fiedler, W., Wikelski, M., and Flack, A. (2018). Synchronization, coordination and collective sensing during thermalling flight of freely migrating white storks. Philos. Trans. Royal Soc. B 373:20170011. doi: 10.1098/rstb.2017.0011
Nathan, R., Monk, C. T., Arlinghaus, R., Adam, T., Alós, J., Assaf, M., et al. (2022). Big-data approaches lead to an increased understanding of the ecology of animal movement. Science 375:eabg1780. doi: 10.1126/science.abg1780
Okura, F. (2022). 3D modeling and reconstruction of plants and trees: a cross-cutting review across computer graphics, vision, and plant phenotyping. Breed. Sci. 72:21074. doi: 10.1270/jsbbs.21074
Péron, G., Calabrese, J. M., Duriez, O., Fleming, C. H., García-Jiménez, R., Johnston, A., et al. (2020). The challenges of estimating the distribution of flight heights from telemetry or altimetry data. Anim. Biotelemetry 8, 1–13.
Pontzer, H., and Wrangham, R. W. (2004). Climbing and the daily energy cost of locomotion in wild chimpanzees: implications for hominoid locomotor evolution. J. Human Evol. 46, 315–333. doi: 10.1016/j.jhevol.2003.12.006
Pretzsch, H. (2009). “Forest dynamics, growth, and yield,” in Forest dynamics, growth and yield, ed. H. Pretzsch (Berlin: Springer), 1–39. doi: 10.1007/978-3-540-88307-4_1
Richards, D. G., and Wiley, R. H. (1980). Reverberations and amplitude fluctuations in the propagation of sound in a forest: implications for animal communication. Am. Nat. 115, 381–399. doi: 10.1086/283568
Rodríguez-Ronderos, M. E., Bohrer, G., Sanchez-Azofeifa, A., Powers, J. S., and Schnitzer, S. A. (2016). Contribution of lianas to plant area index and canopy structure in a Panamanian forest. Ecology 97, 3271–3277. doi: 10.1002/ecy.1597
Rothman, J. M., Chapman, C. A., Struhsaker, T. T., Raubenheimer, D., Twinomugisha, D., and Waterman, P. G. (2015). Long-term declines in nutritional quality of tropical leaves. Ecology 96, 873–878. doi: 10.1890/14-0391.1
Shultz, S., Noë, R., McGraw, W. S., and Dunbar, R. I. M. (2004). A community–level evaluation of the impact of prey behavioural and ecological characteristics on predator diet composition. Proc. R. Soc. B Biol. Sci. 271, 725–732. doi: 10.1098/rspb.2003.2626
Sjöberg, S., Pedersen, L., Malmiga, G., Alerstam, T., Hansson, B., Hasselquist, D., et al. (2018). Barometer logging reveals new dimensions of individual songbird migration. J. Avian Biol. 49:e01821. doi: 10.1111/jav.01821
Thiel, S., Tschapka, M., Heymann, E. W., and Heer, K. (2021). Vertical stratification of seed-dispersing vertebrate communities and their interactions with plants in tropical forests. Biol. Rev. 96, 454–469. doi: 10.1111/brv.12664
Thorpe, S. K. S., Crompton, R. H., and Alexander, R. M. (2007). Orangutans use compliant branches to lower the energetic cost of locomotion. Biol. Lett. 3, 253–256. doi: 10.1098/rsbl.2007.0049
UNEP (2020). The State of the World’s Forests 2020. Forests, Biodiversity and People. Nairobi: United Nations Environment Programme.
Webster, M. M., Atton, N., Hoppitt, W. J., and Laland, K. N. (2013). Environmental complexity influences association network structure and network-based diffusion of foraging information in fish shoals. Am. Nat. 181, 235–244. doi: 10.1086/668825
Wheatley, R., Buettel, J. C., Brook, B. W., Johnson, C. N., and Wilson, R. P. (2021). Accidents alter animal fitness landscapes. Ecol. Lett. 24, 920–934. doi: 10.1111/ele.13705
Willems, E. P., and Hill, R. A. (2009). Predator-specific landscapes of fear and resource distribution: effects on spatial range use. Ecology 90, 546–555. doi: 10.1890/08-0765.1
Willems, E. P., Barton, R. A., and Hill, R. A. (2009). Remotely sensed productivity, regional home range selection, and local range use by an omnivorous primate. Behav. Ecol. 20, 985–992. doi: 10.1093/beheco/arp087
Williams, H. J., and Safi, K. (2021). Certainty and integration of options in animal movement. Trends Ecol. Evol. 36, 990–999. doi: 10.1016/j.tree.2021.06.013
Williamson, R. E., Webb, S. E., Dubreuil, C., Lopez, R., Hernandez, S. C., Fedigan, L. M., et al. (2021). Sharing spaces: niche differentiation in diet and substrate use among wild capuchin monkeys. Anim. Behav. 179, 317–338. doi: 10.1016/j.anbehav.2021.06.002
Wilson, R. P., Liebsch, N., Davies, I. M., Quintana, F., Weimerskirch, H., Storch, S., et al. (2007). All at sea with animal tracks: methodological and analytical solutions for the resolution of movement. Deep Sea Res. Part II Top. Stud.Oceanography 54, 193–210. doi: 10.1016/j.dsr2.2006.11.017
Wu, Q., Xu, K., Wang, J., Xu, M., Gong, X., and Manocha, D. (2021). Reinforcement learning-based visual navigation with information-theoretic regularization. IEEE Robot. Autom. Lett. 6, 731–738. doi: 10.1109/lra.2020.3048668
Keywords: arboreal mammals, foraging, decision-making, navigation, forest
Citation: Harel R, Alavi S, Ashbury AM, Aurisano J, Berger-Wolf T, Davis GH, Hirsch BT, Kalbitzer U, Kays R, Mclean K, Núñez CL, Vining A, Walton Z, Havmøller RW and Crofoot MC (2022) Life in 2.5D: Animal Movement in the Trees. Front. Ecol. Evol. 10:801850. doi: 10.3389/fevo.2022.801850
Received: 25 October 2021; Accepted: 28 April 2022;
Published: 03 June 2022.
Edited by:
Dora Biro, University of Oxford, United KingdomReviewed by:
Richard Bon, Université Toulouse III Paul Sabatier, FranceAlain Houle, Jean-Eudes College, Canada
Miguel De Guinea, The Hebrew University of Jerusalem, Israel
Copyright © 2022 Harel, Alavi, Ashbury, Aurisano, Berger-Wolf, Davis, Hirsch, Kalbitzer, Kays, Mclean, Núñez, Vining, Walton, Havmøller and Crofoot. This is an open-access article distributed under the terms of the Creative Commons Attribution License (CC BY). The use, distribution or reproduction in other forums is permitted, provided the original author(s) and the copyright owner(s) are credited and that the original publication in this journal is cited, in accordance with accepted academic practice. No use, distribution or reproduction is permitted which does not comply with these terms.
*Correspondence: Roi Harel, cmhhcmVsQGFiLm1wZy5kZQ==