- 1Department of Aquatic Sciences and Assessment, Swedish University of Agricultural Sciences, Uppsala, Sweden
- 2School of Natural Resources, University of Nebraska–Lincoln, Lincoln, NE, United States
- 3The PRODEO Institute, San Francisco, CA, United States
- 4The Institute for Mental and Physical Health and Clinical Translation (IMPACT), Deakin University, Geelong, VIC, Australia
- 5Department of Agronomy and Horticulture, University of Nebraska-Lincoln, Lincoln, NE, United States
- 6Center for Resilience in Agricultural Working Lands, University of Nebraska-Lincoln, Lincoln, NE, United States
Human activity causes biome shifts that alter biodiversity and spatial resilience patterns. Rare species, often considered vulnerable to change and endangered, can be a critical element of resilience by providing adaptive capacity in response to disturbances. However, little is known about changes in rarity patterns of communities once a biome transitions into a novel spatial regime. We used time series modeling to identify rare avian species in an expanding terrestrial (southern) spatial regime in the North American Great Plains and another (northern) regime that will become encroached by the southern regime in the near future. In this time-explicit approach, presumably rare species show stochastic dynamics in relative abundance – this is because they occur only rarely throughout the study period, may largely be absent but show occasional abundance peaks or show a combination of these patterns. We specifically assessed how stochastic/rare species of the northern spatial regime influence aspects of ecological resilience once it has been encroached by the southern regime. Using 47 years (1968–2014) of breeding bird survey data and a space-for-time substitution, we found that the overall contribution of stochastic/rare species to the avian community of the southern regime was low. Also, none of these species were of conservation concern, suggesting limited need for revised species conservation action in the novel spatial regime. From a systemic perspective, our results preliminarily suggest that stochastic/rare species only marginally contribute to resilience in a new spatial regime after fundamental ecological changes have occurred.
Introduction
With ongoing environmental change in the Anthropocene, ecosystems are changing rapidly at local, regional, and global scales (Vellend et al., 2017). Such changes can alter their resilience and result in spatial regime shifts (Allen et al., 2016; Sundstrom et al., 2017). For example, multiple global change drivers (including climate warming, species invasions, changing agricultural working landscapes) within the Great Plains of North America affect ecosystems in a south-to-north pattern (Roberts et al., 2019). In the Great Plains, terrestrial regime shifts are especially pervasive and woody plant encroachment causes regime changes from historical grassland regimes to shrubland or woodland regimes (Engle et al., 2008). Entire ecoregions in the southern Great Plains have changed to woodlands in the last century, and many ecoregions in the Great Plains are increasingly at risk of fundamental ecological change and regime shifts in the future (Twidwell et al., 2013a; Wonkka et al., 2019; Pausas and Bond, 2020). Such change is already evident, for instance, in shifting geographic centers of species distributions (Hovick et al., 2016) and native and agricultural plant phenologies (Richardson et al., 2013).
However, this complexity of interacting stressors makes the unambiguous determination of mechanisms steering ecological dynamics difficult because they can confound patterns of both natural and anthropogenic change at multiple scales of space and time. High uncertainty around ecological dynamics further adds to this complexity. Despite this, similar dynamics and large-scale spatial change have been observed in other terrestrial (e.g., altitudinally climbing treelines in the subarctic and temperate regions of the northern hemisphere; Lu et al., 2021), freshwater (arctic boreal lakes; Kuhn and Butman, 2021), and marine environments (western Baltic Sea coast; Eklöf et al., 2020), which highlights the importance of fast broad-scale spatial ecosystems change in recent time.
Ecologists have had a long-lasting interest in the relationship between disturbances and the responses of ecological communities (Pickett and White, 1985; Reynolds, 1993; Brawn et al., 2001). Efforts over the last decades have focused on how the structure and function of species assemblages confer stability to disturbances at local and regional scales (Donohue et al., 2013; Fried-Petersen et al., 2020). This research is more recently extended to explore community-disturbance relationships from a spatial resilience (Allen et al., 2016) and spatial regimes (Sundstrom et al., 2017) perspective, which accounts for the complexity inherent in ecosystems and landscapes. Specifically, spatial resilience and regime concepts build on Holling’s (1973) definition of ecological resilience, an emergent phenomenon of complex systems that accounts for patterns and processes that manifest and operate at distinct spatiotemporal scales, threshold dynamics and non-linear, often abrupt change and the ability of these systems to exist in and shift between alternative regimes (Gunderson, 2000; Baho et al., 2017; Roberts et al., 2021). Understanding biome shifts from a spatial resilience perspective is crucial because once a spatial regime shifts to another regime, exemplified by grasslands changing to woodlands, recovery – a process included in ecological resilience (Allen et al., 2019) – to a previous regime is impeded and costly management required to emulate system conditions that existed prior to change (Angeler et al., 2020).
Historically, research emphasized the role of dominant species in driving biodiversity and community dynamics in response to environmental change, assuming implicitly that they are key players in ecological dynamics while de-emphasizing rare species. In fact, some modeling approaches routinely used by ecologists exclude rare species to not distort ordinations (e.g., canonical correspondence analysis) or disregard species that are not significantly related to model outcomes [e.g., correlated with canonical axes in redundancy analysis (RDA); but see Baker and King, 2010]. However, there is mounting evidence that rare species can play an important role in maintaining ecological pattern-process relationships (e.g., Lyons et al., 2005; Xiong et al., 2021). For instance, Mouillot et al. (2013) found that rare species in alpine meadows, coral reefs, and tropical forests comprised functional trait combinations that were not represented by abundant species. These authors suggested that if rare species go extinct, negative effects on ecosystem processes might result from the subsequent loss of ecosystems’ capacity to cope with disturbances. Such negative effects may occur even if species richness associated with abundant species in these systems is high (Mouillot et al., 2013).
The important role of rare species is further evident in their ability to replace dominant species after perturbation and maintain ecological functions in a grazed Australian rangeland (Walker et al., 1999). Another example, in tropical southwest Texas (United States) rare shrub species with larger root crowns than dominant species were able to compensate for the loss of dominant shrub species to mechanical disturbance by re-sprouting prolifically, thus maintaining a shrub-dominated system despite disturbance (Wonkka et al., 2016). These examples show that rare species may provide a relevant but, to some extent, unpredictable degree of an ecosystem’s capacity to adapt to change (i.e., adaptive capacity). However, due to their rarity, many species have been shown to be particularly vulnerable to environmental change and extinction (Colares et al., 2021). Yet, how rare species of one spatial regime influence a system’s adaptive capacity once it has shifted into a novel spatial regime is not well understood.
In this article, we study the relevance of rare species in the context of spatial resilience and regime change. Such change has recently been demonstrated with shifting biome frontiers in the Great Plains of North America, which were caused by global change (Roberts et al., 2019). We use time series modeling based on RDA, which in this specific modeling approach identifies “deterministic” and “stochastic” species (Baho et al., 2014). This approach is time-explicit which determines the individual temporal patterns of individual species which are then synthesized by the modeling. Presumably rare species show stochastic dynamics in relative abundance because they may occur only in low abundances throughout the study period, may largely be absent but show occasional abundance peaks or show a combination of these patterns. That is, these species are stochastic because they do not show redundant temporal patterns in relation to all other species in the communities. In contrast, deterministic species arise from redundant temporal patterns of individual species abundances, from which the modeling derives patterns of different species groups which differ from each other, based on different patterns of species redundancies in the communities.
From a purely modeling perspective deterministic species are those associated with the temporal scaling patterns identified by the model while stochastic species are unrelated to any scaling patterns identified (Baho et al., 2014). This distinction is specific to the time series modeling used here and may differ with other approaches. In this study we interpret stochastic and deterministic species as analogs of rare and more common or abundant species from an ecological perspective. We will complement our results by showing that stochastic species match to a large degree criteria of rare taxa according to definitions in biodiversity and ecological community research, which do consider time only implicitly (e.g., Gaston, 1994). Although this approach is preliminary and needs further research, it supports the use of the analogous comparison of stochastic and rare species at least in our study. Given the focus on rare species in this article and reconciling the modeling and ecological interpretation, we will refer to these species as “stochastic/rare” species.
We study the relevance of biome-level regime changes using breeding bird communities in the North American Great Plains that have been shown to be useful model systems for such changes (Roberts et al., 2019). We used a time-for-space substitution, an approach commonly used in ecology (Pickett, 1989), especially in a climate change context (Blois et al., 2013). Modern regime shifts often unfold at time scales that far exceed routine monitoring (Spanbauer et al., 2014). Space-for-time substitutions overcome this common problem in regime shift research by comparing spatially independent units that already occur in alternative regimes.
Because of the south-to-north movement of avian spatial regimes in the American Great Plains (Roberts et al., 2019), we assess how stochastic/rare bird species of a northern vulnerable regime may potentially influence stochastic/rare and common species patterns once it gets encroached by the expanding southern regime (Figure 1). In this context, the time series modeling allows us to assess two complementary aspects of spatial resilience, adaptive capacity and ecological resilience: (1) We assess whether stochastic/rare species remain stochastic/rare. Because of the role of stochastic/rare species providing adaptive capacity in response to disturbances in some systems (see above), our study allows us to assess patterns about the degree the stochastic/rare species of the vulnerable regime maintain their importance and contribution for adaptive capacity in the expanding southern spatial regime; (2) We study to what degree stochastic/rare species of the vulnerable spatial regime become likely more common and potentially manifest as “deterministic” in the time series models. This determinism provides insight in the contribution of these species to within-scale and cross-scale resilience attributes inherent in ecological resilience (Peterson et al., 1998) in the expanding spatial regime. We also assess the conservation status of stochastic/rare species contributing to the observed patterns to provide information about potential species vulnerabilities and conservation implications associated with spatial regime changes. The results may provide insight into the dynamically changing spatial resilience of landscapes and how this might affect management and conservation efforts for ecosystems and species with ongoing climate change (Cumming, 2011; Allen et al., 2016).
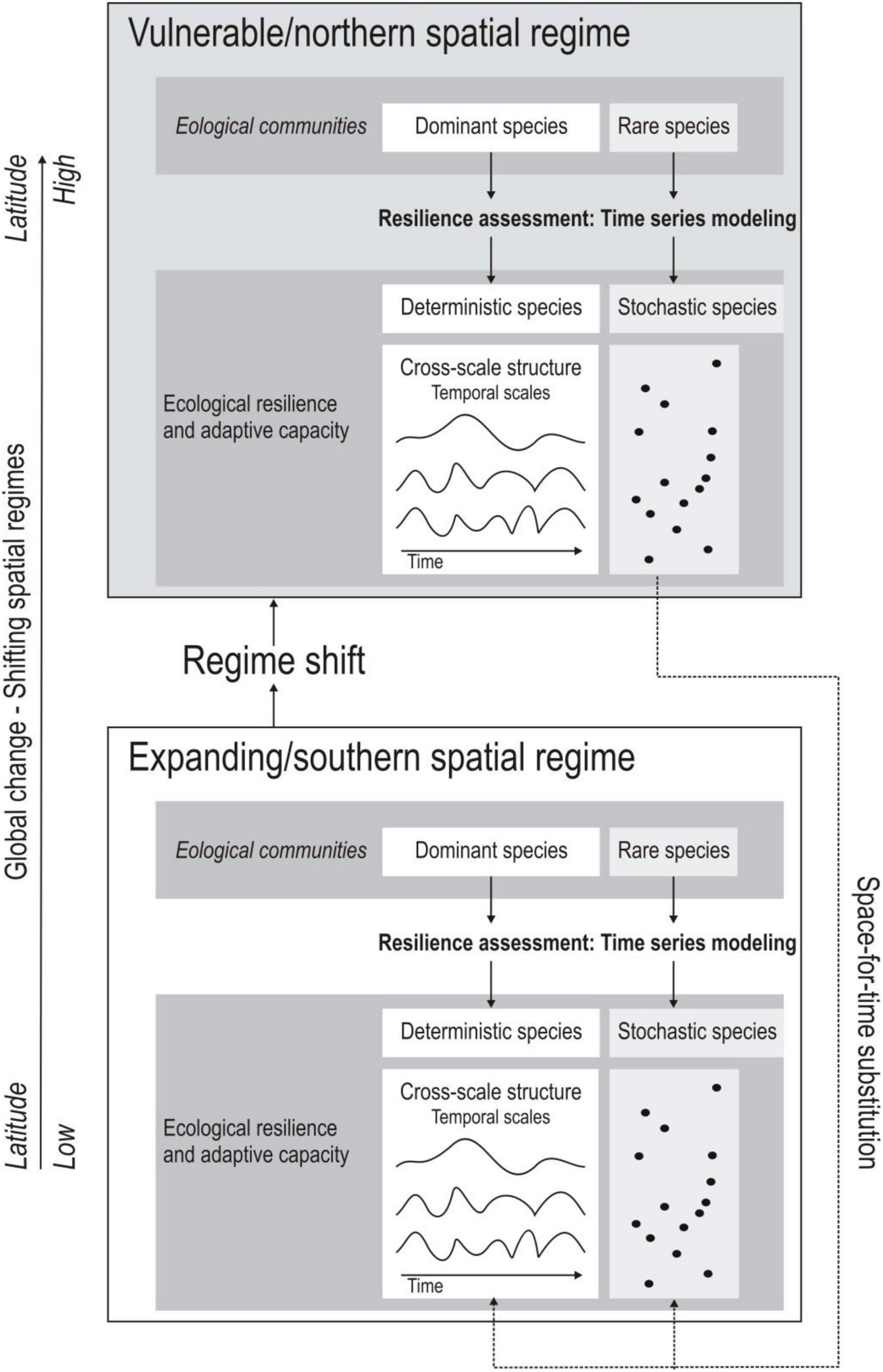
Figure 1. Schematic outlining study design. Time series models using avian communities are used to construct an expanding/southern and vulnerable/northern spatial regime. These regimes account for dominant and rare species with presumably deterministic (symbolized with 3 examples of groups of birds showing similar scale-specific fluctuation frequencies) and stochastic patterns (represented by dots symbolizing species) in the models. The relevance of stochastic species of the northern regime to become deterministic or stochastic once it has been encroached by the southern regime will be assessed.
Materials and Methods
Data and Study Setup
We collected 47 years of data ranging 1968–2014 from the United States Geological Survey breeding bird survey data (BBS) of North America1. The data contain avian community composition that is collected by qualified observers along georeferenced, permanent roadside routes across North America (Sauer et al., 2017). These data are publicly available. Along each ca 39.5 km route, observers make 50 stops once every 0.8 km where they conduct point-count surveys. During each survey, observers stand at the stop and record for 3 min the abundance of all bird species that are visually or aurally detected within a 0.4 km radius. Surveys start 30 min before local sunrise and last until the entire route is finished. To increase uniformity in probability of bird detection, surveys are conducted only on days with little or no rain, high visibility, and low wind.
We removed all aquatic species from the Anseriformes, Gaviiformes, Gruiformes, Pelecaniformes, Phaethontiformes, Phoenicopteriformes, Podicipediformes, Procellariiformes, and Suliformes families from the analysis because of known negative observation biases for waterfowl compared with terrestrial avian families (Veech et al., 2012). We also removed hybrids and unknowns, and we condensed subspecies to their respective species.
Time series data across routes were heterogeneous with many missing data, but three transects with continuous time series for the study period were identified and used for analyses for each of the two regimes studied. Specifically, we used a total of 6 transects, 3 for each spatial regime (Figure 2). Although the transects comprise a low sample number they were representative of their respective regimes: they comprised 82% of the total species pool in the southern regime and 86% in the northern regime. For each regime, we averaged for each year the counts of each species across the three transects. While the averaging resulted in a loss of site-specific temporal patterns of individual species, this approach allowed us to obtain exhaustive species occurrences and facilitated the comparison of species distributions between spatial regimes. For this study, we selected a southern (latitudes: 28.9–29.7; Western Gulf Coastal Plains) and a northern spatial regime (latitudes: 31.8–33.4; South Central Plains) for analyses (Figure 2). These regimes were chosen because of documented biogeographical shifts (northward movement of southern regimes) with ongoing climate change (Roberts et al., 2019).
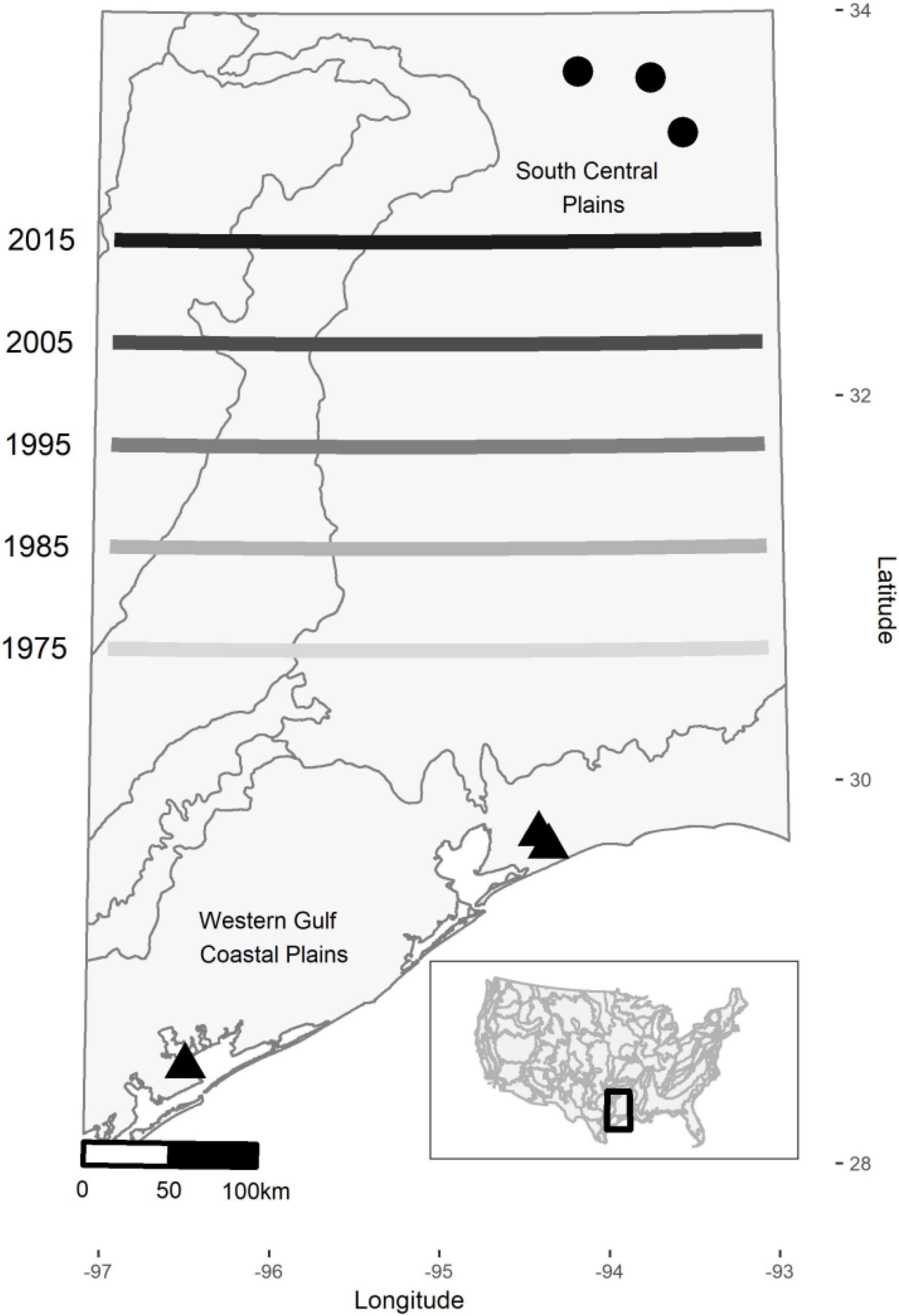
Figure 2. Map showing location of breeding bird survey transects in the southern (triangles: Transect 1, Route ID 13, Latitude/Longitude coordinates 28.52/−96.50; Transect 2, ID 20, Lat./Long. 29.67/−94.34; and Transect 3, ID 21, Lat./Long. 29.74/−94.40) and northern (circles: Transect 1, ID 5, Lat./Long. 33.38/−93.47; Transect 2, ID 6, Lat./Long 33.66/−93.67; and Transect 3, ID 7, Lat./Long. 33.69/−94.12) spatial regimes. Contours show level 3 eco-region delineations based on United States EPA and lines denote northward movements of the southern regime front over time assessed by Roberts et al. (2019).
Statistical Analysis
Time Series Models
Although methods exist that allow for analyses of temporal and space-time data (Legendre and Gauthier, 2014), we limited our data to time series modeling [RDA where time is modeled with Asymmetric Eigenvector Maps (AEM); Baho et al., 2015] of each spatial regime due to the low sample size of our data. All statistical analyses were carried out in R 3.0.2 (R Development Core Team, 2012) using the “aem.time” function [AEM package; Blanchet and Legendre (2013)], packages nlme (Pinheiro et al., 2008), and the “quick PCNM” function (PCNM package; Legendre et al., 2013). AEM were extracted from a set of orthogonal temporal variables that were calculated from the time vector consisting of 47 steps between years 1968 and 2014. These AEMs are used as explanatory variables to model temporal relationships in the BBS data. In the case of AEM, the first variable models linear trends and subsequent variables show sine-wave patterns (Legendre and Legendre, 2012), which allows assessing directional change and different inter-annual and decadal variation in the BBS. These extracted temporal variables are then used as explanatory variables in the time series models using RDA. Two time series models were constructed, one for the southern regime and one for the northern regime, which consisted of 122 species and 111 species, respectively, as response variables.
Redundancy analysis selects significant temporal variables (AEMs) using forward selection. The selected variables are linearly combined in the RDA models to extract temporal structures from the bird species matrices. The modeled temporal patterns that are extracted from the data are collapsed onto significant RDA axes, which are tested through permutation tests. These RDA axes are then used to distinguish deterministic from stochastic species in the analysis. The R software generates linear combination (lc) score plots, which visually present the modeled temporal patterns that are associated with each RDA axis. That is, individual RDA axes indicate fluctuation patterns at different temporal frequencies or scales. Because some time series models of individual transects were not significant, we averaged all bird species raw-abundances from three transects per regime and Hellinger-transformed them prior to the analyses of both spatial regimes (Legendre and Gallagher, 2001). Although specific information of individual transects in terms of numbers and identities of stochastic/rare species is lost due to the averaging, our analyses allows for obtaining biome-focused information about their role for spatial resilience and regime change.
Correlation of Bird Taxa With Modeled Spatial Patterns
We used Spearman rank correlation analysis to relate the temporal trends of raw abundances of individual bird taxa with the modeled temporal patterns (lc scores) associated with the RDA axes of both models. In this way we identified taxa that contributed significantly to the temporal dynamics revealed by the RDA (that is, deterministic species in the time series models which in ecological terms can be interpreted as more common, abundant species contributing to within-scale and cross-scale resilience show significant correlation with RDA axes). Those taxa that were not associated with any significant canonical axis were identified as stochastic/rare species that may presumably be important for adaptive capacity. Specifically, stochastic/rare species showed no significant correlations with any RDA axes revealed by the time series models in the Spearman rank correlation analyses.
Next, we examined whether stochastic/rare species from the northern regime occur as either deterministic and/or stochastic/rare patterns of the southern regime (dotted arrow in Figure 1). This provides insight regarding how species change may alter the spatial resilience of the northern regime once it is invaded by the southern regime with climate change. We ascertained that stochastic/rare species fulfill criteria of rarity using the quartile-method by Gaston (1994). Specifically, in this method the rarity cutoff point is the first quartile of species occurrences, i.e., rare species are the 25 percent of species with the lowest occurrence. We also assessed the conservation status of these species according to the IUCN Red List2 and the United States Endangered Species Act3.
Results
The analyses from the time series modeling of BBS data revealed significant temporal structure in both spatial regimes between 1968 and 2014. The overall variance explained by AEM models was moderately high (adjusted R2 values; northern regime, 0.65; and southern regime 0.63). The models revealed fluctuation patterns at distinct temporal frequencies or scales (Figure 3). AEM revealed temporal dynamics associated with five and six significant RDA axes for the northern and southern spatial regime, respectively. Comparing both regimes, the temporal patterns were similar at RDA 1 and RDA 2 and displayed a marked component of monotonic change in community composition and hump-bell shaped patterns, respectively. The remaining RDA axes indicated higher temporal variability of bird community structure within and between both regimes (Figure 3).
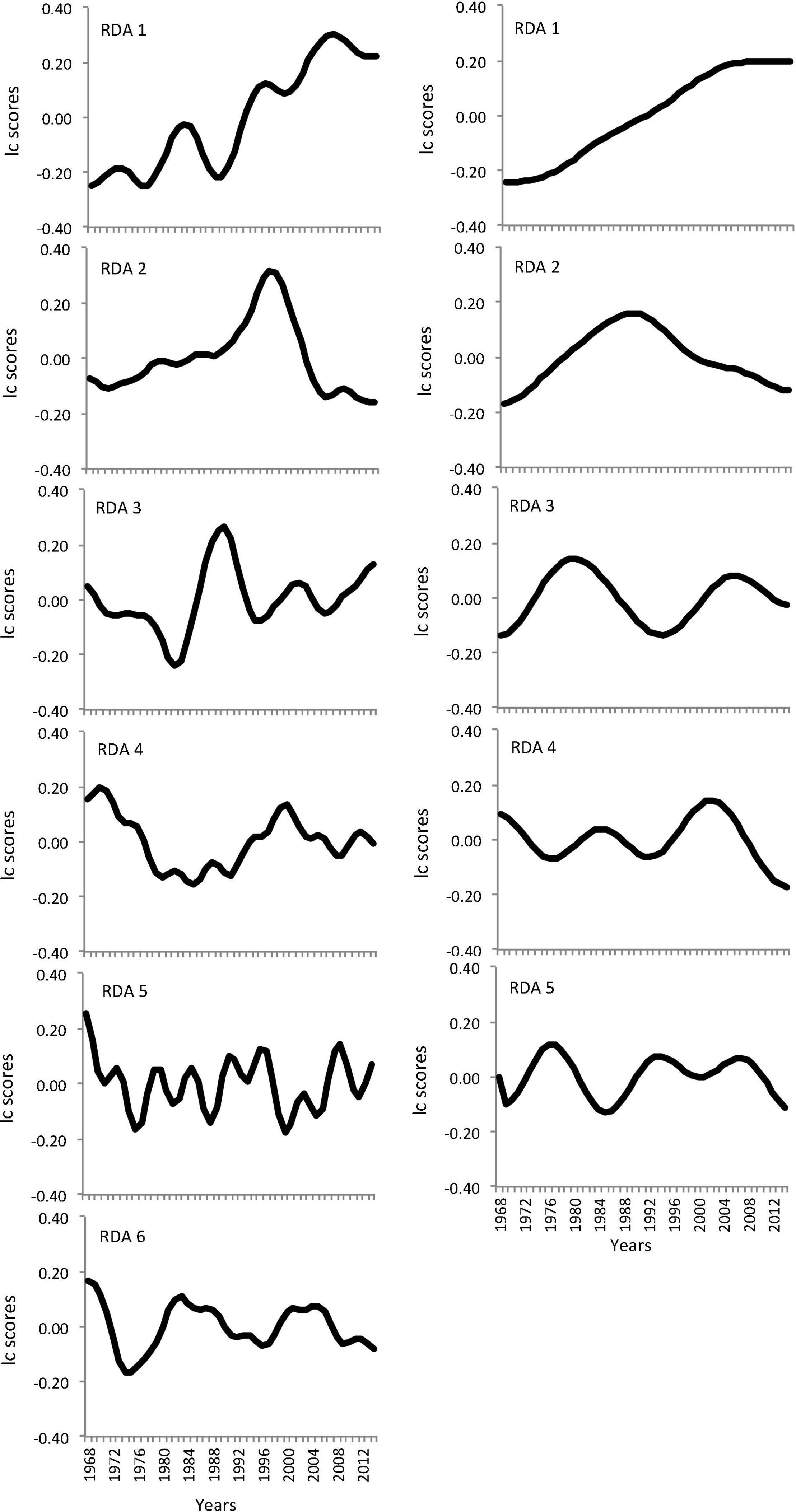
Figure 3. Linear combination (lc) score plots showing significant temporal patterns associated with different RDA axes in the time series models. Panels on the left represent the northern regime that becomes vulnerable to the invasion of the southern regime (right panels) due to climate change.
From the 122 bird species present in the southern regime, 87 (71%) were deterministic and 35 (29%) stochastic/rare. From the 110 taxa present in the northern regime, 86 species (78%) were deterministic, and 24 taxa (22%) stochastic/rare (Figure 4). Across deterministic species, most taxa were correlated with RDA 1 (56 northern regime, 45 southern regime), followed by RDA 2 (19 northern, 12 southern; Table 1). Only a few, generally fewer than 8 species, correlated with the remaining RDA axes, except RDA 4 (19 species) of the southern regime model (Table 1).
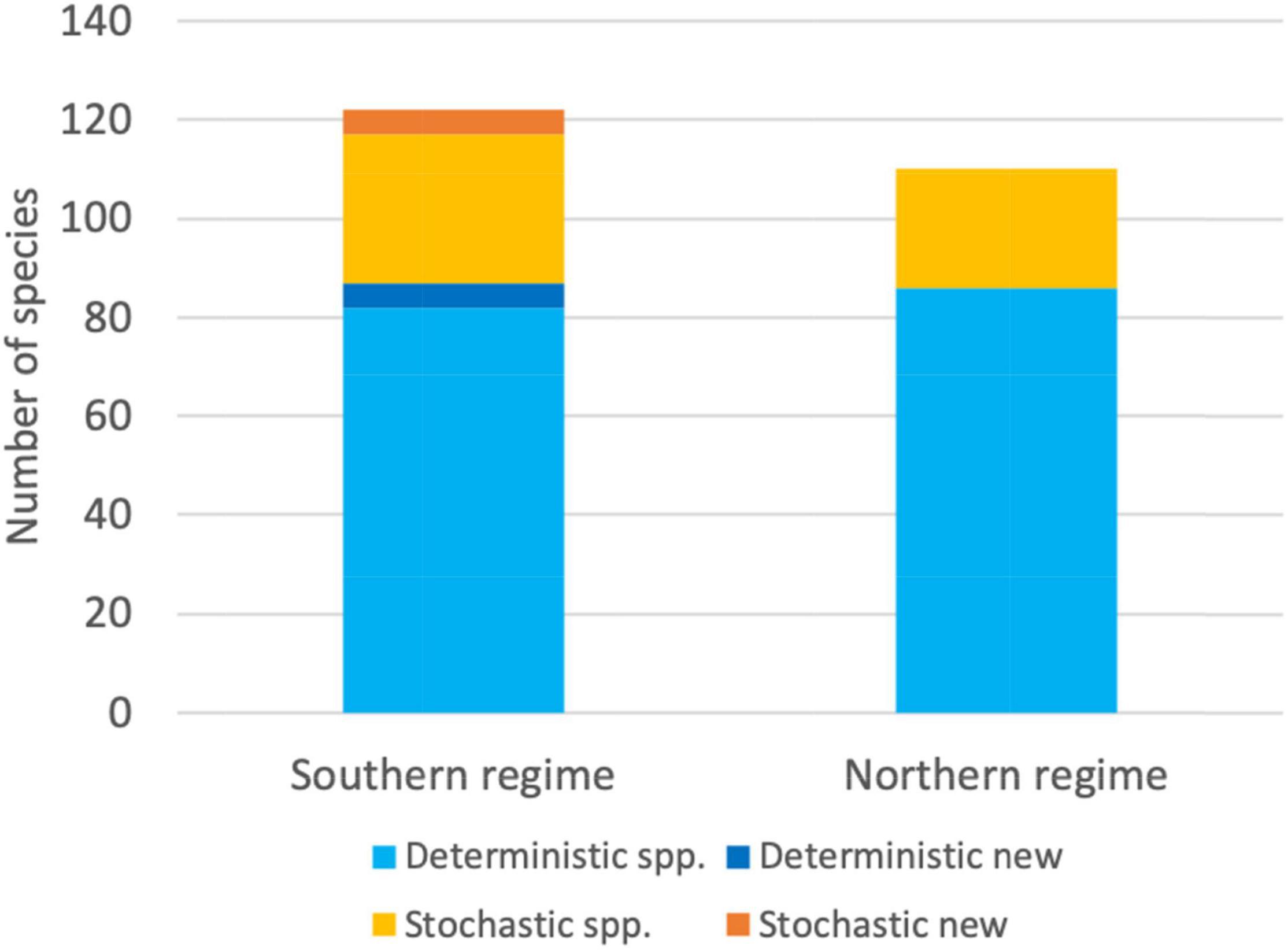
Figure 4. Bar plots showing the number of deterministic (light blue color) and stochastic/rare (yellow) species in the southern and northern spatial regime. Shown are also the stochastic/rare species from the northern regime that became deterministic (“Deterministic new”; dark blue) and remained stochastic/rare (“Stochastic new”, orange) in the southern spatial regime.

Table 1. Number of bird species correlating significantly (P ≤ 0.05) with significant RDA axes in time series models (i.e., deterministic species) of the northern and southern regimes.
Ten stochastic/rare species present in the northern regime were also found in the southern regime (Table 2). From these, 5 species (Thyromanes bewickii, Bubo virginianus, Pandion haliaetus, Tachycineta bicolor, and Vireo flavifrons) remained stochastic/rare and 5 species correlated with different RDA axis of the southern regime (Table 2). Specifically, Dumetella carolinensis and Buteo swainsoni correlated with RDA 1, Eremophila alpestris and Passerina ciris with RDA 2, and Setophaga americana with RDA 3 while no stochastic/rare species of the northern regime were found at RDAs 4, 5, and 6 of the southern regime model. This indicates that stochastic/rare species can become deterministic and contribute to within-scale and cross-scale resilience at different temporal fluctuation frequencies (i.e., temporal scales) in a new regime. None of these species were found to be of conservation concern according to the IUCN red list and the Endangered Species Act. Also, 7 of 10 stochastic/rare species match the rarity criterion according to Gaston (1994; Table 2 and Figure 5).
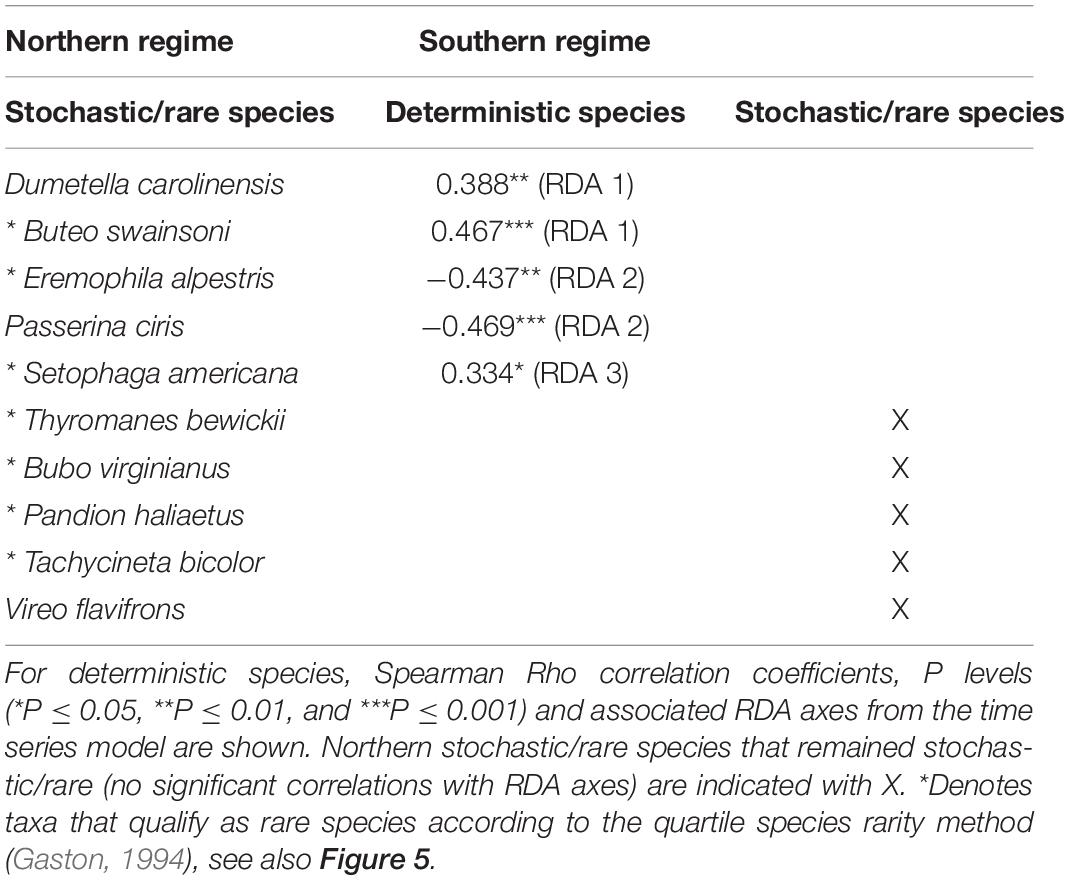
Table 2. Stochastic/rare species from the northern regime and their occurrences as deterministic or stochastic/rare species in the southern regime revealed by Spearman rank correlation analysis.
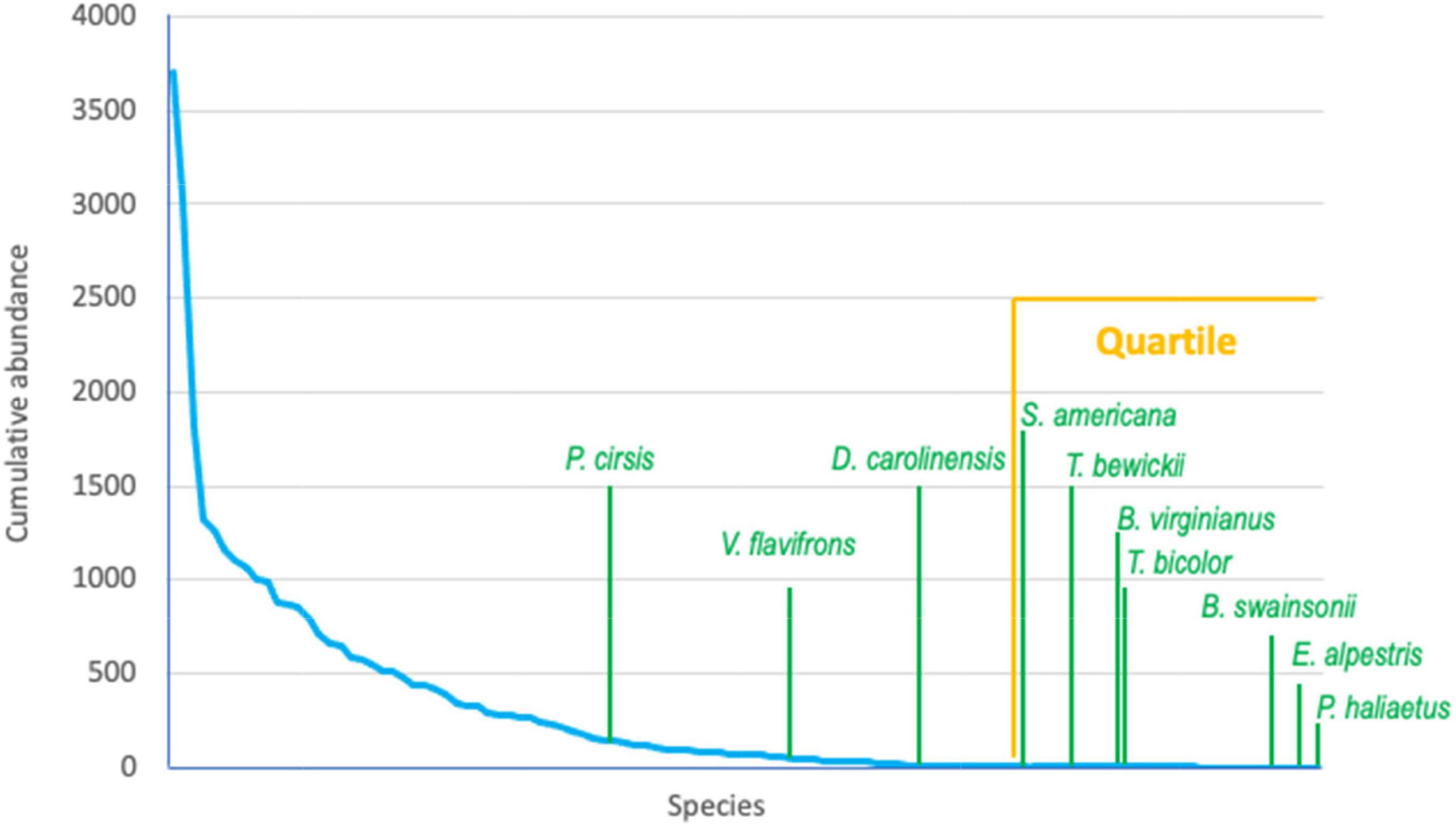
Figure 5. Accumulative species abundance curve of the northern spatial regime according to Gaston’s (1994) species rarity quartile method. Only taxon names for stochastic/rare avian species of the northern spatial regime that become stochastic/rare and deterministic in the expanding southern regime are shown. See also Table 2.
Discussion
We used time series modeling to assess two complementary aspects of spatial resilience: (1) we assessed whether stochastic/rare species remained stochastic/rare, thereby preserving their role for and contributing to adaptive capacity in an expanding spatial regime in the American Great Plains shown by Roberts et al. (2019), and (2) we assessed to what degree stochastic/rare species of a vulnerable spatial regime become deterministic and explain within-scale and cross-scale resilience attributes of ecological resilience in an expanding spatial regime.
Through the use of an analogy, the number of stochastic/rare species was used to estimate their potential role for adaptive capacity and within-scale and cross-scale resilience as proxies of ecological resilience in a novel regime. Results revealed that only 10 out of the 24 stochastic/rare species of the northern regime occurred in the southern regime. Five stochastic/rare species from the northern regime were also stochastic/rare in the southern regime. Of these species T. bewickii, V. flavifrons, and B. virginianus occur in woodlands, T. bicolor in open areas near water, while Pandion bicolor is a habitat generalist4. These range of habitat affinities indicate that stochasticity/rarity is not necessarily associated with a specific ecosystem type, which will be further discussed below.
Based on our analogy and previous research showing the importance of rare species to confer adaptive capacity in relation to disturbances (Walker et al., 1999; Mouillot et al., 2013; Wonkka et al., 2016), our findings suggest that the stochastic/rare species of the northern regime contribute little to the adaptive capacity in the southern regime. However, we acknowledge that the sample size of our study was low and the results therefore need to be viewed with caution.
Similarly, the other set of 5 stochastic/rare species from the northern regime were deterministic in the southern regime. Of these species S. americana and D. carolinensis are typical woodland species including urban woodlands. In contrast, P. ciris, B. swainsoni and E. alpestris are grassland and shrubland species (The Cornell Lab of Ornithology, 2022). Also, as was the case with stochastic/rare species remaining stochastic/rare, the different habitat preferences of these birds suggest that stochastic/rare species becoming deterministic after a spatial regime shift is not necessarily associated with a single ecosystem type. Such a result can be expected for both stochastic/rare and deterministic species because the Great Plains of North America are not dominated by a single habitat type and, for instance, conservation efforts (prescribed burning) contribute to maintaining open, grassland areas, and thus habitat heterogeneity, despite expanding woodlands (Twidwell et al., 2013b).
These five species were associated with three different temporal scales in the southern regime model. Because these scales were relatively species rich, our results suggest that these stochastic/rare species from the northern regime contribute a relatively low degree of within-scale and cross-scale resilience, two proxies of ecological resilience (Peterson et al., 1998), in the southern regime, although the results need to be interpreted with care. However, we were still able to derive some potentially important implications for ecological resilience.
Ecological resilience theory posits that (spatial) regime shifts can cause substantial structural and functional change and ecological reorganization (Angeler and Allen, 2016; Rietkerk et al., 2021). Notwithstanding, our results highlight that stochastic/rare species contribute little to the temporal patterns of the southern and norther regime. This is especially manifested in only 10 stochastic/rare species of the northern regime being represented in the bird community of 122 species of the southern regime. From an ecological community perspective our results suggest that stochastic/rare species per se enact limited structural change, suggesting that they play only a limited role in ecological reorganization in the Great Plains. This also suggests that stochastic/rare species from the northern regime may only leave a marginal “ecological legacy” (Johnstone et al., 2016) once the northern regime becomes invaded by the southern regime.
Contrary to the general notion that rare species dominate ecological communities (Magurran, 2013), our results show that deterministic species, i.e., those associated with significant RDA axes indicating the temporal scaling structure in the time series models, dominated the temporal dynamics in both spatial regimes, with the contribution of stochastic/rare species being below 29%. Although wider comparisons with other ecological communities and ecosystems cannot be made due to the lack of studies using the methodology of this study, this finding is preliminarily in agreement with a lake phytoplankton study, which found stochastic/rare species to contribute less than 34% to community structure (Baho et al., 2014). Research has suggested a relationship between the degree of disturbance and stochastic patterns in community dynamics (e.g., Måren et al., 2018; Santillan et al., 2019). While our study provided insight into how the consequences of disturbance (climate-change mediated biome shifts) influenced patterns of stochastic/rare species, we lacked additional data (environmental, biotic) for a more comprehensive assessments of factors mediating spatial resilience and regime change. Further research is required to provide a more detailed picture about the relationships between disturbances, resilience, regime shifts, stochastic processes, and the likely role of rare (and common) species in these processes.
Our study is based on space-for-time substitutions in which a regime change is only implicit. That is, rather than explicitly assessing regime changes resulting from northward migration of spatial regimes, our approach compared community dynamics between regimes, assuming that over time the northern regime will be encroached by the southern regime. While space-for-time substitutions have been criticized, they are still a valuable alternative to long-term studies (Pickett, 1989), particularly in a climate change context (Blois et al., 2013). Space-for-time substitutions are therefore particularly useful for regime shift research, which is often limited by monitoring data that do not cover relevant scales of ecological change, which can be slow, particularly in a spatial context. There is need to account for both space and time in the assessment of spatial resilience (Cumming, 2011; Allen et al., 2016; Sundstrom et al., 2017). This further underscores the utility of space-for-time substitutions as an important approach in spatial regime shift research, especially given the fast biogeographical changes on a rapidly changing planet.
We conclude by highlighting that none of the stochastic/rare bird species were of conservation concern according to the IUCN Red list and the United States Endangered Species Act. From the perspective of conservation biogeography, this suggests that likely no urgent adaptations of species conservation plans are needed once the northern regime becomes invaded by the southern regime. However, careful interpretation of this implication is needed because our study design and results are agnostic to the potential future biodiversity changes and threat status that may occur due to non-stationary change beyond the temporal horizon of our study.
Data Availability Statement
Publicly available datasets were analyzed in this study. This data can be found here: The data that support the findings of this study are openly available from the North American Breeding Bird Survey at URL https://www.pwrc.usgs.gov/BBS/RawData/, reference: https://doi.org/10.5066/P9HE8XYJ, citation: Pardieck KL, Ziolkowski DJ Jr., Lutmerding M, Aponte V and Hudson M-AR. 2019. North American Breeding Bird Survey Dataset 1966–2018, version 2018.0. United States Geological Survey, Patuxent Wildlife Research Center.
Author Contributions
All authors listed have made a substantial, direct, and intellectual contribution to the work, and approved it for publication.
Funding
This work was supported by Department of Defense Strategic Environmental Research Development Program W912HQ-15-C-0018, Nebraska Game and Parks Commission W-125-R-1 and the University of Nebraska-Lincoln’s Institute of Agriculture and Natural Resources. Additional support was provided by a sabbatical professorship to DGA by the University of Nebraska–Lincoln.
Conflict of Interest
The authors declare that the research was conducted in the absence of any commercial or financial relationships that could be construed as a potential conflict of interest.
Publisher’s Note
All claims expressed in this article are solely those of the authors and do not necessarily represent those of their affiliated organizations, or those of the publisher, the editors and the reviewers. Any product that may be evaluated in this article, or claim that may be made by its manufacturer, is not guaranteed or endorsed by the publisher.
Footnotes
- ^ https://www.usgs.gov/centers/eesc/science/north-american-breeding-bird-survey
- ^ https://www.iucnredlist.org/
- ^ https://www.fws.gov/endangered/species/
- ^ www.animaldiversity.org, www.allaboutbirds.org
References
Allen, C. R., Angeler, D. G., Chaffin, B., Twidwell, D., and Garmestani, A. (2019). Resilience reconciled. Nat. Sustain. 2, 898–900. doi: 10.1038/s41893-019-0401-4
Allen, C. R., Angeler, D. G., Cumming, G. S., Folke, C., Twidwell, D., and Uden, D. R. (2016). Quantifying spatial resilience. J. Appl. Ecol. 53, 625–635. doi: 10.1111/1365-2664.12634
Angeler, D. G., Chaffin, B., Sundstrom, S., Garmestani, A., Pope, K., Uden, D., et al. (2020). Coerced regimes: management challenges in the Anthropocene. Ecol. Soc. 25:4. doi: 10.5751/es-11286-250104
Baho, D. L., Allen, C. R., Garmestani, A. S., Fried-Petersen, H. B., Renes, S. E., Gunderson, L., et al. (2017). A quantitative framework for assessing ecological resilience. Ecol. Soc. 22:17. doi: 10.5751/ES-09427-220317
Baho, D. L., Drakare, S., Johnson, R. K., Allen, C. R., and Angeler, D. G. (2014). Similar resilience attributes in lakes with different management practices. PLoS One 9:e91881. doi: 10.1371/journal.pone.0091881
Baho, D. L., Futter, M. N., Johnson, R. K., and Angeler, D. G. (2015). Assessing temporal scales in time series: comparing methods based on redundancy analysis. Ecol. Complex. 22, 162–168. doi: 10.1186/s12868-016-0283-6
Baker, M. E., and King, R. S. (2010). A new method for detecting and interpreting biodiversity and ecological community thresholds. Methods Ecol. Evol. 1, 25–37. doi: 10.1111/j.2041-210x.2009.00007.x
Blanchet, F. G., and Legendre, P. (2013). AEM: tools to construct asymmetric eigenvector maps (AEM) spatial variablesIn: R Package Version 0.5-1/r118. Available Online at: http://R-Forge.R-project.org/projects/sedar/
Blois, J. L., Williams, J. W., Fitzpatrick, M. C., Jackson, S. T., and Ferrier, S. (2013). Space can substitute for time in predicting climate-change effects on biodiversity. Proc. Natl. Acad. Sci. U. S. A. 110, 9374–9379. doi: 10.1073/pnas.1220228110
Brawn, J. D., Robinson, S. K., and Thompson, F. R. III (2001). The role of disturbance in the ecology and conservation of birds. Annu. Rev. Ecol. Syst. 32, 251–276. doi: 10.1146/annurev.ecolsys.32.081501.114031
Colares, L. F., Lobato, C. M. C., Montag, L. F. D. A., and Dunck Oliveira, B. (2021). Extinction of rare fish predicts an abrupt loss of ecological function in the future of Amazonian streams. Freshw. Biol. 67, 1–12. doi: 10.1111/fwb.13839
Cumming, G. S. (2011). Spatial Resilience In Social-Ecological Systems. Berlin: Springer Science & Business Media.
Donohue, I., Petchey, O. L., Montoya, J. M., Jackson, A. L., McNally, L., Viana, M., et al. (2013). On the dimensionality of ecological stability. Ecol. Lett. 16, 421–429.
Eklöf, J. S., Sundblad, G., Erlandsson, M., Donadi, S., Hansen, J. P., Klemens, B., et al. (2020). A spatial regime shift from predator to prey dominance in a large coastal ecosystem. Commun. Biol. 3:459. doi: 10.1038/s42003-020-01180-0
Engle, D. M., Coppedge, B. R., and Fuhlendorf, S. D. (2008). “From the Dust Bowl to the Green Glacier: Human Activity and Environmental Change in Great Plains Grasslands,” in Western North American Juniperus Communities. Ecological Studies, ed. O. W. Van Auken (New York, NY: Springer).
Fried-Petersen, H. B., Araya-Ajoy, Y. G., Futter, M. N., and Angeler, D. G. (2020). Drivers of long-term invertebrate community stability in changing Swedish lakes. Glob. Change Biol. 26, 1259–1270. doi: 10.1111/gcb.14952
Gaston, K. J. (1994). “What is rarity?,” in Rarity. Population and Community Biology Series, Vol. 13, eds W. E. Kunin and K. J. Gaston (Dordrecht: Springer).
Gunderson, L. H. (2000). Ecological resilience—in theory and application. Annu. Rev. Ecol. Syst. 31, 425–439. doi: 10.1146/annurev.ecolsys.31.1.425
Holling, C. S. (1973). Resilience and stability of ecological systems. Annu. Rev. Ecol. Syst. 4, 1–23. doi: 10.1007/978-3-030-54560-4_1
Hovick, T. J., Allred, B. W., McGranahan, D. A., Palmer, M. W., Elmore, R. D., Fuhlendorf, S. D., et al. (2016). Informing conservation by identifying range shift patterns across breeding habitats and migration strategies. Biodiv. Conserv. 25, 345–356. doi: 10.1007/s10531-016-1053-6
Johnstone, J. F., Allen, C. D., Franklin, J. F., Frelich, L. E., Harvey, B. J., Higuera, P. E., et al. (2016). Changing disturbance regimes, ecological memory, and forest resilience. Front. Ecol. Environ. 14, 369–378. doi: 10.1002/fee.1311
Kuhn, C., and Butman, D. (2021). Declining greenness in Arctic-boreal lakes. Proc. Natl. Acad. Sci. U. S. A. 118:e2021219118. doi: 10.1073/pnas.2021219118
Legendre, P., Borcard, D., Blanchet, F. G., and Dray, S. (2013). PCNM: MEM spatial eigenfunction and principal coordinate analyses. In: R Package Version 2.1-2/r109. Available Online at: http://R-Forge.R-project.org/projects/sedar/
Legendre, P., and Gallagher, E. D. (2001). Ecologically meaningful transformations for ordination of species data. Oecologia 129, 271–280. doi: 10.1007/s004420100716
Legendre, P., and Gauthier, O. (2014). Statistical methods for temporal and space-time analysis of community composition data. Proc. Royal Soc. B: Biol. Sci. 281:20132728. doi: 10.1098/rspb.2013.2728.
Lu, X., Liang, E., Wang, Y., Babst, F., and Camarero, J. J. (2021). Mountain treelines climb slowly despite rapid climate warming. Glob. Ecol. Biogeogr. 30, 305–315. doi: 10.1111/geb.13214
Lyons, K. G., Brigham, C. A., Traut, B. H., and Schwartz, M. W. (2005). Rare species and ecosystem functioning. Conserv. Biol. 19, 1019–1024. doi: 10.1111/j.1523-1739.2005.00106.x
Måren, I. E., Kapfer, J., Aarrestad, P. A., Grytnes, J. -A., and Vandvik, V. (2018). Changing contributions of stochastic and deterministic processes in community assembly over a successional gradient. Ecology 99, 148–157. doi: 10.1002/ecy.2052
Mouillot, D., Bellwood, D. R., Barlato, C., Chave, J., Galzin, R., Harmelin-Vivien, M., et al. (2013). Rare species support vulnerable functions in high-diversity ecosystems. PLoS Biol. 11:e1001569. doi: 10.1371/journal.pbio.1001569
Pausas, J. G., and Bond, W. J. (2020). Alternative biome states in terrestrial ecosystems. Trends Plant Sci. 25, 250–263. doi: 10.1016/j.tplants.2019.11.003
Peterson, G., Allen, C. R., and Holling, C. S. (1998). Ecological resilience, biodiversity, and scale. Ecosystems 1, 6–18. doi: 10.1007/s100219900002
Pickett, S. T. A. (1989). “Space-for-Time Substitution as an Alternative to Long-Term Studies,” in Long-Term Studies in Ecology, ed. G. E. Likens (New York, NY: Springer).
Pickett, S. T. A., and White, P. S. (1985). Ecology of natural disturbance and patch dynamics. Cambridge, Massachusetts: Academic Press.
Pinheiro, J., Bates, D., DebRoy, S., Sarkar, D., and R Core Team (2008). nlme: Linear and Nonlinear Mixed Effects Models. R Package Version 3.1-120. Available Online at: https://CRAN.R-project.org/package=nlme
R Development Core Team (2012). R: A Language and Environment for Statistical Computing. Vienna, Austria: R Foundation for Statistical Computing.
Reynolds, C. S. (1993). “Scales of disturbance and their role in plankton ecology,” in Intermediate Disturbance Hypothesis in Phytoplankton Ecology, eds J. Padisák, C. S. Reynolds, and U. Sommer (Dordrecht: Springer), 157–171. doi: 10.1007/978-94-017-1919-3_15
Richardson, A. D., Keenan, T. F., Migliavacca, M., Ryu, Y., Sonnentag, O., Toomey, M., et al. (2013). Climate change, phenology, and phenological control of vegetation feedbacks to the climate system. Agric. For. Meteorol. 169, 156–173. doi: 10.1016/j.agrformet.2012.09.012
Rietkerk, M., Bastiaansen, R., Banerjee, S., van de Koppel, J., Baudena, M., and Doelman, A. (2021). Evasion of tipping in complex systems through spatial pattern formation. Science 374:eabj0359. doi: 10.1126/science.abj0359
Roberts, C. P., Allen, C. R., Angeler, D. G., and Twidwell, D. (2019). Shifting avian spatial regimes in a changing climate. Nat. Clim. Change 9, 562–566. doi: 10.1038/s41558-019-0517-6
Roberts, C. P., Uden, D. R., Cady, S. M., Allred, B., Fuhlendorf, S., Jones, M. O., et al. (2021). Tracking spatial regimes as an early warning for a species of conservation concern. Ecol. Appl. 32:e02480. doi: 10.1002/eap.2480
Santillan, E., Seshan, H., Constancias, F., Drautz-Moses, D. I., and Wuertz, S. (2019). Frequency of disturbance alters diversity, function, and underlying assembly mechanisms of complex bacterial communities. NPJ Biofilms Microbiomes 5:8. doi: 10.1038/s41522-019-0079-4
Sauer, J. R., Niven, D. K., Hines, J. E., Ziolkowski, D. J. Jr., Pardieck, K. L., and Fallon, J. E. (2017). The North American Breeding Bird Survey, Results and Analysis 1966 - 2015 (Version 2.07.2017). Laurel, MD: USGS Patuxent Wildlife Research Center.
Spanbauer, T. L., Allen, C. R., Angeler, D. G., Eason, T., Fritz, S. C., Garmestani, A. S., et al. (2014). Prolonged instability prior to a regime shift. PLoS One 9:e108936. doi: 10.1371/journal.pone.0108936
Sundstrom, S. M., Eason, T., Nelson, R. J., Angeler, D. G., Barichievy, C., Garmestani, A. S., et al. (2017). Detecting spatial regimes in ecosystems. Ecol. Lett. 20, 19–32. doi: 10.1111/ele.12709
The Cornell Lab of Ornithology (2022). The Cornell Lab of Ornithology. Ithaca, NY, USA: The Cornell Lab of Ornithology.
Twidwell, D., Allred, B. W., and Fuhlendorf, S. D. (2013a). National-scale assessment of ecological content in the world’s largest land management framework. Ecosphere 4, 1–27.
Twidwell, D., Rogers, W. E., Fuhlendorf, S. D., Wonkka, C. L., Engle, D. M., and Weir, J. R. Q. (2013b). The rising Great Plains fire campaign: citizens’ response to woody plant encroachment. Front. Ecol. Environ. 11, e64–e71. doi: 10.1890/130015
Veech, J. A., Small, M. F., and Baccus, J. T. (2012). Representativeness of land cover composition along routes of the North American Breeding Bird Survey. Auk 129, 259–267. doi: 10.1525/auk.2012.11242
Vellend, M., Baeten, L., Becker-Scarpitta, A., Boucher-Lalonde, V., McCune, J. L., Messier, J., et al. (2017). Plant biodiversity change across scales during the Anthropocene. Annu. Rev. Plant Biol. 68, 563–586. doi: 10.1146/annurev-arplant-042916-040949
Walker, B., Kinzig, A., and Langridge, J. (1999). Plant attribute diversity, resilience, and ecosystem function: the nature and significance of dominant and minor species. Ecosystems 2, 95–113. doi: 10.1007/s100219900062
Wonkka, C. L., Twidwell, D., Allred, B. W., Bielski, C. H., Donovan, V. M., Roberts, C. P., et al. (2019). Rangeland vulnerability to state transition under global climate change. Clim. Change 153, 59–78. doi: 10.1007/s10584-018-02365-7
Wonkka, C. L., Twidwell, D., West, J. B., and Rogers, W. E. (2016). Shrubland resilience varies across soil types: implications for operationalizing resilience in ecological restoration. Ecol. Appl. 26, 128–145. doi: 10.1890/15-0066
Keywords: rare species, regime shifts, biome change, biogeography, conservation, spatial regimes, resilience
Citation: Angeler DG, Roberts CP, Twidwell D and Allen CR (2022) The Role of Rare Avian Species for Spatial Resilience of Shifting Biomes in the Great Plains of North America. Front. Ecol. Evol. 10:849944. doi: 10.3389/fevo.2022.849944
Received: 06 January 2022; Accepted: 04 April 2022;
Published: 27 April 2022.
Edited by:
Jasmine Lee, British Antarctic Survey (BAS), United KingdomReviewed by:
Hannah Wauchope, University of Exeter, United KingdomJaime Albino Ramos, University of Coimbra, Portugal
Copyright © 2022 Angeler, Roberts, Twidwell and Allen. This is an open-access article distributed under the terms of the Creative Commons Attribution License (CC BY). The use, distribution or reproduction in other forums is permitted, provided the original author(s) and the copyright owner(s) are credited and that the original publication in this journal is cited, in accordance with accepted academic practice. No use, distribution or reproduction is permitted which does not comply with these terms.
*Correspondence: David G. Angeler, ZGF2aWQuYW5nZWxlckBzbHUuc2U=