- 1College of Plant Protection, Shanxi Agricultural University, Jinzhong, China
- 2Department of Biology, Xinzhou Teachers University, Xinzhou, China
Scale insects (Coccoidea) are morphologically specialized members of the order Hemiptera, with 56 families recognized to date. However, the phylogenetic relationships within and among families are poorly resolved. In this study, to further characterize the phylogenetic relationships among scale insects, an ultraconserved element (UCE) probe set was designed specifically for Coccoidea based on three low-coverage whole genome sequences along with three publicly available genomes. An in silico test including eight additional genomes was performed to evaluate the effectiveness of the probe set. Most scale insect lineages were recovered by the phylogenetic analysis. This study recovered the monophyly of neococcoids. The newly developed UCE probe set has the potential to reshape and improve our understanding of the phylogenetic relationships within and among families of scale insects at the genome level.
Introduction
Scale insects are small plant-feeding, sap-sucking insects that include all members of the superfamily Coccoidea (Gullan and Cook, 2007). Together with aphids (Aphidoidea), jumping plant lice (Psylloidea), and whiteflies (Aleyrodoidea), they constitute the hemipteran suborder Sternorrhyncha (Kondo et al., 2008; Ross et al., 2010). More than 8,400 species belonging to 56 families have been identified, of which 20 are extinct and 36 are extant (Gullan and Cook, 2007; Hodgson and Hardy, 2013; García Morales et al., 2016). Many species of scale insects are cryptic in habit, resembling their host plants (Hodgson and Hardy, 2013). Most adult female scale insects have soft bodies covered by waxy secretions or toughened shields, lacking wings and legs (Hodgson and Hardy, 2013); however, adult males have legs and lack mouthparts (Hodgson et al., 2021). They are significant economic pests (Hodgson and Hardy, 2013) and are among the most invasive insects in the world (Miller et al., 2005), including many agricultural pests (Miller and Davidson, 1990). Scale insects are diverse in many aspects, including variation in genetic and reproductive systems (Normark, 2003, 2004; Ross et al., 2010, 2012; Mongue et al., 2021), types of chromosomes (Blackmon et al., 2016), and endosymbiotic microorganisms (Ross et al., 2012; Sabree et al. (2013); Rosenblueth et al., 2018). Scale insects are also important model organisms for studies of the evolution of extreme polyphagy and host use (Hardy et al., 2016; Peterson et al., 2020), symbioses with endosymbionts (Gruwell et al., 2007; Rosenblueth et al., 2012, 2018; Choi and Lee, 2022), and mutualism with ants (Ben-Dov and Fisher, 2010; Schneider and LaPolla, 2011; Schneider et al., 2018) or wasps (Kapranas and Tena, 2015; Qin et al., 2018).
The classification and composition of Coccoidea has long been controversial and there are many unresolved issues. The scale insects are traditionally divided into two groups, archaeococcoids (∼10% of species) and neococcoids (∼90% of extant species) (Gullan and Cook, 2007; Hodgson, 2014; Vea and Grimaldi, 2016). The monophyly of neococcoids is supported by multiple lines of evidence, including a shared chromosome system called paternal genome elimination (PGE) (Danzig, 1986; Normark, 2003), molecular data [e.g., Gullan and Cook, 2007 (18S); Vea and Grimaldi, 2016 (partial nuclear regions of 18S, 28S, and EF-1a); Yokogawa and Yahara, 2009 (mitochondrial genes COI and COII)], and male morphology (Hodgson and Hardy, 2013). The archaeococcoids have been characterized as non-monophyletic based on morphological (Hodgson and Hardy, 2013) and molecular evidence [Gullan and Cook, 2007 (18S); Vea and Grimaldi, 2016 (partial nuclear regions of 18S, 28S, and EF-1a)]. The extant families Phenacoleachiidae, Pityococcidae, Steingeliidae, and Putoidae did not fall within archaeococcoids and formed separate clades between archaeococcoids and neococcoids based on studies of Hodgson and Hardy (2013) and Vea and Grimaldi (2016). Recently, many studies have explored the phylogenetic relationships based on short DNA fragment for some scale insects, including Diaspididae (Morse and Normark, 2006; Andersen et al., 2010; Schneider et al., 2018; Normark et al., 2019), Coccidae (Choi and Lee, 2022), Pseudococcidae (Downie and Gullan, 2004; Hardy et al., 2008; Schneider and LaPolla, 2011; Choi and Lee, 2022), Eriococcidae (Cook and Gullan, 2004), and Ortheziidae (Vea and Grimaldi, 2012). However, a robust phylogeny revealing relationships within Coccoidea and between this group and other hemipterans is still lacking, irrespective of data type (i.e., morphology or DNA sequences). Coccidologists have continued to develop markers for phylogenetic reconstruction along with new morphological characters for classification.
The estimation of phylogenetic relationships among scale insects requires the following: (1) adequate taxon sampling to cover all families (Buzan et al., 2008; Heath et al., 2008); (2) molecular markers containing enough phylogenetic informative sites (Young and Gillung, 2020); (3) markers that are easily obtained (Buenaventura et al., 2021). Advances in next-generation sequencing have made genome-scale data easier to obtain. Representative methods are transcriptomic sequencing (RNA-seq) (Wang et al., 2009) and hybrid enrichment techniques (Faircloth et al., 2012; Lemmon et al., 2012). These techniques, while effective, have various limitations (Misof et al., 2014; Smith et al., 2014; Young et al., 2016). Transcriptomic sequencing methods require a large quantity of high-quality RNA from fresh specimens preserved specifically for RNA work in liquid nitrogen (Wang et al., 2009; Cronn et al., 2012). Hybrid enrichment techniques, such as anchored hybrid enrichment (AHE) and ultraconserved elements (UCEs), have less stringent quality and quantity restrictions to overcame these limitation (Blaimer et al., 2016; Winker et al., 2018). UCEs are useful for phylogenetic inference (Faircloth and Gilbert, 2017; Gustafson et al., 2019); they are highly conserved regions within the genome that are shared among evolutionarily distant taxa (Bejerano et al., 2004; Zhang Y. M. et al., 2019). UCEs have proven their utility across diverse taxa, including vertebrates [mammals (McCormack et al., 2012; Esselstyn et al., 2017), birds (McCormack et al., 2013; Musher and Cracraft, 2018), fish (Faircloth et al., 2013; Fernando Alda et al., 2018), and amphibians (Newman and Austin, 2016)] and invertebrates [Arachnida (Starrett et al., 2017), Coleoptera (Baca et al., 2017), Diptera (Buenaventura et al., 2021), Hemiptera (Kieran et al., 2019), and Hymenoptera (Faircloth et al., 2015; Branstetter et al., 2017)]. Additionally, UCEs can be used to reconstruct evolutionary relationships at multiple time scales from deep to shallow (Faircloth et al., 2012; McCormack et al., 2012). While UCE baits have been applied to several insect orders, few have been developed for analyses within orders (Van Dam et al., 2019), some studies had reconstructed subfamily, family, superfamily and suborder relationships within Hemiptera (Forthman et al., 2019, 2020; Kieran et al., 2019). Bait sets designed for specific taxa can improve the recovery of loci to some extent (Van Dam et al., 2019). Studies had demonstrated that one can conduct UCE analysis by using low-coverage whole genome sequencing (WGS) (Zhang F. et al., 2019,Zhang Y. M. et al., 2019; Cooper et al., 2020; Wang et al., 2021).
A phylogenetic study should ideally be based on WGS; however, the application of this approach to non-model organisms is limited by high costs and computational requirements. Low-coverage WGS has emerged as a powerful and cost-effective approach for population genomic (Lou et al., 2021) and phylogenomics (Zhang F. et al., 2019) in both model and non-model species in current years. This approach benefits from using as much genetic information from the whole genome as is feasible while reducing the costs of experiments. In the current study, genomic data derived from public databases and low-coverage WGS were used to design a custom UCE probe set specific for Coccoidea based on members of four families (Coccidae, Pseudococcidae, Diaspididae, and Eriococcidae). The effectiveness of the probe set was tested by an in silico test with additional genomic data.
Materials and methods
Study group
We used three publicly available genomes in NCBI and three low-coverage WGS to design a probe set specific to Coccoidea (Table 1). Taxa were chosen to cover representative families of scale insects, such as Coccidae (soft scales), Pseudococcidae (mealybugs), Diaspididae (armored scales), and Eriococcidae (felt scales). The in silico test involved eight additional genomes (seven from NCBI and one from low-coverage WGS) to test the effectiveness of the probe set. We used four genomes as outgroups, including soybean aphid, white-backed planthopper, Asian citrus psyllid, and greenhouse whitefly.
DNA extraction and sequencing
DNA was extracted from scale insects following the manufacturers protocol (Ezup Column Animal Genomic DNA Purification Kit, Sangon Biotech, Shanghai, China), with modifications. (1) An individual fresh female adult was placed on a stereomicroscope and punctured by a sterilized super-thin pin in the thorax. (2) Samples were incubated in proteinase K buffer overnight in a 1.5 mL tube in a 55°C water bath; gently pressing on the specimen with a pin was optional to increase the total amount of DNA. (3) The cuticle was retained for further stain as a voucher specimen while solution was for DNA extraction. DNA library preparation and sequencing were performed on an Illumina NovaSeq 6000 sequencing platform with paired-end 2 × 150 bp read length by Novogene Co., Ltd. (Beijing, China) (Reagent version: NovaSeq Reagent Kits v1.5).
Identification of loci and bait design
Raw sequencing data from four individuals were performed quality checks using FastQC (v0.11.9) (Andrews, 2010); low quality and contaminant reads were removed using fastp (0.21.0) (Chen et al., 2018). Clean reads were conducted de novo assembly using SPAdes (v3.15.2) (Prjibelski et al., 2020). Then, we evaluated the quality of genomes (both low-coverage WGS assembled genomes and those downloaded from databases) by using BUSCO (v5.1.3) (Manni et al., 2021; Figure 1).
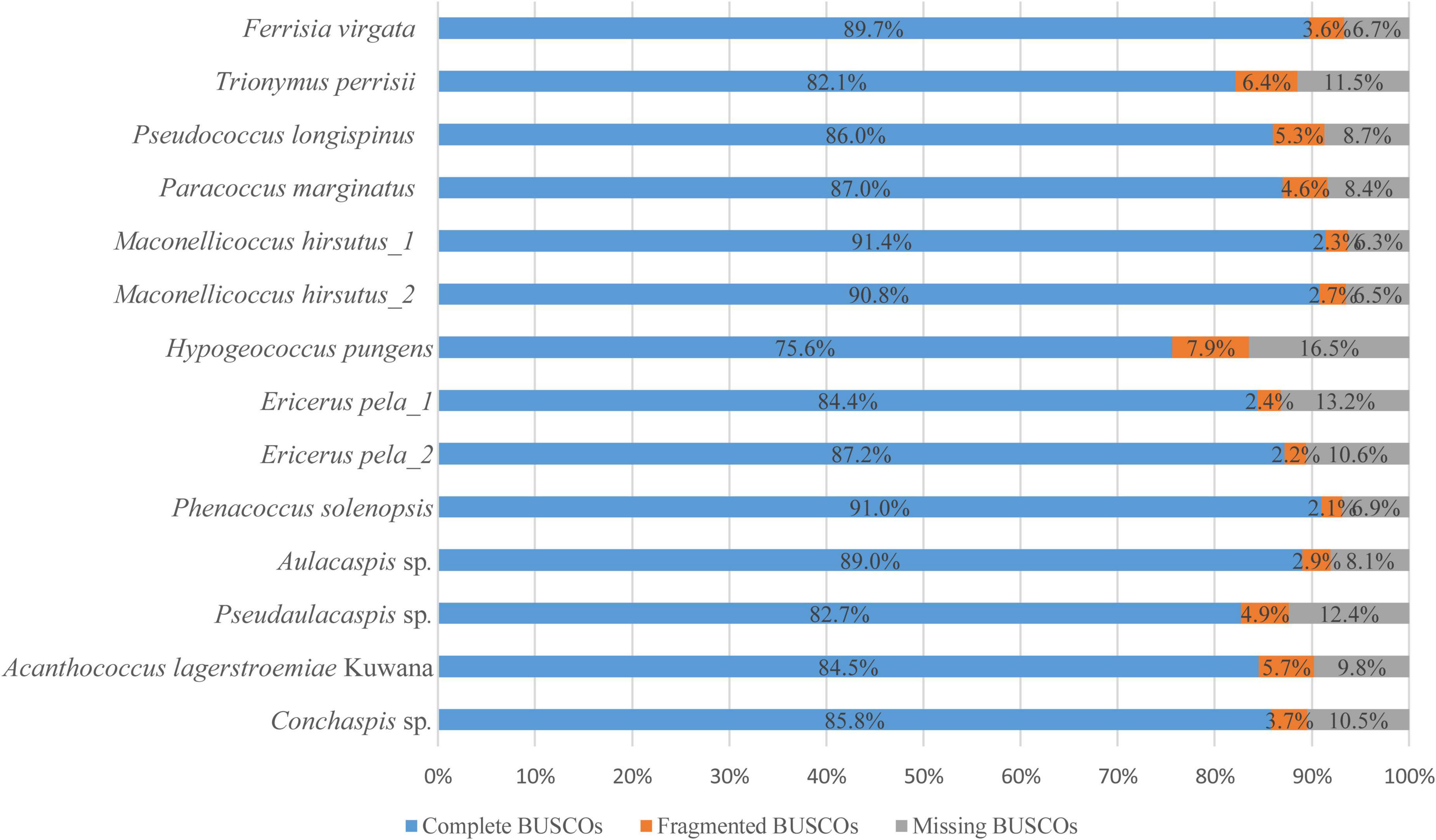
Figure 1. BUSCO evaluation of ingroup genomes. In our study, BUSCO searched every ingroup genomes (4 from low coverage WGS, 10 from NCBI database) against hemiptera dataset, which contained 2,510 universal single-copy orthologs by August 5th 2020. Complete BUSCOs represent total matches of complete single-copy and complete duplicated BUSCOs. Fragmented BUSCOs mean the matches were partial and Missing BUSCOs mean no matches.
We follow Faircloth’s pipeline using Python software package PHYLUCE (v1.7.1) (Faircloth et al., 2012; Faircloth, 2016). It’s worth emphasizing that we refer to temporary bait sets targeting putative conserved loci as “bait” in this study, whereas “probe” refers to the ultimate results of probe design (i.e., the set of RNA probes targeting UCE loci that would actually be synthesized, subject to in silico testing) (Gustafson et al., 2019). We used ART (version 2.5.8) (Huang et al., 2012) to simulated reads from the genomes for alignment to a base genome (i.e., a well-assembled genome centrally located in the phylogeny). We chose the chromosome-level assembled genome of Phenacoccus solenopsis (solenopsis mealybug) as the base genome, as we thought higher genomic assembly metrics would result in better outcomes (Gustafson et al., 2019). Simulated reads were 100 bp paired-end reads at 2 × coverage for each genome, with an insert size of 200 bp; paired reads were then merged. Then, stampy (v1.0.32) (Lunter and Goodson, 2011) was used to align the reads for each genome to the base genome sequence with a substitution rate of 0.05 and insert size of 400 bp. The SAM file was converted to BAM format using SAMtools (Version: 1.7) (Danecek et al., 2021). Next, converted BAM files to BED format using BEDTools (v2.30.0) (Quinlan and Hall, 2010), then sorted the converted BED files and merged overlapping or nearly overlapping intervals. The PHYLUCE (v1.7.1) script “phyluce_probe_strip_masked_loci_from_set” was used to remove putatively conserved intervals shared between other genomes and the base genome in the BED files.
The PHYLUCE (v1.7.1) script “phyluce_probe_get_multi_merge_table” was run to build an SQLite database containing a record of alignment intervals that are shared among taxa. Then, “phyluce_probe_query_multi_merge_table” queried the database and output the number of loci shared by the base genome and other taxa genomes. We then output loci shared between base genome plus 5 taxa (i.e., all other taxa) (Table 2) in BED format. Then, started designing baits to capture these loci by first extracting FASTA sequences (160 bp) from the base genome for temporary bait design using “phyluce_probe_get_genome_sequences_from_bed.” A temporary bait set was designed using “phyluce_probe_get_tiled_probes” ensuring that two baits per locus were selected with a 3 × tiling density overlapping the middle of the targeted locus. Potentially problematic baits with >25% repeat content and GC contents outside the range of 30–70% were removed.
Next, “phyluce_probe_easy_lastz” (v1.04.00) (Harris, 2007) was used to align all baits to themselves to screen duplicates with greater than 50% identity over 50% of the length of the locus. Then, “phyluce_probe_remove_duplicate_hits_from_probes_using _lastz” (v1.04.00) was used to remove duplicate baits from the temporary bait set. These baits designed from the base genome were aligned to other exemplar genomes and an outgroup genome (it helps to bridge the divergence between the outgroup and the exemplar genomes), to see if the bait set works consistently across broad taxa by using “phyluce_probe_run_multiple_lastzs_sqlite” to build an SQLite database and FASTA sequences were extracted using “phyluce_probe_slice_sequence_from_genomes” with buffering to 180 bp for each locus.
We used “phyluce_probe_get_multi_fasta_table” to identify loci that were detected consistently across all genomes. The results were output in an SQLite database. The database was queried to determine how conservative the baits we want to be (Table 3). After identifying loci for enrichment, “phyluce_probe_get_tiled_probe_from_multiple_inputs” was used to write a pre-probe set to a file. This pre-probe set was screened for duplicates using “phyluce_probe_easy_lastz” (v1.04.00) and a final duplicate-free probe set in FASTA format was obtained by using “phyluce_probe_remove_duplicate_hits_from_probes_using_lastz,” (v1.04.00) named “coccoidea-v4-master-probe-list-DUPE-SCREENED.fasta.”
In silico test
To test the performance of the newly designed probe set, an in silico test was performed with 18 additional genomes, 4 of which were outgroups (Aphis glycines, Sogatella furcifera, Diaphorina citri, and Trialeurodes vaporariorum) (Table 1).
We used “phyluce_probe_run_multiple_lastzs_sqlite” to align the probe set to all of the genomes and used “phyluce_probe_slice_sequence_from_genomes” to extract FASTA data for each locus with 400 bp flanking regions on each side. We used “phyluce_assembly_match_contigs_to_probes” to remove duplicates (i.e., the same contig obtained by probes targeting different loci or two supposedly different contigs obtained by probes designed for a single UCE locus). Next, “phyluce_assembly_get_match_counts” and “phyluce_assembly_get_fastas_from_match_counts” (Table 1) were used to extract corresponding FASTA files for each locus into a single file.
Data for conserved loci were aligned using “phyluce_align_seqcap_align” with mafft (v7.475) (Katoh and Standley, 2013; Yamada et al., 2016) and loci with too few taxa (n < 3) were removed. The resulting alignments were trimmed using “phyluce_align_get_gblocks_trimmed_alignments_from _untrimmed” with Gblocks (0.91b) (Castresana, 2000). Then, ‘‘phyluce_align_remove_locus_name_from_files’’ was used to remove the locus names from each of the resulting alignments. Summary statistics across the alignments were obtained using ‘‘phyluce_align_get_align_summary_data’’ and 75, 85, and 95% completeness matrixes were generated using ‘‘phyluce_align_get_only_loci_with_min_taxa.’’ For comparison, we used Hemiptera probe set (Hemiptera 2.7Kv1)1 (Faircloth and Gilbert, 2017) to run an in silico test with same 18 genomes.
Phylogenetic reconstruction of exemplar taxa
Concatenated analyses
We used “phyluce_align_concatenate_alignments” to generate a concatenated matrix in nexus format. To partition UCE data for the phylogenetic analysis, we used the Sliding-Window Site Characteristics method based on site entropies (SWSC-EN) (Tagliacollo and Lanfear, 2018; Zhang et al., 2020). Individual UCEs were divided into three data blocks corresponding to the left flank, center, and right flank, respectively. The resulting data were analyzed using PartitionFinder 2 (Lanfear et al., 2016) with AICc model selection criterion, rcluster scheme (Lanfear et al., 2014), and RAxML (Stamatakis, 2014) search algorithm. Next, IQ-TREE 2 (Minh et al., 2020) was used to reconstruct a maximum likelihood (ML) tree based on the concatenated data and evaluated by 1,000 ultrafast bootstrap approximation (UFBoot) replicates (Hoang et al., 2018). This analysis was repeated for the 75, 85, and 95% completeness matrixes of both our probe set and Hemiptera probe set (Hemiptera 2.7Kv1).
Species tree inference
We used IQ-TREE 2 (Minh et al., 2020) to generate a set of unpartitioned gene trees (where “gene tree” does not specifically refer to gene-based sequences but to UCE sequences) based on ML and found the best-scoring ML gene tree (Mirarab et al., 2016) under the substitution model identified using ModelFinder (Kalyaanamoorthy et al., 2017) and evaluated by 1,000 ultrafast bootstrap approximation (UFBoot) replicates (Hoang et al., 2018). Then, ASTRAL-III (Qin et al., 2018) was run with two files as inputs: one containing ML trees for each locus and another containing the names of all files with bootstrapped trees. After summarizing, we harvested the species tree estimated from the ML gene trees and annotated with node support based on the bootstrap replicates. This analysis was repeated for 75, 85, and 95% completeness matrixes of both our probe set and Hemiptera probe set (Hemiptera 2.7Kv1).
Results
Identification of loci and bait design
The sequencing depths for four species were 26 × (Pseudaulacaspis sp.), 32 × (Aulacaspis sp.), 45 × (Acanthococcus lagerstroemiae Kuwana), and 33 × (Conchaspis sp.). After assembly, the genome sizes were about 295 Mb for Pseudaulacaspis sp., 233 Mb for Aulacaspis sp., 649 Mb for A. lagerstroemiae Kuwana, and 274 Mb for Conchaspis sp. The proportion of complete BUSCOs along with genomes derived from the database ranged from 75.6 to 91.4% (mean = 86.23%) (Figure 1).
We screened out loci shared between the base genome and the genomes of five other exemplar taxa (Table 2). Then, we filtered conserved loci that were consistently detected across six taxa (Table 3). After all, we obtained a total of 3,995 conserved loci and 48,520 probes for the final probe design. The mean number of loci targeted per taxon was 3,561 in our probe set (Table 4).
In silico test
We aligned our probe set to genomes and obtained FASTA files that contained all UCE alignments for all genomes. The mean number of loci was 2,492 per taxon (Table 1). There was no bias in capture performance across ingroups. We generated 75, 85, and 95% completeness matrices from 3,434 UCE alignments. The 75% matrix contained 2,671 alignments representing 13 taxa, 85% matrix contained 781 alignments representing 15 taxa, and 95% matrix contained 146 alignments representing 17 taxa. These three matrices were utilized for a phylogenetic analysis. The mean number of loci for Hemiptera probe set (Hemiptera 2.7Kv1) was 853 per taxon (Table 1). We generated 75, 85, and 95% completeness matrices from 1,821 UCE alignments. The 75, 85, and 95% matrix contained 427, 257, and 96 alignments, respectively.
Phylogenetic reconstruction
In order to evaluate our process of probe design and test, we added two repeated different assembly genomes for the same species: Maconellicoccus hirsutus and Ericerus pela. As expected, they clustered together on the trees.
Analyses of concatenated sequences
In concatenated analysis of data enriched from our probe set, phylogenies based on 75 and 95% completeness matrices shared the same topologies, except for a difference in bootstrap support for one node within Pseudococcidae. We recover a monophyletic Pseudococcidae. Eriococcidae was the sister group to Diaspididae and Coccidae was the sister group to Eriococcidae + Diaspididae. Pseudococcidae was the sister group to Conchaspididae + [Coccidae + [Eriococcidae + Diaspididae]]. The phylogeny based on the 85% completeness matrix differed with respect to the placement of Conchaspididae, which was the sister group to Pseudococcidae (Figures 2–4). Pseudococcidae, Coccidae, Diaspididae, Eriococcidae, and Conchaspididae are traditionally assigned to an informal group referred to as the neococcoids. The Pseudococcidae branch was congruent for the 75, 85, and 95% completeness matrices. Phenacoccus solenopsis (Phenacoccinae) was sister to other species (Pseudococcinae). These findings are consistent with the result of Hardy et al. (2008). In concatenated analysis of data enriched from Hemiptera probe set (Hemiptera 2.7Kv1), phylogenies based on 85 and 95% completeness matrices shared the same topologies, and were consistent with 75 and 95% completeness matrices from Coccoidea probe set. Phylogeny based on the 75% completeness matrix was consistent with 85% completeness matrix from our probe set (Figures 5–7).
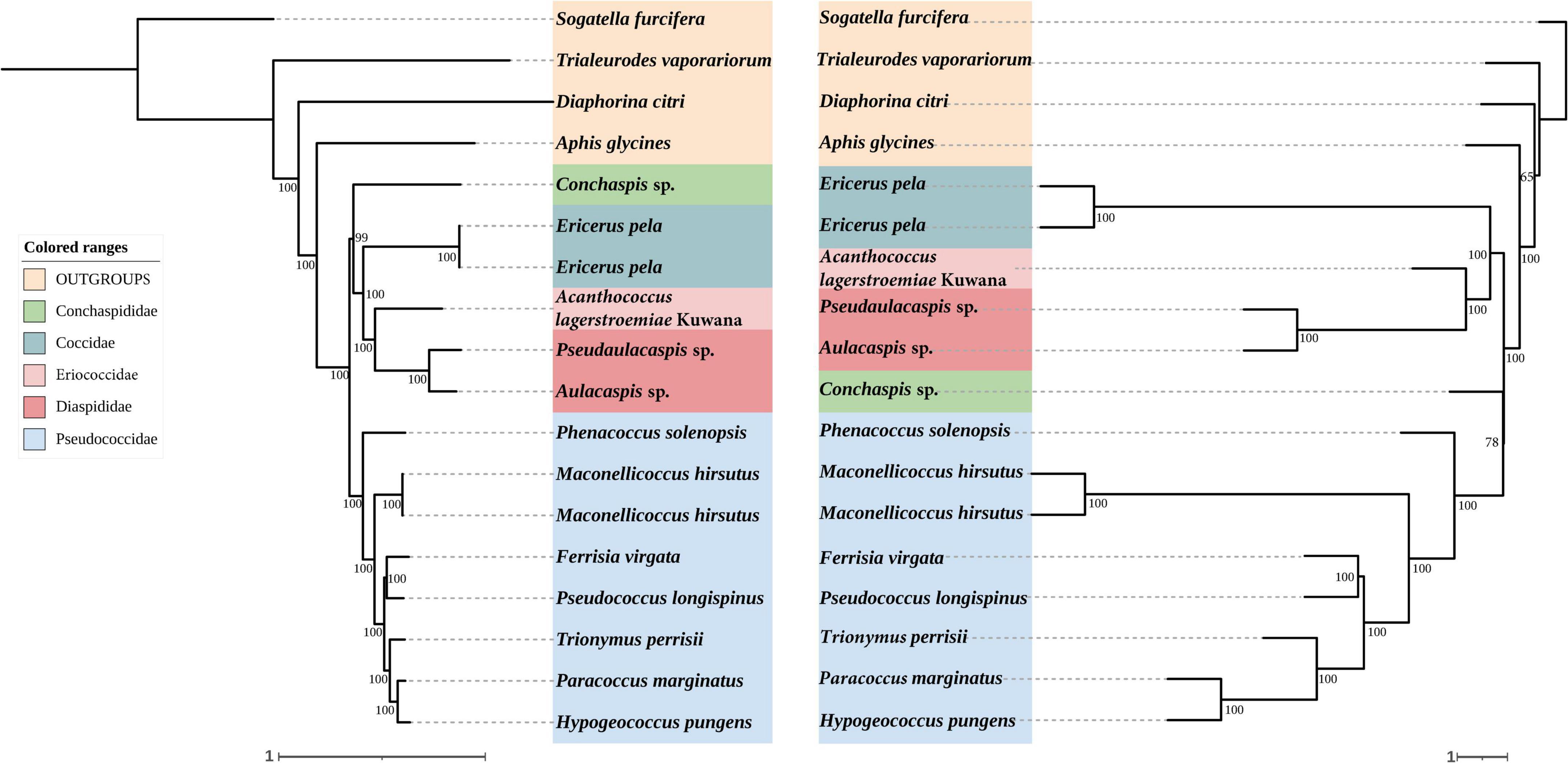
Figure 2. Left: Concatenated tree of 75% completeness matrices from Coccoidea probe set. Node values are bootstrap support. Right: Species tree of 75% completeness matrices from Coccoidea probe set. Node values are bootstrap support.
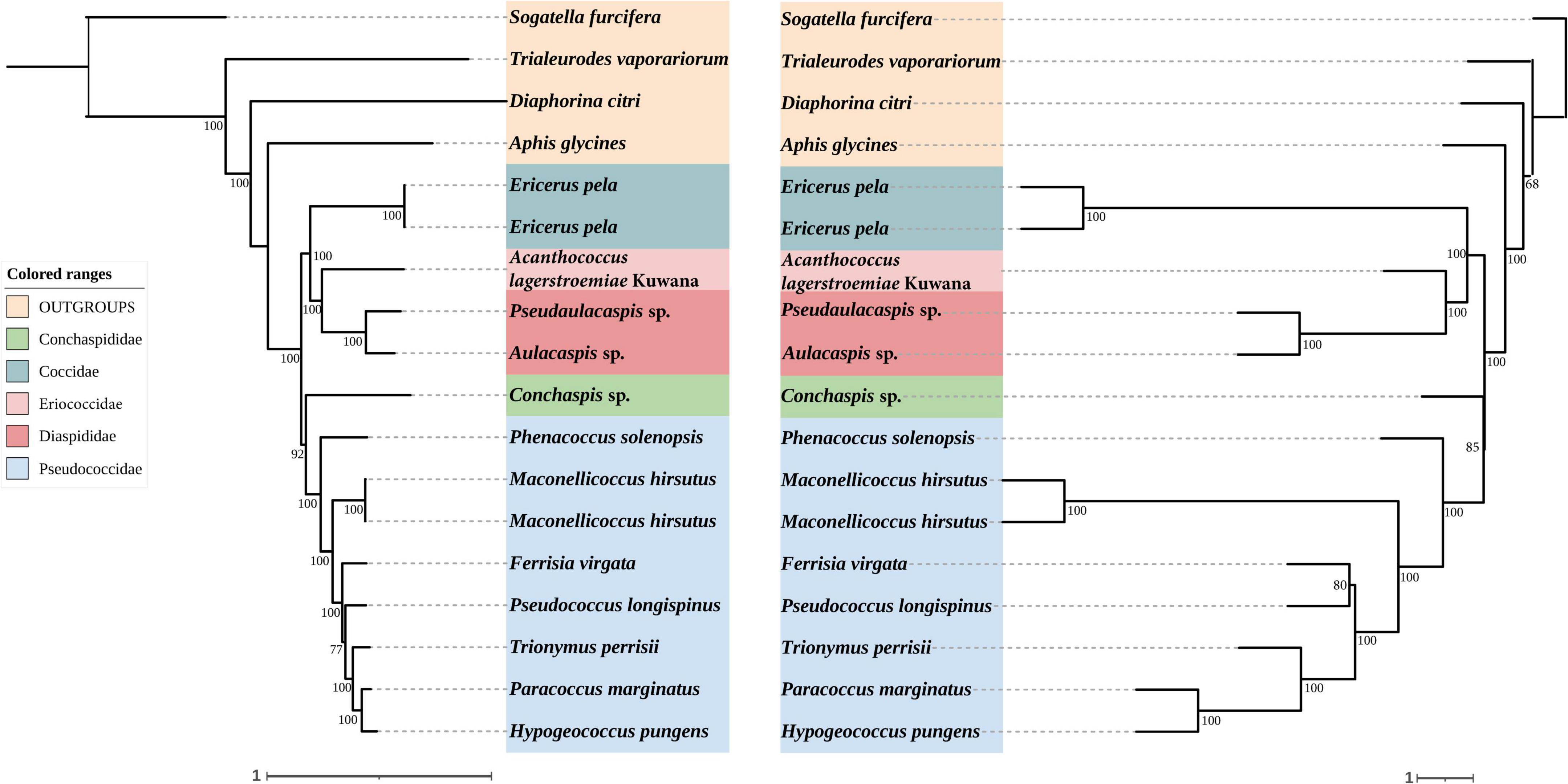
Figure 3. Left: Concatenated tree of 85% completeness matrices from Coccoidea probe set. Node values are bootstrap support. Right: Species tree of 85% completeness matrices from Coccoidea probe set. Node values are bootstrap support.
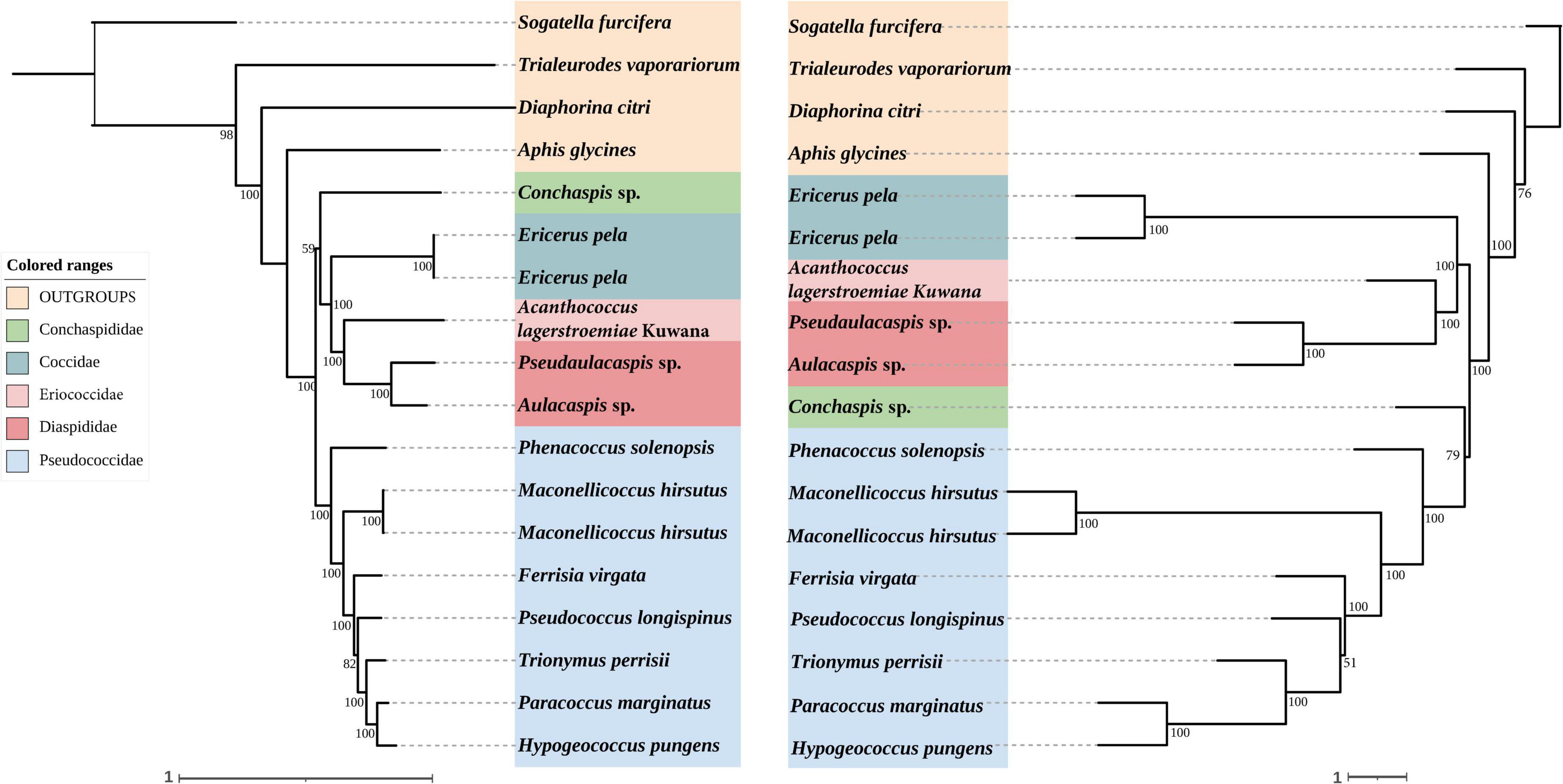
Figure 4. Left: Concatenated tree of 95% completeness matrices from Coccoidea probe set. Node values are bootstrap support. Right: Species tree of 95% completeness matrices from Coccoidea probe set. Node values are bootstrap support.
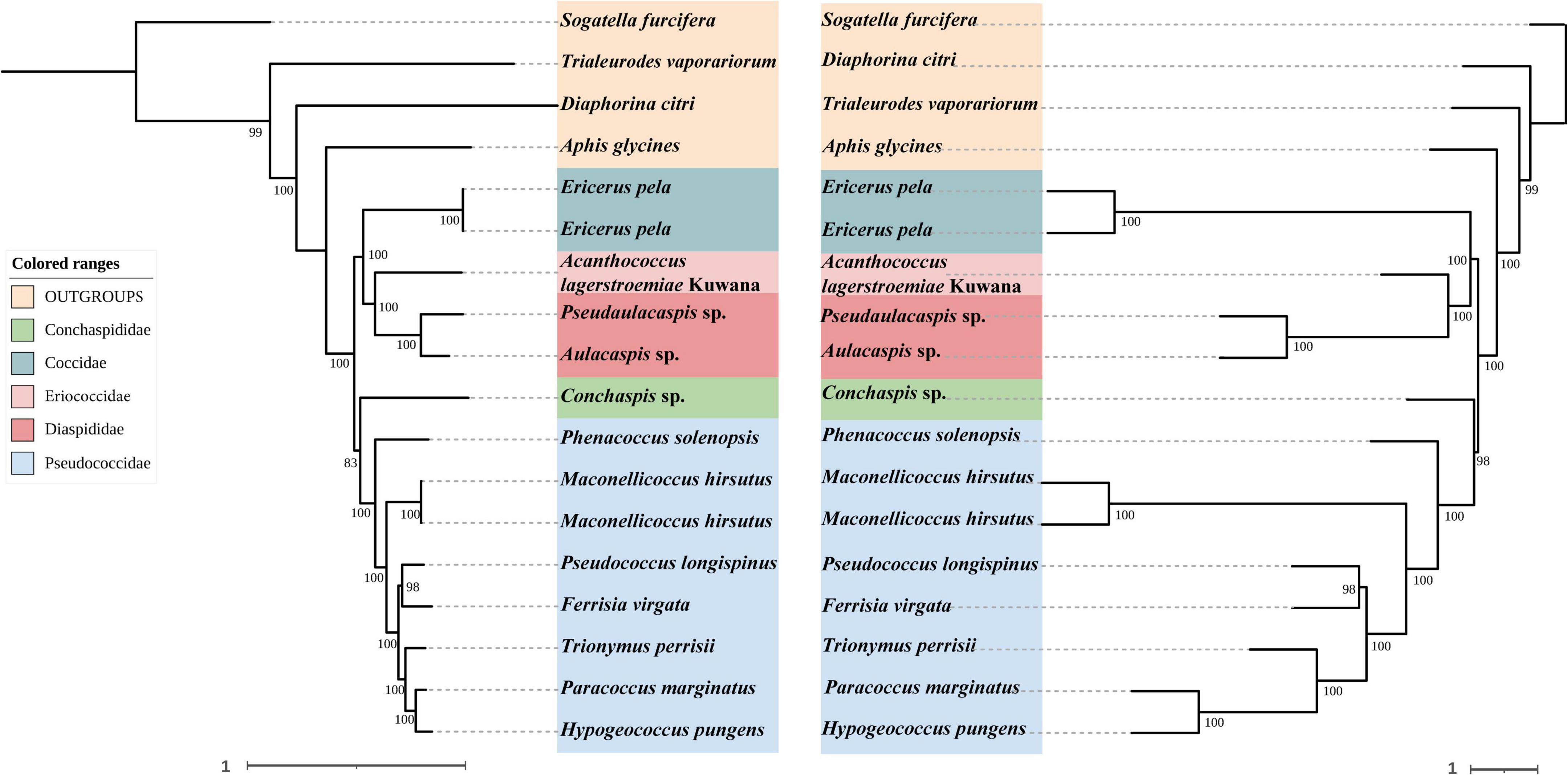
Figure 5. Left: Concatenated tree of 75% completeness matrices from Hemiptera probe set. Node values are bootstrap support. Right: Species tree of 75% completeness matrices from Hemiptera probe set. Node values are bootstrap support.
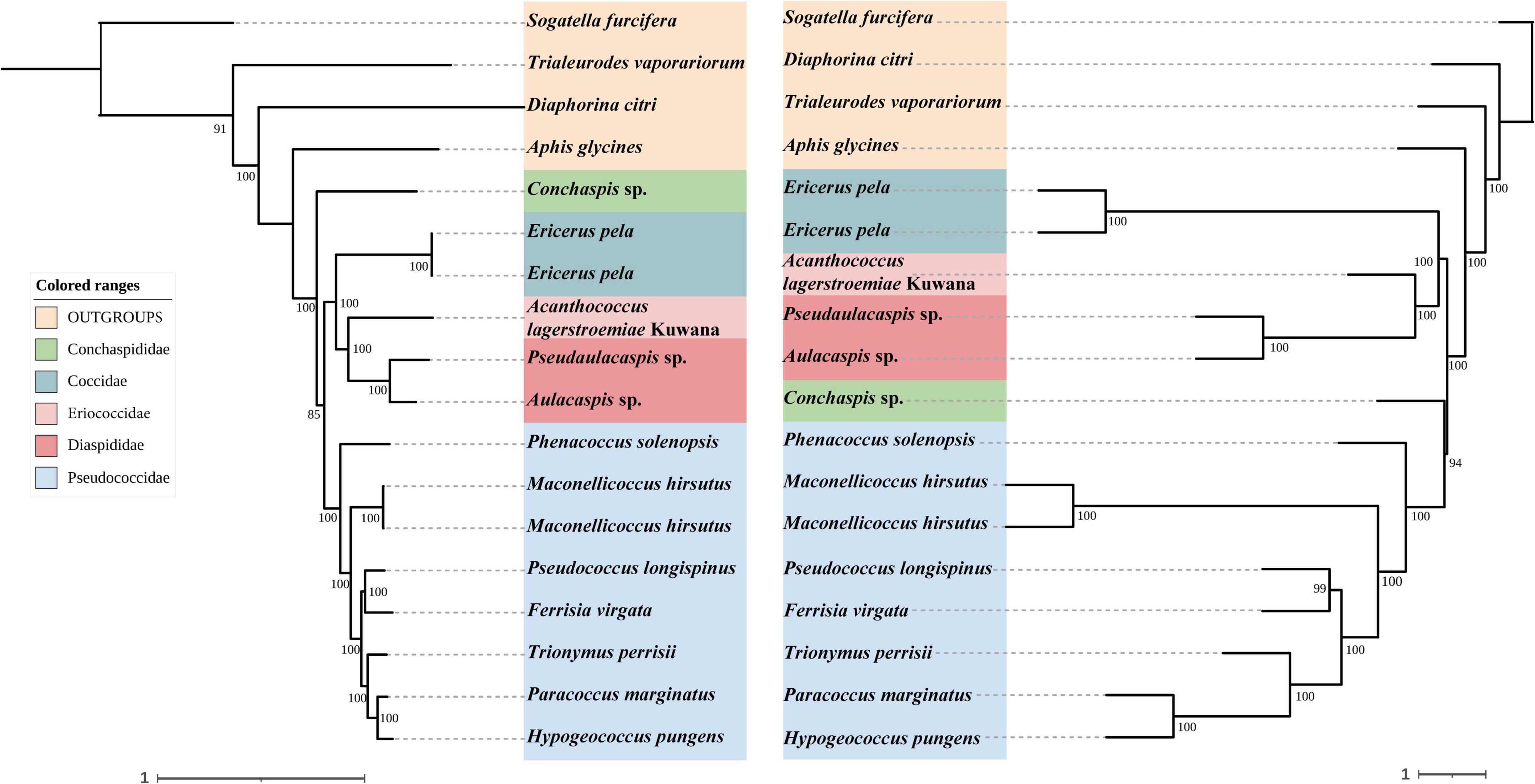
Figure 6. Left: Concatenated tree of 85% completeness matrices from Hemiptera probe set. Node values are bootstrap support. Right: Species tree of 85% completeness matrices from Hemiptera probe set. Node values are bootstrap support.
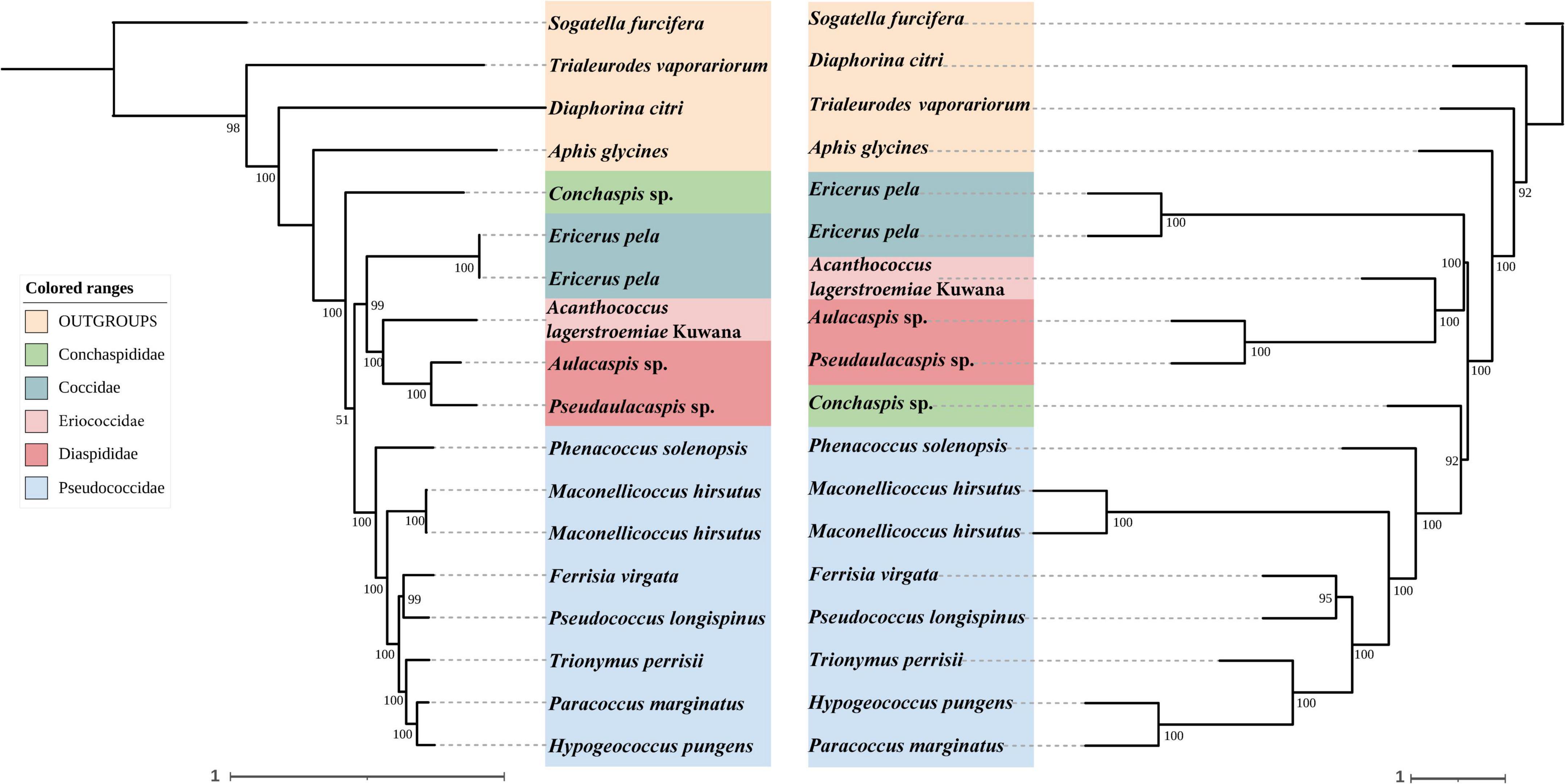
Figure 7. Left: Concatenated tree of 95% completeness matrices from Hemiptera probe set. Node values are bootstrap support. Right: Species tree of 95% completeness matrices from Hemiptera probe set. Node values are bootstrap support.
Species tree inference
The species trees were rooted by using S. furcifera. The species trees based on 75, 85, and 95% completeness matrices returned a consistent topology for data from both probe sets. Conchaspididae was a sister group to Pseudococcidae, and [Conchaspididae + Pseudococcidae] was a sister group to [Coccidae + [Eriococcidae + Diaspididae]]. Within Pseudococcidae, results of concatenated analyses were consistent (Figures 2–7).
Discussion
We designed a probe set specific for Coccoidea and evaluated its effectiveness. Our aim was not to resolve the Coccoidea phylogeny with limited taxon sampling but rather to demonstrate the performance of our probe set and its potential to address issues that were previously insurmountable. The number of UCE loci recovered from Hemiptera probe set (Hemiptera 2.7Kv1) (Faircloth and Gilbert, 2017) was less than that of the Coccoidea probe set, specifically the loci recovered for the outgroups was more than ingroups (Table 1). Species used for Hemiptera probe set design did not contain any members of scale insects in Hemiptera 2.7Kv1. Diaphorina citri was used as base genome in Hemiptera 2.7Kv1, but it was used as one of the outgroups in our study. Base genome and exemplar genomes choice alter the composition of probe set and furthermore affect the loci recovered for phylogenetic analyses, factors affecting these remain underexplored. Meanwhile, the phylogeny trees (both concatenated analyses and species tree inference) inferred from Coccoidea probe set and Hemiptera probe set (Hemiptera 2.7Kv1) differed a little, which demonstrates that the Hemiptera probe set (Hemiptera 2.7Kv1) contains enough informative sites for this limited taxon sampling of scale insects. However, a larger number of UCE loci should meet the need for further work when increasing the taxon sampling. Taxon-specific probe design will be necessary if our concern is more focused taxa. We recovered the monophyletic Pseudococcidae clade. The placement of Conchaspididae in the tree was unstable. Takagi believed that Conchaspididae and Diaspididae closely related (Takagi, 1992). According to Gullan and Cook (2007), Conchaspididae shared some morphological traits and was likely to be a member of larger family. Combined analyses of molecular and morphological traits may clarify the placement of Conchaspididae. For the separation of the two subfamilies, congruence within Pseudococcidae proves the robustness of our probe set at the population level. Based on 2,671 alignments in the 75% completeness matrix, the topology of the concatenated tree and species tree differed slightly from those based on the other completeness matrices. This may be explained by the oversaturation of informative sites under limited taxon sampling. The number of loci needed to resolve the phylogenetic relationships is unclear; however, with the decreasing cost of sequencing and computational resources, it is feasible to utilize more loci for better resolution.
The modified protocol for DNA extraction from an individual fresh specimen while leaving the voucher intact makes it possible to prevent contamination from mixed specimen analyses, especially for tiny insects. The quantity of DNA was sufficient for low-coverage WGS. We used three of four genomes from low-coverage WGS for probe design and left one for the in silico test. The proportion of complete BUSCOs (82.7–89.0%, mean = 85.5%) indicate the integrity of assemblies, and there are an average 2,912 UCE loci (2,447–3,104, mean = 2,912) come from these low-coverage WGS assembly. Together led to a total of 3,995 conserved UCEs in the probe set. We have proved the utility of this “individual specimen low-coverage WGS-UCE” pattern for molecular phylogenetic analyses (Zhang F. et al., 2019).
Our Coccoidea phylogeny was based on 5 of 36 extant families and therefore only reflects a partial portion of the big picture. Owing to the lack of sufficient genome resources, molecular phylogenetic reconstruction of Coccoidea has depended on fragmented nuclear genes. It is inconvenient for replication and further work. Thus, successors of this field can only proceed from the very beginning all over again. Low-coverage WGS provides a viable solution for non-model organisms. The costs are affordable and can easily generate genome scale data (even if not 100% completeness genome, a minimum of 10× raw reads can theoretically cover the whole genome). Additionally, researchers can always back to the raw reads of the genome for regions of interests/new methods in further studies. It enables consecutive analyses of common taxa. In additional, studies have revealed that most flanking regions caught in invertebrate UCEs are exons (Branstetter et al., 2017) or partially exonic regions (Bossert and Danforth, 2018). This is a significant breakthrough because, as proven in Apidae (Bossert et al., 2019), exonic flanking areas recovered by the UCE method and transcriptome sequencing data within these groups may be usefully integrated without the requirement for particular targeted probe sets (Kieran et al., 2019). Our study provides a basis for further combination analyses of different data sources.
Low-coverage WGS also have some limitation, such as low-coverage certainly perform worse for large genomes (Zhang F. et al., 2019). In additional, AHE (Lemmon et al., 2012) and UCEs are might be most widely target capture approaches to develop insect phylogenomic datasets based on reduced representation or low-coverage WGS data sets in current years (Johnson, 2019). These two approaches have fewer limitations in material quality that is degraded DNA or poorly preserved material. Moreover, compare with entire genome or transcriptome, the cost per sample is relatively modest when the probes are developed (Blaimer et al., 2016). Despite there are many similarities between AHE and UCEs, however, the UCEs approach targets highly conserved non-coding regions of the genome, while AHE targets highly conserved regions primarily in the coding portion of the genome. The difference between AHE and UCEs methods is the loci targeted: AHE focuses on fewer loci (300–600) while UCEs target more loci (>1,000) using fewer probes (Faircloth et al., 2015). Compare with AHE, the UCEs have following advantages: (1) UCEs include openly shared resources (probe sets, lab protocols and bioinformatics tools), making it easily to learn; (2) UCE datasets can be easily combinable data across studies using the same probe set, exon or transcriptome (Bossert et al., 2019).
The scale insects possess varied genetic and reproductive systems (Normark, 2003, 2004; Ross et al., 2010, 2012; Husnik and McCutcheon, 2016; Mongue et al., 2021) and occupy an important position in the evolution of insects. Our study added genome-level genomic resources for scale insects and proved the necessity of a set of taxon-specific Coccoidea UCE probes for further study. We have proved the utility of “individual specimen low-coverage WGS-UCE” pattern and it can become a viable routine method for non-model organisms to promote phylogenomic analyses. We expect our probe set to facilitate a comprehensive understanding of the phylogenetic relationships of scale insects.
Data availability statement
The data presented in this study are deposited in the NCBI repository, accession number: PRJNA826350 (https://www.ncbi.nlm.nih.gov/bioproject/PRJNA826350/).
Author contributions
DL, JW, and HZ conceived the study. JW, MN, and HZ obtained funding for the study. DL and JW designed the experiments, analyzed the data, and wrote the first draft of the manuscript. DL, JW, MN, and YL performed the fieldwork. All authors read and approved the final version of the manuscript.
Funding
This work was supported by Shanxi Scholarship Council of China (2020-065), Natural Science Foundation of Shanxi, China (Grant Nos. 202103021224132 and 202103021224331), National Natural Science Foundation of China (Grant No. 32100370), Science and Technology Innovation Funds of Shanxi Agricultural University (2020BQ79), the Excellent Doctoral Award of Shanxi Province for Scientific Research Project (SXBYKY2021024), and Science and Technology Innovation Projects of Universities in Shanxi Province (2021L097).
Conflict of interest
The authors declare that the research was conducted in the absence of any commercial or financial relationships that could be construed as a potential conflict of interest.
Publisher’s note
All claims expressed in this article are solely those of the authors and do not necessarily represent those of their affiliated organizations, or those of the publisher, the editors and the reviewers. Any product that may be evaluated in this article, or claim that may be made by its manufacturer, is not guaranteed or endorsed by the publisher.
Footnotes
References
Andersen, J. C., Wu, J., Gruwell, M. E., Gwiazdowski, R., Santana, S. E., Feliciano, N. M., et al. (2010). A phylogenetic analysis of armored scale insects (Hemiptera: Diaspididae), based upon nuclear, mitochondrial, and endosymbiont gene sequences. Mol. Phylogenet. Evol. 57, 992–1003. doi: 10.1016/j.ympev.2010.05.002
Andrews, S. (2010). FastQC: A quality control tool for high throughput sequence data. Available online at: https://qubeshub.org/resources/fastqc
Baca, S. M., Alexander, A., Gustafson, G. T., and Short, A. E. Z. (2017). Ultraconserved elements show utility in phylogenetic inference of Adephaga (Coleoptera) and suggest paraphyly of ‘Hydradephaga’. Syst. Entomol. 42, 786–795. doi: 10.1111/syen.12244
Bejerano, G., Pheasant, M., Makunin, I., Stephen, S., Kent, W. J., Mattick, J. S., et al. (2004). Ultraconserved elements in the human genome. Science 304, 1321–1325. doi: 10.1126/science.1098119
Ben-Dov, Y., and Fisher, B. L. (2010). The mutualism of Melissotarsus ants and armoured scale insects in Africa and Magadascar: Distribution, host plants and biology. Entomol. Hell. 19, 45–53. doi: 10.12681/eh.11571
Blackmon, H., Ross, L., and Bachtrog, D. (2016). Sex Determination, Sex Chromosomes, and Karyotype Evolution in Insects. J. Hered. 108, 78–93.
Blaimer, B. B., Lloyd, M. W., Guillory, W. X., and Brady, S. G. (2016). Sequence capture and phylogenetic utility of genomic ultraconserved elements obtained from pinned insect specimens. PLoS One 11:e0161531.
Bossert, S., and Danforth, B. N. (2018). On the universality of target-enrichment baits for phylogenomic research. Methods Ecol. Evol. 9, 1453–1460. doi: 10.1111/2041-210x.12988
Bossert, S., Murray, E. A., Almeida, E. A., Brady, S. G., Blaimer, B. B., and Danforth, B. N. (2019). Combining transcriptomes and ultraconserved elements to illuminate the phylogeny of Apidae. Mol. Phylogenet. Evol. 130, 121–131. doi: 10.1016/j.ympev.2018.10.012
Branstetter, M. G., Longino, J. T., Ward, P. S., Faircloth, B. C., and Price, S. (2017). Enriching the ant tree of life: Enhanced UCE bait set for genome-scale phylogenetics of ants and other Hymenoptera. Methods Ecol. Evol. 8, 768–776. doi: 10.1111/2041-210x.12742
Buenaventura, E., Lloyd, M. W., Perilla López, J. M., González, V. L., Thomas-Cabianca, A., and Dikow, T. (2021). Protein-encoding ultraconserved elements provide a new phylogenomic perspective of Oestroidea flies (Diptera: Calyptratae). Syst. Entomol. 46, 5–27. doi: 10.1111/syen.12443
Buzan, E. V., Keystufek, B., Hanfling, B., and Hutchinson, W. F. (2008). Mitochondrial phylogeny of Arvicolinae using comprehensive taxonomic sampling yields new insights. Biol. J. Linn. Soc. 64, 825–835.
Castresana, J. (2000). Selection of Conserved Blocks from Multiple Alignments for Their Use in Phylogenetic Analysis. Mol. Biol. Evol. 17, 540–552.
Chen, S., Zhou, Y., Chen, Y., and Gu, J. (2018). fastp: An ultra-fast all-in-one FASTQ preprocessor. Bioinformatics 34, i884–i890. doi: 10.1093/bioinformatics/bty560
Chinese Academy of Agricultural Sciences Institute of Vegetables and Flowers, (2020). Trialeurodes vaporariorum genome sequencing and assembly; GenBank; Accession Number: GCA_011764245.1
Choi, J., and Lee, S. (2022). Higher classification of mealybugs (Hemiptera: Coccomorpha) inferred from molecular phylogeny and their endosymbionts. Syst. Entomol. 47, 354–370. doi: 10.1111/syen.12534
Cook, L. G., and Gullan, P. J. (2004). The gall-inducing habit has evolved multiple times among the Eriococcid scale insects (Sternorrhyncha: Coccoidea: Eriococcidae). Biol. J. Linn. Soc. 83, 441–452. doi: 10.1111/j.1095-8312.2004.00396.x
Cooper, L., Bunnefeld, L., Hearn, J., Cook, J. M., Lohse, K., and Stone, G. N. (2020). Low coverage genomic data resolve the population divergence and gene flow history of an Australian rain forest fig wasp. Mol. Ecol. 29, 3649–3666. doi: 10.1101/2020.02.21.959205
Cronn, R., Knaus, B. J., Liston, A., Maughan, P. J., Parks, M., Syring, J. V., et al. (2012). Targeted enrichment strategies for next-generation plant biology. Am. J. Bot. 99, 291–311. doi: 10.3732/ajb.1100356
Danecek, P., Bonfield, J. K., Liddle, J., Marshall, J., Ohan, V., Pollard, M. O., et al. (2021). Twelve years of SAMtools and BCFtools. GigaScience 10, 1–4. doi: 10.1093/gigascience/giab008
Danzig, E. M. (1986). Coccids of the Far-Eastern USSR (Homoptera, Coccinea). Phylogenetic Analysis of Coccids in the World Fauna. New Delhi: Amerind Publishing Co.
Downie, D., and Gullan, P. (2004). Phylogenetic analysis of mealybugs (Hemiptera: Coccoidea: Pseudococcidae) based on DNA sequences from three nuclear genes, and a review of the higher classification. Syst. Entomol. 29, 238–260. doi: 10.1111/j.0307-6970.2004.00241.x
Esselstyn, J. A., Oliveros, C. H., Swanson, M. T., and Faircloth, B. C. (2017). Investigating Difficult Nodes in the Placental Mammal Tree with Expanded Taxon Sampling and Thousands of Ultraconserved Elements. Genome Biol. Evol. 9, 2308–2321. doi: 10.1093/gbe/evx168
Faircloth, B. C. (2016). PHYLUCE is a software package for the analysis of conserved genomic loci. Bioinformatics 32, 786–788. doi: 10.1093/bioinformatics/btv646
Faircloth, B. C., Branstetter, M. G., White, N. D., and Brady, S. G. (2015). Target enrichment of ultraconserved elements from arthropods provides a genomic perspective on relationships among Hymenoptera. Mol. Ecol. Resour. 15, 489–501. doi: 10.1111/1755-0998.12328
Faircloth, B. C., and Gilbert, M. (2017). Identifying conserved genomic elements and designing universal bait sets to enrich them. Methods Ecol. Evol. 8, 1103–1112. doi: 10.1111/2041-210x.12754
Faircloth, B. C., McCormack, J. E., Crawford, N. G., Harvey, M. G., Brumfield, R. T., and Glenn, T. C. (2012). Ultraconserved elements anchor thousands of genetic markers spanning multiple evolutionary timescales. Syst. Biol. 61, 717–726. doi: 10.1093/sysbio/sys004
Faircloth, B. C., Sorenson, L., Santini, F., and Alfaro, M. E. (2013). A Phylogenomic Perspective on the Radiation of Ray-Finned Fishes Based upon Targeted Sequencing of Ultraconserved Elements (UCEs). PLoS One 8:e65923. doi: 10.1371/journal.pone.0065923
Fernando Alda, V. A. T., Maxwell, J., Bernt Brandon, T., Waltz William, B., Ludt Brant, C., Faircloth Michael, E., et al. (2018). Resolving Deep Nodes in an Ancient Radiation of Neotropical Fishes in the Presence of Conflicting Signals from Incomplete Lineage Sorting. Syst. Biol. 68, 573–593. doi: 10.5061/dryad.K57430S
Forthman, M., Miller, C. W., and Kimball, R. T. (2019). Phylogenomic analysis suggests Coreidae and Alydidae (Hemiptera: Heteroptera) are not monophyletic. Zool. Scr. 48, 520–534. doi: 10.1111/zsc.12353
Forthman, M., Miller, C. W., and Kimball, R. T. (2020). Phylogenomics of the Leaf-Footed Bug Subfamily Coreinae (Hemiptera: Coreidae). Insect Syst. Divers. 4:2. doi: 10.1093/isd/ixaa009
García Morales, M. D. B., Miller, D. R., Miller, G. L., Ben-Dov, Y., and Hardy, N. B. (2016). ScaleNet: A literature-based model of scale insect biology and systematics. Database 2016:bav118. doi: 10.1093/database/bav118
Gruwell, M. E., Morse, G. E., and Normark, B. B. (2007). Phylogenetic congruence of armored scale insects (Hemiptera: Diaspididae) and their primary endosymbionts from the phylum Bacteroidetes. Mol. Phylogenet. Evol. 44, 267–280. doi: 10.1016/j.ympev.2007.01.014
Gullan, P., and Cook, L. (2007). Phylogeny and higher classification of the scale insects (Hemiptera: Sternorrhyncha: Coccoidea). Zootaxa 1668, 413–425. doi: 10.11646/zootaxa.1668.1.22
Gustafson, G. T., Alexander, A., Sproul, J. S., Pflug, J. M., Maddison, D. R., and Short, A. E. Z. (2019). Ultraconserved element (UCE) probe set design: Base genome and initial design parameters critical for optimization. Ecol. Evol. 9, 6933–6948. doi: 10.1002/ece3.5260
Hardy, N. B., Gullan, P. J., and Hodgson, C. J. (2008). A subfamily-level classification of mealybugs (Hemiptera: Pseudococcidae) based on integrated molecular and morphological data. Syst. Entomol. 33, 51–71. doi: 10.1111/j.1365-3113.2007.00408.x
Hardy, N. B., Peterson, D. A., and Normark, B. B. (2016). Nonadaptive radiation: Pervasive diet specialization by drift in scale insects? Evolution 70, 2421–2428. doi: 10.1111/evo.13036
Harris, R. S. (2007). Improved pairwise alignment of genomic DNA. University Park, PA: The Pennsylvania State University.
Heath, T. A., Hedtke, S. M., and Hillis, D. M. (2008). Taxon sampling and the accuracy of phylogenetic analyses. J. Syst. Evol. 46, 239–257.
Hoang, D. T., Chernomor, O., von Haeseler, A., Minh, B. Q., and Vinh, L. S. (2018). UFBoot2: Improving the Ultrafast Bootstrap Approximation. Mol. Biol. Evol. 35, 518–522. doi: 10.1093/molbev/msx281
Hodgson, C. (2014). Phenacoleachia, Steingelia, Pityococcus and Puto—neococcoids or archaeococcoids? An intuitive phylogenetic discussion based on adult male characters. Acta Zool. Bulg. 6, 41–50.
Hodgson, C., Denno, B., and Watson, G. W. (2021). The Infraorder Coccomorpha (Insecta: Hemiptera). Zootaxa 4979:226227. doi: 10.11646/zootaxa.4979.1.24
Hodgson, C. J., and Hardy, N. B. (2013). The phylogeny of the superfamily Coccoidea (Hemiptera: Sternorrhyncha) based on the morphology of extant and extinct macropterous males. Syst. Entomol. 38, 794–804. doi: 10.1111/syen.12030
Huang, W., Li, L., Myers, J. R., and Marth, G. T. (2012). ART: A next-generation sequencing read simulator. Bioinformatics 28, 593–594. doi: 10.1093/bioinformatics/btr708
Husnik, F., and McCutcheon, J. P. (2016). Repeated replacement of an intrabacterial symbiont in the tripartite nested mealybug symbiosis. Proc. Natl. Acad. Sci. U. S. A. 113, E5416–E5424. doi: 10.1073/pnas.1603910113
Institiute of Insect Sciences, (2019). Phenacoccus solenopsis genome sequencing and assembly; GenBank; Accession Number: GCA_009761765.1
International Psyllid Genome Consortium (2013). Asian citrus psyllid genome sequencing project; GenBank; Accession Number: GCA_000475195.1
Johnson, K. P. (2019). Putting the genome in insect phylogenomics. Curr. Opin. Insect Sci. 36, 111–117.
Kalyaanamoorthy, S., Minh, B. Q., Wong, T. K. F., von Haeseler, A., and Jermiin, L. S. (2017). ModelFinder: Fast model selection for accurate phylogenetic estimates. Nat. Methods 14, 587–589. doi: 10.1038/nmeth.4285
Kapranas, A., and Tena, A. (2015). Encyrtid parasitoids of soft scale insects: Biology, behavior, and their use in biological control. Annu. Rev. Entomol. 60, 195–211. doi: 10.1146/annurev-ento-010814-021053
Katoh, K., and Standley, D. M. (2013). MAFFT Multiple Sequence Alignment Software Version 7: Improvements in Performance and Usability. Mol. Biol. Evol. 30, 772–780. doi: 10.1093/molbev/mst010
Kieran, T. J., Gordon, E. R. L., Forthman, M., Hoey-Chamberlain, R., Kimball, R. T., Faircloth, B. C., et al. (2019). Insight from an ultraconserved element bait set designed for hemipteran phylogenetics integrated with genomic resources. Mol. Phylogenet. Evol. 130, 297–303. doi: 10.1016/j.ympev.2018.10.026
Kondo, T., Gullan, P. J., and Williams, D. J. (2008). Coccidology. The study of scale insects (Hemiptera: Sternorrhyncha: Coccoidea). Cienc. Tecnol. Agropecu. 9, 55–61. doi: 10.21930/rcta.vol9_num2_art:118
Lanfear, R., Calcott, B., Kainer, D., Mayer, C., and Stamatakis, A. (2014). Selecting optimal partitioning schemes for phylogenomic datasets. BMC Evol. Biol. 14:82. doi: 10.1186/1471-2148-14-82
Lanfear, R., Frandsen, P. B., Wright, A. M., Senfeld, T., and Calcott, B. (2016). PartitionFinder 2: New Methods for Selecting Partitioned Models of Evolution for Molecular and Morphological Phylogenetic Analyses. Mol. Biol. Evol. 34, 772–773. doi: 10.1093/molbev/msw260
Lemmon, A. R., Emme, S. A., and Lemmon, E. M. (2012). Anchored hybrid enrichment for massively high-throughput phylogenomics. Syst. Biol. 61, 727–744. doi: 10.1093/sysbio/sys049
Lou, R. N., Jacobs, A., Wilder, A. P., and Therkildsen, N. O. (2021). A beginner’s guide to low-coverage whole genome sequencing for population genomics. Mol. Ecol. 30, 5966–5993. doi: 10.1111/mec.16077
Lunter, G., and Goodson, M. (2011). Stampy: A statistical algorithm for sensitive and fast mapping of Illumina sequence reads. Genome Res. 21, 936–939. doi: 10.1101/gr.111120.110
Manni, M., Berkeley, M. R., Seppey, M., Simao, F. A., and Zdobnov, E. M. (2021). BUSCO Update: Novel and Streamlined Workflows along with Broader and Deeper Phylogenetic Coverage for Scoring of Eukaryotic, Prokaryotic, and Viral Genomes. Mol. Biol. Evol. 38, 4647–4654. doi: 10.1093/molbev/msab199
McCormack, J. E., Faircloth, B. C., Crawford, N. G., Gowaty, P. A., Brumfield, R. T., and Glenn, T. C. (2012). Ultraconserved elements are novel phylogenomic markers that resolve placental mammal phylogeny when combined with species-tree analysis. Genome Res. 22, 746–754. doi: 10.1101/gr.125864.111
McCormack, J. E., Harvey, M. G., Faircloth, B. C., Crawford, N. G., Glenn, T. C., and Brumfield, R. T. (2013). A phylogeny of birds based on over 1,500 loci collected by target enrichment and high-throughput sequencing. PLoS One 8:e54848. doi: 10.1371/journal.pone.0054848
Miller, D. R., and Davidson, J. A. (1990). A list of the armored scale insect pests. World Crop Pests 4, 299–306.
Miller, D. R., Miller, G. L., Hodges, G. S., and Davidson, J. A. (2005). Introduced scale insects (Hemiptera: Coccoidea) of the United States and their impact on U.S. agriculture. Proc. Entomol. Soc. Wash. 107, 123–158.
Minh, B. Q., Schmidt, H. A., Chernomor, O., Schrempf, D., Woodhams, M. D., von Haeseler, A., et al. (2020). IQ-TREE 2: New Models and Efficient Methods for Phylogenetic Inference in the Genomic Era. Mol. Biol. Evol. 37, 1530–1534. doi: 10.1093/molbev/msaa015
Mirarab, S., Bayzid, M. S., and Warnow, T. (2016). Evaluating summary methods for multilocus species tree estimation in the presence of incomplete lineage sorting. Syst. Biol. 65, 366–380. doi: 10.1093/sysbio/syu063
Misof, B., Liu, S., Meusemann, K., Peters, R. S., Donath, A., Mayer, C., et al. (2014). Phylogenomics resolves the timing and pattern of insect evolution. Science 346, 763–767. doi: 10.1126/science.1257570
Mongue, A. J., Michaelides, S., Coombe, O., Tena, A., Kim, D.-S., Normark, B. B., et al. (2021). Sex, males, and hermaphrodites in the scale insect Icerya purchasi. Evolution 75, 2972–2983. doi: 10.1111/evo.14233
Morse, G. E., and Normark, B. B. (2006). A molecular phylogenetic study of armoured scale insects (Hemiptera: Diaspididae). Syst. Entomol. 31, 338–349. doi: 10.1111/j.1365-3113.2005.00316.x
Musher, L. J., and Cracraft, J. (2018). Phylogenomics and species delimitation of a complex radiation of Neotropical suboscine birds (Pachyramphus). Mol. Phylogenet. Evol. 118, 204–221. doi: 10.1016/j.ympev.2017.09.013
Newman, C. E., and Austin, C. C. (2016). Sequence capture and next-generation sequencing of ultraconserved elements in a large-genome salamander. Mol. Ecol. 25, 6162–6174. doi: 10.1111/mec.13909
Normark, B. B. (2003). The evolution of alternative genetic systems in insects. Annu. Rev. Entomol. 48, 397–423. doi: 10.1146/annurev.ento.48.091801.112703
Normark, B. B. (2004). The Strange Case of the Armored Scale Insect and Its Bacteriome. PLoS Biol. 2:e43. doi: 10.1371/journal.pbio.0020043
Normark, B. B., Okusu, A., Morse, G. E., Peterson, D. A., Itioka, T., and Schneider, S. A. (2019). Phylogeny and classification of armored scale insects (Hemiptera: Coccomorpha: Diaspididae). Zootaxa 4616, 1–98. doi: 10.11646/zootaxa.4616.1.1
Peterson, D. A., Hardy, N. B., Morse, G. E., Itioka, T., Wei, J., and Normark, B. B. (2020). Nonadaptive host-use specificity in tropical armored scale insects. Ecol. Evol. 10, 12910–12919. doi: 10.1002/ece3.6867
Poveda-Martínez, D., Aguirre, M. B., Logarzo, G., Hight, S. D., Triapitsyn, S., and Diaz-Sotero, H. (2020). Species complex diversification by host plant use in an herbivorous insect: The source of Puerto Rican cactus mealybug pest and implications for biological control. Ecol. Evol. 10, 10463–10480. doi: 10.1002/ece3.6702
Prjibelski, A., Antipov, D., Meleshko, D., Lapidus, A., and Korobeynikov, A. (2020). Using SPAdes De Novo Assembler. Curr. Protoc. Bioinformatics 70:e102. doi: 10.1002/cpbi.102
Puerto Rico Science, Technology and Research Trust (2019). Aphis glycines genome sequencing and assembly; GenBank; Accession Number: GCA_009761285.1
Qin, Y. G., Zhou, Q. S., Yu, F., Wang, X. B., Wei, J. F., Zhu, C. D., et al. (2018). Host specificity of parasitoids (Encyrtidae) toward armored scale insects (Diaspididae): Untangling the effect of cryptic species on quantitative food webs. Ecol. Evol. 8, 7879–7893. doi: 10.1002/ece3.4344
Quinlan, A. R., and Hall, I. M. (2010). BEDTools: A flexible suite of utilities for comparing genomic features. Bioinformatics 26, 841–842. doi: 10.1093/bioinformatics/btq033
Rosenblueth, M., Martínez-Romero, J., Ramírez-Puebla, S. T., Vera-Ponce de León, A., Rosas-Pérez, T., Bustamante-Brito, R., et al. (2018). Endosymbiotic microorganisms of scale insects. TIP. Rev. Espec. Cienc. Quím. Biol. 21, 53–69. doi: 10.1016/j.recqb.2017.08.006
Rosenblueth, M., Sayavedra, L., Sámano-Sánchez, H., Roth, A., and Martínez-Romero, E. (2012). Evolutionary relationships of flavobacterial and enterobacterial endosymbionts with their scale insect hosts (Hemiptera: Coccoidea). J. Evol. Biol. 25, 2357–2368. doi: 10.1111/j.1420-9101.2012.02611.x
Ross, L., Pen, I., and Shuker, D. M. (2010). Genomic conflict in scale insects: The causes and consequences of bizarre genetic systems. Biol. Rev. 85, 807–828. doi: 10.1111/j.1469-185X.2010.00127.x
Ross, L., Shuker, D. M., Normark, B. B., and Pen, I. (2012). The role of endosymbionts in the evolution of haploid-male genetic systems in scale insects (Coccoidea). Ecol. Evol. 2, 1071–1081. doi: 10.1002/ece3.222
Sabree, Z. L., Huang, C. Y., Okusu, A., Moran, N. A., and Normark, B. B. (2013). The nutrient supplying capabilities of Uzinura, an endosymbiont of armoured scale insects. Environ. Microbiol. 15, 1988–1999. doi: 10.1111/1462-2920.12058
Schneider, S. A., and LaPolla, J. S. (2011). Systematics of the mealybug tribe Xenococcini (Hemiptera: Coccoidea: Pseudococcidae), with a discussion of trophobiotic associations with Acropyga Roger ants. Syst. Entomol. 36, 57–82. doi: 10.1111/j.1365-3113.2010.00546.x
Schneider, S. A., Okusu, A., and Normark, B. B. (2018). Molecular phylogenetics of Aspidiotini armored scale insects (Hemiptera: Diaspididae) reveals rampant paraphyly, curious species radiations, and multiple origins of association with Melissotarsus ants (Hymenoptera: Formicidae). Mol. Phylogenet. Evol. 129, 291–303. doi: 10.1016/j.ympev.2018.09.003
Smith, B. T., Harvey, M. G., Faircloth, B. C., Glenn, T. C., and Brumfield, R. T. (2014). Target capture and massively parallel sequencing of ultraconserved elements for comparative studies at shallow evolutionary time scales. Syst. Biol. 63, 83–95. doi: 10.1093/sysbio/syt061
Stamatakis, A. (2014). RAxML version 8: A tool for phylogenetic analysis and post-analysis of large phylogenies. Bioinformatics 30, 1312–1313. doi: 10.1093/bioinformatics/btu033
Starrett, J., Derkarabetian, S., Hedin, M., Bryson, R. W. Jr., McCormack, J. E., and Faircloth, B. C. (2017). High phylogenetic utility of an ultraconserved element probe set designed for Arachnida. Mol. Ecol. Res. 17, 812–823. doi: 10.1111/1755-0998.12621
Tagliacollo, V. A., and Lanfear, R. (2018). Estimating Improved Partitioning Schemes for Ultraconserved Elements. Mol. Biol. Evol. 35, 1798–1811. doi: 10.1093/molbev/msy069
Takagi, S. (1992). A contribution to conchaspidid systematics (Homoptera: Coccoidea). Insecta Matsumurana 46, 1–71.
University of Delhi (2018). De novo assembly of M.hirsutus, a model organism important for understanding epigenetic regulation; GenBank; Accession Number: GCA_003261595.1
Van Dam, M. H., Trautwein, M., Spicer, G. S., and Esposito, L. (2019). Advancing mite phylogenomics: Designing ultraconserved elements for Acari phylogeny. Mol. Ecol. Resour. 19, 465–475. doi: 10.1111/1755-0998.12962
Vea, I. M., and Grimaldi, D. A. (2012). Phylogeny of ensign scale insects (Hemiptera: Coccoidea: Ortheziidae) based on the morphology of recent and fossil females. Syst. Entomol. 37, 758–783. doi: 10.1111/j.1365-3113.2012.00638.x
Vea, I. M., and Grimaldi, D. A. (2016). Putting scales into evolutionary time: The divergence of major scale insect lineages (Hemiptera) predates the radiation of modern angiosperm hosts. Sci. Rep. 6, 1–11. doi: 10.1038/srep23487
Wang, Y., Zhang, R., Ma, Y., Li, J., Fan, F., Liu, X., et al. (2021). Low-Coverage Whole Genomes Reveal the Higher Phylogeny of Green Lacewings. Insects 12:857. doi: 10.3390/insects12100857
Wang, Z., Gerstein, M., and Snyder, M. (2009). RNA-Seq: A revolutionary tool for transcriptomics. Nat. Rev. Genet. 10, 57–63. doi: 10.1038/nrg2484
Winker, K., Glenn, T. C., and Faircloth, B. C. (2018). Ultraconserved elements (UCEs) illuminate the population genomics of a recent, high-latitude avian speciation event. PeerJ 6:e5735. doi: 10.7717/peerj.5735
Yamada, K. D., Tomii, K., and Katoh, K. (2016). Application of the MAFFT sequence alignment program to large data—reexamination of the usefulness of chained guide trees. Bioinformatics 32, 3246–3251. doi: 10.1093/bioinformatics/btw412
Yang, P., Yu, S., Hao, J., Liu, W., Zhao, Z., Zhu, Z., et al. (2019). Genome sequence of the Chinese white wax scale insect Ericerus pela: The first draft genome for the Coccidae family of scale insects. GigaScience 8:giz113. doi: 10.1093/gigascience/giz113
Yokogawa, T., and Yahara, T. (2009). Mitochondrial phylogeny certified PGL (Paternal Genome Loss) is of single origin and haplodiploidy sensu stricto (arrhenotoky) did not evolve from PGL in the scale insects (Hemiptera: Coccoidea). Genes Genet. Syst. 84, 57–66. doi: 10.1266/ggs.84.57
Young, A. D., and Gillung, J. P. (2020). Phylogenomics-pronciples, opportunities and pitfalls of big-data phylogenetics. Syst. Entomol. 45, 225–247.
Young, A. D., Lemmon, A. R., Skevington, J. H., Mengual, X., Ståhls, G., Reemer, M., et al. (2016). Anchored enrichment dataset for true flies (order Diptera) reveals insights into the phylogeny of flower flies (family Syrphidae). BMC Evol. Biol. 16:143. doi: 10.1186/s12862-016-0714-0
Zhang, F., Ding, Y., Zhu, C. D., Zhou, X., Orr, M. C., Scheu, S., et al. (2019). Phylogenomics from low-coverage whole-genome sequencing. Methods Ecol. Evol. 10, 507–517. doi: 10.1111/2041-210X.13145
Zhang, Y. M., Williams, J. L., and Lucky, A. (2019). Understanding UCEs: A Comprehensive Primer on UsingUltraconserved Elements for Arthropod Phylogenomics. Insect Syst. Divers. 3:3. doi: 10.1093/isd/ixz016
Zhang, Y. M., Buffington, M. L., Looney, C., László, Z., Shorthouse, J. D., Ide, T., et al. (2020). UCE data reveal multiple origins of rose gallers in North America: Global phylogeny of Diplolepis Geoffroy (Hymenoptera: Cynipidae). Mol. Phylogenet. Evol. 153:106949. doi: 10.1016/j.ympev.2020.106949
Keywords: Coccoidea, ultraconserved elements, probe design, low-coverage whole genome sequencing, phylogenomics
Citation: Liu D, Niu M, Lu Y, Wei J and Zhang H (2022) Taxon-specific ultraconserved element probe design for phylogenetic analyses of scale insects (Hemiptera: Sternorrhyncha: Coccoidea). Front. Ecol. Evol. 10:984396. doi: 10.3389/fevo.2022.984396
Received: 02 July 2022; Accepted: 24 August 2022;
Published: 30 September 2022.
Edited by:
Cleber Galvão, Oswaldo Cruz Institute (FIOCRUZ), BrazilReviewed by:
Nan Song, Henan Agricultural University, ChinaTroy J. Kieran, Oak Ridge Institute for Science and Education (ORISE), United States
Copyright © 2022 Liu, Niu, Lu, Wei and Zhang. This is an open-access article distributed under the terms of the Creative Commons Attribution License (CC BY). The use, distribution or reproduction in other forums is permitted, provided the original author(s) and the copyright owner(s) are credited and that the original publication in this journal is cited, in accordance with accepted academic practice. No use, distribution or reproduction is permitted which does not comply with these terms.
*Correspondence: Jiufeng Wei, d2pmZW5nQG53c3VhZi5lZHUuY24=; Hufang Zhang, emhfaHVmYW5nQHNvaHUuY29t