- 1School of Economics, Jiangxi University of Finance and Economics, Nanchang, China
- 2School of Finance, Southwestern University of Finance and Economics, Chengdu, China
- 3College of Economics and Management, China Jiliang University, Hangzhou, China
This paper aims to explore the impact of multiple cropping on farmers’ welfare level and provide the theoretical and empirical basis for solving relative poverty in rural areas in the future. The paper uses data from the field survey of 1,120 farmers in Hubei in 2018 and uses the Endogenous Transformation Regression Model (ESR) and generalized propensity score matching (GPSM) model to construct a counterfactual framework. The paper analyses the effect of multiple cropping on farmers’ relative poverty and examines its mechanism. The result shows that: Multiple cropping of farmland can reduce the relative poverty of farmers through the mechanism of yield improvement path and factor intensification path. Under the counterfactual hypothesis, the relative poverty of farmers would increase by 28.43% if the farmers who participated in the multiple cropping did not; and that the relative poverty of the farmers would decrease by 29.57% if the farmers who did not participate in the multiple cropping participated. From the perspective of multiple cropping, the poverty reduction effect of multiple cropping in paddy fields is higher than that of dry land. From the perspective of the degree of multiple cropping, the poverty reduction effects of paddy fields and dryland will experience an increasing return to scale as the multiple cropping index increases. When the household equivalent scale adjustment coefficient is not used to eliminate the impact of family population structure on the “family per capita comparable income,” the artificially high results estimated by the model cannot truly reflect the poverty-reducing effect of multi-cropping of farmland. This paper argues that the government can guide farmers to choose the suitable mode of multiple cropping to reduce farmers’ vulnerability to relative poverty.
Introduction
Eliminating poverty is a common mission, and China has achieved a world-renowned miracle in solving the problem of rural poverty. In 2020, China achieved the strategic goal of building a moderately prosperous society in an all-round way, with all rural poor people lifted out of poverty. However, this does not mean that China will not experience poverty after 2020 (Tan and Tan, 2021). In 2019, China’s per capita GDP was already in the ranks of upper-middle-income countries, but its current poverty standard is far below the moderate poverty line of $3.2 set by the World Bank for lower-middle-income countries. This means that China’s recent poverty reduction achievements will be greatly reduced if measured by the high-income poverty standard. Even though China has done a lot in solving absolute poverty, the problem of relative poverty still exists (Fan and Zou, 2021). The problem of relative poverty in China is a prominent, deep-seated contradiction that restricts the development of poverty-stricken areas, and poverty occurs from time to time (Su et al., 2021). Therefore, poverty reduction in China still faces many challenges. The task of poverty reduction in China remains daunting, and it is increasingly difficult to reduce poverty through traditional anti-poverty measures. With the decline of land’s social security and income-increasing functions, farmers’ investment time and labor on land continues to decrease, leading to a serious decline in land utilization or even abandonment. The problem of farmland supply has become a major constraint for promoting industrial poverty alleviation smoothly. The resulting “non-agriculturalization” and “agriculturalization” are bound to affect the achievement of agricultural modernization. Has rural land really become a shackle that hinders the development of farmers? What kind of role should farmlands play in relative rural poverty management? Answering the above questions scientifically has significant implications for formulating farmland policies and the future governance of relative poverty.
Literature review
Farmland has always been the material basis for farmers to survive and develop, and plays a basic social security role for farmers. The use of farmland directly affects farmers’ income and poverty (Cai et al., 2019). At present, researchers mainly focus on the impact of farmland consolidation and farmland transfer on poverty (Yan et al., 2019; Zhang et al., 2020, 2022; Wang et al., 2021). Generally speaking, the fragmentation and over-cultivation of farmland will lead to the loss of agricultural production efficiency, accompanied by the phenomenon of spatial aggregation of poverty (Strier, 2019; Aruna et al., 2020; Liu et al., 2020). Farmland consolidation can improve farmland quality and agricultural production environment through farmland reclamation and farmland development (Chen et al., 2019), thereby increasing farmers’ natural capital and household income and alleviating farmers’ poverty. However, the differentiated goals of different farmland remediation models will lead to different poverty reduction effects (Liu et al., 2017), and the public-private partnership (PPP) model has a better poverty reduction effect than the government-led model (Wang et al., 2019). The transfer of farmland will affect the poverty of farmers (Zhang and Sun, 2018), improve the scale operation of farmers and promote poverty reduction through economies of scale (Wu and Lin, 2018). However, the transfer of too much farmland will increase farmers’ investment, resulting in increased investment risks and poverty incidence for farmers (Peng and Zhang, 2015; Wang et al., 2021). Farmers who have transferred their farmland will have more time and energy to increase their income and reduce their poverty through various concurrent business activities (Cai and Xia, 2019; Mihai, 2020; Zhang and Meng, 2021).
Multi-cropping is also an important aspect of measuring farmland use, but there are currently few studies on the relative poverty of farmers and more on the impact of multi-cropping on agricultural production (Battisti et al., 2020; Guo et al., 2021; Shi et al., 2021). From the perspective of farmland systems, scientifically improving the multiple cropping index of farmlands and excavating the potential for intensive utilization of farmland is one of the important ways to increase agricultural production and farmers’ income (Tang et al., 2010, 2015; Wu et al., 2014). The research on the multiple cropping index of farmland has become a frontier and hot research direction in the fields of geography, ecology and agronomy. On the one hand, under the condition that the total amount of farmland remains unchanged, the multi-cropping model can efficiently utilize the existing farmland resources in time and space, thereby increasing the planting area and increasing agricultural yield (Liu, 1997; Xu and Liu, 2014). For example, systems with multiple harvests of the same crop are denser in some Asian rice-based systems, while others are more diversified (Xu and Liu, 2014; Liu et al., 2015), with different types of crops growing together or sequentially and interacting. Higher diversity is expected to increase the sustainability of crop production (Yan et al., 2005; Wu et al., 2007; Li and Li, 2012; Huang and Sun, 2017), pest regulation (Liu, 2002; Zuo et al., 2009; Erb et al., 2013), resistance to climate events (Fan and Wu, 2004; Rogstad and Pelikan, 2013), and reduce fertilizer use in associations with legumes. All of these can also increase production or profitability in the short or long term (Asfaw et al., 2012; Sheridan et al., 2017; Zeng et al., 2020). In cropping systems with a wide variety of crops, multiple crops also allow for the spread and diversification of risk to suit different growing seasons and different crops for personal use or the markets (Rana et al., 2007; Timo and Anni, 2013; Michalscheck et al., 2018). Studies have shown that China’s multi-cropping accounts for 50% of the total farmland, its sown area accounts for about 2/3 of the total sown area, and the crop yield accounts for about 3/4 of the total output (Wang et al., 2022; Yin et al., 2022). Improving the multiple cropping index is one of the important factors for the continuous increase of agricultural production, and has played an important role in ensuring stable agricultural production and reducing poverty (Zhang et al., 2019; Mustafin and Kantarbayeva, 2021; Sun et al., 2021).
On the other hand, multiple cropping patterns and their changes have an important impact on the geophysical and geochemical cycle processes of terrestrial ecosystems, driving regional ecological environment changes (Byela et al., 2021; Xiang et al., 2022). Multi-cropping of different crop combinations will increase the stability of farmland planting systems and improve nutrient recycling and reuse (Amin et al., 2016; Augustine et al., 2018). However, the excessive use of pesticides, fertilizers, and production facilities will lead to soil pollution and reduced water quality, thereby increasing greenhouse gas emissions and destroying the ecological environment of the region (Huang and Sun, 2017). In addition, planting second or third season crops may increase the risk of crop failure (Yang et al., 2010) and drive up production costs (Shi et al., 1998; Zhang, 2000), thereby detrimental to agricultural production and poverty reduction.
The efficiency of farmland use will affect the production efficiency of agriculture. Very low agricultural production efficiency will make agriculture fall into a vicious circle of “low comparative income–low agricultural enthusiasm–low farmland utilization rate–increased poverty of farmers.” (Zhang, 2000; Mthethwa and Wale, 2020; Tang and Cai, 2020; Wang et al., 2021). The key to breaking the vicious cycle of involution in agricultural production is to improve the utilization rate of farmland by farmers. The manner, degree, and intensity of farmland use are all ways to improve the utilization rate of farmlands. The first can be attributed to the question of “what to plant” on farmlands, and the latter two can be attributed to the question of “how to plant” on farmland. Due to the production conditions of farmlands, planting policy guidance, and planting habits, farmers will not change the planting structure of farmlands in the short term. This means that the utilization rate of farmlands will not be affected by “what to plant” in the short term. The degree of farmland use generally refers to the degree of multiple cropping of farmlands, and the intensity of farmland use generally refers to the input of agricultural production factors such as labor, time, chemical fertilizers, and pesticides. The increase in the degree of multiple cropping is usually accompanied by an increase in agricultural production factors. As such, the core of the problem of “how to plant” is the index of multiple cropping of farmlands, which is also one of the effective ways to improve the utilization rate of farmlands and increase the comparative income of agriculture in the short term. Therefore, multiple cropping should alleviate poverty to a certain extent.
In recent years, the comparative efficiency of China’s agriculture has been low, and the enthusiasm of small-scale farmers in farming has also declined (Xu and Liu, 2014; Xiang et al., 2019). The multiple cropping index of high-productivity high-quality farmland in many plains in China is declining (Li and Li, 2012; Xiang et al., 2019). Coupled with crop rotation and fallowing in some areas, this has resulted in a decrease in the actual sown area (Zuo et al., 2009; Yang et al., 2010), which to some extent offsets the positive effect of the increase in yield (Shi et al., 1998; Huang and Sun, 2017). Therefore, it is of great scientific value and practical significance to rethink the multi-cropping of farmland to improve the utilization rate of farmland and reduce poverty given that the extensional expansion of farmland is difficult to meet and the increase of grain yield per unit is more difficult.
Materials and methods
Introduction to the study area
Hubei Province is located in the south-central part of China, and its terrain is an incomplete basin surrounded by mountains on the east, west and north sides and a low and flat middle. Mountainous areas in Hubei Province account for 56%, hills account for 24%, and plain lake areas account for 20%. Most of Hubei Province has a humid subtropical monsoon climate, and the geographical distribution of precipitation shows a decreasing trend from south to north. The main crops in Hubei Province are rice, cotton, rapeseed and soybeans. In 2021, the added value of the primary industry will be 466.167 billion yuan, and the per capita disposable income of rural residents will reach 18,931 yuan. Hubei Province is facing a critical period of transition from traditional agriculture to modern agriculture. Multiple cropping of farmland can improve the level of land use and welfare of farmers. Therefore, it is of great significance to explore the poverty reduction effect of multiple cropping of farmland.
Sample and data source
We use the data from the field survey data of households in Hubei Province in 2018. The survey covered basic information on household population, natural and physical assets, production and operation conditions, land transfer behavior, farmers’ policy cognition, etc. The research site was in the Jianli and Qichun counties of Hubei Province, involving a total of 44 villages in 11 towns. Twenty-six households were investigated in each village, and a total of 1,144 households were investigated. Twenty-four invalid questionnaires were removed, and a total of 1,120 valid samples were obtained. In addition, the farmers in this paper are small farmers with a small operating area.
Definition of variables
Relative poverty
There is currently no fixed method for measuring the relative poverty of rural households. The per capita income of the family has always been the conventional method to measure poverty, but the per capita income does not consider the family structure. If only the per capita income is used to measure, it may lead to the problem of “false poverty.” At present, the mainstream method for measuring relative poverty in the world is 50% of the median per capita income stipulated by the Organization for Economic Co-operation and Development (OECD) (Parodi and Sciulli, 2012; Zhang et al., 2021). This method solves the problem of differences in family structure through the “family equivalent size adjustment coefficient” and then accurately measures the level of family welfare. In addition, due to the long-term and unobservable nature of poverty, this paper does not directly use the per capita income adjusted by the equivalent scale to measure relative poverty. Rather, it calculates the poverty vulnerability of farmers based on per capita income. Poverty vulnerability indicates the probability of farmers falling into poverty or inability to get out of poverty in the future, which is more instructive for future poverty reduction work.
Multiple cropping behavior of farmlands
Multiple cropping is one of the effective ways to improve land intensification, but the difference in farmland conditions will affect the potential farmland’s willingness to engage in multiple cropping. There is more than one type of farmland in our survey, and this paper includes all kinds of farmland, including paddy fields and dryland. This paper focuses on the intention and degree of multiple cropping to investigate the multiple cropping behavior of different types of farmland. The following six variables were used to measure; whether the farmland was engaged in multiple cropping, whether the paddy field had multiple cropping, whether the dryland had multiple cropping, the farmland multiple cropping index, the paddy field multiple cropping index, and the dryland multiple cropping index.
Control variables
In this paper, variables affecting household wealth and farming are selected as control variables. Table 1 is the calculation method and descriptive statistics of the variables. The control variables are selected as follows:
• Hours of work per capita. Higher migrant income is more likely to reduce poverty.
• Family training ratio. Skills training can enhance human capital and enhance job competitiveness.
• Family burden coefficient. A too-large family burden coefficient can easily lead to family poverty.
• Percentage of family coverage. New rural cooperative endowment insurance can effectively alleviate the risk of a family falling into poverty.
• Per capita household area. The greater the land area, the stronger the ability to resist risk.
• Household transfer area. Transferring land is more conducive to scale operations and poverty reduction.
• Irrigation ratio of farmland. Irrigation facilities can increase agricultural productivity and resist natural disasters.
• Number of varieties planted. Diversified planting can share the loss of natural disasters, but too many varieties will lead to the loss of productivity.
• Climate Change. Weather anomalies can affect agricultural production and exacerbate poverty.
Model
Measurement of relative poverty
(1) We calculated the household per capita comparable income. The cost of living varies according to family size, structure, and level of education, and some goods (televisions, refrigerators, air conditioners, etc.) have shared characteristics. Measurements of poverty using household per capita income would result in families with different structures but the same per capita income being classified as homogeneous poor families. Therefore, “household per capita comparable income” needs to be calculated. The equivalent size adjustment coefficient is used to measure the welfare level of families with different characteristics. It is the ratio of the general family expenditure level to the standard family expenditure level. In this paper, the OECD equivalent scale adjustment method was used to assign the coefficient of the first adult in the family to 1, the coefficient of an additional adult to 0.7, and the coefficient of children under 14 years old to 0.5.
(2) Relative poverty measurement method. The three-stage feasible generalized least square method is used to measure the relative poverty. The measurement method is as follows:
In Eq. 1, i means farmers and t means time, Y is the welfare level of farmers (per capita income), Z is the relative poverty standard, Vit is the relative poverty of peasant household i in the period of t, Yi,t+1 is the welfare level of peasant household i in the period of t+1, namely the relative poverty value:
Model of whether the farmland is engaged in multiple cropping
Multiple cropping will increase agricultural output and cost. Assuming that the potential net income per unit of farmer i with and without multiple cropping is and , respectively, and that the condition for selecting multiple cropping is , then the decision model for choosing multiple cropping is:
In Eq. 3, Gi is whether to choose the farmland for multiple cropping. If famers choose the farmland for multiple cropping, Gi will equal 1; otherwise, it will equal 0; and the relative poverty value Vi is:
In Eq. 4, Xi is the covariable, α and β are the parameters to be estimated, and εi is the random error term. In this paper, we take Eq. 3 as the Decision Equation; and Eq. 4 is used as the Outcome Equation.
To simultaneously solve the problems of missing variables and endogeneity, this paper uses the Endogenous Switching Regression (ESR) method proposed by Lokshin and Sajaia (2004). Comparing the expected values of relative poverty of households with and without multiple cropping, the average treatment effect of the treated (ATT) is:
The average treatment effect of the untreated (ATU) is:
Impact of multiple cropping on farmers’ relative poverty
To infer the causal relationship between the multiple cropping index of farmland and relative poverty, an evaluation model that can handle the key variables as continuous variables is needed (Lokshin and Sajaia, 2004). The generalized propensity score matching (GPSM) method can be used for estimation and the operation idea is as follows:
Given the covariable X, the maximum likelihood method is used to estimate the conditional probability distribution H(Ti) of the continuous processing variable T:
The expected value of the result variable Fi when the processing variable is t:
In Eq. 8, N is the sample observation value, is the conditional probability density prediction value of the treatment variable, and the value range of the treatment variable is divided into N subintervals . In each subinterval, the causal effect of the multiple cropping index on the relative poverty of farmers can be estimated.
Empirical results
Table 2 shows the simultaneous estimation results of the multiple cropping of all farmlands and paddy fields, and the relative poverty model of farmers. Columns (1)–(3) of Table 3 are the simultaneous estimation results of the dryland multi-cropping and rural household relative poverty model, and columns (4)–(6) are the estimation results of the instrumental variables of the whole sample.
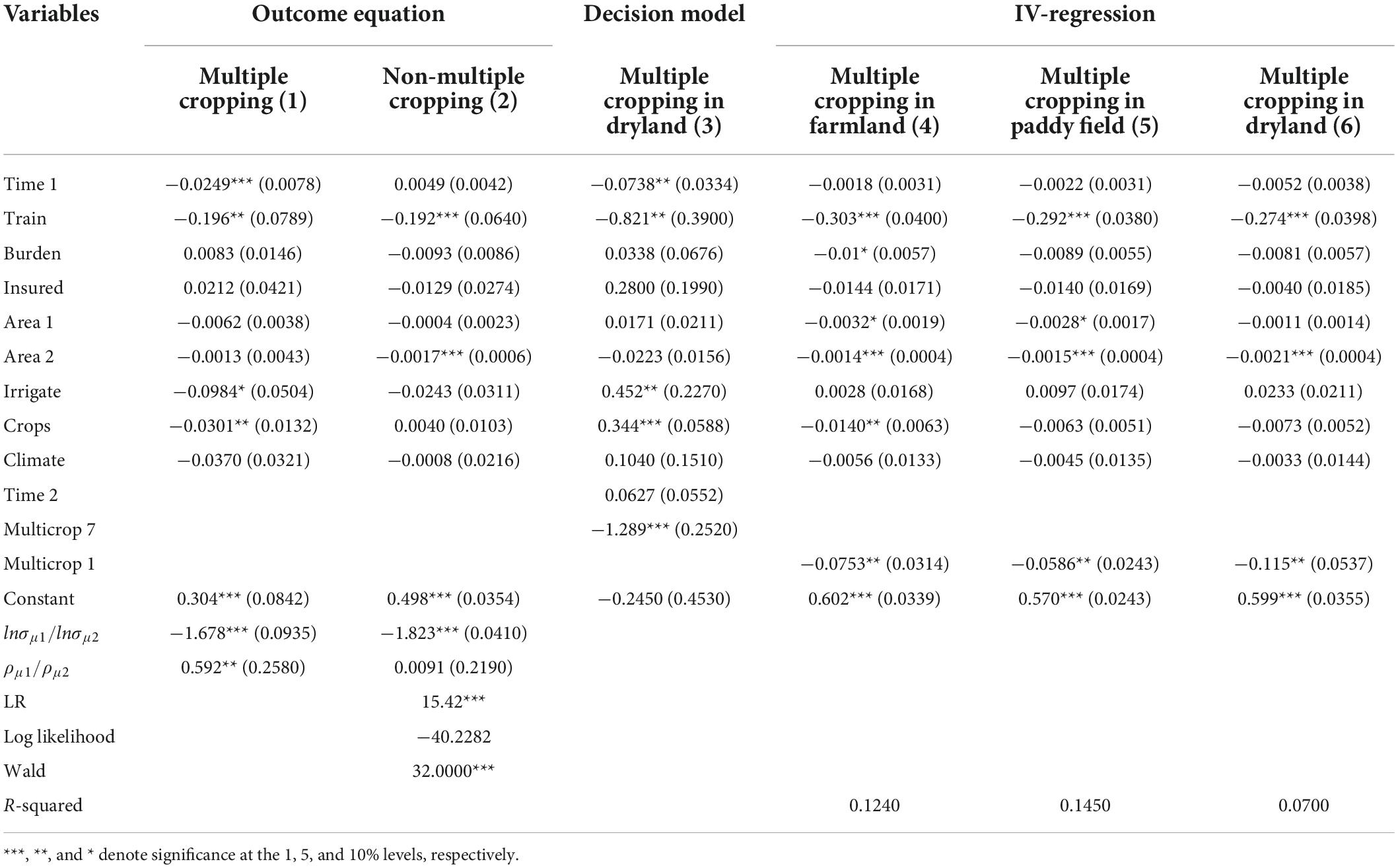
Table 3. Endogenous conversion model estimation results of dryland multiple cropping and the regression results of instrumental variables.
The Endogenous Switching Regression model results and analysis on the relative poverty of farmers by multiple cropping of all farmlands
From the total sample, the LR value at 1% rejects the null hypothesis that the equation and the result equation are independent of each other, while Wald value at 1% passes the test, indicating that the fitting effect is good. ρμ1 and ρμ2 are significant at the 1% level. This indicates that the multiple planting households and the non-multiple planting households are not randomly allocated. Farmers will adjust according to the change of utility before and after multiple planting, so there is a problem of self-selection. The negative ρμ1 indicates that the relative poverty of households with multiple seeds is lower than that of other households in the sample, and the negative ρμ2 indicates that the relative poverty of households without multiple seeds is higher than that of other households in the sample.
Column (1) of Table 2 reports the estimated results of the relative poverty of farmers using multiple cropping. Column (2) is the estimated result of the relative poverty of farmers who have not adopted multiple cropping, and Column (3) is the regression result of the multiple cropping selection model of farmland. The results show that family training significantly reduced the probability of selecting farmlands for multiple cropping. This suggests that the more skilled a household is, the more likely it is to be engaged in off-farm work. The family burden coefficient significantly reduced the probability of selecting farmlands for multiple cropping, because there were more old people and children in the family. A large number of old people and children leads to an insufficient labor force to improve the degree of farmland use. The per capita household area significantly increased the probability of multiple cropping of farmland, because land scale management can improve income and increase the willingness to adopt multiple cropping. The transferred area of households significantly increased the probability of multiple cropping of farmland, indicating that households had a strong enthusiasm for farming, and the land utilization rate would be improved to the maximum extent after the transfer of land. The number of cultivated varieties significantly promoted the probability of multiple cropping of farmland, which indicated that farmers tried not to leave land idle under the condition of considering the seasonal growth of different types of crops in order to maximize the use of farmland. Abnormal climate change significantly reduces the probability of multiple cropping of farmland. Extreme weather change will lead to agricultural yield reduction or even failure. Therefore, climate change will cause farmers to reduce agricultural input by reducing the multiple cropping of farmland.
To verify the effectiveness of instrumental variables, family farming time and village average multiple cropping index were considered in the model as instrumental variables for regression. The estimation results show that family farming time and village average multiple cropping index have a significant impact on the decision of multiple cropping, but have an insignificant impact on the relative poverty of farmers. Since the number of instrumental variables is greater than the number of endogenous variables, the instrumental variables need to be tested for over-identification and weak instrumental variables. The F-test statistic value of the instrumental variables in the first stage of the model is 25.22, greater than the empirical rule of the F-test statistic value of 10. This indicates that the model does not have weak instrumental variables. The P-value of the over-identification test result is 0.000, indicating that there is no over-identification problem in the model. Family farming time can significantly increase the probability of multiple cropping of farmland. This is because families allocate more time to agricultural production, which will increase land utilization. The average multiple cropping index in the village can significantly increase the probability of multiple cropping of farmland, indicating that there is a “same group effect” in a rural society.
The Endogenous Switching Regression estimation results of different types of farmland multiple cropping on the relative poverty of farmers
Column (1)–(3) of Table 3 shows the impact of multiple cropping decisions on farmers’ relative poverty in dryland, and Column (4)–(6) estimates the results of instrumental variables in the full sample. From the dryland samples, the LR and Wald values indicate that the model has a good fitting effect. ρμ1 and ρμ2 indicate the existence of a self-selection problem. Column (4) and (5) of Table 2 are the estimated results of farmers’ relative poverty to multiple cropping in paddy fields and non-multiple cropping in paddy fields. Column (6) shows the regression results of the selection and decision model of multiple cropping in paddy fields. The per capita labor time will significantly reduce the probability of multiple cropping of paddy fields, which may be because household labor time occupies agricultural labor time to some extent, and households with more labor will not improve the land use degree too much. The per capita area of a household will significantly increase the probability of multiple cropping of paddy field, while the transferred area of a household will significantly reduce the probability of multiple cropping of paddy fields. The irrigation ratio of farmland will significantly reduce the probability of multiple cropping in paddy fields, possibly because good irrigation facilities can guarantee agricultural production efficiency, effectively improve farmers’ enthusiasm for farming, and improve the degree of farmland use. The instrumental variable of family farming time was not significant, but the instrumental variable of the average village multiple cropping index was significant at the 1% level, indicating that the endogeneity problem should be solved. In the model, the F-test statistic of the instrumental variable in the first stage is 26.35, which is greater than the empirical rule of F-test statistic 10. This indicates that there is no weak instrumental variable in the model. The P-value of the over-identification test result is 0.000, indicating that there is no over-identification problem in the model.
The treatment effect of multiple cropping decision on farmers’ relative poverty
The treatment effect of a multiple cropping decision on the relative poverty of farmers is shown in Table 4. In general, the multiple cropping decision has a significant negative effect on the relative poverty of farmers. Among them, the ATT value shows that, under the counterfactual condition, the relative poverty of farmers who have actually adopted multiple cropping of farmland will increase by 0.1302 units or 28.43% when they do not adopt multiple cropping of farmland. According to the ATU value, the relative poverty of the farmers who did not adopt multiple cropping of farmland will decrease by 0.1308 units, or 29.57%, when they adopt multiple cropping of farmlands for agricultural production. This indicates that multiple cropping of farmland can effectively reduce the relative poverty of the farmers. Furthermore, the endogenous transformation regression model and counterfactual framework were used to analyze the treatment effect of multiple cropping of different types of farmland on households’ relative poverty. The average treatment effect of multiple cropping decision of all types of farmland had significant negative influence. Among them, the ATT value showed that if farmers with multiple cropping of paddy fields and dryland did not adopt multiple cropping of farmland, their relative poverty would increase by 0.1482 and 0.0331, respectively. This translates to increases of 30.4 and 7.6%, respectively. The ATU value showed that if the farmers who did not engage in multiply cropping in paddy fields and dryland adopted the double cropping in farmland, their relative poverty would decrease by 0.2193 and 0.0917, respectively, or by 30.4 and 7.6%, respectively.
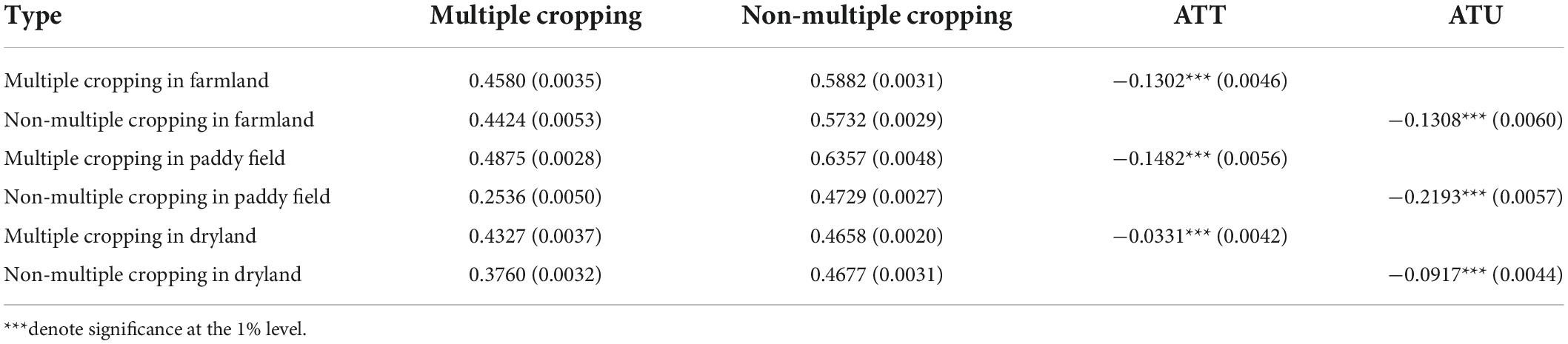
Table 4. The average treatment effect of multiple cropping of various types of farmland on relative poverty.
In conclusion, adopting multiple cropping in paddy fields can reduce the relative poverty of farmers better than adopting multiple cropping in dryland. The possible reasons are as follows: First, paddy fields mostly plant wheat, rice and other field crops. Field crops generally consume less labor and time than cash crops, thus saving labor and increasing family income. Second, because the main crop of paddy fields is grain, the grain income is low. Therefore, the index of multiple cropping in dryland was higher than that of paddy fields, and the space for the improvement of multiple cropping in paddy fields was larger. Third, paddy fields planted with food crops can get more agricultural subsidies, stimulating farmers to improve the degree of multiple cropping of paddy fields to a certain extent.
To test the robustness of the endogenous transformation regression model, Columns (5)–(7) of Table 3 presents the regression results of instrumental variables based on all farmland samples, paddy field samples and dryland samples. The comparison between the results of the instrumental variable estimation and those of the ESR model shows that the instrumental variable method overestimates the effect of the multiple cropping decision on the relative poverty of farmers. However, the results of the instrumental variable estimation are consistent with the results of the ESR model in significance and direction. To more vividly reflect the effect of the decision of multiple cropping of farmland on the reduction of households’ relative poverty, Figure 1 lists the probability density distribution map of households’ relative poverty under the scenarios of multiple cropping of farmland or not. Figure 1A shows that, in the counterfactual case, the probability density distribution curve of relative poverty shifts to the right if the households adopting multiple cropping of farmland do not adopt it, indicating that the sample selection bias is solved, the multiple cropping of farmland will significantly reduce the households’ relative poverty (ATT = −0.1302). As shown in Figure 1B, in the counterfactual case, if farmers who do not adopt multiple cropping of farmland adopted it, the probability density distribution curve of their relative poverty would shift to the left, indicating that the relative poverty of farmers without multiple cropping of farmland would be reduced after the adoption of multiple cropping (ATT = −0.1308). Due to the limitation of space, the probability density map of relative poverty of farmers’ multiple cropping in paddy field samples and dryland samples was not provided.
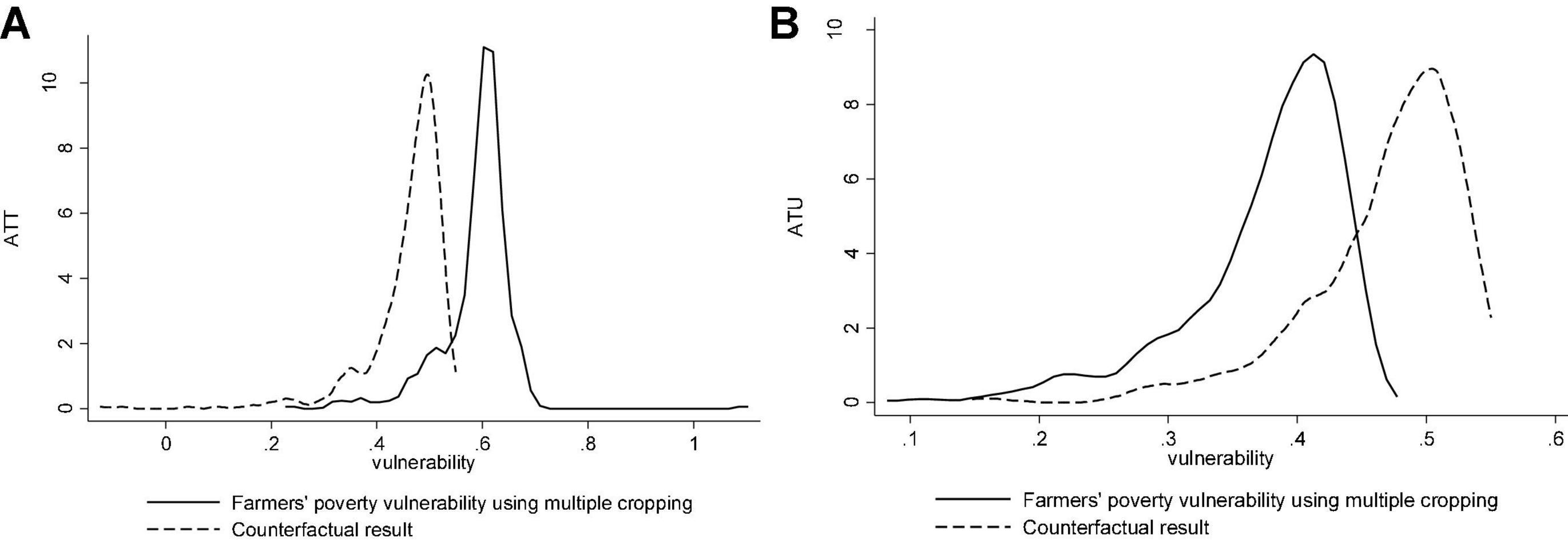
Figure 1. Relative poverty probability density of farmers with multiple crops (A) and no multiple crops (B).
Generalized propensity score matching estimation of the degree of multiple cropping on the relative poverty of farmers
The endogenous transformation regression model was used to evaluate the processing effect of multiple cropping on the relative poverty of farmers, but the processing variable of ESR model could not be a continuous variable. The GPSM model was used to evaluate the net benefits of the multiple cropping index on the relative poverty of farmers. First, the Fractional Logit model was used to estimate the generalized propensity score, and a balance test was conducted on the samples. The test results are shown in Table 5. All the covariables had no significant effect on relative poverty, indicating that the selection of covariables was reasonable. According to the balance test idea of Hirano and Imbens (2004), 0.2 and 0.6 were taken as critical points, and the samples were divided into three groups to test the difference of conditional mean values of matching variables in three subintervals after multiple cropping matching of different types of farmland. The mean deviation is not significant, indicating that there is no systematic difference between the matching variables. Thus, it meets the assumption of balance. Then, the conditional expectation of the relative poverty of multi-cropping households of different types of farmland was calculated using the second-order approximation formula. The results showed that the estimated coefficients of the multi-cropping index and its square of different types of farmland were significant at the 1% level, and the estimated coefficients of the propensity score variable and its square were significant at the 1% level. The interaction between multiple cropping index and propensity score variables is significant at the 5% level.
Table 6 shows the estimated results of the GPSM processing effect. According to Eq. 8, the expected value and marginal change of agricultural production costs of different types of land circulation areas at different treatment levels can be estimated. According to the estimated results, the treatment effects of multiple cropping index in all farmlands, paddy fields, and dryland experienced a change from positive to negative effects. However, not all positive effects passed the significance test, while all negative effects passed the significance test. The treatment effects of the three types of farmland all showed an increasing trend with the increase of multiple cropping index, but the marginal effects were different among plots. From the perspective of the relative poverty of farmers, when the multiple cropping index of different types of farmland was at the treatment level of 0∼0.03, increasing the multiple cropping index of farmland would reduce the relative poverty of farmers, but the effect was not obvious. When the treatment level exceeded 0.3, the treatment effect of multiple cropping index of different types of farmland improved more obviously. The main reason is that when the multiple cropping index of farmland is low, farmers do not make full use of land, which is inevitably not conducive to reducing the relative poverty. When the multiple cropping index of farmland exceeds a certain threshold, the effect of the multiple cropping index of farmland on reducing the relative poverty of farmers will continue to expand. From the perspective of multiple cropping index of different types of farmland, the marginal effect of multiple cropping in paddy fields at different treatment levels was significantly higher than that in dryland, and the increasing trend of the marginal effect of multiple cropping in paddy fields was more obvious. The possible reason is that under the same multiple cropping index tillage condition, multiple cropping of paddy field can increase family income more significantly, while significantly reducing the relative poverty of farmers.
According to the expected value and marginal effect, the treatment effect function of different types of farmland multiple cropping indices on the relative poverty of farmers can be obtained. Figures 2A–C represent the functional diagrams of the treatment effects of the total cropland MCI, the paddy field MCI, and the dryland MCI on the relative poverty of farmers, respectively. The solid line in Figure 2 represents the functional relationship between the multiple cropping index of different types of farmlands and the relative poverty of farmers. The two dotted lines outside the two represent the 95% upper and lower confidence limits of the GPS estimation function, respectively. Figure 2 clearly shows that the effect of the multi-cropping index of farmland in reducing the relative poverty of farmers does increase in scale. Also, the curve of the treatment effect of the multi-cropping index of paddy fields is significantly more curved, indicating that the treatment effect of multi-cropping in paddy fields is greater.
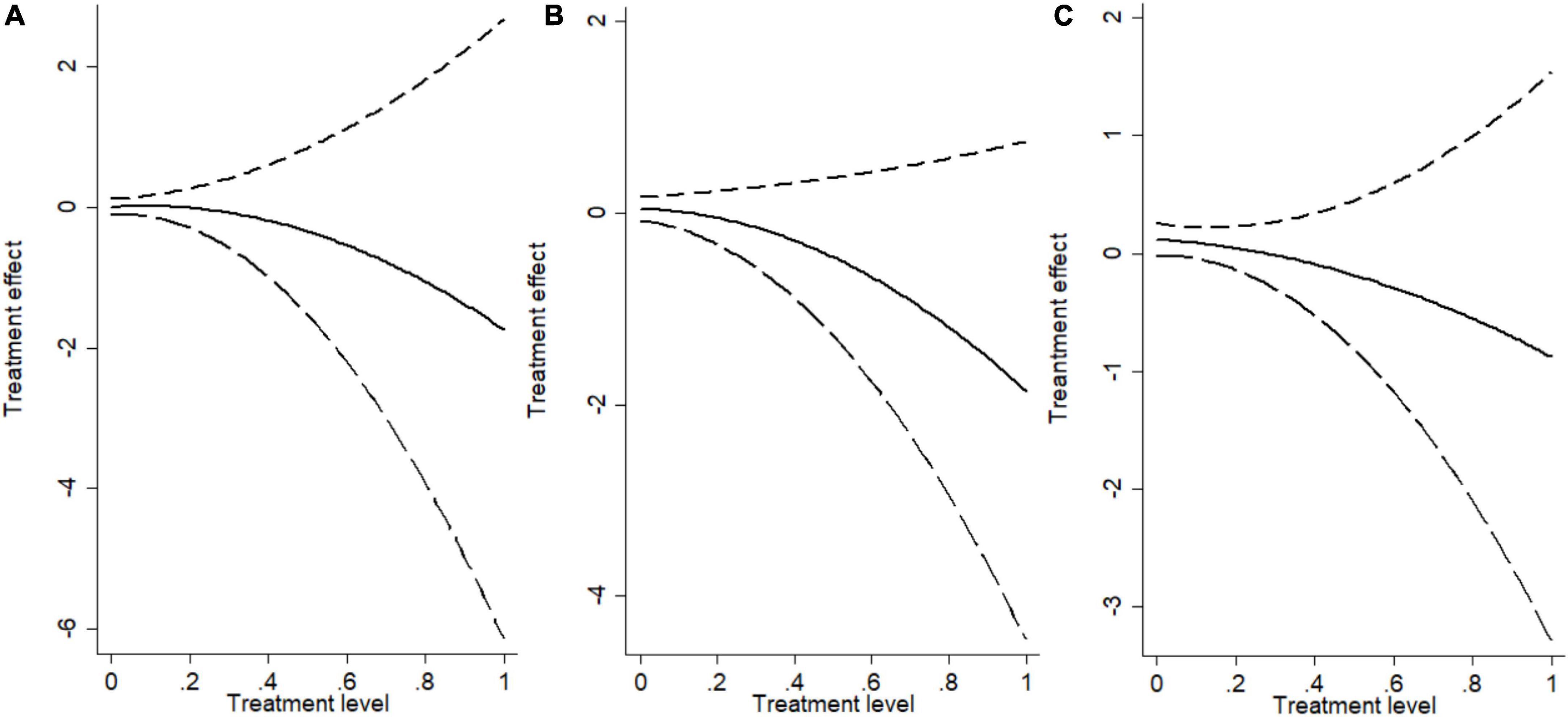
Figure 2. Schematic diagram of the treatment effect function of all farmland (A), paddy field (B), and dryland (C) multiple cropping index on the relative poverty.
The mechanism of multiple cropping to the relative poverty of farmers
The multiple cropping of farmlands may affect the relative poverty of farmers through two paths. One is by increasing agricultural planting income and reducing the relative poverty of farmers through the yield improvement path; the other is by improving factor utilization rate and reducing the agricultural planting cost and the relative poverty of farmers through the factor intensive path. The mediating effect method was used to test the above two action paths (Peng et al., 2019).
Test the mediating effect of yield increase
To quantify the yield increase, the average output per unit was used as a mediating variable. Column (1) in Table 7 shows that the multiple cropping of farmland has a significant impact on the relative poverty of farmers, with an estimated coefficient of −0.0305. Column (2) shows that the multiple cropping index of farmland can significantly increase agricultural output, which means that the multiple cropping of farmland increases agricultural income. The two variables in Column (3) have passed the significance test, indicating that the impact of the mediator variable on the relative poverty of farmers is still significant after controlling for the influence of multiple cropping of farmland. According to the significance of each variable parameter estimate, the mediating effect of average output per unit exists, but it is a partial mediating effect, and the proportion of the mediating effect in the total utility is 0.31. This means that about 31% of the impact of multiple cropping of farmland on the relative poverty of farmers in the sample area is realized through the mediating effect of the variable of average output per unit. In other words, the multiple cropping of farmland affects “farmers” income by changing the agricultural per unit output value, which to a certain extent verifies the authenticity of the existence of the path of yield improvement.
Test of mediating effect of factor intensive path
Column (5) shows that the multiple cropping of farmlands can significantly improve the agricultural factor utilization ratio, indicating that the factor intensification after participating in the multiple cropping of farmland can indeed help to improve the agricultural factor utilization ratio. Column (6) shows that after controlling for the effect of the multiple cropping variable, factory utilization rate, as a mediating variable, still has a significant inhibiting effect on the relative poverty of farmers. As the estimated value of correlation coefficient in Column (4) ∼ (6) all passed the significance test, it could be concluded that there was a mediating effect of factory utilization variables. However, it was still a partial mediating effect rather than a complete mediating effect, and the proportion of the mediating effect to the total effect was 0.19. Therefore, it can be concluded that about 19% of the impact of multiple cropping of farmland on the relative poverty of farmers is realized through the mediating role of factor use rate variable. This means that the multiple cropping of farmland improves the agricultural factor use rate and thus increases the income level of farmers, which to some extent confirms the authenticity of the existence of the factor intensive path.
Further study: Analysis without the equivalent scale adjustment factor
Furthermore, the relative poverty value and the difference of the effect of multiple cropping on poverty reduction of farmland were used without adjusting for household equivalent size. Table 8 shows the values of family size, per capita income, and relative poverty before and after adjusting for equivalent size. The last line in the table is the mean value of each variable. The table shows that the family size in the sample ranges from 1 to 10 people, with an average value of 4.1, which is reflective of the actual situation in China’s rural areas. The adjusted family size ranges from 1 to 4.81, with an average value of 2.43. Both the number of families and the mean value are significantly smaller than those before the adjustment. The pre-adjustment value of per capita income is significantly less than the adjusted per capita income, and the pre-adjustment value of relative poverty value is significantly greater than the adjusted relative poverty. If the household demographic structure is not considered and a direct calculation on the basis of family per capita income is done, there will be a serious interference to measure poverty, causing a “false poverty” problem.
Then, the relative poverty value calculated based on unadjusted household per capita income was used for regression analysis. Table 9 estimates the average treatment effect of multiple cropping of various types of farmland on relative poverty using the endogenous transformation model. The table shows that the multiple cropping behavior of all types of farmlands has a significant negative impact on the relative poverty of farmers, indicating that the regression model has a strong stability. Under the counterfactor condition, the relative poverty of farmers who have actually adopted the method of multiple cropping of farmland will increase by 0.1504 units, with an increase ratio of 31.7. The estimated results of ATU show that the relative poverty of farmers who did not adopt the multi-cropping method would be reduced by 0.1019 units, with a decrease ratio of 27.2%. The estimated results of ATT show that if farmers with multiple cropping in paddy fields and multiple cropping in dryland do not adopt multiple cropping in their farmlands, their relative poverty will increase by 0.1772 and 0.1129, or 35.3 and 25.3%, respectively. The estimated results of ATU show that if the farmers with non-multiple cropping in paddy fields and non-multiple cropping in dryland adopted the method of multiple cropping in farmland, their relative poverty would be reduced by 0.1626 and 0.2041, respectively, with a decrease ratio of 26.3 and 72.3%. In general, the ATT treatment effect of multiple cropping of all types of farmland is higher than the adjusted estimated value, indicating that the estimated result of unadjusted family per capita income will be higher.
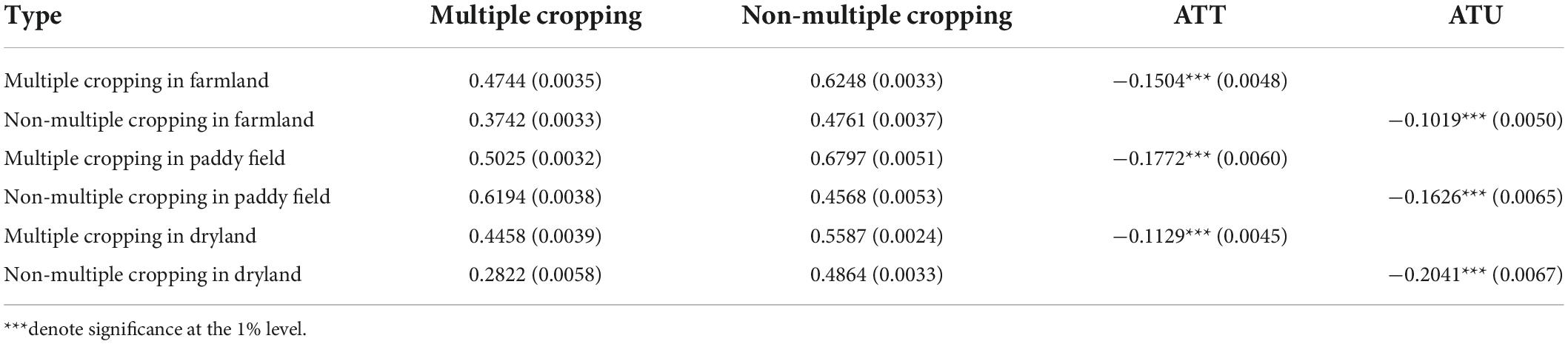
Table 9. The average treatment effect of multiple cropping of various types of farmland on relative poverty before the equivalent scale adjustment.
Table 10 shows the net effect of the farmland multiple cropping index on the relative poverty of farmers estimated by the GPSM method before adjusting the equivalent scale. The table shows that the negative treatment effect of the multiple cropping index of each type of farmland passed the significance test. The treatment effect of the three types of farmland showed an increasing trend with the increase of the multiple cropping index, but the treatment effect of the multiple cropping index of paddy fields was higher than that of dryland, indicating that the estimated results had a strong robustness. The per capita income before adjustment is significantly smaller than the adjusted per capita income, and the relative poverty before adjustment is significantly larger than the relative poverty after adjustment. It shows that if the family per capita income is directly calculated without dealing with the family population structure, it will seriously interfere with the poverty measurement, and even cause the problem of “false poverty.”
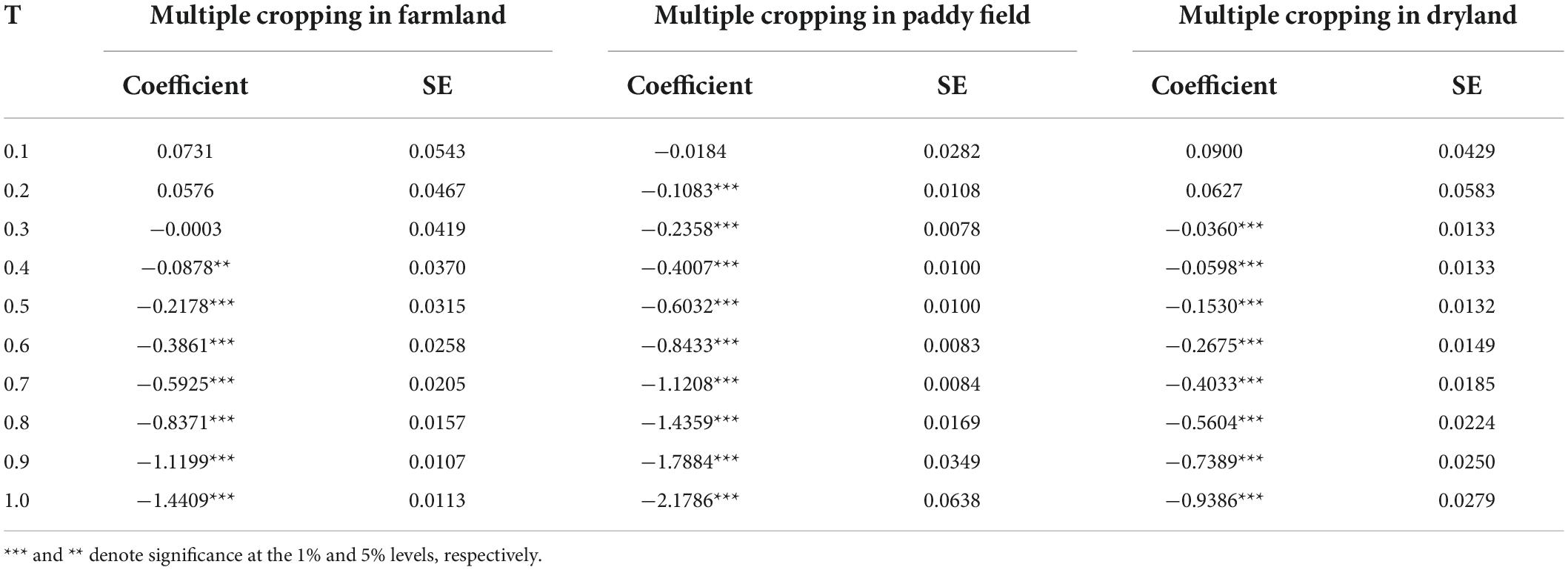
Table 10. Estimated results of generalized propensity score matching treatment effects before equivalence scale adjustment.
Conclusion and policy insight
Based on a field survey of 1,120 rural households in Hubei Province, this paper analyzed the impact of multiple cropping of farmlands on the relative poverty of farmers. It used the endogenous transformation regression model and GPSM model. The research conclusions are as follows: First, in general, the multiple cropping of farmlands can increase relative poverty, and this conclusion is confirmed by counterfactual test. Second, the poverty reduction effect of multiple cropping on different farmland types is different, and the poverty reduction effect of multiple cropping in paddy fields is higher than that of dryland. Third, all the treatment effects of the multiple cropping index of farmlands, paddy fields, and dryland changed from positive to negative, but not all the positive effects passed the significance test, while all the negative effects passed the significance test. The treatment effect of the three types of farmland showed an increasing trend with the increase of multiple cropping index, but the marginal effect was different with the farmland type, and the poverty reduction effect of multiple cropping in paddy fields was greater. Fourth, the per capita working time, the proportion of household training, the proportion of households participating in insurance, and abnormal climate changes have a significant inhibitory effect on the multi-cropping of farmlands. The per capita area of households, the transferred area of households, the proportion of cultivated land irrigated, and the number of planted varieties play significant roles in promoting the multi-cropping of farmers’ farmland. The treatment effects of the three types of farmland showed an increasing trend with the increase of the multiple cropping index, but there were differences in the marginal effects of the plot types, and the poverty reduction effect of the multiple cropping of paddy fields was greater. Fifth, multi-cropping of cultivated land not only directly affects the relative poverty of farmers, but also affects the relative poverty of farmers through the partial mediation effect of the yield improvement path and the factor intensification path. The mediating effects of these two paths account for 31 and 19% of the total utility, respectively. Sixth, it is necessary to use the equivalent scale adjustment method to scientifically measure the welfare level of farmers, so as to reduce the impact of family structure on the measurement of farmers’ welfare level. Moreover, when the government formulates support policies, it needs to consider both the population size and family structure of farmers.
The following insight can be drawn from this research: First, while increasing farmers’ income through improving agricultural productivity, multiple cropping of farmland can also reduce the relative poverty of farmers. Therefore, it is of great practical significance to further promote the pattern of multiple cropping of farmland to improve the relative poverty of farmers. Second, there are significant differences in the poverty reduction effects of multiple cropping of different types of farmland, which helps to clarify the mechanism of multiple cropping of farmland on the improvement of farmland use efficiency and promote the rational choice of multiple cropping mode of farmland. Third, the government should correctly guide different types of farmers to rationally choose the corresponding pattern of multiple cropping of farmland considering the group differences in poverty reduction under multiple cropping of farmland. The government should also give preferential policies to farmers with inferior endowments. Fourth, there is a need to strengthen the construction of farmland-related supporting infrastructure and the corresponding technical support for the multiple cropping of farmland. This will help create conditions that further improve the multiple cropping of farmers’ farmland.
However, this paper has certain limitations. This paper focuses on the impact of multi-cropping paddy fields and dryland on the relative poverty of farmers, but does not analyze the poverty reduction effect of multiple cropping of farmland in different terrains. Topography is an objective factor affecting multiple cropping. Although the control variables have been used in this paper, it is still important to study the subdivision of cultivated land topography. In addition, although the process of using statistical data to calculate the multiple cropping index is simple, the calculation results have problems such as information lag, inability to reflect the spatial heterogeneity within the statistical unit, and low precision. Remote sensing technology has been used to monitor cropland MCI due to its advantages of large scale, high timeliness, and low cost, but it is generally used to measure cropland MCI at the regional level. Due to the limited measurement methods and the research object of farmers, this paper mainly uses statistical data to calculate the multiple cropping index. In the future, remote sensing technology can be used to more accurately measure the farmland multiple cropping index at the farmer level. In addition, due to the limited data, this paper only uses the data from the farmer survey in Hubei Province to analyze the poverty reduction effect of multiple cropping on farmland. China’s land area is relatively large, and the effect of multiple cropping of farmland in different regions should be different. Therefore, the findings of this paper need to be further tested with more data from other provinces or other countries.
Data availability statement
The datasets presented in this study can be found in online repositories. The names of the repository/repositories and accession number(s) can be found in the article/supplementary material.
Author contributions
JP conceived of the idea for this study. LC and BY conducted the statistical analysis. XZ and ZH contributed to the final write-up. All authors contributed to the article and approved the submitted version.
Funding
This research was funded by the National Natural Science Foundation of China (No. 72063012), MOE (Ministry of Education in China) Humanities and Social Sciences Youth Foundation (No. 20YJC790103), General Project of Science and Technology Research of Jiangxi Provincial Department of Education (No. GJJ210526), and Jiangxi Province Postdoctoral Research Routine Funding Project (No. 2020RC23).
Acknowledgments
The valuable comments and suggestions of editors and reviewers are gratefully acknowledged.
Conflict of interest
The authors declare that the research was conducted in the absence of any commercial or financial relationships that could be construed as a potential conflict of interest.
Publisher’s note
All claims expressed in this article are solely those of the authors and do not necessarily represent those of their affiliated organizations, or those of the publisher, the editors and the reviewers. Any product that may be evaluated in this article, or claim that may be made by its manufacturer, is not guaranteed or endorsed by the publisher.
References
Amin, T., Timothy, D., Meehan, J., Gary, R., Michael, F., and Claudio, G. (2016). SmartScape™: A web-based decision support system for assessing the tradeoffs among multiple ecosystem services under crop-change scenarios. Comput. Electron. Agric. 121, 108–121. doi: 10.1016/j.compag.2015.12.003
Aruna, S., James, C., and Michelle, J. (2020). The Samagra anti-poverty programme in Madhya Pradesh: Integrating household data, overcoming silo-problems and leaving nobody behind. Dev. Policy Rev. 39, 435–449. doi: 10.1111/dpr.12502
Asfaw, S., Lipper, L., Dalton, T., and Audi, P. (2012). Market participation, on-farm crop diversity and household welfare: Micro-evidence from Kenya. Environ. Dev. Econ. 17, 579–601. doi: 10.1017/S1355770X12000277
Augustine, A., Ayantunde, O., and Cofie, J. (2018). Multiple uses of small reservoirs in crop-livestock agro-ecosystems of Volta basin: Implications for livestock management. Agric. Water Manag. 204, 81–90. doi: 10.1016/j.agwat.2018.04.010
Battisti, R., Ferreira, M. D. P., Tavares, E. B., Knapp, F. M., Bender, F. D., Casaroli, D., et al. (2020). Rules for grown soybean-maize cropping system in Midwestern Brazil: Food production and economic profits. Agric. Syst. 182:102850. doi: 10.1016/j.agsy.2020.102850
Byela, T., Herbert, N., Razack, L., Boscow, O., and Coretha, K. (2021). Long-rains crops, short-rains crops, permanent crops and fruit crops: The ‘hidden’ multiple season-cropping system for adaptation to rain variability by smallholder farms. J. Environ. Manag. 278:111407. doi: 10.1016/j.jenvman.2020.111407
Cai, J., and Xia, X. (2019). Can Lan transfer ease or aggravate the multidimensional poverty of households? Res. Environ. Yangtze Basin 2, 2971–2979. doi: 10.11870/cjlyzyyhj201912017
Cai, J., Wang, T., Xia, X., Chen, Y., Lv, H., and Li, N. (2019). Analysis on the choice of livelihood strategy for peasant households renting out Farmland: Evidence from western poverty-stricken areas in China. Sustainability 11:1424. doi: 10.3390/su11051424
Chen, Y., Wu, J., Wang, H., Ma, J., Su, C., and Wang, K. (2019). Evaluating the soil quality of newly created farmland in hilly and gully areas on the Loess Plateau. China. J. Geogr. Sci. 29, 791–802. doi: 10.1007/s11442-019-1628-8
Erb, K., Haberl, H., Jepsen, M., Kuemmerle, T., Linder, M., Muller, D., et al. (2013). A conceptual framework for analysing and measuring land-use intensity. Cur. Opin. Environ. Sustain. 5, 464–470. doi: 10.1016/j.cosust.2013.07.010
Fan, J., and Wu, B. (2004). A study on cropping index potential based on GIS. J. Remote Sens. 8, 637–644. doi: 10.3321/j.issn:1007-4619.2004.06.014
Fan, Z., and Zou, W. (2021). From anti-poverty campaign to common prosperity: Dynamic identification of relative poverty and quantitative decomposition of poverty changes in China. China Ind. Ecol. 10, 59–77. doi: 10.19581/j.cnki.ciejournal.2021.10.010
Guo, W., Wu, T., Jiang, G., Pu, L., Zhang, J., Xu, F., et al. (2021). Spatial distribution, environmental risk and safe utilization zoning of soil heavy metals in Farmland, subtropical China. Land 10:569. doi: 10.3390/land10060569
Hirano, K., and Imbens, G. (2004). “The propensity score with continuous treatments,” in Applied bayesian modeling and causal inference from incomplete-data perspectives, eds A. Gelman and X. L. Meng (West Sussex: Wiley InterScience), 73–84
Huang, G., and Sun, D. (2017). Development situation and research progress of multiple cropping in China. Chin. Agric. Sci. Bull. 33, 35–43. doi: 10.3389/fpls.2017.01157
Li, L., and Li, M. (2012). Improving the multiple cropping index is an effective approach to ensure national food security in China. Manag. Exp. 3, 26–28. doi: 10.3969/j.issn.1003-1154.2012.03.009
Liu, H., Bi, R., and Wen, B. (2017). Determination of conflict between coal mining and water and soil resources utilization and land consolidation strategy of resource destruction in river basin. Trans. Chin. Soc. Agric. Eng. 33, 238–249. doi: 10.11975/j.issn.1002-6819.2017.14.033
Liu, X. (2002). Farming system and farming system regional planning in China. J. China Agric. Res. Reg. Plan. 23, 11–15. doi: 10.7621/cjarrp.1005-9121.20020568
Liu, X., Chen, F., and Wu, Y. (2015). Multiple cropping—the principal part of China’s agriculture. Crops 6, 1–9. doi: 10.16035/j.issn.1001-7283.2015.06.001
Liu, Y., Liao, H., Li, J., Li, T., Cai, J., and He, T. (2020). Measurement and mechanism analysis of relative poverty of farming households in the post-2020 period: A case study of Changshou District, Chongqing Municipality. Prog. Geogr. 39, 960–971. doi: 10.18306/dlkxjz.2020.06.007
Lokshin, M., and Sajaia, Z. (2004). Maximum likelihood estimation of endogenous switching regression models. Stata J. 4, 282–289. doi: 10.1177/1536867X0600600301
Michalscheck, M., Groot, J., Kotu, B., Hoeschle, I., Kuivanen, K., Descheemaeker, K., et al. (2018). Model results versus farmer realities. Operationalizing diversity within and among smallholder farm systems for a nuanced impact assessment of technology packages. Agric. Syst. 162, 164–178. doi: 10.1016/j.agsy.2018.01.028
Mihai, V. (2020). Poverty reduction through land transfers? The World Bank’s titling reforms and the making of “subsistence” agriculture. World Dev. 135:105058. doi: 10.1016/j.worlddev.2020.105058
Mthethwa, S., and Wale, E. (2020). Household vulnerability to food insecurity in rural South Africa: Evidence from a nationally representative survey data. Int. J. Environ. Res. Public Health 18:1917. doi: 10.3390/ijerph18041917
Mustafin, A., and Kantarbayeva, A. (2021). Resource competition and technological diversity. PLoS One 16:e0259875. doi: 10.1371/journal.pone.0259875
Parodi, G., and Sciulli, D. (2012). Disability and lowincome persistence in Italian households. Int. J. Manp. 33, 9–26. doi: 10.1108/01437721211212501
Peng, J., Wu, H., and Qin, X. (2019). Impact of land transfer to the vulnerability of poor farmers. China Life Sci. 33, 67–75. doi: 10.11994/zgtdkx.20190408.084615
Peng, K., and Zhang, A. (2015). Farmers’ vulnerability in farmland conversion: Analytical framework and empirical research on five provinces in central China. J. Nat. Res. 30, 1798–1810. doi: 10.11849/zrzyxb.2015.11.002
Rana, R., Garforth, C., and Sthapit, B. (2007). Influence of socio-economic and cultural factors in rice varietal diversity management on-farm in Nepal. Agric. Hum. Values 24, 461–472. doi: 10.1007/s10460-007-9082-0
Rogstad, S., and Pelikan, S. (2013). Plant species restoration: Effects of different founding patterns on sustaining future population size and genetic diversity. Sustainability 5, 1304–1316. doi: 10.3390/su5031304
Sheridan, H., Keogh, B., Anderson, A., Carnus, T., McMahon, B., Green, S., et al. (2017). Farmland habitat diversity in Ireland. Land Use Policy 63, 206–213. doi: 10.1016/j.landusepol.2017.01.031
Shi, C., Qu, L., Zhang, Q., and Li, X. (2021). A systematic review on comprehensive sloping farmland utilization based on a perspective of scientometrics analysis. Agric. Water Manag. 244:106564. doi: 10.1016/j.agwat.2020.106564
Shi, J., Liu, M., and Li, J. (1998). Multicropping and sustainable development in grain production in China. Agric. Res. Arid Areas 16, 51–57. doi: 10.3969/j.issn.1001-3601.2011.04.062
Strier, R. (2019). Resisting neoliberal social work fragmentation: The wall-to-wall alliance. Soc. Work 64, 339–345. doi: 10.1093/sw/swz036
Su, F., Fan, B., Huang, D., Kan, L., and Luo, W. (2021). Relative poverty management in post-poverty era: International reference and policy orientation. China Soft Sci. 12, 73–83. doi: 10.3969/j.issn.1002-9753.2021.12.008
Sun, Z., Zhao, L., Wang, S., Zhang, H., Wang, X., and Wan, Z. (2021). Targeted poverty alleviation and households’ livelihood strategy in a relation-based society: Evidence from Northeast China. Int. J. Environ. Res. Public Health 18:1747. doi: 10.3390/ijerph18041747
Tan, X., and Tan, Q. (2021). China’s anti-poverty strategy towards 2035. Issues Agric. Ecol. 12, 126–136. doi: 10.13246/j.cnki.iae.2021.12.008
Tang, F., and Cai, G. (2020). Temporal and spatial characteristics of land use transformation and it’s the evolution of landscape patterns in typical karst regions: A case study of Renhuai City. Sci. Technol. Eng. 20, 4860–4870. doi: 10.3969/j.issn.1671-1815.2020.12.037
Tang, H., Wu, W., Yang, P., Zhou, Q., and Chen, Z. (2010). Recent progresses in monitoring crop spatial patterns by using remote sensing technologies. Sci. Agric. Sin. 43, 2879–2888. doi: 10.3864/j.issn.0578-1752.2010.14.006
Tang, H., Wu, W., Yu, Q., Xia, T., Yang, P., and Li, Z. (2015). Key research priorities for agricultural land system studies. Sci. Agric. Sin. 48, 900–910. doi: 10.3864/j.issn.0578-1752.2015.05.08
Timo, S., and Anni, H. (2013). Opportunity costs of providing crop diversity in organic and conventional farming: Would targeted environmental policies make economic sense? Eur. Rev. Agric. Econ. 40, 441–462. doi: 10.1093/erae/jbs029
Wang, H., Yang, T., Chen, J., Bell, S., Wu, S., Jiang, Y., et al. (2022). Effects of free-air temperature increase on grain yield and greenhouse gas emissions in a double rice cropping system. Field Crops Res. 281:108489. doi: 10.1016/j.fcr.2022.108489
Wang, W., Feng, Y., Zhang, D., and Chen, S. (2019). The poverty-reducting and Income-increasing effects of rural land consolidation in different modes based on the PSM-DID method. China Life Sci. 33, 80–88. doi: 10.11994/zgtdkx.20191210.142657
Wang, W., Lan, Y., and Wang, X. (2021a). Impact of livelihood capital endowment on poverty alleviation of households under rural land consolidation. Land Use Policy 109:105608. doi: 10.1016/j.landusepol.2021.105608
Wang, W., Luo, X., Zhang, C., Song, J., and Xu, D. (2021b). Can land transfer alleviate the poverty of the elderly? Evidence from rural China. Int. J. Environ. Res. Public Health 18:11288. doi: 10.3390/ijerph182111288
Wu, Q., and Lin, H. (2018). Research on land circulation models for poverty reduction. Soc. Sci. Yunnan 4, 132–140. doi: 10.3969/j.issn.1002-6924.2014.07.026
Wu, W., Yang, P., Tang, H., Ongaro, L., and Shibasaki, R. (2007). Regional variability of effects of land use system on soil properties. Sci. Agric. Sin. 40, 1697–1702. doi: 10.1016/S1671-2927(07)60185-0
Wu, W., Yu, Q., Verburg, P., You, L., Yang, P., and Tang, H. (2014). How could agricultural land systems contribute to raise food production under global change? J. Integr. Agric. 13, 1432–1442. doi: 10.1016/S2095-3119(14)60819-4
Xiang, M., Yu, Q., and Wu, W. (2019). From multiple cropping index to multiple cropping frequency: Observing cropland use intensity at a finer scale. Ecol. Indic. 101, 892–903. doi: 10.1016/j.ecolind.2019.01.081
Xiang, M., Yu, Q., Li, Y., Shi, Z., and Wu, W. (2022). Increasing multiple cropping for land use intensification: The role of crop choice. Land Use Policy 112:105846. doi: 10.1016/j.landusepol.2021.105846
Xu, X., and Liu, L. (2014). CropSysChina. Acta Geogr. Sin. 69, 49–53. doi: 10.3974/geodb.2014.01.08.v1
Yan, H., Liu, J., and Cao, M. (2005). Remote sensing multiple cropping index variations in China during 1980-2000. Acta Geogr. Sin. 60, 559–566. doi: 10.3321/j.issn:0375-5444.2005.04.004
Yan, Z., Yang, Z., and Zhong, W. (2019). Research on the path and countermeasures of to promotion of poverty alleviation and development by differential land policy. Theory Month. 4, 104–110. doi: 10.14180/j.cnki.1004-0544.2019.04.015
Yang, X., Liu, Z., and Chen, F. (2010). The possible effects of global warming on cropping systems in China I. The possible effects of climate warming on northern limits of cropping systems and crop yields in China. Sci. Agric. Sin. 43, 329–336. doi: 10.3864/j.issn.0578-1752.2010.02.013
Yin, Y., Hou, X., Liu, J., Zhou, X., and Zhang, D. (2022). Detection and attribution of changes in cultivated land use ecological efficiency: A case study on Yangtze River economic belt. China. Ecol. Indian 137:108753. doi: 10.1016/j.ecolind.2022.108753
Zeng, L., Li, X., and Ruiz, M. (2020). The effect of crop diversity on agricultural eco-efficiency in China: A blessing or a curse? J. Clean. Prod. 276:124243. doi: 10.1016/j.jclepro.2020.124243
Zhang, C., He, H., and Mokhtar, A. (2019). The impact of climate change and human activity on spatiotemporal patterns of multiple cropping index in south west China. Sustainability 11:5308. doi: 10.3390/su11195308
Zhang, D., Wang, W., Zhou, W., Zhang, X., and Zuo, J. (2020). The effect on poverty alleviation and income increase of rural land consolidation in different models: A China study. Land Use Policy 99:104989. doi: 10.1016/j.landusepol.2020.104989
Zhang, H. (2000). The problems of concerning the response of China’s cropping systems to global climatic changes: The effects of climatic changes on cropping system in China. Agric. Meteorol. 21, 9–13. doi: 10.3969/j.issn.1000-6362.2000.02.003
Zhang, J., and Sun, Z. (2018). Poverty reduction and realizating ways of Peasantry’s Land contractual management rights: Analyzing the tension of “three transformations” in P City, G Province. J. Nanjing Agric. Univ. 18, 91–102.
Zhang, M., and Meng, M. (2021). Alleviation effect of agricultural land conversion on multi-dimensional poverty of targeted households: Based on propensity score matching. Res. Soil. Water Conserv. 28, 384–389. doi: 10.13869/j.cnki.rswc.2021.04.041
Zhang, N., Kou, X., and Liu, R. (2021). Rural poverty reduction effect and efficiency of fiscal instruments: An analysis based on three relative poverty lines. China Rur. Ecol. 1, 49–71.
Zhang, Y., Wang, W., and Feng, Y. (2022). Impact of different models of rural land consolidation on rural household poverty vulnerability. Land Use Policy 99:104989. doi: 10.1016/j.landusepol.2021.105963
Keywords: multiple cropping, relative poverty, poverty vulnerability, endogenous transformation regression model, generalized propensity score matching, household equivalent size adjustment, welfare level
Citation: Peng J, Chen L, Yu B, Zhang X and Huo Z (2022) Effects of multiple cropping of farmland on the welfare level of farmers: Based on the perspective of poverty vulnerability. Front. Ecol. Evol. 10:988757. doi: 10.3389/fevo.2022.988757
Received: 11 August 2022; Accepted: 06 September 2022;
Published: 29 September 2022.
Edited by:
Dingde Xu, Sichuan Agricultural University, ChinaReviewed by:
Tian Yun, Zhongnan University of Economics and Law, ChinaChen Fuqiao, Chinese Academy of Agricultural Sciences (CAAS), China
Copyright © 2022 Peng, Chen, Yu, Zhang and Huo. This is an open-access article distributed under the terms of the Creative Commons Attribution License (CC BY). The use, distribution or reproduction in other forums is permitted, provided the original author(s) and the copyright owner(s) are credited and that the original publication in this journal is cited, in accordance with accepted academic practice. No use, distribution or reproduction is permitted which does not comply with these terms.
*Correspondence: Bingwen Yu, eXViaW5nd2VuQGp4dWZlLmVkdS5jbg==; Zenghui Huo, aHVvemVuZ2h1aUBjamx1LmVkdS5jbg==