- Modelling Evidence and Policy Group, School of Natural and Environmental Sciences, Newcastle University, Newcastle upon Tyne, United Kingdom
Established widespread populations of invasive vertebrates can be challenging to eradicate and for small to medium-sized vertebrates, such as squirrels, is often not deemed feasible. However, long-term trapping campaigns are necessary to limit spread or reduce impacts. The scale of trapping programmes is often limited by budgets that are not commensurate to the scale of the problem. On-going costs and fundraising are key factors used by decision makers to assess the feasibility of complete removal or long-term management options of established populations. Predicting the time and effort required to successfully manage Invasive Alien Species (IAS) remains a challenge, particularly where different strategies need to be employed across different spatial scales and habitat types, and in response to changing population densities. Statistical methods such as removal models can be applied to quantify population abundance during management operations and could inform planning of resource requirements. However, in practice, the lack of awareness of data requirements results in unsuited or non-standardized data collection. We present data from an invasive grey squirrel (Sciurus carolinensis) management project in the United Kingdom as a case study to illustrate common issues that impact on quantifying project outcomes. We focus on human and operational aspects of implementation and suggest ways of prioritizing analytical requirements. Insights consider conservation targets, data collection and operational design and may be of relevance to stakeholders, analysts, field operators and managers.
1. Introduction
Conservation of threatened species includes their protection from threats. One of the major threats is Invasive Alien Species (IAS), and successful IAS management has prevented species extinctions (Bolam et al., 2020). Many vertebrate IAS eradications have been documented, often removing small rodents from small, uninhabited islands using poisons (Robertson et al., 2017). Poison bait strategies are relatively well-established with good guidance on feasibility and spatiotemporal deployment of campaigns (Broome et al., 2014; Keitt et al., 2015) but are not suitable where non-target species are of concern. Instead, live capture trapping followed by humane dispatch is the main removal method for medium-sized invasive mammals but can be labor-intensive and costly, as traps can be dispersed across large landscapes and require regular checking.
In the UK, the invasive grey squirrel Sciurus carolinensis is subject to live capture trapping campaigns as a conservation measure to protect the native red squirrel Sciurus vulgaris. Best practice guidance provides technical information for practitioners (e.g., trap types, trap setting, Gill et al., 2019; UK Forestry Standard Technical Note, 2019; Smith et al., 2022) and much of the activity is carried out unmeasured. While live capture trapping campaigns for invasive mammal eradications have been documented and summarized (Robertson et al., 2017; Mill et al., 2020), only in a few cases is sufficient detail reported to assess the effort and resource required for successful eradication (Parkes et al., 2014).
For coordination of long-term management (e.g., where the objective is to reduce impact through reduction in density or removal from a discrete area) guidance on cost-effective strategies of live capture campaigns and methods to demonstrate success are lacking. Below we highlight the need for models and metrics for long-term management, and then discuss the impact that data availability can have on assessing management impact and predicting management costs using the Red Squirrels United project as a case study. We suggest these elements are considered by project managers when designing trapping strategies.
1.1. Measuring success, need for models
Removal models are designed to estimate population abundance as individuals are extirpated through control operations: they are therefore well-suited for estimating IAS populations as they are managed (Davis et al., 2016). Model outcomes are instrumental to the evidence-based analysis of trends in the effectiveness of control operations; they can inform management locally and support guidance for effective control (McGeoch and Jetz, 2019).
In animal populations, local abundance is expected to vary seasonally and through years with naturally occurring processes of population dynamics (e.g., births and deaths) and movement. Additionally, when the population is invasive and controlled, removals may affect density-related processes (e.g., change in availability via change in spatial dispersion). By structuring removal data collected via a robust design approach (with repeated random sampling during a “closed” period and estimation of natural population dynamics during subsequent “open” periods) removal models may account for changes relating to population dynamics and temporary emigration (Kéry and Royle, 2015; Zhou et al., 2019). Remaining changes in removal data may then be attributed to the control operations.
Various model formulations exist that target specific challenges but removal models remain complex with ongoing developments (Udell et al., 2022) and challenging data requirements. The estimation procedure will be compromised when operations are undertaken that do not allow detection of a decrease in population abundance. For instance, expending effort where individuals are rare may be counterintuitive to practitioners but will result in a lack of data preventing quantification of the trapping effort required where population densities are low. Rotation of trapping activities between densely populated areas without observing a decrease in captures may appear to be successful (animals are constantly removed) but the efficiency cannot be quantified with a removal model. Trapping where capture rates are low could be conducted with the use of automated trap monitoring or smart traps that reduce the need for human resource (e.g., alerting operator when a trap door closes via mobile phone, Martin, 2022).
Lastly, removal data may also contain common sources of bias (e.g., detectability or observer bias, spatial clustering of effort, preferential sampling) that may lead to a biased estimation of population trends (Link W.A. et al., 2018; Link W.W. et al., 2018) and should be avoided when initially designing field operations in time and space. Subsequent monitoring is also encouraged (Ramsey et al., 2010).
1.2. Metrics of successful management
Catch per unit effort (CPUE) is a commonly used metric to standardize capture rates to facilitate comparison. Trapping campaigns routinely report CPUE as the number of animals removed per ‘trap-night’, a unit that has been used as a metric of management success for over 100 years (Grinnell, 1914). However, its interpretation remains ambiguous: 500 trap-nights may imply running 50 traps for 10 nights or 100 traps for 5 nights (or any combination). The aggregation does not allow assessment of the distinct contribution of trap number and duration as separate, yet interacting, factors affecting removal success. As a result, reported management protocols are unreproducible and uninstructive. This ambiguity also challenges reliable costing of control operations as materials and rangers’ time are budgeted separately.
1.3. Making management decisions to facilitate success
Managers with limited resources must choose where and when to use them. Trapping operations tend to be focused where the problem appears worst on the ground, rather than using a strategic approach to collect data to inform models to improve knowledge and understanding of the impact of management. Often the vast landscapes where IAS management is required cannot be covered adequately under the operational logistics (number of staff, travel time, working hours) of the available resources. Funder requirements can contribute to poor decision-making by simplistic operational targets of number of animals removed or tight timelines rather than objectives of density reduction or spread limitation. Any systematic biases in the operational management decisions will impact the data collected and the interpretation of the model outputs. Ultimately this hampers our ability to learn about effective management strategies and how these may change with population density.
With the available statistical methods and advances in understanding about data recording being recognized as important for conservation, it is of concern that modeling approaches are not routinely used by managers to demonstrate progress. We seek to improve awareness of how management decision-making impacts data quality and the ability to make reliable assessment of the impact of control operations. We discuss the impact of landscape dynamics, operational decisions and resource deployment and their interactions as barriers to being able to document success. We evidence each theme with data from a case study of grey squirrel control to illustrate the issues and present evidence of costed control strategies.
2. A case study of invasive grey squirrel control in the UK
The control of grey squirrels in the UK is not centrally coordinated but undertaken in multiple areas by local groups, charities and wildlife organizations with a shared interest in conservation of the red squirrel or reduction of impact of grey squirrels on woodlands (MacKenna, 2020). Local removals of a widespread and highly dispersive mammal such as the squirrel can be inefficient or ineffectual as remnant populations will disperse back into cleared areas. Long-term management is often needed but obtaining repeated funding to carry out activity in the same areas year on year is challenging (Mill et al., 2020).
The Red Squirrels United (RSU) programme was a 5-year collaboration funded by EU LIFE14 and National Lottery Heritage Fund, and the first in bringing together a partnership of academics, practitioners and volunteers across Northern Ireland, Northern England and Wales in areas of red squirrel conservation to assess management effectiveness and community awareness.
The RSU project was an opportunity to quantify and contrast the impact of the management strategies of invasive grey squirrel in distinct and well-defined contexts of invasion. The perceived density and distribution of grey and red squirrel populations were major drivers of conservation goals in each project area. In practice the management implemented also varied with resource availability and local habitat context (e.g., forestry plantation to urban gardens and parkland). Previous experience in grey squirrel management influenced protocols and monitoring, data recording and collection, and eventually data quality.
The intention to use removal models to assess management impact was ultimately prevented as data availability or quality was not sufficient to fulfill the model requirements. We outline obstacles the project faced in attempting to quantify conservation actions and make recommendations to improve data quality to support analysis of management impact.
2.1. Metrics and units of trapping effort
Trapping effort was compared across the different geographic contexts and landscapes; the smallest area trapped was approximately 420 km2 and composed of well-connected patches of self-contained woodlands, the largest area trapped was 4,000 km2 of forestry plantation. Grey squirrel densities were considered higher in fragmented woodlands than in forestry blocks, according to sightings, but this was not measured systematically.
Landscape features such as size of woodlands and the seasonal ecology of squirrels were expected to influence trapping effort (we recorded area covered, number of traps, duration) noting when natural food sources (such as tree seeds, Gill et al., 2019) were high, trapping is deemed less successful. Published advice for grey squirrel control is approximately one trap per hectare (Gill et al., 2019) but in practice we found this to be two traps per hectare on average (Figure 1). Where grey squirrel density was perceived to be high, more intensive trapping was conducted throughout the year trapping areas were large (annual average 7.89–11.61 ha), trap density was high at approximately. Three traps per hectare, sessions were long (>4 days) as well as pre-baiting (>2.5 days), potentially reflecting a pattern of working through the week and closing traps over the weekend. Where perceived densities were not as high, trapping was more seasonal with higher trap densities and shorter pre-baiting and control sessions (approximately 2 days) where controlled areas were smallest (4.37 traps ha−1 for an annual average area of 3.43–3.45 ha−1). These were generally in a patchy landscape of woodlands around urban and agricultural land.
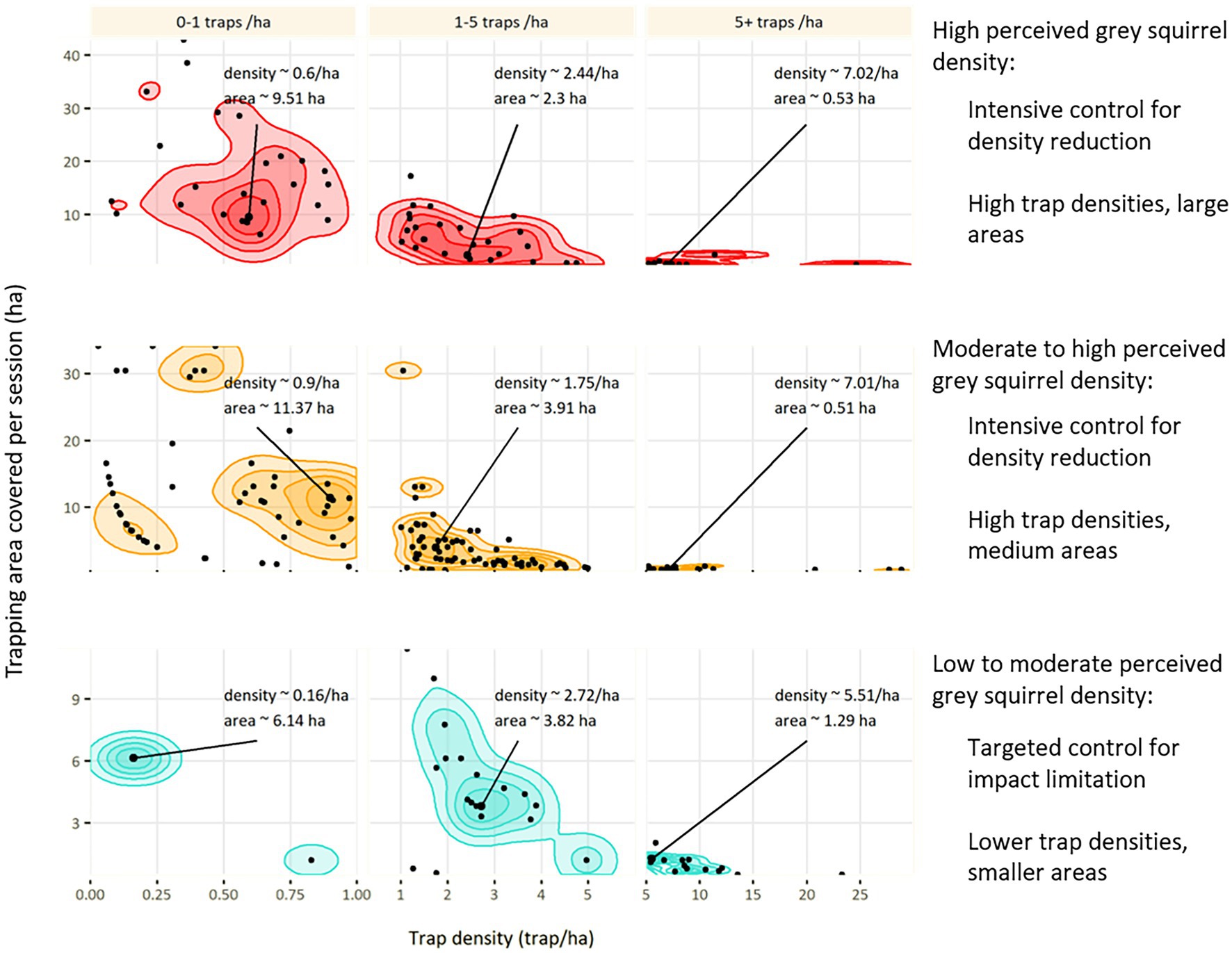
Figure 1. Observed relationship between trapping area and trapping density. The black dots represent the matched parameter values recorded by operators during each session throughout the duration of the project; surfaces of similar point density are illustrated by isopleths (average values are specified for the highest density surfaces). Trap density was partitioned into levels to assess associated trends. Each row represents a different project area associated with a distinct perceived stage of invasion and management goal. Note the varying limits of both the x and y axis.
Very low trapping densities over large areas can be where grey squirrels have been removed and the populations density is low or where the grey squirrel density is not known and a low density of traps are set out to monitor a large area efficiently. We found that larger areas had low trap densities at all perceived levels of grey squirrel density (Figure 1, left column), likely reflecting limitations or lack of resources, such as operator effort and time, rather than a decision in strategy.
Trapping densities should ideally be calculated as the number of traps set over an area defined using individual trap locations. However, the importance of geo-referencing in estimating control effort was not prioritized by all managers, so a precise computation of the area was not always possible. As illustrated on Figure 1, trapping densities could not be assumed to be constant. So, where absent, the trapped area was estimated on-site or post-hoc by the operator and used with the recorded number of traps set to derive the trap density.
Here we emphasize that determining how trap density will be recorded consistently to avoid having to make estimates should be an intrinsic part of efficient strategy planning. Metrics such as trap density or CPUE may be familiar metrics to managers however the relevance and adequacy of how these are determined and recorded should be reassessed against each project goals.
2.2. Operational design for good data: Considering model requirements
The design of a trapping strategy is affected by a number of both general and site-specific challenges that do not relate to the conservation goals or the squirrel ecology but will ultimately affect the analysis of the data collated and their analysis or interpretation. Management decisions reflect a balance between resources available, the landscape to cover and logistics. Manpower, often dictated by available budget, determines the number of traps deployed and the duration of each trapping session. RSU partners typically had one or two practitioners, increasing up to six working seasonally. Trapping was routinely halted for weekends and holidays, with varying trapping durations (e.g., between 2 and 24 h, four to 5 days weekly), although short trapping sessions were insufficient to observe a decline in capture rates thereby preventing adequate application of removal models.
The data recorded by RSU practitioners evidenced preferential trapping locations toward sites associated with ease of access or proximity to roads. Familiarity with the area may also be a factor for site selection, leading to selective revisiting of sites by practitioners rather than a randomized attribution of control site locations between practitioners. Observed variation in capture rates between trapping locations may reflect differences in approach or experience (e.g., trap placement, baiting techniques) or an inherent factor about the locations practitioners are working in, rather than the squirrel behavior and ecology as intended.
A lack of strategic direction will invite short-term management objectives, and result in practitioners visiting sites they consider more densely populated with IAS and therefore problematic, rather than prioritizing a spatial distribution of their effort that respects the underlining statistical constraints that condition a model applicability. Employing practitioners to conduct trapping also encourages their interest to lie closer to high capture rates rather than reaching overall conservation goals. Maintaining trapping effort where densities are or become low can seem counterintuitive, so it should be explicitly planned since regardless of the spread of data in time and space, if repeated sampling is routinely interrupted as the IAS density is reduced, our ability to make predictions about the remaining population will remain limited.
Effective and efficient data flows remain a challenge when implementing long-term invasive species management (Mill et al., 2020) but are needed to document and evidence management success. In the RSU case study, we routinely found that data entry work was undervalued with insufficient time dedicated and limited training. Desktop-based skills are also not necessarily an expectation of the role when rangers are recruited, so potential training needs for data entry should be evaluated and addressed.
Ideally datasets should be structured and adhere to well-defined standards in order to avoid common challenges that prevent data integration including sampling bias, bad practices preventing scaling and unbalanced data (Zipkin et al., 2021). While digital innovation can help resolve many of these issues, there is a danger that, without co-ordination, different projects invest and design their own individual data-collecting mechanisms, making data sharing and comparison at a broad scale more challenging or impossible (Wilson, 2018). Systems that allow integrating monitoring and sightings data are becoming more common and allow the implementation of citizen science approaches to provide data at a range of scales, and can be targeted to specific locations.
2.3. Impact on costing and future IAS management
Operational costs of trapping to remove invasive species will scale with the stage of invasion (and hence target species density) and management objectives (complete removal or spread/impact reduction). We modeled costs based on the observed trapping densities and capture rates to provide some guidance for future management planning (Table 1). We found the highest operational costs were where squirrel density was in greatest density and reduction was the management objective. Capture rates are high but the resource requirements are also high. RSU data showed that a single practitioner can operate up to 50 traps in a session and undertake the most trapping sessions per week for the highest capture rates, but this also varied seasonally. A more strategic, targeted trapping strategy was employed where the perceived IAS density was moderate, this approach required fewer traps and reduced costs. At its peak, trapping success rates can make this a cost-effective strategy however this is likely to be dependent on the landscape under control.
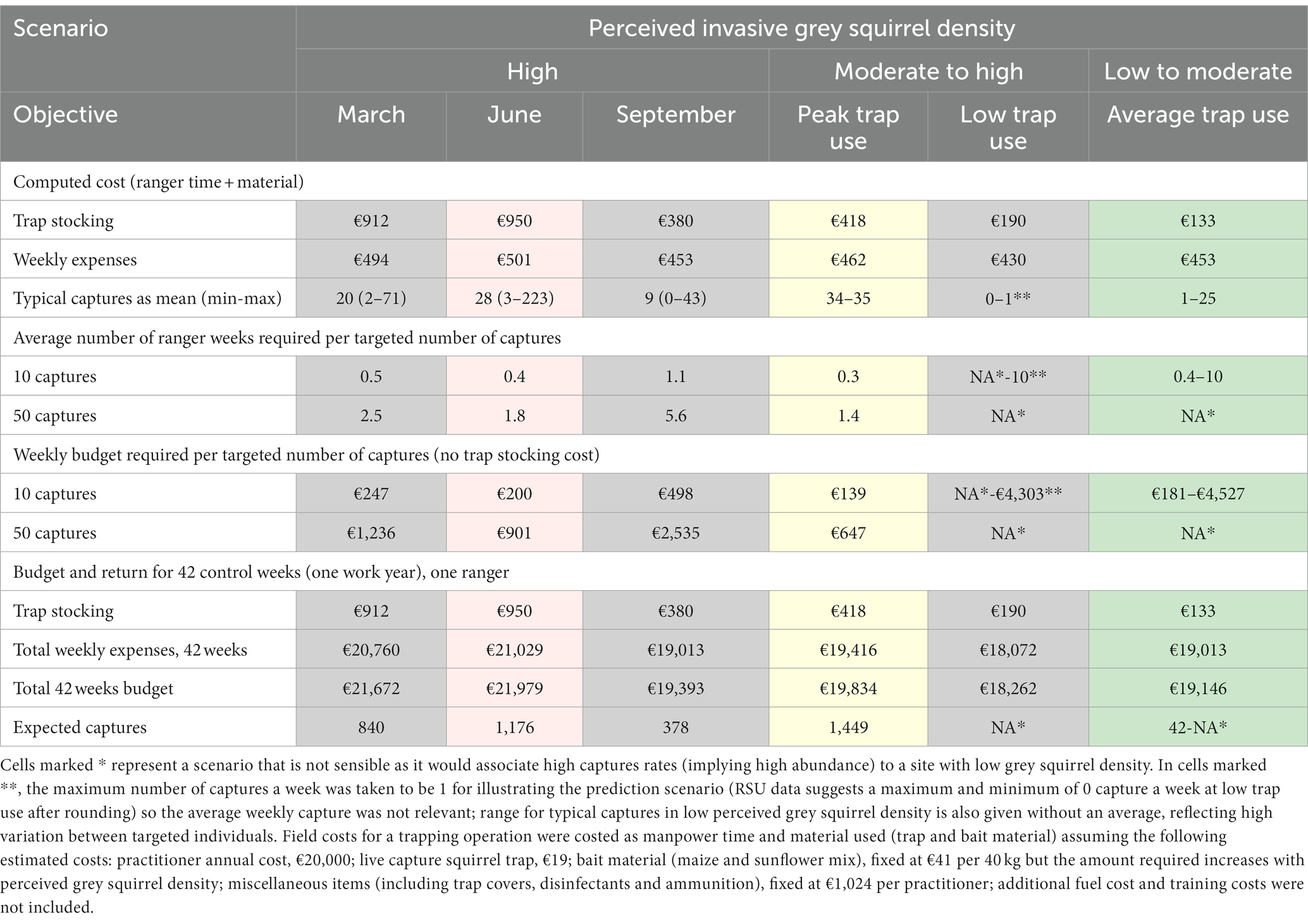
Table 1. Estimation of the time and cost required for a given number of grey squirrel captures under different combinations (high to low) of perceived IAS density and season.
The greatest uncertainty in operational costs occurs when the target species density is low. This can occur at the start of an invasion or at the latter stages of an eradication when the approach is targeted and focuses on single individuals. Operational costs are highly dependent on the time to detect and capture individuals. Average costs remain high and the number of captures can be very low. Remote monitoring, for example through camera traps, can be used to improve detection rates.
In the longer term, landscape connectivity strategies are key to maintaining areas free of grey squirrels. At that stage, costs associated with monitoring for IAS (re)invasion may be mitigated by focusing monitoring and/or trapping to key potential points the IAS is likely to use for (re)entry, identified by least cost pathways mapping.
3. Recommendations for management decisions
To allow management objectives and effectiveness of methods to be comparable between areas or across different projects, standardized metrics are needed. Assessing the effectiveness of a control strategy requires a measure of control effort that is clear about the context, scale and units of the intervention, even when similar operations were previously undertaken. For invasive mammal programs we recommend that removals are recorded alongside a measurement of control effort that documents space and time in a standardized way, such as daily applied trapping density and duration. Trapping strategies must aim to avoid bias such as clustering and preferential site visits, and plan for repeated site visits with robust design. Data recording must be enforced with clean entry, efficient storage, and dedicated time for operators including training if relevant. Only when these data are collected routinely will we be able to make evidenced based predictions about the cost effectiveness of control programs.
The collaboration of field practitioners and analysts across the RSU partnership led to a proposed framework for modeling the impact of control operations on the IAS population abundance (van der Waal and Mill, 2020). The framework allows managers to consider the competing impacts inherent with the landscape they are working in. They can make informed operational decisions, given their resource limitations, to maximize the data quality required for robust assessment of removal operations, and to optimize knowledge transfer and best practice in measuring management success.
Data availability statement
The original contributions presented in the study are included in the article/supplementary material, further inquiries can be directed to the corresponding author.
Ethics statement
Ethical review and approval was not required for the animal study because this work was analytical, we did not undertake field work.
Author contributions
All authors listed have made a substantial, direct, and intellectual contribution to the work and approved it for publication.
Funding
The Red Squirrels United project was co-financed by the European Union’s LIFE Programme (LIFE14 NAT/UK/000467) and the National Lottery Heritage Fund.
Acknowledgments
Red Squirrels United data considered in this perspective article were collated from Wildlife Trust for Lancashire, Manchester and North Merseyside, Red Squirrels Trust Wales, Northumberland Wildlife Trust, Ulster Wildlife and The Wildlife Trusts of South & West Wales.
Conflict of interest
The authors declare that the research was conducted in the absence of any commercial or financial relationships that could be construed as a potential conflict of interest.
The handing editor TA declared a past co-authorship with the author AM.
Publisher’s note
All claims expressed in this article are solely those of the authors and do not necessarily represent those of their affiliated organizations, or those of the publisher, the editors and the reviewers. Any product that may be evaluated in this article, or claim that may be made by its manufacturer, is not guaranteed or endorsed by the publisher.
References
Bolam, F. C., Mair, L., Angelico, M., Brooks, T. M., Burgman, M., Hermes, C., et al. (2020). How many bird and mammal extinctions has recent conservation action prevented? bioRxiv. doi: 10.1101/2020.02.11.943902
Broome, K, Cox, A., Golding, C., Cromarty, P., Bell, P., and McClelland, P. (2014). Rat eradication using aerial baiting: Current agreed best practice used in New Zealand (Version 3.0). New Zealand Department of Conservation Internal Document, Department of Conservation, Wellington, New Zealand (2014).
Davis, A. J., Hooten, M. B., Miller, R. S., Farnsworth, M. L., Lewis, J., Moxcey, M., et al. (2016). Inferring invasive species abundance using removal data from management actions. Ecol. Appl. 26, 2339–2346. doi: 10.1002/eap.1383
Gill, R., Ferryman, M., Shuttleworth, C., Lurz, P. W. W., Mill, A., Robertson, P., et al. (2019). Controlling grey squirrels in forests and woodlands in the UK. Forest research and forestry commission. UK forestry standard technical note.
Grinnell, J. (1914). A survey of the mammals of the lower Colorado Valley. University of California Publication Zoology 12, 1–92.
Keitt, B., Griffiths, R., Boudjelas, S., Broome, K., Cranwell, S., Millett, J., et al. (2015). Best practice guidelines for rat eradication on tropical islands. Biol. Conserv. 185, 17–26. doi: 10.1016/j.biocon.2014.10.014
Kéry, M., and Royle, J. A. (2015). Applied hierarchical modeling in ecology: Analysis of distribution, abundance and species richness in R and BUGS: Vol. 1: Prelude and static models. Elsevier Inc.
Link, W. W., Converse, S. J., Adams, A. A. Y., and Hostetter, N. J. (2018). Analysis of population change and movement using robust design removal data. J. Agric. Biol. Environ. Stat. 23, 463–477. doi: 10.1007/s13253-018-0335-8
Link, W. A., Schofield, M. R., Barker, R. J., and Sauer, J. R. (2018). On the robustness of N-mixture models. Ecology 99, 1547–1551. doi: 10.1002/ecy.2362
MacKenna, M. (2020). Red squirrel (Sciurus vulgaris) conservation: Conservation status, threats and efficacy of conservation measures. Queen’s University Belfast.
Martin, A. R. (2022). Reliability and effective use of electronic trap monitoring systems based on cellular networks. Biol. Invasions 24, 1247–1251. doi: 10.1007/s10530-021-02713-2
McGeoch, M., and Jetz, W. (2019). Measure and reduce the harm caused by biological invasions. One Earth 1, 171–174. doi: 10.1016/j.oneear.2019.10.003
Mill, A. C., Crowley, S. L., Lambin, X., McKinney, C., Maggs, G., Robertson, P., et al. (2020). The challenges of long-term invasive mammal management: lessons from the UK. Mammal Rev. 50, 136–146. doi: 10.1111/mam.12186
Parkes, J., Fisher, P., Robinson, S., and Aguirre-Munoz, A. (2014). Eradication of feral cats from large islands: an assessment of the effort required for success. N. Z. J. Ecol. 38, 307–314.
Ramsey, D. S. L., Parkes, J. P., Will, D., Hanson, C. C., and Campbell, K. J. (2010). Quantifying the success of feral cat eradication, San Nicolas Island, California. N. Z. J. Ecol. 35, 163–173.
Robertson, P. A., Adriaens, T., Lambin, X., Mill, A., Roy, S., Shuttleworth, C. M., et al. (2017). The large-scale removal of mammalian invasive alien species in northern Europe. Pest Manag. Sci. 73, 273–279. doi: 10.1002/ps.4224
Smith, K. G., Nunes, A. L., Aegerter, J., Baker, S. E., Di Silvestre, I., Ferreira, C. C., et al. (2022). A manual for the management of vertebrate invasive alien species of union concern, incorporating animal welfare. 1st Edn Technical report prepared for the European Commission within the framework of the contract no. 07.027746/2019/812504/SER/ENV.D.2.
Udell, B., Martin, J., Romagosa, C., Waddle, H., Johnson, F., et al. (2022). Open removal models with temporary emigration and population dynamics to inform invasive animal management. Ecol. Evol. 12:e9173. doi: 10.1002/ece3.9173
UK Forestry Standard Technical Note (2019). Controlling grey squirrels in forests and woodlands in the UK isbn:978-1-83915-004-3.
van der Waal, Z., and Mill, A.C. (2020). Red squirrels united - evolving IAS grey squirrel management techniques in the UK and Ireland (D3 – Ecosystem function restoration assessment: Outcomes of monitoring evaluations on conservation actions, final report). Available at: www.redsquirrelsunited.org.uk/wp-content/uploads/2020/04/NU-Final-Eco-Assessment.pdf
Wilson, S. (2018). Haunting and the knowing and showing of qualitative research. Sociol. Rev. 66, 1209–1225. doi: 10.1177/0038026118769843
Zhou, M., McCrea, R. S., Matechou, E., Cole, D. J., and Griffiths, R. A. (2019). Removal models accounting for temporary emigration. Biometrics 75, 24–35. doi: 10.1111/biom.12961
Keywords: eradication, Sciurus carolinensis, removal models, invasion stages, measuring success
Citation: van der Waal Z and Mill AC (2023) Evidencing success: Data requirements to model the impacts of long-term management of invasive squirrels. Front. Ecol. Evol. 11:1105091. doi: 10.3389/fevo.2023.1105091
Edited by:
Tim Adriaens, Research Institute for Nature and Forest (INBO), BelgiumReviewed by:
Bram D’Hondt, Research Institute for Nature and Forest (INBO), BelgiumLucas Wauters, University of Insubria, Italy
Copyright © 2023 van der Waal and Mill. This is an open-access article distributed under the terms of the Creative Commons Attribution License (CC BY). The use, distribution or reproduction in other forums is permitted, provided the original author(s) and the copyright owner(s) are credited and that the original publication in this journal is cited, in accordance with accepted academic practice. No use, distribution or reproduction is permitted which does not comply with these terms.
*Correspondence: Zelda van der Waal, emVsZGEudmFuZGVyd2FhbEBuZXdjYXN0bGUuYWMudWs=