- 1School of Earth Sciences and Engineering, Hohai University, Nanjing, China
- 2Department of Geography, School of Arts and Sciences, National University of Mongolia, Ulaanbaatar, Mongolia
- 3Baotou Normal College, Inner Mongolia University of Science & Technology, Baotou, China
- 4College of Geographical Science, Inner Mongolia Normal University, Hohhot, China
- 5Inner Mongolia Key Laboratory of Remote Sensing & Geography Information System, Inner Mongolia Normal University, Hohhot, China
Irrigated crops have experienced a significant global expansion. The biophysical response of climate change to irrigated crop expansion in different regions, particularly in terms of monitoring the influence mechanism of nighttime land surface temperature (LST) change, however, remains insufficiently explored. Taking the three northeastern provinces of China as our study area, we apply window analysis, partial correlation analysis, and geographical detector to quantitatively characterize the spatial and temporal distribution pattern of daytime and nighttime LST (diurnal LST) and biophysical parameters, and the main driving mechanism of diurnal LST change. The results showed that irrigated crop expansion led to asymmetric changes in daytime (−2.11 ± 0.2°C, 97.4%) and nighttime (0.64 ± 0.2°C, 79.9%) LST. ΔLSTDT had a negative correlation with ΔLE (63%), but a positive correlation with ΔSSR and ΔH (91% and 77%). This revealed that the cooling effect caused by the superposition of the output latent heat flux and the absorbed solar shortwave radiation was greater than its heating effect. ΔLSTNT and ΔLE had a positive connection across 69% of the region. ΔLSTNT demonstrated a negative correlation with ΔSSR and ΔH in 82% and 75% of the regions, respectively. At this time, the superposition of latent heat flux and heating potential term produces a greater heating effect. The explanatory power of the single factor (the mean of q<0.50) of biophysical parameters for diurnal LST variation was significantly smaller than that of the interaction factor (the mean of q>0.50, p<0.01). This study shows more detailed dynamic information of diurnal LST and biophysical parameters from 8day scale. The findings highlighted the critical role of asymmetric changes in the diurnal surface thermal environment caused by irrigated crop expansion in the global climate from a land surface hydrothermal energy balance perspective.
1 Introduction
By 2050, the world population is expected to reach 9.2 billion, increasing the food demand by 70–110% (Bajželj et al., 2014; van Dijk et al., 2021). To ensure an adequate food supply in the future, intensive irrigated agriculture has been developing rapidly. The Food and Agriculture Organization of the United Nations (FAO) reports that as of 2017, there are approximately 167 million hectares of irrigated crops globally, of which more than 90% are located in Northeast Asia (Delzeit et al., 2017; FAO, 2018). The expansion of irrigated crops fundamentally alters the biogeochemical and biophysical (albedo, sensible heat flux, and latent heat flux) processes between the atmosphere and the land surface. It indirectly alters the seasonal cycle of carbon–water–energy of the land–atmosphere interface and plays a critical role in regulating the regional and global climate (Feddema et al., 2005; Windisch et al., 2021). Among these changes, the influence of biophysical processes on climate is more prominent at regional and short-time series scales (Arora and Montenegro, 2011; Zhang et al., 2014a), and these persistent changes in regional climates will threaten future grain yield (Liang et al., 2018; Gaupp et al., 2020). Therefore, understanding the regional climate effects of irrigated crop expansion from a mechanism perspective will provide a scientific basis for sustainable regional agriculture and food development.
Currently, observation data and model simulation are used mainly to study the influence of irrigated crop expansion on climate (Zhao et al., 2012; Zhu et al., 2012). Observational data, the most reliable in situ measurement method, are used to quantify the climate’s response to irrigated crop expansion by comparing temperature differences between irrigated and rainfed crops or the differences before and after the conversion of rainfed crops to irrigated crops. However, the data essentially provide point information (Christy et al., 2006). Because of the complex land surface coverage conditions and significant terrain differences, point information cannot fully represent the large-scale situation of a region. In addition, the background information (e.g., the characteristics of the surrounding features) of irrigated crops of different sites is different. Therefore, it is difficult to distinguish temperature differences caused by a combination of irrigated crop expansion and other surrounding instabilities. Model research has compared the differences between the simulation results of different models (coupled or uncoupled) and the results of control experiments (Huang et al., 2009; Wei et al., 2016). Therefore, accurately simulating each sector of irrigation experiments is a crucial step toward achieving effective results. For example, the use of a climate-coupled model to simulate the response of temperature to global irrigation indicated that the intensity of irrigation cooling varied considerably regionally, which may have been caused by factors such as irrigation range and irrigation amount (Lobell et al., 2009). However, the quantitative description of the location, time, method, and number of irrigated crops remains uncertain. Therefore, we used the window-searching strategy proposed by previous studies to eliminate issues like inconsistent background information of climate observation data and uncertainties in the attribution of temperature changes caused by model simulations (Li et al., 2016). Within the same moving window, pairs can be used to calculate differences in land surface parameters between the study object and its surrounding land cover types. It is possible to ensure the consistency of climate background, environment, and topography of different land use and cover types within the window by setting up appropriately sized moving windows (Malyshev et al., 2015; Schultz et al., 2016; Winckler et al., 2017). This method, as a complementary method for investigating temperature change, is used to identify the effects of irrigated crop expansion on local land surface temperature (LST).
Previous studies have simulated the effect of irrigated crop expansion on contemporary climate using models and have concluded that the effect of irrigated crop expansion is negligible on a global average scale (Sacks et al., 2009). However, the effect of irrigated crop expansion has been significant on a regional scale, with varying results. In the humid tropics, for example, the expansion of irrigated crops has caused thermal effects both during the day and at night, whereas it is a source of cold during the day and a source of warmth during the night in arid and semi-arid areas, especially during the dry season (Adegoke et al., 2003; Yang et al., 2020). However, the relative magnitude of daytime cooling and nighttime warming varies by region(Zhu et al., 2011). In the North China Plain, the nighttime warming effect of irrigated crops is greater than the daytime cooling effect, which has increase the regional temperature (Chen and Jeong, 2018). Conversely, in Northeast China, the expansion of irrigated crops has led to more significant daytime cooling effect than the nighttime warming effect during the growing season, resulting in a cooler local region (Liu et al., 2018; Yu and Liu, 2019).
Current research focuses on the influence mechanism of LST change from two aspects: biogeochemical processes and biophysical parameters (Bonan et al., 1992). The former emits or absorbs greenhouse gases, such as carbon dioxide, methane, and nitrous oxide, directly affecting carbon emissions and sequestration in the atmosphere (Pongratz et al., 2010). Studies have revealed that the expansion of irrigated crops has increased greenhouse gas concentrations in the atmosphere, eventually leading to a rise in the average temperature during the growing season (Chen et al., 2013; Sun et al., 2017). The latter directly disturbs the surface energy and water balance by changing the surface albedo, evapotranspiration, roughness, and specific emissivity (Moon et al., 2020). The study of the response of biophysical parameters to irrigated crop expansion, however, remains limited, which is reflected mainly in the trade-off effect between the degree of influence of radiative and nonradiative processes during different growing seasons. It is generally accepted that albedo, latent heat flux, and sensible heat flux are the dominant factors of LST variation (Du et al., 2017; He et al., 2020). Daytime LST decreases during the early growing season (May–June), and this is mainly because the cooling effect of latent heat flux is greater than the warming effect of albedo. Daytime LST variation in July–September is influenced by the synergistic effect of albedo and latent heat flux (Liu et al., 2018; Liu et al., 2019b; Pan et al., 2020; Liu et al., 2022). Through temperature response models, it has been found that local nonradiative processes (i.e., evapotranspiration and sensible heat) dominate the daytime cooling of irrigated crops and that radiation processes (i.e., albedo) play a secondary role (Zhang et al., 2022). The contribution of nonradiative processes to the daytime LST variation had seasonal characteristics. However, previous studies have only made a qualitative comparison of the relative contributions of radiative and nonradiative mechanisms to LST changes and the seasonal response of LST changes to energy factors remains controversial (Xin et al., 2020). Meanwhile, these studies have ignored the influence of superposition of energy factors (e.g., latent heat flux, solar incident shortwave radiation) on LST. Furthermore, the influence mechanism of nighttime LST change is more complex (Chen et al., 2022; Lian et al., 2022), and it has not been thoroughly discussed in prior research. We assessed the potential influencing factors of nighttime LST increase in terms of thermal inertia generated by net surface energy during the day and atmospheric inverse radiation at night caused by latent heat flux in this study.
The three eastern provinces of China are located in the hinterland of Northeast Asia, characterized by flat topography and abundant water and soil resources. Since the economic reforms in 1978, a large area of wetlands has been reclaimed to ensure the supply of national food production and to meet the demands of local economic development (Dong et al., 2016). A combination of these factors provided a natural experimental site for studying the mechanisms of climate change caused by the expansion of irrigated crops. In this study, we used the window-searching strategy to calculate the differences in surface parameters between irrigated crop and non irrigated crop to remove the influence of climatic background and topographic factors on LST changes. Then, we portrayed the spatial–temporal responses of daytime and nighttime land surface temperature (diurnal LST) changes to surface radiant energy (e.g., incident radiant energy, outgoing radiant energy, and net effect) quantitatively and comprehensively, from the perspective of surface energy superposition. This information has enriched the research system on the mechanism of the climate impact of irrigated crop expansion. Specifically, we examined the following: (1) How do latent heat flux, solar shortwave radiation energy, and heating potential term lead to the decrease of average daytime LST during the growing season? (2) What are the main controlling factors of the increase in nighttime LST? Are they direct effects on the nighttime LST increase? (3) On the spatial scale, which of the interaction effects of single-energy factor (first order) and double-energy factor (second order) has a higher degree of explanation for LST changes?
2 Materials and methods
2.1 Study area
The study area is located in high-latitudes of Northeast Asia, which cover Heilongjiang, Jilin, and Liaoning provinces (38.7–53.5°N, 121.1–123.6°E) (Figures 1A, B), with a total area of about 7.9×105 km2. This region is at the junction of the cold temperate zone, mid-temperate zone, humid and semi-humid climate. This area is cold-dry in winter and warm-humid in summer. The annual average temperature is −3–10°C. The maximum temperature decreases each year, while the minimum temperature increases during the period 2000–2020 (Figure 1C). The annual precipitation ranges between 400 and 1100 mm, with the majority falling between July and September. Its vast plains, fertile soil and sufficient water-heat state provide excellent natural conditions for the growth of single-season rice. The expansion rate of rice area during 2015–2020 is as high as 1.76×103 km2/a (Figure 1D). The rapid development of intensive irrigated agriculture has made it an important commercial grain base in China.
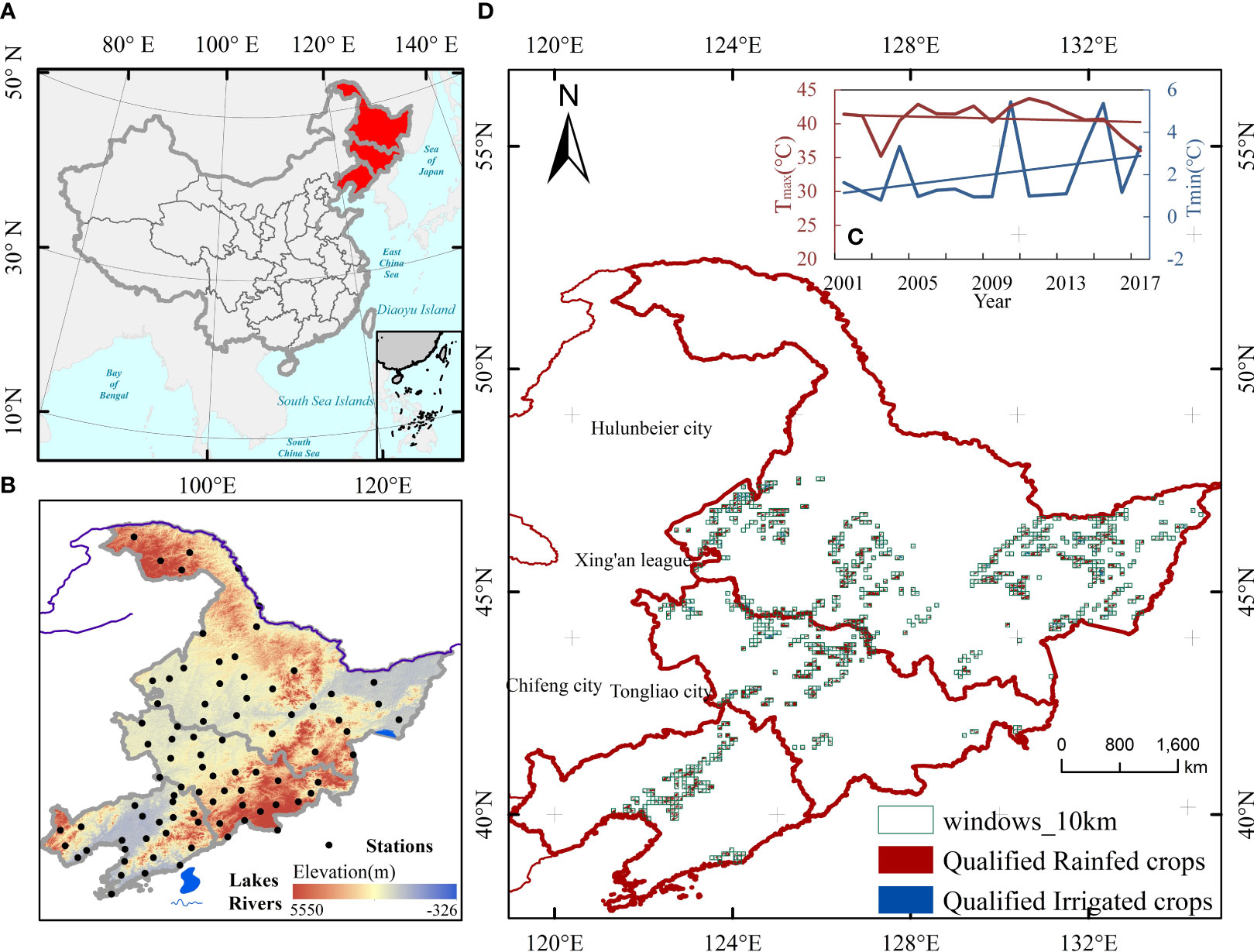
Figure 1 (A, B) Geographic location and elevation of Northeast China. (C) Climate situation of Northeast China since 2001. (D) Spatial distribution map of Irrigated crops expansion from 2015 to 2020.
2.2 Data and data processing
Land surface temperature (LST) data from the 8-day 1-km Terra/MODIS products (MOD11A2). As Terra/MODIS overpasses at around 10:30 and 22:30, which are close to the time of daily maximum and minimum air temperatures, so MOD11A2 LSTs at 10:30 and 22:30 were defined daytime LST (LSTDT) and nighttime LST (LSTNT), accordingly (Wan et al., 2015).
Latent heat flux (LE) is the flux of heat from the Earth’s surface to the atmosphere that is associated with evaporation of water at the surface, and is estimated by Terra/MODIS Evapotranspiration/Latent Heat Flux product (MOD16A2) that includes 500 m 8-day Evapotranspiration (ET), Latent Heat Flux (LE), Potential ET (PET) and Potential LE (PLE). MOD16A2 calculates global LE based on the Penman-Monteith (PM) equation, and its mean absolute bias is within 0.31–0.33 mm day−1 (Mu et al., 2011).
Albedo data are derived from 500 m daily albedo Model dataset (MCD43A3). The data include white-sky (directional hemispherical reflectance) and black-sky (dual hemispherical reflectance) albedos for seven MODIS individual bands and three broad bands (0.3–0.7 μm, 0.7–5.0 μm, and 0.3–5.0 μm) (Schaaf et al., 2002). As black-sky and white-sky albedo are highly correlated and have small difference, this study simply chooses white-sky albedo for 0.3–5.0 μm to indicate the total energy reflected by the earth surface.
Downward Shortwave Radiation (DSR) from Global Land Surface Satellite (GLASS), which is the world’s first high spatial-temporal resolution radiation product (daily, 0.05°). It mainly uses multiple polar orbits and geostationary satellite data to establish cloudy and cloudless radiative transfer models based on the look-up table algorithm. Using MODIS band data and cloud products, the inversion results of two MODIS observation sensors are combined. The measured coefficient is good 0.83 and the root mean square error is 115.0 W m−2 (Zhang et al., 2014b).
The land use/cover data from 2015 to 2020 are derived from the China Cropping Pattern Map. This data is based on GlobeLand30 global cultivated land data, which use mapping algorithm of phenological and threshold of pixel purity, the first planting map of three major crops (rice, maize and wheat) in China based on 500 m MODIS was obtained. The overall classification accuracy was 89%, the kappa coefficient was 0.85, the rice producer accuracy was 93%, and the dryland was 83%–90% (Qiu et al., 2022). We mainly selected irrigated and rainfed crops by the following criteria, as described in the literature (Abera et al., 2019). Assuming six layers for 2015–2020, the dominant land cover type was assigned to each pixel through an automatic selection method, i.e., the ones in the six 500 m pixels should all belong to the same category, or else removed from our analysis. Ultimately, pixels with rice in all six layers for 2015–2020 were defined as irrigated crops, and pixels with corn in a single pixel in all six layers, wheat, or a pixel with both corn and wheat were defined as rainfed crops. Eventually, all products’ temporal and spatial resolutions were unified to 1 km and 8-day scales, respectively. Additionally, the projection was unified to match the original projection of MODIS data to facilitate the calculation between products. See Table 1 for details of data products.
2.3 Methodology
This paper is mostly based on data from multiple remote sensing sources. Window-searching strategy is used to find irrigated crops and rainfed crops that have the same climate setting. On the basis of this, the spatial and temporal characteristics of diurnal land surface temperature and land surface biophysical parameters of rainfed crops and irrigated crops are examined. Lastly, by combining the pearson partial correlation analysis and the geo-detector model, the main biophysical mechanisms that cause the diurnal land surface temperature to change were studied on both the spatial and temporal scales. The exact process is shown in Figure 2.
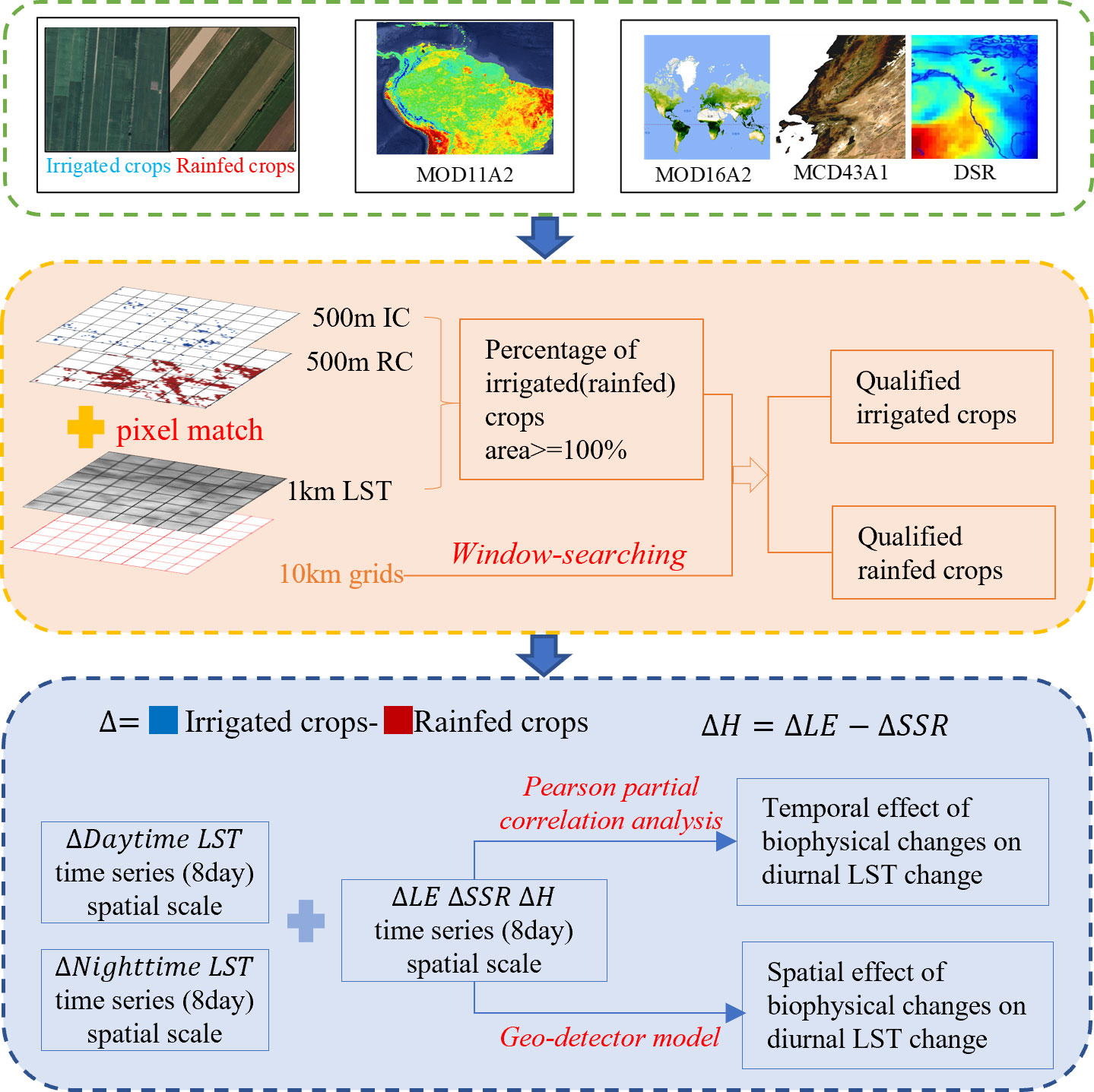
Figure 2 Flowchart of this study. IC, irrigated crops; RC, rainfed crops; LE, latent heat flux; SSR, solar shortwave radiation; H, heating potential term.
2.3.1 Quantization impacts of irrigated crop expansion on land surface parameters
Land surface temperature: We assessed the biophysical impact on LST changes through hypothetical irrigated crop expansion, which does not happen in reality. This methodology was developed by evaluating the temperature change induced by afforestation and deforestation (Li et al., 2015). The potential impact of irrigated crops on LST was expressed as the LST difference between irrigated crops and nearby rainfed crops in 2015–2020:
where represents daytime (DT) or nighttime (NT); represents or ; and represent average daytime and nighttime LSTs in 2015–2020 for irrigated crops and rainfed crops, respectively (8 days in growing season); and represent monthly average (mean of from May to October) daytime and nighttime LST in 2015–2020, respectively, and and represent the diurnal LST difference in irrigated crops and the diurnal LST difference in rainfed crops, respectively. Negative () indicated the cooling effect of irrigated crops; otherwise, it indicated a warming effect. A negative suggested that the diurnal LST difference was smaller in the irrigated crops compared to non-irrigated crops, and vice versa.
Intrinsic biophysical mechanics: We calculated the biophysical differences in solar shortwave radiation (SSR), latent heat flux (LE), and heating potential term (H) between irrigated crops and nearby non-irrigated crops in the same way as the previous calculation. To directly compare the relative effects of surface radiant energy changes on , which were caused by the difference between albedo and latent heat flux, we introduced the downward shortwave radiation (DSR). The solar shortwave radiation energy SSR (SSR = DSR (1-Albedo)), which was absorbed by the land surface, was calculated by DSR and albedo (Schultz et al., 2017). We refer to (Li et al., 2015) for the definition of a heating potential term as the difference in absorbed solar shortwave radiation and latent heat fluxes between irrigated crops and nearby non-irrigated crops in 2015–2020 is given by
where and represent the difference in solar shortwave radiation energy and latent heat flux on the 8-day scale for irrigated crops and rainfed crops in 2015–2020, respectively; and represent the monthly average (mean of and from May to October) solar shortwave radiation energy and latent heat flux for irrigated crops and rainfed crops in 2015–2020, respectively. Negative () indicated that the heating potential term absorbed and released by the irrigated crops was greater than that of the surrounding non-irrigated crops, and vice versa.
2.3.2 Window-searching strategy
Because the 1-km spatial resolution of MODIS products is relatively coarse, it might cause “mixed pixels” and add uncertainties of land surface parameters. To extract relatively pure MODIS pixels, we aggregated the 500-m land use and cover data with the 1-km MODIS LST resolution by calculating the area percentage of irrigated crops and rainfed crops within 1 km × 1 km grids and chose “pure” grids with an area percentage of 100% for irrigated crops (46,163 grids) and rainfed crops (34,797 grids).
We used the “window-searching strategy” to identify the relationship between surface energy parameters of irrigated crops and rainfed crops over a valid geospatial space range (Li et al., 2016; Shen et al., 2019). We defined a 10 km × 10 km moving window covering 100 1 km × 1 km pixels. If both the paddy field and rainfed crop pixels were located within one moving window, this was defined as a valid comparison sample, within which we could calculate the average , , , , and .
For the potential impact of irrigated crop expansion, the total number of valid windows was 802 (Figure 1C). The window-searching strategy guaranteed that irrigated crop and rainfed crop pixels within the surrounding neighborhood were under similar climate forcing and minimized the uncertainties of local environment backgrounds (e.g., terrain, elevation wind, MODIS viewing zenith angle).
2.3.3 Spatio-temporal correlation analysis
Pearson partial correlation analysis: The variation of land surface biophysical parameters was the main factor affecting the variation of diurnal LST at the local scale. We used the Pearson partial correlation analysis method to explore the individual effects of latent heat flux, solar shortwave radiation energy, and heating potential term changes on diurnal LST changes on the temporal scale during the growing season. These results revealed the essential characteristics of diurnal LST warming and cooling changes (Umair et al., 2020).
Geo-detector model: Geo-detector is a statistical method used to reveal the driving factors behind by spatial heterogeneity. In this study, our core idea was that the spatial distribution of the two factors with correlation would be similar and mainly runs the model in R studio environment. We used the factor detector and the interactive detector to judge the spatial effect of the change of a single-surface energy factor and the interaction of the change of two-surface energy factors on the diurnal LST changes (Zhu et al., 2020). This degree of explanation is measured by the q-value, and the statistical model is as follows:
where n = 1, …, m indicates the subregions (irrigated crops (46163 grids) and rainfed crops (34797 grids)) of variable or ( and ); was the number of moving windows for the entire region (802); and indicate the variances of n of subregions and the whole area, respectively. Larger q-values indicate a stronger explanatory power of driver variation on diurnal LST variations, while the opposite indicates that the explanatory power is weaker; q = 1 indicates that the change of driving factors completely controls the spatial distribution of diurnal LST variations, and the explanatory power reaches 100%.
The factor detector determined the main controlling factor of LST changes mainly by judging the relative magnitude of q-values of multiple single factors. The interaction detector compared the relative magnitudes of q-values of two single factors (e.g., , ) and two single-factor interactions (e.g., ) to determine the type of interaction (divided into five categories) Table 2.
3 Results
3.1 Temporal response of LST and biophysical parameters to irrigation crop expansion
We identified the differences between irrigated crops and rainfed crops in the process when the underlying surface releases latent heat flux and absorbs solar shortwave radiation energy. To reflect the change in the surface energy signal of the prospective growth of irrigated crops, we quantitatively assessed the differences in diurnal LST, latent heat flux, net solar shortwave radiation energy, and heating potential term of irrigated crops and rainfed crops in 2015–2020. During the growing season, the daytime (10:30) and nighttime (22:30) LST differences showed asymmetry (Figures 3A, B). Among these differences, the daytime LST average decreased by 2.11 ± 0.21°C, whereas it increased by 0.64 ± 0.19°C at nighttime, and the daytime cooling range was significantly bigger than the nightly warming range (1.47°C). The order of was as follows: the early growing season (−3.56 ± 0.27°C) > the mid-growing season (−1.35 ± 0.21°C) > the late-growing season (−1.34 ± 0.16°C). The temporal variation characteristics of were consistent with those of , which reached the maximum value (1.98 ± 0.25°C) in the early growing season and the minimum value (−0.76 ± 0.15°C) in the late-growing season. The average values of and were 9.03°C and 11.78°C, respectively, and the average value of δ was −2.75 ± 0.23°C. The main reason for the decrease in was that irrigated crops were subjected to both daytime cooling and nighttime warming (Figure 3C).
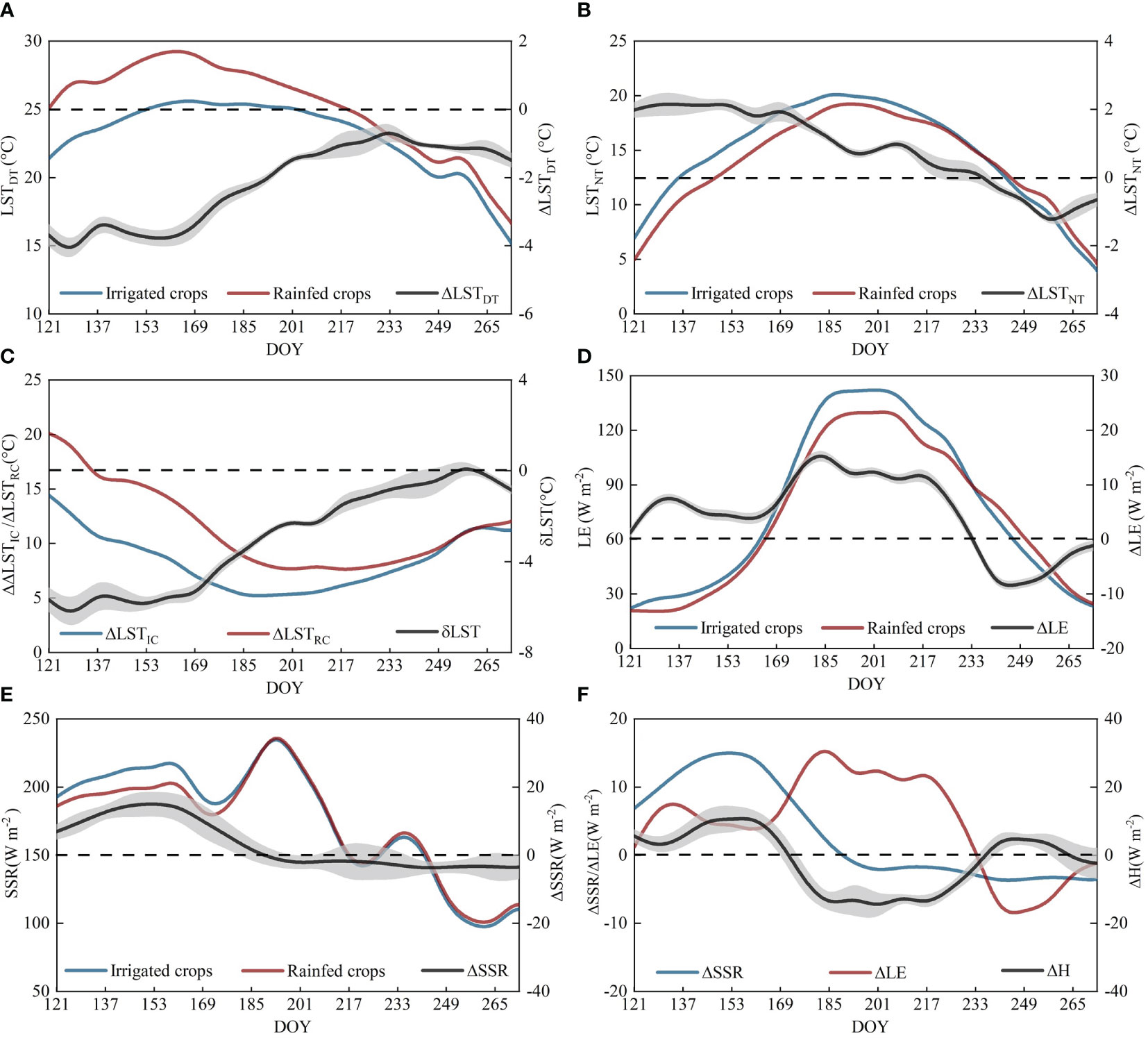
Figure 3 Mean 8-day cycle of land surface parameters of irrigated crops and the adjacent rainfed crops, and their differences during the growing season of 2015–2020. (A) Daytime LST, (B) nighttime LST, (C) diurnal LST, (D) latent heat flux (LE), (E) solar shortwave radiation (SSR), (F) heating potential term (H). Grey shadows represent standard deviation.
The average difference of latent heat fluxes was 4.05 ± 1.01 W m−2 (Figure 3D). This reached its maximum during the mid-growing season (10.35 ± 1.02 W m−2), and the sign of average values of the early growing season (6.01 ± 0.78W m−2) and late-growing season (−3.50 ± 0.92 W m−2) were opposite. The average difference of solar shortwave radiation energy was 2.49 ± 3.12 W m−2. The maximum difference (11.23 ± 3.44 W m−2) in the early growing season was significantly larger than the average value of the entire growing season, whereas the average difference in other months was −2.18 ± 3.02 W m−2. The mean value of the difference of SSR during the whole growing season was greater than zero (Figure 3E). The average difference in heating potential term for DOY = 121–177, was 5.22 ± 2.17 W m−2. The difference of surface heating potential term was the smallest in the late-growing season (0.01 ± 2.94 W m−2; Figure 3F), because the absorbed solar shortwave radiation and the latent heat flux that was released by the surface were similar.
3.2 Spatial response of LST and biophysical parameters to irrigation crop expansion
We observed significant spatial differences in multiyear mean daytime and nighttime LST of irrigated and rainfed crops in 2015–2020. showed an overall high in the west and low in the east (Figure 4A), with a mean value of −2.11°C, which was concentrated in the range of −3°C to 1°C (Figure 4B). In more than 62% of the regions, the was between −2°C and −5°C. Compared with low latitudes, the daytime LST of irrigated crops decreased significantly at high latitudes. The in the eastern region was slightly lower than that in the western region (Figure 4C), with a mean value of 0.64°C, which was concentrated in the range of −2°C to 2°C. In more than 77% of the regions, the was between 0°C and 1°C (Figure 4D). Similarly, was bigger in the high latitudes than that in the low latitudes, which mainly showed a warming effect. Because of the low temperature at high latitudes, the non-irrigated crops entered the growing season late. Low latitudes, on the other hand, are close to the ocean, and heavy rainfall during the growing season reduces the surface heat difference between paddy fields and drylands.
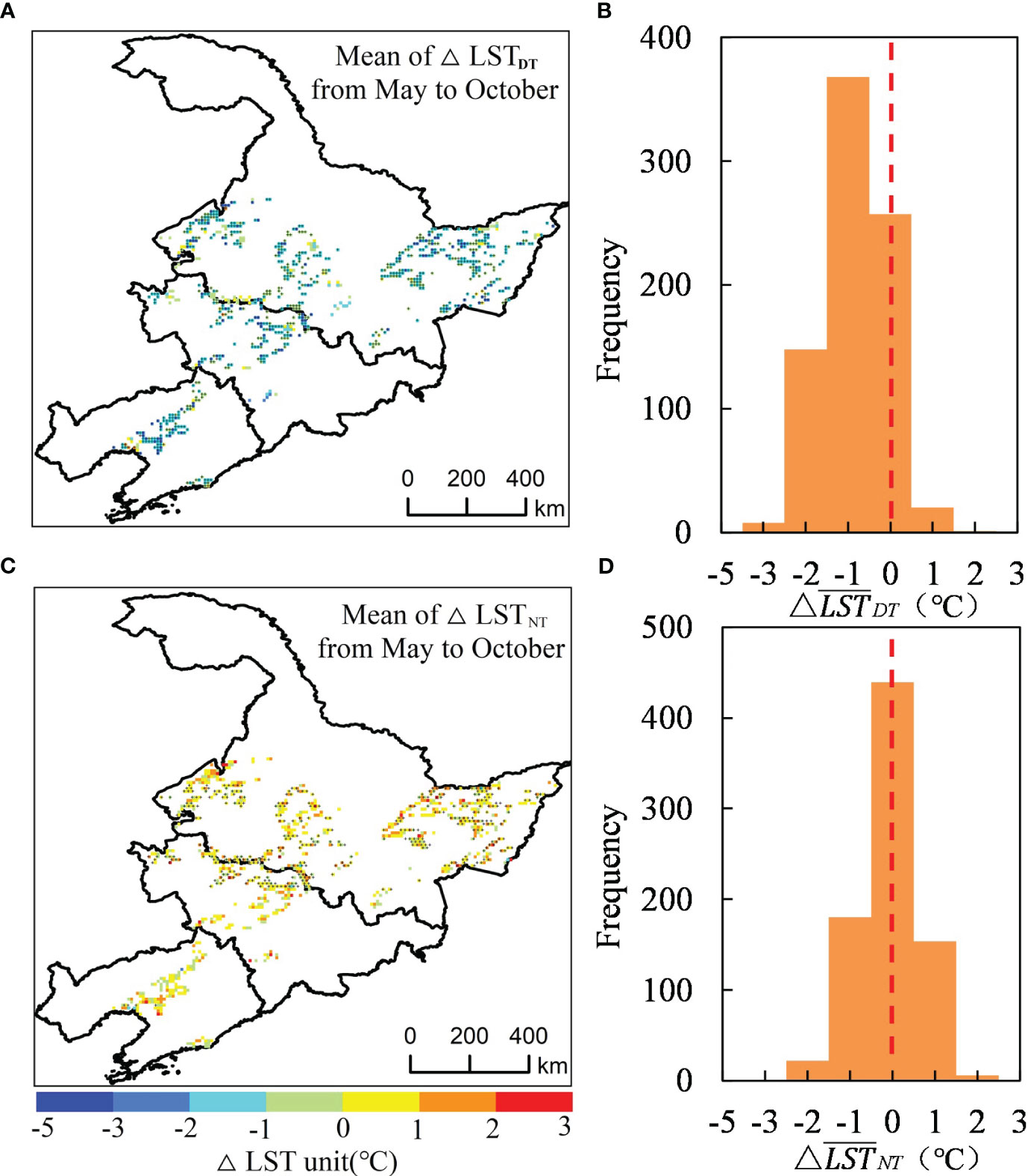
Figure 4 The land surface temperature differences of spatial patterns, latitudinal distributions, and frequency histograms of irrigated crops and the adjacent rainfed crops during the growing season of 2015–2020. (A, B) The spatial distribution map and the histogram of . (C, D) The spatial distribution map and the histogram of . Grids with crosses indicate that the differences are significant at 95% by t-test, grey shadows represent standard deviation.
From May to October, the showed a gradual decrease (Table 3), with a maximum difference (−3.7±0.3) in May (the early irrigation period) and a minimum difference (−0.9±0.3) in August (the vegetation bloom period). The trended upward and then downward, with the greatest difference in June and the smallest difference in September.
We also observed differences in the spatial distribution of , , and of irrigated crops and rainfed crops in 2015–2020 (Figures 5A, C, E). The change patterns of , and were similar, and all showed a weak decreasing trend with an increase in latitude. was more than zero in 70.2% of the locations, and was more than zero in 80.6% of the areas. During the entire growing season, irrigated crops released and absorbed less heating potential term than rainfed crops, and the percentage of heating potential term reduction regions was 53.2% (Figures 5B, D, F).
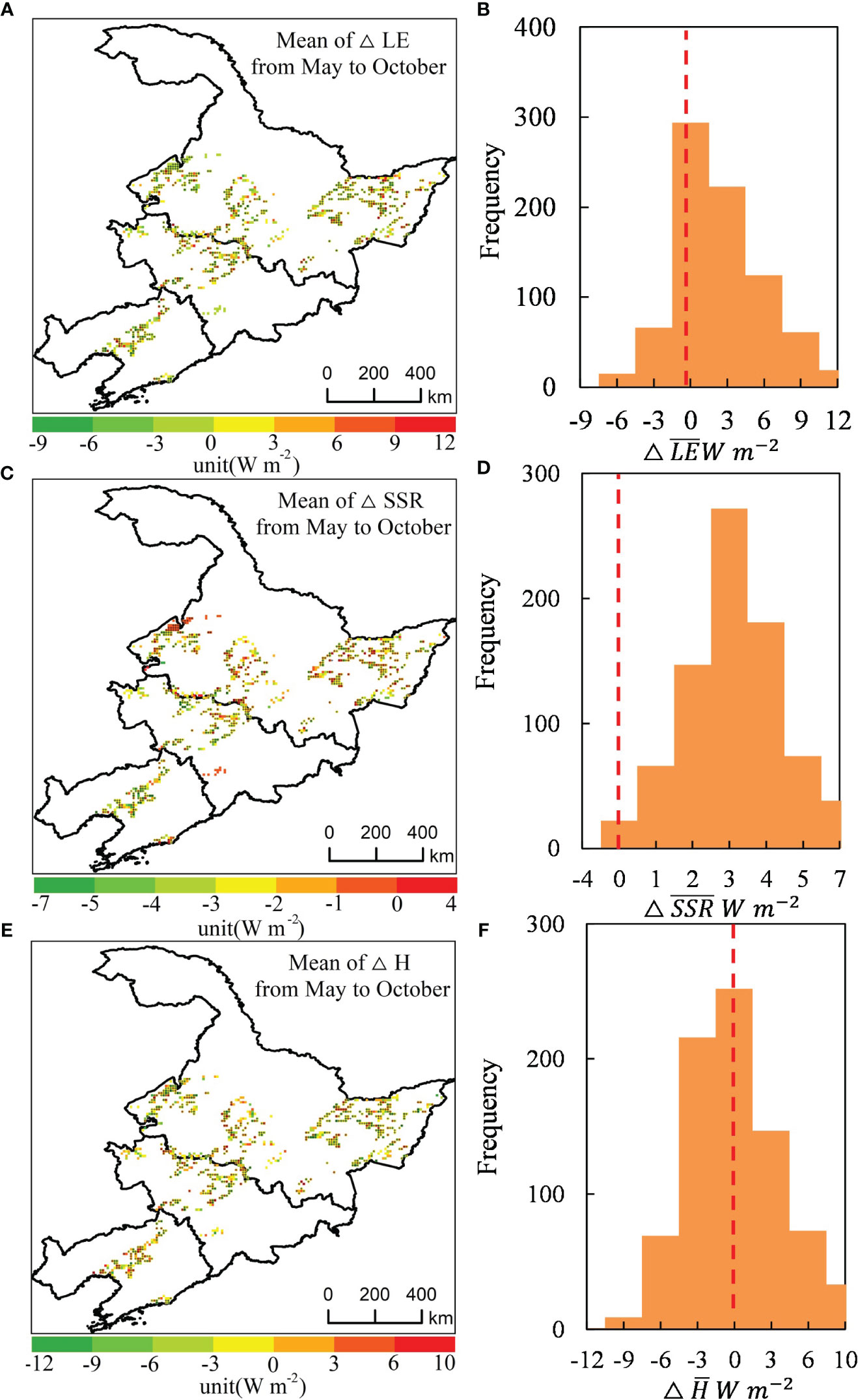
Figure 5 The land surface radiation energy differences of spatial patterns, latitudinal distributions, and frequency histograms of irrigated crops and the adjacent rainfed crops during the growing season of 2015–2020. (A, B) The spatial distribution map and the histogram of . (C, D) The spatial distribution map and the histogram of . (E, F) The spatial distribution map and the histogram of . Grids with crosses indicate that the differences are significant at 95% by t-test, grey shadows represent standard deviation.
The extreme values of and appeared in opposite months (Table 4). reached the maximum in July (12.7±0.9 W m−2) and the minimum in October (0.2±0.9 W m−2). The value of reached its highest point in June (11.4±4.2 W m−2) and its lowest point in July (−1±2.4 W m−2). The heating potential term difference was determined by a combination of latent heat flux and solar shortwave radiation energy.
3.3 Biophysical spatiotemporal driving mechanism of diurnal LST change
3.3.1 Temporal effect of biophysical changes on diurnal LST change
We used latent heat flux and solar shortwave radiation energy and their summation to directly determine the change characteristics of LST. On the seasonal scale, was negatively correlated with , which accounted for 63% of the area (Figure 6A); furthermore, 15% of the regions had highly significant negative correlation. This research demonstrated that when between irrigated and rainfed crops grew, so did . In May–August, the latent heat flux released by irrigated crops was much greater than that released by rainfed crops (> 0), resulting in a cool effect, while < 0 from September to October. Finally, the average daytime LST of irrigated crops was lower than that of rainfed crops during the growing season. Thus, it indicates that the strong cooling effect of latent heat flux in May–August offsets the weak heating effect in September–October. We observed a positive correlation between and , which accounted for 91% of the area (Figure 6B), and indicated that the greater the difference in solar shortwave radiation energy, the greater the difference in daytime LST. Compared with rainfed crops, irrigated crops absorbed more solar shortwave radiation energy in May–June, and less in July–October. However, the was still less than zero, indicating that the cooling effect of high latent heat flux offset the weak warming effect of low solar shortwave radiant energy. was positively correlated with (Figure 6C), which accounted for 77% of the area. The < 0 in entire growing season, eventually produced a cooling effect. This finding revealed that there was a lag effect in the impact of land surface energy.
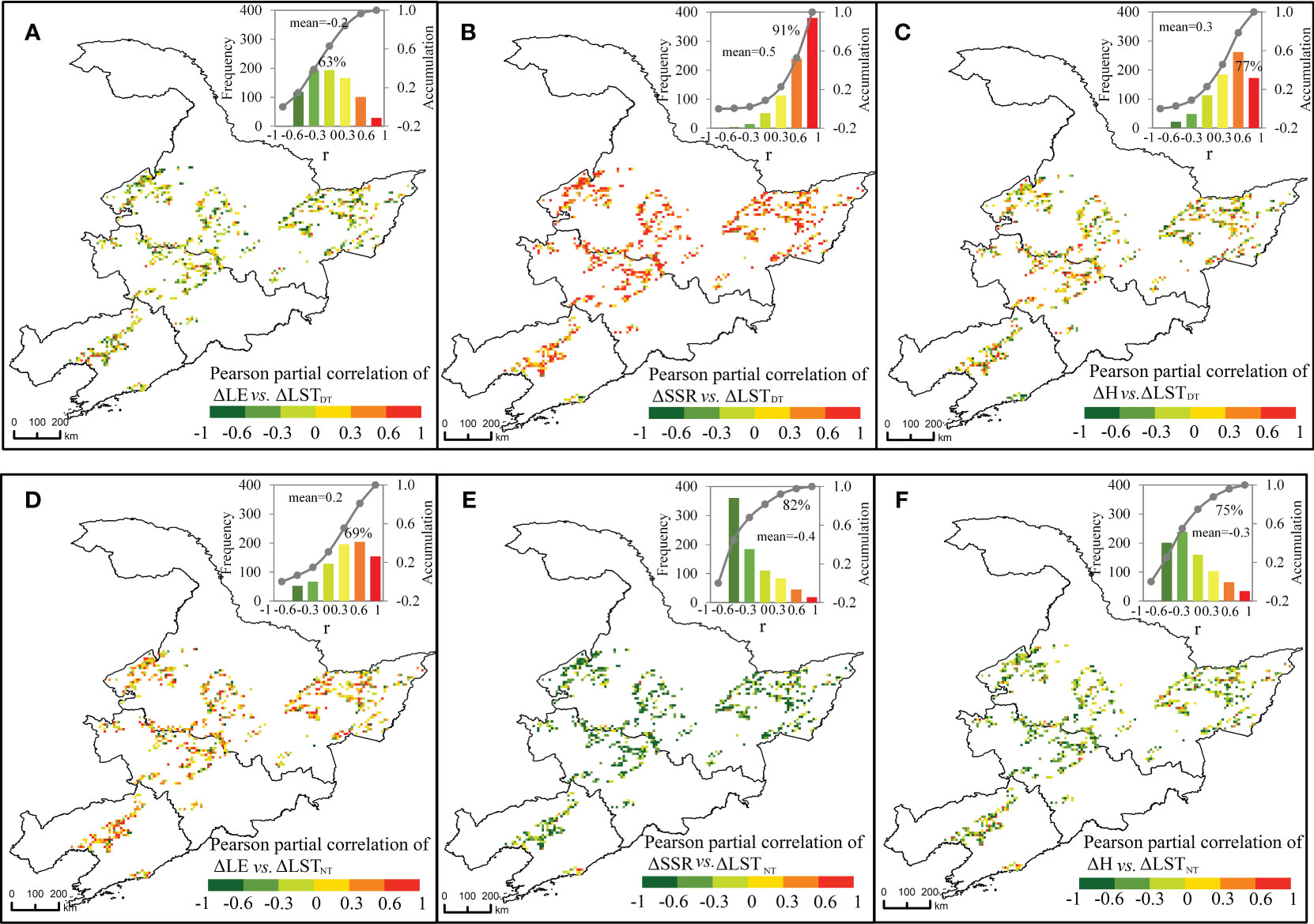
Figure 6 Spatial distribution of time scale correlation and their frequency histograms between diurnal LST change and biophysical parameters change during growing seasons. (A–C) Correlation between ΔLSTDT and ΔLE, ΔSSR, ΔH. (D–F) Correlation between ΔLSTNT and ΔLE, ΔSSR, ΔH.
On the seasonal scale, was positively correlated with , and its area accounted for 69% of the total (Figure 6D). Highly significant positive correlation and substantial positive correlation accounted for 44.6% and 24.4% of the total. This result indicated that with an increase in , its gradually increased. and were inversely associated, as were and , and their regions accounted for 82% and 75%, respectively (Figures 6E, F). Thus, as and increased, the decreased steadily. From May to October, irrigated crops had a higher average latent heat flux and a lower average cumulative heating potential term throughout the day than rainfed crops. Finally, irrigated crops provided nighttime warmth. This result showed that the warming effect of latent heat flux was greater than the cooling effect of daytime-accumulated heating potential term. Therefore, the asymmetry of daytime and nighttime LST variation was caused mainly by the different controlling factors of land surface energy.
3.3.2 Spatial effect of biophysical changes on diurnal LST change
The physical energy of surface components and surrounding ground types underwent lateral exchange. Changes in latent heat flux, solar shortwave radiant energy, and heating potential term factor and their interactions on spatial scales directly determined the spatial distribution patterns of daytime LST changes. As shown in Table 5, the single-factor and had the strongest explanatory power for the spatial distribution of in September (q=0.57, p<0.01) and October (q=0.43, p<0.01), respectively, whereas had significant explanatory power in all of May–October; the mean of the q-value was 0.45.

Table 5 The q-value of the single effects for different driving mechanism on ΔLSTDT, *representing p<0.05, **representing p<0.01.
The latent heat flux, solar shortwave radiant energy, and heating potential term were superimposed, and their interaction also determined . The effect of interaction factors was significantly greater than the single factor effect (Table 5, Figure 7A), and their q-values were all greater than 0.5. Overall, had a more significant effect on than , whereas had the slightest impact. The trends of the monthly variation of the explanatory power of interaction factors on were consistent, and all of them peaked in August (q=0.92, 0.85, and 0.84). Among these factors, had non-linear enhancement throughout the growing season, whereas both and had two-factor and non-linear enhancements over the growing season (Figure 7B).
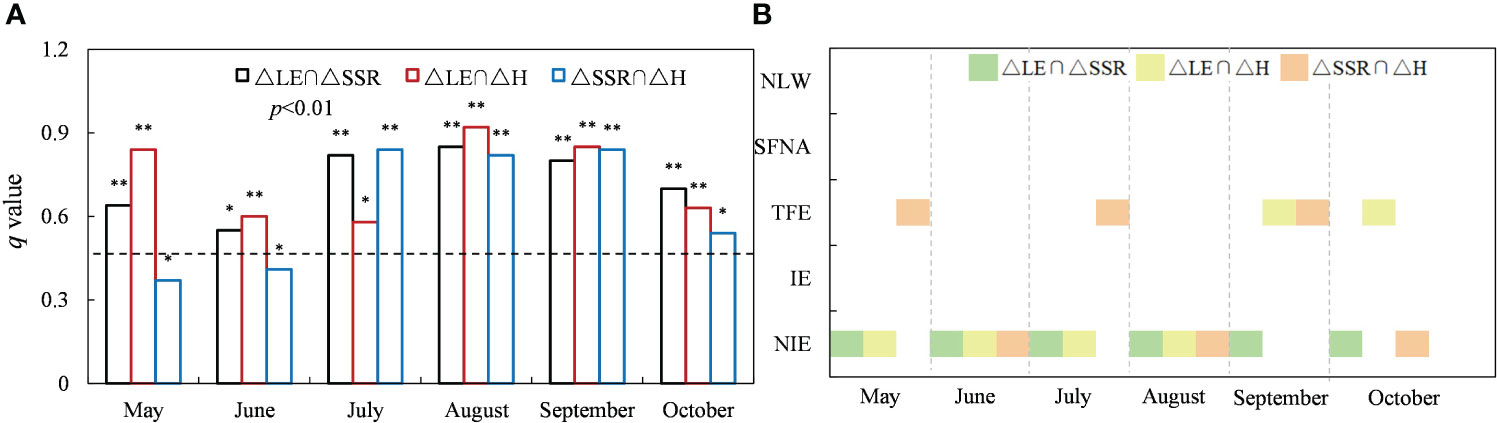
Figure 7 The spatial correlation between ΔLE (ΔSSR and ΔH) and ΔLSTDT from May to October. (A) Interaction detection results (q-value). (B) Interaction detector classifications. *representing p<0.05, **representing p<0.01.
Corresponding to the time scale, at the spatial scale, changes in the land surface energy factor affected the to a lesser extent than the , due to the cumulative transfer in time weakened the effects of land surface energy factor. The explanatory power of the single components , , and for the nighttime LST rise was best in August (q = 0.39, 0.3, and 0.56) and poorest in September, all with a q-value of 0.1. (Table 6). The at the surface was also the primary determining element for nighttime irrigated crop warming.

Table 6 The q-value of the single effects for different driving mechanism on ΔLSTNT. *representing p<0.05, **representing p<0.01.
The explanatory power of the interaction factor on the was significantly greater than the single-factor effect (Table 6, Figure 8A). Consistent with the influence mechanism of the , had a greater degree of action on than ∩ . The explanatory power of the interaction factors was the strongest in August (q=0.71, 0.82, and 0.80) and the weakest in June (q<0.5). The interaction effects of and were non-linear enhancements, except for August (two-factor enhancement), but was all non-linear enhancements throughout the growing season (Figure 8B).
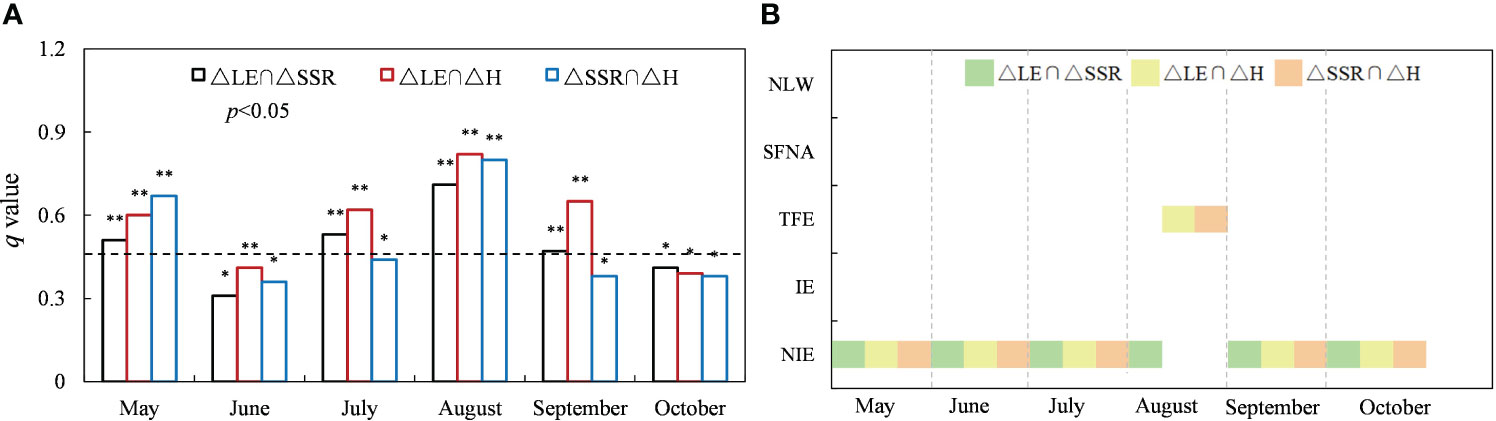
Figure 8 The spatial correlation between ΔLE (ΔSSR and ΔH) and ΔLSTNT from May to October. (A) Interaction detection results (q-value). (B) Interaction detector classifications. *representing p<0.05, **representing p<0.01.
4 Discussion
4.1 Analysis of the time variation of LST and biophysical parameters
The effect of the expansion of irrigated crops on land surface temperature in cold regions China has attracted widespread attention. It is generally believed that during the growing season, the change range of LST is between 1.3°C and 2.0°C during daytime and between 0.8°C and 1.1°C during nighttime (Liu et al., 2018; Liu et al., 2019a; Yu and Liu, 2019; Liu et al., 2022). Previous researches have explored the state of surface temperature variation on a seasonal or interannual scale. This work, we gathered more detailed dynamic information through a fine time scale in 8 day (Figures 9A, B). In addition, we averaged the time series information over nearly 5 years to prevent extreme climate disturbances, such as extreme heat, heat waves, and droughts. Previous studies analyzing the response mechanisms of land surface temperature changes have divided the growing season into different stages (e.g., early growing season, late-growing season) on a monthly scale, and thus, the details of LST time series changes were weakened. Because of the high sensitivity of land surface temperature, the inconsistency of surrounding features (urban, farmland) and climatic conditions (wind speed, wind direction) significantly affected the regional scale LST (Li et al., 2013; Ma et al., 2022). Therefore, when exploring the influence mechanism of LST changes in different process frameworks as well as different research areas, the growing season should be accurately divided according to the crop farming time in the study area. To effectively capture the response mechanism’s temporal dynamic characteristics (Figures 9C–F).
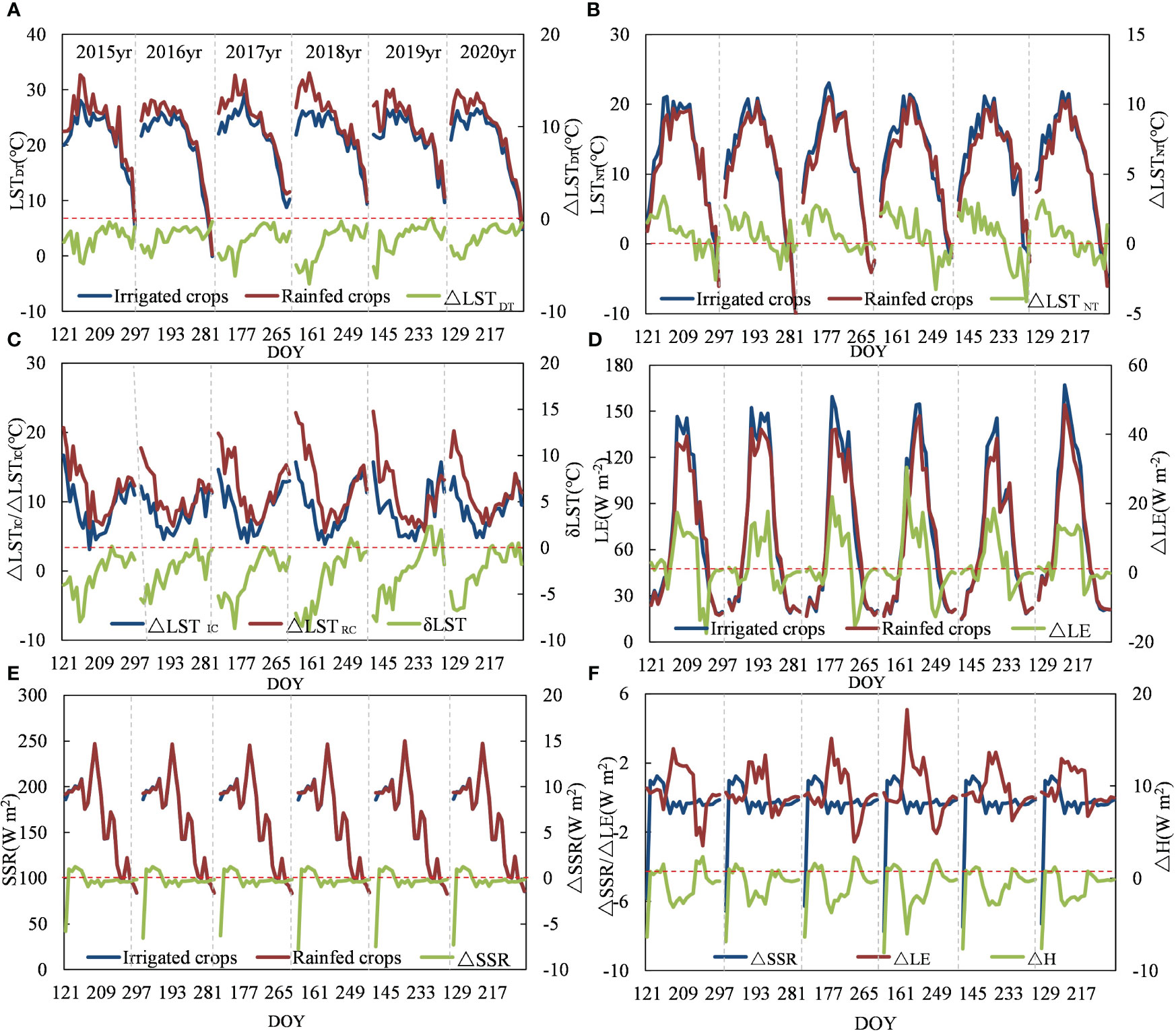
Figure 9 Temporal variation of land surface parameters of irrigated crops and the adjacent rainfed crops, and their differences from 2015 to 2020 (DOY=121–297). (A) Daytime LST, (B) nighttime LST, (C) diurnal LST, (D) latent heat flux, (E) solar shortwave radiation, (F) heating potential term.
4.2 Analysis of the spatial variation of LST and biophysical parameters
We found significant spatial heterogeneity in the diurnal LST differences from May to October. Among these distinctions was the irrigation time for paddy fields in early May, when the water content of the subsurface was much higher than that of non-irrigated crops (Figures 10A, 11B). During the day, irrigation crops had a high evapotranspiration capacity, but at night, the specific heat capacity of water was greater than that of bare soil, which played a role in heat preservation (Jin et al., 2016). The paddy fields were tillered in mid-June, when the surrounding crops were cultivated, and the difference in surface water-heat energy between low vegetation and bare soil was large (Figures 10B, 11B), which eventually led to a significant variation in LST between the irrigated and non-irrigated crops (Dong et al., 2014). July–August was the booting period of irrigated crops, when the land surfaces of irrigated and non-irrigated crops were covered with a large amount of vegetation at the same time. The land surface evapotranspiration capacity, water consumption-renewal capacity, and solar shortwave radiation absorption capacity of irrigated crops were comparable to non-irrigated crops (Figures 10C, D, 11C, D) (Gorguner and Kavvas, 2020; Jiang et al., 2021). September–October was the crop harvesting period, when bare soil was the major land surface cover type and the soil moisture content of irrigated and non-irrigated crops was similar (Cierniewski and Ceglarek, 2018; Rojas et al., 2020), and eventually, the difference in harvesting time-points led to heterogeneity in their diurnal LST differences on a spatial scale (Figures 10E, F, 11E, F). Moreover, the cold regions of China had a vast north–south span, and the distribution of solar shortwave radiation varied substantially across latitudes, resulting in a climate with significant latitudinal zonality. As a result, the study area is spatially split based on crop harvest time points and climate variances to investigate the interpretation of surface water-heat energy balance to surface temperature changes in different regions and at different harvesting time points.
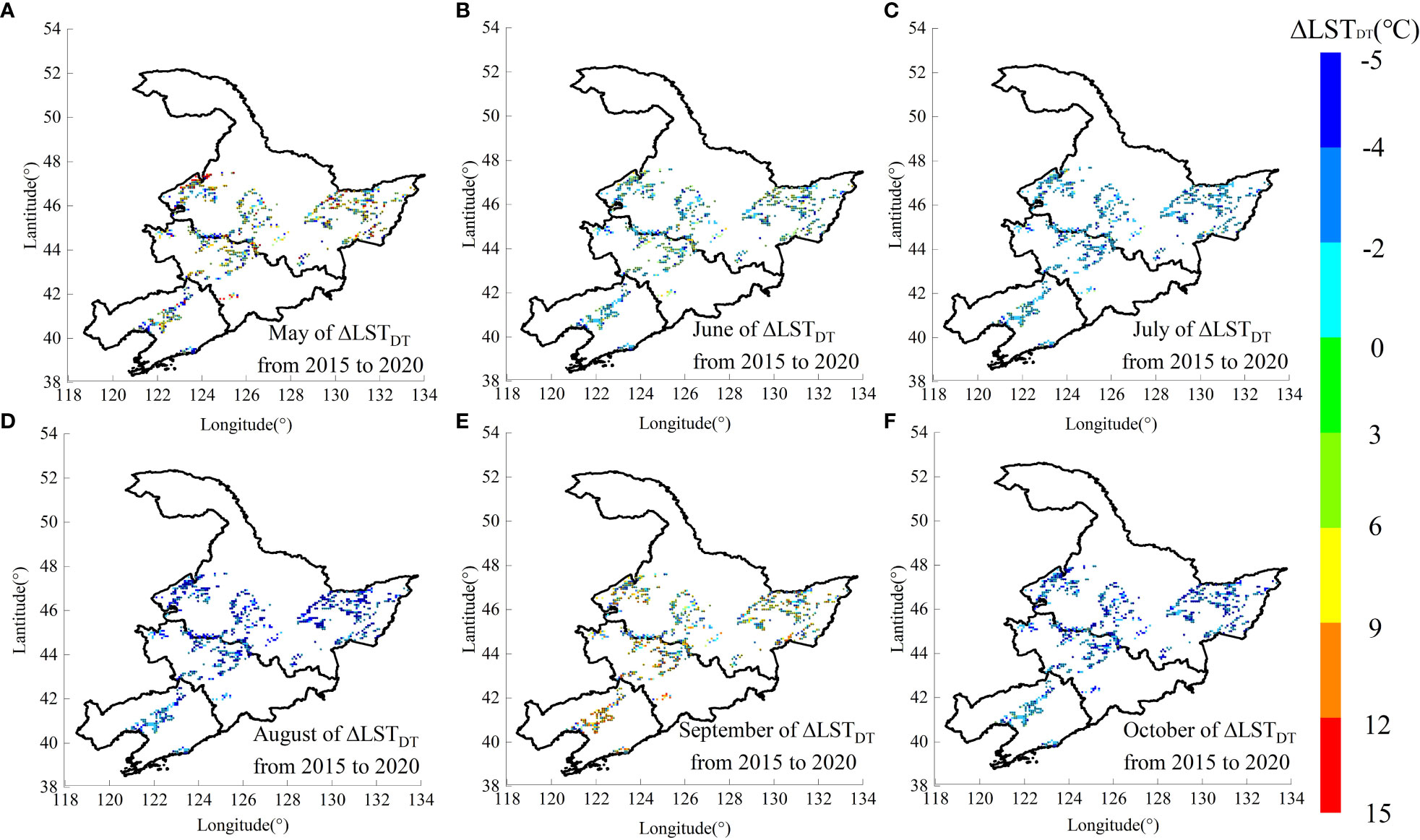
Figure 10 Monthly spatial variation of mean daytime land surface temperature from 2015 to 2020. (A) Daytime LST in May, (B) daytime LST in June, (C) daytime LST in July, (D) daytime LST in August, (E) daytime LST in September, (F) daytime LST in October.
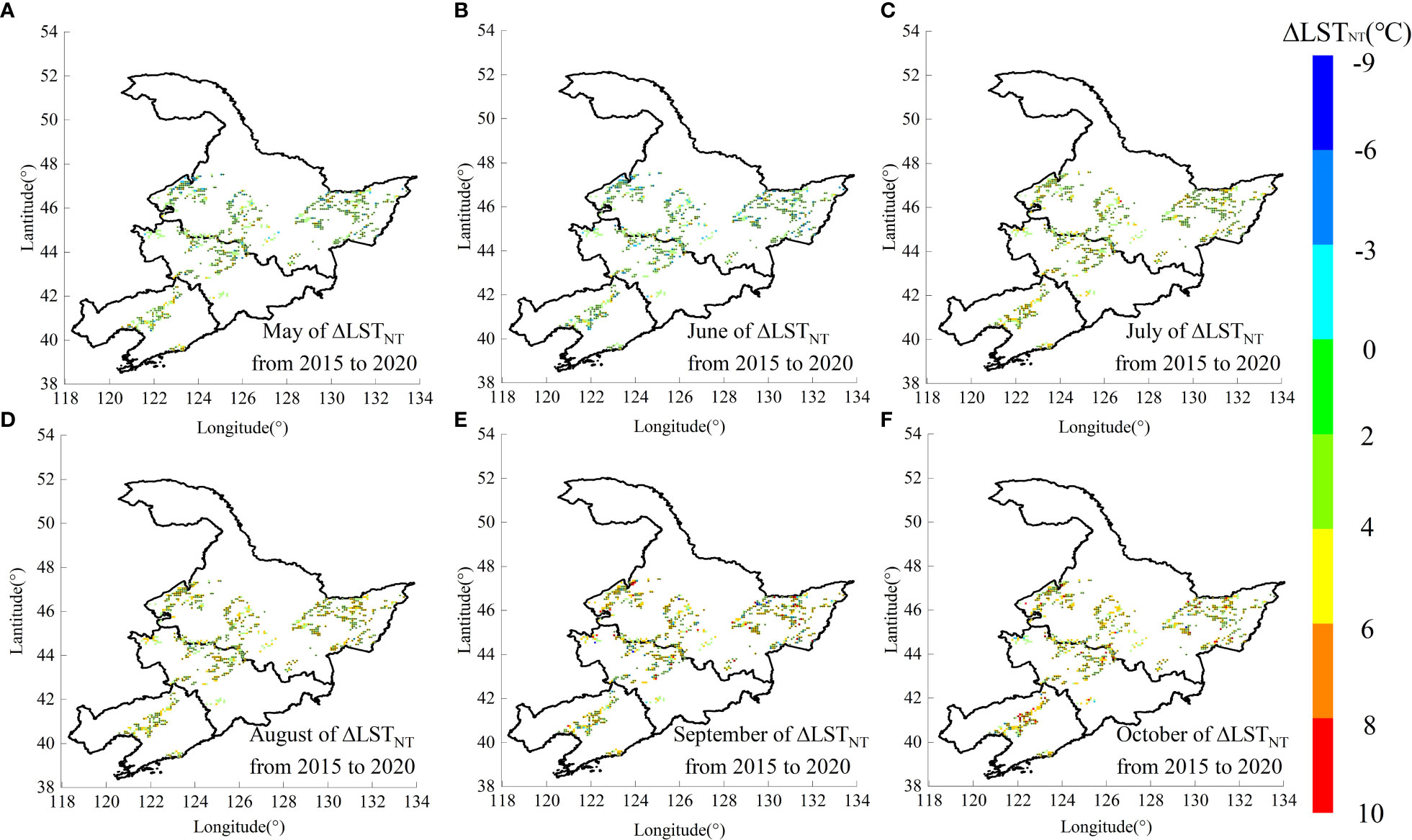
Figure 11 Monthly spatial variation of mean daytime land surface temperature from 2015 to 2020. (A) Nighttime LST in May, (B) nighttime LST in June, (C) nighttime LST in July, (D) nighttime LST in August, (E) nighttime LST in September, (F) nighttime LST in October.
4.3 Exploring the effect mechanism of diurnal LST change
Numerous studies have found that irrigation crop expansion influence the spatial–temporal distribution of regional and even global climate primarily through biogeochemical processes and biophysical parameters. Among them, biophysical parameters play a larger role at regional scales than biogeochemical processes, such as the high latitudes of the Northern Hemisphere (Brovkin et al., 2006; Alkama and Cescatti, 2016). Many studies have investigated the effects of land use/cover changes (wetland to cropland or dryland to artificial wetland) on LST in the high-latitude regions, and most of them have explored the main influencing mechanisms of daytime on irrigated farmland changes from the perspectives of non-radiative mechanisms (evapotranspiration) and radiative mechanisms (albedo) (Liu et al., 2018; Liu et al., 2019a; Yu and Liu, 2019). In contrast, our study focuses on analyzing how the heating potential term responds to diurnal LST variations and finds that the LST variations are mainly due to the relative magnitudes of the surface energy income term (solar incident shortwave radiation), and the surface energy expenditure term (latent heat flux). Among them, irrigated crops ultimately lead to a decrease in the heating potential term during the growing season due to more latent heat flux exported from the surface and less solar shortwave radiation absorbed, which is consistent with the findings of the existing studies that there is a cooling effect on irrigated crops (Zhu et al., 2011; Yang et al., 2020; Zhang et al., 2022).
As the influencing factors of nighttime LST were complex, the results of current studies on the influencing mechanism of nighttime LST change have not been fully proved (Yuan et al., 2022). Therefore, it remains challenging to explore the main influencing mechanism of nighttime LST, which is an issue for both afforestation and deforestation. (Peng et al., 2014; Li et al., 2016; Shen et al., 2019; Yuan et al., 2022). Existing studies have found that land use/cover changes in northeastern China enhance atmospheric water vapor mainly through evapotranspiration, which enhances downward atmospheric longwave radiation and strengthens the nighttime greenhouse warming effect through measured data (Li et al., 2017). We focused on the effect of the interaction between nighttime atmospheric inverse radiation as well as daytime thermal inertia on nighttime LST changes. However, it has also been suggested that the magnitude of the specific heat capacity of surface components is also a major mechanism influencing the nighttime LST, especially between regions with large differences in surface water content (Cao et al., 2019; Shen et al., 2022). Therefore, to accurately evaluate the main controlling factors of nighttime LST warming, we should comprehensively analyze the potential impact mechanism of warming from the multifactor perspective.
4.4 Uncertainties and future work
In this work, we assumed that irrigated crop expansion occurred in the region during 2015–2020. We emphasized the biophysical effects of “potential” changes in irrigated crops (paired comparison with surrounding non-irrigated crops) on diurnal LST change to accurately obtain more valid window samples and remove the climate background differences caused by long time series and large spans. Another concept that corresponds to “potential” change, namely the application of “actual” change, was reflected in a typical land use and cover changes (afforestation or deforestation.) study. They found that the effects of “potential” and “actual” changes in deforestation (afforestation) on diurnal LST were similar in most regions. So whether the impacts of potential and actual changes in irrigated crops on diurnal LST are consistent. We also wondered how the biophysical mechanisms affected the diurnal LST in the actual changes of irrigated crops. This study showed that the expansion of irrigated crops produced a cooling effect during the daytime and whether its effect could offset global warming caused by long-term human activities. In addition, the cooling effect of irrigated crops with different planting patterns was different, which raised the question of whether or not the cooling effect of high-density irrigated crops lasted longer than low-density. These issues require further exploration.
Because the input parameters of the observed MODIS LST products were not refined between irrigated and non-irrigated crops, they did not consider the species specificity between different crops. As a result, the differences in LST between crops were underestimated. Furthermore, the current thermal infrared remote-sensing satellites are characterized by mutually exclusive spatial–temporal resolutions, and it is difficult for a single remote-sensing sensor to obtain LST data with high spatial–temporal resolutions. Following that, we used a multisource data fusion approach to obtain an all-weather, high-spatial-resolution LST dataset to meet the needs for fine monitoring of LST.
5 Conclusions
The expansion of irrigated crops in the Northeast China significantly altered regional climate, as revealed by our synergetic investigation based on multi-source remote sensing satellite data and the pairwise comparison method. We found an asymmetric variation in diurnal LST, with daytime LST varying more than nighttime LST. The daytime cooling and nighttime warming effects were mainly due to the fact that latent heat fluxes released from irrigated crops is greater than the solar shortwave radiation absorbed, resulting in less heating potential term. The variation in the average land surface parameters had spatial heterogeneity during the growing season. The diurnal LST variation was affected by different energy factors, and its response to land surface energy was accompanied by a lag effect. followed a decreasing trend as increased, but it followed an increasing trend as and increased. This result indicated that the decrease in was mainly due to the cooling effect of thermal energy of output and input of irrigated crops to offset the warming effect. showed an increasing trend with increasing and a decreasing trend with increasing and . This result demonstrated that the increase in the was due to the fact that the latent heat flux of irrigated crops and the accumulated daytime heating potential term had a greater warming effect than the cooling effect at night from May to October. The degree of influence of the interaction factor on the difference of diurnal LST was significantly greater than the single factor effect. Our study provided a new perspective for comprehending how human activities have an impact on regional, and even global climate change by revealing the spatiotemporal pattern of the biophysical effects of irrigated crop expansion.
Data availability statement
The original contributions presented in the study are included in the article/supplementary material. Further inquiries can be directed to the corresponding author.
Author contributions
XL: Conceptualization, Methodology, Validation, Formal analysis, Investigation, Data Curation, Writing – Original Draft, Visualization. QH: Writing – Review & Editing, Supervision, Funding acquisition. KX: Writing – Review & Editing, Visualization. BV: Supervision, Funding acquisition. YB: Supervision, Project administration. All authors contributed to the article and approved the submitted version.
Funding
All authors appreciated the financially support for the present study which was founded by the International Cooperation and Exchange Program of National Natural Science Foundation of China (41961144019) and key research base of humanities and social sciences in universities of Inner Mongolia Autonomous Region-Yinshan Cultural Research Center research project (23YSYJ00014).
Conflict of interest
The authors declare that the research was conducted in the absence of any commercial or financial relationships that could be construed as a potential conflict of interest.
Publisher’s note
All claims expressed in this article are solely those of the authors and do not necessarily represent those of their affiliated organizations, or those of the publisher, the editors and the reviewers. Any product that may be evaluated in this article, or claim that may be made by its manufacturer, is not guaranteed or endorsed by the publisher.
References
Abera T. A., Heiskanen J., Pellikka P., Rautiainen M., Maeda E. E. (2019). Clarifying the role of radiative mechanisms in the spatio-temporal changes of land surface temperature across the Horn of Africa. Remote Sens. Environ. 221, 210–224. doi: 10.1016/j.rse.2018.11.024
Adegoke J. O., Pielke R. A. Sr., Eastman J., Mahmood R., Hubbard K. G. (2003). Impact of irrigation on midsummer surface fluxes and temperature under dry synoptic conditions: A regional atmospheric model study of the US High Plains. Monthly Weather Rev. 131 (3), 556–564. doi: 10.1175/1520-0493(2003)131<0556:IOIOMS>2.0.CO;2
Alkama R., Cescatti A. J. S. (2016). Biophysical climate impacts of recent changes in global forest cover. Science 351, 600–6046273. doi: 10.1126/science.aac8083
Arora V. K., Montenegro A. J. N. G. (2011). Small temperature benefits provided by realistic afforestation efforts. Nat. Geosci. 4 (8), 514–518. doi: 10.1038/ngeo1182
Bajželj B., Richards K. S., Allwood J. M., Smith P., Dennis J. S., Curmi E., et al. (2014). Importance of food-demand management for climate mitigation. Nat. Climate Change 4 (10), 924–929.
Bonan G. B., Pollard D., Thompson S. L. (1992). Effects of boreal forest vegetation on global climate. Nature 359, 716–718. doi: 10.1038/359716a0
Brovkin V., Claussen M., Driesschaert E., Fichefet T., Kicklighter D., Loutre M. F., et al. (2006). Biogeophysical effects of historical land cover changes simulated by six earth system models of intermediate complexity. Climate Dynamics 26, 587–600. . doi: 10.1007/s00382-005-0092-6
Cao Q., Wu J., Yu D., Wang W. J. A., Meteorology F. (2019). The biophysical effects of the vegetation restoration program on regional climate metrics in the Loess Plateau, China. Agric. For. Meteorology 268, 169–180. doi: 10.1016/j.agrformet.2019.01.022
Chen C., Ge J., Guo W., Cao Y., Liu Y., Luo X., et al. (2022). The biophysical impacts of idealized afforestation on surface temperature in china: Local and nonlocal effects. J. Climate 35 (23), 7833–7852. doi: 10.1175/JCLI-D-22-0144.1
Chen W., Wang Y., Zhao Z., Cui F., Gu J., Zheng X. (2013). The effect of planting density on carbon dioxide, methane and nitrous oxide emissions from a cold paddy field in the sanjiang plain, northeast china. Agriculture Ecosyst. Environ. 178, 64–70. doi: 10.1016/j.agee.2013.05.008
Chen X., Jeong S.-J. (2018). Irrigation enhances local warming with greater nocturnal warming effects than daytime cooling effects. Environ. Res. Lett. 13 (2), 024005. doi: 10.1088/1748-9326/aa9dea
Christy J. R., Norris W. B., Redmond K., Gallo K. P. (2006). Methodology and results of calculating central California surface temperature trends: evidence of human-induced climate change? J. Climate 19 (4), 548–563. doi: 10.1175/JCLI3627.1
Cierniewski J., Ceglarek J. (2018). Annual dynamics of shortwave radiation of bare arable lands on a global scale incorporating their roughness. Environ. Earth Sci. 77 (23), 1–17. doi: 10.1007/s12665-018-7956-7
Delzeit R., Zabel F., Meyer C., Václavík T. (2017). Addressing future trade-offs between biodiversity and cropland expansion to improve food security. Regional Environ. Change 17 (5), 1429–1441. doi: 10.1007/s10113-016-0927-1
Dong Z., Wang Z., Liu D., Song K., Li L., Jia M., et al. (2014). Mapping wetland areas using landsat-derived NDVI and LSWI: A case study of west songnen plain, northeast china. J. Indian Soc. Remote Sens. 42, 569–576. doi: 10.1007/s12524-013-0357-1
Dong J., Xiao X., Zhang G., Menarguez M. A., Choi C. Y., Qin Y., et al. (2016). Northward expansion of paddy rice in northeastern asia during 2000 -2014. Geophysical Res. Lett. 43 (8), 3754–3761. doi: 10.1002/2016GL068191
Du H., He H. S., Wu Z., Wang L., Zong S., Liu J. (2017). Human influences on regional temperature change -comparing adjacent plains of china and russia. Int. J. Climatology 37 (6), 2913–2922. doi: 10.1002/joc.4888
FAO. (2018). Food and agriculture organization of the United Nations. Available at: http://faostat.
Feddema J. J., Oleson K. W., Bonan G. B., Mearns L. O., Buja L. E., Meehl G. A., et al. (2005). The importance of land-cover change in simulating future climates. Science 310 (5754), 1674–1678. doi: 10.1126/science.1118160
Gaupp F., Hall J., Hochrainer-Stigler S., Dadson S. (2020). Changing risks of simultaneous global breadbasket failure. Nat. Climate Change 10 (1), 54–57. doi: 10.1038/s41558-019-0600-z
Gorguner M., Kavvas M. L. (2020). Modeling impacts of future climate change on reservoir storages and irrigation water demands in a Mediterranean basin. Sci. Total Environ. 748, 141246. doi: 10.1016/j.scitotenv.2020.141246
He Y., Lee E., Mankin J. S. (2020). Seasonal tropospheric cooling in Northeast China associated with cropland expansion. Environ. Res. Lett. 15 (3), 034032. doi: 10.1088/1748-9326/ab6616
Huang Y., Yu Y., Zhang W., Sun W., Liu S., Jiang J., et al. (2009). Agro-C: A biogeophysical model for simulating the carbon budget of agroecosystems. Agric. For. Meteorology 149 (1), 106–129. doi: 10.1016/j.agrformet.2008.07.013
Jiang F., Xie X., Liang S., Wang Y., Zhu B., Zhang X., et al. (2021). Loess plateau evapotranspiration intensified by land surface radiative forcing associated with ecological restoration. Agric. For. Meteorology 311, 108669. doi: 10.1016/j.agrformet.2021.108669
Jin C., Xiao X., Dong J., Qin Y., Wang Z. (2016). Mapping paddy rice distribution using multi-temporal Landsat imagery in the Sanjiang Plain, northeast China. Front. Earth Sci. 10 (1), 49–62. doi: 10.1007/s11707-015-0518-3
Li Z.-L., Tang B. H., Wu H., Ren H., Yan G., Wan Z., et al. (2013). Satellite-derived land surface temperature: Current status and perspectives. Remote Sens. Environ. 131, 14–37. doi: 10.1016/j.rse.2012.12.008
Li Y., Zhao M., Motesharrei S., Mu Q., Kalnay E., Li S. (2015). Local cooling and warming effects of forests based on satellite observations. Nat. Commun. 6 (1), 6603. doi: 10.1038/ncomms7603
Li Y., Zhao M., Mildrexler D. J., Motesharrei S., Mu Q., Kalnay E., et al. (2016). Potential and actual impacts of deforestation and afforestation on land surface temperature. J. Geophysical Research: Atmospheres 121 (24), 14–372. doi: 10.1002/2016JD024969
Li X., Zhang X., Zhang L. (2017). Observed effects of vegetation growth on temperature in the early summer over the northeast China plain. Atmosphere 8 (6), 97. doi: 10.3390/atmos8060097
Lian X., Jeong S., Park C. E., Xu H., Li L. Z., Wang T., et al. (2022). Biophysical impacts of northern vegetation changes on seasonal warming patterns. Nat. Commun. 13 (1), 3925. doi: 10.1038/s41467-022-31671-z
Liang S., Li Y., Zhang X., Sun Z., Sun N., Duan Y., et al. (2018). Response of crop yield and nitrogen use efficiency for wheat-maize cropping system to future climate change in northern china. Agric. For. Meteorology 262, 310–321. doi: 10.1016/j.agrformet.2018.07.019
Liu W., Dong J., Du G., Zhang G., Hao Z., You N., et al. (2022). Biophysical effects of paddy rice expansion on land surface temperature in northeastern asia. Agric. For. Meteorology 315, 108820. doi: 10.1016/j.agrformet.2022.108820
Liu T., Yu L., Bu K., Yan F., Zhang S. (2018). Seasonal local temperature responses to paddy field expansion from rain-fed farmland in the cold and humid Sanjiang Plain of China. Remote Sens. 10 (12), 2009. doi: 10.3390/rs10122009
Liu T., Yu L., Zhang S. J. (2019a). Impacts of wetland reclamation and paddy field expansion on observed local temperature trends in the Sanjiang Plain of China. J. Geophysical Research: Earth Surface 124 (2), 414–426. doi: 10.1029/2018JF004846
Liu T., Yu L., Zhang S. J. S. R. (2019b). Land surface temperature response to irrigated paddy field expansion: a case study of semi-arid western Jilin Province, China. Sci. Rep. 9 (1), 1–8. doi: 10.1038/s41598-019-41745-6
Lobell D., Bala G., Mirin A., Phillips T., Maxwell R., Rotman D. (2009). Regional differences in the influence of irrigation on climate. J. Climate 22 (8), 2248–2255. doi: 10.1175/2008JCLI2703.1
Ma Y., Zhou J., Liu S., Zhang W., Zhang Y., Xu Z., et al. (2022). Estimation of evapotranspiration using all-weather land surface temperature and variational trends with warming temperatures for the river source region in southwest china. J. Hydrology 613, 128346. doi: 10.1016/j.jhydrol.2022.128346
Malyshev S., Shevliakova E., Stouffer R. J., Pacala S. W. (2015). Contrasting local versus regional effects of land-use-change-induced heterogeneity on historical climate: analysis with the GFDL earth system model. J. Climate 28 (13), 5448–5469. doi: 10.1175/JCLI-D-14-00586.1
Moon M., Li D., Liao W., Rigden A. J., Friedl M. A. (2020). Modification of surface energy balance during springtime: The relative importance of biophysical and meteorological changes. Agric. For. Meteorology 284, 107905. doi: 10.1016/j.agrformet.2020.107905
Mu Q., Zhao M., Running S. W. (2011). Improvements to a MODIS global terrestrial evapotranspiration algorithm. Remote Sens. Environ. 115 (8), 1781–1800. doi: 10.1016/j.rse.2011.02.019
Pan T., Zhang C., Kuang W., Luo G., Du G., Yin Z.. (2020). Large-scale rain-fed to paddy farmland conversion modified land-surface thermal properties in Cold China. Sci. Total Environ. 722, 137917. doi: 10.1016/j.scitotenv.2020.137917
Peng S. S., Piao S., Zeng Z., Ciais P., Zhou L., Li L. Z., et al. (2014). Afforestation in china cools local land surface temperature. Proc. Natl. Acad. Sci. 111 (8), 2915–2919. doi: 10.1073/pnas.1315126111
Pongratz J., Reick C., Raddatz T., Claussen M. (2010). Biogeophysical versus biogeochemical climate response to historical anthropogenic land cover change. Geophysical Res. Lett. 37 (8). doi: 10.1029/2010GL043010
Qiu B., Hu X., Chen C., Tang Z., Yang P., Zhu X., et al. (2022). Maps of cropping patterns in china during 2015 -2021. Sci. Data 9 (1), 479. doi: 10.1038/s41597-022-01589-8
Rojas F., Rubio C., Rizzo M., Bernabeu M., Akil N., Martín F. (2020). Land use and land cover in irrigated drylands: a long-term analysis of changes in the mendoza and tunuyán river basins, argentin-2018). Appl. Spatial Anal. Policy 13, 875–899. doi: 10.1007/s12061-020-09335-6
Sacks W. J., Cook B. I., Buenning N., Levis S., Helkowski J. H. (2009). Effects of global irrigation on the near-surface climate. Climate Dynamics 33 (2), 159–175. doi: 10.1007/s00382-008-0445-z
Schaaf C. B., Gao F., Strahler A. H., Lucht W., Li X., Tsang T., et al. (2002). Albedo nadir reflectance products from MODIS. Remote Sens. Environ. 83 (1-2), 135–148. doi: 10.1016/S0034-4257(02)00091-3
Schultz N. M., Lawrence P. J., Lee X. J. (2017). Global satellite data highlights the diurnal asymmetry of the surface temperature response to deforestation. J. Geophysical Research: Biogeosciences 122 (4), 903–917. doi: 10.1002/2016JG003653
Schultz N. M., Lee X., Lawrence P. J., Lawrence D. M., Zhao L. J. (2016). Assessing the use of subgrid land model output to study impacts of land cover change. J. Geophysical Research: Atmospheres 121 (11), 6133–6147. doi: 10.1002/2016JD025094
Shen W., Li M., Huang C., He T., Tao X., Wei A. (2019). Local land surface temperature change induced by afforestation based on satellite observations in guangdong plantation forests in china. Agric. For. Meteorology 276, 107641. doi: 10.1016/j.agrformet.2019.107641
Shen X., Liu Y., Liu B., Zhang J., Wang L., Lu X., et al. (2022). Effect of shrub encroachment on land surface temperature in semi-arid areas of temperate regions of the Northern Hemisphere 320, 108943. doi: 10.1016/j.agrformet.2022.108943
Sun M., Zhang Y., Ma J., Yuan W., Li X., Cheng X.. (2017). Satellite data based estimation of methane emissions from rice paddies in the Sanjiang Plain in northeast China. PloS One 12 (6). doi: 10.1371/journal.pone.0176765
Umair M., Kim D., Ray R. L., Choi M. J. A., Meteorology F. (2020). Evaluation of atmospheric and terrestrial effects in the carbon cycle for forest and grassland ecosystems using a remote sensing and modeling approach. Agric. For. Meteorology 295, 108187. doi: 10.1016/j.agrformet.2020.108187
van Dijk M., Morley T., Rau M. L., Saghai Y. (2021). A meta-analysis of projected global food demand and population at risk of hunger for the period 2010–2050. Nat. Food 2 (7), 494–501. doi: 10.1038/s43016-021-00322-9
Wan Z., Hook S., Hulley G. (2015). MOD11A2 MODIS/Terra land surface temperature/emissivity 8-day L3 global 1km SIN grid V006. Nasa Eosdis Land Processes Daac 10, 10.5067. doi: 10.5067/MODIS/MOD11A2.006
Wei Y., Tang D., Ding Y., Agoramoorthy G. (2016). Incorporating water consumption into crop water footprint: A case study of China’s South–North Water Diversion Project. Sci. Total Environ. 545, 601–608. doi: 10.1016/j.scitotenv.2015.12.062
Winckler J., Reick C. H., Pongratz J. J. (2017). Robust identification of local biogeophysical effects of land-cover change in a global climate model. J. Climate 30 (3), 1159–1176. doi: 10.1175/JCLI-D-16-0067.1
Windisch M. G., Davin E. L., Seneviratne S. I. (2021). Prioritizing forestation based on biogeochemical and local biogeophysical impacts. Nat. Climate Change 11 (10), 867–871. doi: 10.1038/s41558-021-01161-z
Xin F., Xiao X., Dong J., Zhang G., Zhang Y., Wu X., et al. (2020). Large increases of paddy rice area, gross primary production, and grain production in northeast china during 2000–2017. Sci. Total Environ. 711, 135183. doi: 10.1016/j.scitotenv.2019.135183
Yang Q., Huang X., Tang Q. (2020). Irrigation cooling effect on land surface temperature across China based on satellite observations. Sci. Total Environ. 705, 135984. doi: 10.1016/j.scitotenv.2019.135984
Yu L., Liu T. J. R. S. (2019). The impact of artificial wetland expansion on local temperature in the growing season—The case study of the Sanjiang Plain, China. Remote Sens. 11 (24), 2915. doi: 10.3390/rs11242915
Yuan G., Tang W., Zuo T., Li E., Zhang L., Liu Y. (2022). Impacts of afforestation on land surface temperature in different regions of china. Agric. For. Meteorology 318, 108901. doi: 10.1016/j.agrformet.2022.108901
Zhang W., Jansson C., Miller P. A., Smith B., Samuelsson P. J. B. (2014a). Biogeophysical feedbacks enhance the Arctic terrestrial carbon sink in regional Earth system dynamics. Biogeosciences 11 (19), 5503–5519. doi: 10.5194/bg-11-5503-2014
Zhang X., Liang S., Zhou G., Wu H., Zhao X. (2014b). Generating Global LAnd Surface Satellite incident shortwave radiation and photosynthetically active radiation products from multiple satellite data. Remote Sens. Environ. 152, 318–332. doi: 10.1016/j.rse.2014.07.003
Zhang Z., Lin A., Zhao L., Zhao B. (2022). Attribution of local land surface temperature variations response to irrigation over the North China Plain. Sci. Total Environ. 826, 154104. doi: 10.1016/j.scitotenv.2022.154104
Zhao Y., Fang Y., Cui C. (2012). Effects of irrigation on precipitation in the arid regions of Xinjiang, China. J. Arid Land 4 (2), 132–139. doi: 10.3724/SP.J.1227.2012.00132
Zhu X., Liang S., Pan Y. (2012). Observational evidence of the cooling effect of agricultural irrigation in Jilin, China. Climatic Change 114 (3), 799–811. doi: 10.1007/s10584-012-0435-3
Zhu X., Liang S., Pan Y., Zhang X., Sensing R. (2011). Agricultural irrigation impacts on land surface characteristics detected from satellite data products in Jilin Province, China. IEEE J. Selected Topics Appl. Earth Observations Remote Sens. 4 (3), 721–729. doi: 10.1109/JSTARS.2011.2106152
Keywords: biophysical parameters, water thermal energy balance, regional climate change, land use and cover, geo-detector model
Citation: Li X, Hai Q, Xia K, Vandansambuu B and Bao Y (2023) The biophysical effects of potential changes in irrigated crops on diurnal land surface temperature in Northeast China. Front. Ecol. Evol. 11:1208601. doi: 10.3389/fevo.2023.1208601
Received: 03 May 2023; Accepted: 23 August 2023;
Published: 28 September 2023.
Edited by:
Manoj Kumar, Forest Research Institute (FRI), IndiaReviewed by:
Keshav Tyagi, Forest Research Institute (FRI), IndiaLingxue Yu, Chinese Academy of Sciences (CAS), China
Copyright © 2023 Li, Hai, Xia, Vandansambuu and Bao. This is an open-access article distributed under the terms of the Creative Commons Attribution License (CC BY). The use, distribution or reproduction in other forums is permitted, provided the original author(s) and the copyright owner(s) are credited and that the original publication in this journal is cited, in accordance with accepted academic practice. No use, distribution or reproduction is permitted which does not comply with these terms.
*Correspondence: Quansheng Hai, NjYwNjNAYnR0Yy5lZHUuY24=