Similarity visualization of soundscapes in ecology and music
- 1Institut de Systématique, Évolution, Biodiversité (ISYEB), Muséum national d’Histoire naturelle, CNRS, Sorbonne Université, EPHE, Université des Antilles, Paris, France
- 2Equipe Recherche en Arts, Spectacles et Musique (RASM), Centre d’histoire Culturelle des Sociétés Contemporaines (CHCSC), Université d'Évry Paris-Saclay, Boulevard François Mitterrand, Évry Cedex, France
The concept of a soundscape is found in both ecology and music studies. Nature soundscapes and soundscape compositions are analyzed by both disciplines, respectively, to understand their biological diversity and ecosystem functioning and to interpret their compositional structure. A major challenge for both disciplines is visualizing the information embedded in a large variety of soundscapes and to share it with different audiences, from non-professionals to experts. To analyze soundscapes, both disciplines have independently developed similarity visualizations. However, no attempt has been made yet to combine these two fields of research to improve our ecological and musical perception of environmental sounds through shared similarity analysis methods. In this paper, we introduce a new visualization tool, the soundscape chord diagram (SCD), a circular similarity representation method that can be applied to any type of soundscape, either in ecoacoustics or electroacoustic studies. Our approach consists of visualizing spectral similarities between predefined sound segments based on the computation of a β-diversity acoustic index and on automatic clustering. SCDs were tested on two ecoacoustic forest databases and two electroacoustic soundscape compositions. SCDs were performant for the identification of specific acoustic events and highlighted known diel periods for nature soundscapes and written parts for soundscape compositions. This new visualization tool allows us to easily decipher the structure of musical and ecological acoustic data. SCDs could be applied to a large variety of soundscapes and promote their knowledge and preservation. This study opens a new way of investigating soundscapes at the interface between ecology and music, bringing together science and the arts.
1 Introduction
Listening to and interpreting information encoded in the sounds emanating from ecosystems is central to the survival and welfare of many living organisms. Sound is used, among other reasons, by individuals to find food sources, communicate with congeners, navigate, or avoid predators (Bradbury and Vehrencamp, 2011). Individual acoustic sources generate large sound mixes whose structure and dynamics can reflect local or large-scale ecological patterns and dynamics (Sueur and Farina, 2015). Sound environments are also an important source of inspiration for artists, particularly for composers, who include them in their musical works with different degrees of transformations, from light to deep editing processes (Sueur et al., 2019; Pasoulas, 2020). Following the seminal works of Southworth (1969) and Schafer (1977), the ensemble of sounds emanating from a site at a given time has been defined as a soundscape. The term soundscape has been used in different contexts, including social, acoustic, psychoacoustic, geography, ecological, musical, and artistic studies (Truax, 1999; Westerkamp, 2002; Farina, 2014; Barchiesi et al., 2015; Pasoulas, 2020; Grinfeder et al., 2022b).
The intention to study and preserve soundscapes was largely driven by field recordists, musicians, and electroacoustic composers mostly working in urban, rural, and sometimes natural environments as was the case for the World Soundscape Project at the end of the 1960s and the beginning of the 1970s (Schafer, 1977; Truax, 2008). Electroacoustic is a “music [that] refers to any music in which electricity has had some involvement in sound registration and/or production other than that of simple microphone recording or amplification” (Landy, 1999). In electroacoustics, a soundscape is the recording of the sounds of an environment from the moment there is the will to transmit about a place (Westerkamp, 2002). A soundscape can also be a composition or arrangement of different sound and musical elements (Truax, 1999) referring to a real or transformed cultural, political, social and environmental context (Westerkamp, 2002). A soundscape is also perceived during a sound diffusion through the arrangement of an electronic acoustic space made of different sound sources (Truax, 2012; Mancero Baquerizo, 2019). Musicological research analyzes these soundscapes based on electroacoustic compositional techniques.
From an ecological perspective, a soundscape is a set of sounds that can be analyzed and decomposed to access information about populations, communities, ecosystems, or landscapes states (e.g., Pijanowski et al., 2011). In that case, a soundscape can be decomposed into three categories: the biophony, which includes all biotic sounds, the geophony, which refers to natural but abiotic sounds, and the anthropophony, which groups all sounds produced by human activities (Krause, 1987). Soundscapes are non-fixed processes at the crossroads between perceptions of a situated environment from individual and subjective points of view and objective physical entities. Analytical strategies are developed to understand them and to infer ecological information.
A major challenge of ecoacoustics and electroacoustic studies is visualizing the information embedded in a large variety of soundscapes, from raw recordings in nature areas ranging from a few minutes to several years to sound composition made up of multiple transformations along multiple temporal scales. Visualization should be a powerful tool to easily navigate and analyze audio samples (Towsey et al., 2014; Couprie, 2015; Phillips et al., 2018) and share information in science or education (Couprie, 2015; Couprie, 2018). Ecoacoustics and electroacoustic studies have developed parallel tools for visualizing soundscapes, historically based mainly on the spectrogram. In ecoacoustics, a recent development offers original displays such as long-term spectrograms, false-color spectrograms, sound element polar histograms, and species diel plots (Towsey et al., 2014; Gasc et al., 2018; Phillips et al., 2018). At the same time, electroacoustic studies propose self-similarity matrix (SSM), brightness standard deviation (BStD), and arc diagram solutions (Wattenberg, 2002; Couprie, 2015).
Similarity (resp. dissimilarity) visualization is a solution developed independently by both disciplines but is still rarely explored. Similarity analyses have the advantage of showing the distance between different components and thus revealing structural and temporal information within a sonic environment. In ecoacoustics, this type of analysis mainly relies on dissimilarity measures through the computation of β acoustic indices that have been developed to compare pairs of recordings (Sueur et al., 2014). Beta acoustic indices can be used to identify specific events (Lellouch et al., 2014) and determine spatial and temporal differences at the community or landscape level (Rodriguez et al., 2014; Sueur et al., 2014). In electroacoustics, similarities can be estimated along the timeline of a piece. In that case, similarities can show musical form, structural variations, recurrences, and breaking points, or more simply can guide listening and navigation along the audio file (Couprie, 2015; Couprie, 2022b). Therefore, similarity visualization is a way to highlight structural relationships within a soundscape.
In this paper, we introduce a new visualization, the soundscape chord diagram (SCD), a similarity representation method that can be applied to any type of soundscape, either in ecoacoustics or in electroacoustic studies. The aim of the SCD is to visualize similarities between sound segments on a clock-like layout. The chord diagram is a circular plot already used in genetics (e.g., gene ontology cluster similarities; Xin et al., 2022), economics (e.g., connections of emission transfer between major economies; Deng et al., 2022), migration studies (e.g., human migration between parishes; Gietel-Basten, 2020), and landscape ecology (e.g., percentage of land-use cover changes; Ferrara et al., 2021). To the best of our knowledge, chord diagrams were previously used once for bird sound clustering (Hakim and Mahmood, 2021), but not at the soundscape level, and once in music for visual exploration of song collections, but not for musical analysis (Ono et al., 2015).
The SCD was tested on two ecoacoustic forest databases and two electroacoustic soundscape compositions, representing a wide range of nature and composed soundscapes. The SCD revealed acoustic events and singular elements and highlighted known diel periods for nature soundscapes as studied in ecoacoustics and written parts for soundscape as composed in electroacoustics. This new visualization tool therefore appears to be a performant tool in contrasted acoustic contexts building an original bridge between distant but still interconnected disciplines.
2 Materials and methods
2.1 Ecoacoustic case studies
The soundscapes of two protected forest ecosystems were recorded and analyzed, namely a tropical forest in the Réserve naturelle des Nouragues in French Guiana and an alpine forest in the Parc Naturel Régional du Haut-Jura in the East of France mainland. These two forests are acoustically monitored to assess long-term climate change effects in two different biogeographical and climate contexts.
The Nouragues tropical forest is a lowland tropical forest located in the center of French Guiana (4°05′N, 52°41′W). The forest is part of a nature reserve, which includes the CNRS Nouragues Research Station. The climate is equatorial. The mean temperature is 26.3°C with a weak thermal amplitude of 2°C and a mean annual rainfall of 2,861 mm (average amount of rainfall from 1992 to 2012) (Ulloa et al., 2018). The annual weather cycle is composed of two main seasons, a dry season from September to December and a rainy season from January to June. A secondary short dry season occurs in March within the rainy season (Grimaldi and Riéra, 2001). Sunrise varies between 06:24 am and 06:38 am and sunset varies between 06:28 pm and 06:46 pm. The forest is a dense evergreen forest with a high density of trees, usually reaching a height of 30–45 m (Rodriguez et al., 2014). The local biodiversity is particularly high (Bongers et al., 2001), including 749 bird species (Birdlife International, 2023). The native occupants of the site, the Noraks, disappeared during the eighteenth century (Charles-Dominique, 2001). Anthropic pressure is particularly low; the site currently inhabited only at the CNRS research station, and the nearest village (Regina) is 60 km away from the station (Rodriguez et al., 2014).
Risoux Forest is a temperate cold climax forest located in the French Jura Mountains close to Switzerland. The climate is continental. The mean temperature is 5.5°C with important snowfalls (> 2.50 m). The annual weather cycle is composed of four main seasons characterized by an extended winter period from December to April. The forest is characterized by mid-mountain vegetation dominated by the European spruce (Picea abies). The forest is inhabited by a rich animal diversity, including key species such as the European lynx (Lynx lynx), the Grey wolf (Canis lupus), and the Western Capercaillie (Tetrao urogallus). Risoux Forest is crossed by 26 km of roads, hiking and cross-country skiing trails, and is overflown by aircraft corridors. Aircraft noise is present 75% of the time in the soundscape of this protected forest habitat (Grinfeder et al., 2022a). This strong human presence is accentuated by hunting and commercial logging.
The soundscapes of these two contrasted forests were obtained using autonomous recording units (Songmeter SM4, Wildlife Acoustics Inc., Concord, MA, USA), each equipped with two omnidirectional microphones. In the Nouragues forest, a single recorder (4°2′N; 52°40′W) was attached to a tree at a height of 1.50 m so that the built-in left channel recorded the understory, and the right channel was positioned at a height of 40 m to record the canopy. In Risoux Forest, four recorders were installed at heights of 2.50 m. In both forests, the recorders were programmed to record every 15 min for 1 min at each site (1’ on, 14’ off). Audio recordings were saved under uncompressed .wav format with a 44.1 kHz sampling frequency, 16-bit depth, and 4 dB gain for the Nouragues forest and 16 dB gain for the Risoux forest. These recordings were part of long-term ecoacoustic programs that started in February 2019 in the Nouragues forest and in July 2018 in the Risoux forest. A complete year of recordings was selected for each site, from February 20, 2019 at 00:00 am to the February 19, 2020 at 11:45 pm. For the Risoux forest, one of the four recorders was selected (46°32′N; 6°06′E). The resulting audio database included 38,728 audio files for a total of 645 hours of recording for the Nouragues forest and 35,040 audio files for a total of 584 hours of recording for the Risoux soundscape. The difference in the sampling effort was due to differences in equipment failure.
2.2 Electroacoustic case studies
Electroacoustic music analyses were carried out on two masterpieces which differ in compositional methods (e.g., with or without editing, mixing, or sound transformations) and types of sound materials (e.g., processed or unprocessed recordings, synthesized sounds). These two musical pieces are representative of electroacoustic soundscape compositions.
“Presque Rien n°1 ou Le lever du jour au bord de la mer’’ was composed by Luc Ferrari in 1967–1970. Luc Ferrari was a French composer (1929–2005) who was a pioneer in electroacoustic music. He was one of the first to compose “anecdotal compositions”, which is “music that employs recognisable sounds more for their “anecdotic” or narrative aspect than for their abstract potential” (Caesar, 1992). “Presque Rien n°1” is a soundscape composition work, which attempts to reconstitute as realistically as possible a summer sunrise in Vela Luka fishing village (42°57’N 16°43’E) of the Adriatic Sea (former Yugoslavia, now in Croatia). In this composition, Ferrari mixed sea sounds, cricket and cicada choruses, domestic animal vocalizations, human voices, and other anthropic sounds such as boat engines. This musical piece is part of the cycle “Presque Rien”, a term that refers to a “homogeneous and natural place, not urban, which has particular acoustic qualities (transparency and depth), where one can hear far and near without excess, on the scale of the ear as we say on a human scale, without technology, where nothing is dominant so that the different sound inhabitants each have their say and the superimposition of this small world of life never becomes more than a mere nothing” (Caux, 2002). Recordings and editing were made on magnetic stereo tape. The microphones were placed on the windowsill of the house where Luc Ferrari lived, turned towards the sea. Sound recordings were made between 04:00 am and 06:00 am in the summer of 1967. From this large quantity of recordings, a selection of sound materials and a manual editing (cutting, splicing) was made to compose a continuous soundscape. A digital version was obtained in 1995 with three tracks lasting 06’50’’, 09’20’’, and 04’41’’ (Ferrari, 1995). We worked on a .wav file (20’54’’) obtained by rejoining the three tracks in their original order with the digital audio workstation Reaper (release 6.46).
“Sud” was composed by Jean-Claude Risset in 1984–85, commissioned by the French Ministry of Culture. Jean-Claude Risset was a French composer (1938–2016) whose work was based on his scientific and musical training. He was a leader in computer music, especially in synthesis techniques. “Sud” is a hybrid work on a seaside soundscape, at the interface between phonography, synthetic music, realism, and imagination. This piece evolves over three movements where natural, instrumental, and synthetic sounds interact and merge into each other, creating sound chimeras. Sound recordings were made in the Massif des Calanques, next to Marseille (France), and a digital synthesis was carried out in Marseille, partly with the computer program MUSIC V (Ina-GRM/LMA, CNRS Marseille). These sounds were processed (e.g., modulation, reverb, spatialization) and mixed in the GRM studios. A digital version (Risset, 1988) contains the three movements (Sud 1 or La mer le matin - 09’51’’, Sud 2 or Appel - 05’49’’, and Sud 3 or Le profil de la mer - 08’15’’). We worked on a single file (23’57’’) in .wav format obtained by assembling the three parts with the digital audio workstation Reaper (release 6.46).
2.3 Frequency filtering
In Risoux Forest, geophony (wind and rain) and antropophony (aircraft only) are the main components of the soundscape (Grinfeder et al., 2022a). In this special case, to study the structure of the biophony, it was necessary to reduce noise due to anthropophony and geophony. Processing was therefore applied to the files to reduce pseudo-stationary noise, such as wind, heavy rain or aircraft, and to preserve the non-stationary sound events in the soundscape (e.g., birdsong, crackling, woodpecker percussion, water drops). A two-step procedure was applied: (1) a low-cut frequency filter (0–500 Hz) with a 16th order digital butterworth filter from the Python package scipy, and (2) a log minimum mean square error (log-MMSE) algorithm (Ephraim and Malah, 1984) implemented in the Python package logmmse 1.5 to remove uncorrelated additive noise. The parameters of the log-MMSE were 9 for the initial noise, 4,096 samples for the window size, and 0.9 for the noise threshold.
2.4 Signal analysis
For natural soundscapes, one minute per hour was selected so that the temporal sampling was reduced but preserved. Working only on these sound segments preserves the temporal sampling while reducing the large size of the database. For composed soundscapes, the audio files were divided into contiguous segments so that the temporal organization of the compositions was fully conserved. In this study, “Presque Rien n°1” was divided into one-minute segments. “Sud” was divided into 30-second segments because this composition presents rapid variations. The mean frequency spectrum of each segment was obtained by computing a short-term frequency Fourier transform (STFT) made of 256 samples tapered with a Hanning window and no-overlap between successive analysis windows.
2.5 Similarity links
Acoustic similarity between all pairwise segments was assessed using the 1-Dcf index based on cumulative frequency dissimilarity (Lellouch et al., 2014). The index was bounded between 0 and 1, a value of 0 indicating a total absence of similarity and an index of 1 a perfect similarity. The index was computed to obtain a similarity matrix for each dataset using the R package seewave (Sueur et al., 2008a). Then, the similarity matrix dataset was normalized between 0 and 1. When comparing several matrices to each other, the similarity data were scaled according to the highest value of all the matrices. The strongest links between audio segments were selected by applying a threshold to the similarity index. This threshold was heuristically adapted according to each dataset to maximize readability.
2.6 Clustering
To highlight a temporal structure in the datasets, that is, to identify parts in both natural or composed soundscapes, segments were clustered based on the mean frequency spectra with a Hierarchical Clustering Analysis (HCA). HCA is a clustering method that requires specifying the k number of clusters to be found. As we had no a priori information on the numbers of clusters occurring in each dataset, the number of clusters was determined by running the HCA with k > 1. For each partition, the within-cluster inertia was calculated. The best partition was the one with the highest relative loss of inertia between the partition with the k cluster and the partition with (k+1) clusters. The clusters were determined using the HCPC function of the R package FactoMineR (Lê et al., 2008).
2.7 Visualization
Single and multiple chord diagrams were used to circularly display the similarity between audio segments through links. Segments were organized around a circle as circular arcs in a clockwise direction. The length of each circular arc was proportional to the importance of the total similarity with the other segments. Except for multiple chord diagram comparisons, the sizes of the circular arcs were normalized to the maximum similarity of all the days. Spectral similarity (1-Dcf) was represented by the width of the links that connected the segments to each other. The clusters as defined by the HCA were grouped under the same color. For the multiple chord diagram representations, the colors displayed correspond to the HCA calculated on all the mean frequency spectra of selected days. Chord diagrams were obtained using the R package circlize (Gu et al., 2014). The complete process is summarized in Figure 1.
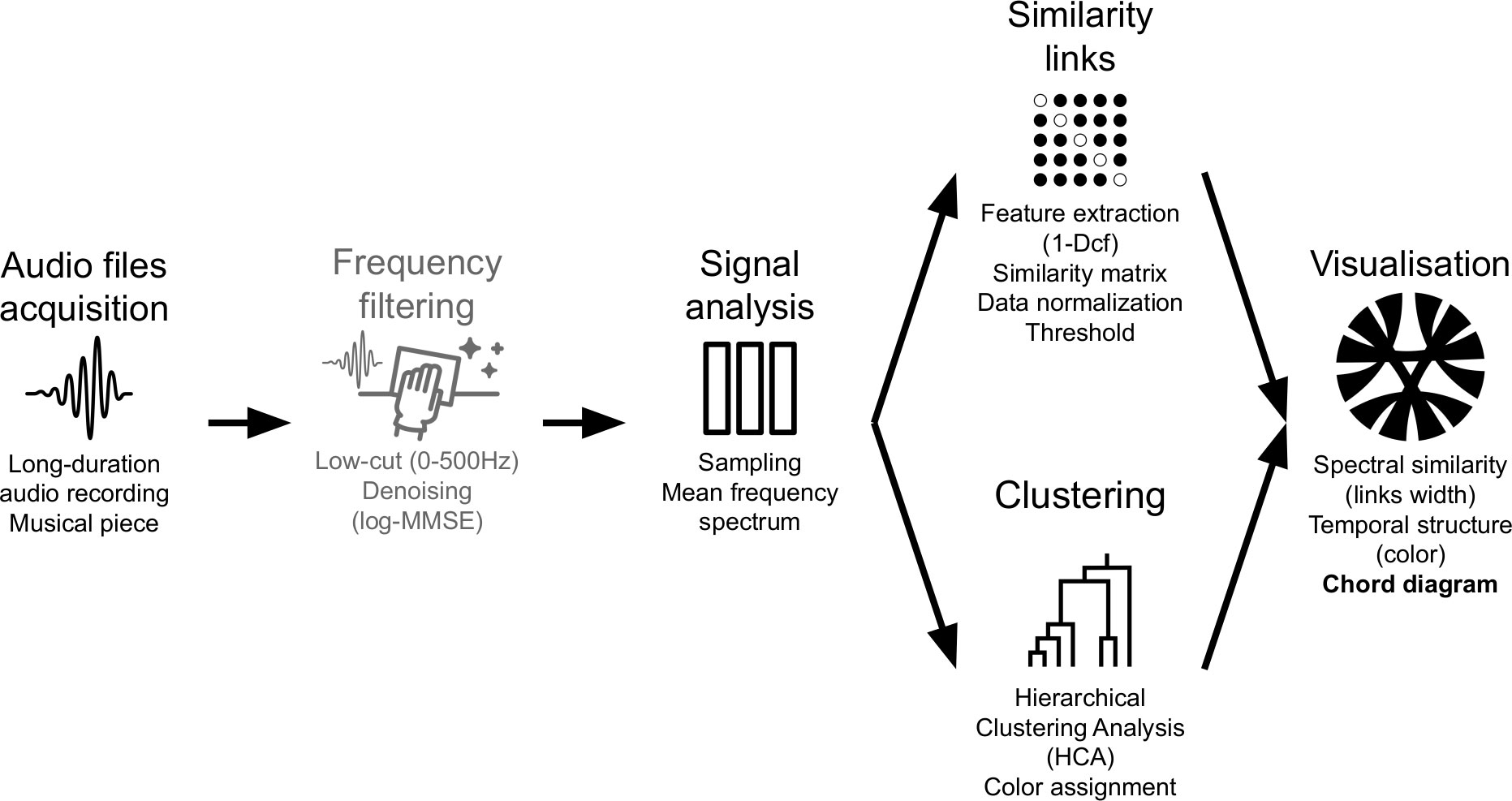
Figure 1 Soundscape chord diagram workflow. From recording to visualization, the process goes through frequency filtering (optional), mean frequency spectrum computation, spectral similarity estimation through a distance calculation, and hierarchical clustering analysis.
3 Results
3.1 Ecoacoustic case studies
Nouragues Forest. As an example, the SCD analysis applied to a single day (09/18/2019) revealed a structure divided into three groups (HCA k = 3), corresponding to three successive time periods: (1) a long night and dawn period from 00:00 am to 08:00 am (green color), (2) a diurnal period from 09:00 am to 06:00 pm (yellow color), and a night period from 07:00 pm to 11:00 pm (red color) (Figure 2). The diurnal period, dominated by stridulating insects in the 7–9 kHz frequency band, was particularly well defined, showing rare similarity links with the other two night periods. In contrast, the two night periods shared some similarities. Over the complete year of recording, the HCA revealed three general clusters. The two largest clusters (green and yellow colors) were relatively homogeneous throughout the year (Figure 3). They corresponded to (1) a day period from 09:00 am/10:00 am to 18:00 pm/19:00 pm and (2) a night period from 19:00 pm/20:00 pm to 08:00 am/09:00 am. On many days, there were regular similarity links between the nocturnal and diurnal groups, around 08:00 am to 10:00 am recordings revealing a transition period between nocturnal and diurnal sounds. The third cluster (red color), smaller in size and present in 57% of the SCD, corresponded to heavy rain revealing a rainy period in May and December, and a dry period in September. A chord diagram computed at 06:00 pm, one day per week, over the entire year, revealed three clusters with two of them corresponding to heavy rain events (blue color) and the chorus of the leaf litter frog Adenomera andrea (orange color) from November 13, 2019 to February 12, 2020 (Figure 4). The segments that compose each of the clusters share similarity links almost exclusively between segments of the same cluster. This underlines the strong acoustic identity of each of the sound events.
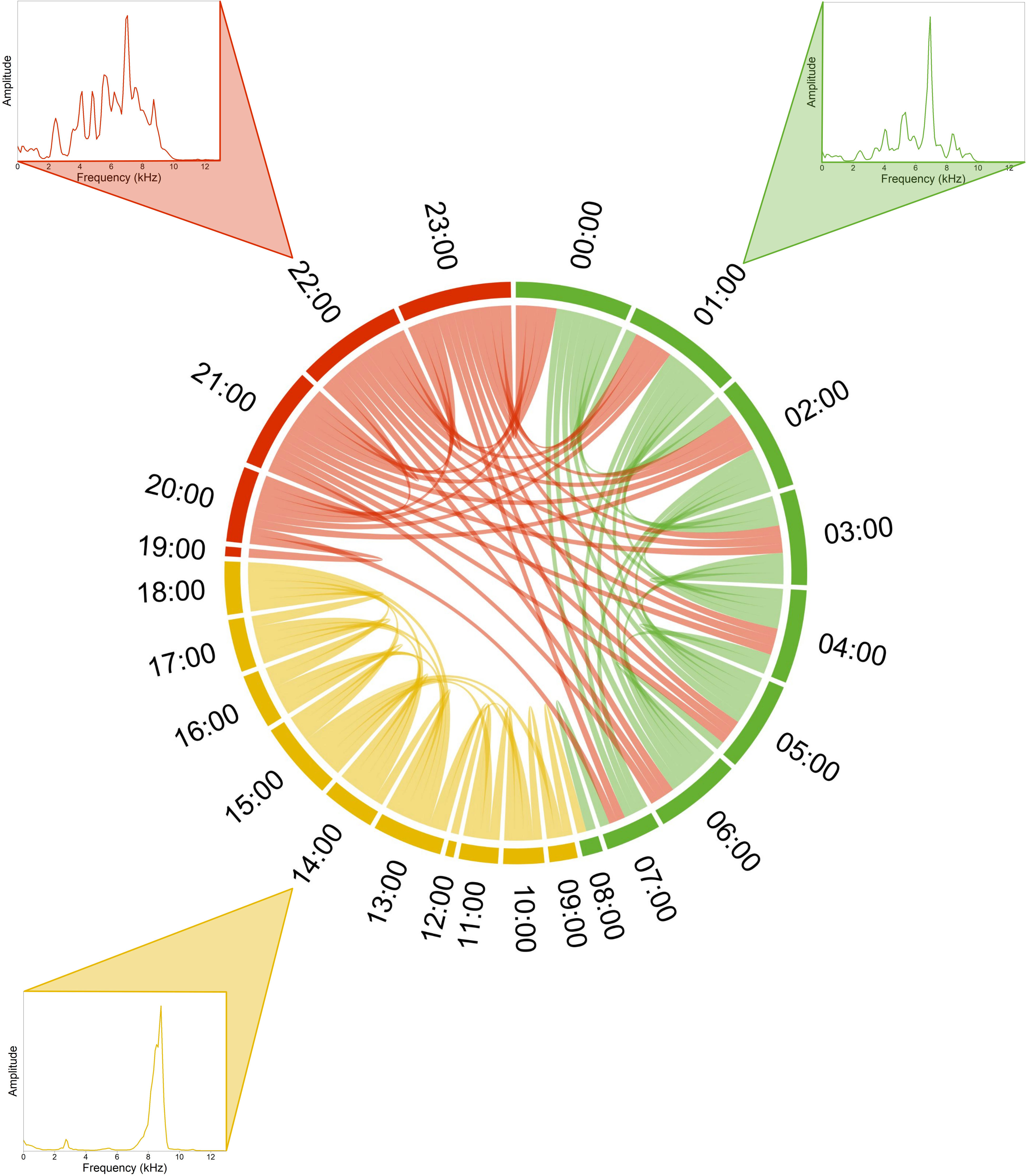
Figure 2 Soundscape chord diagram of a complete recording day in the Nouragues tropical forest. The SCD shows the acoustic similarity within a day during the dry season (September 18, 2019). Midnight (00h00) is positioned in the North direction (0°) and hours run in a clockwise manner. The size of circular arcs and the number of links between recordings (1 min/hour) are proportional to spectral similarity computed with the spectral index (1-Dcf). Links with a similarity below 0.7 are not shown. Colors correspond to the three clusters automatically found by the hierarchical clustering analysis (HCA). Representative mean frequency spectra illustrate each cluster, namely at 22:00 for the first part of the night (red cluster), 01:00 for the second part of the night (green cluster), and at 14:00 for the day (yellow cluster).
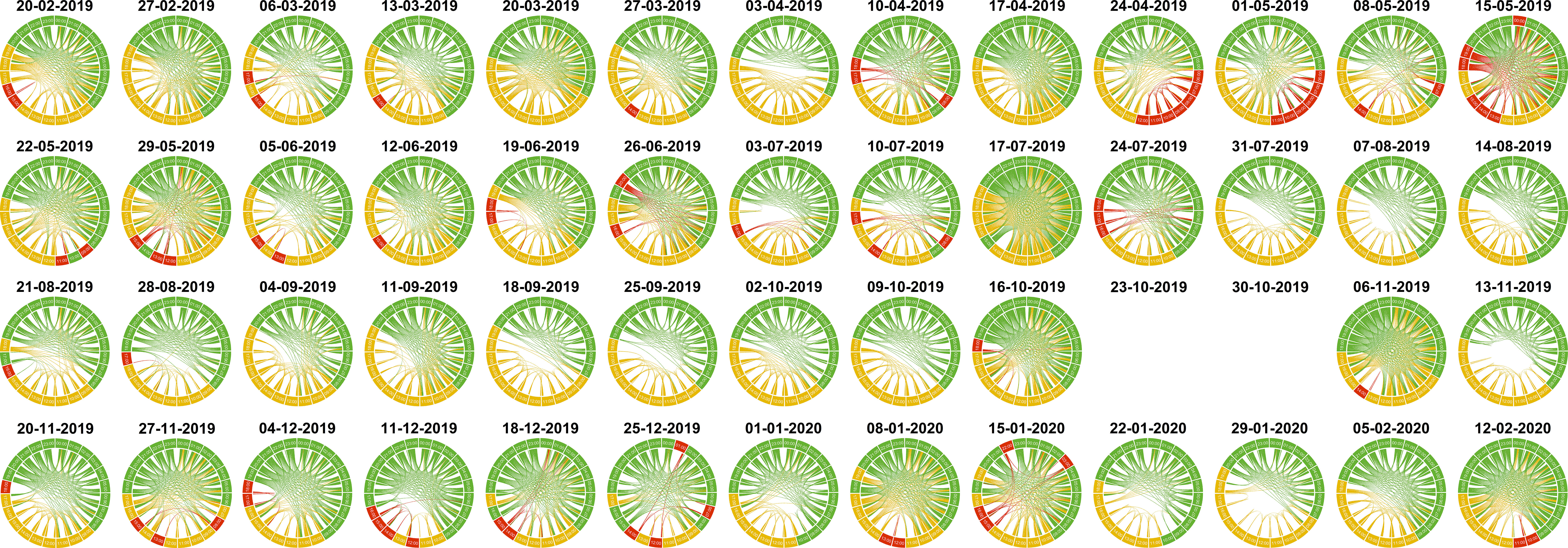
Figure 3 Soundscape chord diagrams for a complete recording year in the Nouragues tropical forest. A SCD was computed every seven days from February 20, 2019 to February 19, 2020. Midnight (00h00) is positioned in the North direction (0°), hours run in a clockwise manner, and days run from left to right and up to down. Size of each circular arc has been normalized to the maximum similarity importance of all the days. Links with a similarity below 0.7 are not shown. Colors correspond to the three clusters automatically found by the hierarchical clustering analysis (HCA) applied to the complete dataset, so that the colors of all SCD are homologous. The green color corresponds to the night cluster, yellow color to the diurnal cluster, and red color to the heavy rain cluster. October 23, 2019 and October 30, 2019 are missing due to recording failures.
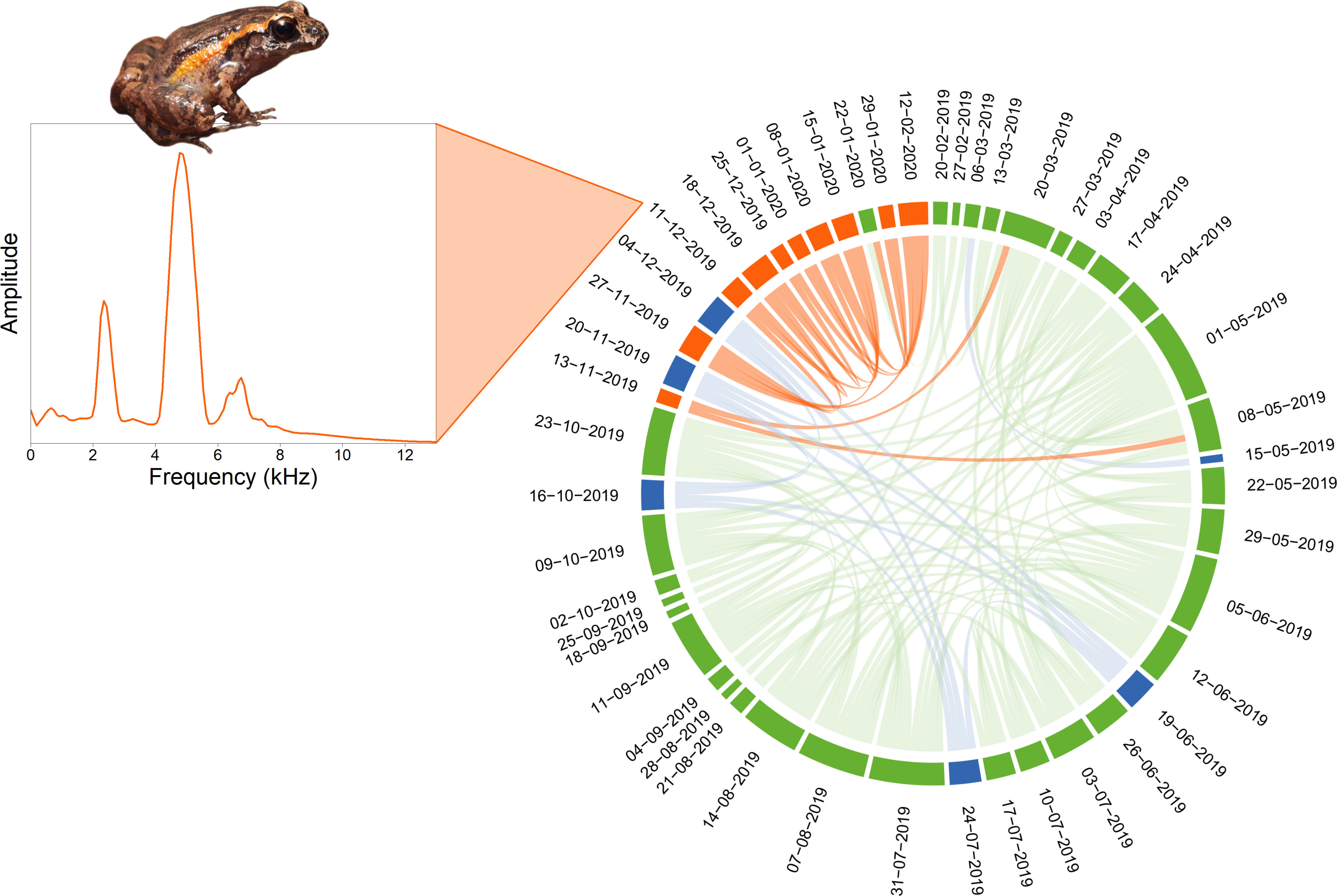
Figure 4 Soundscape chord diagram for a complete recording year at 18:00 in the Nouragues tropical forest. The SCD shows the acoustic similarity between days at the same hour, every seven days for a year from February 20, 2019 to February 19, 2020. The first day of recording is positioned in the North direction (0°) and days run in a clockwise manner. The size of circular arcs and the number of links between recordings (1 min/hour) are proportional to spectral similarity computed with the spectral index (1-Dcf). Links with a similarity below 0.9 are not shown. Colors correspond to the three clusters automatically found by the hierarchical clustering analysis (HCA). The mean frequency spectrum of the December 11, 2019 recording is shown to illustrate the orange cluster corresponding to the chorus of the litter frog Adenomera andrea that dominates the soundscape during the rainy season, from mid-November to mid-February. The blue color corresponds to the heavy rain cluster. Picture by Quentin Martinez.
Risoux Forest. SCD analysis carried out on a single day (06/25/2019) revealed a structure divided into three groups (k = 3), corresponding to three successive time periods: (1) a night period from 11:00 pm to 04:00 am (blue color), (2) a day period mostly from 09:00 am to 06:00 pm (yellow color), and (3) dawn and dusk periods from 06:00 am to 08:00 am and from 07:00 pm to 10:00 pm (orange color) (Figure 5). The night period, which was particularly silent, was specifically well defined, showing rare similarities with other times of the day. In contrast, the day time periods and dawn-dusk periods shared strong similarities. When exploring the year pattern with one SCD generated every seven days, three clusters emerged: (1) a very quiet soundscape (yellow color), (2) a soundscape dominated by the biophony and other sounds (e.g., leaf rubbing, chainsaw) (blue color), and (3) heavy rain (orange color) (Figure 6). However, no general consistent pattern could be observed over the year, except the highlight of sudden events such as heavy rains (e.g., 11/06/2019). A chord diagram computed at 06:00 am, one day per week, over the entire year (Figure 7), revealed an overall strong similarity between all audio files except for one period from April 10, 2019 to July 17, 2019, due to a spring dawn chorus with a frequency peak at 3–4 kHz (e.g., 06/05/2019), corresponding to the frequency range of local bird songs.
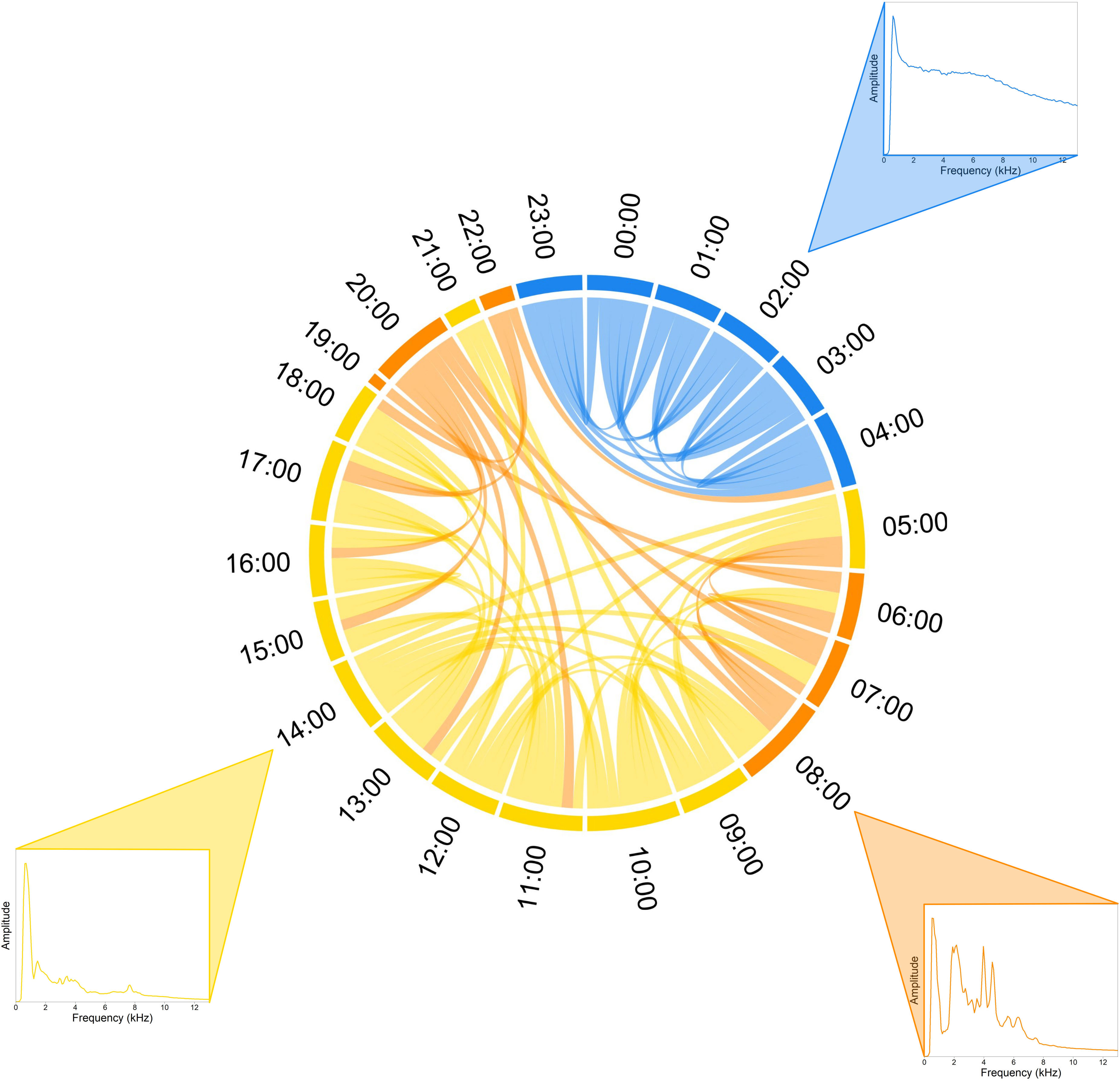
Figure 5 Soundscape chord diagram of a complete recording day in the Risoux temperate forest. The SCD shows the acoustic similarity within a day during spring (June 25, 2019). Midnight (00h00) is positioned in the North direction (0°) and hours run in a clockwise manner. The sizes of circular arcs and the numbers of links between recordings (1 min/hour) are proportional to spectral similarity computed with the spectral index (1-Dcf). Links with a similarity below 0.8 are not shown. Colors correspond to the three clusters automatically found by the hierarchical clustering analysis (HCA). Representative mean frequency spectra illustrate each cluster, namely at 02:00 for the night (blue cluster), 08:00 for the dawn and dusk choruses (orange cluster), and at 14:00 for the day (yellow cluster).
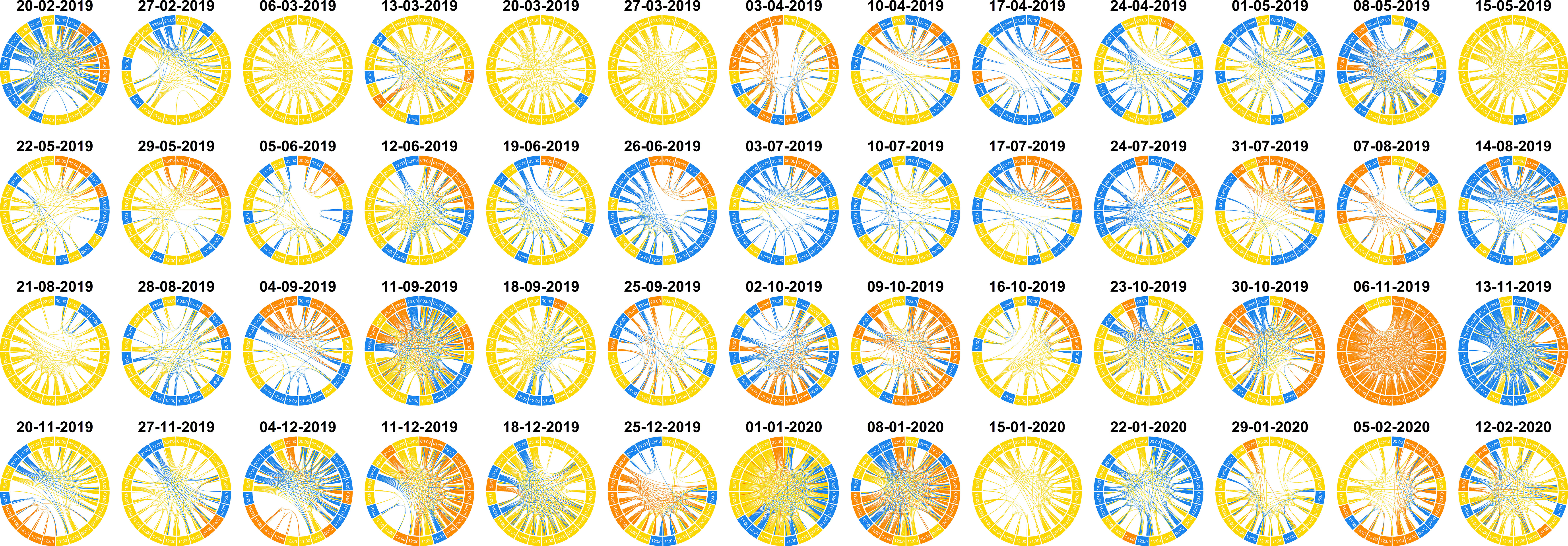
Figure 6 Soundscape chord diagrams for a complete recording year in the Risoux temperate forest. A SCD was computed every seven days from February 20, 2019 to February 19, 2020. Midnight (00h00) is positioned in the North direction (0°), hours run in a clockwise manner, and days run from left to right and up to down. Recordings were processed with a low-cut frequency filter (0-500Hz) and denoising (log-MMSE) procedures. The size of each circular arc has been normalized to the maximum similarity importance of all the days. Links with a similarity below 0.8 are not shown. Colors correspond to the three clusters automatically found by the hierarchical clustering analysis (HCA) applied to the complete dataset, so that the colors of all SCDs are homologous. Yellow color corresponds to a very quiet soundscape, blue color corresponds to a soundscape dominated by biophony and other sounds (e.g., leaf rubbing, chainsaw), and orange color corresponds to heavy rain.
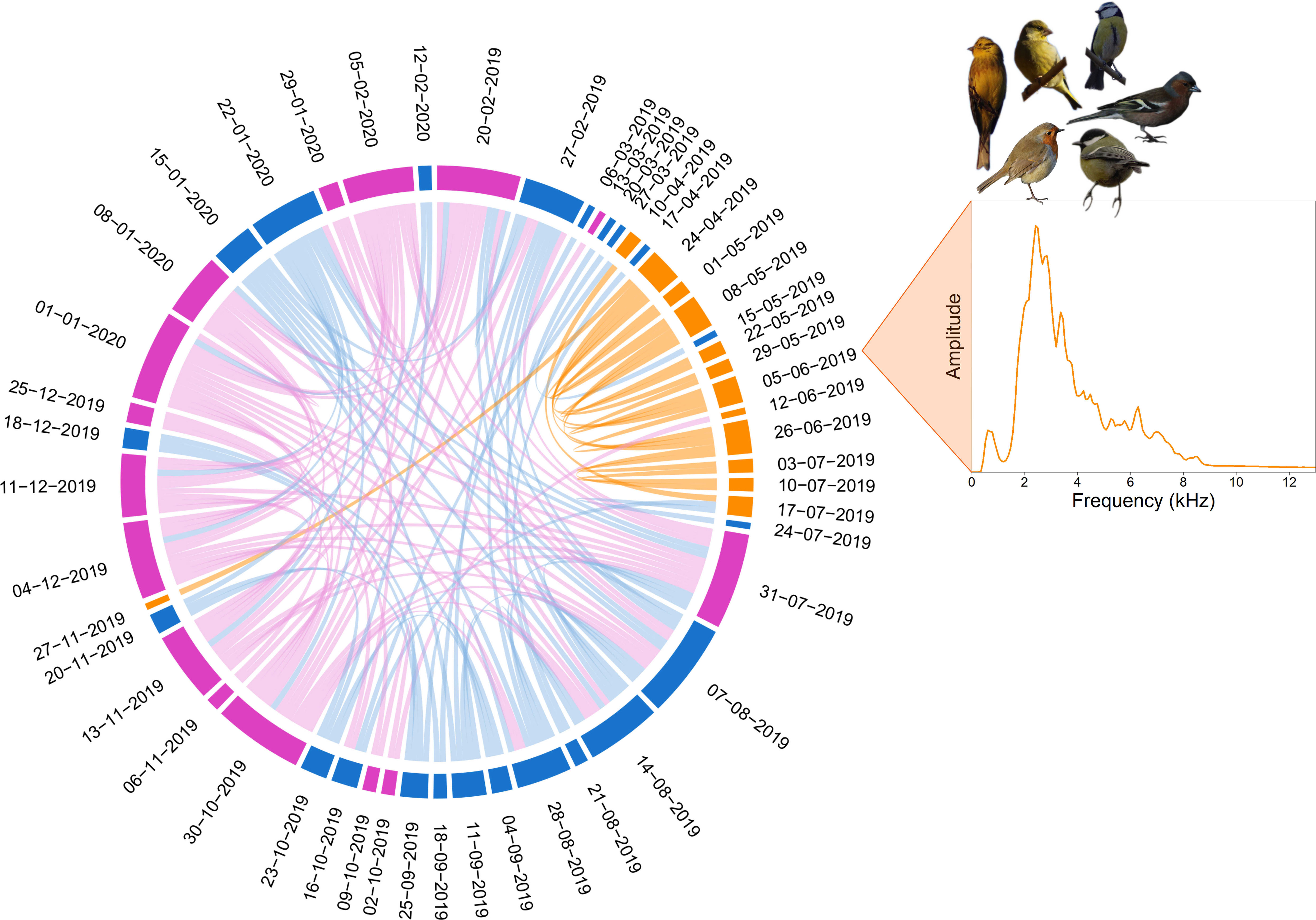
Figure 7 Soundscape chord diagram for a complete recording year at 06:00 in the Risoux temperate forest. The SCD shows the acoustic similarity between days at the same hour, every seven days for a year from February 20, 2019 to February 19, 2020. The first day of recording is positioned in the North direction (0°) and days run in a clockwise manner. The sizes of circular arcs and the numbers of links between recordings (1 min/hour) are proportional to spectral similarity computed with the spectral index (1-Dcf). Links with a similarity below 0.9 are not shown. Colors correspond to the three clusters automatically found by the hierarchical clustering analysis (HCA). The mean frequency spectrum of the June 5, 2019 recording illustrates an orange cluster corresponding to the bird dawn chorus that dominates the soundscape during spring, from mid-April to mid-July. Pictures by Maxime Le Cesne.
3.2 Electroacoustic case studies
“Presque rien n°1” by Ferrari. The SCD computed on “Presque Rien n°1” (Figure 8) revealed three sections: (1) section one from 00’00’’ to 08’00’’ (yellow color), (2) section two from 08’00’’ to 16’00’’ (red color), and (3) section three from 16’00’’ to 20’00’’ (purple color). These three sections matched with the original sections designed by Luc Ferrari: (1) part one from 00’00’’ to 06’50’’, (2) part two from 06’50’’ to 16’10’’, (3) part three from 16’10’’ to 20’51’’. The only difference between the structure proposed by the SCD and the editing work was related to the segment 07’00’’ to 08’00’’, associated to part one in the chord diagram and to part two in Luc Ferrari montage. This segment corresponded to a transition between two scenes. The first part sets the scene of the fishing village with a succession of sounds mainly of anthropic origin (e.g., human voices, domestic animals voices, motors). The second part is characterized by human voices and songs, almost continuous engines, and the regular but light presence of cicadas. In the third part, engines disappear but cicadas gradually increase and invade the soundscape. The few human voices give way to them. Listening to the piece gives the feeling of progressive and slow changes but the chord diagram reveals clear-cut and intentional changes in the sound material supporting the three-part structure. The first two parts had differentiated sound signatures but also shared quite strong similarities, probably linked to the use of the same anthropophonic materials. The last part had a more particular sound signature, mainly due to the strong presence of cicadas of the species Cicada orni. This common Mediterranean species produces a calling song characterized by a dominant frequency at 4.5 kHz, which makes the third part very distinctive (Sueur et al., 2008b).
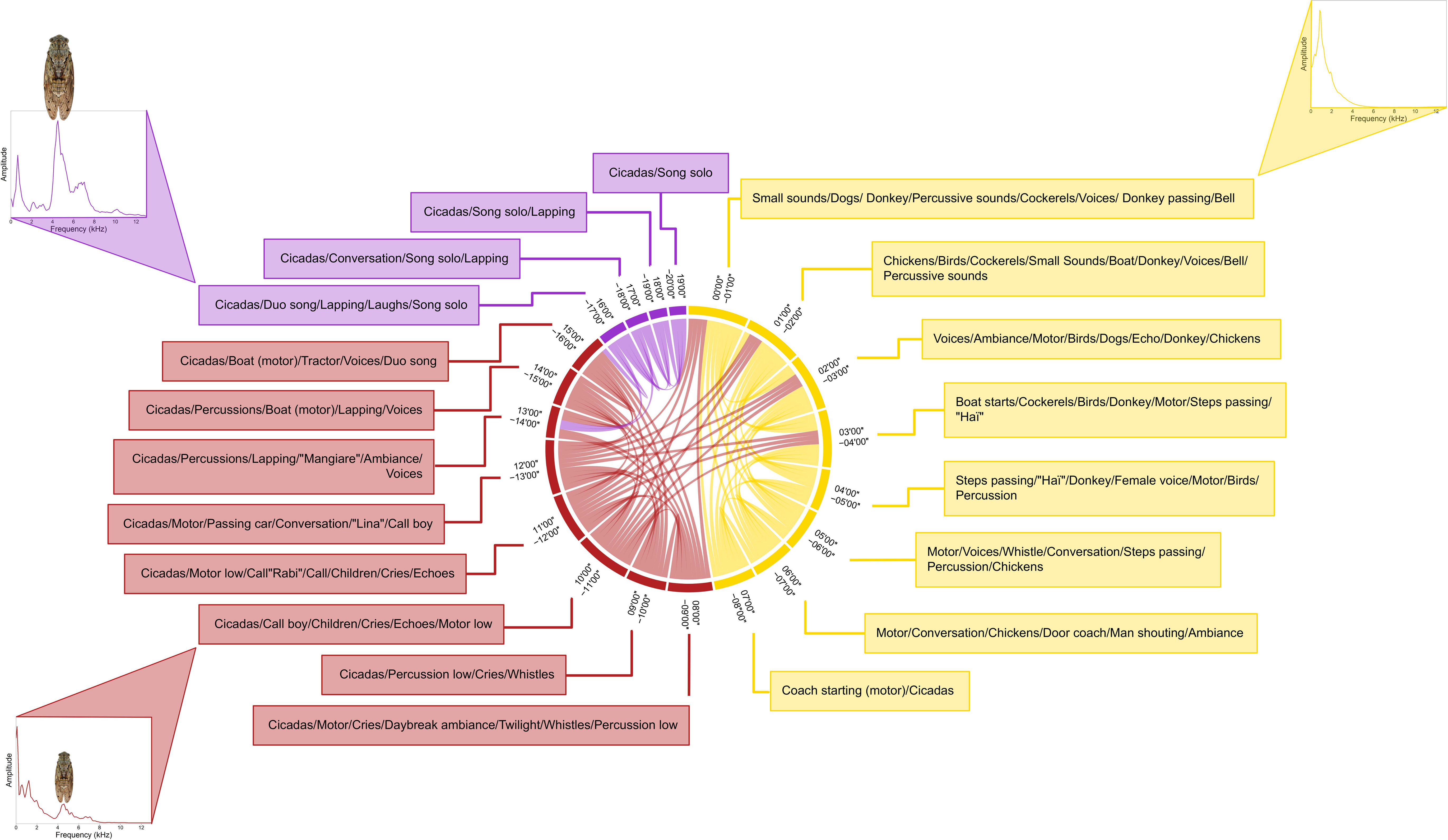
Figure 8 Soundscape chord diagram for the electroacoustic piece Presque Rien n°1 by Luc Ferrari. The SCD shows the acoustic similarity within the soundscape composition. The beginning is positioned in the North direction (0°) and time runs in a clockwise manner for a total duration of 20’54’’. The sizes of circular arcs and the numbers of links between successive 1’ segments are proportional to spectral similarity computed with the spectral index (1-Dcf). Links with a similarity below 0.85 are not shown. Colors correspond to the three clusters automatically found by the hierarchical clustering analysis (HCA). These clusters correspond to the composer’s editing plan as shown by text boxes around the circle. Representative mean frequency spectra illustrate each cluster, namely at the 00’ to 01’ segment (yellow cluster), 10’ to 11’ segment (red cluster), and at 16’ to 17’ segment (purple cluster). The frequency peak due to the sound activity of the Mediterranean cicada Cicada orni is highlighted in the two last clusters (red and purple clusters). Picture by Jérôme Sueur.
“Sud” by Risset. The SCD analysis carried out on Sud by Jean-Claude Risset could not reveal a particular structure as the time sections were almost all connected to each other (Figure 9). There was, however, one cluster that was well defined, which indicated the repetition of a specific element, a carillon (Figure 9, purple cluster).
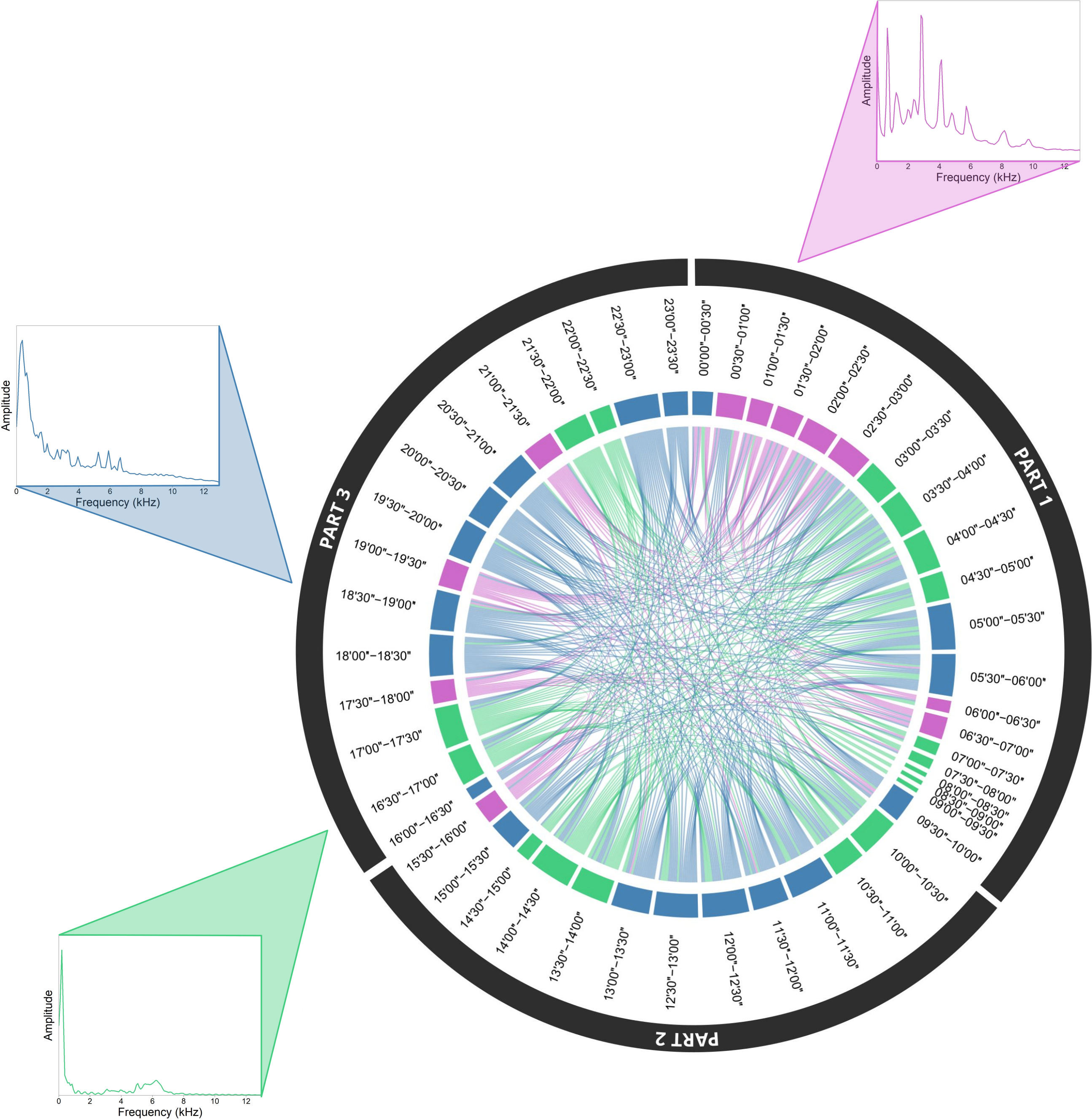
Figure 9 Soundscape chord diagram for the electroacoustic piece Sud by Jean-Claude Risset. The SCD shows the acoustic similarity within the soundscape composition. The beginning is positioned in the North direction (0°) and time runs in a clockwise manner for a total duration of 23’57’’. The sizes of circular arcs and the numbers of links between successive 30’’ segments are proportional to spectral similarity computed with the spectral index (1-Dcf). Links with a similarity below 0.85 are not shown. Colors correspond to the three clusters automatically found by the hierarchical clustering analysis (HCA). The composer’s editing plan is shown by black arc circles around the circle. Representative mean frequency spectra illustrate each cluster, namely at the 01’00’’ to 01’30’’ segment (pink cluster), 16’30’’ to 17’00’’ segment (green cluster), and at 18’30’’ to 19’00’’ segment (blue cluster).
4 Discussion
We designed a visualization tool for ecologists and musicologists so that global spectral information on a variety of soundscapes, both natural and composed, can be obtained and shared with a large audience. This visual solution can improve the knowledge on the acoustic structure, ecological function, and social perception of soundscapes so they can be better preserved (Dumyahn and Pijanowski, 2011). Sound visualization is a central theme of research in both ecology and musicology. The main challenge in ecoacoustics is to summarize in a single plot long-term programs including thousands of recording hours (Towsey et al., 2014; Phillips et al., 2018; Towsey et al., 2018; Metcalf et al., 2023). Several visualization techniques have already been proposed, particularly false-color spectrograms, which can reveal peculiar events or detect species by combining the visualization of three acoustic indices (Towsey et al., 2018) and polar histograms or diel plots based on clustering techniques (Phillips et al., 2018). Even if the spectrogram is widely used for music analysis, musicology offers, among others, the self-similarity matrix (SSM), a 2-dimensional similarity representation to visualize the time structure and highlight acoustic singularities (Foote and Cooper, 2001; Couprie, 2015; Couprie, 2022a). The brightness standard deviation (BStD) combines in a single waveform three audio descriptors so timbre dynamics can be assessed (Malt and Jourdan, 2015). Eventually, the arc diagrams link repeated series of notes with arcs along a single linear axis (Wattenberg, 2002). All these solutions have been mainly applied to classical and popular styles but less to electroacoustic music and even more rarely to soundscape compositions. Here, the SCD offers a new complementary technique focused on frequency spectrum similarity, a rarely explored approach.
Whether applied to short- or long-term audio, SCD returns specific time-limited events at the same time as global image of soundscape. In ecoacoustics, the SCD could show the main day periods in both tropical and temperate forests and highlight time-limited events such as tropical frog choruses, temperate bird dawn choruses, or tropical heavy rains. In music, the SCD could automatically find sections as described in the original editing scheme of the composer and reveal the occurrence of similar sound elements. The SCD can also be applied at different scales of observations. In ecoacoustics, the most intuitive method is a 24-hour recording following a clock design, but also several successive days (several SCD) or several days on a single SCD at the time of recording. The SCD was applied here to middle duration pieces (~ 25’), but it could be tested on longer pieces or to a complete chorus so that the structure of a composer’s work could be estimated.
These preliminary tests of heterogeneous data confirmed the potential of the SCD for finding structures in both ecoacoustic recordings and musical pieces. Built on frequency similarity, the SCD was, however, less relevant for ecoacoustic recordings containing continuous noise such as wind or anthropophony or for music pieces with unstructured spectral content. The SCD can also fail to identify brief sections that only differ in their time and/or energy properties. The SCD therefore seems to be more appropriate for slowly evolving and spectrally well-organized soundscapes. In addition, the time sampling linked to the duration of the segments determines the spectral and temporal information that is visualized and thus, the emerging structure. It is therefore important to adapt the size of the segments to each soundscape and to each type of information to be visualized.
Alpha acoustic indices, assessing the within-recording diversity, have been thoroughly used in ecoacoustics, while β indices, i.e., the between-recording similarity, has been neglected (Sueur et al., 2014; Buxton et al., 2018; Bradfer-Lawrence et al., 2019; Alcocer et al., 2022). The 1-Dcf acoustic index, which has the advantage of reducing a bias towards high values compared to other similarity indices (Lellouch et al., 2014), proved to be informative not only for ecoacoustic recordings as it was designed for but also for musical pieces. To the best of our knowledge, this is the first attempt to transfer analytical tools from ecological sciences to musicology. The reverse process would imply the use of audio descriptors extensively used in music, such as inharmonicity, zero crossing rate (ZCR) or mel-frequency cepstral coefficients (MFCCs) (Couprie, 2022a; Couprie, 2022b; Lerch, 2023), on ecoacoustic recordings, reinforcing the art and science bridging.
The SCD follows a successive step process. Each step can be changed or tuned so that the final graphical output can be optimized for specific needs. For instance, the raw recordings of the Risoux temperate forest were filtered with a butterworth filter and the log-MMSE algorithm, but any other filtering processes could be applied. Then, the mean spectrum was computed but other spectrum summaries could also have been computed, such as the median spectrum. Moreover, other frequency representations, such as the mel frequency spectrum, could be calculated. In a similar way, both similarity distance and clustering method could be replaced by other distances and methods, respectively, if they prove to be more appropriate.
The SCD is a circular representation that supposes a cyclic structure in the recordings analyzed. In ecoacoustics, such cyclicity agrees with diel and annual cycles but is more questionable for musical pieces. The SCD can clearly show some compositional techniques, as in Sud, where all the parts of the piece, from beginning to end, are spectrally linked. However, to give a cyclical image of a music piece that has been constructed in a linear way can give a distorted interpretation of the composer’s intention. In some cases, an “uncoiled” version of the SCD might be more adapted, in a similar way to the arc diagram that follows the note structure linearly (Wattenberg, 2002).
In many transdisciplinary projects it is necessary to find a common language and a balance between simplicity of use and technical quality. An easy-to-use feature will soon be available in the next version of iAnalyse, a digital musicology software developed by Pierre Couprie (Couprie, 2022a). Its use will be possible for non-specialists, especially for musicologists. A function named scd() is available in the R package seewave vs. 2.2.2 (Sueur et al., 2008a).
The different points discussed previously highlight a fundamental point in the use of representation tools in scientific analysis. Data visualizations result from a succession of determinant choices. As for any visualization tool, SCD’s final appearance depends on the choice of the similarity acoustic index, the normalization of the data, the duration of the window segmentation, the filter frequency limits, the parameters of the Fourier transform, the clustering method, and the threshold applied to the data, which only presents the most representative information and hides the most discrete of it. It is therefore not recommended to fix these analysis parameters that should be tuned by the user. We can also highlight the choice made on the colors which impact on our interpretation of the data. The choice of color must be carefully considered to limit this bias and make the information accessible to people with color vision deficiencies (Crameri et al., 2020). Therefore, it is advised to test for different visualizations to optimize the quality of the information provided to the reader. We also recommend contextualizing the raw data and data visualizations to limit our social and cultural interpretation biases. Listening back to the audio files also seems necessary for a proper interpretation.
Soundscape chord diagrams allow us to visually analyze both musical and ecological acoustic data. Using this workflow and the multiple adaptations it offers, it is possible to analyze a large diversity of soundscapes. The interpretation of SCDs is intuitive and could be made interactive with online applications for a large audience promoting the knowledge and preservation of soundscapes. The approach we are proposing opens many routes of research by combining the different techniques and concepts proposed in ecology and music.
Data availability statement
The raw data supporting the conclusions of this article will be made available by the authors, without undue reservation.
Author contributions
AdB: Conceptualization, Data curation, Formal analysis, Funding acquisition, Methodology, Visualization, Writing – original draft. PC: Conceptualization, Supervision, Writing – review & editing, Funding acquisition. FM: Writing – review & editing, Methodology. SH: Writing – review & editing, Methodology. JS: Supervision, Writing – review & editing, Conceptualization, Funding acquisition.
Funding
The author(s) declare financial support was received for the research, authorship, and/or publication of this article. This work is part of a PhD project granted by the Collegium Musicae of Sorbonne University. The work conducted in the Risoux forest was supported by the Parc Naturel Régional du Haut-Jura, which received funding from the Région Bourgogne-Franche-Comté, the Région Auvergne-Rhône-Alpes, and the DREAL Bourgogne-Franche-Comté. The work conducted in the Nouragues forest was supported by Labex CEBA which received funding from Investissements d’Avenir ANR-10-LABX-25-01.
Acknowledgments
We warmly thank Marie-Pierre Reynet and Julien Barlet for their support in the Parc Naturel Régional du Haut-Jura. We received great help from Elodie Courtois, Philippe Gaucher, Nina Marchand, Patrick Châtelet, and Florian Jeanne at the CNRS Nouragues research station. We also thank François Husson for his assistance and the two referees, Felipe N. Moreno-Gómez and Marco Gamba, for their helpful comments on the manuscript.
Conflict of interest
The authors declare that the research was conducted in the absence of any commercial or financial relationships that could be construed as a potential conflict of interest.
Publisher’s note
All claims expressed in this article are solely those of the authors and do not necessarily represent those of their affiliated organizations, or those of the publisher, the editors and the reviewers. Any product that may be evaluated in this article, or claim that may be made by its manufacturer, is not guaranteed or endorsed by the publisher.
References
Alcocer I., Lima H., Sugai L. S. M., Llusia D. (2022). Acoustic indices as proxies for biodiversity: a meta-analysis. Biol. Rev. 97, 2209–2236. doi: 10.1111/brv.12890
Barchiesi D., Giannoulis D., Stowell D., Plumbley M. D. (2015). Acoustic scene classification: classifying environments from the sounds they produce. IEEE Signal Proc. Mag. 32 (3), 16−34. doi: 10.1109/MSP.2014.2326181
Birdlife International (2023) Avibase Bird checklist of the world. Available at: https://avibase.bsc-eoc.org/ (Accessed 20 October 2023).
Bongers F., Charles-Dominique P., Forget P.-M., Théry M. (2001). Nouragues (Dordrecht: Springer Netherlands). doi: 10.1007/978-94-015-9821-7
Bradbury J. W., Vehrencamp S. L. (2011). Principles of Animal Communication (Sunderland, MA: Sinauer Associates).
Bradfer-Lawrence T., Gardner N., Bunnefeld L., Bunnefeld N., Willis S. G., Dent D. H. (2019). Guidelines for the use of acoustic indices in environmental research. Methods Ecol. Evol. 10, 1796–1807. doi: 10.1111/2041-210X.13254
Buxton R. T., McKenna M. F., Clapp M., Meyer E., Stabenau E., Angeloni L. M., et al. (2018). Efficacy of extracting indices from large-scale acoustic recordings to monitor biodiversity. Conserv. Biol. 32, 1174–1184. doi: 10.1111/cobi.13119
Caesar R. (1992). The Composition of Electroacoustic Music. [PhD Thesis]. Norwich, UK: University of East Anglia.
Charles-Dominique P. (2001). “The field station,” in Nouragues: Dynamics and Plant-Animal Interactions in a Neotropical Rainforest Monographiae Biologicae. Eds. Bongers F., Charles-Dominique P., Forget P.-M., Théry M. (Dordrecht: Springer Netherlands), 19–30. doi: 10.1007/978-94-015-9821-7_3
Couprie P. (2015). La visualisation du son et de ses paramètres pour l’analyse de la musique acousmatique. Dossier d’habilitation à diriger des recherches (Paris, FR: Université Paris-Sorbonne).
Couprie P. (2018). "Methods and tools for transcribing electroacoustic music," in Proceedings of the 4th International Conference on Technologies for Music Notation and Representation (Montréal, CA: TENOR 2018), 7–16.
Couprie P. (2022a). Analytical approaches to electroacoustic music improvisation. Organ. Sound 27, 117–130. doi: 10.1017/S1355771821000571
Couprie P. (2022b). "Designing Sound Representations For Musicology," in Sound and Music Computing Conference (SMC) (Saint-Etienne, FR: Université de Saint-Etienne and INRIA and GRAME), 563–569.
Crameri F., Shephard G. E., Heron P. J. (2020). The misuse of colour in science communication. Nat. Commun. 11, 5444. doi: 10.1038/s41467-020-19160-7
Deng J., Zhang Z.-Y., Yang Q., Wu X.-D. (2022). Overview of non-methane volatile organic compounds for world economy: From emission source to consumption sink. Energy Nexus 6, 100064. doi: 10.1016/j.nexus.2022.100064
Dumyahn S. L., Pijanowski B. C. (2011). Soundscape conservation. Landsc. Ecol. 26, 1327–1344. doi: 10.1007/s10980-011-9635-x
Ephraim Y., Malah D. (1984). Speech enhancement using a minimum-mean square error short-time spectral amplitude estimator. IEEE Trans. Acoust. Speech Signal Process. 32, 1109–1121. doi: 10.1109/TASSP.1984.1164453
Farina A. (2014). “Soundscape and landscape ecology,” in Soundscape Ecology: Principles, Patterns, Methods and Applications. Ed. Farina A. (Dordrecht: Springer Netherlands), 1–28. doi: 10.1007/978-94-007-7374-5_1
Ferrara A., Biró M., Malatesta L., Molnár Z., Mugnoz S., Tardella F. M., et al. (2021). Land-use modifications and ecological implications over the past 160 years in the central Apennine mountains. Landsc. Res. 46, 932–944. doi: 10.1080/01426397.2021.1922997
Foote J., Cooper M. L. (2001). "Visualizing Musical Structure and Rhythm via Self-Similarity," in Proceedings of the 2001 International Computer Music Conference (Havana, CU: ICMC).
Gasc A., Gottesman B. L., Francomano D., Jung J., Durham M., Mateljak J., et al. (2018). Soundscapes reveal disturbance impacts: biophonic response to wildfire in the Sonoran Desert Sky Islands. Landsc. Ecol. 33, 1399–1415. doi: 10.1007/s10980-018-0675-3
Gietel-Basten S. (2020). Circular visualisation of historical migration in England in the long eighteenth-century. Heliyon 6, e05490. doi: 10.1016/j.heliyon.2020.e05490
Grimaldi M., Riéra B. (2001). “Scales of ambient light variation,” in Nouragues: Dynamics and Plant-Animal Interactions in a Neotropical Rainforest Monographiae Biologicae. Eds. Bongers F., Charles-Dominique P., Forget P.-M., Théry M. (Dordrecht: Springer Netherlands), 19–30. doi: 10.1007/978-94-015-9821-7_3
Grinfeder E., Haupert S., Ducrettet M., Barlet J., Reynet M.-P., Sèbe F., et al. (2022a). Soundscape dynamics of a cold protected forest: dominance of aircraft noise. Landsc. Ecol. 37, 567–582. doi: 10.1007/s10980-021-01360-1
Grinfeder E., Lorenzi C., Haupert S., Sueur J. (2022b). What do we mean by “Soundscape”? A functional description. Front. Ecol. Evol. 10. doi: 10.3389/fevo.2022.894232
Gu Z., Gu L., Eils R., Schlesner M., Brors B. (2014). circlize Implements and enhances circular visualization in R. Bioinformatics 30, 2811–2812. doi: 10.1093/bioinformatics/btu393
Hakim A., Mahmood M. T. (2021). Automated birdsong clustering and interactive visualization tool. Pak. J. Agric. Sci. 58, 1395–1403. doi: 10.21162/PAKJAS/21.195
Landy L. (1999). Reviewing the musicology of electroacoustic music: a plea for greater triangulation. Organ. Sound 4, 61–70. doi: 10.1017/S1355771899001077
Lê S., Josse J., Husson F. (2008). FactoMineR: an R package for multivariate analysis. J. Stat. Software 25, 1–18. doi: 10.18637/jss.v025.i01
Lellouch L., Pavoine S., Jiguet F., Glotin H., Sueur J. (2014). Monitoring temporal change of bird communities with dissimilarity acoustic indices. Methods Ecol. Evol. 5, 495–505. doi: 10.1111/2041-210X.12178
Lerch A. (2023). “An introduction to audio content analysis,” in Applications in Signal Processing and Music Informatics (Piscataway, NJ: IEE Press and Wiley).
Malt M., Jourdan E. (2015). “Le ‘BSTD’ – Une représentation graphique de la brillance et de l’écart type spectral, comme possible représentation de l’évolution du timbre sonore,” in L’analyse musicale aujourd’hui (Paris, FR: Delatour).
Mancero Baquerizo D. (2019). Composition musicale et modélisation de l’espace hétérophonique des Soundscape Compositions Vol. 8 (Paris, FR: Université Paris). PhD thesis.
Metcalf O., Abrahams C., Ashington B., Baker E., Bradfer-Lawrence T., Browning E., et al. (2023). Good practice guidelines for long-term ecoacoustic monitoring in the UK. (Manchester, UK: The UK Acoustics Network). Available at: https://acoustics.ac.uk/.
Ono J., Corrêa D., Ferreira M., Mello R., Nonato L. (2015). "Similarity graph: Visual exploration of song collections," in SIPGRAPI 2015: 28th Conference on Graphics, Patterns and Images. (Los Alamitos, CA: IEEE).
Phillips Y. F., Towsey M., Roe P. (2018). Revealing the ecological content of long-duration audio-recordings of the environment through clustering and visualisation. PloS One 13, e0193345. doi: 10.1371/journal.pone.0193345
Pijanowski B. C., Villanueva-Rivera L. J., Dumyahn S. L., Farina A., Krause B. L., Napoletano B. M., et al. (2011). Soundscape ecology: the science of sound in the landscape. BioScience 61, 203–216. doi: 10.1525/bio.2011.61.3.6
Rodriguez A., Gasc A., Pavoine S., Grandcolas P., Gaucher P., Sueur J. (2014). Temporal and spatial variability of animal sound within a neotropical forest. Ecol. Inform. 21, 133–143. doi: 10.1016/j.ecoinf.2013.12.006
Schafer R. M. (1977). Our Sonic Environment and the Tuning of the World: The Soundscape (Rochester, VT: Destiny Books).
Southworth M. (1969). The sonic environment of cities. Environ. Beh. 1, 49–70. doi: 10.1177/001391656900100104
Sueur J., Aubin T., Simonis C. (2008a). Seewave, a free modular tool for sound analysis and synthesis. Bioacoustics 18, 213–226. doi: 10.1080/09524622.2008.9753600
Sueur J., Farina A. (2015). Ecoacoustics: the ecological investigation and interpretation of environmental sound. Biosemiotics 8, 493–502. doi: 10.1007/s12304-015-9248-x
Sueur J., Farina A., Gasc A., Pieretti N., Pavoine S. (2014). Acoustic indices for biodiversity assessment and landscape investigation. Acta Acustica United Acustica 100, 772–781. doi: 10.3813/AAA.918757
Sueur J., Krause B., Farina A. (2019). Climate change is breaking earth’s beat. Trends Ecol. Evol. 34, 971–973. doi: 10.1016/j.tree.2019.07.014
Sueur J., Windmill J. F. C., Robert D. (2008b). Sexual dimorphism in auditory mechanics: tympanal vibrations of Cicada orni. J. Exp. Biol. 211, 2379–2387. doi: 10.1242/jeb.018804
Towsey M., Zhang L., Cottman-Fields M., Wimmer J., Zhang J., Roe P. (2014). Visualization of long-duration acoustic recordings of the environment. Proc. Comput. Sci. 29, 703–712. doi: 10.1016/j.procs.2014.05.063
Towsey M., Znidersic E., Broken-Brow J., Indraswari K., Watson D. M., Phillips Y., et al. (2018). Long-duration, false-colour spectrograms for detecting species in large audio data-sets. JEA 2, 1–1. doi: 10.22261/JEA.IUSWUI
Truax B. (1999). The World Soundscape Project’s Handbook for Acoustic Ecology (Vancouver, BC: CD-ROM edition, Cambridge Street Publishing).
Truax B. (2008). Soundscape Composition as Global Music: Electroacoustic music as soundscape. Organ. Sound 13 (2), 103–109. doi: 10.1017/S1355771808000149
Truax B. (2012). "From soundscape documentation to soundscape composition," in Proceedings of the Acoustics 2012 Nantes Conference (Nantes, FR: Acoustics 2012), 2103–2107.
Ulloa J. S., Aubin T., Llusia D., Bouveyron C., Sueur J. (2018). Estimating animal acoustic diversity in tropical environments using unsupervised multiresolution analysis. Ecol. Indic. 90, 346–355. doi: 10.1016/j.ecolind.2018.03.026
Wattenberg M. (2002). "Arc diagrams: visualizing structure in strings," in IEEE Symposium on Information Visualization. INFOVIS 2002 (Boston, USA: IEEE), 110–116. doi: 10.1109/INFVIS.2002.1173155
Westerkamp H. (2002). Linking soundscape composition and acoustic ecology. Organ. Sound 7 (1), 51–56. doi: 10.1017/S1355771802001085
Keywords: visual analysis, ecoacoustics, electroacoustic, acoustic monitoring, musical data, music analysis
Citation: de Baudouin A, Couprie P, Michaud F, Haupert S and Sueur J (2024) Similarity visualization of soundscapes in ecology and music. Front. Ecol. Evol. 12:1334776. doi: 10.3389/fevo.2024.1334776
Received: 07 November 2023; Accepted: 08 January 2024;
Published: 07 February 2024.
Edited by:
Almo Farina, University of Urbino Carlo Bo, ItalyReviewed by:
Marco Gamba, University of Turin, ItalyFelipe N. Moreno-Gómez, Universidad Católica del Maule, Chile
Copyright © 2024 de Baudouin, Couprie, Michaud, Haupert and Sueur. This is an open-access article distributed under the terms of the Creative Commons Attribution License (CC BY). The use, distribution or reproduction in other forums is permitted, provided the original author(s) and the copyright owner(s) are credited and that the original publication in this journal is cited, in accordance with accepted academic practice. No use, distribution or reproduction is permitted which does not comply with these terms.
*Correspondence: Adèle de Baudouin, adele.de-baudouin@mnhn.fr