- Division of Gastroenterology, The First Affiliated Hospital, Sun Yat-sen University, Guangzhou, China
Background: Infliximab is effective in inducing and maintaining remission in patients with Crohn’s disease (CD), but primary non-response (PNR) occurs in 10-30% of cases. We investigated whether serum biomarkers are effective in predicting PNR in patients with CD.
Methods: From January 2016 to April 2020, a total of 260 patients were recruited to this prospective and retrospective cohort study. Serum samples were collected at baseline and week 2 of infliximab treatment. Serum levels of 35 cytokines were assessed in 18 patients from the discovery cohort and were further evaluated in the 60-patient cohort 1. Then, candidate cytokines and other serological biomarkers were used to construct a predictive model by logistic regression in a 182-patient cohort 2. PNR was defined based on the change of CD activity index or clinical symptoms.
Results: Among the 35 cytokines, matrix metalloproteinase 3(MMP3) and C-C motif ligand 2 (CCL2) were two effective serum biomarkers associated with PNR in both the discovery cohort and cohort 1. In cohort 2, serum level of MMP3, CCL2 and C-reactive protein (CRP) at 2 weeks after infliximab injection were independent predictors of PNR, with odds ratios (95% confidence interval) of 1.108(1.059-1.159), 0.940(0.920-0.965) and 1.102(1.031-1.117), respectively. A PNR classifier combining these three indicators had a large area under the curve [0.896(95% CI:0.895-0.897)] and negative predictive value [0.918(95%CI:0.917-0.919)] to predict PNR to infliximab.
Conclusions: MMP3, CCL2, and CRP are promising biomarkers in prediction of PNR to infliximab, and PNR classifier could accurately predict PNR and may be useful in clinical practice for therapy selection.
Introduction
The introduction of infliximab (IFX), a chimeric monoclonal antibody against tumour necrosis factor (TNF)-α, has significantly improved therapy to induce and maintain remission in Crohn’s disease (CD) (1). However, 10-30% of patients receiving IFX are non-responsive during induction therapy (primary non-response, PNR) (2). Furthermore, IFX therapy is expensive and may give rise to some adverse events, such as infusion reactions and infections (1). Incorrect use of IFX in PNR patients would delay treatment as well as lead to disease progression (3). Thus, it is important to explore a method for precisely predicting PNR in patients of CD.
Previous studies have demonstrated that clinical characteristics (4, 5), including disease duration, age at diagnosis, concomitant use of immunosuppressant drugs, and disease location or behaviour, were associated with the efficacy of IFX. Besides, genetic polymorphisms (6, 7), microbiome (8) and serum biomarkers (5, 9) have also been studied with regard to response to IFX. Although some risk factors of PNR were confirmed, the mechanisms underlying PNR have not been broadly defined. It has been widely proposed that non-TNF-α mediated inflammation may result in PNR to IFX and some pro-inflammatory pathways could even be regulated by TNFα blockade (10). Therefore, we believe that the inflammatory state of patients could affect the IFX response, and alterations of inflammatory cytokines in serum might be an appropriate biomarker to predict PNR.
Previous studies have shown that inflammatory cytokines, including TNF-α, interleukin (IL)-6, IL-1β, IL-17A, IL-23, and IL-12, may be predictive factors of PNR to IFX (11–13). However, these studies only found the discrepant expression levels of the cytokines between the respondent and non-respondent groups, and none of these cytokines has been widely used in clinical practise. Thus, identifying effective serum cytokines that affect therapeutic failure could help select the most appropriate patients for IFX treatment. In this prospective and retrospective cohort study, we assessed circulating inflammatory cytokines levels before and after the administration of IFX to identify potential serum biomarkers, and then constructed a model in prediction of PNR to IFX in CD patients.
Patients and Methods
Study Design and Patient Population
This was a prospective and retrospective, single-centre cohort study, approved by the institutional ethics committee (IEC) for Clinical Research and Animal Trials of the First Affiliated Hospital of Sun Yat-sen University (No. 2019-383). Informed consent was obtained from all patients. Patients with a definite diagnosis of CD and receiving IFX therapy in the First Affiliated Hospital of Sun Yat-sen University were consecutively included in the study from January 2016 to April 2020. Other inclusion criteria were as follows: (1) treatment with 5 mg/kg IFX intravenously at week 0, 2, and 6; (2) naïve to anti-TNF and other biologicals therapy; and (3) CD activity index (CDAI) of ≥150 before IFX treatment. Exclusion criteria included the following: (1) lack of evaluation for disease activity at baseline or at week 14 after the first treatment with IFX; (2) unavailable blood samples at baseline and week 2; (3) delayed infusion; (4) pregnancy; and (5) lost to follow-up.
The Montreal system was used to classify the location and behaviour of CD patients (14). The CDAI was calculated for all patients at baseline and week 14 after IFX therapy. At baseline, clinical characteristics including sex, age at first IFX therapy, age at diagnosis, disease duration, body mass index, disease location and behaviour, presence of extraintestinal manifestations, history of smoking and surgery, concomitant azathioprine, and CDAI score were recorded. The endoscopic activity of patients was assessed using the Simple Endoscopic Score for Crohn’s Disease (SESCD). C-reactive protein (CRP), erythrocyte sedimentation rate (ESR), albumin, haemoglobin, white blood cell, neutrophil, lymphocytes, and platelets counts were assessed at baseline and week 2 after IFX therapy.
PNR of IFX was defined as a failure of the CDAI to drop more than 70 points or to <150 at week 14 after IFX administration. Situations in which patients received an alternative therapy schedule such as an escalation of corticosteroid therapy, switching to other agents or having the surgery before week 14 were also defined as PNR.
In this prospective and retrospective study, a total of 260 patients were eligible (Figure 1). Retrospective single-centre cohorts were designed to screen (discovery cohort, n=18) and validate (Cohort 1, n=60) the candidate cytokines. The first 9 primary responders and 9 non-responders constituted a discovery cohort to identify potential biomarkers associated with PNR. Serum levels of 35 cytokines at baseline and week 2 after IFX therapy were examined by the Luminex cytokine multiplex assay in 18 patients from the discovery cohort. The latter 60 patients were recruited into cohort 1 to further verify and screen the candidate cytokines selected from the discovery cohort. Thereafter, a prospective single-centre cohort (cohort 2, n=182) was recruited from May 2018 to April 2020 in the First Affiliated Hospital of Sun Yat-sen University, which were used to construct a predictive model based on the findings from cohort 1 and do internal validation through the bootstrap validation approach.
Sample Collection
The blood samples were collected into serum separator tubes (BD Biosciences, Franklin Lakes, NJ, USA) before intravenous injection of Infliximab at baseline and week 2. The tubes were centrifuged at 1900× g for 15 minutes, then the serum samples were aliquoted with 0.5ml tubes (Ambion, Foster City, CA) and stored at −80°C until analyses.
Assay for Serum Biomarkers
Serum levels of 35 cytokines, including C-C motif ligand (CCL)2, CCL4, CCL11, CCL20, CCL25, CCL26, CX3CL1, CXCL8, CXCL11, ADAMTS13, adiponectin, α-2-macroglobulin, matrix metalloproteinase (MMP)3, insulin-like growth factor binding protein-1, TNF-α, IL-1ß, IL-2, IL-4, IL-6, IL-7, IL-10, IL-11, IL-12 p70, IL-13, IL-17, IL-18, IL-23, IL-27, IL-28A, IL-28B, IL-31, IL-33, IL-34, and IL-36ß, at baseline and week 2 in discovery cohort were measured using a Luminex cytokine assay kit (LXSAHM-35) and a Luminex X-200 instrument according to the manufacturer’s protocol (R&D Systems, Minneapolis, MN, USA).
Enzyme-linked immunosorbent assay (ELISA) was used to measure levels of the molecules selected after the analysis of Luminex results obtained in the discovery cohort. In cohort 1 and 2, serum MMP3 levels were measured using a quantitative sandwich enzyme immunoassay (R&D Systems) at baseline and week 2. The serum CCL2 concentration was measured at week 2 using an ELISA kit (Abcam, Cambridge, UK, Ab179886). Serum IL-7 levels were measured using an ELISA kit (R&D Systems). Other indicators, such as CRP, ESR, albumin and so on, were tested with standard institutional protocols by the laboratory physicians at the First Affiliated Hospital of Sun Yat-sen University.
Statistical Analyses
Continuous and categorical variables were described as medians with interquartile ranges and frequency with percentages, respectively. The non-parametric Wilcoxon and chi-square tests for continuous and categorical variables, respectively, were used to assess differences between responders and non-responders. The False Discovery Rate (Benjaminiand-Hochberg method) was performed to adjust the p-value in the comparison of 35 serum biomarkers between primary responders and non-responders in the discovery cohort. A p-value of <0.05 denoted statistical significance. Multivariate logistic regression (enter) was used to evaluate the relationship between the clinical or serological variables with the significant differences in the univariate analysis and the outcome of PNR among all patients in cohort 2, and also in patients with determined response status by CDAI in cohort 2. The variables with a p-value of <0.05 in the multivariate analyses were defined as independent predictive indicators, and were selected to construct a logistic regression model, the PNR classifier. Multivariate logistic regression was also performed to calculate the odds ratio (OR) of the independent predictive indicators and PNR classifier in predicting PNR. The area under the receiver operating characteristics curve (AUC), sensitivity, specificity, positive predictive value and negative predictive value were used to assess the predictive ability of the model, and their respective mean [95% confidence interval (CI)] were calculated by bootstrapping with 2,000 replications. Moreover, the bootstrap validation approach was also used to assess the internal validation of the independent predictive indicators and the PNR classifier for predicting PNR in cohort 2. R software, version 4.0.0, was used for statistical analyses.
Results
Patients Characteristics
Between January 2016 and April 2020, 260 patients were included in this prospective and retrospective cohort study (Figure 1). Baseline characteristics for discovery cohort, and cohorts 1 and 2 are documented in Supplement Table 1 and Table 1, respectively. Only perianal disease (p<0.001) and serum albumin concentration (p=0.019) were significantly different between the two cohorts. In PNR patients, only ESR (p=0.015) was significantly different between cohort 1 and cohort 2 (Supplement Table 2). To evaluate the disease activity at week 14 more objectively, we performed a comparison of CRP, ESR and SESCD between primary responders and primary non-responders (Supplement Table 3). The results showed that primary non-responders had higher CRP, ESR and SESCD levels than primary responders at week 14 in both cohort 1 (CRP: p<0.001; ESR: p<0.001; SESCD: p<0.001) and cohort 2 (CRP: p<0.001; ESR: p<0.001; SESCD: p<0.001).
Distinct Serum Cytokine Profiles in CD Patients Receiving IFX Treatment
In the discovery cohort, Luminex multiplex assay was performed using serum samples collected before IFX treatment and at week 2. Through non-parametric Wilcoxon test, we found that the serum CCL2 level at week 2 (p=0.013), MMP3 level at baseline (p=0.050), MMP3 level at week 2 (p=0.008), and the change of IL-7 from baseline to week 2 (ΔIL-7) (p=0.050) were significantly different between the primary responders and non-responders (Supplement Table 4). However, after adjusting the p-value by False Discovery Rate, none of the indicators was significantly different.
To verify the discrepant levels of these four serum indicators, we performed further analyses by ELISA in cohort 1. MMP3 level at baseline (p=0.040), MMP3 level at week 2 (p=0.004) and CCL2 level at week 2 (p=0.001) showed significant difference between primary non-responders and responders, while ΔIL-7 showed no significant change (p=0.094) (Table 2). Furthermore, we observed that CCL2 and MMP3 levels at week 2 were significantly altered in the multivariate analysis; the odds ratios (ORs) (95% CI) were 0.903 (0.877-0.986, p=0.010) and 1.203 (1.039-1.394, p=0.008), respectively. The receiver operating characteristics analysis revealed that CCL2 [AUC (95% CI): 0.790 (0.786-0.793)] and MMP3 [AUC (95% CI): 0.764 (0.760-0.767)] levels at week 2 could better predict PNR than MMP3 at baseline [AUC (95% CI): 0.683 (0.678-0.687)] and ΔIL-7 [AUC (95% CI): 0.632 (0.627-0.637)] (Figure 2).

Table 2 Comparison of serum biomarkers detected by ELISA between primary non-responders and responders in cohort 1.
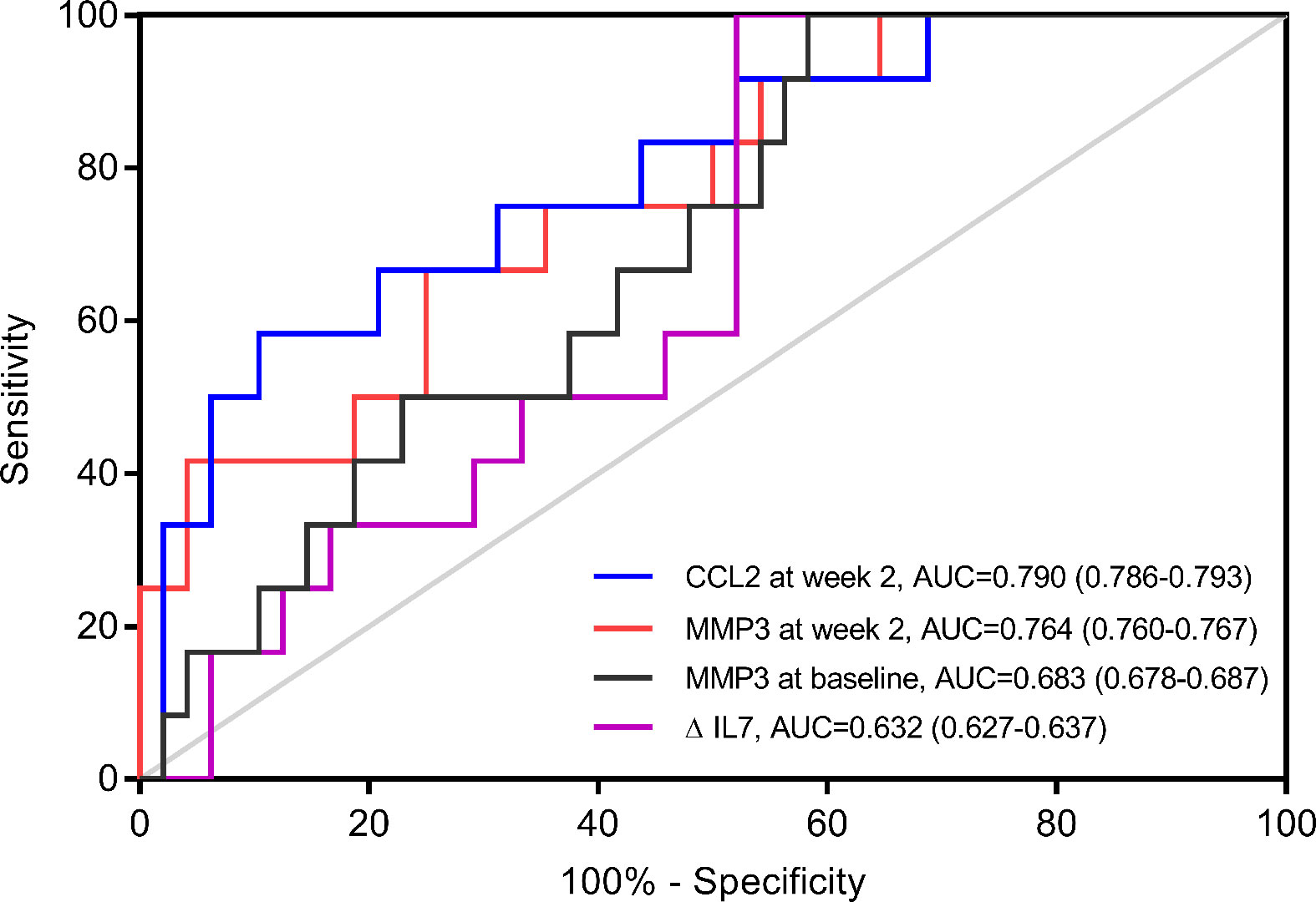
Figure 2 Receiver operating characteristics (ROC) analyses of potential predictors in cohort 1. ROC Curve of change of IL-7 from baseline to week 2 (ΔIL-7), matrix metalloproteinase (MMP) 3 at baseline, MMP3 at week 2 and C-C motif ligand (CCL) 2 at week 2 for predicting primary non-response (n=12) in cohort 1 (n=60).
CCL2, MMP3, and CRP at Week 2 Are Independently Predictive of PNR
Considering that CCL2 and MMP3 at week 2 were the most important predictors of PNR to IFX, we further analysed these two indicators, as well as other clinical and serological markers, in cohort 2.
Univariate analyses showed that three clinical characteristics and eight serological indicators, including age at first IFX therapy (p=0.044), disease duration (p=0.002), CD behaviour (p=0.001), neutrophil percentage (p=0.010), lymphocytes counts(p=0.042), lymphocyte percentage (p=0.007), platelet-to-lymphocyte ratio (p=0.028), neutrophil-to-lymphocyte ratio (p=0.008), CRP (p<0.001), MMP3 (p<0.001) and CCL2 levels (p<0.001) at week 2, were significantly different between primary non-responders and responders (Table 3 and Supplemental Table 5).
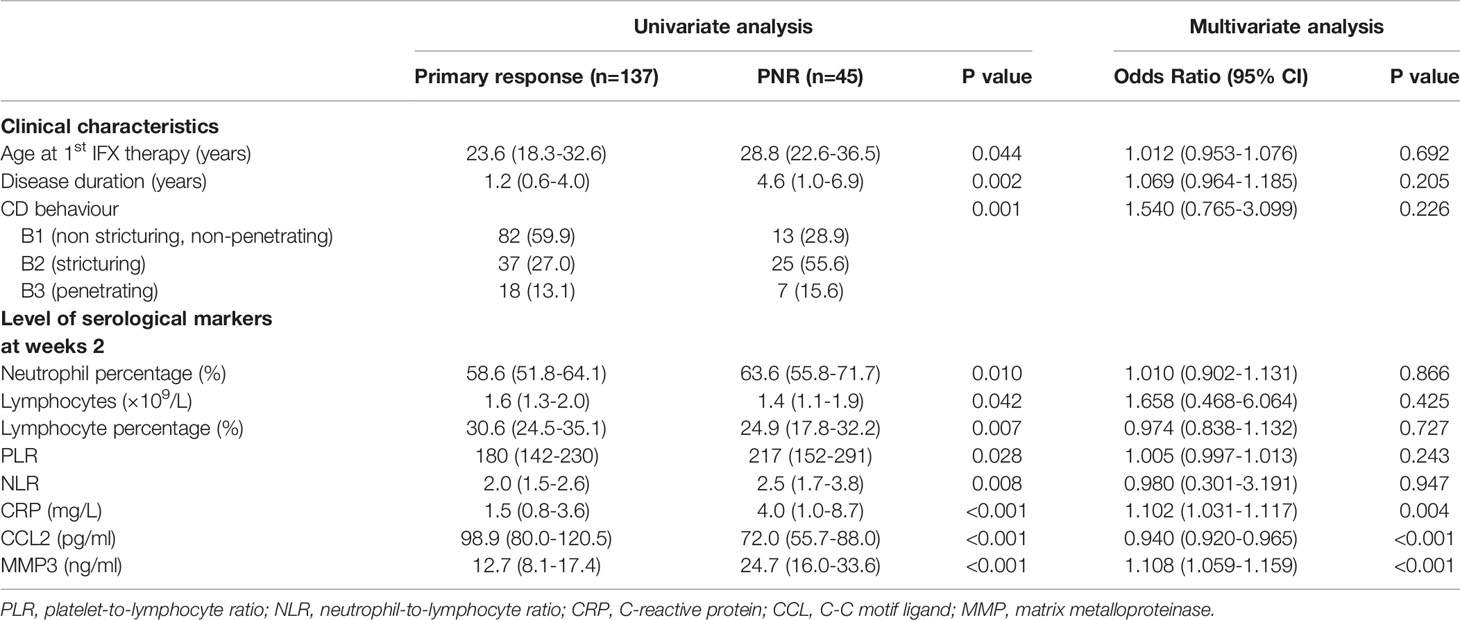
Table 3 Significant univariate association of clinical characteristics and serological markers for primary non-response in cohort 2.
Multivariate analyses of all patients in cohort 2 revealed that only CRP [OR 1.102 (95%CI: 1.031-1.117), p=0.004], MMP3 [OR 1.108 (95%CI: 1.059-1.159), p<0.001], and CCL2 levels at week 2 [OR 0.940 (95%CI: 0.920-0.965), p<0.001] were independently associated with PNR (Table 3). Another multivariate analysis was performed in patients with determined response status by CDAI, and the result was similar to that obtained while including all patients (Supplement Table 6).
A Novel Model for Predicting PNR
The predictive values of CRP, CCL2, and MMP3 at week 2 are shown in Table 4. Both CRP and MMP3 had large specificity [(95% CI): 0.768 (0.765-0.770) and 0.799 (0.796-0.802), respectively], whereas CCL2 had high sensitivity [0.783 (0.778-0.788)], but low specificity [0.687 (0.681-0.692)].
To better predict PNR, we built a PNR classifier combining serum levels of CRP, CCL2, and MMP3 at week 2 through logistic regression, and the variance inflation factors of CRP, MMP3 and CCL2 was equal to 1.004, 1.008 and 1.012, respectively. PNR classifier had a large AUC of 0.896 (95% CI: 0.895-0.897) to predict PNR (Table 4). PNR classifier also had the largest specificity [0.888 (95%CI: 0.885-0.890)], negative predictive value [0.918 (95%CI: 0.917-0.919)] and positive predictive value [0.703 (95%CI: 0.699-0.708)] in the prediction of PNR among these 4 indicators. The OR for getting PNR in patients with a value of PNR classifier > 0.331 was 6.467 (95% CI: 1.928-21.687; p=0.002), after adjustment for covariates (Supplement Table 7). Furthermore, patients with serum concentration of MMP3 > 19.15pg/ml [OR (95% CI): 8.478 (2.307-31.160); p=0.001] or CRP > 3.79 mg/L [OR (95% CI): 6.314 (1.956-20.377); p=0.002] also had the high risk to getting PNR.
Discussion
IFX therapy is currently an important choice to induce and maintain remission in CD patients, but approximately 30% of patients exhibit PNR to IFX (1, 2). To achieve the goal of treating each patient at the right time with the optimal drug, it is necessary to detect which group of patients are unlikely to benefit from IFX treatment. In this prospective and retrospective cohort study of 260 CD patients, we found that clinical characteristics such as older age at first IFX therapy, longer disease duration, and stricturing behaviour were significantly associated with PNR to IFX. Furthermore, serum CRP, MMP3, and CCL2 levels at week 2 after IFX injection were the three independent predictors for PNR. Additionally, to better monitor CD, we further established a PNR classifier incorporating these three inflammation mediators, which was demonstrated to have a larger AUC (0.898 [95% CI: 0.837-0.947]) in discriminating primary non-responders from primary responders than using CRP, MMP3 or CCL2 independently. Thus, it can effectively identify patients who are suitable for IFX induction therapy.
MMP3 is a pattern of the large family of zinc dependent matrix metalloproteinases (MMPs), which plays an active role in the pathogenesis of inflammation in inflammatory bowel disease (IBD). The expression and secretion of MMPs are sensitive to the condition of intestinal inflammation, and are either augmented or moderated by a series of inflammatory cytokines, especially TNF-α (15). MMP3 is regarded as a crucial effector molecule of inflammatory cells, while it can also modify cytokines and chemokines (15). Thus, the up-regulated expression of MMPs prompts an initiation phase of acute inflammation. In our study, a high level of serum MMP3 at week 2 from baseline manifested as an effective predictor of PNR to IFX. Similarly, previous study had found that a high serum MMP3 concentration at week 14 was associated with a loss of response after 52 weeks of IFX treatment (16). Biancheri et al. showed that MMP3 was involved in cleaving anti-TNF agents, such as infliximab, adalimumab, and etanercept, in the colon mucosa of inflammatory bowel disease patients (17). This may explain the predictive function of MMP3 in PNR to IFX. However, whether MMP3 can predict a loss of response to other anti-TNF drugs has not been studied.
CCL2, namely monocyte chemotactic protein 1, is a CC-type chemokine that plays an important role in regulating migration and infiltration of monocytes, lymphocytes, or other immunocytes to inflammation sites in the pathogenesis of chronic inflammatory diseases such as CD (18–20). Previous studies have shown that the expression of CCL2 in intestinal epithelial cells, mucosa, circulating monocytes, or plasma was significantly increased in CD patients, especially those with active CD, compared to healthy individuals (21–23). Thus, CCL2 may be a pro-inflammatory factor in CD. Swedish patients with ulcerative colitis responding to infliximab therapy have been shown to express decreased levels of CCL2 in the serum at Week 2 (24). However, it is confusing that CD patients with PNR had lower serum CCL2 concentrations at week 2 after IFX treatment than responders in our study. A hypothesis that might explain this phenomenon is that the high serum concentration of CCL2 in primary responders is attributable to the decrease in CCR2 expression. A recent study showed that serum CCL2 was removed in a CCR2-dependent but G-protein independent manner, and when CCR2 expression decreased, serum CCL2 concentrations increased (25). Furthermore, anti-TNF non-responders with inflammatory bowel disease had upregulated CCR2 expression (26), which might cause lower serum CCL2 levels than those seen in the responders. Although this theoretical explanation makes sense, experimental validation is lacking. Therefore, it is attractive to research the change and function of the CCL2-CCR2 axis in CD patients before and after anti-TNF therapy for further studies.
CRP is one of the most common biomarkers used in clinical practice and is also useful for monitoring clinical or endoscopic activity in CD patients (27, 28). Post-injection CRP levels are a potential predictor for loss of response to anti-TNF therapy (29, 30). However, it remains controversial whether the baseline CRP level can predict loss of response to anti-TNF therapy in CD (31, 32). Our study found that serum CRP at week 2, but not baseline, was an independent predictor for PNR. However, CRP reflects the status of generic inflammation, not specific inflammatory pathways. In this study, we combined CRP with MMP3 and CCL2 levels, which are closely correlated with the pathogenesis of CD, and developed a well-founded PNR classifier.
Previous studies have demonstrated that the mechanisms underlying PNR are multifactorial. These mechanisms include disease characteristics, drug and treatment strategy-related factors, and inflammation and immune status of the patients (5). Disease duration, phenotype, and location may contribute to primary nonresponse. However, the role of patient-related characteristics was unclear because of controversial outcomes of different studies (5). Drug related factors includes through drug levels and the accumulation of anti-TNF antibodies. A recent study demonstrated that serum trough levels of IFX at week 2 could predict short-term clinical efficacy of IFX (33). Moreover, low IFX levels and high anti-TNF antibody levels to IFX at week 2 could predict PNR to IFX, with AUCs of 0.68 and 0.78, respectively (34). Nevertheless, the response status could be altered by changing the IFX dose or the combination of immunosuppressive drugs, and the effect of through drug levels and the anti-TNF antibodies could not count as primary mechanisms in the process of PNR. Emerging evidence suggest that primary nonresponse occurs in the patients with a disease course of non-TNF-driven inflammatory process. It has been thought that non-TNF-driven inflammatory processes were mainly involved in the mechanisms underlying PNR. Our research mainly focused on the non-TNF-driven inflammatory processes. We assumed that the changes of inflammatory states, which is presented by the alterations of the cytokines, chemokines and other inflammatory related factors, were significantly different between patients with TNF-driven inflammatory pathway and those were not. In the end, we explored MMP3 and CCL2 which were correlated with PNR.
There are some limitations to our study. From an analysis perspective, the reference cytokine subset we used as the discovery cohort to identify potential biomarkers is not a complete compendium of all of the immune related factors in the CD patients. Nevertheless, these potential factors have all been previously described in CD, which could compensate for the selection bias to some extent. In addition, the initial screening of cytokines in the discovery cohort was based on only 18 patients, which might be insubstantial to yield conclusive data. However, we screened potential biomarkers through analyses based not only on these 18 patients but also the patients in Cohort 1. we verified these potential cytokines selected from the discovery cohort in cohort 1 by univariate and multivariate analyses, which could reduce the incidence of false positives to a certain extent. Moreover, we did not detect IFX trough levels or anti-TNF antibody concentrations in the patients. These two indicators were thought to be able to predict loss of response of IFX (35, 36). However, this study mainly discussed the primary non-response of IFX, while trough serum concentrations of drug and anti-TNF antibodies are probably more relevant for the secondary loss of response (10). Another limitation is that all patients in our study are Chinese, which might make our results with limited generalisability.
In conclusion, our prospective and retrospective cohort study showed that CCL2 and MMP3 at week 2 play important roles in predicting PNR to IFX in CD patients. Furthermore, a PNR classifier was developed and proved effective in predicting PNR. However, further studies need to be performed on other patient populations to further substantiate our findings.
Data Availability Statement
The raw data supporting the conclusions of this article will be made available by the authors, without undue reservation.
Ethics Statement
This study was approved by the IEC for Clinical Research and Animal Trials of the First Affiliated Hospital of Sun Yat-sen University (No. 2019-383). Written informed consent to participate in this study was provided by the participants’ legal guardian/next of kin. Written informed consent was obtained from the individual(s), and minor(s)’ legal guardian/next of kin, for the publication of any potentially identifiable images or data included in this article.
Author Contributions
All authors were responsible for the study concept and design. LL and RC: drafting of manuscript. LL, RC, YZ, and GZ analyzed the serum samples and interpretation of the data. RC and LL performed the statistical analysis. BC ZZ, MC, and SZ: critical revision of manuscript for important intellectual content. All authors contributed to the article and approved the submitted version.
Funding
This project was supported by grants from the National Natural Science Foundation of China (#81630018, #81670498, #81870374, #82070538), Guangzhou Science and Technology Department (#202002030041), Guangdong Science and Technology (#2017A030306021, #2020A1515010249), China Postdoctoral Science Foundation (#2019M653228), and Science and Technology Innovation Young Talents of Guangdong Special Support Plan (#2016TQ03R296).
Conflict of Interest
The authors declare that the research was conducted in the absence of any commercial or financial relationships that could be construed as a potential conflict of interest.
Supplementary Material
The Supplementary Material for this article can be found online at: https://www.frontiersin.org/articles/10.3389/fimmu.2021.646673/full#supplementary-material
References
1. Hanauer SB, Feagan BG, Lichtenstein GR, Mayer LF, Schreiber S, Colombel JF, et al. Maintenance Infliximab for Crohn’s Disease: The ACCENT I Randomised Trial. Lancet (2002) 359:1541–9. doi: 10.1016/S0140-6736(02)08512-4
2. Gisbert JP, Panes J. Loss of Response and Requirement of Infliximab Dose Intensification in Crohn’s Disease: A Review. Am J Gastroenterol (2009) 104:760–7. doi: 10.1038/ajg.2008.88
3. Rosenblum H, Amital H. Anti-TNF Therapy: Safety Aspects of Taking the Risk. Autoimmun Rev (2011) 10:563–8. doi: 10.1016/j.autrev.2011.04.010
4. Kennedy NA, Heap GA, Green HD, Hamilton B, Bewshea C, Walker GJ, et al. Predictors of Anti-TNF Treatment Failure in Anti-TNF-Naive Patients With Active Luminal Crohn’s Disease: A Prospective, Multicentre, Cohort Study. Lancet Gastroenterol Hepatol (2019) 4:341–53. doi: 10.1016/S2468-1253(19)30012-3
5. Gisbert JP, Chaparro M. Predictors of Primary Response to Biologic Treatment [Anti-TNF, Vedolizumab, and Ustekinumab] in Patients With Inflammatory Bowel Disease: From Basic Science to Clinical Practice. J Crohns Colitis (2020) 14:694–709. doi: 10.1093/ecco-jcc/jjz195
6. Hlavaty T, Pierik M, Henckaerts L, Ferrante M, Joossens S, van Schuerbeek N, et al. Polymorphisms in Apoptosis Genes Predict Response to Infliximab Therapy in Luminal and Fistulizing Crohn’s Disease. Aliment Pharmacol Ther (2005) 22:613–26. doi: 10.1111/j.1365-2036.2005.02635.x
7. Barber GE, Yajnik V, Khalili H, Giallourakis C, Garber J, Xavier R, et al. Genetic Markers Predict Primary Non-Response and Durable Response to Anti-TNF Biologic Therapies in Crohn’s Disease. Am J Gastroenterol (2016) 111:1816–22. doi: 10.1038/ajg.2016.408
8. Zhou Y, Xu ZZ, He Y, Yang Y, Liu L, Lin Q, et al. Gut Microbiota Offers Universal Biomarkers Across Ethnicity in Inflammatory Bowel Disease Diagnosis and Infliximab Response Prediction. mSystems (2018) 3. doi: 10.1128/mSystems.00188-17
9. Chen P, Zhou G, Lin J, Li L, Zeng Z, Chen M, et al. Serum Biomarkers for Inflammatory Bowel Disease. Front Med (2020) 7:123. doi: 10.3389/fmed.2020.00123
10. D’Haens GR, Panaccione R, Higgins PD, Vermeire S, Gassull M, Chowers Y, et al. The London Position Statement of the World Congress of Gastroenterology on Biological Therapy for IBD With the European Crohn’s and Colitis Organization: When to Start, When to Stop, Which Drug to Choose, and How to Predict Response? Am J Gastroenterol (2011) 106(2):199–212. doi: 10.1038/ajg.2010.392
11. Ogawa K, Matsumoto T, Esaki M, Torisu T, Iida M. Profiles of Circulating Cytokines in Patients With Crohn’s Disease Under Maintenance Therapy With Infliximab. J Crohns Colitis (2012) 6:529–35. doi: 10.1016/j.crohns.2011.10.010
12. Martinez-Borra J, Lopez-Larrea C, Gonzalez S, Fuentes D, Dieguez A, Deschamps EM, et al. High Serum Tumor Necrosis Factor-Alpha Levels are Associated With Lack of Response to Infliximab in Fistulizing Crohn’s Disease. Am J Gastroenterol (2002) 97:2350–6. doi: 10.1111/j.1572-0241.2002.05990.x
13. Andus T, Herfarth H, Obermeier F, Kuehbacher T, Mascheretti T, Thriene T, et al. Prediction of Response to Treatment With Infliximab (Remicade) in a German Prospective Open-Label Multicenter Trial in Refractory Crohn’s Disease. Gastroenterology (2001) 120:A621. doi: 10.1016/S0016-5085(08)83089-2
14. Gomollón F, Dignass A, Annese V, Tilg H, Van Assche G, Lindsay JO, et al. 3rd European Evidence-Based Consensus on the Diagnosis and Management of Crohn’s Disease 2016: Part 1: Diagnosis and Medical Management. J Crohns Colitis (2017) 11:3–25. doi: 10.1093/ecco-jcc/jjw168
15. O’Shea NR, Smith AM. Matrix Metalloproteases Role in Bowel Inflammation and Inflammatory Bowel Disease: An Up to Date Review. Inflammation Bowel Dis (2014) 20:2379–93. doi: 10.1097/MIB.0000000000000163
16. Barberio B, D’ Incà R, Facchin S, Gasperina M, Fohom Tagne CA, Cardin R, et al. Matrix Metalloproteinase 3 Predicts Therapeutic Response in Inflammatory Bowel Disease Patients Treated With Infliximab. Inflamm Bowel Dis (2020) 26:756–63. doi: 10.1093/ibd/izz195
17. Biancheri P, Brezski RJ, Di Sabatino A, Greenplate AR, Soring KL, Corazza GR, et al. Proteolytic Cleavage and Loss of Function of Biologic Agents That Neutralize Tumor Necrosis Factor in the Mucosa of Patients With Inflammatory Bowel Disease. Gastroenterology (2015) 149:1564–74.e3. doi: 10.1053/j.gastro.2015.07.002
18. Melgarejo E, Medina MÁ, Sánchez-Jiménez F, Urdiales JL. Monocyte Chemoattractant Protein-1: A Key Mediator in Inflammatory Processes. Int J Biochem Cell Biol (2009) 41:998–1001. doi: 10.1016/j.biocel.2008.07.018
19. Moser B, Loetscher P. Lymphocyte Traffic Control by Chemokines. Nat Immunol (2001) 2:123–8. doi: 10.1038/84219
20. He J, Song Y, Li G, Xiao P, Liu Y, Xue Y, et al. Fbxw7 Increases CCL2/7 in CX3CR1hi Macrophages to Promote Intestinal Inflammation. J Clin Invest (2019) 129:3877–93. doi: 10.1172/JCI123374
21. Grip O, Janciauskiene S, Lindgren S. Circulating Monocytes and Plasma Inflammatory Biomarkers in Active Crohn’s Disease: Elevated Oxidized Low-Density Lipoprotein and the Anti-Inflammatory Effect of Atorvastatin. Inflamm Bowel Dis (2004) 10:193–200. doi: 10.1097/00054725-200405000-00003
22. Reinecker HC, Loh EY, Ringler DJ, Mehta A, Rombeau JL, MacDermott RP. Monocyte-Chemoattractant Protein 1 Gene Expression in Intestinal Epithelial Cells and Inflammatory Bowel Disease Mucosa. Gastroenterology (1995) 108:40–50. doi: 10.1016/0016-5085(95)90006-3
23. Christophi GP, Rong R, Holtzapple PG, Massa PT, Landas SK. Immune Markers and Differential Signaling Networks in Ulcerative Colitis and Crohn's Disease. Inflammation Bowel Dis (2012) 18:2342–56. doi: 10.1002/ibd.22957
24. Magnusson MK, Strid H, Isaksson S, Bajor A, Lasson A, Ung K, et al. Response to Infliximab Therapy in Ulcerative Colitis is Associated With Decreased Monocyte Activation, Reduced CCL2 Expression and Downregulation of Tenascin C. J Crohn’s Colitis (2015) 9:56–65. doi: 10.1093/ecco-jcc/jju008
25. Kaushansky N, Bakos E, Becker-Herman S, Shachar I, Ben-Nun A. Circulating Picomolar Levels of CCL2 Downregulate Ongoing Chronic Experimental Autoimmune Encephalomyelitis by Induction of Regulatory Mechanisms. J Immunol (2019) 203:1857–66. doi: 10.4049/jimmunol.1900424
26. Gaujoux R, Starosvetsky E, Maimon N, Vallania F, Bar-Yoseph H, Pressman S, et al. Cell-Centred Meta-Analysis Reveals Baseline Predictors of Anti-TNFα non-Response in Biopsy and Blood of Patients With IBD. Gut (2019) 68:604–14. doi: 10.1136/gutjnl-2017-315494
27. Ma C, Battat R, Khanna R, Parker CE, Feagan BG, Jairath V. What is the Role of C-Reactive Protein and Fecal Calprotectin in Evaluating Crohn’s Disease Activity? Best Pract Res Clin Gastroenterol (2019) 38–39:101602. doi: 10.1016/j.bpg.2019.02.004
28. Mosli MH, Zou G, Garg SK, Feagan SG, MacDonald JK, Chande N, et al. C-Reactive Protein, Fecal Calprotectin, and Stool Lactoferrin for Detection of Endoscopic Activity in Symptomatic Inflammatory Bowel Disease Patients: A Systematic Review and Meta-Analysis. Am J Gastroenterol (2015) 110:802–19; quiz 820. doi: 10.1038/ajg.2015.120
29. Rodrigues-Pinto E, Magro F, Santos-Antunes J, Vilas-Boas F, Lopes S, Nunes A, et al. High C-Reactive Protein in Crohn’s Disease Patients Predicts Nonresponse to Infliximab Treatment. J Crohns Colitis (2013) 7:S208. doi: 10.1016/S1873-9946(13)60516-4
30. Reinisch W, Wang Y, Oddens BJ, Link R. C-Reactive Protein, an Indicator for Maintained Response or Remission to Infliximab in Patients With Crohn’s Disease: A Post-Hoc Analysis From ACCENT I. Aliment Pharmacol Ther (2012) 35:568–76. doi: 10.1111/j.1365-2036.2011.04987.x
31. Colombel JF, Sandborn WJ, Reinisch W, Mantzaris GJ, Kornbluth A, Rachmilewitz D, et al. Infliximab, Azathioprine, or Combination Therapy for Crohn’s Disease. N Engl J Med (2010) 362:1383–95. doi: 10.1056/NEJMoa0904492
32. Sprakes MB, Ford AC, Warren L, Greer D, Hamlin J. Effcacy, Tolerability, and Predictors of Response to Infliximab Therapy for Crohn’s Disease: A Large Single Centre Experience. J Crohns Colitis (2012) 6:143–53. doi: 10.1016/j.crohns.2011.07.011
33. Gonczi L, Vegh Z, Golovics PA, Rutka M, Gecse KB, Bor R, et al. Prediction of Short- and Medium-Term Efficacy of Biosimilar Infliximab Therapy. Do Trough Levels and Antidrug Antibody Levels or Clinical And Biochemical Markers Play the More Important Role? J Crohns Colitis (2017) 11:697–705. doi: 10.1093/ecco-jcc/jjw203
34. Bar-Yoseph H, Levhar N, Selinger L, Manor U, Yavzori M, Picard O, et al. Early Drug and Anti-Infliximab Antibody Levels for Prediction of Primary Nonresponse to Infliximab Therapy. Aliment Pharmacol Ther (2018) 47:212–8. doi: 10.1111/apt.14410
35. Ding NS, Hart A, De Cruz P, Levesque BG, Vermeire S, Sandborn WJ. Systematic Review: Predicting and Optimising Response to Anti-TNF Therapy in Crohn’s Disease - Algorithm for Practical Management. Aliment Pharmacol Ther (2016) 43:30–51. doi: 10.1111/apt.13445
36. Papamichael K, Gils A, Rutgeerts P, Levesque BG, Vermeire S, Sandborn WJ, et al. Role for Therapeutic Drug Monitoring During Induction Therapy With TNF Antagonists in IBD: Evolution in the Definition and Management of Primary Nonresponse. Inflamm Bowel Dis (2015) 21:182–97. doi: 10.1097/MIB.0000000000000202
Keywords: Crohn’s disease, primary non-response, infliximab, serum biomarkers, prediction
Citation: Li L, Chen R, Zhang Y, Zhou G, Chen B, Zeng Z, Chen M and Zhang S (2021) A Novel Model Based on Serum Biomarkers to Predict Primary Non-Response to Infliximab in Crohn’s Disease. Front. Immunol. 12:646673. doi: 10.3389/fimmu.2021.646673
Received: 27 December 2020; Accepted: 07 July 2021;
Published: 22 July 2021.
Edited by:
Silvano Sozzani, Sapienza University of Rome, ItalyReviewed by:
Gerard Dijkstra, University Medical Center Groningen, NetherlandsBarbara Bottazzi, University of Milan, Italy
Copyright © 2021 Li, Chen, Zhang, Zhou, Chen, Zeng, Chen and Zhang. This is an open-access article distributed under the terms of the Creative Commons Attribution License (CC BY). The use, distribution or reproduction in other forums is permitted, provided the original author(s) and the copyright owner(s) are credited and that the original publication in this journal is cited, in accordance with accepted academic practice. No use, distribution or reproduction is permitted which does not comply with these terms.
*Correspondence: Shenghong Zhang, c2hlbmdob25nemhhbmdAMTYzLmNvbQ==; emhzaGgzQG1haWwuc3lzdS5lZHUuY24=
†These authors have contributed equally to this work and share first authorship