- 1Department of Anesthesiology and Critical Care Medicine, Tianjin Nankai Hospital, Tianjin Medical University, Tianjin, China
- 2Department of Anesthesia, The First Affiliated Hospital of Wenzhou Medical University, Wenzhou, Zhejiang, China
- 3Department of General Practice, Central Health Center of Yayang Town, Taishun County (Yayang Branch of Medical Community of Taishun County People’s Hospital), Wenzhou, Zhejiang, China
- 4Key Laboratory of Intelligent Treatment and Life Support for Critical Diseases of Zhejiang Province, Wenzhou Medical University, Wenzhou, China
Background: Septic shock occurs when sepsis is related to severe hypotension and leads to a remarkable high number of deaths. The early diagnosis of septic shock is essential to reduce mortality. High-quality biomarkers can be objectively measured and evaluated as indicators to accurately predict disease diagnosis. However, single-gene prediction efficiency is inadequate; therefore, we identified a risk-score model based on gene signature to elevate predictive efficiency.
Methods: The gene expression profiles of GSE33118 and GSE26440 were downloaded from the Gene Expression Omnibus (GEO) database. These two datasets were merged, and the differentially expressed genes (DEGs) were identified using the limma package in R software. Gene Ontology (GO) and Kyoto Encyclopedia of Genes and Genomes (KEGG) pathway enrichments of DEGs were performed. Subsequently, Lasso regression and Boruta feature selection algorithm were combined to identify the hub genes of septic shock. GSE9692 was then subjected to weighted gene co-expression network analysis (WGCNA) to identify the septic shock-related gene modules. Subsequently, the genes within such modules that matched with septic shock-related DEGs were identified as the hub genes of septic shock. To further understand the function and signaling pathways of hub genes, we performed gene set variation analysis (GSVA) and then used the CIBERSORT tool to analyze the immune cell infiltration pattern of diseases. The diagnostic value of hub genes in septic shock was determined using receiver operating characteristic (ROC) analysis and verified using quantitative PCR (qPCR) and Western blotting in our hospital patients with septic shock.
Results: A total of 975 DEGs in the GSE33118 and GSE26440 databases were obtained, of which 30 DEGs were remarkably upregulated. With the use of Lasso regression and Boruta feature selection algorithm, six hub genes (CD177, CLEC5A, CYSTM1, MCEMP1, MMP8, and RGL4) with expression differences in septic shock were screened as potential diagnostic markers for septic shock among the significant DEGs and were further validated in the GSE9692 dataset. WGCNA was used to identify the co-expression modules and module–trait correlation. Enrichment analysis showed significant enrichment in the reactive oxygen species pathway, hypoxia, phosphatidylinositol 3-kinases (PI3K)/Protein Kinase B (AKT)/mammalian target of rapamycin (mTOR) signaling, nuclear factor-κβ/tumor necrosis factor alpha (NF-κβ/TNF-α), and interleukin-6 (IL-6)/Janus Kinase (JAK)/Signal Transducers and Activators of Transcription 3 (STAT3) signaling pathways. The receiver operating characteristic curve (ROC) of these signature genes was 0.938, 0.914, 0.939, 0.956, 0.932, and 0.914, respectively. In the immune cell infiltration analysis, the infiltration of M0 macrophages, activated mast cells, neutrophils, CD8 T cells, and naive B cells was more significant in the septic shock group. In addition, higher expression levels of CD177, CLEC5A, CYSTM1, MCEMP1, MMP8, and RGL4 messenger RNA (mRNA) were observed in peripheral blood mononuclear cells (PBMCs) isolated from septic shock patients than from healthy donors. Higher expression levels of CD177 and MMP8 proteins were also observed in the PBMCs isolated from septic shock patients than from control participants.
Conclusions: CD177, CLEC5A, CYSTM1, MCEMP1, MMP8, and RGL4 were identified as hub genes, which were of considerable value in the early diagnosis of septic shock patients. These preliminary findings are of great significance for studying immune cell infiltration in the pathogenesis of septic shock, which should be further validated in clinical studies and basic studies.
1 Introduction
Sepsis is a life-threatening organ dysfunction disease that is characterized as an unusual systemic reaction to what is sometimes an otherwise ordinary infection (1). Septic shock is a subtype of sepsis with circulatory and cellular or metabolic dysfunction (2). Both sepsis and septic shock lead to high mortality and morbidity rates and represent a heavy societal burden across the world (3). By identifying the root causes and relevant diagnostic biomarkers of septic shock, we can intervene early and effectively, resulting in a significant reduction in the mortality rates associated with sepsis. Therefore, it is crucial to research the biological mechanisms involved in septic shock and explore the potential indicators for early diagnosis.
It is believed that immune cells and immune-related pathways significantly contribute to the development of septic shock; this is based on growing clinical and experimental evidence supporting the association between the overactivation of innate immune effector cells and uncontrolled inflammation leading to tissue damage and organ failure in severe septicemia observed in both human subjects and animal models (4–6). Judith Hellman et al. proposed that sepsis disrupts the balance of the redox state toward a pro-oxidative state. This is characterized by the excessive production of reactive oxygen and nitrogen species, mitochondrial dysfunction, and damage to the antioxidant system. It results in microvascular dysfunction and multiple organ failure (7). Research has shown that activating the phosphatidylinositol 3-kinases (PI3K)/AKT/mammalian target of rapamycin (mTOR) pathway and inhibiting the nuclear factor-κβ (NF-κβ) signaling pathway can have a protective effect on organs in the pathological and physiological state of sepsis (8).
To better understand the dysregulated immune responses to infection that are sepsis and septic shock, biomarkers have been evaluated for providing information beyond what is available using other metrics, and could therefore help inform clinical decision-making and potentially improve patient management (9). It has been reported that several proposed biomarkers, such as C-reactive protein (CRP) and procalcitonin (PCT), could complement clinical evaluation and help physicians to make therapeutic decisions (10); however, the role of biomarkers in septic shock diagnosis remains undefined (11). In addition, there are few prognosis specificity markers that have performed well in preventing septic development from reaching a severe phase (12–14). Therefore, this study aims to explore the value of immune-related genes in the early diagnosis of septic shock at the genetic level. A better understanding of the role of immune regulatory genes in promoting the diagnosis of septic shock is of great significance and can provide new ideas for the accurate diagnosis and treatment of septic shock.
In this study, we conducted a comprehensive bioinformatics analysis based on a large sample size to screen for promising gene markers for septic shock. The microarray datasets for septic shock were downloaded from the Gene Expression Omnibus (GEO) database. Subsequently, differentially expressed genes (DEGs) analyses were performed for both the patients with septic shock and control participants. In sepsis, genes play their role through networks of co-expression genes with alike biological roles. The recognition of co-expressing patterns can offer more inspiration on septic pathways. Therefore, we also analyzed the relationship between gene expression and phenotypes via weighted gene co-expression network analysis (WGCNA) in septic shock (15, 16). Then, hub genes screened from DEGs for septic shock and those identified using WGCNA were intersected and analyzed. Functional and pathway analyses were also performed, and the relationships of proteins were investigated.
The infiltration of inflammatory cells and activation of immune-related pathways are critical features in sepsis and septic shock (17, 18). In addition, inflammatory cytokines such as interleukin-1β (IL-1β), IL-6, IL-18, tumor necrosis factor alpha (TNF-α), and interferon-gamma (IFN-γ) have been reported to play an essential role in the development of sepsis and septic shock (19, 20). However, the changes in immune cell types in response to septic shock are still unclear. The critical role of particular immune cells and immune-related pathways in septic shock was confirmed by these molecular and cellular experiments, but few studies have been conducted that explore the correlation of genes and immune cells or the overall landscape in big data. Hence, we conducted an overall description of the immune-related landscape of septic shock in this study. Finally, the diagnostic values of hub genes were evaluated by ROC analyses and the measurement of mRNA concentration and protein expression levels, and we also conducted a preliminary verification of data credibility.
The results given in the present study could be conducive to comprehensively understanding septic shock pathogenesis, identifying the molecular mechanisms that are involved in the pathological process, and providing insights into novel treatment and therapeutic targets for drugs.
2 Materials and methods
2.1 Extraction of peripheral blood mononuclear cells from clinical blood samples
Our study is a forward-looking, observation-originated investigation involving septic patients from the intensive care unit (ICU) of the First Affiliated Hospital of Wenzhou Medical University from June 2021 to November 2021. All patients in our study had reached the consentaneous standards of the Third International Consensus Definitions for Sepsis and Septic Shock (Sepsis-3) (11). Septic shock patients exhibited persistently low blood pressure, which required vasopressors for the maintenance of MAP ≥ 65 mmHg and sera lactic acid levels > 2 mmol/L (18 mg/dL) despite sufficient fluid resuscitation. Patients who were < 18 years old or who had potential acute non-infection organic damage and shock were filtered out.
Blood samples were collected in ethylenediaminetetraacetic acid (EDTA) tubes immediately upon patient admission, before septic shock occurred. Blood samples from 30 control participants were also collected, followed by the immediate extraction of peripheral blood mononuclear cells (PBMCs). The isolation of PBMCs from whole blood is based on the density differences between PBMCs and other components of the peripheral blood. The origins of this method go back to the 1960s, when Arne Boyum first described the separation of white blood cells using Ficoll and other density gradient media. Since then, the technique has been refined, but the underlying principles have remained the same: by density gradient centrifugation, separation of blood components according to their density is possible with the help of a density gradient medium (e.g., Lymphoprep or Ficoll) containing sodium diatrizoate, polysaccharides, and water, reaching a density of 1,077 g/mL. The density gradient medium facilitates the aggregation of red blood cells. The medium is denser than lymphocytes, monocytes, and platelets, but less dense than granulocytes and red blood cells. Accordingly, erythrocytes and most of the granulocytes will sediment and pellet at the bottom of the tube after centrifugation; after this phase, the density gradient medium is found. The top layer consists of plasma and platelets. Mononuclear cells band at the interface between the plasma and the density gradient medium. Subsequently, two washing steps at a lower speed help to remove the remaining platelets (21). The PBMCs obtained were stored at –80°C for subsequent experimental research.
2.2 Real-time quantitative PCR
The RNA from cells was extracted using Trizol (Invitrogen) and reverse transcribed into cDNA through a synthesis kit containing specific primers and SYBR green reaction mix (Takara Clontech, Dalian, Japan). Specific primers were customized by Sangon Biotechnology Co., Ltd. (Shanghai, China), with the presented sequence shown in Table 1. Real-time qPCR was performed, followed by the calculation of relative gene expression levels using the 2–ΔΔCT approach. Each primer sequence is presented in Table 1.
2.3 Western blotting
Proteins were extracted from PBMCs, after which the protein concentration was measured using a bicinchoninic acid (BCA) protein assay (Beyotime, P0012, Shanghai, China). An equal number of proteins (20 μg) per sample was separated by 10% sodium dodecyl sulphate-polyacrylamide gel electrophoresis (SDS-PAGE), then transferred to a Polyvinylidene difluoride (PVDF) membrane (Millipore). Subsequently, the membranes were incubated with anti-CD177 (HUABIO, ER65526, 1:1,000) and anti-MPP8 (HUABIO, ER63980, 1:1,000) overnight after being blocked, firstly by 5% skim milk at room temperature for 2 h, and thereafter by a horseradish peroxidase (HRP)-conjugated secondary antibody. The images were scanned and quantified using Image Lab software (Bio-Rad).
2.4 Data collection and ethics statement
Relative clinical information was documented, including patient demographics and diagnostic results, and also their PCT, lactic acid, and CRP levels. Acute Physiology and Chronic Health Evaluation (APACHE) II scores were recorded at the onset of septic shock and sequential organ failure assessment (SOFA) scores were recorded in the first 24 hours after the diagnosis of septic shock. Clinical test results and hospital death rate data were also collected within the first 24 hours of ICU admission. Our research (clinical trial registration number: ChiCTR2100053564) was approved by the ethics committee of the First Affiliated Hospital of Wenzhou Medical University, Zhejiang, China, and recorded at ClinicalTrials.gov., in accordance with the protocols in the Declaration of Helsinki, with informed consent provided by each participating patient.
2.5 Microarray data
The gene expression profiles of GSE33118, GSE26440 (22), and GSE9692 (23) were downloaded from the GEO database (http://www.ncbi.nlm.nih.gov/geo/) (24). The GSE33118 data collection includes the gene expression profiles of 20 septic shock patients and 42 control participants; blood specimens were obtained within 24 h of the septic shock diagnosis. The samples in the GSE26440 data collection were for 98 children with septic shock and 32 control pediatric patients. Children who were 10 years old in the pediatric intensive care unit (PICU) and who met the pediatrics-specific standards for septic shock were enrolled (25). After obtaining written informed consent, blood specimens were acquired within 24 h of their initial presentation to the PICU. The specimens in the GSE9692 data collection were also collected; these were for 42 septic shock patients who were minors, and 15 healthy donors. The patients who had one or more serious coexisting diseases or who were undergoing immunosuppression treatment were filtered out. The septic shock diagnoses were referred to the American College of Chest Physicians/Society of Critical Care Medicine (ACCP/SCCM) standards.
2.6 Identification of DEGs
We downloaded the GSE33118 datasets, which contained the expression profiles of 150 groups of patients, and GSE26440, which contained the expression profiles of the normal group (n = 42) and the disease group (n = 108) that were related to septic shock from the GEO database. To obtain the critical information from the two datasets, the merging and pre-processing of raw data were conducted using the surrogate variable analysis (SVA) package (26) from R software. Principal component analysis (PCA), a multivariate regression analysis, was used to distinguish samples with multiple measurements (27, 28). We used PCA to show the uniformity of the two datasets after correction. In addition, the limma package (29) was used to conduct a differential analysis of the combined database comprising these two databases; gene screening conditions with p < 0.05 and | Log2FC | > 1 were used as the selection criteria for filtering septic shock DEGs.
2.7 Functional enrichment analysis of DEGs and PPI network construction
Gene Ontology (GO), a major bioinformatics tool for annotating genes and analyzing biological process, and Kyoto Gene Genome Encyclopedia (KEGG), a database resource for understanding high-level functions and biological systems from large-scale molecular datasets generated by high-throughput experimental technologies, were used to further investigate the biological functions and signaling pathways involved in the occurrence and development of septic shock. The protein–protein interaction (PPI) networks of the septic shock DEGs were predicted through the retrieval pool Search Tool for the Retrieval of Interacting Genes/Proteins (STRING). The statistical significance was set at a minimum overlap of ≥3 and p ≤ 0.01. Afterward, the Cytoscape program was used to establish and realize the visualization of molecule mutual effect nets (30). In the PPI network, the target proteins were represented by nodes, whereas the predicted or validated interactions between proteins were represented by edges.
2.8 Lasso regression and Boruta feature selection process
Lasso logistic regression was used for feature selection of the diagnostic markers of disease. The Lasso algorithm uses the generalized linear model net (GLMNet) software package (version 4.1–2). In addition, Boruta is a feature selection algorithm that randomly disrupts each real feature in order, evaluates the importance of each feature, and iteratively removes features with low correlation to find the best variable. In this study, the Boruta package (version 7.0.0) was used for feature selection, and 500 trees were constructed to further identify the diagnostic value of these biomarkers for disease.
2.9 WGCNA analysis
Through the establishment of a weight-added genetic co-expressing net, our team searched for co-expressed genetic modules and explored the correlation between gene networks and phenotypes, along with the core genes (15). The WGCNA-R packet (version 1.70–3) was adopted to establish the co-expression net of all genes in the dataset, and the genes with the top 5,000 variance were selected via such arithmetic for further assays, in which the soft liminal value was 14. The weight-added adjacent matrix was converted into a topological overlap matrix (TOM) to speculate the net connection level. The adjacency matrix is a matrix composed of weighted correlation values between genes, whereas TOM is a new distance matrix that is transformed by the adjacency matrix to reduce noise and false correlation. The topological overlap matrix considers not only the direct relationship between genes, but also the possible multiple indirect relationships. This information can be used to construct a network for subsequent analysis.
The hierarchy clustering approach was adopted to establish the cluster tree framework of TOM matrix. Diverse offshoots of the cluster tree represent diverse genetic modules, and the diverse colors denote diverse modules. As per the weight-added association coefficient of genes, the genes were categorized in terms of their expressing features. Genes with similar features were classified into a module, and massive genes were divided into multi-modules via genetic expressing features. After genes were clustered, a heatmap was plotted to visualize and calculate the intermodule correlation. The correlation between modules and clinical traits was further evaluated to determine the modules associated with septic shock for analysis.
2.10 Veen and circos analysis
The Venn diagram, which is a widely used diagram that exhibits the relationship between multiple sets, is probably the most intuitive form of data visualization, superior to heat maps and tables when the number of sets is fewer than five (31). In addition, we used the Circlize and Corrplot packages to plot the interaction diagram of core genes expressing positive and negative correlation.
2.11 GeneMANIA analysis
GeneMANIA is an elastic, easy-to-use PPI net construction data center for the visualization of function networks between genes and the analysis of gene roles and mutual effects. The database can set up the data resource of genetic nodal points and has various biological information assay approaches, such as physical mutual effect, genetic co-expression, genetic co-location, genetic enriching assay, and database forecast. In this study, the core gene net was generated using GeneMANIA to explore its possible mechanism in septic shock.
2.12 Gene set difference analysis
Gene set difference analysis (GSVA) is a non-parameter, non-supervised approach for assessing the enrichment of transcriptomic genomes. Through the all-round scoring of the intriguing genetic sets, GSVA transforms the genetic-level variations into pathway-level variations and afterwards judges the biology role of the samples. The genetic sets used in this study were acquired from Molecular Signatures Data Center 7.0, and the GSVA arithmetic was adopted for the all-round scoring of every genetic set to assess the underlying biological function variations of diverse specimens.
2.13 Receiver operating characteristic curve analysis
MedCalc statistical software (www.medcalc.org) was used to carry out receiver operating characteristic (ROC) curve assay and to identify the idiosyncrasy, susceptibility, probability ratio, and favorable and unfavorable prediction scores for all probable liminal values of the ROC curve. The genetic scores were forecasted as per the ROC curve assay.
2.14 CIBERSORT analysis of immune infiltration
CIBERSORT algorithm (32), which is a gene expression-based arithmetic that represents cell composition based on pre-processed gene expression profiles, was utilized to evaluate immune cell infiltration in septic shock. LM22 (22 immune cell types) in CIBERSORT is a signature gene expression matrix used to estimate the proportion of leukocytes. Data from control participants and patients with septic shock were analyzed using the CIBERSORT algorithm to infer the relative proportions of 22 immune infiltrating cells, with the sum of all estimated immune cell type scores in each sample equal to 1. In this study, the influence of genes on immune infiltration was evaluated. CIBERSORT (http://CIBERSORT.stanford.edu/) was used to quantify the level of immune cell infiltration in each sample, and Spearman’s correlation analysis was performed on gene expression level and immune cell content.
2.15 Statistical analysis
Our team undertook statistic assays via GraphPad Prism 8, SPSS 21.0, and R program 4.0. The normal distribution successive variates were expressed as averages ± SD and were studied via an independent Student’s t-test or one-way ANOVA. P-values < 0.05 were deemed to be statistically significant.
3 Manuscript formatting
3.1 Identification of DEGs, functional enrichment analysis, and construction of PPI networks
Datasets related to septic shock were downloaded from the GEO database, then the GSE33118 and GSE26440 datasets were merged. A total of 150 gene expression profiles were included, containing 108 septic shock patients and 42 controls participants. The batch effect was eliminated by sva package, which is presented in a principal component analysis (PCA) diagram (Figures 1A, B). In total, 975 differentially expressed genes (DEGs) were identified by screening via the limma package in R software, of which 30 were upregulated genes (Figure 1C, red dots) (p < 0.05).
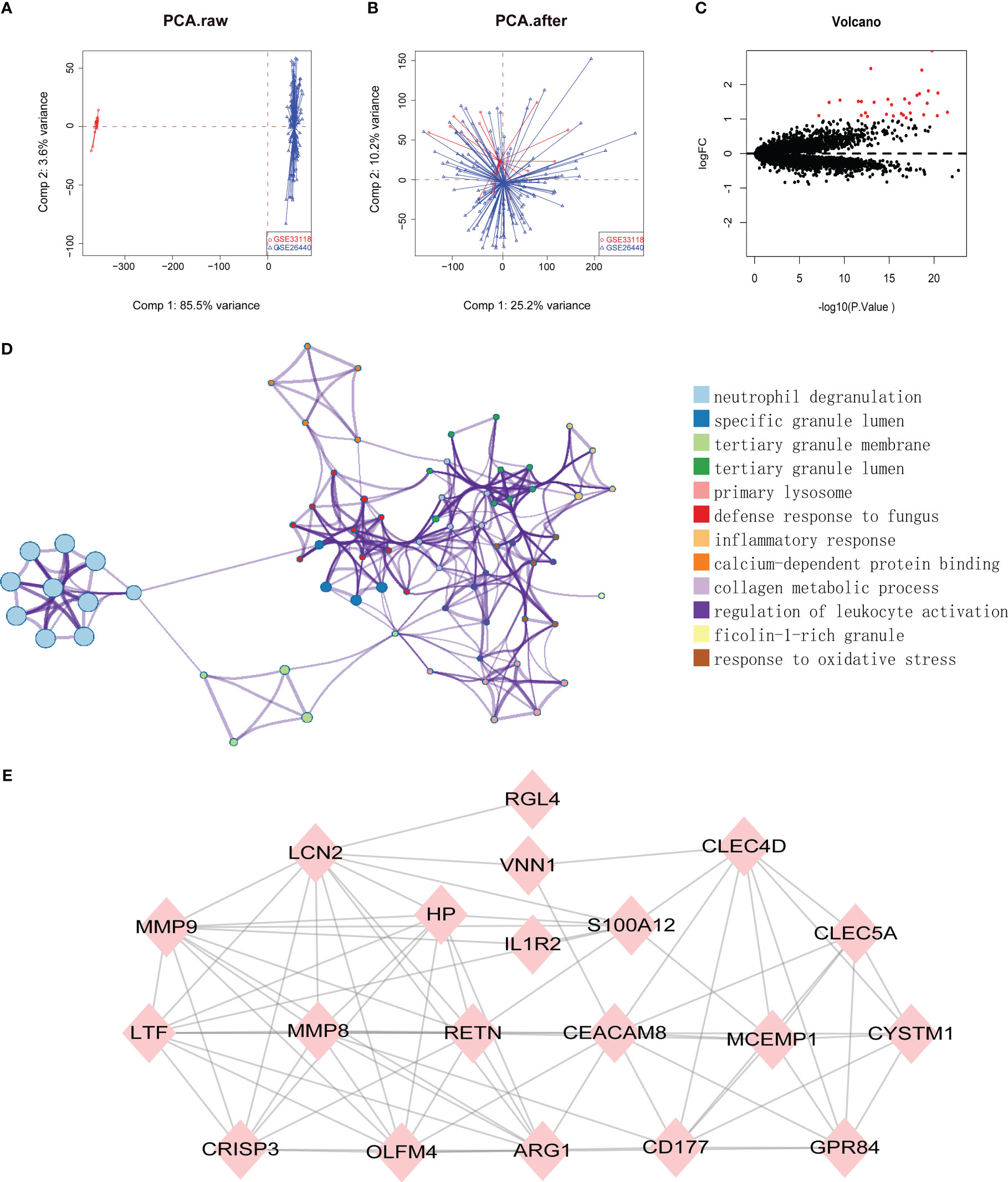
Figure 1 (A, B) SVA algorithm was used to correct the chip, and PCA (Principal Component Analysis) diagram was used to show the results after correction. The results showed that the interchip batch effect was eliminated after SVA algorithm correction. (C) Volcano plot of gene expression profile data between normal group and septic shock group. Red dots: significantly up-regulated genes; Black dots: nondifferentially expressed genes. P<0.05 and |log2 Foldchange |>1 were considered as significant. (D) The classification netplot denoted the associations of genes and GO terms as a net to indicate the enriched pathways, including neutrophil degranulation, SGL, TGL, and primary Lysosome and other pathways. (E) The PPI networks based on DEGs involved 30 key genes.
Subsequently, GO functional enrichment analysis and KEGG pathway analysis were conducted, suggesting that neutrophil degranulation, specific granule lumen, tertiary granule membrane, tertiary granule lumen, and primary lysosome and other pathways may play important roles in septic shock (Figure 1D). Using the PPI network, 30 genetic variations that may be associated with septic shock were identified. They were CYSTM1, MCEMP1, GYG1, MMP8, HP,HPR, GPR84, RGL4, CD177, RETN, BMX, CLEC5A, CA4, IL1R2, S100A12, ARG1, PFKFB3, ANKRD22, ANXA3, CLEC4D, MMP9, OLFM4, MS4A4A, VNN1, LCN2, TDRD9, LTF, OLAH, CEACAM8, and CRISP3. These are shown in Figure 1E.
3.2 Identification of hub genes
To further identify the hub genes among the differential genes, we combined Lasso regression and Boruta feature selection algorithm to screen out the characteristic genes of septic shock patients. The results of Lasso regression showed that six differential genes were identified as hub genes of septic shock (Figure 2A, Supplementary Table 1), 24 differential genes were identified as the hub genes of septic shock by Boruta algorithm (Figure 2B, Supplementary Table 2), and six hub genes were screened out after the two were intersected (Table 2): the CD177 molecule (CD177), C-type lectin domain containing 5A (CLEC5A), cysteine rich transmembrane module containing 1 (CYSTM1), mast cell expressed membrane protein 1 (MCEMP1), matrix metallopeptidase 8 (MMP8), and ral guanine nucleotide dissociation stimulator like 4 (RGL4).
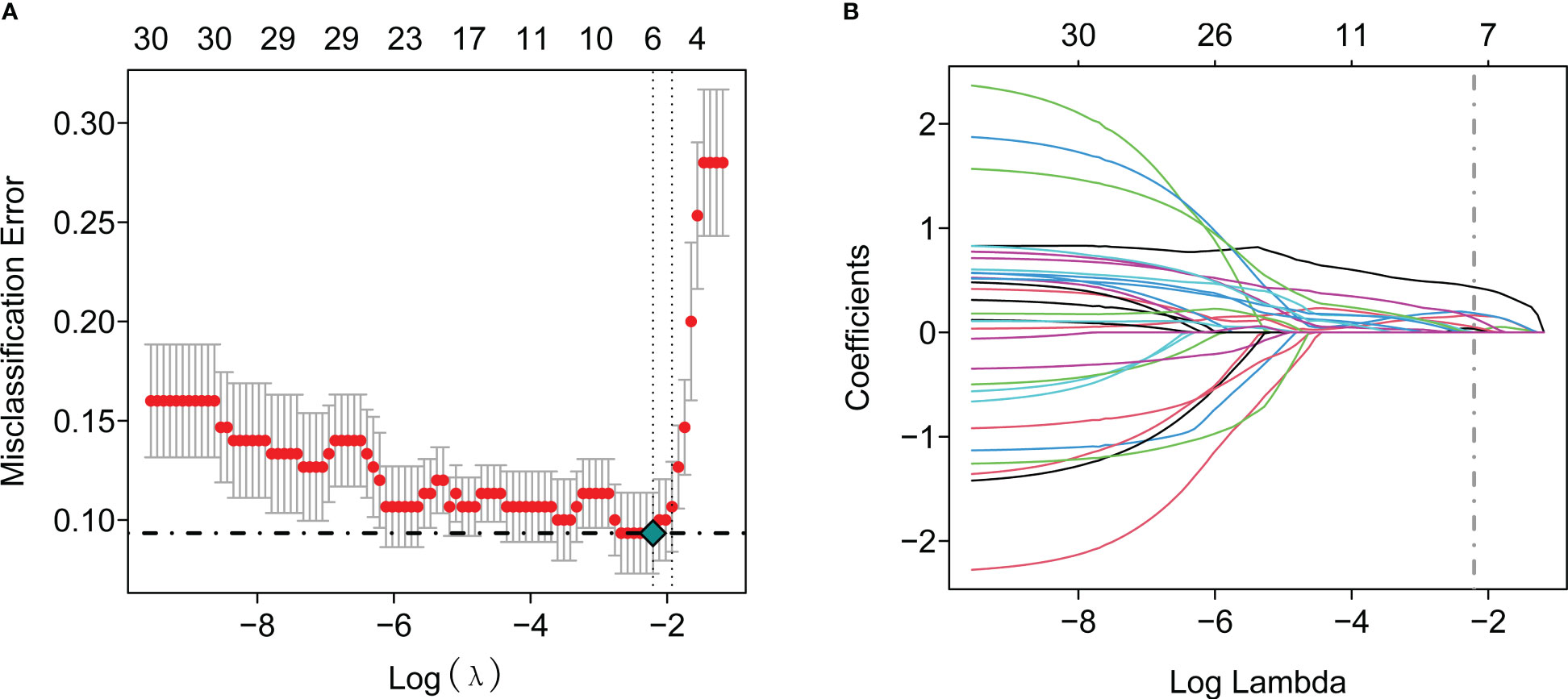
Figure 2 The Lasso regression and Boruta feature selection algorithm were used to screen out the characteristic genes in septic shock. (A) 6 differential genes were identified as key genes of septic shock by Lasso regression. (B) 24 differential genes were identified as key genes of septic shock by Boruta algorithm.
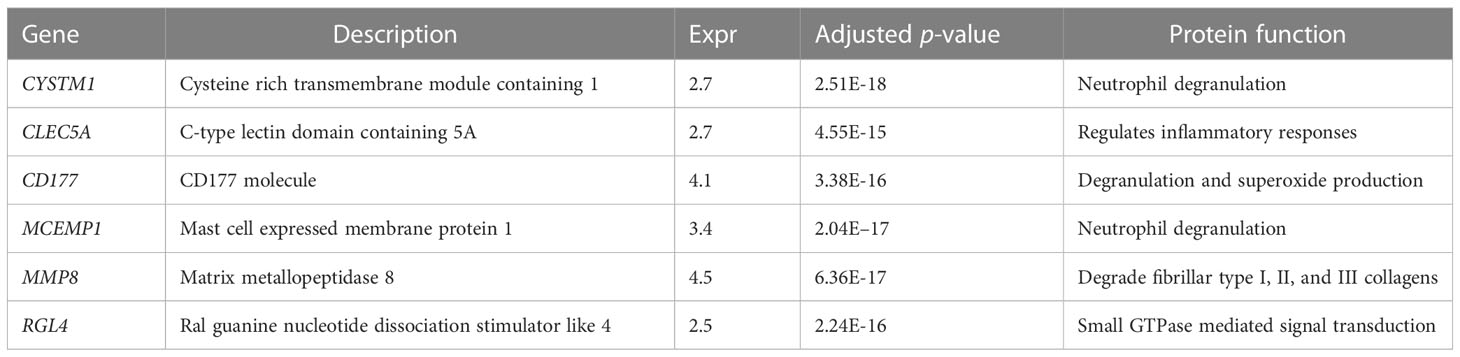
Table 2 The six genes screened out by Lasso regression and Boruta selection algorithm in septic shock.
3.3 Construction of co-expression modules and module–trait correlation
Raw microarray data from GSE9692 were used to construct the gene co-expression networks through WGCNA package to prune the gene modules of co-expression and explore the relationship between gene network and clinic features in the network. In samples of control and septic shock groups, there were no outliers detected in the expression matrix of the top 5,000 genes, whose expression variance was within the first quartile. To construct the correlation matrix, the Pearson correlation coefficient was utilized to calculate the correlation coefficient of expression between genes. An appropriate soft-thresholding power β = 14 was determined in the WGCNA package; here, the soft thresholding power is based on two criteria: the lowest power at which the scale-free topology fit index reached 0.90; and the connectivity measurements decrease considerably (Figures 3A, B).
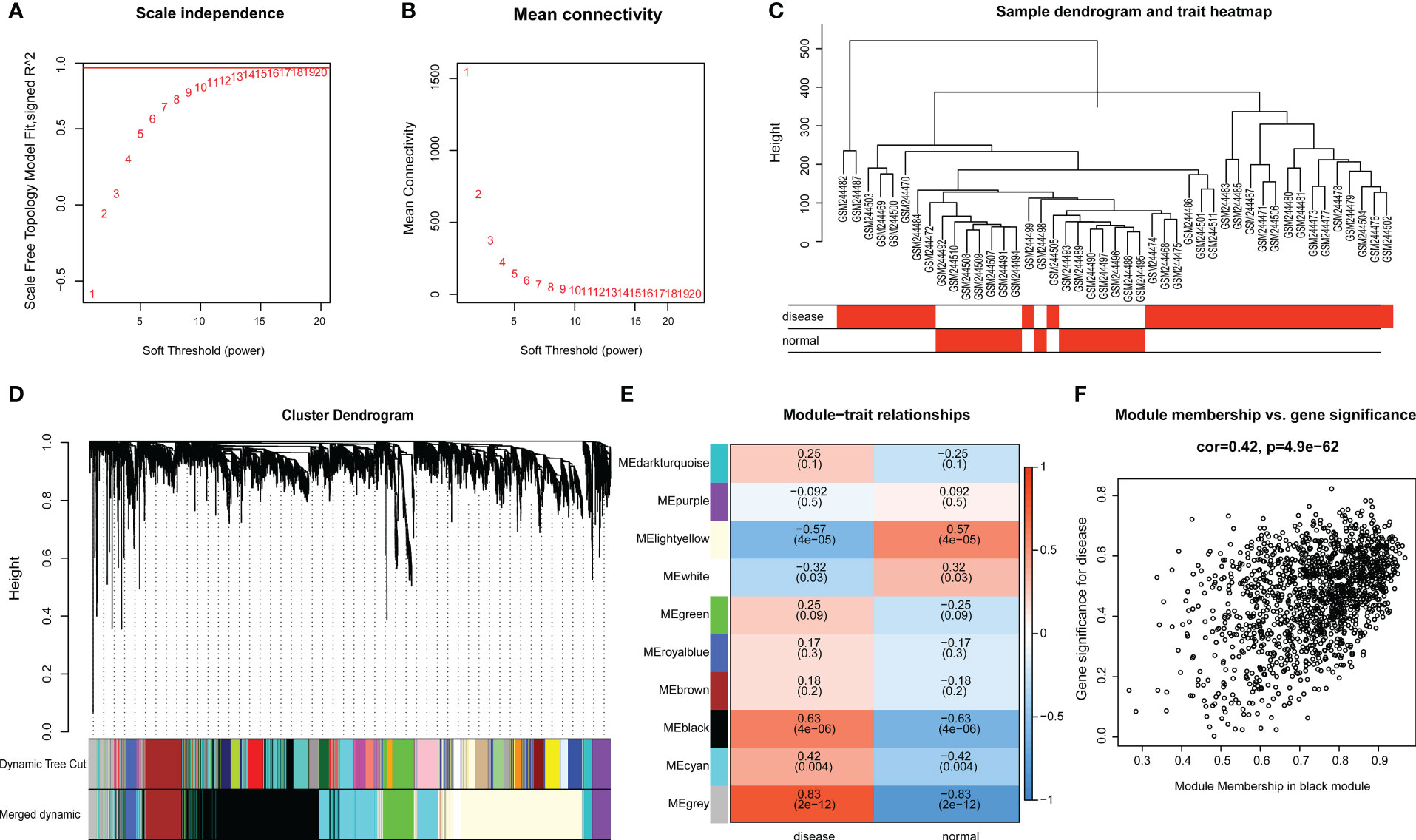
Figure 3 (A, B) Scale-free network construction in the co-expression network. Scale independence and mean connectivity analysis (β = 14). (C) Clustering tree construction. Merging of modules with similar expression profiles. (D) Module identification. Network dendrogram based on differential measurement and identified module colors, each node represents a gene, and the vertical axis denotes the degree of topological differences between genes, i.e. a larger distance between vertical coordinates indicates a larger topological difference, meaning a weaker co-expression correlation; the horizontal axes represent different modules, with each color denoting a module and the width of color bar indicating the gene number in the module; Dynamic Tree Cut represents modules initially obtained through average-linkage hierarchical clustering. Merged colors denote the reconstructed modules after merging similar modules. (E) Correlation of module and trait. Heatmap for the correlation between modules and septic shock traits. The horizontal axes represent different modules. The color of each cell indicates the corresponding module–trait correlation, a deeper red color suggesting stronger positive correlation and a deeper blue color suggesting stronger negative correlation. The value in each cell denotes the correlation score, and the value in the bracket below denotes the significance (P-value). (F) The association between modules and disease phenotype, the scatter plot of module eigengenes in the black module (COR = 0.42, P = 4.9E-62).
The topological overlap matrix (TOM) was constructed from the adjacency matrix and used to cluster genes with high topological overlap into modules. The clustering dendrogram was then pruned using the dynamic tree cut method to further classify the modules (Figure 3C). However, we considered that some genes with high similarity were not clustered together, owing to the number of initially classified modules being relatively large. To avoid excessive module numbers that would complicate subsequent analysis, the module eigengenes in each module were clustered using a similarity threshold of 0.25 to group similar modules together. Finally, gene modules were detected based on TOM matrix, and 10 genetic modules were identified herein. They were the black (1426), brown (348), cyan (792), dark-turquoise (94), green (317), gray (283), light-yellow (1394), purple (166), royal-blue (108), and white (72) modules (Figure 3D).
The module–trait relationship heatmap (Figure 3E) was generated by analyzing the correlation between the constructed modules and traits and calculating their significance. The BLACK modules were found to be significantly associated with disease traits, indicating their role in the pathogenesis and progression of septic shock. Through further analysis of the association between modules and features, we discovered that the BLACK module exhibited the highest correlation with disease phenotype (COR = 0.42, p = 4.9E-62) (Figure 3F). Therefore, the BLACK module was selected for the subsequent analysis as septic shock-correlated modules.
3.4 Functional classification and pathway enrichment of hub genes
We used the six hub genes that were identified as hub genes (shown in Table 2) to intersect with the BLACK module and found that all six hub genes intersected with the BLACK module, indicating that the results were in line with expectations (Figure 4A).
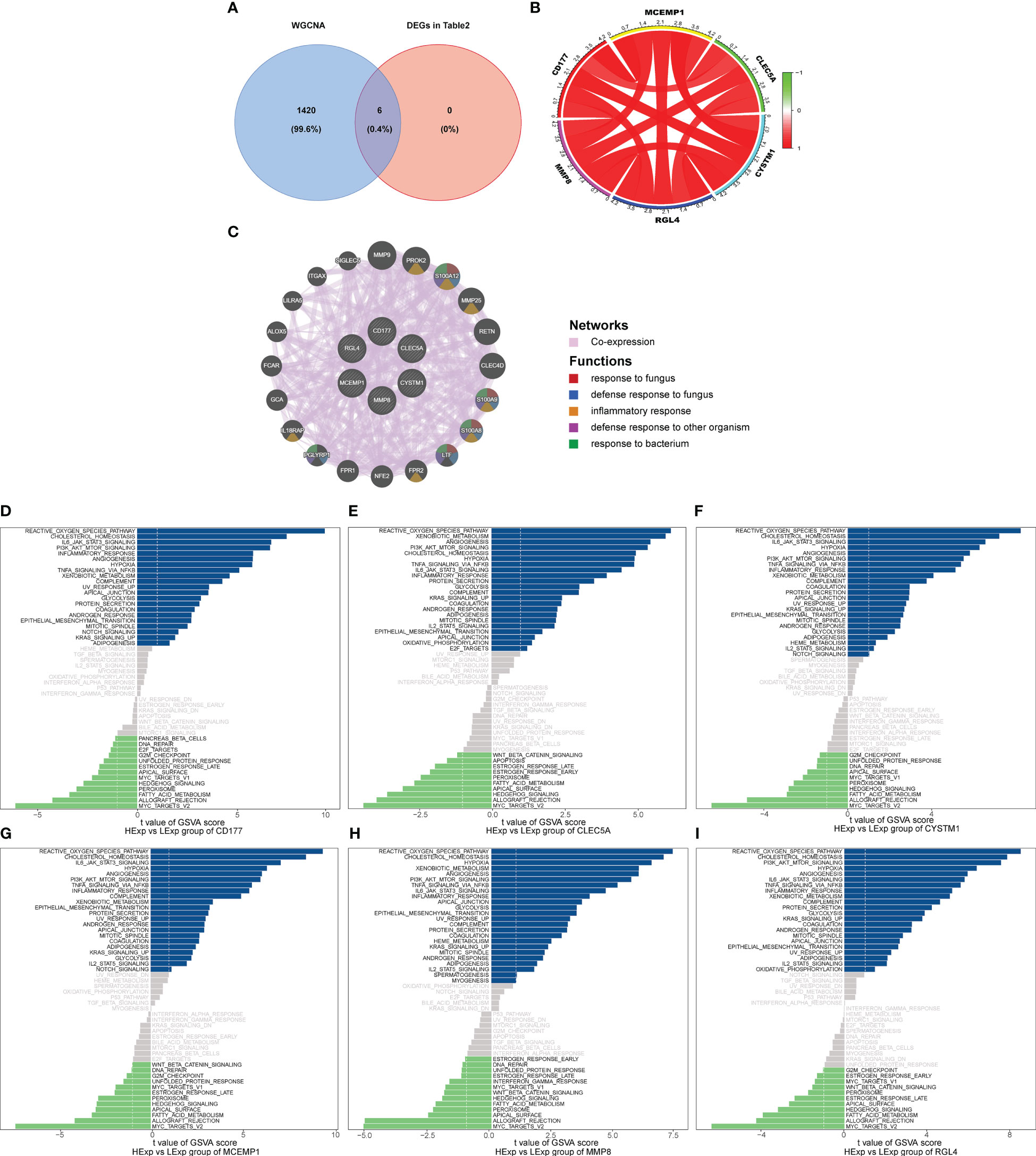
Figure 4 (A) Venn analysis for overlap of BLACK module target genes and six key DEGs (Identified from the intersection of Lasso regression and Boruta feature selection algorithm). (B) The interaction of positive and negative core genes was identified by circlize package and corrplot package. (C) The core gene net was generated through GeneMANIA to explore functions, including response to fungus, defense response to bacterium, inflammatory response, defense response to other organism and response to bacterium. The GSVA arithmetic was adopted for the all-round scoring of every genetic set, (D) CD177, (E) CLEC5A, (F) CYSTM1, (G) MCEMP1, (H) MMP8 and (I) RGL4 to assess the underlying molecule mechanisms where the key genes affect the development of septic shock, such as the reactive oxygen species pathway, hypoxia, PI3K/AKT/mTOR signaling, NFK-β/TNFa and IL6/JAK/STAT3.
Subsequently, we used the “circlize” package and the “corrplot” package to plot the interaction of positive and negative core genes. Green represents a negative correlation and red represents a positive correlation. We found that CD177, CLEC5A, CYSTM1, MCEMP1, MMP8, and RGL4 exhibited significant positive correlation with each other (Figure 4B). As shown in Figure 4C, the core gene net was generated through GeneMANIA to explore its possible mechanism in the disease, such as the response to fungus, defense response to bacterium, inflammatory response, and defense response to another organism.
Finally, we explored the specificity signal paths participating in the six hub genes to unveil the underlying molecule mechanisms where the hub genes impact the development of septic shock. GSVA then was used to determine the pathways in the following six genes: CD177, CLEC5A, CYSTM1, MCEMP1, MMP8, and RGL4. Among the abundant pathways involved in the six genes, we found several correlation pathways related to reactive oxygen species, namely hypoxia, PI3K/AKT/mTOR signaling, NFκ-β/TNFα, and IL6/JAK/STAT3, suggesting that the hub genes influence the development of septic shock by regulating these pathways (Figures 4D–I).
3.5 ROC analyses of biomarker genes
We also explored the predictive ability of hub genes on the occurrence and development of septic shock through the ROC curve of diagnostic efficacy verification. The higher the AUC value, the better the predictive ability. The results showed that the AUC values of the six core genes were CD177-AUC: 0.938 (0.899–0.978), CLEC5A-AUC: 0.914 (0.860–0.968), CYSTM1-AUC: 0.939 (0.900–0.979), MCEMP1-AUC: 0.956 (0.926–0.986), MMP8-AUC: 0.932 (0.890–0.974), and RGL4-AUC: 0.914 (0.863–0.965) (Figures 5A–F).
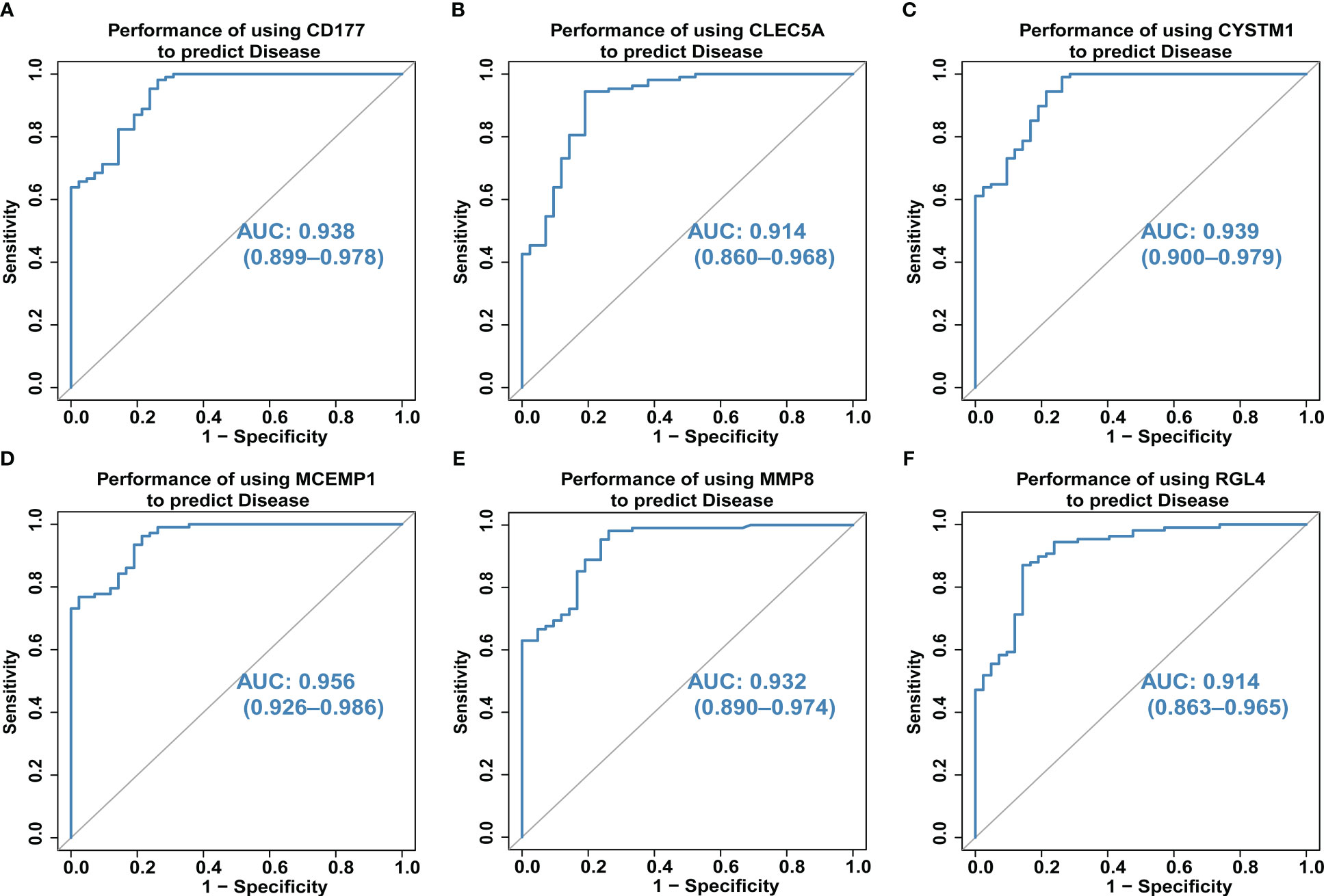
Figure 5 (A–F) The predictive ability of hub genes (CD177, CLEC5A, CYSTM1, MCEMP1, MMP8 and RGL4) on the occurrence and development of septic shock was explored through the ROC curve. The higher the AUC value, the better the predictive ability. The AUC for all six genes was >0.9 for ROC analysis.
3.6 Analysis of immune cell infiltration
The CIBERSORT algorithm was used to evaluate the proportion of different infiltrating immune cell types in the control and septic shock groups. The GEO expression array data were used to investigate the fraction of infiltrated immune cells. From 150 samples, 42 cases from the healthy donors group and 108 cases from septic shock group were eligible for CIBERSORT (p-value < 0.05). As shown in Figure 6A, the fraction of immune cells varied significantly among the samples and groups. The top five highest-infiltrating fractions in septic shock were M0 macrophages, activated mast cells, neutrophils, CD8+T cells, and I B cells. Follicular helper T cells and resting dendritic cells were present in lower quantities, inversely. Moreover, in general, higher proportions of M0 macrophages, activated mast cells, and neutrophils (p-value < 0.001) were found in the tissue of septic shock patients than in that of the healthy donors. In addition, we found a lower proportion of CD8+ T ceInaiveIlls, naive CD4+ T cells, gamma delta T cells, and resting natural killer (NK) cells in the tissue of septic shock patients than in that of the healthy donors (p-value < 0.05) (Figure 6B).
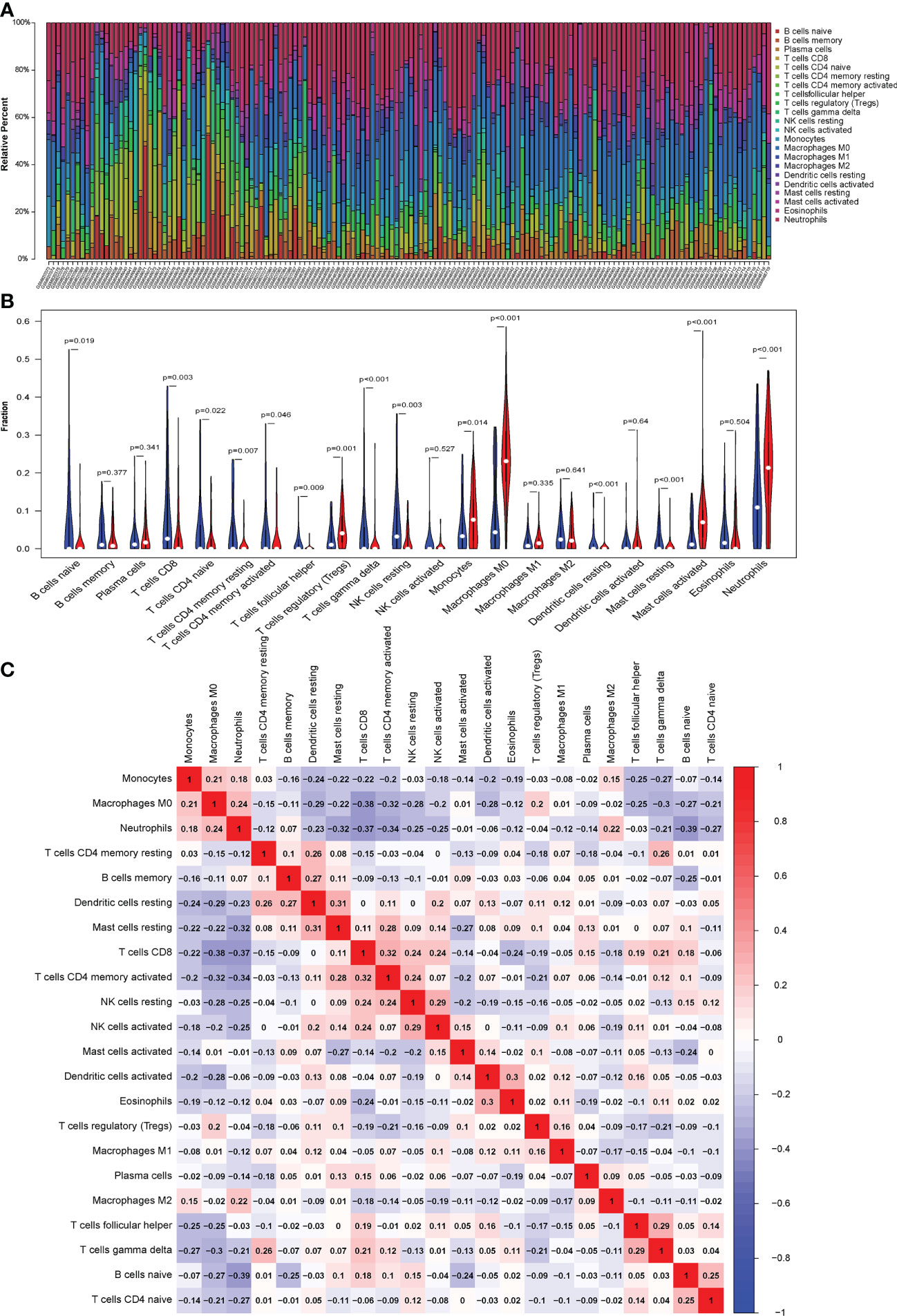
Figure 6 (A) CIBERSORT was used to quantify the fraction of 22 subsets of immune cells in septic shock. X axis: each GEO sample; Y axis: percentage of each kind of immune cells. (B) The violin graph shows the difference of immune infiltration between normal individuals and septic shock sufferers. The normal group is shown in blue and septic shock group is shown in red. P-Value < 0.05. (C) The co-expression patterns among fractions of immune cells. Red: positive correlation; blue: negative correlation.
We conducted a correlation analysis of infiltrated immune cells in septic shock and found multiple pairs of positively and negatively related immune cells (Figure 6C). The score represents the degree of correlation. CD8+ T cells and activated CD4+ memory T cells showed the most synergistic effIand naive B cells and neutrophils showed the most competitive effect.
3.7 Hub genes and immune infiltration
In this study, we analyzed the association between hub genes and immunity invasion in the septic shock dataset, so that the underlying molecular mechanism of hub genes affecting the development of septic shock was further explored. Correlation analysis with immune cells showed that all six hub genes were related to immune cells. In addition, the results in Figures 7A–F show that all six genes were positively related to M0 macrophages and neutrophils (p-value < 0.01) and negatively related to resting dendritic cells (p-value < 0.01).
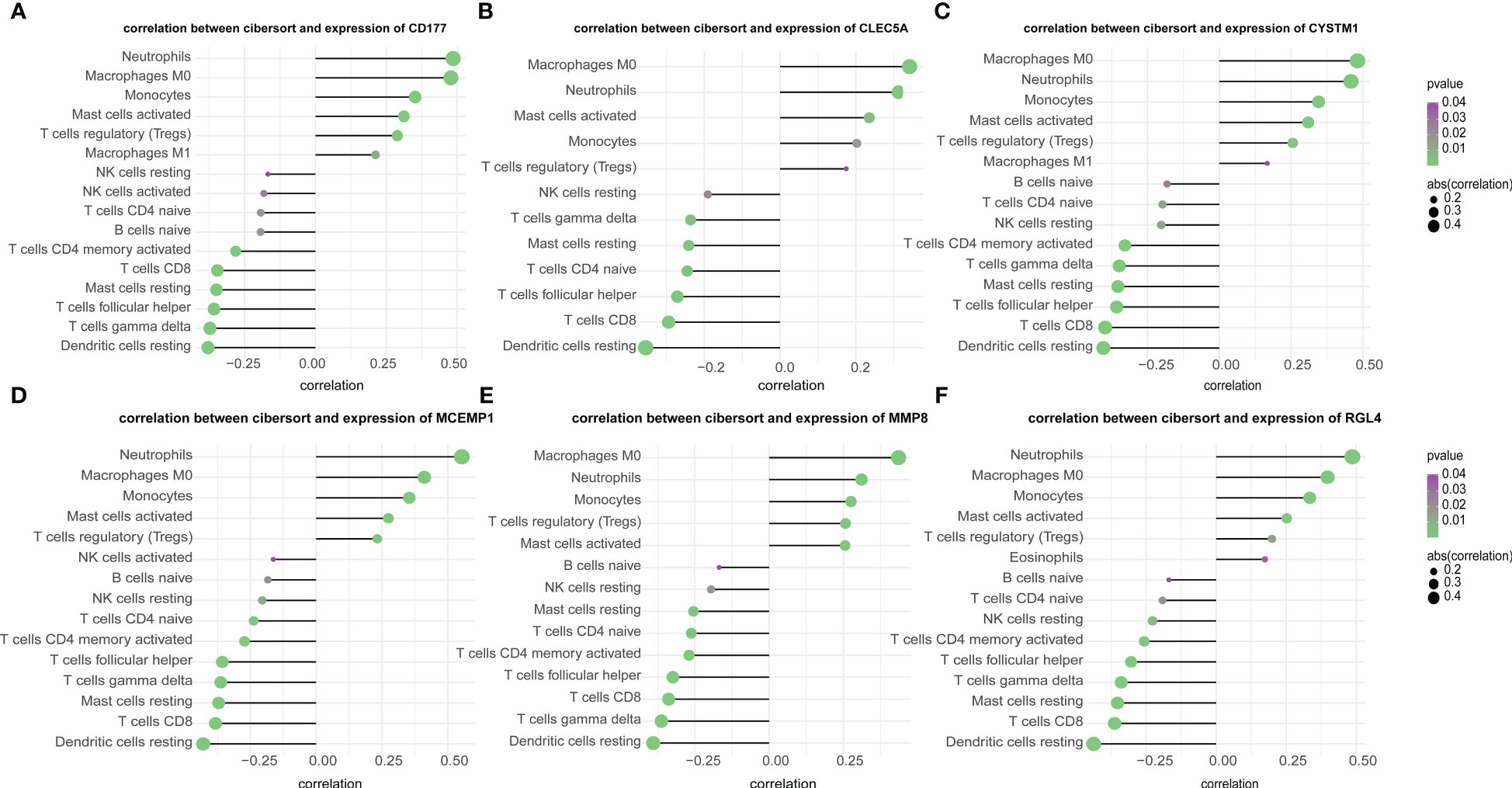
Figure 7 (A–F) Correlation of immune cells and 6 key genes. The all 6 genes (A) CD177, (B) CLEC5A, (C) CYSTM1, (D) MCEMP1, (E) MMP8 and (F) RGL4 were potently related to the similar kind of immunocyte content.
The microenvironment primarily comprises immunocytes, the extracellular matrix (ECM), various growing factors, inflammation factors, and specific physicochemical properties, all of which remarkably influence the diagnosis and susceptibility of clinical treatment of diseases. Therefore, we further supplemented the correlation analysis to explore the relationship between the six hub genes and immunomodulatory genes (extended data are in Supplementary Figures 1A–D). From the an integrated repository portal for tumor-immune system interactions (TISIDB) database of immune activation genes, immunosuppression, chemokines, and chemokine receptors, we extracted four types of immune-regulatory genes; of these, six hub genes were found by correlation analysis to be significantly associated with immune activation (i.e., CD27, CD68, ENTPD1, ICOS, TMEM173), immunosuppression (i.e., BTLA, CD244, CD274, HAVCR2, TGFB1), chemokines (i.e., CCL20, CXCL1, CXCL10, CXCL16.) and chemokine receptors (i.e., CCR1, CCR6, CXCR1, CXCR3).
According to those comprehensive bioinformatics analyses, the aforementioned six hub genes may be considered new septic shock biomarkers, and further research is needed to verify these preliminary findings.
3.8 Preliminary validation analysis of biomarker genes
To determine the clinical correlation of the discoveries made in this study, we undertook an RT-PCR analysis of peripheral blood mononuclear cells (PBMCs) from 30 septic shock patients and 28 healthy donors. The basic features are presented in Table 3. The age and sex distributional results had no statistical differences between these two groups. The most common infectious site was the lung (46.6%), then the abdomen and other organs (20.0%). Our studies revealed that the septic shock group displayed a greater total length of hospital stay and ICU stay in contrast to the healthy donors (p < 0.005). The APACHE II and SOFA values of the septic shock group were remarkably elevated in contrast to the healthy donors (p < 0.0001). The mRNA levels of CD177, CLEC5A, CYSTM1, MCEMP1, MMP8, and RGL4 were remarkably incremented in the septic shock group (n = 30) compared with the control group (n = 28) (Figures 8A–F). Meanwhile, the expression levels of CD177 and MMP8, as determined by Western blotting, were found to be upregulated in the PBMCs from septic shock patients (n = 4) compared with those from healthy donors (n = 4) (Figure 9). We found that these data exhibited a consistent trend with the bioinformatics analysis.
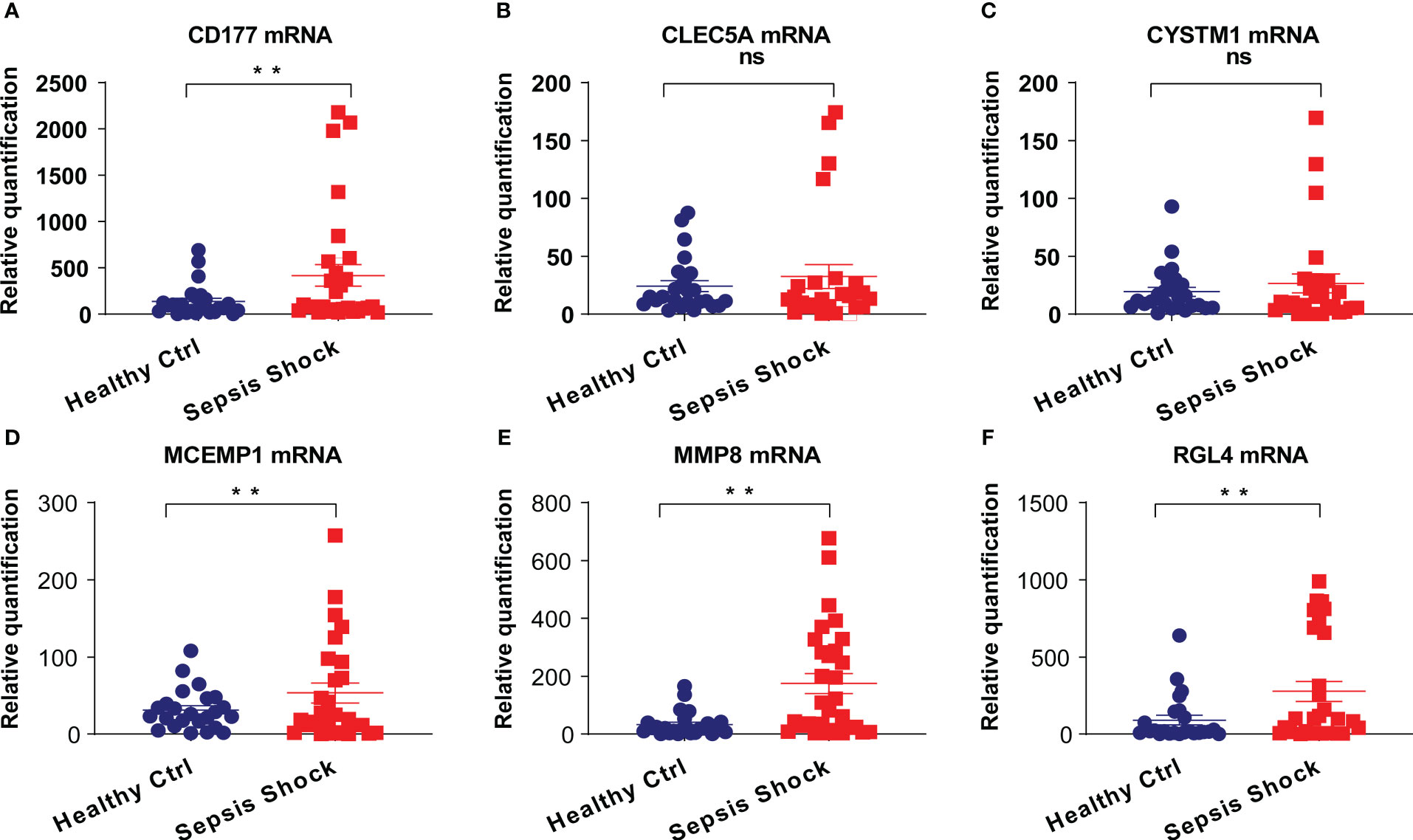
Figure 8 (A–F) The mRNA expression of CD177, CLEC5A, CYSTM1, MCEMP1, MMP8 and RGL4 were identified in PBMCs of sepsis sufferers and normal individuals via qRT-PCR. **P<0.05 vs relevant normal individuals; ns, no significance.
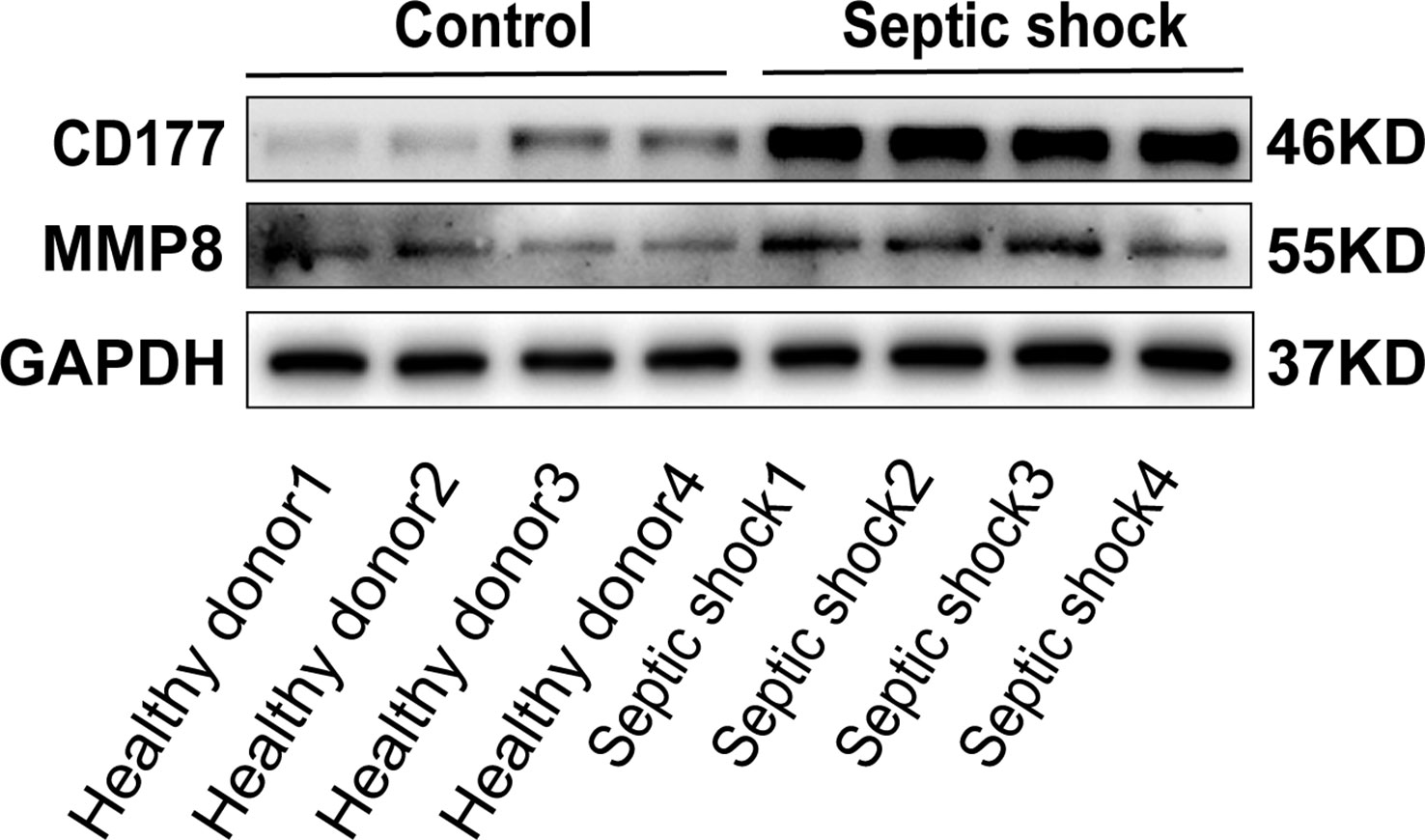
Figure 9 The protein expression of CD177 and MMP8 were identified in PBMCs of sepsis sufferers and health donors via Western blot.
4 Discussion
Currently, the mortality caused by sepsis and septic shock is still remarkably high. Early detection, suitable categorization, and timely treatment administered during the incipient period of septic shock are vital for decreasing the death rate. Biological information assays allow us to reveal the molecule mechanisms of illness onset and progression, offering a new and valid method to acquire the underlying diagnosis markers and treatment targets that can prevent and treat septic shock. In the present study, we first identified 30 genetic variations from the combined GSE33118 and GSE26440 datasets, and then combined Lasso regression and Boruta feature selection algorithm to screen out six key genes that are involved in septic shock. Subsequently, through intersecting genes between key genes screened from DEGs for septic shock and those identified using WGCNA, we finally identified six new biomarkers of septic shock, namely CD177, CLEC5A, CYSTM1, MCEMP1, MMP8, and RGL4. The PPI networks have proven to be useful in the analysis of many kinds of disease. The STRING online database was used to construct PPI networks in which all protein-coding genes in a genome are grouped and organized. These six biomarkers were also verified by comprehensive bioinformatics analyses of gene expression profiles and a preliminary gene expression assay.
MMP8 is a Zn+2-reliant endopeptidase of the MMP family, which is mainly produced by neutrophilic cells as a proenzyme, and is stimulated by the ROS generated from the stimulated neutrophilic cells; hence, MMP-8 is vital for acute and persistent inflammatory activities (33, 34). MMP8 is related to the stimulation of proinflammatory cytokine TNF-α (35, 36), which eventually activates inflammatory activities throughout the body. MMP8 participates in the homoeostasis of the exocellular area, the expression of which is mainly realized by monocytes and macrophages. It also participates in the supportive activities of inborn immunity (37). The elevated MMP-8 activities facilitate the etiology of numerous illnesses, such as atherosis, lung fibrosis, and septic disease (38). Pedro Martínez-Paz et al. contrasted septic sufferers separated into septic shock and non-septic shock groups in postsurgical cases (39), then discovered that the septic shock cases registered greater sera MMPs expressing in contrast to the non-septic shock cases and the healthy donors. All discoveries reveal that MMPs can be underlying diagnosis biomarkers of septic shock.
CD177 serves as a vital neutrophilic cell gene encoding the glycoprotein of the membrane and the expression of such gene is increased during bacterial infections, burns, and pregnancy (40, 41). The identification of CD177 mRNA concentration has also become a helpful diagnosis method to differentiate these illnesses (42, 43), whereas its function in septic progression is still elusive. The CD177 gene might serve as an assumed marker or medicine target for septic shock.
Artificial intelligence systems have identified that the novel genetic marker CLEC5A helps to predict sepsis severity or mortality; CLEC5A had been identified previously and associated with sepsis mortality (44). Recent findings have suggested that CLEC5A (45) might be further implicated in NETosis. The polymorphic nucleus neutrophilic cells, facilitating inflammation representatively, also play roles in immunoregulation functions. A recent study found that CLEC5A, related to dengue, a virus-caused, life-threatening illness (46), is a vital element related to the regulation of the inborn immunoresponse against microbial infectious diseases in mice. This study showed that the expression of CLEC5A was increased in septic shock patients, and that the degree of increase was related to the degree of organic function disorder.
CYSTM1 is a comparatively elusive gene and the biological information assay displays an effect in resisting various matters involving DNA-injuring agents such as oxidizer, H2O2 mitomycin C, and the underlying membranous destabilization agent. The variation of the oxidation-reduction potential (ORP) of the membranous substances by CYSTM proteins may also impact the absorption of some substances and enable the quenching of underlying harmful free radicals (47). Thus, consistently across eukaryotes, CYSTM1 seems to play an effect in stress reaction or resistance, especially against harmful matters (48). The discoveries made in this study indicate that the CYSTM1 gene might serve as a novel marker for septic shock.
MCEMP1 is responsible for the encoding of a single-pass trans-membranous protein and participates in the modulation of MC differentiative activities or immunoresponses (49). MCs deteriorate septic disease via damaging the phagocyte activity of resident macrophages, hence enabling the proliferative activities of regional and systemic microbe agents (50). Jian-Xin Chen et al. verified that the miR-125-mediation MCEMP1 suppression could decrease the sera levels of TNF-α, IL-1β, and IL-6, and the programmed cell death facilitated the T leukomonocyte activity, hence attenuating the immune activity of sepsis mice (51). These discoveries reveal that MCEMP1 can be an underlying diagnosis marker for septic shock.
Yidan Sun et al. evidenced that the reduced expression of RGL4 was remarkably related to unfavorable prognostic results and immunocyte infiltrative activities in lung adenocarcinoma (LUAD) cases and highlighted the utilization of RGL4 as a new prediction marker for the prognostic results of LUAD (52). RGL4 might also be utilized in combination with the immuno-checkpoint method to reveal the advantages of immunity therapy.
In addition, these six potential biomarkers have considerable prospects in other diseases. Reports have shown that MMP8, which has a strong correlation with the development of skin cancers (53), particularly breast (54), melanoma (55), and tongue (56), could diminish or accelerate cancer progression via its breaking down of the ECM and cleavage of cell adhesion proteins (57). CD177 modulates the function and homeostasis of tumor-infiltrating regulatory T cells, one of the major immunosuppressive cell types in cancer (58). Tong et al. have reported that CLEC5A correlated with immunosuppression in glioma patients (59); meanwhile, CLEC5A was identified as an immune-related prognostic gene of ovarian cancer based on the immune microenvironment (39). In addition, bioinformatics analysis demonstrated that CYSTM1, a relatively unknown gene, plays a role in stress response (48). Gang Hu et al. were the first to report that the MCEMP1 gene had a significant association with the prognosis of gastric cancer (60). Moreover, the expression of RGL4 has been found to be correlated with a variety of tumor-infiltrating immune cells, such as neutrophils, CD8+ T cells, and memory B cells (52).
However, the pathways of CD177, CLEC5A, CYSTM1, MCEMP1, MMP8, and RGL4 in the development of septic shock remain unclear. Our study suggested that these core genes influenced the progression of septic shock by regulating these pathways, including the reactive oxygen species pathway, hypoxia, PI3K/AKT/mTOR signaling, NFκ-β/TNFα, and IL6/JAK/STAT3. Further relevant experimental assays should be carried out to verify the potential functions of critical genes and pathways in septic shock.
In this study, the CIBERSORT algorithm was used to quantify the abundance of each tumor microenvironment (TME) cell infiltration in the septic shock patient and healthy donors. In total, 22 subpopulations of immune cells, including B cells, plasma cells, T cells, NK cells, monocytes, macrophages, dendritic cells, mast cells, eosinophils, and neutrophils, were evaluated. A significant difference in immune cell infiltration was found between septic shock patients and control participants. Meanwhile, Spearman’s correlation analysis was conducted to evaluate the correlation between related DEGs and each TME infiltration cell type content.
The AUROCs of the six genes in the early diagnosis of septic shock were remarkable, indicating a better predictive efficiency than classical biomarkers such as CRP and PCT. Moreover, the intervention with the signal pathways that are related to these six hub genes may help us to achieve a better treatment response and prognosis for septic shock patients. Our study had inevitable limitations. First, to guarantee universalization and reduce selective bias to a minimum, more verification must be put in place, and larger numbers of septic shock patients and healthy donors should be involved. Second, in this study, our team merely highlighted markers that originated from white blood cells in circulating blood. Circulation markers related to cells from other organs with function disorders, such as tissue macrophages and blood vessel cells, may also participate in an intricate clinical process of septic disease, but these were not investigated in the present study. Last, as we obtained most of our data from specimens of septic shock who were minors and healthy donors minors through the biological information assay, the outcomes cannot be uncritically applied to adult septic cases. Further experimental analysis is still required to validate these findings. In addition, from the vast GEO database, not all septic shock samples could be included; thus, GSE33118, GSE26440, and GSE9692 were chosen in this study, owing to their relatively large sample sizes and representativeness. However, the sample sizes of the three datasets used in this study were small; thus, from a bioinformatics perspective, the results should be further verified, and an increased number of samples should be studied to increase the credibility of results. Our study has shown that in septic patients the mRNA levels of the CD177, CLEC5A, CYSTM1, MCEMP1, MMP8, and RGL4 genes were higher in septic patients than in control participants, suggesting the reliability of the preliminary clinical specimen. There is a lack of statistical difference between CLEC51 and CYSTM1 among the six genes tested in an mRNA expression analysis. In addition, only the protein levels of CD177 and MMP8 were verified owing to the limited experimental time. In a nutshell, a sufficient sample size for validating these hub genes both in terms of their RNA and protein levels is needed for further exploration.
5 Conclusion
In the present study, six hub genes with expression differences in septic shock were screened by broadly used biological information assays and WGCNA by integrating multiple datasets. The results revealed six helpful genes that can be used to explore the biomarkers or molecule mechanisms of sepsis shock.
Data availability statement
The original contributions presented in the study are included in the article/Supplementary material. Further inquiries can be directed to the corresponding author.
Ethics statement
The study (clinical trial registration number: ChiCTR2100053564) was approved by the Institutional Review Board of the First Affiliated Hospital of Wenzhou Medical University, Wenzhou, China, and has been registered at ClinicalTrials.gov. The patients/participants provided their written informed consent to participate in this study.
Author contributions
CK, YZ, JW, and MQ conceived and designed the experiments. CK, YZ, and MQ executed the experiments and analyzed the samples. CK, YZ, and MQ analyzed the data. CK wrote the first version of the manuscript. JW contributed to the revision of this article. XX contributed significantly to conducting the supplementary experiments required for the revisions. All authors interpreted the data and critically revised the manuscript. All authors contributed to the article and approved the submitted version.
Funding
We acknowledge the funding received from the Zhejiang Provincial Natural Science Foundation (LQ21H150007) and Wenzhou Municipal Science and Technology Bureau (Y20180122).
Acknowledgments
We are grateful for the experimental instruments provided by the Institute of Translational Medicine of the First Affiliated Hospital of Wenzhou Medical University, Wenzhou, China.
Conflict of interest
The authors declare that the research was conducted in the absence of any commercial or financial relationships that could be construed as a potential conflict of interest.
Publisher’s note
All claims expressed in this article are solely those of the authors and do not necessarily represent those of their affiliated organizations, or those of the publisher, the editors and the reviewers. Any product that may be evaluated in this article, or claim that may be made by its manufacturer, is not guaranteed or endorsed by the publisher.
Supplementary material
The Supplementary Material for this article can be found online at: https://www.frontiersin.org/articles/10.3389/fimmu.2023.1184700/full#supplementary-material
Supplementary Figure 1 | (A) Correlation analysis of immune activation genes and 6 hub genes. (B) Correlation analysis of immunosuppression and 6 hub genes. (C) Correlation analysis of chemokines and 6 hub genes. (D) Correlation analysis of chemokine receptors and 6 hub genes. Asterisk represent significant, red for positive correlation, blue for negative correlation.
Abbreviations
DEGs, differentially expressed genes; WGCNA, Weighted Gene Co-Expression Network Analysis; GSVA, gene set enrichment analysis; qPCR, quantitative Polymerase Chain Reaction; ROC, receiver operating characteristic; CRP, C-reactive protein; PCT, procalcitonin; GEO, Gene Expression Omnibus; ICU, intensive care unit; APACHE, Acute Physiology and Chronic Health Evaluation; SOFA, sequential organ failure assessment; PBMCs, peripheral blood mononuclear cells; PICU, pediatric intensive care unit; ACCP/SCCM, American College of Chest Physicians/Society of Critical Care Medicine; SVA, Surrogate Variable Analysis; PPI, protein protein interaction; STRING, Search Tool for the Retrieval of Interacting Genes; MCODE, Molecular Complex Detection; GLMNet, generalized linear model net; TOM, topological overlap matrix; SVM, support vector machine; GO, Gene Ontology; KEGG, Kyoto Encyclopedia of Genes and Genomes; PCA, Principal component analysis; CLEC5 A, C-type lectin domain containing 5 A; CYSTM1, cysteine rich transmembrane module containing 1; MCEMP1, mast cell expressed membrane protein 1; MMP8, matrix metallopeptidase 8; RGL4, ral guanine nucleotide dissociation stimulator like 4; ORP, oxidation-reduction potential; LUAD, Lung adenocarcinoma.
References
1. Faix JD. Biomarkers of sepsis. Crit Rev Clin Lab Sci (2013) 50:23–36. doi: 10.3109/10408363.2013.764490
2. Fernando SM, Rochwerg B, Seely AJE. Clinical implications of the third international consensus definitions for sepsis and septic shock (Sepsis-3). Cmaj (2018) 190:E1058–e1059. doi: 10.1503/cmaj.170149
3. Fleischmann-Struzek C, Mellhammar L, Rose N, Cassini A, Rudd KE, Schlattmann P, et al. Incidence and mortality of hospital- and ICU-treated sepsis: results from an updated and expanded systematic review and meta-analysis. Intensive Care Med (2020) 46:1552–62. doi: 10.1007/s00134-020-06151-x
4. Bosmann M, Ward PA. The inflammatory response in sepsis. Trends Immunol (2013) 34:129–36. doi: 10.1016/j.it.2012.09.004
5. Delano MJ, Ward PA. The immune system's role in sepsis progression, resolution, and long-term outcome. Immunol Rev (2016) 274:330–53. doi: 10.1111/imr.12499
6. Hotchkiss RS, Monneret G, Payen D. Sepsis-induced immunosuppression: from cellular dysfunctions to immunotherapy. Nat Rev Immunol (2013) 13:862–74. doi: 10.1038/nri3552
7. Joffre J, Hellman J. Oxidative stress and endothelial dysfunction in sepsis and acute inflammation. Antioxid Redox Signal (2021) 35:1291–307. doi: 10.1089/ars.2021.0027
8. Shang X, Lin K, Yu R, Zhu P, Zhang Y, Wang L, et al. Resveratrol protects the myocardium in sepsis by activating the phosphatidylinositol 3-kinases (PI3K)/AKT/Mammalian target of rapamycin (mTOR) pathway and inhibiting the nuclear factor-κB (NF-κB) signaling pathway. Med Sci Monit (2019) 25:9290–8. doi: 10.12659/MSM.918369
9. Pierrakos C, Velissaris D, Bisdorff M, Marshall JC, Vincent JL. Biomarkers of sepsis: time for a reappraisal. Crit Care (2020) 24:287. doi: 10.1186/s13054-020-02993-5
10. Evans L, Rhodes A, Alhazzani W, Antonelli M, Coopersmith CM, French C, et al. Surviving sepsis campaign: international guidelines for management of sepsis and septic shock 2021. Intensive Care Med (2021) 47:1181–247. doi: 10.1007/s00134-021-06506-y
11. Singer M, Deutschman CS, Seymour CW, Shankar-Hari M, Annane D, Bauer M, et al. The third international consensus definitions for sepsis and septic shock (Sepsis-3). Jama (2016) 315:801–10. doi: 10.1001/jama.2016.0287
12. Sinha M, Jupe J, Mack H, Coleman TP, Lawrence SM, Fraley SI. Emerging technologies for molecular diagnosis of sepsis. Clin Microbiol Rev (2018) 31:e00089-17. doi: 10.1128/CMR.00089-17
13. Cecconi M, Evans L, Levy M, Rhodes A. Sepsis and septic shock. Lancet (2018) 392:75–87. doi: 10.1016/S0140-6736(18)30696-2
14. Kumar S, Tripathy S, Jyoti A, Singh SG. Recent advances in biosensors for diagnosis and detection of sepsis: a comprehensive review. Biosens Bioelectron (2019) 124-125:205–15. doi: 10.1016/j.bios.2018.10.034
15. Zhang B, Horvath S. A general framework for weighted gene co-expression network analysis. Stat Appl Genet Mol Biol (2005) 4. doi: 10.2202/1544-6115.1128
16. Langfelder P, Horvath S. WGCNA: an r package for weighted correlation network analysis. BMC Bioinf (2008) 9:559. doi: 10.1186/1471-2105-9-559
17. Annane D, Sharshar T. Cognitive decline after sepsis. Lancet Respir Med (2015) 3:61–9. doi: 10.1016/S2213-2600(14)70246-2
18. Fang M, Zou T, Yang X, Zhang Z, Cao P, Han J, et al. Discovery of novel pterostilbene derivatives that might treat sepsis by attenuating oxidative stress and inflammation through modulation of MAPKs/NF-κB signaling pathways. Antioxidants (Basel) (2021) 10(9):1333. doi: 10.3390/antiox10091333
19. Venet F, Monneret G. Advances in the understanding and treatment of sepsis-induced immunosuppression. Nat Rev Nephrol (2018) 14:121–37. doi: 10.1038/nrneph.2017.165
20. Nedeva C, Menassa J, Duan M, Liu C, Doerflinger M, Kueh AJ, et al. TREML4 receptor regulates inflammation and innate immune cell death during polymicrobial sepsis. Nat Immunol (2020) 21:1585–96. doi: 10.1038/s41590-020-0789-z
21. Riedhammer C, Halbritter D, Weissert R. Peripheral blood mononuclear cells: isolation, freezing, thawing, and culture. Methods Mol Biol (2016) 1304:53–61. doi: 10.1007/7651_2014_99
22. Wynn JL, Cvijanovich NZ, Allen GL, Thomas NJ, Freishtat RJ, Anas N, et al. The influence of developmental age on the early transcriptomic response of children with septic shock. Mol Med (2011) 17:1146–56. doi: 10.2119/molmed.2011.00169
23. Cvijanovich N, Shanley TP, Lin R, Allen GL, Thomas NJ, Checchia P, et al. Validating the genomic signature of pediatric septic shock. Physiol Genomics (2008) 34:127–34. doi: 10.1152/physiolgenomics.00025.2008
24. Barrett T, Wilhite SE, Ledoux P, Evangelista C, Kim IF, Tomashevsky M, et al. NCBI GEO: archive for functional genomics data sets–update. Nucleic Acids Res (2013) 41:D991–5. doi: 10.1093/nar/gks1193
25. Goldstein B, Giroir B, Randolph A. International pediatric sepsis consensus conference: definitions for sepsis and organ dysfunction in pediatrics. Pediatr Crit Care Med (2005) 6:2–8. doi: 10.1097/01.PCC.0000149131.72248.E6
26. Leek JT, Johnson WE, Parker HS, Jaffe AE, Storey JD. The sva package for removing batch effects and other unwanted variation in high-throughput experiments. Bioinformatics (2012) 28:882–3. doi: 10.1093/bioinformatics/bts034
27. Hild M, Beckmann B, Haas SA, Koch B, Solovyev V, Busold C, et al. An integrated gene annotation and transcriptional profiling approach towards the full gene content of the drosophila genome. Genome Biol (2003) 5:R3. doi: 10.1186/gb-2003-5-1-r3
28. Zhao W, Wang D, Zhao J, Zhao W. Bioinformatic analysis of retinal gene function and expression in diabetic rats. Exp Ther Med (2017) 14:2485–92. doi: 10.3892/etm.2017.4805
29. Ritchie ME, Phipson B, Wu D, Hu Y, Law CW, Shi W, et al. Limma powers differential expression analyses for RNA-sequencing and microarray studies. Nucleic Acids Res (2015) 43:e47. doi: 10.1093/nar/gkv007
30. Shannon P, Markiel A, Ozier O, Baliga NS, Wang JT, Ramage D, et al. Cytoscape: a software environment for integrated models of biomolecular interaction networks. Genome Res (2003) 13:2498–504. doi: 10.1101/gr.1239303
31. Gao CH, Yu G, Cai P. ggVennDiagram: an intuitive, easy-to-Use, and highly customizable r package to generate Venn diagram. Front Genet (2021) 12:706907. doi: 10.3389/fgene.2021.706907
32. Gentles AJ, Newman AM, Liu CL, Bratman SV, Feng W, Kim D, et al. The prognostic landscape of genes and infiltrating immune cells across human cancers. Nat Med (2015) 21:938–45. doi: 10.1038/nm.3909
33. Van Lint P, Libert C. Matrix metalloproteinase-8: cleavage can be decisive. Cytokine Growth Factor Rev (2006) 17:217–23. doi: 10.1016/j.cytogfr.2006.04.001
34. Uhel F, Azzaoui I, Grégoire M, Pangault C, Dulong J, Tadié JM, et al. Early expansion of circulating granulocytic myeloid-derived suppressor cells predicts development of nosocomial infections in patients with sepsis. Am J Respir Crit Care Med (2017) 196:315–27. doi: 10.1164/rccm.201606-1143OC
35. Silswal N, Singh AK, Aruna B, Mukhopadhyay S, Ghosh S, Ehtesham NZ. Human resistin stimulates the pro-inflammatory cytokines TNF-alpha and IL-12 in macrophages by NF-kappaB-dependent pathway. Biochem Biophys Res Commun (2005) 334:1092–101. doi: 10.1016/j.bbrc.2005.06.202
36. Lee EJ, Han JE, Woo MS, Shin JA, Park EM, Kang JL, et al. Matrix metalloproteinase-8 plays a pivotal role in neuroinflammation by modulating TNF-α activation. J Immunol (2014) 193:2384–93. doi: 10.4049/jimmunol.1303240
37. Quintero PA, Knolle MD, Cala LF, Zhuang Y, Owen CA. Matrix metalloproteinase-8 inactivates macrophage inflammatory protein-1 alpha to reduce acute lung inflammation and injury in mice. J Immunol (2010) 184:1575–88. doi: 10.4049/jimmunol.0900290
38. Morla S, Desai UR. Discovery of sulfated small molecule inhibitors of matrix metalloproteinase-8. Biomolecules (2020) 10(8):1166. doi: 10.3390/biom10081166
39. Martínez-Paz P, Aragón-Camino M, Gómez-Sánchez E, Lorenzo-López M, Gómez-Pesquera E, Fadrique-Fuentes A, et al. Distinguishing septic shock from non-septic shock in postsurgical patients using gene expression. J Infect (2021) 83:147–55. doi: 10.1016/j.jinf.2021.05.039
40. Stroncek DF, Caruccio L, Bettinotti M. CD177: a member of the ly-6 gene superfamily involved with neutrophil proliferation and polycythemia vera. J Transl Med (2004) 2:8. doi: 10.1186/1479-5876-2-8
41. Göhring K, Wolff J, Doppl W, Schmidt KL, Fenchel K, Pralle H, et al. Neutrophil CD177 (NB1 gp, HNA-2a) expression is increased in severe bacterial infections and polycythaemia vera. Br J Haematol (2004) 126:252–4. doi: 10.1111/j.1365-2141.2004.05027.x
42. Demaret J, Venet F, Plassais J, Cazalis MA, Vallin H, Friggeri A, et al. Identification of CD177 as the most dysregulated parameter in a microarray study of purified neutrophils from septic shock patients. Immunol Lett (2016) 178:122–30. doi: 10.1016/j.imlet.2016.08.011
43. Rasooli A, Ghafari E, Saedi H, Miri S. Expression changes of CD177 and MPO as novel biomarkers in lung tissue of CLP model rats. Turk J Med Sci (2018) 48:1321. doi: 10.3906/sag-1806-223
44. Banerjee S, Mohammed A, Wong HR, Palaniyar N, Kamaleswaran R. Machine learning identifies complicated sepsis course and subsequent mortality based on 20 genes in peripheral blood immune cells at 24 h post-ICU admission. Front Immunol (2021) 12:592303. doi: 10.3389/fimmu.2021.592303
45. Chen ST, Li FJ, Hsu TY, Liang SM, Yeh YC, Liao WY, et al. CLEC5A is a critical receptor in innate immunity against listeria infection. Nat Commun (2017) 8:299. doi: 10.1038/s41467-017-00356-3
46. Chen ST, Lin YL, Huang MT, Wu MF, Cheng SC, Lei HY, et al. CLEC5A is critical for dengue-virus-induced lethal disease. Nature (2008) 453:672–6. doi: 10.1038/nature07013
47. Venancio TM, Balaji S, Aravind L. High-confidence mapping of chemical compounds and protein complexes reveals novel aspects of chemical stress response in yeast. Mol Biosyst (2010) 6:175–81. doi: 10.1039/B911821G
48. Venancio TM, Aravind L. CYSTM, a novel cysteine-rich transmembrane module with a role in stress tolerance across eukaryotes. Bioinformatics (2010) 26:149–52. doi: 10.1093/bioinformatics/btp647
49. Li K, Wang SW, Li Y, Martin RE, Li L, Lu M, et al. Identification and expression of a new type II transmembrane protein in human mast cells. Genomics (2005) 86:68–75. doi: 10.1016/j.ygeno.2005.03.006
50. Dahdah A, Gautier G, Attout T, Fiore F, Lebourdais E, Msallam R, et al. Mast cells aggravate sepsis by inhibiting peritoneal macrophage phagocytosis. J Clin Invest (2014) 124:4577–89. doi: 10.1172/JCI75212
51. Chen JX, Xu X, Zhang S. Silence of long noncoding RNA NEAT1 exerts suppressive effects on immunity during sepsis by promoting microRNA-125-dependent MCEMP1 downregulation. IUBMB Life (2019) 71:956–68. doi: 10.1002/iub.2033
52. Sun Y, Zhang Y, Ren S, Li X, Yang P, Zhu J, et al. Low expression of RGL4 is associated with a poor prognosis and immune infiltration in lung adenocarcinoma patients. Int Immunopharmacol (2020) 83:106454. doi: 10.1016/j.intimp.2020.106454
53. Balbín M, Fueyo A, Tester AM, Pendás AM, Pitiot AS, Astudillo A, et al. Loss of collagenase-2 confers increased skin tumor susceptibility to male mice. Nat Genet (2003) 35:252–7. doi: 10.1038/ng1249
54. Decock J, Hendrickx W, Thirkettle S, Gutiérrez-Fernández A, Robinson SD, Edwards DR. Pleiotropic functions of the tumor- and metastasis-suppressing matrix metalloproteinase-8 in mammary cancer in MMTV-PyMT transgenic mice. Breast Cancer Res (2015) 17:38. doi: 10.1186/s13058-015-0545-8
55. Gutiérrez-Fernández A, Fueyo A, Folgueras AR, Garabaya C, Pennington CJ, Pilgrim S, et al. Matrix metalloproteinase-8 functions as a metastasis suppressor through modulation of tumor cell adhesion and invasion. Cancer Res (2008) 68:2755–63. doi: 10.1158/0008-5472.CAN-07-5154
56. Korpi JT, Kervinen V, Mäklin H, Väänänen A, Lahtinen M, Läärä E, et al. Collagenase-2 (matrix metalloproteinase-8) plays a protective role in tongue cancer. Br J Cancer (2008) 98:766–75. doi: 10.1038/sj.bjc.6604239
57. Dufour A, Overall CM. Missing the target: matrix metalloproteinase antitargets in inflammation and cancer. Trends Pharmacol Sci (2013) 34:233–42. doi: 10.1016/j.tips.2013.02.004
58. Kim MC, Borcherding N, Ahmed KK, Voigt AP, Vishwakarma A, Kolb R, et al. CD177 modulates the function and homeostasis of tumor-infiltrating regulatory T cells. Nat Commun (2021) 12:5764. doi: 10.1038/s41467-021-26091-4
59. Tong L, Li J, Choi J, Pant A, Xia Y, Jackson C, et al. CLEC5A expressed on myeloid cells as a M2 biomarker relates to immunosuppression and decreased survival in patients with glioma. Cancer Gene Ther (2020) 27:669–79. doi: 10.1038/s41417-019-0140-8
Keywords: septic shock, WGCNA, biomarker, bioinformatic analysis, prognosis
Citation: Kong C, Zhu Y, Xie X, Wu J and Qian M (2023) Six potential biomarkers in septic shock: a deep bioinformatics and prospective observational study. Front. Immunol. 14:1184700. doi: 10.3389/fimmu.2023.1184700
Received: 12 March 2023; Accepted: 15 May 2023;
Published: 08 June 2023.
Edited by:
Takashi Karako, National Center For Global Health and Medicine, JapanReviewed by:
Di Xie, Shanghai Jiao Tong University, ChinaXin Ouyang, Guangdong Provincial People’s Hospital, China
Gustavo Sganzerla, Dalhousie University, Canada
Copyright © 2023 Kong, Zhu, Xie, Wu and Qian. This is an open-access article distributed under the terms of the Creative Commons Attribution License (CC BY). The use, distribution or reproduction in other forums is permitted, provided the original author(s) and the copyright owner(s) are credited and that the original publication in this journal is cited, in accordance with accepted academic practice. No use, distribution or reproduction is permitted which does not comply with these terms.
*Correspondence: Meizi Qian, cWlhbm1laXppQHd6aG9zcGl0YWwuY24=
†These authors have contributed equally to this work and share first authorship