- 1Division of Critical Care Medicine, Department of Pediatrics, Penn State University College of Medicine, Hershey, PA, United States
- 2Department of Molecular and Precision Medicine, Penn State University College of Medicine, Hershey, PA, United States
- 3Critical Illness and Sepsis Research Center, Penn State College of Medicine, Hershey, PA, United States
- 4Division of Pediatric Hematology/Oncology, Department of Pediatrics, Penn State University College of Medicine, Hershey, PA, United States
- 5Division of Critical Care Medicine, Department of Anesthesiology and Perioperative Medicine, Penn State University College of Medicine, Hershey, PA, United States
- 6Division of Biostatistics and Bioinformatics, Department of Public Health Sciences, Penn State University College of Medicine, Hershey, PA, United States
Introduction: Recent studies of adult sepsis patients demonstrate the existence of two subphenotypes that differ in risk of mortality: a hyper-inflammatory subphenotype with a high risk of mortality, and a hypo-inflammatory or “not hyper-inflamed” subphenotype with a relatively lower risk of mortality. We recently investigated the association of organ dysfunction with ex vivo immune profiling in sixty (60) critically ill adult patients with sepsis. In this secondary analysis we measured cytokine biomarkers with an automated, microfluidic immunoassay device (Ella™) and sought to investigate the functional immune profiles of patients in the hyper/hypo-inflammatory subphenotype groups.
Methods: Subjects were consecutively identified adults, older than 18 years, and enrolled within 48 hours of sepsis onset. Whole blood cytokine analysis was performed in all patients. Additionally, ex vivo cytokine production was measured following 4h of stimulation. Cytokine concentrations were measured using the Ella™ automated immunoassay system.
Results: Subjects were divided into hypo-inflammatory (42 patients) and hyper-inflammatory (18 patients) subtypes using a previously validated parsimonious model based on concentrations of IL-6, TNFR1 and bicarbonate. The hyper- and hypo-inflammatory clusters demonstrated a near four-fold difference in 30-day mortality (44.4% vs 11.9%, p=0.0046). Following 4h of ex vivo stimulation with LPS, TNF production was lower in the hyper-inflammatory group as compared with the hypo-inflammatory group (p=0.0159). Ex vivo phorbol 12-myristate 13-acetate (PMA)-stimulated IFN-γ production (4h) by whole blood did not differ between groups.
Conclusions: These data further validate the use of IL-6, TNFR1 and bicarbonate to discern inflammatory sub-groups of patients with critical illness. They also confirm the observation that the presence of the hyper-inflammatory subphenotype is often accompanied by a compensatory anti-inflammatory response syndrome. Future investigations should focus on prospective validation of this panel for prognostic enrichment of clinical research studies.
Introduction
Sepsis is characterized by dynamic disruptions in the immune system, often leading to an increased susceptibility to secondary infections, long-term health complications, and death (1–5). Biomarkers have shown significant promise in prognostication following acute respiratory distress syndrome (ARDS) (6–10) and sepsis (11, 12): interleukin (IL)-6, IL-8 (otherwise known as C-X-C motif ligand-8 or CXCL8), and tumor necrosis factor receptor 1 (TNFR1). Furthermore, these biomarkers have helped to identify a hyper-inflammatory subphenotype of ARDS and sepsis, characterized by increased mortality as compared with its hypo-inflamed counterpart (10, 13).
Our prior investigation has synthesized existing evidence regarding the prognostic value of whole blood responses to ex vivo lipopolysaccharide (LPS) stimulation of blood in sepsis (14). Reduced TNF production following LPS stimulation remains a hallmark of “immunoparalysis,” a state of immune dysfunction characterized by diminished immune capacity to respond effectively to infections (1, 15, 16). Patients in this state are at a substantially higher risk for developing secondary infections and death (17). However, an exclusive focus on LPS responses may overlook other vital components of immune function, such as lymphocyte count and activity (14, 18).
Both immunoparalysis, indicated by reduced ex vivo cytokine production, and hyper-inflammation, characterized by elevated levels of IL-6, IL-8 and TNFR1, are associated with worse outcomes in sepsis. To investigate the apparent paradox between these two states, we examined the relationship between inflammatory subphenotypes as determined by plasma cytokine and bicarbonate concentrations during critical illness, and ex vivo cytokine production.
Materials and methods
Methodological approach and participant selection
This is a secondary analysis of a recently published study (19). Ethical approval was obtained from the Human Studies Protection Office of the Penn State College of Medicine (#15328, 7/30/2020). Procedures were followed in accordance with ethical standards established by the Human Subjects Protection Office and with the Helsinki Declaration of 1975. The Modified Early Warning Scoring (MEWS) scoring-based algorithm was used to identify critically ill patients with possible sepsis (20, 21) from November 2021 to August 2023. Dual, independent investigator reviews ensured unbiased selection of patients meeting inclusion criteria from electronically flagged patient records. Informed consent was obtained from patients having decision-making capacity, or from the legally authorized healthcare representatives of patients lacking decision-making capacity.
Inclusion and exclusion parameters
Eligible septic candidates were consecutively identified adults, >18 years, within 48 hours of critical illness onset. Sepsis was defined by Sepsis-3 criteria, namely a change in SOFA score of two or more in the setting of clinically suspected or microbiologically proven infection (22). Non-survivors were defined as those deceased within 30 days post-enrollment. Patients exhibiting active hematologic malignancies and those receiving immune-altering therapies were excluded.
Clinical variables and data retrieval
Clinical data, including whole blood counts and cell differentials, were retrieved from hospital records and post-discharge interviews. Illness severity was measured using Charlson Comorbidity Index (23) and APACHE II score (24–26). Outcomes included organ dysfunction via SOFA score; 30-day mortality, readmission, and infection rates. Secondary infection was defined using the CDC/NHSN definitions (27). Blood samples were collected early, within 48h of ICU admission, for leukocyte analysis, cytokine analysis, and cytokine quantification after ex vivo stimulation tests, with supernatants frozen for later analysis.
Cytokine analysis
Whole blood was collected in sodium heparin tubes and ex vivo cytokine production was performed on the day of collection. Plasma was separated by centrifugation (400 g x 5 min). Plasma was aliquoted and stored at -80°C until subsequent batch analysis. Cytokines were measured using the Ella™ microfluidic automated immunoassay system (Bio-Techne, MN). Biomarkers were measured by batch analysis by Ella™ using custom manufactured 32 sample x 4 analyte cartridges (28–45) (Supplementary Table 1). Plasma samples were thawed and diluted 1:10 with sample diluent 13, and 50 µL of diluted sample were loaded into each sample inlet, and data was acquired utilizing the Simple Plex Runner software v.3.7.2.0 (ProteinSimple).
Ex vivo stimulation and cytokine quantification
Whole blood was collected in sodium heparin tubes. As previously described (19) 50 μL whole blood was diluted ten-fold in HEPES-buffered RPMI media, then exposed to designated stimulants (46, 47). Whole blood samples from each study participant were exposed to separate conditions: (1) 500 pg/mL lipopolysaccharide (LPS) from Salmonella enterica strain abortus equi, or (2) 10 ng/mL phorbol 12-myristate 13-acetate (PMA) with 1 μg/mL ionomycin. Following incubation for 4 hours at 5% carbon dioxide and 37 °C, and subsequent centrifugation, supernatants were frozen at -80 °C until the time of analysis. The Ella™ automated immunoassay system (Bio-Techne, Minneapolis, MN) was used for triplicate measurement of interferon-gamma (IFN-γ), tumor necrosis factor (TNF), and interleukin (IL)-6. Data was acquired utilizing the Simple Plex Runner software v.3.7.2.0 (ProteinSimple).
Intracellular cytokine staining
Multicolor flow cytometry was performed on a 17-color Becton Dickinson (BD) FACSSymphony. Blood was obtained from three separate healthy adult controls, ages 40-50, on the morning of stimulation. After 3h of ex vivo stimulation, brefeldin A was added to cause cytokine accumulation in cells. After 4h whole blood cells were surface stained with fluorescent-labeled antibodies (BD or BioLegend) and intracellular staining for TNF and IFN-γ was performed using Cytofix/Cytoperm (BD) kit.
Statistics
Using Prism (v9.5) and JMP® Pro (v18.0.2), with a significance threshold of 0.05, we summarized variables descriptively. Continuous variables’ distributions were evaluated via histograms, probability plots, and normality tests. Demographic comparisons between groups utilized Chi-square and two-sample t-tests. Due to the non-normal distribution of the cytokine data, log10 transformation was applied before modeling. Designation of the inflammatory group (hypo-inflammatory vs. hyper-inflammatory) was determined using a parsimonious mathematical model with IL-6 (pg/mL), TNFR1 (pg/mL) and bicarbonate (mEq/L) values as variables that was previously validated for patients with ARDS (7).
Results
Study population and inflammatory clusters
In the primary study, we enrolled a total of 60 individuals with sepsis. Detailed demographic profiles and clinical outcomes of these patients have been previously described (19). Multiple cytokines were measured in sepsis patients using custom Ella™ cartridges. Using a previously validated parsimonious classifier model (7) based on IL-6, TNFR1 and bicarbonate levels, subjects were divided into two clusters: hyper-inflammatory (defined by elevated IL-6, TNFR1 and low bicarbonate levels, n=18, red), and hypo-inflammatory (n=42, blue) groups (Figure 1). The classifier model is a logistic regression-based model comprised of IL-6, sodium bicarbonate and TNFR1. For the model, IL-6 and TNFR1 were log-transformed after the addition of one (+1). The coefficients used for the model were detailed in previous manuscripts (7, 48). We used the validated cutoff of 0.5 for this analysis.
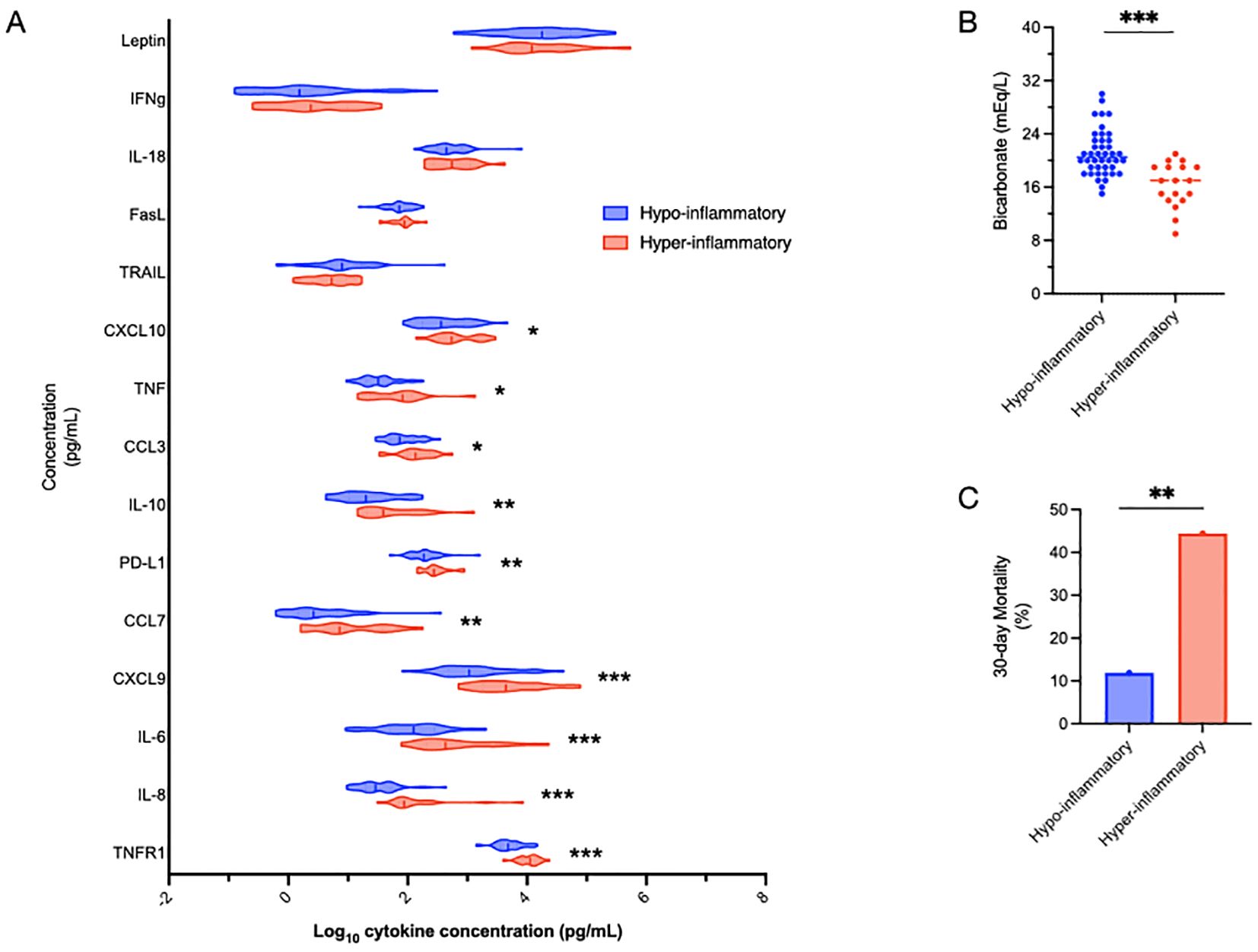
Figure 1. Defining inflammatory subphenotypes by IL-6, TNFR1 and bicarbonate. Sepsis patients were divided into hyper-inflammatory (red) and hypo-inflammatory (blue) groups using IL-6, TNFR1 and bicarbonate levels based on a previously validated model (48). The association of other cytokines with the inflammatory groups is shown, with cytokines listed in order of increasing significant differences between the groups (A). Bicarbonate, the non-cytokine variable in the model, by definition, was significantly different (***p<0.001) between the groups (B). Mortality in the hyper-inflammatory group was significantly higher than the hypo-inflammatory group (44.4% vs 11.9%, **p<0.01) (C). Comparisons between the groups were performed using t-tests (A, B) and chi-squared tests (C). *p<0.05.
Not surprisingly, TNFR1, IL-8 and IL-6 levels were significantly (***p<0.001) different between the inflammatory subphenotypes (Figure 1A). Also not surprisingly, given that bicarbonate is part of the validated model, bicarbonate levels were significantly lower in the hyper-inflammatory cluster (Figure 1B). 30-day mortality was almost four-fold higher (p=0.0046) in the hyper-inflammatory (44.4%) group than the hypo-inflammatory (11.9%) cluster (Figure 1B).
Functional immune responses
We investigated the “functional immune response” by quantifying the amount of cytokines produced by whole blood in response to precise amounts of immune stimulation. We quantified total TNF production after 4h of ex vivo stimulation of fresh whole blood with LPS. Interestingly, patients from the hyper-inflammatory cluster demonstrated lower ex vivo TNF production as compared to the hypo-inflammatory group (407±442 vs 729±637 pg/mL, p = 0.0159 (Figure 2A). As a side experiment, we investigated what cell types were producing TNF in response to LPS stimulation using intracellular cytokine staining (ICS)(Supplementary Figure 1). Patients with sepsis had reduced TNF production as compared to healthy controls (Supplementary Figures 2, 4). While CD15+ neutrophils produced some TNF, by far the largest producers of TNF in the ex vivo assay are CD14+ monocytes (Supplementary Figures 2–4).
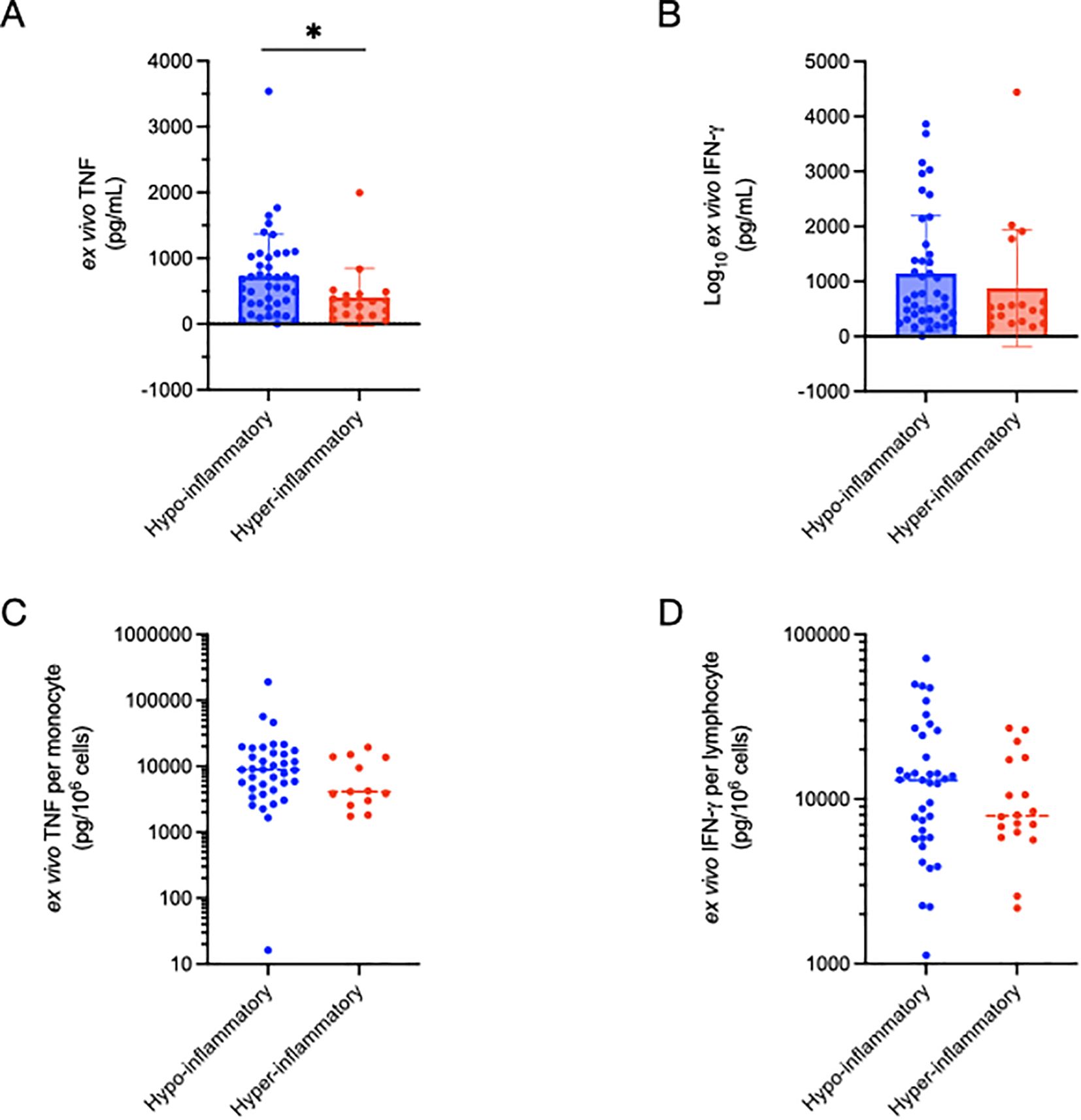
Figure 2. Comparison of functional immune profiling as determined by ex vivo cytokine production between inflammatory subphenotypes. Total TNF production (4h) was significantly (*p=0.016) lower in the hyper-inflammatory cluster (A), but not when corrected for absolute monocyte count (C). Ex vivo IFN-γ production did not differ between the inflammatory clusters in total (B), or after correction for absolute lymphocyte count (D). Significance was determined using non-parametric (Wilcoxon signed-rank) testing.
To control for the potential impact of leukopenia on TNF production, we normalized TNF levels to monocyte counts calculated using daily complete blood count with automated differential cell count analysis. TNF production per monocyte (pg TNF/106 cells) did not differ between the inflammatory clusters. In contrast to TNF production in response to LPS stimulation, the total amount of IFN-γ production (pg/mL) following PMA/ionomycin stimulation did not differ between the hyper- and hypo-inflammatory groups (Figure 2C), nor was there a difference after normalizing to lymphocyte count (pg IFN-γ/106 cells) (Figure 2D).
Discussion
We have recently described the prognostic value of rapid, functional immune profiling to predict organ dysfunction in critically ill patients (19). This secondary analysis extends our prior investigation by examining the relationship between previously-described, hyper- and hypo-inflammatory subphenotypes of sepsis (11, 12) and ex vivo TNF production in our cohort (6–10, 12). By combining measurements of IL-6 and TNFR1 levels in whole blood, combined with bicarbonate levels, with ex vivo production of cytokines in response to stimulation of whole blood, we observed that hyper-inflamed patients produce less TNF following ex vivo LPS stimulation, as compared with hypo-inflammatory sepsis patients. Ex vivo TNF production by whole blood has typically been ascribed to monocytes (49). We were able to confirm this using intracellular cytokine staining (Supplementary Figures 1–4). The white blood cell and absolute monocyte counts were not different between the inflammatory subphenotypes. Therefore, we expected to see less TNF production per monocyte in the hyper-inflammatory sub-group, but this result was not significant (p=0.138, Wilcoxon two-sample test). This is likely due to our sample size being underpowered to detect the difference. Furthermore, the AMC was based on automated differential counts from the clinical laboratory which can be fairly inaccurate (50).
These results support the existing paradigm of a concurrent systemic inflammatory (SIRS) and compensatory anti-inflammatory (CARS) response in acute sepsis (15, 51). In our cohort, being in the hyper-inflammatory subgroup increased the 30-day risk of mortality nearly 4-fold. Further research needs to be performed to develop strategies rapidly identify this group, and to provide anti-inflammatory interventions. The ongoing NICHD supported PRECISE trial (52) is examining the role of IL-1 blockade using anakinra to decrease organ dysfunction. It is also plausible that targeted anti-inflammatory agents may restore ex vivo TNF production.
Our work adds to a growing body of literature that demonstrates the value of IL-6, IL-8 and TNFR1 concentrations for prognostic enrichment of clinical cohorts. IL-6 and IL-8 are very tightly correlated: R2 0.95 in our cohort of sepsis patients. While IL-6 is described as one of the big three pyrogens (IL-1, IL-6, TNF), IL-1 and TNF seem to be the main drivers of inflammation, while IL-6 serves as a quality biomarker (53). IL-8, more correctly named CXCL8 (54), is a chemokine secreted by toll-like receptor-expressing cells. It binds to CXCR1 with a higher affinity as compared with CXCR2, and it functions as neutrophil chemoattractant (55). TNFR1 is one of two TNF receptors that are cleaved from cell surfaces following TNF signaling. TNFR1 is ubiquitously expressed, whereas the expression of TNFR2 is restricted to immune cells (56, 57). The use of TNFR1 as a prognostic biomarker of mortality in critical illness is well established. Elevated TNFR1 levels likely reflect both prior and ongoing TNF signaling following a pro-inflammatory insult (58).
The role of the ex vivo TNF production assay for clinical use is unclear (14). In the present study ex vivo TNF did not predict 30-day mortality. The assay is likely a better predictor of risk of secondary infection, but this study was underpowered to detect any association. While the ex vivo TNF production assay is relatively easy to perform, it is time intensive and requires a 4h incubation step. Also, comparisons of the assay results across laboratories is not standardized.
The methods for conducting ex vivo LPS-stimulated TNF production assays vary across laboratories and investigators (14). Our laboratory utilizes the same LPS source (Salmonella enterica) and dose (500 pg/mL) as Dr. Hall from Nationwide Children’s Hospital (15, 16). As a result, our TNF measurements may differ from those obtained by other sepsis research laboratories. The same limitation is also true of the PMA/ionomycin-induced IFN-γ assay.
The chief limitation of the present study is the relatively small sample size, which likely limited our ability to detect some significant differences between the two subphenotypes.
Strengths of our study include the use of a rapid, established and semi-automated immunoassay system for cytokine measurement directly from plasma, and of supernatants for the ex vivo stimulation assays. Because of the Ella™ system’s attributes, which include high sensitivity, a large dynamic measurement range, rapid analysis, and high reproducibility (19, 46, 47), we envision the proliferation of its use as a clinical instrument for on-site cytokine measurements in the near future. Future studies should focus on the rapid differentiation of the two subphenotypes with targeted interventions.
Data availability statement
The raw data supporting the conclusions of this article will be made available by the authors, without undue reservation.
Ethics statement
The studies involving humans were approved by Penn State University Institutional Review Board. The studies were conducted in accordance with the local legislation and institutional requirements. The participants provided their written informed consent to participate in this study.
Author contributions
EH: Conceptualization, Formal Analysis, Writing – original draft, Writing – review & editing. DM: Funding acquisition, Writing – review & editing. AS: Data curation, Investigation, Writing – review & editing. SZ: Writing – review & editing, Data curation, Formal Analysis. AB: Conceptualization, Funding acquisition, Supervision, Writing – review & editing.
Funding
The author(s) declare that financial support was received for the research and/or publication of this article. This study was supported by National Institute of General Medical Sciences, grant #K08GM138825 (ASB) and #R35 R35GM150695 (ASB).
Conflict of interest
EH is a consultant for Swedish Orphan Biovitrum SOBI pharmaceuticals.
The remaining authors declare that the research was conducted in the absence of any commercial or financial relationships that could be construed as a potential conflict of interest.
Generative AI statement
The author(s) declare that no Generative AI was used in the creation of this manuscript.
Publisher’s note
All claims expressed in this article are solely those of the authors and do not necessarily represent those of their affiliated organizations, or those of the publisher, the editors and the reviewers. Any product that may be evaluated in this article, or claim that may be made by its manufacturer, is not guaranteed or endorsed by the publisher.
Supplementary material
The Supplementary Material for this article can be found online at: https://www.frontiersin.org/articles/10.3389/fimmu.2025.1520848/full#supplementary-material
Supplementary Table 1 | Biomarker selection.
Abbreviations
CINS, critically ill and non-septic; CXCL8, C-X-C motif chemokine ligand 8 (AKA, IL-8); IFNγ, interferon-gamma; IL-8, interleukin-8 (AKA, CXCL8); LPS, lipopolysaccharide, or endotoxinNa; PMA, phorbol 12-myristate 13-acetate; TNF, tumor necrosis factor; TNFR1, tumor necrosis factor receptor 1.
References
1. Frazier WJ, Hall MW. Immunoparalysis and adverse outcomes from critical illness. Pediatr Clin North Am. (2008) 55:647–68, xi. doi: 10.1016/j.pcl.2008.02.009
2. Zhao GJ, Li D, Zhao Q, Song JX, Chen XR, Hong GL, et al. Incidence, risk factors and impact on outcomes of secondary infection in patients with septic shock: an 8-year retrospective study. Sci Rep. (2016) 6:38361. doi: 10.1038/srep38361
3. Hotchkiss RS, Monneret G, Payen D. Sepsis-induced immunosuppression: from cellular dysfunctions to immunotherapy. Nat Rev Immunol. (2013) 13:862–74. doi: 10.1038/nri3552
4. Venet F, Monneret G. Advances in the understanding and treatment of sepsis-induced immunosuppression. Nat Rev Nephrol. (2018) 14:121–37. doi: 10.1038/nrneph.2017.165
5. Davenport EE, Burnham KL, Radhakrishnan J, Humburg P, Hutton P, Mills TC, et al. Genomic landscape of the individual host response and outcomes in sepsis: a prospective cohort study. Lancet Respir Med. (2016) 4:259–71. doi: 10.1016/S2213-2600(16)00046-1
6. Sinha P, Delucchi KL, Chen Y, Zhuo H, Abbott J, Wang C, et al. Latent class analysis-derived subphenotypes are generalisable to observational cohorts of acute respiratory distress syndrome: a prospective study. Thorax. (2022) 77:13–21. doi: 10.1136/thoraxjnl-2021-217158
7. Sinha P, Delucchi KL, McAuley DF, O’Kane CM, Matthay MA, Calfee CS. Development and validation of parsimonious algorithms to classify acute respiratory distress syndrome phenotypes: a secondary analysis of randomised controlled trials. Lancet Respir Med. (2020) 8:247–57. doi: 10.1016/S2213-2600(19)30369-8
8. Sinha P, Churpek MM, Calfee CS. Machine learning classifier models can identify acute respiratory distress syndrome phenotypes using readily available clinical data. Am J Respir Crit Care Med. (2020) 202:996–1004. doi: 10.1164/rccm.202002-0347OC
9. Delucchi K, Famous KR, Ware LB, Parsons PE, Thompson BT, Calfee CS, et al. Stability of ARDS subphenotypes over time in two randomised controlled trials. Thorax. (2018) 73:439–45. doi: 10.1136/thoraxjnl-2017-211090
10. Calfee CS, Delucchi K, Parsons PE, Thompson BT, Ware LB, Matthay MA, et al. Subphenotypes in acute respiratory distress syndrome: latent class analysis of data from two randomised controlled trials. Lancet Respir Med. (2014) 2:611–20. doi: 10.1016/S2213-2600(14)70097-9
11. Mikacenic C, Price BL, Harju-Baker S, O’Mahony DS, Robinson-Cohen C, Radella F, et al. A two-biomarker model predicts mortality in the critically ill with sepsis. Am J Respir Crit Care Med. (2017) 196:1004–11. doi: 10.1164/rccm.201611-2307OC
12. Sinha P, Kerchberger VE, Willmore A, Chambers J, Zhuo H, Abbott J, et al. Identifying molecular phenotypes in sepsis: an analysis of two prospective observational cohorts and secondary analysis of two randomised controlled trials. Lancet Respir Med. (2023) 11(11):965–74. doi: 10.1016/S2213-2600(23)00237-0
13. Sinha P, Delucchi KL, Thompson BT, McAuley DF, Matthay MA, Calfee CS, et al. Latent class analysis of ARDS subphenotypes: a secondary analysis of the statins for acutely injured lungs from sepsis (SAILS) study. Intensive Care Med. (2018) 44:1859–69. doi: 10.1007/s00134-018-5378-3
14. Wheelwright J, Halstead ES, Knehans A, Bonavia AS. Ex vivo endotoxin stimulation of blood for predicting survival in patients with sepsis: A systematic review. CHEST Crit Care. (2023) 1(3):100029. doi: 10.1016/j.chstcc.2023.100029
15. Hall MW, Greathouse KC, Thakkar RK, Sribnick EA, Muszynski JA. Immunoparalysis in pediatric critical care. Pediatr Clin North Am. (2017) 64:1089–102. doi: 10.1016/j.pcl.2017.06.008
16. Hall MW, Knatz NL, Vetterly C, Tomarello S, Wewers MD, Volk HD, et al. Immunoparalysis and nosocomial infection in children with multiple organ dysfunction syndrome. Intensive Care Med. (2011) 37:525–32. doi: 10.1007/s00134-010-2088-x
17. Muszynski JA, Thakkar R, Hall MW. Inflammation and innate immune function in critical illness. Curr Opin Pediatr. (2016) 28:267–73. doi: 10.1097/MOP.0000000000000352
18. Mazer MB, CC C, Hanson J, Mannion D, Turnbull IR, Drewry A, et al. A whole blood enzyme-linked immunospot assay for functional immune endotyping of septic patients. J Immunol. (2021) 206:23–36. doi: 10.4049/jimmunol.2001088
19. Samuelsen AM HE, Lehman EB, McKeone DJ, Bonavia AS. Predicting organ dysfunction in septic and critically ill patients: A prospective cohort study using rapid ex vivo immune profiling. Crit Care Explor. (2024) 6(7):e1106. in print. doi: 10.1097/CCE.0000000000001106
20. Subbe CP, Kruger M, Rutherford P, Gemmel L. Validation of a modified Early Warning Score in medical admissions. QJM. (2001) 94:521–6. doi: 10.1093/qjmed/94.10.521
21. Gardner-Thorpe J, Love N, Wrightson J, Walsh S, Keeling N. The value of Modified Early Warning Score (MEWS) in surgical in-patients: a prospective observational study. Ann R Coll Surg Engl. (2006) 88:571–5. doi: 10.1308/003588406X130615
22. Singer M, Deutschman CS, Seymour CW, Shankar-Hari M, Annane D, Bauer M, et al. The third international consensus definitions for sepsis and septic shock (Sepsis-3). JAMA. (2016) 315:801–10. doi: 10.1001/jama.2016.0287
23. Charlson ME, Pompei P, Ales KL, MacKenzie CR. A new method of classifying prognostic comorbidity in longitudinal studies: development and validation. J Chronic Dis. (1987) 40:373–83. doi: 10.1016/0021-9681(87)90171-8
24. Knaus WA, Draper EA, Wagner DP, Zimmerman JE. APACHE II: a severity of disease classification system. Crit Care Med. (1985) 13:818–29. doi: 10.1097/00003246-198510000-00009
25. Ferreira FL, Bota DP, Bross A, Melot C, Vincent JL. Serial evaluation of the SOFA score to predict outcome in critically ill patients. Jama J Am Med Assoc. (2001) 286:1754–8. doi: 10.1001/jama.286.14.1754
26. Vincent JL, de Mendonca A, Cantraine F, Moreno R, Takala J, Suter PM, et al. Use of the SOFA score to assess the incidence of organ dysfunction/failure in intensive care units: Results of a multicenter, prospective study. Crit Care Med. (1998) 26:1793–800. doi: 10.1097/00003246-199811000-00016
27. Horan TC, Andrus M, Dudeck MA. CDC/NHSN surveillance definition of health care-associated infection and criteria for specific types of infections in the acute care setting. Am J Infect Control. (2008) 36:309–32. doi: 10.1016/j.ajic.2008.03.002
28. Wong HR, Caldwell JT, Cvijanovich NZ, Weiss SL, Fitzgerald JC, Bigham MT, et al. Prospective clinical testing and experimental validation of the Pediatric Sepsis Biomarker Risk Model. Sci Transl Med. (2019) 11. doi: 10.1126/scitranslmed.aax9000
29. Wong HR, Cvijanovich NZ, Anas N, Allen GL, Thomas NJ, Bigham MT, et al. Improved risk stratification in pediatric septic shock using both protein and mRNA biomarkers. PERSEVERE-XP. Am J Respir Crit Care Med. (2017) 196:494–501. doi: 10.1164/rccm.201701-0066OC
30. Nguyen J, Thompson JM, Balcarcel DR, Alder MN, McKeone DJ, Halstead ES, et al. Immunocompromised children with acute respiratory distress syndrome possess a distinct circulating inflammatory profile. Crit Care Explor. (2023) 5:e0844. doi: 10.1097/CCE.0000000000000844
31. Yehya N, Zinter MS, Thompson JM, Lim MJ, Hanudel MR, Alkhouli MF, et al. Identification of molecular subphenotypes in two cohorts of paediatric ARDS. Thorax. (2024) 79:128–34. doi: 10.1136/thorax-2023-220130
32. McKeone DJ, Mathewson M, Dalal PG, Spear D, Umstead TM, Hicks SD, et al. Cytokine panels and pediatric acute respiratory distress syndrome: A translational investigation. Pediatr Crit Care Med. (2020) 21:e1084–e93. doi: 10.1097/PCC.0000000000002531
33. Lin H, Scull BP, Goldberg BR, Abhyankar HA, Eckstein OE, Zinn DJ, et al. IFN-gamma signature in the plasma proteome distinguishes pediatric hemophagocytic lymphohistiocytosis from sepsis and SIRS. Blood Adv. (2021) 5:3457–67. doi: 10.1182/bloodadvances.2021004287
34. Debaugnies F, Mahadeb B, Nagant C, Meuleman N, De Bels D, Wolff F, et al. Biomarkers for early diagnosis of hemophagocytic lymphohistiocytosis in critically ill patients. J Clin Immunol. (2021) 41:658–65. doi: 10.1007/s10875-020-00950-z
35. Lacroix L, Papis S, Mardegan C, Luterbacher F, L’Huillier A, Sahyoun C, et al. Host biomarkers and combinatorial scores for the detection of serious and invasive bacterial infection in pediatric patients with fever without source. PloS One. (2023) 18:e0294032. doi: 10.1371/journal.pone.0294032
36. van Houten CB, de Groot JAH, Klein A, Srugo I, Chistyakov I, de Waal W, et al. A host-protein based assay to differentiate between bacterial and viral infections in preschool children (OPPORTUNITY): a double-blind, multicentre, validation study. Lancet Infect Dis. (2017) 17:431–40. doi: 10.1016/S1473-3099(16)30519-9
37. Fan Z, Kernan KF, Qin Y, Canna S, Berg RA, Wessel D, et al. Hyperferritinemic sepsis, macrophage activation syndrome, and mortality in a pediatric research network: a causal inference analysis. Crit Care. (2023) 27:347. doi: 10.1186/s13054-023-04628-x
38. Dolin HH, Papadimos TJ, Stepkowski S, Chen X, Pan ZK. A novel combination of biomarkers to herald the onset of sepsis prior to the manifestation of symptoms. Shock. (2018) 49:364–70. doi: 10.1097/SHK.0000000000001010
39. Song J, Park DW, Moon S, Cho HJ, Park JH, Seok H, et al. Diagnostic and prognostic value of interleukin-6, pentraxin 3, and procalcitonin levels among sepsis and septic shock patients: a prospective controlled study according to the Sepsis-3 definitions. BMC Infect Dis. (2019) 19:968. doi: 10.1186/s12879-019-4618-7
40. Karakike E, Giamarellos-Bourboulis EJ. Macrophage activation-like syndrome: A distinct entity leading to early death in sepsis. Front Immunol. (2019) 10:55. doi: 10.3389/fimmu.2019.00055
41. Karampela I, Chrysanthopoulou E, Skyllas G, Christodoulatos GS, Kandri E, Antonakos G, et al. Circulating leptin, soluble leptin receptor and free leptin index in critically ill patients with sepsis: a prospective observational study. Minerva Anestesiol. (2021) 87:880–90. doi: 10.23736/S0375-9393.21.15368-4
42. Sun S, Chen Y, Liu Z, Tian R, Liu J, Chen E, et al. Serum-soluble PD-L1 may be a potential diagnostic biomarker in sepsis. Scand J Immunol. (2021) 94:e13049. doi: 10.1111/sji.13049
43. Cohen J, Carlet J. INTERSEPT: an international, multicenter, placebo-controlled trial of monoclonal antibody to human tumor necrosis factor-alpha in patients with sepsis. Int Sepsis Trial Study Group Crit Care Med. (1996) 24:1431–40. doi: 10.1097/00003246-199609000-00002
44. Oved K, Cohen A, Boico O, Navon R, Friedman T, Etshtein L, et al. A novel host-proteome signature for distinguishing between acute bacterial and viral infections. PloS One. (2015) 10:e0120012. doi: 10.1371/journal.pone.0120012
45. Langedijk AC, Rengerink KO, Harding E, Wensing A, van Slooten R, Israeli Y, et al. Natural variability of TRAIL, IP-10, and CRP in healthy adults - The “HERACLES” study. Cytokine. (2024) 176:156530. doi: 10.1016/j.cyto.2024.156530
46. Bonavia AS, Samuelsen A, Chroneos ZC, Halstead ES. Comparison of rapid cytokine immunoassays for functional immune phenotyping. Front Immunol. (2022) 13:940030. doi: 10.3389/fimmu.2022.940030
47. Bonavia AS, Samuelsen A, Liang M, Hanson J, McKeone D, Chroneos ZC, et al. Comparison of whole blood cytokine immunoassays for rapid, functional immune phenotyping in critically ill patients with sepsis. Intensive Care Med Exp. (2023) 11:70. doi: 10.1186/s40635-023-00556-w
48. Sinha P, Calfee CS, Cherian S, Brealey D, Cutler S, King C, et al. Prevalence of phenotypes of acute respiratory distress syndrome in critically ill patients with COVID-19: a prospective observational study. Lancet Respir Med. (2020) 8:1209–18. doi: 10.1016/S2213-2600(20)30366-0
49. Hall MW, Gavrilin MA, Knatz NL, Duncan MD, Fernandez SA, Wewers MD. Monocyte mRNA phenotype and adverse outcomes from pediatric multiple organ dysfunction syndrome. Pediatr Res. (2007) 62:597–603. doi: 10.1203/PDR.0b013e3181559774
50. Hubl W, Tlustos L, Erath A, Andert S, Bayer PM. Proposed reference method for peripheral-blood monocyte counting using fluorescence-labelled monoclonal antibodies. Cytometry. (1996) 26:69–74. doi: 10.1002/(SICI)1097-0320(19960315)26:1<69::AID-CYTO11>3.0.CO;2-Q
51. Denstaedt SJ, Singer BH, Standiford TJ. Sepsis and nosocomial infection: patient characteristics, mechanisms, and modulation. Front Immunol. (2018) 9:2446. doi: 10.3389/fimmu.2018.02446
52. VanBuren JM, Hall M, Zuppa AF, Mourani PM, Carcillo J, Dean JM, et al. The design of nested adaptive clinical trials of multiple organ dysfunction syndrome children in a single study. Pediatr Crit Care Med. (2023) 24:e635–e46. doi: 10.1097/PCC.0000000000003332
53. Takahama M, Patil A, Richey G, Cipurko D, Johnson K, Carbonetto P, et al. A pairwise cytokine code explains the organism-wide response to sepsis. Nat Immunol. (2024) 25:226–39. doi: 10.1038/s41590-023-01722-8
54. Yoshimura T. Discovery of IL-8/CXCL8 (The story from Frederick). Front Immunol. (2015) 6:278. doi: 10.3389/fimmu.2015.00278
55. Harada A, Sekido N, Akahoshi T, Wada T, Mukaida N, Matsushima K. Essential involvement of interleukin-8 (IL-8) in acute inflammation. J Leukoc Biol. (1994) 56:559–64. doi: 10.1002/jlb.56.5.559
56. Bergquist M, Samuelsson L, Larsson A, Tyden J, Johansson J, Lipcsey M. TNFR1, TNFR2, neutrophil gelatinase-associated lipocalin and heparin binding protein in identifying sepsis and predicting outcome in an intensive care cohort. Sci Rep. (2020) 10:15350. doi: 10.1038/s41598-020-72003-9
57. Pimentel-Muinos FX, Seed B. Regulated commitment of TNF receptor signaling: a molecular switch for death or activation. Immunity. (1999) 11:783–93. doi: 10.1016/S1074-7613(00)80152-1
Keywords: sepsis, biomarker, phenotype, cytokines, critical illness, humans
Citation: Halstead ES, McKeone DJ, Samuelsen AM, Zhou S and Bonavia AS (2025) Functional immune profiling of hyper- and hypo-inflammatory subphenotypes of critical illness: a secondary analysis. Front. Immunol. 16:1520848. doi: 10.3389/fimmu.2025.1520848
Received: 31 October 2024; Accepted: 18 April 2025;
Published: 09 May 2025.
Edited by:
Beate E. Kehrel, University Hospital Münster, GermanyReviewed by:
Ivana Kawikova, National Institute of Mental Health, CzechiaCarolyn Calfee, University of California, San Francisco, United States
Copyright © 2025 Halstead, McKeone, Samuelsen, Zhou and Bonavia. This is an open-access article distributed under the terms of the Creative Commons Attribution License (CC BY). The use, distribution or reproduction in other forums is permitted, provided the original author(s) and the copyright owner(s) are credited and that the original publication in this journal is cited, in accordance with accepted academic practice. No use, distribution or reproduction is permitted which does not comply with these terms.
*Correspondence: E. Scott Halstead, ZWhhbHN0ZWFkQHBlbm5zdGF0ZWhlYWx0aC5wc3UuZWR1