- 1Department of Pulmonary Medicine, The University of Texas MD Anderson Cancer Center, Houston, TX, United States
- 2UTHealth Houston Graduate School of Biomedical Sciences, The University of Texas M.D. Anderson Cancer Center, Houston, TX, United States
- 3Department of Translational Molecular Pathology, The University of Texas MD Anderson Cancer Center, Houston, TX, United States
- 4Department of Melanoma Medical Oncology, The University of Texas MD Anderson Cancer Center, Houston, TX, United States
- 5Department of Infectious Diseases, Infection Control & Employee Health, The University of Texas MD Anderson Cancer Center, Houston, TX, United States
- 6Department of General Internal Medicine, The University of Texas MD Anderson Cancer Center, Houston, TX, United States
- 7Department of Molecular & Cellular Oncology, The University of Texas MD Anderson Cancer Center, Houston, TX, United States
Introduction: K-ras mutant lung adenocarcinoma (KM-LUAD) is a difficult-to-treat cancer subtype in which chronic inflammation pervades the tumor immune microenvironment (TIME). Pro-inflammatory pathways dampen the response to treatments, including immune checkpoint inhibitors, necessitating therapies that target this inflammatory signaling network in the TIME. One of the lynchpins of chronic inflammation in KM-LUAD is signal transducer and activator of transcription 3 (STAT3).
Methods: Here, we tested the anti-tumor and early immunotherapeutic efficacy of TTI-101, a selective small-molecule inhibitor of canonical STAT3 signaling, in a K-rasG12D mutant lung cancer mouse model (CC-LR).
Results: Treatment of CC-LR mice with TTI-101 resulted in reduced tumor burden while increasing dendritic cell (DC) and T helper 1 (Th1) infiltration into the TIME. TTI-101 treatment decreased pY-STAT3 expression in tumors with accompanying increases in several NF-κB anti-tumor target genes including CXCL9, a chemokine for primed T cells. Transcriptional profiling of the TIME revealed improved immune activation and anti-tumor skewing, as well as B cell signaling enrichment. Analysis of human LUAD data demonstrated negative correlations between STAT3 and Th1/DC infiltration, with DC infiltration also conferring improved survival in LUAD patients with low STAT3.
Discussion: Our results highlight the importance of STAT3 in driving early tumorigenesis and offer a preventative treatment window for high-risk individuals and patients with early-stage KM-LUAD.
1 Introduction
A quarter of global cancer-related deaths are attributable to lung cancer, of which 40% are histologically classified as lung adenocarcinoma (LUAD) (1–3). A large plurality (25%) of LUAD cases feature a driver mutation in K-ras, earning the moniker K-ras mutant LUAD (KM-LUAD) (3). Patients with KM-LUAD, especially those with a history of cigarette smoking, carry a poor prognosis, with K-ras mutations leading to hyperactive RAF-MEK-ERK signaling and unchecked cellular proliferation (2, 4, 5). While direct inhibition of K-ras has been attempted with some recent success, resistance mechanisms eventually appear, reversing any initial benefits (6, 7). As a result, new therapeutic strategies independent of K-ras signaling are urgently needed to provide better care for an exceedingly large cohort of lung cancer patients.
One promising treatment modality lies in targeting tumor-promoting inflammation. Tumor-promoting inflammation occurs when an initial anti-tumor immune response shifts from acute to chronic, and K-ras is well described as an intrinsic driver of this type of inflammation (8, 9). While the inflammation itself physically damages the lung tissue and promotes tumor proliferation, the flavor of inflammation changes from strongly T helper 1 (Th1) to other non-productive phenotypes such as Th17 and M2-type macrophage polarization (10). We and others have shown the importance of immune cells such as Th17s, myeloid-derived suppressor cells (MDSCs), M2-like macrophages, and neutrophils in lung cancer development via production of cytokines like interleukin 6 (IL-6), IL-17, IL-22, and IL-1β (11–13). In particular, we have shown that targeting IL-6 strongly reduces tumor-promoting inflammation and improves tumor burden in a murine KM-LUAD model (14). The importance of IL-6 signaling has become recognized clinically, with IL-6 neutralizing antibodies currently under study in concert with immune checkpoint inhibitors (NCT04691817).
While targeting IL-6 presents an excellent therapeutic opportunity, this modality is limited to the extracellular space. An alternative strategy to targeting IL-6 is to block key downstream mediators in the pathway, one such being signal transducer and activator of transcription 3 (STAT3). STAT3 plays a key role in pro-tumor machinery across many cell types of the tumor immune microenvironment (TIME). Activated STAT3 within tumor cells has been shown to promote tumor development through transcriptional activation of gene targets that improve cancer survival, stemness, proliferation, and invasiveness (15). In immune cells, STAT3 signaling encourages autocrine production of IL-6 that sustains tumor-promoting inflammation along with immunosuppressive factors such as IL-10 and TGFβ which blunt the anti-tumor response (16). STAT3 activation can occur independently of IL-6 targeting, as it lies downstream to other cytokines including IL-10, IL-22, and IL-27, which are broadly pro-tumor in nature and found in abundance in KM-LUAD (12, 17). Moreover, the use of a small molecule inhibitor enables the targeting of STAT3 in the cytoplasm or nucleus, and such an inhibitor can be upscaled for mass production more easily than a monoclonal antibody (18).
Despite STAT3 representing an attractive therapeutic target, there is disagreement as to the role of STAT3 in early versus late-stage tumors. Most groups have shown that late stage blocking of STAT3 is favorable for tumor clearance (19–28). However, others have shown that targeting STAT3 prior to or during tumorigenesis leads to a loss of epithelial cell identity and a worsening of disease (29, 30). To elucidate the role of STAT3 in early KM-LUAD development, we tested a selective small molecule STAT3 inhibitor, TTI-101, currently in phase II clinical trials, including treatment of idiopathic pulmonary fibrosis and hepatocellular carcinoma (NCT05671835 and NCT05440708, respectively), in a mouse model of KM-LUAD called CC-LR (31). Here, we profile the immune cells, cytokines, chemokines, and transcriptome of the TIME in CC-LR mice to illustrate the effects of STAT3 inhibition across a wide array of cell types. Mice treated during the initial stages of tumorigenesis displayed significant reduction in tumor burden, reprogramming of the TIME chemokine profile, and augmented dendritic cell (DC) and Th1 responses, supporting the hypothesis that STAT3 contributes to early-stage KM-LUAD development.
2 Methods
2.1 Cell culture
MDA-F471 cells, a Gprc5a-/- Kras-mutant LUAD cell line with STAT3 hyperactivation, were developed as previously described (32). Cells were cultured in complete growth medium consisting of DMEM (GenDEPOT) supplemented with 10% fetal bovine serum (FBS) (GenDEPOT) and 1% penicillin-streptomycin (GenDEPOT) and maintained at 37°C in a humidified atmosphere with 5% CO2. Cells were passaged upon reaching 80-90% confluency. Regular mycoplasma testing was provided by the MD Anderson Cytogenetics and Cell Authentication Core.
Cell viability was measured by MTT assay. Cells were seeded in 96-well plates at a density of 5,000 cells per well in 100 µL of complete growth medium and incubated overnight at 37°C in a 5% CO2 atmosphere in serum-free medium containing TTI-101 diluted serially 1:1 from 100-12.5 μM in DMSO; equivalent volumes of DMSO were added to vehicle control wells. After treatment, 10 µL of MTT solution (5 mg/mL in PBS) were added to each well to achieve a final concentration of 0.5 mg/mL. Wells of each concentration were set up in triplicate. Plates were incubated for 4 hours at 37°C to allow for formazan formation. Following incubation, medium was removed and replaced with 100 µL of DMSO to dissolve formazan crystals. The plates were gently shaken for 10–15 minutes to ensure complete dissolution. Absorbance was measured at 570 nm using a microplate reader, with a reference wavelength of 630 nm for background correction. Cell viability was calculated by normalizing absorbance values to untreated control wells.
2.2 Mouse husbandry and treatments
CCSPCre/LSL-K-rasG12D (CC-LR) mice were generated as previously described (14). Briefly, C57BL/6 background mice with the LSL-K-rasG12D allele were crossed with mice with Cre recombinase under the control of the club cell secretory protein (CCSP) locus. The resulting mice gain expression of mutant K-ras in the lung epithelium, leading to spontaneous tumor formation that parallels KM-LUAD pathology. All mice were housed under specific pathogen–free conditions and handled in accordance with the guidelines of the IACUC of MD Anderson Cancer Center. Mice were monitored daily for evidence of disease or distress. Both male and female mice were used in this study, and data were analyzed to check for sex-specific effects, which were not seen.
TTI-101 was provided by Dr. David J Tweardy under an approved material transfer agreement with Tvardi Therapeutics, Inc. Mice receiving TTI-101 intraperitoneally (i.p.) were given 100 mg/kg TTI-101 in DMSO five times weekly from 10-to-14 weeks of age (N = 8 controls, 9 TTI-101-treated). Oral gavage (o.g.) delivery of TTI-101 was formulated at 50 mg/kg in Labrasol: PEG 400 at a ratio of 3:2 and given once daily from 10-to-14 weeks of age (N = 21 controls, 25 TTI-101-treated). Mice were weighed daily to determine drug dose and to monitor for weight loss, a possible sign of toxicity or poor gavage technique. Dosing was performed as previously described (33, 34).
2.3 Mouse necropsy, bronchoalveolar lavage, plasma, and histology preparation
To harvest samples, 14-week-old CC-LR mice were anesthetized by i.p. injection of 0.8 mL of 0.25 mg/mL 2,2,2-tribromoethanol (Avertin, Sigma). Cannulas (Luer) were inserted into the tracheas and sutured into place, and lung surface tumors were then counted.
For bronchoalveolar lavage fluid (BALF) collection, a portion of mice received two sequential instillations of 1 mL of PBS via the cannulas. Blood was collected from the inferior vena cava and centrifuged at 2,370 x G for 5 minutes, and supernatants were collected as plasma. The lungs of these mice later underwent perfusion with 10 mL of PBS injected into the right ventricle. The lung tissue was then snap frozen in liquid nitrogen for downstream RNA and protein analyses. BALF total white blood cell (WBC) count was then obtained on a hemocytometer. Cytocentrifugation and subsequent Wright-Giemsa (Sigma) staining were performed to measure major immune cell lineages.
For histology preparation, a portion of mice had blood drawn and were perfused as described above, after which the lungs were inflated with 10% buffered formalin (Sigma) for 10 minutes via the cannulas. Lungs were removed and stored in formalin for 24 hours, followed by 24 hours in PBS. Lungs were then passed through an automated tissue embedder (Leica Biosystems) to make formalin fixed paraffin embedded (FFPE) blocks.
2.4 Quantification of TTI-101 drug concentrations in plasma
Plasma collected as above was mixed with stabilizer solution containing 20 mg/mL of NaF, 25 mg/mL of Na2SO3, and 25 mg/mL of L-ascorbic acid in ddH2O with 1X PBS at a 1:1 ratio. Aliquots of plasma from mice were spiked with 5ul of deuterated TTI-101(D7) as the internal standard (IS) to a final concentration of 5 μg/mL. Calibration standards and QC samples of TTI-101 were prepared by spiking 5 μL of the relevant working solutions of TTI-101 and SI into 100 μL of blank normal plasma. TTI-101 was extracted through one-step liquid-liquid extraction (LLE) using methyl tert-butyl ether (MTBE). Samples were reconstituted in 100 μL of methanol before analysis. The LC-MS/MS analysis was performed on a QTRAP 5500 Sciex hybrid quadrupole-linear ion trap system with a turbo ion spray source coupled to a Sciex LC Exion liquid chromatography system. Data acquisition and quantification were conducted using Analyst 1.6. (Redwood City, CA, USA). Chromatographic separation was achieved using a Synergi™ 4 µm Fusion-RP 80 Å, LC Column 50 x 2 mm at a temperature of 40°C with a 3 min linear gradient at 500 μL/mL. The aqueous mobile phase (solvent A) was created as follows: 0.1% (vol/vol) formic acid and 5 mM ammonium acetate in ddH2O. The organic phase (solvent B) was 0.1% formic acid and 5 mM ammonium acetate in methanol. MRM monitoring in positive mode (ESI+) was used to detect TTI-101 and the IS, with m/z 472.093 > 301.1 for TTI-101 and m/z 479.200 > 301.1 for IS. The calibration curve for TTI-101 was generated from the peak area ratio of TTI-101 to the peak area of its internal standard TTI-101(d7) using the Linear regression analysis with 1/X weight over the range of 0.03-30uM. All LC-MS/MS reagents including methanol, water, ammonium acetate, and formic acid were obtained from Honeywell Fluka (Morris Plains, NJ). Methyl tert-butyl ether (MTBE) was obtained from Sigma Aldrich. The C18 Synergi™ 4 µm Fusion-RP 80 Å LC column (50 × 2 mm) was purchased from Phenomenex, (Torrance, CA, USA).
2.5 Tissue histology
Hematoxylin and Eosin (H&E) staining was performed on multiple 5 μm-thick cuts from FFPE lungs, with individual sections separated by at least 50 μm. Slides were scanned at 4X magnification and stitched together on a BZ-X810 microscope (Keyence). Normal lung and lesion areas were manually annotated in ImageScope 12.4.3 (Leica Biosystems).
Immunohistochemistry (IHC) for Ki-67 (1:200, ab16667, Abcam) and pY-STAT3 (Tyr705) (1:200, 9145S, Cell Signaling Technology) were performed as previously described (14). Ki-67+ and pY-STAT3+ nuclei within tumors were quantified using ImageJ (NIH) as previously described (35). Additionally, pY-STAT3 staining and lesion type in o.g. CC-LR mice was quantified using HALO Indica Labs image analysis platform (version 3.5.3577.108).
Multiplex immunofluorescence staining (Lunaphore COMET) was performed on FFPE-derived sections as described in Wang et al. (36). Antibodies against major immune markers were sequentially stained, imaged, and inactivated to build multicolor image overlays. Images were imported into Visiopharm for segmentation, gating, and quantification (see Supplementary Table 1 for full antibody panel and cell segmentation logic).
2.6 RNA extraction and qRT-PCR analysis
Mouse lung RNA was extracted by placing cryopreserved lung tissue into tubes containing chrome plated steel beads and QIAzol (Qiagen). Tubes were then shaken on a Mini-BeadBeater 16 (BioSpec) for 10–20 seconds twice, incubating samples on ice between each round. Homogenates were then passed through a QIAshredder (Qiagen) for further cellular lysis. RNA extraction was then performed using the RNeasy Mini kit (Qiagen) following the manufacturer’s instructions. cDNA was made using the qScript cDNA SuperMix (Quanta Biosciences). qRT-PCR was run using SYBR Green FastMix (Quanta Bioscience) on a CFX96 Touch™ Real-Time PCR Detection System (Bio-Rad). Beta-actin (Actb) or CD45 (Ptprc) were used as housekeeping genes, with results presented as fold change using the ΔΔCt method. Primers used are listed in Supplementary Table 2.
2.7 Protein extraction and analysis
Mouse lung protein was extracted by homogenizing cryopreserved lung tissue in tubes containing chrome plated steel beads. For total protein, RIPA buffer (Sigma) with protease + phosphatase inhibitor cocktail (Thermo Fisher Scientific) was used; for nuclear and cytosolic fractionation, buffers from the NE-PER Nuclear Protein Extraction Kit (Pierce) were used. After incubating for 30 minutes on ice, samples were centrifuged at 18,620 x G for 30 minutes at 4°C, with supernatants collected. Protein concentration was determined using a bicinchoninic acid assay (Thermo Fisher Scientific) according to the manufacturer’s instructions.
BALF supernatants were analyzed by Olink Proximity Extension Assay using the Olink Target 48 (Olink Proteomics) panel for mouse immune markers. Samples were run by the Houston Methodist Research Institute Immunoediting Core according to the manufacturer’s instructions. Data were provided as NPX values (normalized protein expression with log2-type transformation) and pg/mL (absolute quantification).
Immunoblotting was performed as previously described (37), with nuclear fractions used for STAT3 detection and total protein used for p65. Primary antibodies were against STAT3 (1:1000; catalog 4904S, Cell Signaling Technology), p-p65 (Ser536) (1:1000, catalog 3033S, Cell Signaling), total p65 (1:1000, catalog ab32536, Abcam), histone H3 (1:1000; catalog 4499S, Cell Signaling Technology), and β-actin (1:1000; catalog 4970S, Cell Signaling Technology).
The NF-κB (p65) binding activity was measured using the NF-κB (p65) Transcription Factor Assay Kit (Cayman Chemical Company) as previously described (13), with 10 μg of nuclear extracts run in duplicate. Optical density (OD) was measured at 450 nm, and fold binding activity was calculated by normalizing to control ODs.
2.8 Flow cytometry
Flow cytometry was performed as previously described (13): Briefly, lungs were harvested as mentioned above but were then manually cut using scissors into a paste and then digested for 30–45 minutes at 37°C in 1 mg/mL collagenase IV (Gibco) in RPMI (GenDEPOT). Digested samples were then mechanically dissociated into single-cell suspensions using 70 μm nylon mesh (Falcon) and subjected to RBC lysis. Cells were then resuspended in FACS buffer (1X PBS, Sigma; 2 mM EDTA, Millipore Sigma; 1% FBS, GenDEPOT) for staining. 3 x 106 cells per sample were allocated to separate myeloid and lymphoid panels (see Supplementary Table 3 for complete antibody list and panel composition). Myeloid samples underwent Fc blocking with anti-CD16/CD32 (clone 2.4G2, Tonbo) concurrent with surface staining for 30 minutes on ice, followed by fixation with 1% formaldehyde. Lymphoid samples underwent intracellular cytokine stimulation: cells were cultured in RPMI (GenDEPOT) containing 50 ng/mL phorbol 12-myristate 13-acetate (PMA, Sigma), 500 ng/mL ionomycin (Sigma), 1 μL/mL GolgiStop (BD Biosciences), and 0.7 μL/mL GolgiPlug (BD Biosciences) for 4–6 hours at 37°C. After surface staining, cells were permeabilized with the FoxP3 Transcription Factor Staining Kit (Invitrogen) for intracellular/intranuclear markers. All data were acquired using an LSRFortessa X-20 (BD) and analyzed with FlowJo software, version 10 (Tree Star). Gating strategies are shown in Supplementary Figure 5.
2.9 Bulk RNA sequencing and GSEA
Following RNA extraction as described above, whole-transcriptome sequencing (RNA-seq), library construction, and gene set enrichment analysis (GSEA) were performed by Novogene (Beijing, China) using standard protocols, including quality control, library preparation with poly(A) selection, and paired-end sequencing on the Illumina platform. Data were processed and analyzed following Novogene’s recommended RNA-seq pipeline. GSEA results were generated by comparison with GO Enrichment gene sets.
2.10 Cellular deconvolution and TCGA analysis
RNA-seq immune profiles were deconvoluted from bulk RNA-seq data using the xCell (38) and CIBERSORT (39) algorithms in R (version 3.5.1; R Project for Statistical Computing). Gene expression data from LUAD patients, including both males and females, were obtained from TCGA (https://portal.gdc.cancer.gov). Linear regression was run to correlate immune cell infiltration with STAT3 expression. Overall survival was calculated for patients compared to B cell infiltration, and overall survival for patients with low STAT3 expression was compared to DC infiltration.
2.11 Statistics
Data are presented as mean ± SEM. Comparisons between control and TTI-101-treated groups were calculated by a 2-tailed t test, with P < 0.05 being statistically significant. All statistical analyses were performed in GraphPad Prism (Version 10.3.1).
2.12 Data availability statement
Data were generated by the authors and are available on request.
3 Results
3.1 TTI-101 reduces growth of tumor cells in KM-LUAD models
To test the effectiveness of TTI-101 on K-ras mutant cancer cells, we first treated a mouse-derived KM-LUAD cell line with increasing concentrations of TTI-101. The MDA-F471 mouse LUAD cell line, which is K-ras mutant and STAT3-addicted (32), demonstrated a dose-dependent decrease in viability upon treatment with TTI-101, with an IC50 of 14.74 μM (Supplementary Figure 1A).
We next examined TTI-101 in vivo using a KM-LUAD mouse model possessing K-rasG12D expression under the control of club cell secretory protein (CC-LR). TTI-101 was formulated in DMSO and delivered intraperitoneally (i.p.) at 100 mg/kg five days per week from 10-to-14 weeks-of-age. This treatment timeline was chosen in order to test for any tumor-preventative effects, since adenoma formation is evident at 14 weeks of age in the CC-LR model (40). Fourteen-week-old TTI-101-treated mice displayed significant reduction in tumor burden as measured by surface tumor number (Supplementary Figure 1B) and the ratio of tumor to healthy lung area by histology (Supplementary Figures 1C, D). Tumors from these mice showed reduced levels of activated STAT3 (STAT3 phosphorylated on Y705; pY-STAT3) using both pY-STAT3 immunohistochemistry (IHC; Supplementary Figures 1E, F) and Luminex bead-based assays (Supplementary Figure 1G).
To facilitate its potential use in patients, we next examined the effect of TTI-101 treatment (50 mg/kg once daily) in CC-LR mice again from 10-to-14 weeks of age using TTI-101 formulated in Labrasol/PEG-400 that allowed for its administration by oral gavage (o.g.) (41). Plasma TTI-101 levels reached 1952 +/- 914.5 ng/mL in treated mice and were below the lower limit of quantitation (LLOQ) for controls (Supplementary Table 4). Importantly, we witnessed a 39% reduction in tumor burden (p = 0.0053; Figures 1A–C). In addition, the number of Ki-67+ tumor cell nuclei was decreased, indicating reduced tumor cell proliferation (Figures 1D, E). As previously demonstrated, TTI-101 was well tolerated with no evidence of toxicity or weight loss observed (Figure 1F).
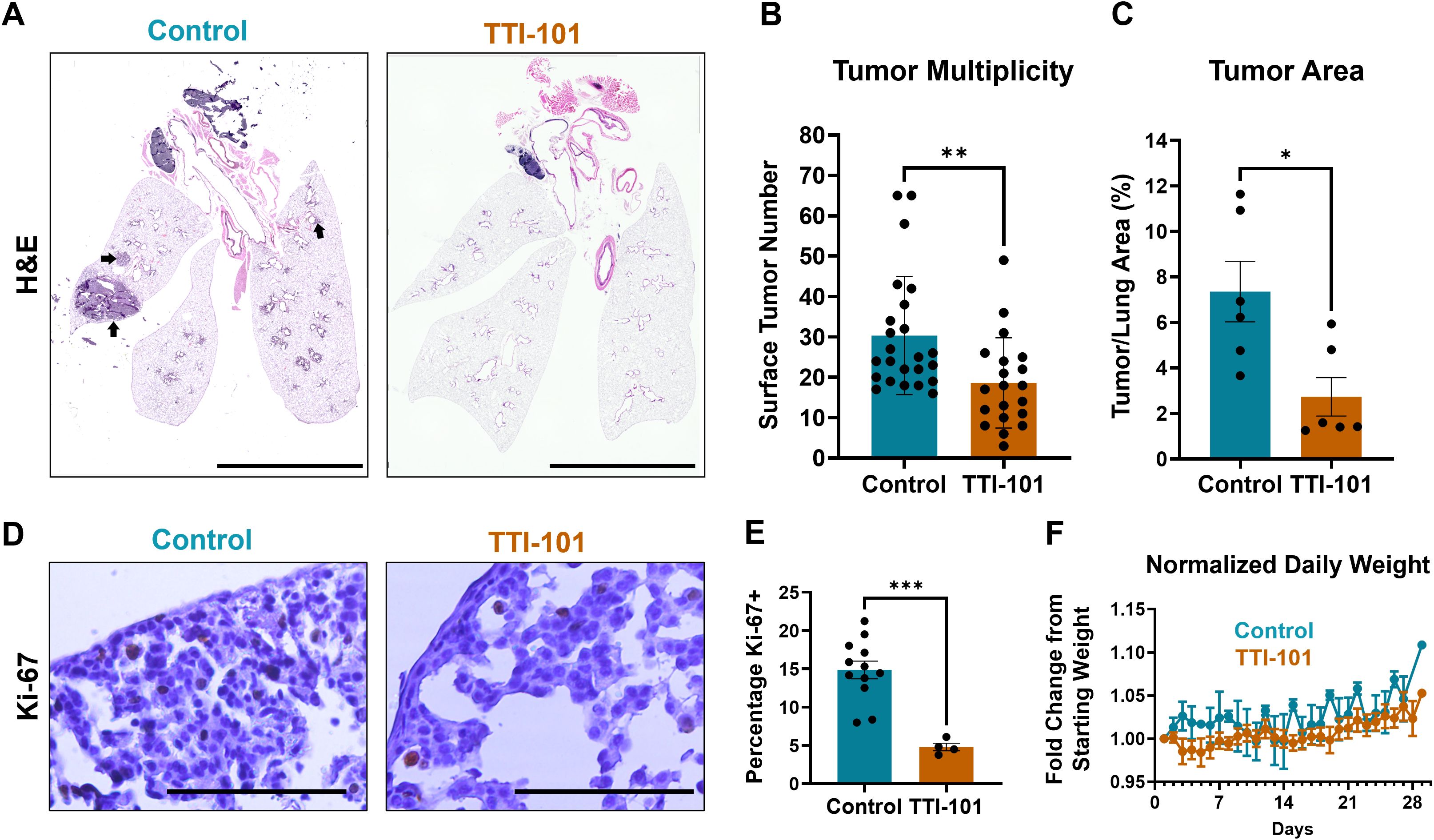
Figure 1. STAT3 inhibition reduces development and growth of lung tumors. (A) Representative stitched photomicrographs of H&E-stained sections of 14-week-old CC-LR mouse lungs either untreated or given TTI-101 by oral gavage (o.g.); arrows indicate representative tumor areas; original magnification, 4X; scale bar is 5 mm. (B) Tumor burden as measured by manual counting of lung surface tumors (N = 21-25). (C) Percentage of tumor area over total lung area (N = 6). (D) Representative photomicrographs of Ki-67-stained sections and quantified in (E) (N = 4-12); scale bar is 200 μm. (F) Weight, normalized to initial weight, of CC-LR mice given 50 mg/kg TTI-101 o.g. from 10-to-14 weeks-of-age. Data represent mean ± SEM. ***P < 0.001, **P < 0.01, *P < 0.5 by unpaired t test.
3.2 STAT3 inhibition reduces STAT3 signaling while skewing to an NF-κB-driven chemokine profile
To determine if STAT3 signaling was impacted by TTI-101, we measured STAT3 in CC-LR mice treated with TTI-101 by o.g. Early lesions displayed a lower density of pY-STAT3+ cells (Figures 2A, B), and immunoblotting of nuclear fractions from whole lung protein showed decreased translocation of STAT3 into the nucleus (Figures 2C, D). Since there is known modulation between STAT3 and NF-κB signaling (42), we performed immunoblotting to assess for NF-κB pathway components. However, we saw no changes in protein abundance of the NF-κB nuclear effector subunit p65 (Supplementary Figures 2A, B). To check for possible changes in transcriptional activity, we performed a p65 DNA binding assay, which measures the ability of the NF-κB p65 subunit to bind its transcriptional target sequences. Using this method, we saw a trend for increased p65 DNA binding (Figure 2E).
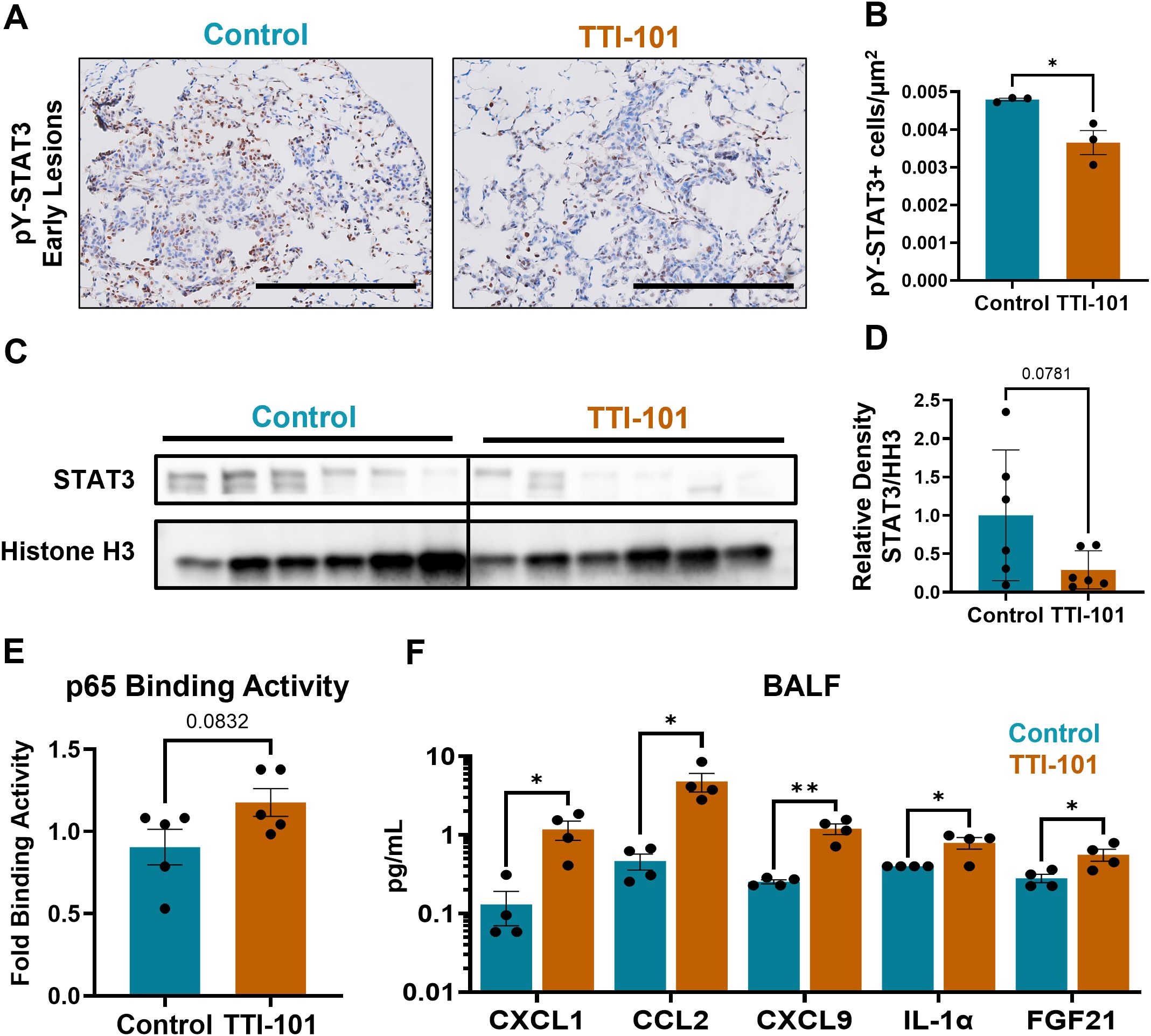
Figure 2. STAT3 inhibition reduces STAT3 signaling intensity while skewing to an NF-κB-driven chemokine profile. (A) Representative photomicrographs of pY-STAT3-stained sections in early lesions in CC-LR mice (N = 3); magnification, 20X; scale bar is 200 μm. (B) Quantification of pY-STAT3+ cells from CC-LR mice by μm2 of lesion area. (C) Immunoblot of STAT3 and histone H3 (HH3; loading control) in nuclear fractionated lung tissue lysates, quantified in (D) as relative density of STAT3 to HH3 (N = 6). (E) p65 DNA binding assay, with fold binding activity calculated based on OD of control samples (N = 5). (F) Olink protein extension assay analytes from bronchoalveolar lavage fluid (BALF) (N = 4). Data represent mean ± SEM. **P < 0.01, *P < 0.5 by unpaired t test.
To assess changes in the TIME and any downstream effects of STAT3 inhibition, we performed an Olink Proximity Extension Assay on bronchoalveolar lavage fluid (BALF) from CC-LR mice. BALF was chosen as a means of biomarker discovery and facilitating clinical translation because cytokine changes in BALF are more reflective of the secretory products of airway immune cells. Olink of BALF revealed upregulation of various soluble mediators (Figure 2F): Three chemokines, CXCL1, CCL2, and CXCL9, were upregulated, which provide chemotactic impetus to neutrophils, monocytes, and primed T cells respectively (43–45). IL-1α, a pro-inflammatory cytokine (46), was also upregulated. These four markers are notable as being transcriptional targets of NF-κB (47), although CXCL9 is also strongly induced by IFNγ (48). Normalized protein expression (NPX) data from Olink showed upregulation of other NF-κB targets: CCL22, CSF1, CSF2, CSF3, CXCL2, and TNFα (Supplementary Figure 2C). We also noted an increase in FGF21, a factor which promotes cholesterol metabolism and is known to cause exhaustion in T cells (49).
Collectively, these results indicate a possible shift in lung tumors from STAT3- to NF-κB-driven inflammation, with the reprogramming of the TIME evidenced primarily by changes in chemokine composition rather than prolific cytokine profile alterations.
3.3 STAT3 inhibition increases DC and Th1 proportion within the TIME
To better understand changes in the immune cell populations within the TIME, we performed flow cytometry of CC-LR mouse lungs treated with TTI-101 by o.g. In the myeloid compartment, the most significant changes were seen in dendritic cells (DCs), with classical type 1 (cDC1), classical type 2 (cDC2), and monocytic DC (Mo-DC) proportions elevated in the lung (Figures 3A, B). It should be noted, however, that our myeloid gating strategy was unable to satisfactorily delineate alveolar macrophages (AMs) and cDC1s, as both are CD11b- CD11c+. Therefore, we treated these cells as a lumped group labeled cDC1/AM. cDC2s displayed trends for increased MHC-II expression (Supplementary Figure 3C), indicating activation and antigen presentation ability. Flow cytometry of BALF indicated a trending increase in cDC2 infiltration and Ly6G low immature granulocytes (Supplementary Figure 3B), which may have migrated in response to elevated CCL2 and CXCL1 respectively (Figure 2F). Immature granulocytes may develop into polymorphonuclear MDSCs (PMN-MDSCs), and these findings will be explored in greater depth later.
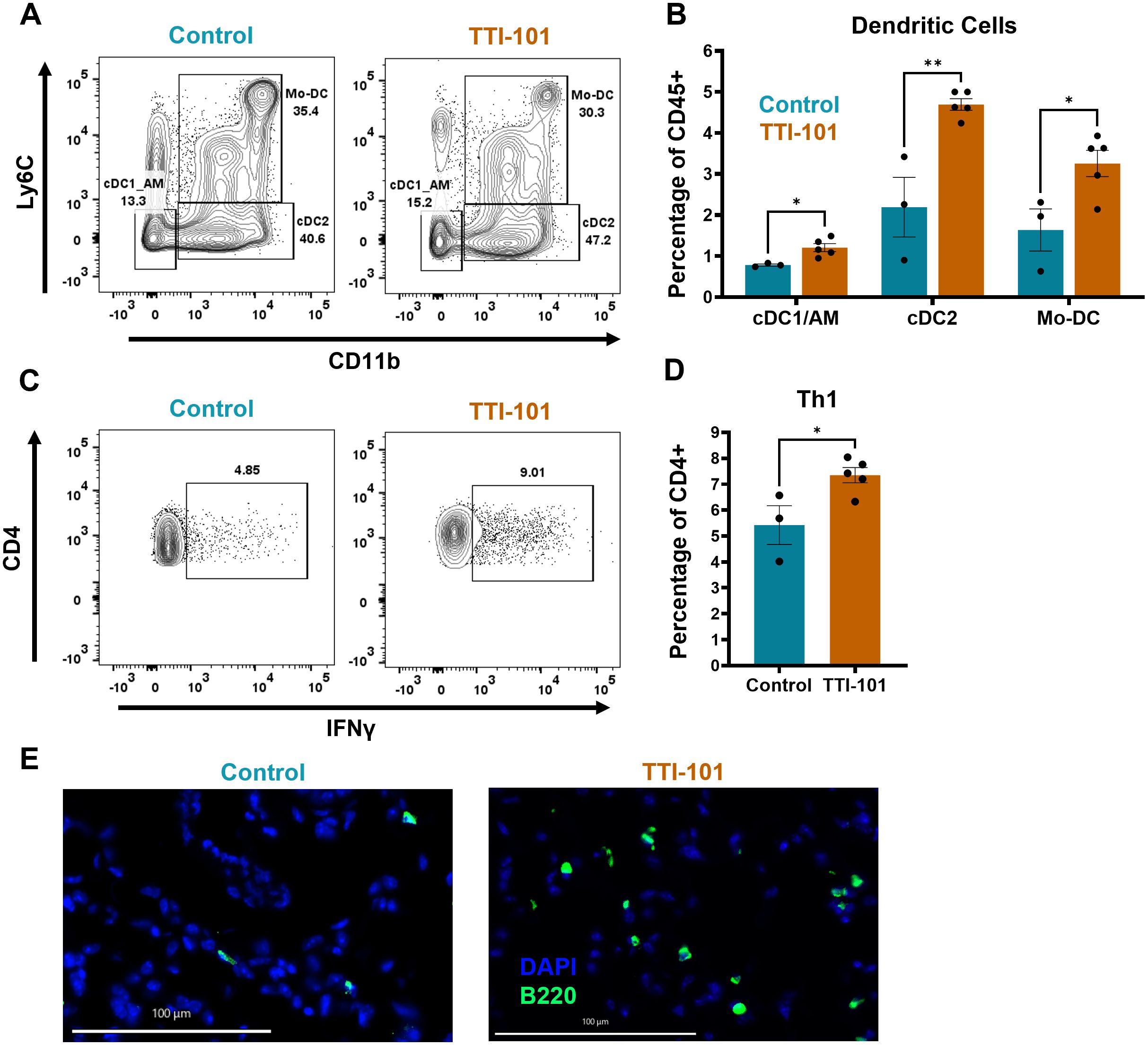
Figure 3. STAT3 inhibition increases DC and Th1 proportion within the TIME. (A) Representative dot plots of myeloid cell flow cytometry analysis pre-gated on CD11c+ cells; expression of Ly6C and CD11b is used to delineate classical type 1 DCs (cDC1s)/alveolar macrophages (AMs), cDC2s, and monocytic DCs (Mo-DCs), and is quantified in (B) as a percentage of CD45+ cells (N = 3-5). (C) Representative dot plots of lymphoid cell flow cytometry analysis with ex vivo restimulation by PMA/Ionomycin, pre-gated on CD3+ cells; expression of CD4 and IFNγ is used to mark T helper 1 (Th1) cells and is quantified in (D) as a percentage of CD4+ cells (N = 3-5). (E) Representative multiplex immunofluorescence (COMET) images for B cells (B220+); original magnification, 20X; scale bar is 100 µm. Data represent mean ± SEM. **P < 0.01, *P < 0.5 by unpaired t test.
Within the lymphoid compartment, we noted an increase in IFNγ-producing CD4+ helper T cells (Th1s) (Figures 3C, D). IFNγ transcripts (Ifng) were significantly elevated as measured by qRT-PCR, and expression of T-bet (Tbx21), the defining Th1 lineage transcription factor, trended higher (Supplementary Figure 3D). However, no significant changes were seen in other T cell subtypes (data not shown). We also noted a trend for increased B cell proportion by flow cytometry (Supplementary Figure 3E), an observation corroborated by immunofluorescence imaging of lung sections showing some heterogeneous increase in B cell infiltration into the lung (Figure 3E, Supplementary Figure 3F). Taken together, our findings suggest that STAT3 inhibition by TTI-101 augments DC infiltration into the TIME and bolsters the Th1 response.
3.4 Transcriptomic profiling indicates STAT3 inhibition broadly increased immune activation, particularly DCs and B cells
To better survey the effects of TTI-101 in our model in an unbiased manner, we performed whole-transcriptome sequencing (RNA-seq) of mRNA from whole lung lysates. Applying a cutoff rate of FDR > 2, we discovered 398 genes that were differentially expressed following STAT3 inhibition (Figure 4A). Gene set enrichment analysis (GSEA) by GO enrichment indicated downregulated pathways related to microtubules and cilia (Supplementary Figure 4A). Additionally, C2cd4b, a marker of acute inflammation (50), and Dlk1, a driver of Wnt signaling, lung repair and stemness, and cancer stemness (51–53), were downregulated (Figure 4A). However, a larger number of pathways related to immune activation were found to be significantly enriched (Figure 4B). Broad T, B, and myeloid cell activation pathways were seen, including cell adhesion, cell migration, B cell receptor (BCR) signaling, immune proliferation, and more. Several transcripts in these pathways were upregulated, including Blk (T cell activation) and Cd79a (mature B cells) (Figure 4A). GSEA also supported our findings of increased Th1/DC activation as well as enrichment of inhibitory gene sets governing Ras and ERK1/2 (Figure 4B), indicating that TTI-101 mitigates hyperactive K-ras signaling.
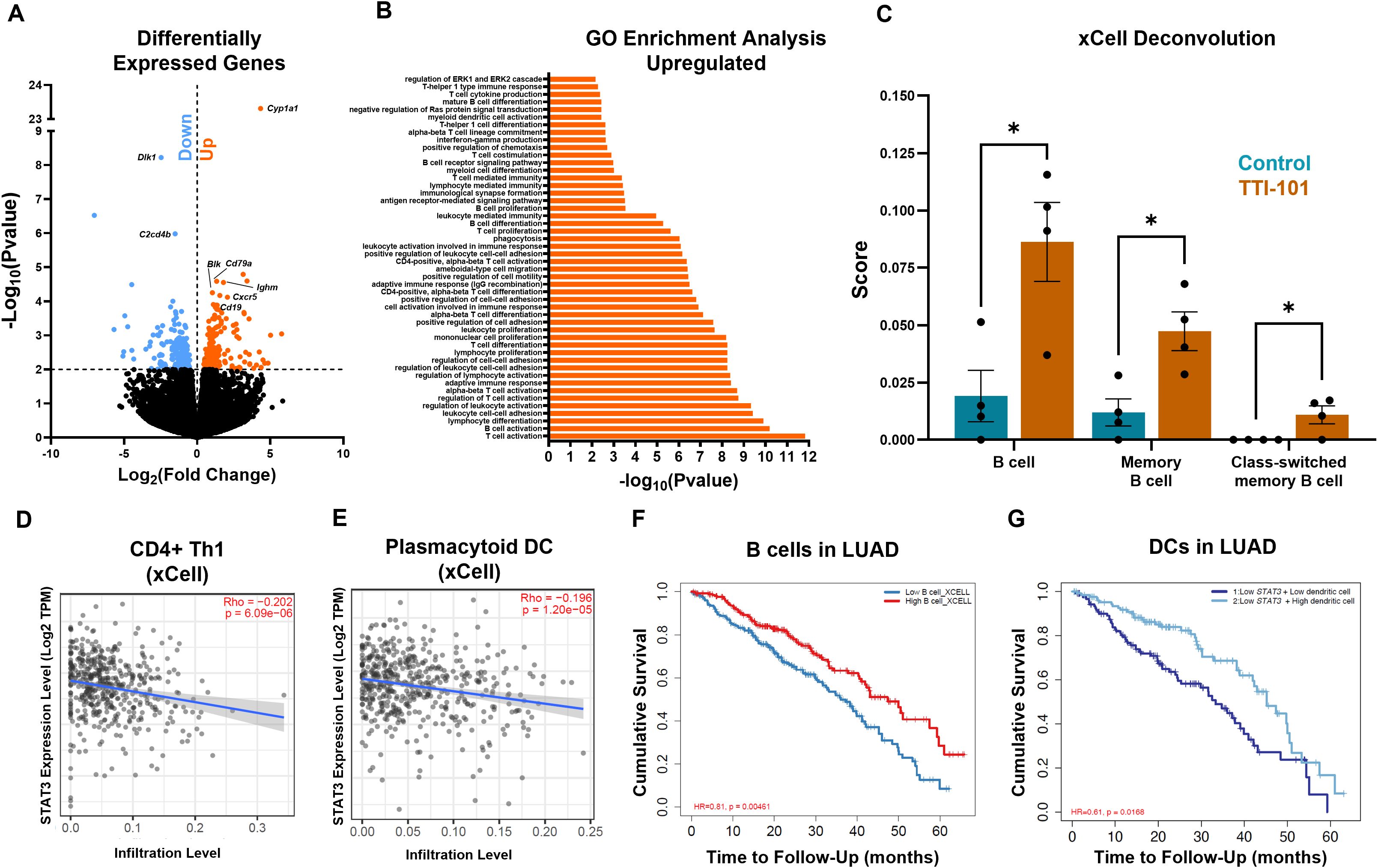
Figure 4. Transcriptomic profiling indicates STAT3 inhibition broadly increases immune activation. (A) Volcano plot of upregulated (orange) and downregulated (blue) genes following TTI-101 treatment in CC-LR mice (N = 4). (B) Go enrichment GSEA showing the top enriched pathways (N = 4). (C) Cellular deconvolution score of RNA-seq data by xCell. Correlation of STAT3 expression in LUAD patients (adjusted by stage) vs. Th1 (D) and plasmacytoid DC (E) infiltration (N = 515). Survival curves of B cells in LUAD (F) and DCs in LUAD with low STAT3 expression (G). Data represent mean ± SEM. *P < 0.5 by unpaired t test.
We next deconvoluted the bulk RNA-seq data to quantify immune cell infiltration. Using the xCell deconvolution method (38), we saw significant enrichment in B cells, memory B cells, and class-switched memory B cells (Figure 4C). B cell genes were seen to be upregulated, including Ighm (Ig mu heavy chain), Cd19 (B cell lineage), and Cxcr5 (B cell chemotaxis) (Figure 4A). Using xCell, we also noticed a higher score for common lymphoid progenitors (CLPs) (Supplementary Figure 4B). Use of CIBERSORT deconvolution (39) revealed a decrease in M2 score (Supplementary Figure 4C). Deconvolution scores were correlated with LUAD data from The Cancer Genome Atlas (TCGA): Th1s and plasmacytoid DCs negatively correlated with STAT3 expression in LUAD tumors (Figures 4D, E), a pattern we also noticed for CLPs (Supplementary Figure 4D). Owing to the B cell phenotype found in our RNA-seq data, we queried TCGA for LUAD patients and B cell infiltration. Our results showed that higher B cell infiltration led to increased cumulative survival (Figure 4F). Since we were operating in the setting of STAT3 inhibition, we narrowed our analysis to patients with low STAT3 expression. We found that patients with low STAT3 showed improved survival with higher infiltration of DCs (Figure 4G), supporting our finding of increased DC infiltration in CC-LR mice. These data suggest that DC tumor infiltration may serve as a predictor of response to STAT3 inhibition while suggesting a role for B cells in the anti-tumor response engendered by TTI-101.
4 Discussion
In this study, we demonstrated that inhibiting STAT3 with TTI-101 is an efficacious means of targeting a myriad of pro-tumor STAT3 functions across the tumor and immune compartments. In CC-LR mice, STAT3 inhibition attenuated tumor burden and tumor proliferation. Immunologically, we observed substantial reprogramming of the TIME: in addition to decreased STAT3 signaling, we observed upregulation of many NF-κB targets, including monocyte and T cell chemokines (CCL2 and CXCL9). Flow cytometry profiling of the lung revealed changes in both the myeloid and lymphoid compartments: DC proportions were higher following treatment with TTI-101, with a corresponding increase in Th1s. Increased immune activation was reinforced by differential gene analysis, with profiles for T, B, and myeloid cell activation showing significant positive enrichment, particularly for B cells. Comparisons with LUAD data from TCGA data likewise indicated inverse relationships between STAT3 expression and Th1 and DC infiltration. B cell and DC infiltration even correlated with improved survival.
Our results shed light on the temporal nature of STAT3 in lung tumorigenesis. Some studies have reported that early Stat3 ablation is pro-tumorigenic (29, 30). These studies showed that deletion of Stat3 in the lung epithelium concurrent with K-rasG12D induction fostered KM-LUAD development, growth, and lethality. The temporal nature of STAT3 function was illustrated by Zhou et al., who demonstrated that preemptive targeting of STAT3 prior to carcinogen exposure resulted in increased K-ras mutation rate, inflammation, and tumorigenesis. However, once tumors were established, Stat3 deletion proved integral to reducing tumor growth (26). In our hands, we were able to pharmacologically target STAT3 after initial tumor formation but saw decreased tumor growth. Therefore, we conclude that STAT3 inhibition is a viable means of treating KM-LUAD between initial tumor formation and tumor establishment.
Additionally, our results show that targeting STAT3 does not only affect tumor cells but repolarizes the TIME to an anti-tumor phenotype. Skewing from STAT3 to NF-κB signaling may explain the resulting changes in secreted factors. The NF-κB target CCL2, which is a known monocyte chemokine, is also a DC chemotactic factor (54) and may well explain the increased abundance of DCs within the lung. Likewise, CXCL9 is known to recruit primed T cells to sites of inflammation (45) and may correspond with our finding of increased Th1 prevalence.
DCs are well known to play an essential role in priming T cell-mediated anti-tumor immune responses (55, 56), and the trend for cDC2s to upregulate their MHC-II expression is indicative of increased activation and antigen presentation (57). While STAT3 is known to play an important role in DC maturation (58, 59), DC-specific knockouts of STAT3 in mice result in DCs that are fewer in number but greater in anti-tumor function and Th1 priming ability via IL-12 secretion (20, 60). Therefore, we suspect that TTI-101 enhances DC antigen presentation and T cell priming to elicit an anti-tumor response.
Th1 function is likewise influenced by STAT3 signaling: STAT3-derived IL-6 and TGFβ drive CD4 differentiation away from Th1 towards Th17, a CD4 subtype that is often pro-tumorigenic through IL-17-mediated recruitment of MDSCs (61–64). STAT3 likewise is important for regulatory T cell (Treg) differentiation via TGFβ (65), and deletion of STAT3 in the murine T cell compartment obliterates Treg tumor infiltration (66). In our hands, STAT3 inhibition using TTI-101 in the CC-LR model seems to promote Th1 function.
Evidence of anti-tumor synergy between myeloid and T cells is also mirrored in our RNA-seq results, with numerous anti-tumor immune signaling pathways showing significant enrichment. It is also telling that in LUAD tumors with low STAT3 expression, DC infiltration is predictive of survival. In subsequent studies, we will focus more on the DC:T interaction and the priming process in response to TTI-101.
It is worth noting that while we only saw a trending increase in B cell abundance in the lung, transcriptomic profiling indicated increased BCR signaling and B cell activity, and cellular deconvolution predicted increased B cell enrichment. Humoral immunity and tertiary lymphoid structures (TLSs) are known positive prognostic indicators in KM-LUAD (67, 68); while we saw no evidence of TLSs (data not shown), it is likely that humoral immunity plays a role in the response to TTI-101 and merits further investigation.
We also detected TTI-101-induced gene transcript changes that suggest potential pathways for the emergence of TTI-101 resistance. For example, FGF21, which inhibits CD8 T cells by boosting cholesterol metabolism (49), was upregulated in BALF and may herald blunting of the anti-tumor responses. Similarly, increased CXCL1, a known neutrophil chemokine (43), could lead to recruitment of PMN-MDSCs, which are immunosuppressive and a negative prognostic indicator in KM-LUAD (10).
While this study demonstrated the efficacy of STAT3 inhibition via TTI-101 in reducing tumor burden and in reprogramming inflammation in KM-LUAD, several limitations should be addressed. First, we have studied the role of TTI-101 in a preventative setting in CC-LR mice. Our early treatment regimen is most translatable to high-risk patients who, through improved early screening, may benefit from a prophylactic intervention. However, providing the drug once tumors are established or increasing drug exposure may yield different results, and we plan to study STAT3 inhibition at later timepoints moving forward. Second, although we observed increased immune activation, including DC and Th1 responses, the precise mechanism of immune priming and the durability of this response remain unclear. While we see tumor-intrinsic effects of STAT3 inhibition in terms of reduced tumor cell proliferation, we do not see changes in angiogenesis or apoptosis markers (data not show). This suggests that tumor-extrinsic STAT3 function in DCs and T cells play a marked role. In the same vein, we cannot precisely define if one DC subset or another is playing a greater role, and we cannot rule out a role for AMs, since they were indistinguishable from cDC1s in our gating. Additionally, while transcriptomic analyses suggested increased B cell enrichment and activity, our flow and COMET results are limited and only trending. The functional contribution of humoral immunity and its role in the anti-tumor response in this model warrant further investigation. Moreover, the CCR2/CCL2 pathway recruits mononuclear MDSCs (M-MDSCs) into tumors, in addition to monocytes and DCs (69).
We will focus future studies on longitudinal monitoring of M- and PMN-MDSC recruitment to further dissect the interplay between MDSCs, DCs, T cells, and B cells in shaping anti-tumor immunity. Since the use of TTI-101 alone did not completely abrogate lung tumor burden, and since TTI-101 did not appear to alter CD8 number or their cytokine profiles (data not shown), we plan to combine TTI-101 with anti-PD-1 to see if there are synergistic effects that could boost cytotoxicity. In addition, it will be interesting to test combination therapy with K-ras inhibitors, conventional chemotherapy, and other immunotherapeutic interventions such as DC vaccination to improve DC function and T cell priming. As this study was limited to the early-stage lung tumor setting, we also plan to study the effects of TTI-101 in later-stage tumors alone and in combination with the other therapies mentioned.
In conclusion, our findings establish STAT3 inhibition via TTI-101 as a promising early therapeutic/preventative approach for KM-LUAD that can reduce tumor burden, reprogram the TIME, and enhance anti-tumor immunity.
Data availability statement
The original contributions presented in this article are deposited in the GEO repository, accession number GSE294807. The individual samples are stored in GSM8920639-GSM8920646.
Ethics statement
Ethical approval was not required for the study involving humans in accordance with the local legislation and institutional requirements. Written informed consent to participate in this study was not required from the participants or the participants’ legal guardians/next of kin in accordance with the national legislation and the institutional requirements. The animal study was approved by IACUC of MD Anderson Cancer Center. The study was conducted in accordance with the local legislation and institutional requirements.
Author contributions
MC: Formal Analysis, Funding acquisition, Investigation, Methodology, Project administration, Resources, Validation, Visualization, Writing – original draft, Writing – review & editing. ZR: Data curation, Formal Analysis, Investigation, Software, Validation, Visualization, Writing – review & editing. SC: Formal Analysis, Investigation, Software, Validation, Visualization, Writing – review & editing. AK: Formal Analysis, Investigation, Visualization, Writing – review & editing. BY: Formal Analysis, Investigation, Visualization, Writing – review & editing. LH: Formal Analysis, Software, Validation, Visualization, Writing – review & editing. TE: Formal Analysis, Investigation, Validation, Visualization, Writing – review & editing. MK: Formal Analysis, Investigation, Validation, Visualization, Writing – review & editing. SL: Formal Analysis, Investigation, Visualization, Writing – review & editing. SP: Formal Analysis, Investigation, Visualization, Writing – review & editing. MR: Formal Analysis, Investigation, Visualization, Writing – review & editing. AT: Formal Analysis, Investigation, Visualization, Writing – review & editing. CR: Formal Analysis, Investigation, Visualization, Writing – review & editing. KL: Formal Analysis, Investigation, Visualization, Writing – review & editing. MG: Formal Analysis, Investigation, Visualization, Writing – review & editing. SD: Formal Analysis, Investigation, Visualization, Writing – review & editing. NK: Formal Analysis, Investigation, Visualization, Writing – review & editing. CC: Formal Analysis, Investigation, Visualization, Writing – review & editing. WV: Formal Analysis, Investigation, Visualization, Writing – review & editing. MZ: Formal Analysis, Investigation, Visualization, Writing – review & editing. SA: Formal Analysis, Investigation, Software, Visualization, Writing – review & editing. LS: Funding acquisition, Resources, Software, Supervision, Writing – review & editing. EO: Formal Analysis, Resources, Software, Supervision, Writing – review & editing. HK: Funding acquisition, Methodology, Project administration, Resources, Software, Supervision, Writing – review & editing. SE: Project administration, Resources, Software, Supervision, Writing – review & editing. DT: Conceptualization, Funding acquisition, Methodology, Project administration, Resources, Software, Supervision, Writing – review & editing. SM: Conceptualization, Funding acquisition, Investigation, Methodology, Project administration, Resources, Software, Supervision, Validation, Writing – original draft, Writing – review & editing.
Funding
The author(s) declare that financial support was received for the research and/or publication of this article. This study was supported by an NIH/NCI R01 grant (R01CA225977) awarded to SM, an R01 grant (1R01CA279452-01A1) awarded to DT and SM, and R01 grants awarded to HK and SM (R01CA248731 and R01CA272863). MC was supported by an F31 fellowship (F31CA261121) from NIH/NCI. SL, SP, AT, and SA were supported by the CPRIT Research Training Award CPRIT Training Program (RP 210028). CC was supported by the King Foundation High School Summer.
Conflict of interest
The authors declare that the research was conducted in the absence of any commercial or financial relationships that could be construed as a potential conflict of interest.
The author(s) declared that they were an editorial board member of Frontiers, at the time of submission. This had no impact on the peer review process and the final decision.
Generative AI statement
The author(s) declare that no Generative AI was used in the creation of this manuscript.
Publisher’s note
All claims expressed in this article are solely those of the authors and do not necessarily represent those of their affiliated organizations, or those of the publisher, the editors and the reviewers. Any product that may be evaluated in this article, or claim that may be made by its manufacturer, is not guaranteed or endorsed by the publisher.
Supplementary material
The Supplementary Material for this article can be found online at: https://www.frontiersin.org/articles/10.3389/fimmu.2025.1575181/full#supplementary-material
References
1. Siegel RL, Miller KD, Jemal A. Cancer statistics, 2019. CA: Cancer J Clin. (2019) 69:7–34. doi: 10.3322/caac.21551
2. Stellman SD, Takezaki T, Wang L, Chen Y, Citron ML, Djordjevic MV, et al. Smoking and lung cancer risk in American and Japanese men: an international case-control study. Cancer Epidemiol Prev Biomarkers. (2001) 10:1193–9.
3. Goldstraw P, Ball D, Jett JR, Le Chevalier T, Lim E, Nicholson AG, et al. Non-small-cell lung cancer. Lancet. (2011) 378:1727–40. doi: 10.1016/S0140-6736(10)62101-0
4. Cully M. Closing the door on KRAS-mutant lung cancer. Nat Rev Drug Discovery. (2016) 15:747–7. doi: 10.1038/nrd.2016.216
5. Jančík S, Drábek J, Radzioch D, Hajdúch M. Clinical relevance of KRAS in human cancers. BioMed Res Int. (2010) 2010. doi: 10.1155/2010/150960
6. Mayekar MK, Bivona TG. Current landscape of targeted therapy in lung cancer. Clin Pharmacol Ther. (2017) 102:757–64. doi: 10.1002/cpt.v102.5
7. Ash LJ, Busia-Bourdain O, Okpattah D, Kamel A, Liberchuk A, Wolfe AL. KRAS: biology, inhibition, and mechanisms of inhibitor resistance. Curr Oncol. (2024) 31:2024–46. doi: 10.3390/curroncol31040150
8. Greten FR, Grivennikov SI. Inflammation and cancer: triggers, mechanisms, and consequences. Immunity. (2019) 51:27–41. doi: 10.1016/j.immuni.2019.06.025
9. Ji H, Houghton A, Mariani T, Perera S, Kim C, Padera R, et al. K-ras activation generates an inflammatory response in lung tumors. Oncogene. (2006) 25:2105–12. doi: 10.1038/sj.onc.1209237
10. Deng S, Clowers MJ, Velasco WV, Ramos-Castaneda M, Moghaddam SJ. Understanding the complexity of the tumor microenvironment in K-ras mutant lung cancer: finding an alternative path to prevention and treatment. Front Oncol. (2019) 9:1556. doi: 10.3389/fonc.2019.01556
11. Chang SH, Mirabolfathinejad SG, Katta H, Cumpian AM, Gong L, Caetano MS, et al. T helper 17 cells play a critical pathogenic role in lung cancer. Proc Natl Acad Sci. (2014) 111:5664–9. doi: 10.1073/pnas.1319051111
12. Khosravi N, Caetano MS, Cumpian AM, Unver N, De la Garza Ramos C, Noble O, et al. IL22 promotes Kras mutant lung cancer by induction of a pro-tumor immune response and protection of stemness properties. Cancer Immunol Res. (2018) 6:788–97. doi: 10.1158/2326-6066.CIR-17-0655
13. Yuan B, Clowers MJ, Velasco WV, Peng S, Peng Q, Shi Y, et al. Targeting IL-1β as an immunopreventive and therapeutic modality for K-ras-mutant lung cancer. JCI Insight. (2022) 7. doi: 10.1172/jci.insight.157788
14. Caetano MS, Zhang H, Cumpian AM, Gong L, Unver N, Ostrin EJ, et al. IL6 blockade reprograms the lung tumor microenvironment to limit the development and progression of K-ras-mutant lung cancer. Cancer Res. (2016) 76:3189–99. doi: 10.1158/0008-5472.CAN-15-2840
15. Clowers MJ, Moghaddam SJ. Cell type-specific roles of STAT3 signaling in the pathogenesis and progression of K-ras mutant lung adenocarcinoma. Cancers (Basel). (2022) 14. doi: 10.3390/cancers14071785
16. Carpenter RL, Lo HW. STAT3 target genes relevant to human cancers. Cancers (Basel). (2014) 6:897–925. doi: 10.3390/cancers6020897
17. Roca Suarez AA, Van Renne N, Baumert TF, Lupberger J. Viral manipulation of STAT3: Evade, exploit, and injure. PLoS Pathog. (2018) 14:e1006839. doi: 10.1371/journal.ppat.1006839
18. Castelli MS, McGonigle P, Hornby PJ. The pharmacology and therapeutic applications of monoclonal antibodies. Pharmacol Res Perspect. (2019) 7:e00535. doi: 10.1002/prp2.v7.6
19. Gotthardt D, Putz EM, Straka E, Kudweis P, Biaggio M, Poli V, et al. Loss of STAT3 in murine NK cells enhances NK cell-dependent tumor surveillance. Blood. (2014) 124:2370–9. doi: 10.1182/blood-2014-03-564450
20. Iwata-Kajihara T, Sumimoto H, Kawamura N, Ueda R, Takahashi T, Mizuguchi H, et al. Enhanced cancer immunotherapy using STAT3-depleted dendritic cells with high Th1-inducing ability and resistance to cancer cell-derived inhibitory factors. J Immunol. (2011) 187:27–36. doi: 10.4049/jimmunol.1002067
21. Kujawski M, Kortylewski M, Lee H, Herrmann A, Kay H, Yu H. Stat3 mediates myeloid cell-dependent tumor angiogenesis in mice. J Clin Invest. (2008) 118:3367–77. doi: 10.1172/JCI35213
22. Rébé C, Végran F, Berger H, Ghiringhelli F. STAT3 activation: A key factor in tumor immunoescape. JAKSTAT. (2013) 2:e23010. doi: 10.4161/jkst.23010
23. Looyenga BD, Hutchings D, Cherni I, Kingsley C, Weiss GJ, Mackeigan JP. STAT3 is activated by JAK2 independent of key oncogenic driver mutations in non-small cell lung carcinoma. PLoS One. (2012) 7:e30820. doi: 10.1371/journal.pone.0030820
24. Tong M, Wang J, Jiang N, Pan H, Li D. Correlation between p-STAT3 overexpression and prognosis in lung cancer: A systematic review and meta-analysis. PLoS One. (2017) 12:e0182282. doi: 10.1371/journal.pone.0182282
25. Yu H, Pardoll D, Jove R. STATs in cancer inflammation and immunity: a leading role for STAT3. Nat Rev Cancer. (2009) 9:798–809. doi: 10.1038/nrc2734
26. Zhou J, Qu Z, Yan S, Sun F, Whitsett JA, Shapiro SD, et al. Differential roles of STAT3 in the initiation and growth of lung cancer. Oncogene. (2015) 34:3804–14. doi: 10.1038/onc.2014.318
27. Zhou Z, Wang M, Li J, Xiao M, Chin YE, Cheng J, et al. SUMOylation and SENP3 regulate STAT3 activation in head and neck cancer. Oncogene. (2016) 35:5826–38. doi: 10.1038/onc.2016.124
28. Zhou J, Qu Z, Sun F, Han L, Li L, Yan S, et al. Myeloid STAT3 promotes lung tumorigenesis by transforming tumor immunosurveillance into tumor-promoting inflammation. Cancer Immunol Res. (2017) 5:257–68. doi: 10.1158/2326-6066.CIR-16-0073
29. D’Amico S, Shi J, Martin BL, Crawford HC, Petrenko O, Reich NC. STAT3 is a master regulator of epithelial identity and KRAS-driven tumorigenesis. Genes Dev. (2018) 32:1175–87. doi: 10.1101/gad.311852.118
30. Grabner B, Schramek D, Mueller KM, Moll HP, Svinka J, Hoffmann T, et al. Disruption of STAT3 signalling promotes KRAS-induced lung tumorigenesis. Nat Commun. (2015) 6:6285. doi: 10.1038/ncomms7285
31. Lewis KM, Bharadwaj U, Eckols TK, Kolosov M, Kasembeli MM, Fridley C, et al. Small-molecule targeting of signal transducer and activator of transcription (STAT) 3 to treat non-small cell lung cancer. Lung Cancer. (2015) 90:182–90. doi: 10.1016/j.lungcan.2015.09.014
32. Fujimoto J, Kadara H, Men T, Pelt van C, Lotan D, Lotan R. Comparative functional genomics analysis of NNK tobacco-carcinogen induced lung adenocarcinoma development in Gprc5a-knockout mice. PLoS One. (2010) 5:e11847. doi: 10.1371/journal.pone.0011847
33. Bharadwaj U, Eckols TK, Xu X, Kasembeli MM, Chen Y, Adachi M, et al. Small-molecule inhibition of STAT3 in radioresistant head and neck squamous cell carcinoma. Oncotarget. (2016) 7:26307–30. doi: 10.18632/oncotarget.v7i18
34. Kasembeli MM, Singhmar P, Ma J, Edralin J, Tang Y, Adams C, et al. TTI-101: A competitive inhibitor of STAT3 that spares oxidative phosphorylation and reverses mechanical allodynia in mouse models of neuropathic pain. Biochem Pharmacol. (2021) 192:114688. doi: 10.1016/j.bcp.2021.114688
35. Crowe AR, Yue W. Semi-quantitative determination of protein expression using immunohistochemistry staining and analysis: an integrated protocol. Bio Protoc. (2019) 9. doi: 10.21769/BioProtoc.3465
36. Wang B, Reville PK, Yassouf MY, Jelloul FZ, Ly C, Desai PN, et al. Comprehensive characterization of IFNγ signaling in acute myeloid leukemia reveals prognostic and therapeutic strategies. Nat Commun. (2024) 15:1821. doi: 10.1038/s41467-024-45916-6
37. Gong L, da Silva Caetano M, Cumpian AM, Daliri S, Flores Garza A, Chang SH, et al. Tumor necrosis factor links chronic obstructive pulmonary disease and K-ras mutant lung cancer through induction of an immunosuppressive pro-tumor microenvironment. Oncoimmunology. (2016) 5:e1229724. doi: 10.1080/2162402X.2016.1229724
38. Aran D. Cell-type enrichment analysis of bulk transcriptomes using xCell. Methods Mol Biol. (2020) 2120:263–76. doi: 10.1007/978-1-0716-0327-7_
39. Newman AM, Liu CL, Green MR, Gentles AJ, Feng W, Xu Y, et al. Robust enumeration of cell subsets from tissue expression profiles. Nat Methods. (2015) 12:453–7. doi: 10.1038/nmeth.3337
40. Moghaddam SJ, Li H, Cho SN, Dishop MK, Wistuba II, Ji L, et al. Promotion of lung carcinogenesis by chronic obstructive pulmonary disease–like airway inflammation in a K-ras–induced mouse model. Am J Respir Cell Mol Biol. (2009) 40:443–53. doi: 10.1165/rcmb.2008-0198OC
41. McCartney F, Jannin V, Chevrier S, Boulghobra H, Hristov DR, Ritter N, et al. Labrasol® is an efficacious intestinal permeation enhancer across rat intestine: Ex vivo and in vivo rat studies. J Control Release. (2019) 310:115–26. doi: 10.1016/j.jconrel.2019.08.008
42. Deng S, Ramos-Castaneda M, Velasco WV, Clowers MJ, Gutierrez BA, Noble O, et al. Interplay between estrogen and Stat3/NF-κB driven immunomodulation in lung cancer. Carcinogenesis. (2020) 41:1529–42. doi: 10.1093/carcin/bgaa064
43. De Filippo K, Dudeck A, Hasenberg M, Nye E, van Rooijen N, Hartmann K, et al. Mast cell and macrophage chemokines CXCL1/CXCL2 control the early stage of neutrophil recruitment during tissue inflammation. Blood. (2013) 121:4930–7. doi: 10.1182/blood-2013-02-486217
44. Lin Z, Shi JL, Chen M, Zheng ZM, Li MQ, Shao J, et al. CCL2: An important cytokine in normal and pathological pregnancies: A review. Front Immunol. (2022) 13:1053457. doi: 10.3389/fimmu.2022.1053457
45. Ozga AJ, Chow MT, Luster AD. Chemokines and the immune response to cancer. Immunity. (2021) 54:859–74. doi: 10.1016/j.immuni.2021.01.012
46. Mantovani A, Barajon I, Garlanda C. IL-1 and IL-1 regulatory pathways in cancer progression and therapy. Immunol Rev. (2018) 281:57–61. doi: 10.1111/imr.2018.281.issue-1
47. Liu T, Zhang L, Joo D, Sun SC. NF-κB signaling in inflammation. Signal Transduct Target Ther. (2017) 2:17023–. doi: 10.1038/sigtrans.2017.23
48. Berthoud TK, Dunachie SJ, Todryk S, Hill AV, Fletcher HA. MIG (CXCL9) is a more sensitive measure than IFN-gamma of vaccine induced T-cell responses in volunteers receiving investigated malaria vaccines. J Immunol Methods. (2009) 340:33–41. doi: 10.1016/j.jim.2008.09.021
49. Hu C, Qiao W, Li X, Ning ZK, Liu J, Dalangood S, et al. Tumor-secreted FGF21 acts as an immune suppressor by rewiring cholesterol metabolism of CD8. Cell Metab. (2024) 36:630–647.e638. doi: 10.1016/j.cmet.2024.01.005
50. Han F, Qian L, Zhang Y, Liu P, Li R, Chen M. C2CD4A/B variants in the predisposition of lung cancer in the Chinese Han population. Funct Integr Genomics. (2022) 22:331–40. doi: 10.1007/s10142-022-00827-x
51. Pittaway JFH, Lipsos C, Mariniello K, Guasti L. The role of delta-like non-canonical Notch ligand 1 (DLK1) in cancer. Endocr Relat Cancer. (2021) 28:R271–87. doi: 10.1530/ERC-21-0208
52. Finn J, Lipsos C, Mariniello K, Guasti L. Dlk1-mediated temporal regulation of notch signaling is required for differentiation of alveolar type II to type I cells during repair. Cell Rep. (2019) 26:2942–54.e2945. doi: 10.1016/j.celrep.2019.02.046
53. Grassi ES, Pietras A. Emerging roles of DLK1 in the stem cell niche and cancer stemness. J Histochem Cytochem. (2022) 70:17–28. doi: 10.1369/00221554211048951
54. Hao Q, Vadgama JV, Wang P. CCL2/CCR2 signaling in cancer pathogenesis. Cell Commun Signal. (2020) 18:82. doi: 10.1186/s12964-020-00589-8
55. Mempel TR, Henrickson SE, Von Andrian UH. T-cell priming by dendritic cells in lymph nodes occurs in three distinct phases. Nature. (2004) 427:154–9. doi: 10.1038/nature02238
56. Wculek SK, Cueto FJ, Mujal AM, Melero I, Krummel MF, Sancho D. Dendritic cells in cancer immunology and immunotherapy. Nat Rev Immunol. (2020) 20:7–24. doi: 10.1038/s41577-019-0210-z
57. Cella M, Engering A, Pinet V, Pieters J, Lanzavecchia A. Inflammatory stimuli induce accumulation of MHC class II complexes on dendritic cells. Nature. (1997) 388:782–7. doi: 10.1038/42030
58. Laouar Y, Welte T, Fu XY, Flavell RA. STAT3 is required for Flt3L-dependent dendritic cell differentiation. Immunity. (2003) 19:903–12. doi: 10.1016/S1074-7613(03)00332-7
59. Park SJ, Nakagawa T, Kitamura H, Atsumi T, Kamon H, Sawa S, et al. IL-6 regulates in vivo dendritic cell differentiation through STAT3 activation. J Immunol. (2004) 173:3844–54. doi: 10.4049/jimmunol.173.6.3844
60. Kortylewski M, Kujawski M, Wang T, Wei S, Zhang S, Pilon-Thomas S, et al. Inhibiting Stat3 signaling in the hematopoietic system elicits multicomponent antitumor immunity. Nat Med. (2005) 11:1314–21. doi: 10.1038/nm1325
61. Golubovskaya V, Wu L. Different subsets of T cells, memory, effector functions, and CAR-T immunotherapy. Cancers (Basel). (2016) 8. doi: 10.3390/cancers8030036
62. Karin N. The development and homing of myeloid-derived suppressor cells: from a two-stage model to a multistep narrative. Front Immunol. (2020) 11:557586. doi: 10.3389/fimmu.2020.557586
63. Akbay EA, Koyama S, Liu Y, Dries R, Bufe LE, Silkes M, et al. Interleukin-17A promotes lung tumor progression through neutrophil attraction to tumor sites and mediating resistance to PD-1 blockade. J Thorac Oncol. (2017) 12:1268–79. doi: 10.1016/j.jtho.2017.04.017
64. Chalmin F, Mignot G, Bruchard M, Chevriaux A, Végran F, Hichami A, et al. Stat3 and Gfi-1 transcription factors control Th17 cell immunosuppressive activity via the regulation of ectonucleotidase expression. Immunity. (2012) 36:362–73. doi: 10.1016/j.immuni.2011.12.019
65. Zheng SG, Wang J, Wang P, Gray JD, Horwitz DA. IL-2 is essential for TGF-beta to convert naive CD4+CD25- cells to CD25+Foxp3+ regulatory T cells and for expansion of these cells. J Immunol. (2007) 178:2018–27. doi: 10.4049/jimmunol.178.4.2018
66. Pallandre JR, Brillard E, Créhange G, Radlovic A, Remy-Martin JP, Saas P, et al. Role of STAT3 in CD4+CD25+FOXP3+ regulatory lymphocyte generation: implications in graft-versus-host disease and antitumor immunity. J Immunol. (2007) 179:7593–604. doi: 10.4049/jimmunol.179.11.7593
67. Laumont CM, Banville AC, Gilardi M, Hollern DP, Nelson BH. Tumour-infiltrating B cells: immunological mechanisms, clinical impact and therapeutic opportunities. Nat Rev Cancer. (2022) 22:414–30. doi: 10.1038/s41568-022-00466-1
68. Teillaud JL, Houel A, Panouillot M, Riffard C, Dieu-Nosjean MC. Tertiary lymphoid structures in anticancer immunity. Nat Rev Cancer. (2024) 24:629–46. doi: 10.1038/s41568-024-00728-0
Keywords: LUAD, K-ras, stat3, DC, Th1, tumor-promoting inflammation
Citation: Clowers MJ, Rahal Z, Cho S-N, Krishna A, Yuan B, Hamana Zorrilla LG, Eckols TK, Kasembeli MM, Liu S, Peng S, Ramos-Castaneda M, Thompson AL, Rodriguez Reyna CI, Larsen KE, Grimaldo MT, Deng S, Karimi N, Chou C, Velasco WV, Zarghooni M, Alekseev S, Solis Soto LM, Ostrin EJ, Kadara H, Ekmekcioglu S, Tweardy DJ and Moghaddam SJ (2025) Selective inhibition of canonical STAT3 signaling suppresses K-ras mutant lung tumorigenesis and reinvigorates anti-tumor immunity. Front. Immunol. 16:1575181. doi: 10.3389/fimmu.2025.1575181
Received: 11 February 2025; Accepted: 07 April 2025;
Published: 28 April 2025.
Edited by:
Tatiana Akimova, University of Pennsylvania, United StatesReviewed by:
Peter R. Lowe, Merus N.V., NetherlandsRahul Shivahare, The Ohio State University, United States
Copyright © 2025 Clowers, Rahal, Cho, Krishna, Yuan, Hamana Zorrilla, Eckols, Kasembeli, Liu, Peng, Ramos-Castaneda, Thompson, Rodriguez Reyna, Larsen, Grimaldo, Deng, Karimi, Chou, Velasco, Zarghooni, Alekseev, Solis Soto, Ostrin, Kadara, Ekmekcioglu, Tweardy and Moghaddam. This is an open-access article distributed under the terms of the Creative Commons Attribution License (CC BY). The use, distribution or reproduction in other forums is permitted, provided the original author(s) and the copyright owner(s) are credited and that the original publication in this journal is cited, in accordance with accepted academic practice. No use, distribution or reproduction is permitted which does not comply with these terms.
*Correspondence: Seyed Javad Moghaddam, c21vZ2hhZGRAbWRhbmRlcnNvbi5vcmc=