- 1Center for Ecological Research, Kyoto University, Kyoto, Japan
- 2Precursory Research for Embryonic Science and Technology, Japan Science and Technology Agency, Kawaguchi, Japan
- 3Center for Advanced Intelligence Project, RIKEN, Tokyo, Japan
- 4Institute for Advanced Biosciences, Keio University, Tsuruoka, Japan
- 5Intestinal Microbiota Project, Kanagawa Institute of Industrial Science and Technology, Kawasaki, Japan
- 6Transborder Medical Research Center, University of Tsukuba, Tsukuba, Japan
Constructing biological communities is a major challenge in both basic and applied sciences. Although model synthetic communities with a few species have been constructed, designing systems consisting of tens or hundreds of species remains one of the most difficult goals in ecology and microbiology. By utilizing high-throughput sequencing data of interspecific association networks, we here propose a framework for exploring “functional core” species that have great impacts on whole community processes and functions. The framework allows us to score each species within a large community based on three criteria: namely, topological positions, functional portfolios, and functional balance within a target network. The criteria are measures of each species’ roles in maximizing functional benefits at the community or ecosystem level. When species with potentially large contributions to ecosystem-level functions are screened, the framework also helps us design “functional core microbiomes” by focusing on properties of species groups (modules) within a network. When embedded into agroecosystems or human gut, such functional core microbiomes are expected to organize whole microbiome processes and functions. An application to a plant-associated microbiome dataset actually highlighted potential functional core microbes that were known to control rhizosphere microbiomes by suppressing pathogens. Meanwhile, an example of application in mouse gut microbiomes called attention to poorly investigated bacterial species, whose potential roles within gut microbiomes deserve future experimental studies. The framework for gaining “bird’s-eye” views of functional cores within networks is applicable not only to agricultural and medical data but also to datasets produced in food processing, brewing, waste water purification, and biofuel production.
Introduction
Constructing biological communities composed of multiple species is a major challenge not only in basic ecology and microbiology but also in various fields of applied sciences (Stenuit and Agathos, 2015; Paredes et al., 2018; Toju et al., 2018; Venturelli et al., 2018). Biological systems consisting of multiple species are expected to provide functions unavailable with single species and they can be more resistant to biotic and abiotic environmental perturbations than single-species systems (Brenner et al., 2008; Kazamia et al., 2012; Sadoudi et al., 2012; Kitada et al., 2018). In medicine, optimal sets of bacteria have been explored to optimize and stabilize gut microbiomes of humans, thereby controlling host health by administering such “microbial cocktails” (Faith et al., 2010; De Roy et al., 2014; Ratner, 2015). In agriculture, engineering of microbiomes associated with roots and leaves is the key to maintain crop production under severe biotic and abiotic conditions in the field (Busby et al., 2017; Paredes et al., 2018; Toju et al., 2018). In bioenergy industry, oil-producing algal cultures, whose production can be dropped by microbial contamination, may be stabilized by addition of microbial communities resistant to species colonizing from the environment (Kazamia et al., 2012). Despite the huge potential of application, interdisciplinary attempts to integrate ecology, microbiology, and information science for designing and synthesizing biological communities are still in their infancy (Brenner et al., 2008; Stenuit and Agathos, 2015).
One way to design biological communities with favorable functions is to start from gradually adding species to simple systems (Shou et al., 2007; De Roy et al., 2014; Paredes et al., 2018). At the first step, among tens or hundreds of candidate species, species with preferable functions, such as abilities to suppress pathogenic species within host animals/plants, are selected (Jacobsen et al., 1999; Ahmad et al., 2008). After the single-species screening, pairs of species potentially forming facilitative interactions are selected (Han et al., 2006; Cassan et al., 2009). By using these pairs of functional species as building blocks, the complexity of synthetic communities may be gradually increased. Because this “bottom-up” approach is based on existing platforms of screening species with favorable functions, an increasing number of studies have yielded productive and insightful results (De Roy et al., 2014; Paredes et al., 2018). However, it has not yet been examined whether this pioneering approach ultimately allows us to optimize functions of communities composed of hundreds or thousands of species. As nonlinearity is often observed in systems consisting of multiple species (Hsieh et al., 2005; Benincà et al., 2008; Ushio et al., 2018), the bottom-up approach assuming additive effects of species or pairs of species may lead to local optima, but not global optima, of community compositions. While the bottom-up approach would be powerful and productive in designing communities with relatively small numbers of species, alternative strategies are required when we try to manage systems that inevitably contain hundreds or thousands of species (e.g., human gut microbiomes and plant-associated microbiomes).
In designing synthetic communities with hundreds or thousands of species, network science provides “bird’s-eye” views of key elements (species) that can determine community-level dynamics within complex webs of interactions/associations between species (Barberán et al., 2012; Faust et al., 2012; van der Heijden and Hartmann, 2016; Layeghifard et al., 2017). Studies based on network analyses of biological communities have inferred hub species that are located at the central positions within networks of interspecific associations (Barberán et al., 2012; Agler et al., 2016). As those hub species are linked with many other species, they potentially have profound effects on the dynamics of whole communities (Berry and Widder, 2014; Layeghifard et al., 2017; Toju et al., 2017). While the bottom-up approach mentioned above explore promising species only from pools of species with specific functions (e.g., species with direct positive effects on host animals/plants), the bird’s-eye approach highlights species based on their potential for organizing webs of interactions. By embedding species selected in the bird’s-eye approach at the early stage of community assembly, we may be able to control ecosystem processes and functions based on priority effects (Fukami, 2015; Sprockett et al., 2018) of biological communities (Toju et al., 2018). Nonetheless, metrics used in exploration of hub, core, or keystone species (e.g., degree, betweenness, closeness, and eigenvector centralities) (Newman, 2010) are too simple to evaluate potential effects of species on whole community dynamics and functions. Moreover, there have been few analytical frameworks for inferring sets of species with which we can maximize the functions and stability of biological communities.
In this study, we propose a framework for designing sets of species that can maximize demanded functions at the community or ecosystem level. By inputting data of microbe–microbe network topologies, properties of each species [or operational taxonomic units (OTUs)], and conditions of samples (e.g., health conditions of host animal/plant health), the platform allows us to explore “functional core species” and “functional core microbiomes,” which are expected to promote co-existence of species differing in their functions. With the index proposed in this study, compositions of functional core microbiomes are designed to block species with negative functions (e.g., pathogens and pests). The framework also allows us to increase the robustness of ecosystem-level functions against stochastic loss of component species based on optimization of functional redundancy and balance within microbiomes. We applied the analytical platform to datasets of plant-associated microbiomes in the rhizosphere and mouse gut microbiomes. Furthermore, a candidate taxon of the functional core microbes designated in the plant microbiome analysis was subjected to an inoculation experiments using crop plants. We also show how to apply the proposed framework to laboratory co-culture systems. Overall, this study provides a framework for maximizing ecosystem-level functions and stability in construction of microbiomes or any other types of systems consisting of multiple species.
Materials and Methods
Step 0: Assumptions
To apply the method detailed below to infer potential core species/taxa and thereby design functional core microbiomes, we assume that two types of data matrices are available for a focal microbial system. One is a data matrix of a microbe-to-microbe network, in which interactions or associations between each pair of microbial species are described (Friedman and Alm, 2012; Kurtz et al., 2015; Suzuki et al., 2017; Ushio et al., 2018; Figure 1A). There are a number of methods for inferring interactions or associations between species/taxa. Among them, co-occurrence network analyses, which evaluate patterns of co-occurrences across samples (Friedman and Alm, 2012; Kurtz et al., 2015), have been used frequently in microbiome studies (Toju et al., 2016b). Meanwhile, because co-occurrence patterns themselves can derive not only from direct interactions between species/taxa but also from sharing of environmental preferences (niches) (Toju et al., 2018), alternative approaches have been proposed (Sugihara et al., 2012; Deyle et al., 2016; Suzuki et al., 2017). For example, time-series analyses represented by empirical dynamic modeling (Sugihara et al., 2012; Deyle et al., 2016), transfer entropy (Schreiber, 2000), and sparse S-map (Suzuki et al., 2017) allow us to estimate the direction and strength of interactions between species/taxa. These methods have been used in some pioneer studies in ecology and microbiology (Suzuki et al., 2017; Ushio et al., 2018), although they remain inapplicable to systems that lack time-series data of microbiome dynamics. Both types (co-occurrence and time-series analyses) of network data are applicable to our framework detailed below, while requirement and limitation of each network analytical method should be taken into account in interpreting results.
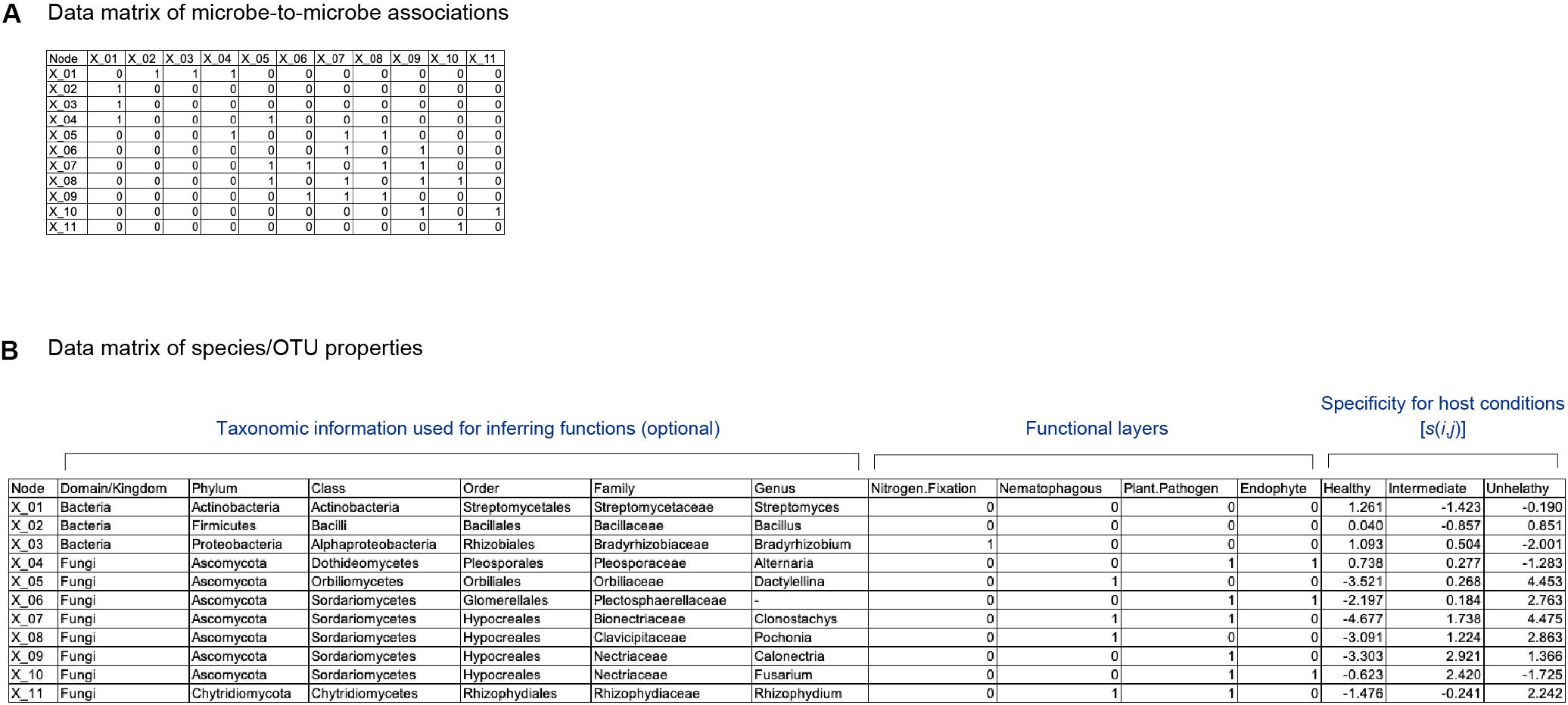
Figure 1. Schematic example of input data. (A) Matrix representing network topology of associations between species/OTUs/ASVs. (B) Data file representing properties of each species/OTU/ASV. The presence (1) and absence (0) of each function is designated for each species/OTU/ASV. Specificity of a species (i) to a sample condition (j) [s(i,j)] is also shown for each species/OTU/ASV.
The other input matrix used in the following analysis indicates presence/absence of each physiological/ecological function for each species/taxon (Figure 1B). Multiple types of functions can be set in the input data matrix (hereafter, functional layers). At each functional layer, presence/absence of a specific function (e.g., nitrogen-fixing ability or suppression of pathogens) is described for each species/taxon. If genome data are available for each species/taxa, each functional layer may represent presence/absence of each gene or KEGG orthology (Kanehisa et al., 2011; Kanehisa et al., 2015).
Step 1: Estimating Functional Coreness
We develop a framework for scoring each species in terms of its potential roles in optimizing microbiome-scale (community-scale) functions. The criteria included in the framework is (1) topological positions of a focal species within a network, (2) community-scale functional portfolios, and (3) community-scale balance of functions. Hereafter, we assume a network dataset consisting of species for simplicity: however, the methods detailed below is applicable to datasets composed of genera, strains, or operational taxonomic units (OTUs).
To evaluate the topological position of a focal species i [T(i)], we use the betweenness centrality metric (Freeman, 1977) defined as follows:
where σk,l is the number of shortest paths between species k and l, and σk,l(i) is the number of shortest paths between species k and l that pass through the focal species i (Figure 2A). In calculating the betweenness scores, network topology representing only positive interactions/associations between species should be considered: i.e., betweenness scores derived from networks including negative interactions/associations are misleading, although inference of negative interactions between pairs of species (Toju et al., 2016b), per se, may be of some help in selecting best sets of functional core microbes in the later process. Along with the classic betweenness metric (Freeman, 1977), the weighted betweenness metric that uses network edge weights (Brandes, 2001) is applicable as well.
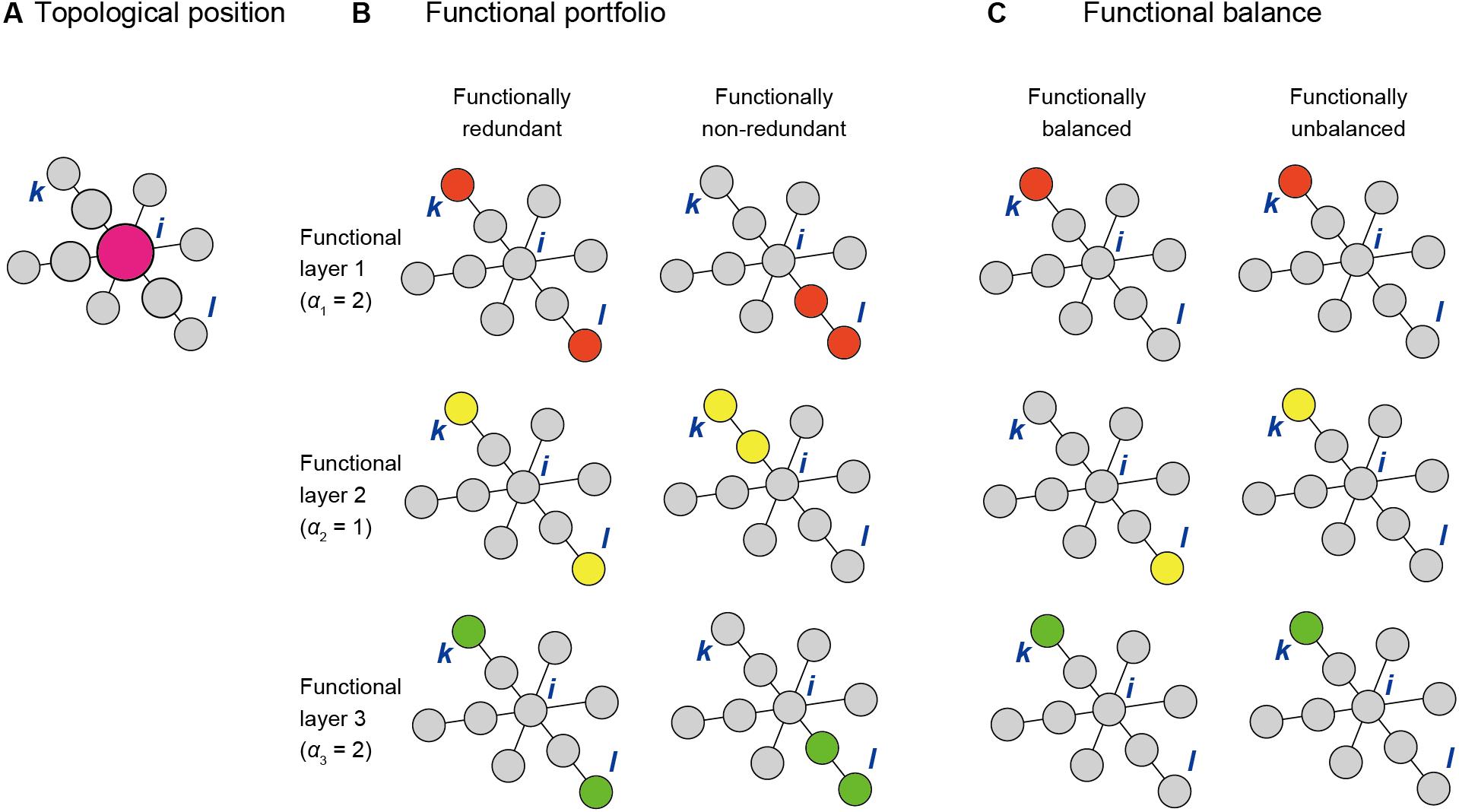
Figure 2. Three criteria for scoring species within a network. (A) Topological positions within a network. A species (or OTU/ASV) i is highly scored if it is located within the shortest path connecting pairs of other species within a network (e.g., species k and l). (B) Functional redundancy. The score of species i depends on the functional properties of species k and l as determined the weighting parameter (αn) and the functional redundancy parameter (βn) of each functional layer. (C) Functional balance. The score of species i depends on functional properties of species k and l as determined by the balancing parameter γ and the weighting parameter αn [optional: regulated as δ + (1−δ)αn].
We next take into account microbial functions by multiplying the betweenness score of species i by the term representing functional profiles of species k and l. For each species i, an index evaluating topological positions and functional portfolios [TP(i)] is given as follows:
where αn is weight for functional layer n, fn,k is presence (1) or absence (0) of a function in layer n of species k, fn,l is presence (1) or absence (0) of a function (or gene) at layer n of species l, and N is the number of functional layers (Figure 2B). The function z (fn,k + fn,l) is defined as z (0) = 0, z (1) = 1, and z (2) = βn, where βn is the parameter representing functional redundancy (Peterson et al., 1998; Rosenfeld, 2002; Ley et al., 2006) at layer n. When species i is located within the shortest paths connecting species k and l and either or both species k and l has (have) function n (i.e., fn,k + fn,l = 1 or 2), the focal species i has a high score in the above index TP(i). The parameter βn scales the degree to which functional redundancy is introduced for function n: when two species interconnected by species i within shortest paths have function n (i.e., fn,k + fn,l = 2), species i is weighted in proportion to βn. For analyses based on amplicon sequencing (DNA metabarcoding), FAPROTAX (Louca et al., 2016) or FUNGuild (Nguyen et al., 2016) programs may be used to infer potential functions of each species or OTU. Alternatively, reference databases of whole genomes may be used for designating the presence/absence of respective functional genes for each species or OTU.
Another important criterion in exploring core species is community-scale balance of functions. For each species i, an index evaluating topological positions, functional portfolios, and functional balance [TPB(i)] is given as follows:
where Dn = δ + (1−δ)αn, γ(0≤γ≤1) is a parameter for balancing, and δ(0≤δ≤1) is a parameter for determining the usage of the number of functional layers or the weight when calculating the balancing factor (Figure 2C). When only a small proportion of species among those interconnected by species i within the shortest paths disproportionately have most functions, the focal species i is penalized. In other words, by adding the third term (), species that interconnect sets of other species differing in their functional compositions are highly evaluated.
Finally, we extend the index TPB(i) by taking into account situations in which input data include microbial species with deleterious functions (e.g., pathogens; αn < 0). The “functional coreness” of species i [Cfunc(i)] is then calculated as:
where N+ is a set of functional layers with positive αn values (αn > 0), N− is a set of functional layers with negative αn values (αn < 0).
Step 2: Designing Core Microbiomes
Once functional coreness of each species within a network was calculated, core microbiomes can be designed as sets of two or more species with highest scores. In this process, the stability of designed core microbiomes will be maximized by selecting pairs or groups of candidate core species within the same network modules. Because groups of species in the same network module are those that can co-exist in the environment or within the same host organisms, choosing components of core microbiomes within a network module will be the key to design robust microbiomes. Various types of methods are available for inferring network modules (Guimera and Amaral, 2005; Lancichinetti et al., 2011).
When compositions of core microbiomes are arranged for each network module, we then need to choose one of the network modules whose candidate core microbiomes will be used in experimental studies on synthetic microbiomes. An effective way is to choose network modules that show highest mean functional coreness or largest sums of functional coreness. Alternatively, if additional information is available regarding “soundness” of samples that were used in the estimation of microbe-to-microbe networks (e.g., health conditions of host individuals), we can evaluate network modules based on the observed sample conditions. To evaluate modules within a microbe-to-microbe network based on conditions of samples (e.g., host animal or plant health conditions), we may need to infer how species constituting each network module show specificity to favorable/unfavorable sample conditions.
As discussed in a previous study (Toju et al., 2019), specificity of a species i to sample condition j [s(i,j); e.g., healthy or unhealthy condition of host individuals in a human gut microbiome study] can be calculated based on a randomization analysis as follows:
where Noriginal(i,j) is the mean number of the sequencing reads of species i observed in condition j samples in an original data matrix and Nrandomized(i,j) is the mean number of the sequencing reads of species i across condition j samples in randomized matrices (Figure 3). Mean and SD denote mean and standard deviation, respectively. There are various types of methods for making randomized network matrices (Vázquez et al., 2007; Dormann et al., 2009; Toju et al., 2014): shuffling of labels denoting sample conditions is the simplest way to make randomized matrices, although it should be considered carefully whether the assumptions of each randomization method are applicable to each dataset. The number of randomized matrices (usually, 10,000 or more) should be carefully determined as well. Instead of the above metrics based on the mean number of sequencing reads, the number of condition j samples from which species i was observed (Toju et al., 2016a), for example, can be used in estimation of species × sample condition specificity.
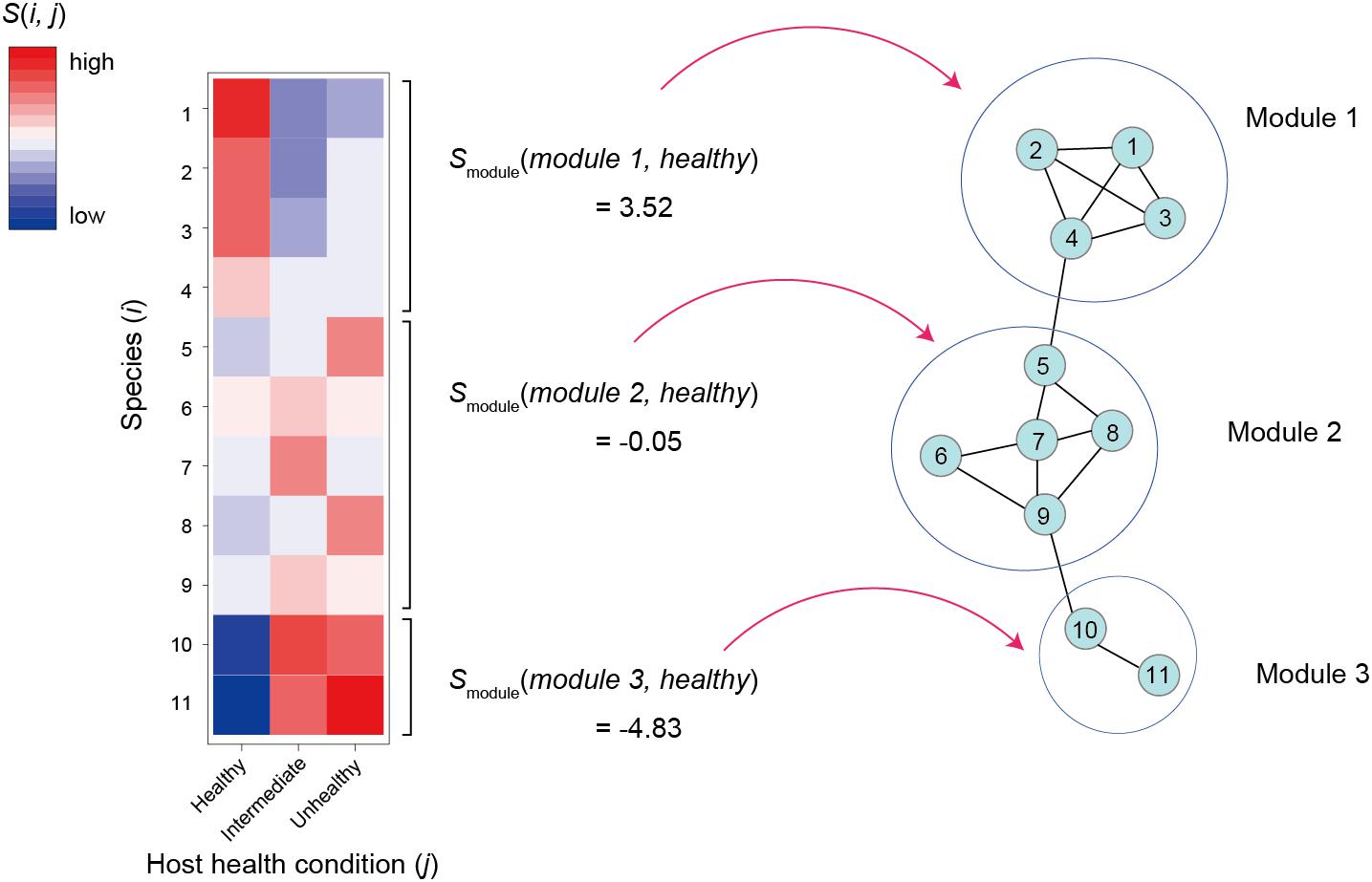
Figure 3. Relationship between network modules and host sample conditions. For the first step, specificity to a host sample conditions [s(i,j)] is calculated or each species/OTU/ASV as defined in the equation 5. The s(i,j)values are then averaged across members of each network module (h) by focusing on a host sample condition (j). The obtained smodule(h,j) scores of a favorable sample condition are used as criteria for ranking network modules.
Once specificity scores for respective combinations of species and sample conditions were obtained, we can then evaluate how species in a module, on average, represent sample conditions as follows:
where smodule(h,j) is mean specificity of species in network module h to sample condition j (sample condition score of a module) and |Mh| is size of a set of species belonging to module h (Figure 3). This kind of metric allows us to rank microbe-to-microbe network modules depending on their associations with favorable/unfavorable conditions of samples. Network modules with highest smodule(h,j) value for favorable sample conditions are potential targets of core microbiome design because they are expected to include species promoting or associated with host animal/plant health (or benign conditions of water/soil samples).
Regarding the number of optimal core species in designing core microbiomes, there has been few criteria (Toju et al., 2018). One way of determining the number of core species used for core microbiome design is to add candidate core species in an order from the highest Cfunc(i) values until it decreases disruptively. It also remains to be examined whether core microbiomes should be constituted exclusively by member of network modules with highest smodule(h,j) or they should include members of other modules with high smodule(h,j) in certain proportions.
Application 1: Soybean Rhizosphere Microbiome
The algorithm for exploring core species was applied to the data of a plant-associated microbiome (Toju and Tanaka, 2019). The data represent rhizosphere microbiome structures of the 128 individual soybean plants (Glycine max) collected in the field of Kyoto University, Kyoto, Japan (35.033N, 135.785E). In the field, soybean plants heavily attacked by root-knot nematodes (Meloidogyne sp.), benign (healthy) plants, and plants showing intermediate phenotypes were recorded and the information of host sample conditions was used in interpreting results on microbiome analyses (Toju and Tanaka, 2019).
The OTU data consisting of bacterial 16S and fungal internal transcribed spacer (ITS) sequences were analyzed with the SpiecEasi method (Kurtz et al., 2015) in order to infer co-occurrence network topology (Toju and Tanaka, 2019). After removing network edges with negative values, the network data was converted into a binary format. Because betweenness centrality could not be calculated for small disconnected sub-networks consisting of a few species, only the largest sub-network involving 300 out of 318 bacterial and fungal OTUs were analyzed in the following process. The number of optimal network modules within the largest sub-network was estimated based on a modularity score (Clauset et al., 2004) using the igraph package (Csardi and Nepusz, 2006) of R 3.5.2.
In the input file indicating functions of each OTU (i.e., network node) within the network, six functional layers were incorporated. For example, nitrogen fixing ability, which was inferred using the program FAPROTAX 1.1 (Louca et al., 2016), and potential nematode-suppressing ability discussed in the original study (Toju and Tanaka, 2019) were included as functional layers with positive αn values. Likewise, based on the functional profiling with the FUNGuild program (Nguyen et al., 2016), endophytic fungi and plant-pathogenic fungi were included with positive and negative αn values, respectively. Details of the functional layers and parameter setting are shown in the caption of Figure 4. The input data and R scripts are provided as Supplementary Data S1.
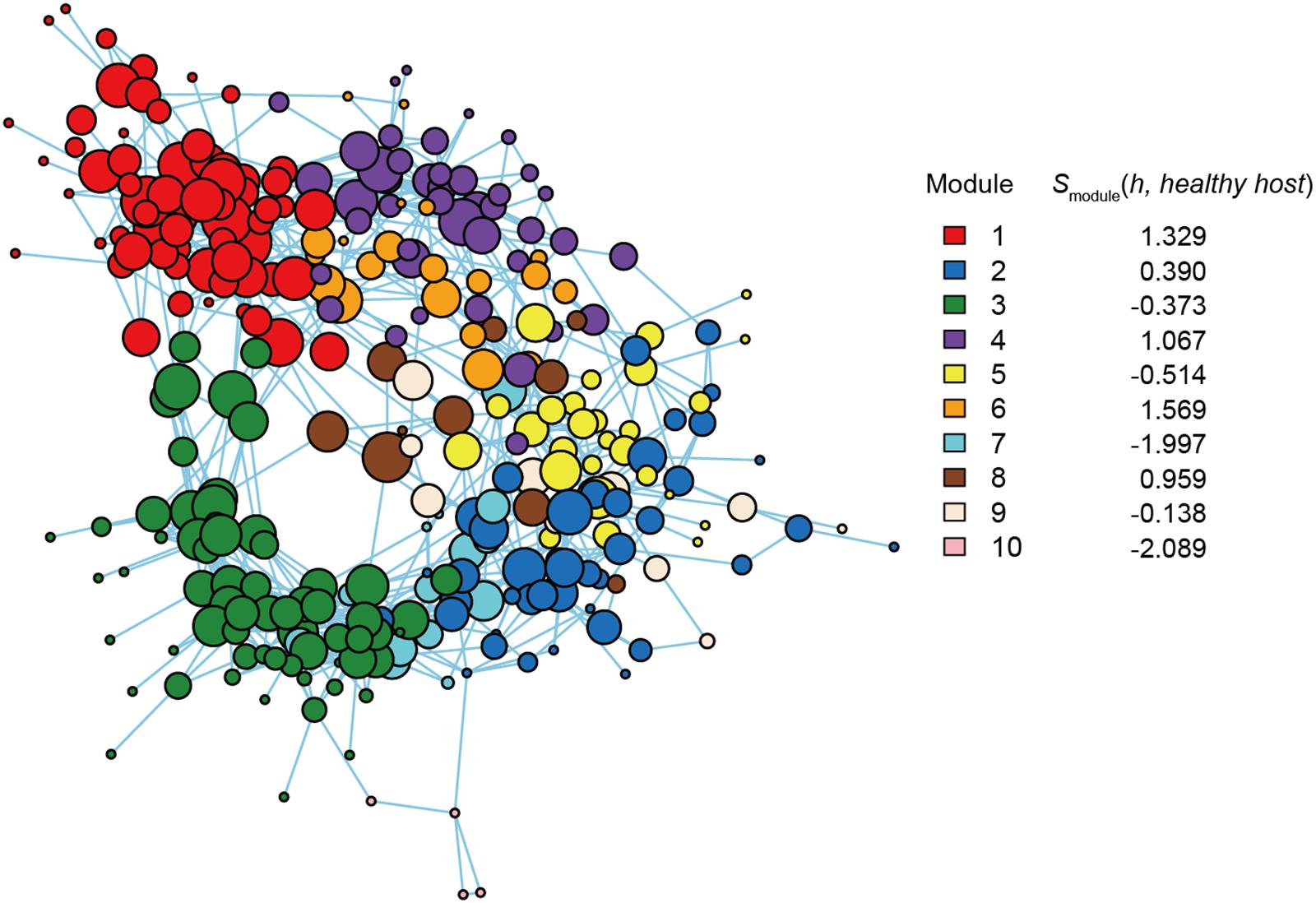
Figure 4. Functional coreness analysis for the dataset of the soybean rhizosphere microbiome. Networks depicting co-occurrence patterns of prokaryote and fungal OTUs are shown. Size of nodes is roughly proportional to functional coreness scores. Specificity to healthy soybeans is calculated for each network module. wo functional layers were included in the analysis (layer 1, nitrogen fixation; layer 2, nematode-attacking function; layer 3, plant pathogenicity; layer 4, fungal parasitism; layer 5, endophytic guild; layer 6, saprotrophy). The parameters used are as follows: weight for each functional layer, α1 = 1, α2 = 1, α3 = –1, α4 = –1, α5 = 1, and α6 = 1; functional redundancy of each functional layer, β1 = 2, β2 = 2, β3 = 1, β4 = 1, β5 = 2, and β6 = 1; a parameter for balancing, γ = 1; an additional parameter for balancing, δ = 0.
Application 2: Mouse Gut Microbiome
We next applied the algorithm to the data of a gut microbiome study using mice as hosts (Ishii et al., 2018). In the previous study, mice were fed with the diet whose nutritional compositions was equivalent to daily human nutritional content in the United States (as described in a report distributed by National Research Council of United States) (Hashimoto et al., 2009) and with normal (control) diet CA-1 chow (CLEA Japan, Inc., Meguro, Tokyo, Japan). Five and six mice were fed with American diet and normal diet, respectively, and fecal samples of each mouse individual was collected at 8, 12, 24, 36, and 52 weeks of age (Ishii et al., 2018). The fecal samples were then subjected to 16S rRNA sequencing analyses and prokaryote biome data of the 55 samples (11 mice × 5 time points) were obtained.
The published sequencing data were processed in the pipeline of the program DADA2 (Callahan et al., 2016) and the taxonomic assignment of the output amplicon sequence variants (ASVs) was performed with the SILVA reference database v.132 (Quast et al., 2012). Within the list of ASVs, the taxa whose positive effects on host mice/humans were shown in previous studies (e.g., Lactobacillus) (Gorbach, 2000; Bravo et al., 2011; Ritze et al., 2014) and those possibly having negative effects on hosts (e.g., Erysipelatoclostridium) (Zakham et al., 2019) were designated at separate functional layers. Co-occurrence patterns of the ASVs across the 55 samples were analyzed with the SpiecEasi program: ASVs appearing in 15 or more samples were subjected to the analysis. The network depicting co-occurrence patterns was subdivided into two main clusters (sub-networks) including 38 and 33 ASVs, respectively, and many small clusters consisting of 1-3 ASVs. For each of the two main sub-networks, the functional coreness analyses detailed above were performed. Details of the functional layers and parameter setting are shown in the caption of Figure 5. The input data and R scripts are provided as Supplementary Data S2.
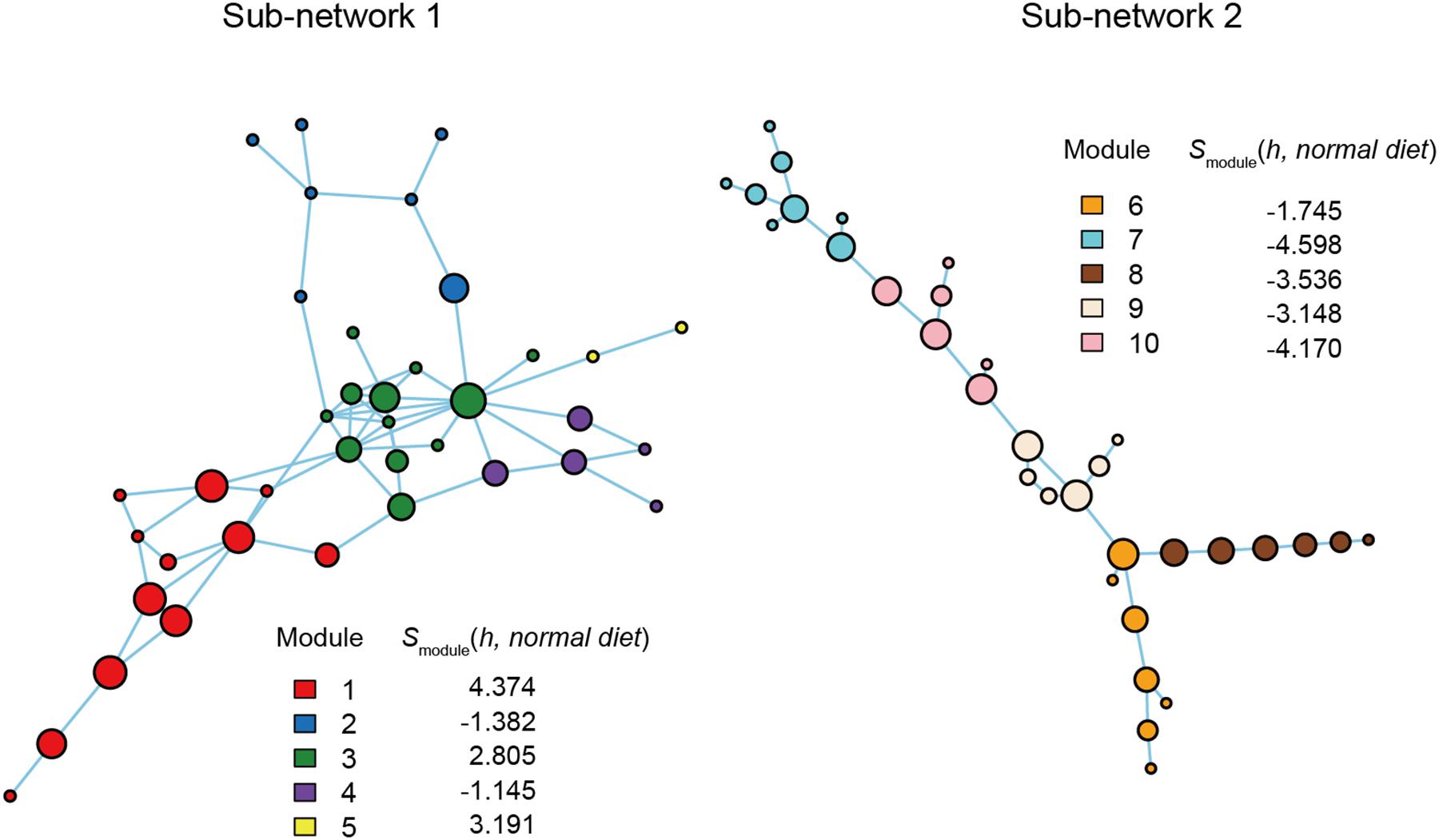
Figure 5. Functional coreness analysis for the dataset of the mouse gut microbiome. Networks depicting co-occurrence patterns of prokaryote ASVs are shown. Size of nodes is roughly proportional to functional coreness scores. Specificity to normal diet over American diet is calculated for each network module. Small network clusters (sub-networks) consisting of 1–3 ASVs are not shown. Two functional layers were included in the analysis (layer 1, potential positive effects on host health; layer 2, potential negative effects on host health). The parameters used are as follows: weight for each functional layer, α1 = 1 and α2 = –1; functional redundancy of each functional layer, β1 = 1 and β2 = 1; a parameter for balancing, γ = 1; an additional parameter for balancing, δ = 0.
Application 3: Laboratory Co-cultures
We also targeted artificial microbiomes maintained under a laboratory environmental condition for future use of the algorithm in industrial applications. We set up laboratory co-culture systems by introducing soil-derived microbes to liquid media. The source soil was sampled in the forest of Center for Ecological Research, Kyoto University (34.971N, 135.960E) and then it was sieved with 2-mm mesh. The 5 g of sieved soil was mixed in 30 mL of autoclaved distilled water and then the water was diluted by 10-folds by autoclaved distilled water. The water containing microbiomes (200 μL) was then introduced into 2-mL deep well plate, in which 800 μL of liquid medium was installed beforehand in each well. Three types of media were used: Media A [0.5% (w/v) milled oatmeal (Nisshoku Oats; Nippon Food Manufacturer)], Media B [0.5% (w/v) milled oatmeal + 0.5% (w/v) peptone (Bacto Peptone, BD)], and Media C (0.5% (w/v) peptone). For each medium type, 16 replicate samples were set up. After pre-culture for 5 days, 200 μL of co-culture liquid was collected from each sample every 24 h and then 200 μL fresh medium was added to the continual co-culture. The sampling of co-culture media was continued for 31 days, yielding, in total, 1,488 samples (3 medium types × 16 replicates × 31 days). Of the sampled 200 μL co-culture, 25 μL was mixed with 50 μL of buffer containing Sodium Dodecyl Sulfate (0.0025%), ethylenediaminetetraacetic acid (0.125 mM), Tris (1 mM), NaCl (4 nM), and Proteinase K (×1/100). The sample-buffer mixtures were processed with the temperature profile of 37°C for 60 min followed by 95°C for 10 min and then they were vortexed for 10 min to extract DNA.
To reveal the microbiome structure of each sample, Prokaryote 16S ribosomal RNA region was PCR-amplified with the forward primer 515f (Caporaso et al., 2011) fused with 3–6-mer Ns for improved Illumina sequencing quality (Lundberg et al., 2013) and the forward Illumina sequencing primer (5′- TCG TCG GCA GCG TCA GAT GTG TAT AAG AGA CAG- [3–6-mer Ns] – [515f] -3′) and the reverse primer 806rB (Apprill et al., 2015) used with 3–6-mer Ns and the reverse sequencing primer (5′- GTC TCG TGG GCT CGG AGA TGT GTA TAA GAG ACA G [3–6-mer Ns] − [806rB]-3′) (0.2 μM each). The DNA-polymerase–buffer system of KOD One (Toyobo) was used with the temperature profile of 35 cycles at 98°C (denaturation) for 10 s, 55°C (annealing of primers) for 5 s, and 68°C (extension) for 30 s. To prevent generation of chimeric sequences, the ramp rate through the thermal cycles was set to 1°C/s (Stevens et al., 2013). Illumina sequencing adaptors were then added to respective samples in the supplemental PCR using the forward fusion primers consisting of the P5 Illumina adaptor, 8-mer indexes for sample identification (Hamady et al., 2008) and a partial sequence of the sequencing primer (5′- AAT GAT ACG GCG ACC ACC GAG ATC TAC AC − [8-mer index] − TCG TCG GCA GCG TC -3′) and the reverse fusion primers consisting of the P7 adaptor, 8-mer indexes, and a partial sequence of the sequencing primer (5′- CAA GCA GAA GAC GGC ATA CGA GAT − [8-mer index] − GTC TCG TGG GCT CGG -3′). KOD One was used with a temperature profile of 8 cycles at 98°C for 10 s, 55°C for 5 s, and 68°C for 30 s (ramp rate = 1°C/s). The PCR amplicons of the samples were then pooled after a purification/equalization process with the AMPureXP Kit (Beckman Coulter). Primer dimers, which were shorter than 200 bp, were removed from the pooled library by supplemental purification with AMpureXP: the ratio of AMPureXP reagent to the pooled library was set to 0.6 (v/v) in this process. The sequencing library was processed with an Illumina MiSeq sequencer (run center: KYOTO-HE; 2 × 250 bp; 15% PhiX spike-in).
The raw sequencing data were converted into FASTQ files using the program bcl2fastq 1.8.4 distributed by Illumina (DDBJ DRA accession: DRA010262). The output FASTQ files were demultiplexed with the program Claident v0.2. 2018.05.29 (Tanabe, 2018). The 16S rRNA dataset was subsequently processed with the program DADA2. The sample × ASV matrix obtained was subjected to a co-occurrence network analysis with the Spiec-Easi program: ASVs that appeared in less than 50 samples were omitted in the analysis. The network depicting co-occurrence patterns was subdivided into two main clusters (sub-networks) including 70 and 20 ASVs and many small clusters consisting of single ASVs. For each of the two main sub-networks, functional coreness analyses were performed. Properties of each ASV were inferred with FAPROTAX and chemoheterotrophy (αn = 1) and pathogenicity for humans (αn = −1) were included as functional layers. Details of the functional layers and parameter setting are shown in the caption of Figure 6. The input data and R scripts are provided as Supplementary Data S3.
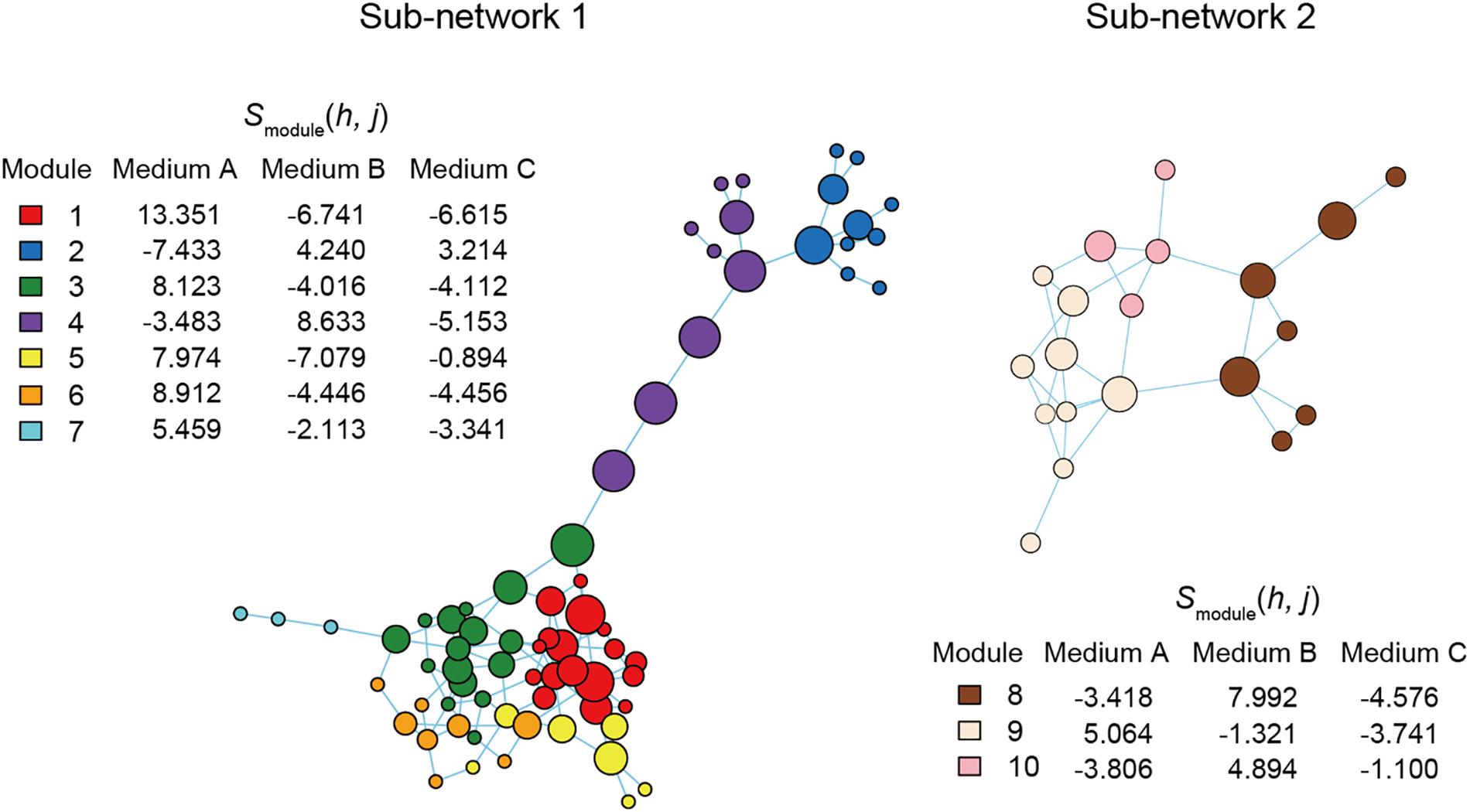
Figure 6. Functional coreness analysis for the dataset of the co-culture experiment. Networks depicting co-occurrence patterns of prokaryote ASVs are shown. Size of nodes is roughly proportional to functional coreness scores. Specificity to Medium A is calculated for each network module. The two largest network clusters (sub-networks) are shown: other clusters consisted of single ASVs. Two functional layers were included in the analysis (layer 1, chemohetrotrophy; layer 2, human pathogenicity). The parameters used are as follows: weight for each functional layer, α1 = 1 and α2 = –1; functional redundancy of each functional layer, β1 = 2 and β2 = 1; a parameter for balancing, γ = 1; an additional parameter for balancing, δ = 0.
Inoculation Experiment of a Core Microbe
We performed a preliminary experiment to examine effects of an inferred core species on host plant growth based on the results on the soybean rhizosphere microbiome (application 1). Among OTUs with highest functional coreness [Cfunc(i)] within modules with high sample condition scores [smodule(h,j)], we chose one whose close relatives were available for inoculation experiments. A Trichoderma fungal strain isolated from the site close to the Kyoto University field (Trichoderma sp. strain KUCER00000218) was selected and used in the inoculation experiment (Supplementary Figure S1). The fungal isolate was cultured in 1.5% (w/v) malt extract (BD). Grown hyphae were washed with autoclaved distilled water and were subsequently fragmented using a mill (OML-1, Osaka Chemical Co., Ltd.). To make inoculum of the Trichoderma fungus, the fragmented hyphae were then introduced into a fungus culture bag (Shinkoen) filled with the mixture of autoclaved leaf mold, wood chips (sawdust), and rice bran (3:1:1). We also made a fungus culture bag with no fungal inoculum as a control. After the Trichoderma hyphae spread within the fungus culture bag, the inoculum was mixed with autoclaved potting soil (“Silver Soil,” Kanea) consisting mainly of fermented bark, peat moss, and coconut peat by the proportion of 1:9. For the rapid evaluation of plant growth responses, seeds of a Brassicaceae plant species, Brassica rapa var. perviridis (cultivar “Komatsuna Wase,” Atariya Co., Ltd.), were sawn to the mixed soil. Three seeds were sawn in each pot (40 × 40 × 45 mm). In total, 20 pots were made for both inoculation and control experiments (Experiment A). To examine the reproducibility of the results on plant growth responses, an additional experiment using the same brassica species (B. rapa var. perviridis) and a tomato (Solanum lycopersicum) cultivar “Marmande” (Daiousyokai Co., Ltd.) were performed (two seeds per pot; 20 pots for each plant species for each inoculum/control experiment) (Experiment B). The pots were kept under a 12L/12D light condition at 25°C. After 27 days and 21 days, the Experiment A and B plant samples, respectively, were harvested to measure their dry shoot mass.
Results
Application 1: Soybean Rhizosphere Microbiome
Within the microbe–microbe co-occurrence network, Modules 1, 4, 6, and 8 represented healthy host plants (Figure 4). Among the modules, Module 1 included bacterial and fungal OTUs with highest functional coreness scores such as fungi allied to Penicillium janthinellum, Trichoderma asperellum, or Chaetomium sp., and a bacterium in the phylum Chloroflexi (Table 1). Of the microbial OTUs, the Penicillium OTU had the highest functional coreness score but its host specificity for healthy plants was relatively weak (Table 1). Meanwhile, the Trichoderma OTU, whose ITS sequence completely matched reference database sequences of T. asperellum, T. harzianum, and T. viride, showed the third highest functional coreness and high host specificity for healthy plants (Table 1). The Penicillium and Trichoderma OTUs showed higher scores of functional coreness than OTUs in other modules within the soybean microbiome (Table 1).
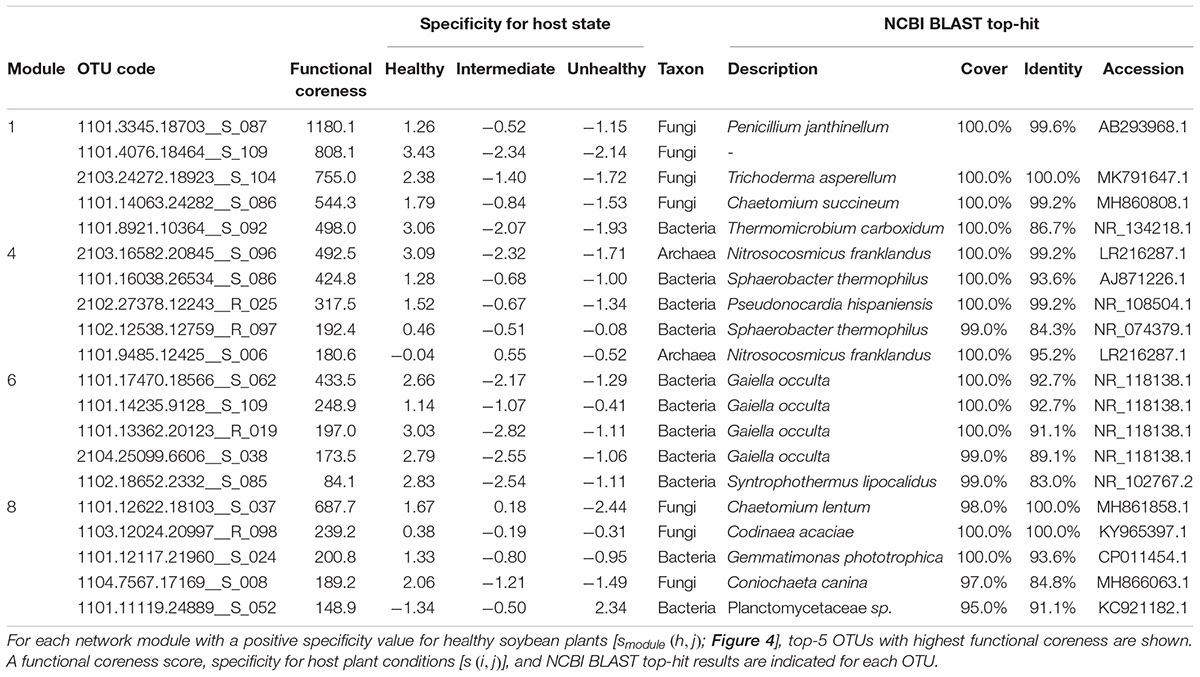
Table 1. Prokaryote and fungal OTUs with highest functional coreness in the dataset of the soybean rhizosphere microbiome.
Within Module 4, archaeal OTUs in the genus Nitrosocosmicus and actinobacterial OTUs phylogenetically allied to Pseudonocardia and Sphaerobacter showed highest scores of functional coreness (Table 1). In Module 6, OTUs allied to the actinobacterial genus Gaiella and an OTU distantly allied to the Firmicutes genus Syntrophothermus displayed high functional coreness (Table 1). In Module 8, the list of microbes with high functional coreness involved fungal OTUs allied to Chaetomium, Codinaea, and Coniochaeta, and bacterial OTUs allied to Gemmatimonas and Planctomycetaceae sp. (Table 1).
Application 2: Mouse Gut Microbiome
Within the network depicting the co-occurrence patterns of bacteria in mouse gut, Modules 1, 3, and 5 represented high average specificity for normal diet over American diet conditions (Figure 5). As Module 5 included only two ASVs, it was omitted in the downstream analysis. In Module 1, ASVs phylogenetically allied to Roseburia, Faecalitalea, Clostridium, Bacteroides, and Breznakia showed high scores of functional coreness. Although four of them showed low 16S rRNA sequence similarity to bacteria in the NCBI database, an ASV allied closely allied to Bacteroides caecimuris (Table 2). In Module 3, an ASV allied to Muribaculum intestinale and one allied to Lactobacillus murinus displayed high functional coreness scores and high 16S rRNA sequence similarity to bacteria in public database sequences (Table 2). Other ASVs in the module showed low functional coreness and their taxonomic identity was uncertain (Table 2).
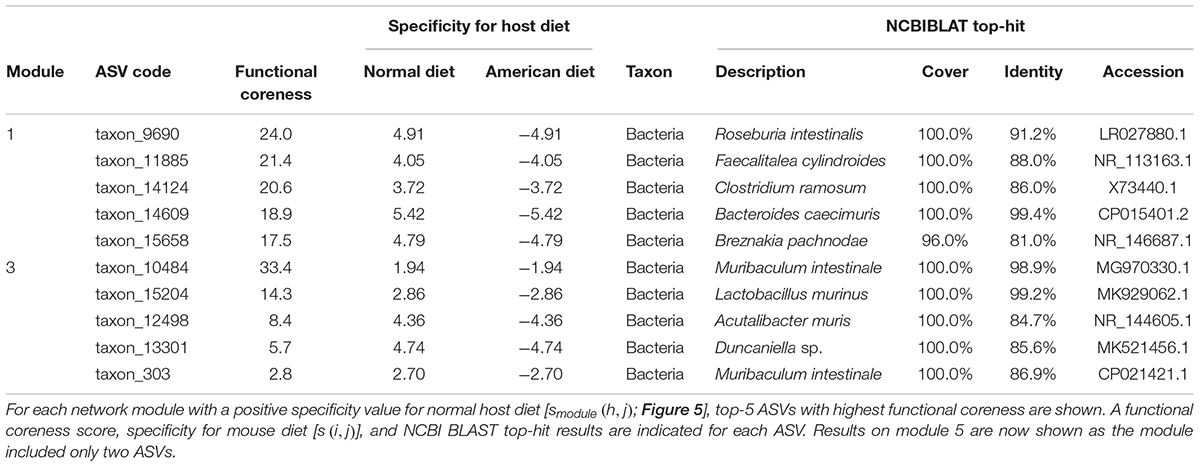
Table 2. Prokaryote ASVs with highest functional coreness in the dataset of the mouse gut microbiome.
Application 3: Laboratory Co-cultures
For simplicity, we here report results on the two network modules with highest specificity values for Medium A (oatmeal) (Figure 6). Within Module 1, which had the highest specificity to Medium A, bacteria in the genera Mucilaginibacter, Terriglobus, and Paraburkholderia showed highest functional coreness (Table 3). Likewise, in Module 3, bacteria in the genera Mucilaginibacter, Sphingomonas, Bdellovibrio, Clostridium, and Pelosinus were highly ranked in terms of functional coreness (Table 3).
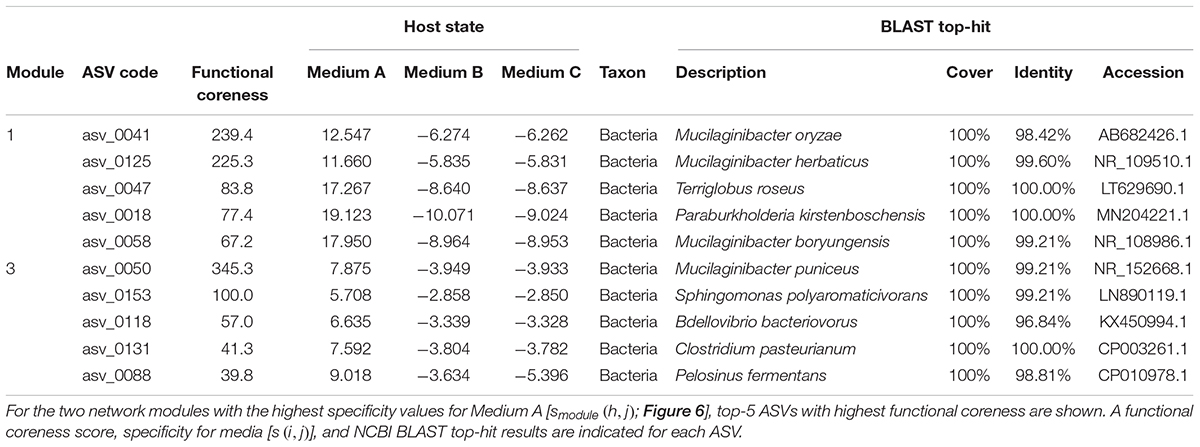
Table 3. Prokaryote ASVs with highest functional coreness in the dataset of the co-culture experiment.
Inoculation Experiment of a Core Microbe
In Experiment A, shoot dry mass of the host Brassica plants was 75.7 (SD = 34.3) mg for control (uninoculated) samples and 258.8 (SD = 139.9) mg for samples inoculated with the selected Trichoderma strain (ANOVA: F1,78 = 64.7, P < 0.0001; Figure 7A). This positive effect of the Trichoderma strain on Brassica host growth was confirmed in an additional inoculation experiment (Experiment B) (ANOVA: F1,71 = 74.3, P < 0.0001): mean shoot dry mass was 14.9 (SD = 9.0) mg for control samples and 212.3 (SD = 137.1) mg for inoculated samples (Figure 7B):. Similar growth promotion effects were observed also in an experiment in which the Trichoderma strain was inoculated to tomato plants (ANOVA: F1,61 = 30.5, P < 0.0001): mean shoot dry mass was 34.4 (SD = 17.9) mg for control samples and 95.4 (SD = 58.9) mg for inoculated samples (Figure 7B).
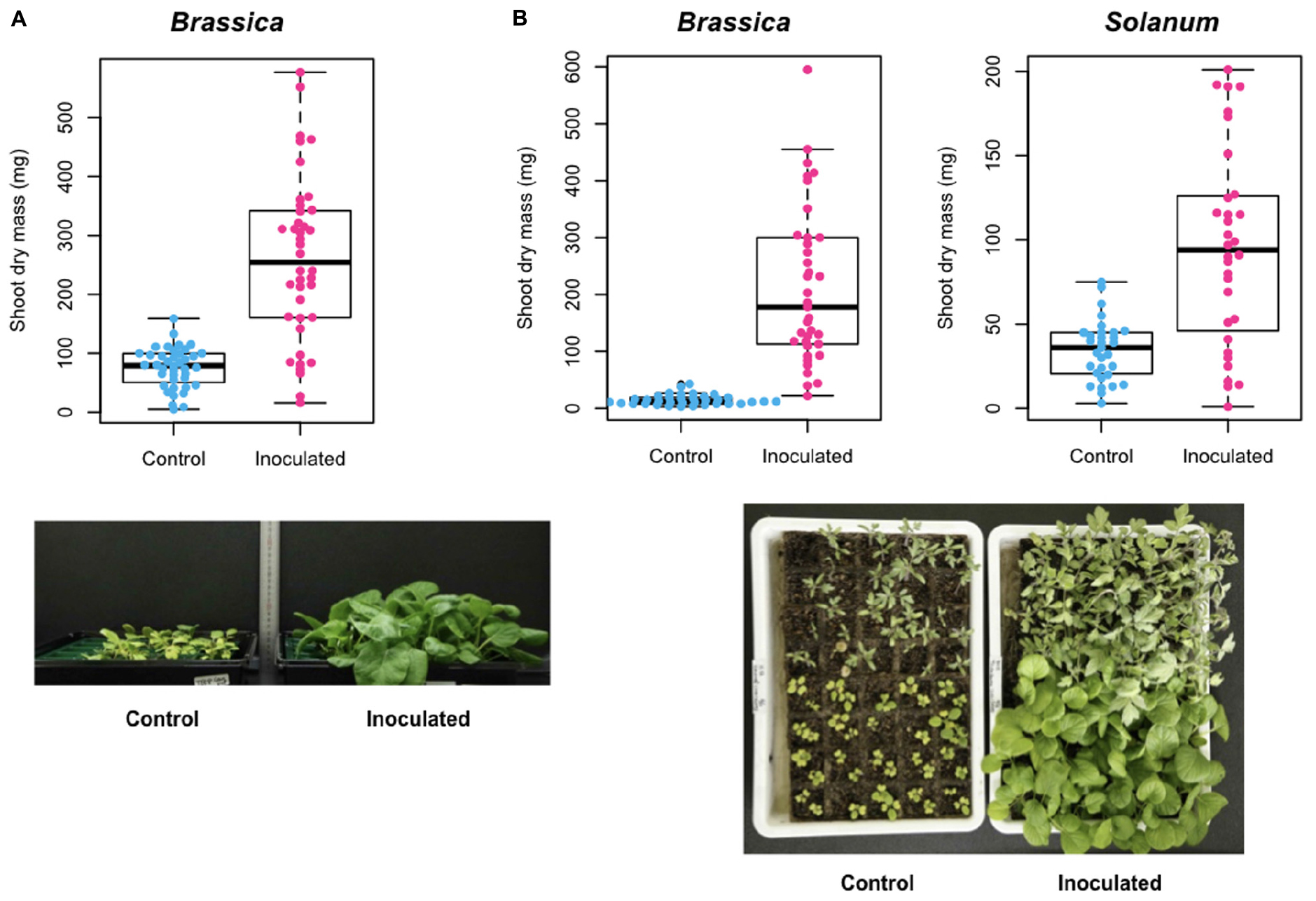
Figure 7. Inoculation experiment of a functional core. (A) Experiment A. Shoot dry mass of Brassica rapa var. perviridis is shown for individual plants uninoculated/inoculated with Trichoderma sp. strain KUCER00000218. (B) Experiment B. Shoot dry mass of B. rapa var. perviridis (left) and Solanum lycopersicum (right) is shown for individual plants uninoculated/inoculated with Trichoderma sp. strain KUCER00000218. In the photograph, B. rapa var. perviridis and S. lycopersicum are found in the lower and upper parts, respectively.
Discussion
A fundamental limitation in synthesizing biological communities is “the curse of dimensionality.” If libraries of plant-growth promoting microbes are available, best species/stain pairs that maximize plant growth might be found through round-robin experiments (e.g., inoculation of microbial pairs on animal/plant hosts). However, the success of this bottom-up approach depends on the size of libraries. Even in a relatively simple situation in which 50 candidate species/stains are available, the 1,225 combinations (50C2) should be examined to find best pairs. The number of combinations to be tested inflates with that of candidate species/strains and the size of synthesized communities. Even in a moderate case in which a five-species community is designed based on libraries including 500 species, the number of possible combinations to test exceeds 2.6 × 1011 (500C5). Thus, synthetic community design requires pre-screening of compatibility between species based on genomic information (Qin et al., 2010; Arumugam et al., 2011; Bai et al., 2015).
By utilizing accumulated information of microbial functions and reference genomes, we herein proposed a “bird’s-eye view” approach of systematically exploring best combinations of species/strains that potentially maximize functionality at the community/ecosystem level. Although similar framework for designing microbiomes has been discussed in a previous study, its application was limited to selection of best pairs of microbial species (Toju et al., 2018). Moreover, the previous method did not allow to optimize community-scale characteristics of designed core microbiomes. The workflow proposed in this study starts from scoring potential contributions of each species/strain to the community-level functions. The method allows us to design synthetic communities with arbitrary number of core species/strains based on three criteria. First, species that potentially intermediate interactions between other species are highlighted based on their topological positions within a network (Figure 2A). Betweenness centrality metric (Freeman, 1977; Brandes, 2001) was used to evaluate each species’ position in light of shortest paths connecting pairs of other species within a network. Second, portfolios of functions at the community level is designed by setting weights of respective functions and the degree of each function’s redundancy (Figure 2B). By giving negative weighting parameters to unfavorable functions (αn < 0), potential assembly of pathogens/pests is penalized. In addition, we may control functional stability of synthetic communities against accidental loss of species by increasing functional redundancy of whole microbiomes. That is, even if a species with key functions is lost (goes extinct), ecosystem-level functions may be maintained if there are other species with the same functions within the community (Peterson et al., 1998; Rosenfeld, 2002; Ley et al., 2006). Third, we can determine balance of functions throughout an association/interaction network (Figure 2C). Communities in which major functions are provided by a few species may be less resistant to perturbations than those in which important functions are distributed across the network. Interactions between functional portfolios and functional balance are set by a balancing parameter (γ). After calculating scores that represent species’ contributions to ecosystem-level functions (functional coreness), the framework allows us to design synthetic communities with arbitrary number of core species/strains (functional core microbiomes). Alternative candidates of functional core microbiomes are evaluated by focusing on properties of network modules (Figures 3−6).
One important benefit of our approach is that it estimates whole-system-scale contributions of each species. Species mutualistic to animal/plant hosts in a single-species context does not necessarily maximize whole-system functions in multi-species contexts. In other words, species with neutral, or even slightly negative, direct effects on their hosts may play pivotal roles at the community level. Our index scores each species based on how it potentially mediates interactions between all pairs of other species with specific functions, thereby evaluating webs of indirect interactions (Ohgushi, 2005; Guimarães et al., 2017). If species with high functional coreness are introduced at early stages of community assembly, they are expected to support colonization of peripheral species with favorable functions and block entry of species with unfavorable effects (pests or pathogens) into the ecosystem (Toju et al., 2018). In general, microbial species introduced into communities earlier are more likely to persist in the communities, controlling assembly of follower (latecomer) species (Fukami, 2015; Werner and Kiers, 2015; Sprockett et al., 2018). The strategy of embedding core species/strains whose priority effects (Toju et al., 2018) lead whole community structures toward favorable functional profiles deserves further theoretical investigations.
Also importantly, synthetic communities designed by our method are expected to be more resistant to stochastic extinctions and external perturbations than communities designed without considering functional redundancy and possible colonization (contamination) of species from surrounding environments (Stachowicz et al., 1999; Rosenfeld, 2002; Allison and Martiny, 2008). Microbiomes in agroecosystems, human gut, and biofuel production plants are inevitably affected by stochastic loss and immigration of species (Hooper and Gordon, 2001; Morris and Monier, 2003; Kazamia et al., 2012). Given that even communities with a few species often show unpredictable dynamics (Benincà et al., 2008; Sugihara et al., 2012; Deyle et al., 2016; Ushio et al., 2018), keeping community compositions of synthetic communities in practical applications is extremely difficult. Our bird’s-eye approach allows us to deploy backups of functional species, thereby increasing constancy of functional profiles at the ecosystem level. Maximizing robustness of ecosystem-level functions is the key to design synthetic communities with high application values in agriculture, medicine, and industry.
When functional coreness of each species is calculated, we then need to consider sets of functional core species (i.e., functional core microbiomes). Because species belonging to the same network modules are expected to coexist, stable functional core microbiomes would be designed by combining multiple species with highest functional coreness in a target module. We then need to choose a best network module with which a core microbiome is designed. Choosing the module that includes species showing the highest functional coreness within a whole network is a possible solution. Alternatively, properties of modules per se can give us a criterion for choosing among modules (Figure 3): see Morone et al. (2019); Kitsak et al. (2010) for an additional criterion for selecting modules based on topological features. If there are some modules with equally favorable properties, they may be entitled as alternative sources of functional core microbiomes (Toju et al., 2018). For a module, a functional core microbiome would be designed by listing candidate species from the top until functional coreness drops disruptively: in general, inferring optimal number of functional core species is an important issue to be addressed in future studies.
In an application to plant-associated microbiome data, we found that the bird’s-eye approach actually highlighted species with great impacts on other species within microbiomes (Figure 4 and Table 1). For example, the fungus with the highest functional coreness was identified as Penicillium janthinellum, which had been known to show antibacterial properties (Marinho et al., 2005). Likewise, the fungus with the third highest functional coreness was phylogenetically allied to T. asperellum, T. harzianum, and T. viride, which are known to induce plants’ resistance against pathogens (Yedidia et al., 2003; Shoresh et al., 2005; Wu et al., 2017) and suppress various pathogens such as Athelia rolfsii, Rhizoctonia solani, Pythium aphanidermatum, and Fusarium spp. (Elad et al., 1980; Sivan, 1984; Dolatabadi et al., 2012). These abilities possibly contribute to organization of favorable microbiomes in the rhizosphere. Moreover, a phylogenetically close Trichoderma species (T. hamatum) not only increases resistance of hosts against pathogens but also directly promotes plant growth (Studholme et al., 2013). A preliminary inoculation experiment using a fungal strain belonging to the Trichoderma clade (Supporting Figure S1) confirmed the positive effects of the Trichoderma group on preferable host plant conditions (Figure 7). These results suggest that our method can highlight species with both microbiome-regulating abilities and direct positive effects on hosts, while species with only the former characteristics, in principle, can be explored as well. In agricultural applications, those functional core species may be used to activate immune systems of host seeds or seedlings (Kloepper et al., 2004; Pieterse et al., 2014; Mauch-Mani et al., 2017). When inoculated seeds/seedlings are introduced into croplands, the embedded core microbes will block pathogens/pests and recruit species with diverse positive functions from native microbiomes, thereby maximizing microbial functions at the agroecosystem level (Toju et al., 2018).
In contrast to the results on plant-associated microbiomes, those on a mouse gut microbiome dataset spotlighted many unidentified microbes (i.e., microbes with low sequence identities with reference databases) and microbes with unknown functions as candidates of functional cores (Figure 5 and Table 2). Among bacterial ASVs with high functional coreness, only three were unambiguously allied to known taxa (>97% identities with database sequences; Table 2). Of the three, Lactobacillus murinus was known to produce antibacterial compounds (Nardi et al., 2005; Elayaraja et al., 2014) and show positive probiotic effects on host animals (Perelmuter et al., 2008), while the remaining two species, Bacteroides caecimuris and Muribaculum intestinale, have been reported with unknown functions (Lagkouvardos et al., 2016; Brugiroux et al., 2017; Osaka et al., 2017; Medvecky et al., 2018). Detailed analyses on the genomic structures of those bacteria will help us infer roles of those poorly investigated bacteria in gut microbiomes.
Although we put the emphasis of this study on microbiomes associated with plants and animals, the method itself can be applied to other types of microbial (and non-microbial) systems. In this respect, we performed a pilot study based on a laboratory co-culture experiment (Figure 6). The list of bacteria with high functional coreness itself (Table 3) does not provide any inference for industrial applications and results on such experiments would differ depending on used media. Our aim here was to show the entire process of functional coreness analyses in laboratory co-culture systems, which should be optimized for purposes of each trial. Technologies for controlling co-culture systems themselves are prospective in various industrial fields such as food processing, brewing, waste water purification, and biofuel production (Kazamia et al., 2012; Sadoudi et al., 2012; Wang et al., 2019). In addition, experimental co-culture systems can be used to keep “unculturable” microbes that cannot be maintained in single-species culture (Pham and Kim, 2012; Stewart, 2012), increasing repertoires of microbial resources used for screening in drug discovery (Pettit, 2009; Bertrand et al., 2014).
The validity and power of the approach proposed herein need to be examined in future studies with more comprehensive datasets of microbiomes. The rough functional categorization implemented in this study should be replaced with background profiling of genes and metabolic pathways based on reference genome analyses. Building systematic ways for gaining functional information of respective microbial species/strains is an emergent task. From the aspect of application, experimental tests (e.g., inoculations to animal/plant hosts) should be conducted to examine whether designed functional core microbiomes actually increase community- or ecosystem-level functions and stability. Through feedback between in silico community design and its experimental evaluation, parameters in the index [Cfunc(i)] need to be optimized in each system. Comparison of performance of designed communities between bottom-up and bird’s-eye approaches is also necessary. Sophistication of methods for evaluating community stability (McCann, 2000; Allesina and Tang, 2012; Ushio et al., 2018) and those for identifying alternative stable states (Scheffer et al., 2001; Beisner et al., 2003; Suding et al., 2004) and tipping points (Scheffer et al., 2009; Dai et al., 2012; Suzuki et al., 2019) in community dynamics is also awaited. Furthermore, while the present method uses only undirected-graph information of positive interactions, incorporating information of various types of interactions (e.g., mutualism, competition, commensalism, etc.) in directed-graph format (Deyle et al., 2016; Ushio et al., 2018) may enhance the utility of the network-based approach. Albeit in its infancy, interdisciplinary science of synthetic community design is cultivating frontiers in microbiology.
Data Availability Statement
The datasets generated for this study can be found in the Supplementary Material of this article.
Author Contributions
HT conceived and designed the work. HT and MA developed the method. HT, YH, and HF performed the experiment. HT analyzed the data based on inputs from SF and CI. HT wrote the manuscript. All authors contributed to the article and approved the submitted version.
Funding
This work was financially supported in part by the JST PRESTO (JPMJPR16Q6 to HT), JSPS KAKENHI (18H04009 to HT and 18H04805 to SF), JST ERATO (JPMJER1902 to SF), and AMED-CREST (JP19gm1010009 to SF), the Takeda Science Foundation (to SF), the Food Science Institute Foundation (to SF), the Program for the Advancement of Research in Core Projects under Keio University’s Longevity Initiative (to SF), and Human Frontier Science Program (RGP0029/2019 to HT).
Conflict of Interest
The authors declare that the research was conducted in the absence of any commercial or financial relationships that could be construed as a potential conflict of interest.
Kyoto University has applied for the patent related to this article.
Acknowledgments
We thank reviewers for their comments that improved the manuscript.
Supplementary Material
The Supplementary Material for this article can be found online at: https://www.frontiersin.org/articles/10.3389/fmicb.2020.01361/full#supplementary-material
References
Agler, M. T., Ruhe, J., Kroll, S., Morhenn, C., Kim, S.-T., Weigel, D., et al. (2016). Microbial hub taxa link host and abiotic factors to plant microbiome variation. PLoS Biol. 14:e1002352. doi: 10.1371/journal.pbio.1002352
Ahmad, F., Ahmad, I., and Khan, M. (2008). Screening of free-living rhizospheric bacteria for their multiple plant growth promoting activities. Microbiol. Res. 163, 173–181. doi: 10.1016/j.micres.2006.04.001
Allesina, S., and Tang, S. (2012). Stability criteria for complex ecosystems. Nature 483, 205–208. doi: 10.1038/nature10832
Allison, S. D., and Martiny, J. B. (2008). Resistance, resilience, and redundancy in microbial communities. Proc. Natl. Acad. Sci. U.S.A. 105, 11512–11519. doi: 10.1073/pnas.0801925105
Apprill, A., Mcnally, S., Parsons, R., and Weber, L. (2015). Minor revision to V4 region SSU rRNA 806R gene primer greatly increases detection of SAR11 bacterioplankton. Aquat. Microb. Ecol. 75, 129–137. doi: 10.3354/ame01753
Arumugam, M., Raes, J., Pelletier, E., Le Paslier, D., Yamada, T., Mende, D. R., et al. (2011). Enterotypes of the human gut microbiome. Nature 473, 174–180. doi: 10.1038/nature09944
Bai, Y., Müller, D. B., Srinivas, G., Garrido-Oter, R., Potthoff, E., Rott, M., et al. (2015). Functional overlap of the Arabidopsis leaf and root microbiota. Nature 528, 364–369. doi: 10.1038/nature16192
Barberán, A., Bates, S. T., Casamayor, E. O., and Fierer, N. (2012). Using network analysis to explore co-occurrence patterns in soil microbial communities. ISME J. 6, 343–351. doi: 10.1038/ismej.2011.119
Beisner, B. E., Haydon, D. T., and Cuddington, K. (2003). Alternative stable states in ecology. Front. Ecol. Environ. 1, 376–382.
Benincà, E., Huisman, J., Heerkloss, R., Jöhnk, K. D., Branco, P., Van Nes, E. H., et al. (2008). Chaos in a long-term experiment with a plankton community. Nature 451, 822–825. doi: 10.1038/nature06512
Berry, D., and Widder, S. (2014). Deciphering microbial interactions and detecting keystone species with co-occurrence networks. Front. Microbiol. 5:219. doi: 10.3389/fmicb.2014.00219
Bertrand, S., Bohni, N., Schnee, S., Schumpp, O., Gindro, K., and Wolfender, J.-L. (2014). Metabolite induction via microorganism co-culture: a potential way to enhance chemical diversity for drug discovery. Biotechnol. Adv. 32, 1180–1204. doi: 10.1016/j.biotechadv.2014.03.001
Brandes, U. (2001). A faster algorithm for betweenness centrality. J. Math. Sociol. 25, 163–177. doi: 10.1080/0022250x.2001.9990249
Bravo, J. A., Forsythe, P., Chew, M. V., Escaravage, E., Savignac, H. M., Dinan, T. G., et al. (2011). Ingestion of Lactobacillus strain regulates emotional behavior and central GABA receptor expression in a mouse via the vagus nerve. Proc. Natl. Acad. Sci. U.S.A. 108, 16050–16055. doi: 10.1073/pnas.1102999108
Brenner, K., You, L., and Arnold, F. H. (2008). Engineering microbial consortia: a new frontier in synthetic biology. Trends Biotechnol. 26, 483–489. doi: 10.1016/j.tibtech.2008.05.004
Brugiroux, S., Beutler, M., Pfann, C., Garzetti, D., Ruscheweyh, H.-J., Ring, D., et al. (2017). Genome-guided design of a defined mouse microbiota that confers colonization resistance against Salmonella enterica serovar Typhimurium. Nat. Microbiol. 2:16215.
Busby, P. E., Soman, C., Wagner, M. R., Friesen, M. L., Kremer, J., Bennett, A., et al. (2017). Research priorities for harnessing plant microbiomes in sustainable agriculture. PLoS Biol. 15:e2001793. doi: 10.1371/journal.pbio.2001793
Callahan, B. J., Mcmurdie, P. J., Rosen, M. J., Han, A. W., Johnson, A. J. A., and Holmes, S. P. (2016). DADA2: high-resolution sample inference from Illumina amplicon data. Nat. Methods 13, 581–583. doi: 10.1038/nmeth.3869
Caporaso, J. G., Lauber, C. L., Walters, W. A., Berg-Lyons, D., Lozupone, C. A., Turnbaugh, P. J., et al. (2011). Global patterns of 16S rRNA diversity at a depth of millions of sequences per sample. Proc. Natl. Acad. Sci. U.S.A. 108, 4516–4522. doi: 10.1073/pnas.1000080107
Cassan, F., Perrig, D., Sgroy, V., Masciarelli, O., Penna, C., and Luna, V. (2009). Azospirillum brasilense Az39 and Bradyrhizobium japonicum E109, inoculated singly or in combination, promote seed germination and early seedling growth in corn (Zea mays L.) and soybean (Glycine max L.). Eur. J. Soil Biol. 45, 28–35. doi: 10.1016/j.ejsobi.2008.08.005
Clauset, A., Newman, M. E., and Moore, C. (2004). Finding community structure in very large networks. Phys. Rev. E 70:066111. doi: 10.1103/PhysRevE.70.066111
Csardi, G., and Nepusz, T. (2006). The igraph software package for complex network research. Interjournal Complex Syst. 1695, 1–9.
Dai, L., Vorselen, D., Korolev, K. S., and Gore, J. (2012). Generic indicators for loss of resilience before a tipping point leading to population collapse. Science 336, 1175–1177. doi: 10.1126/science.1219805
De Roy, K., Marzorati, M., Van Den Abbeele, P., Van De Wiele, T., and Boon, N. (2014). Synthetic microbial ecosystems: an exciting tool to understand and apply microbial communities. Environ. Microbiol. 16, 1472–1481. doi: 10.1111/1462-2920.12343
Deyle, E. R., May, R. M., Munch, S. B., and Sugihara, G. (2016). Tracking and forecasting ecosystem interactions in real time. Proc. R. Soc. Lond. Ser. B 283:20152258. doi: 10.1098/rspb.2015.2258
Dolatabadi, H. K., Goltapeh, E. M., Mohammadi, N., Rabiey, M., Rohani, N., and Varma, A. (2012). Biocontrol potential of root endophytic fungi and Trichoderma species against Fusarium wilt of lentil under in vitro and greenhouse conditions. J. Agric. Sci. Technol. 14, 407–420.
Dormann, C. F., Fründ, J., Blüthgen, N., and Gruber, B. (2009). Indices, graphs and null models: analyzing bipartite ecological networks. Open Ecol. J. 2, 7–24. doi: 10.2174/1874213000902010007
Elad, Y., Chet, I., and Katan, J. (1980). Trichoderma harzianum: a biocontrol agent effective against Sclerotium rolfsii and Rhizoctonia solani. Dis. Contr. Pest Manag. 70, 119–121.
Elayaraja, S., Annamalai, N., Mayavu, P., and Balasubramanian, T. (2014). Production, purification and characterization of bacteriocin from Lactobacillus murinus AU06 and its broad antibacterial spectrum. Asian Pac. J. Trop. Biomed. 4, S305–S311. doi: 10.12980/APJTB.4.2014C537
Faith, J. J., Rey, F. E., O’donnell, D., Karlsson, M., Mcnulty, N. P., Kallstrom, G., et al. (2010). Creating and characterizing communities of human gut microbes in gnotobiotic mice. ISME J. 4, 1094–1098. doi: 10.1038/ismej.2010.110
Faust, K., Sathirapongsasuti, J. F., Izard, J., Segata, N., Gevers, D., Raes, J., et al. (2012). Microbial co-occurrence relationships in the human microbiome. PLoS Comput. Biol. 8:e1002606. doi: 10.1371/journal.pcbi.1002606
Friedman, J., and Alm, E. J. (2012). Inferring correlation networks from genomic survey data. PLoS Comput. Biol. 8:e1002687. doi: 10.1371/journal.pcbi.1002687
Fukami, T. (2015). Historical contingency in community assembly: integrating niches, species pools, and priority effects. Ann. Rev. Ecol. Evol. Syst. 46, 1–23. doi: 10.1146/annurev-ecolsys-110411-160340
Gorbach, S. L. (2000). Probiotics and gastrointestinal health. Am. J. Gastroenterol. 95, S2–S4. doi: 10.1016/s0002-9270(99)00806-0
Guimarães, P. R. Jr., Pires, M. M., Jordano, P., Bascompte, J., and Thompson, J. N. (2017). Indirect effects drive coevolution in mutualistic networks. Nature 550, 511–514. doi: 10.1038/nature24273
Guimera, R., and Amaral, L. A. N. (2005). Cartography of complex networks: modules and universal roles. J. Stat. Mech. 2005, P02001-1–P02001-13. doi: 10.1088/1742-5468/2005/02/P02001
Hamady, M., Walker, J. J., Harris, J. K., Gold, N. J., and Knight, R. (2008). Error-correcting barcoded primers for pyrosequencing hundreds of samples in multiplex. Nat. Methods 5, 235–237. doi: 10.1038/nmeth.1184
Han, H. S., Supanjani, and Lee, K. D. (2006). Effect of co-inoculation with phosphate and potassium solubilizing bacteria on mineral uptake and growth of pepper and cucumber. Plant Soil Environ. 52, 130–136. doi: 10.17221/3356-Pse
Hashimoto, H., Arai, T., Mori, A., Kawai, K., Hikishima, K., Ohnishi, Y., et al. (2009). Reconsideration of insulin signals induced by improved laboratory animal diets, Japanese and American diets, in IRS-2 deficient mice. Exp. Clin. Endocrinol. Diabetes 117, 577–586. doi: 10.1055/s-0029-1225352
Hooper, L. V., and Gordon, J. I. (2001). Commensal host-bacterial relationships in the gut. Science 292, 1115–1118. doi: 10.1126/science.1058709
Hsieh, C.-H., Glaser, S. M., Lucas, A. J., and Sugihara, G. (2005). Distinguishing random environmental fluctuations from ecological catastrophes for the North Pacific Ocean. Nature 435, 336–340. doi: 10.1038/nature03553
Ishii, C., Nakanishi, Y., Murakami, S., Nozu, R., Ueno, M., Hioki, K., et al. (2018). A metabologenomic approach reveals changes in the intestinal environment of mice fed on American diet. Int. J. Mol. Sci. 19:4079. doi: 10.3390/ijms19124079
Jacobsen, C. N., Nielsen, V. R., Hayford, A., Møller, P. L., Michaelsen, K., Paerregaard, A., et al. (1999). Screening of probiotic activities of forty-seven strains of Lactobacillus spp. by in vitro techniques and evaluation of the colonization ability of five selected strains in humans. Appl. Environ. Microbiol. 65, 4949–4956. doi: 10.1128/aem.65.11.4949-4956.1999
Kanehisa, M., Goto, S., Sato, Y., Furumichi, M., and Tanabe, M. (2011). KEGG for integration and interpretation of large-scale molecular data sets. Nucleic Acids Res. 40, D109–D114. doi: 10.1093/nar/gkr988
Kanehisa, M., Sato, Y., Kawashima, M., Furumichi, M., and Tanabe, M. (2015). KEGG as a reference resource for gene and protein annotation. Nucleic Acids Res. 44, D457–D462.
Kazamia, E., Aldridge, D. C., and Smith, A. G. (2012). Synthetic ecology–a way forward for sustainable algal biofuel production? J. Biotechnol. 162, 163–169. doi: 10.1016/j.jbiotec.2012.03.022
Kitada, Y., Muramatsu, K., Toju, H., Kibe, R., Benno, Y., Kurihara, S., et al. (2018). Bioactive polyamine production by a novel hybrid system comprising multiple indigenous gut bacterial strategies. Sci. Adv. 4:eaat0062. doi: 10.1126/sciadv.aat0062
Kitsak, M., Gallos, L. K., Havlin, S., Liljeros, F., Muchnik, L., Stanley, H. E., et al. (2010). Identification of influential spreaders in complex networks. Nat. Phys. 6, 888–893. doi: 10.1038/Nphys1746
Kloepper, J. W., Ryu, C.-M., and Zhang, S. (2004). Induced systemic resistance and promotion of plant growth by Bacillus spp. Phytopathology 94, 1259–1266. doi: 10.1094/PHYTO.2004.94.11.1259
Kurtz, Z. D., Mueller, C. L., Miraldi, E. R., Littman, D. R., Blaser, M. J., and Bonneau, R. A. (2015). Sparse and compositionally robust inference of microbial ecological networks. PLoS Comput. Biol. 11:e1004226. doi: 10.1371/journal.pcbi.1004226
Lagkouvardos, I., Pukall, R., Abt, B., Foesel, B. U., Meier-Kolthoff, J. P., Kumar, N., et al. (2016). The mouse intestinal bacterial collection (miBC) provides host-specific insight into cultured diversity and functional potential of the gut microbiota. Nat. Microbiol. 1:16131.
Lancichinetti, A., Radicchi, F., Ramasco, J. J., and Fortunato, S. (2011). Finding statistically significant communities in networks. PLoS One 6:e18961. doi: 10.1371/journal.pone.0018961
Layeghifard, M., Hwang, D. M., and Guttman, D. S. (2017). Disentangling interactions in the microbiome: a network perspective. Trends Microbiol. 25, 217–228. doi: 10.1016/j.tim.2016.11.008
Ley, R. E., Peterson, D. A., and Gordon, J. I. (2006). Ecological and evolutionary forces shaping microbial diversity in the human intestine. Cell 124, 837–848. doi: 10.1016/j.cell.2006.02.017
Louca, S., Parfrey, L. W., and Doebeli, M. (2016). Decoupling function and taxonomy in the global ocean microbiome. Science 353, 1272–1277. doi: 10.1126/science.aaf4507
Lundberg, D. S., Yourstone, S., Mieczkowski, P., Jones, C. D., and Dangl, J. L. (2013). Practical innovations for high-throughput amplicon sequencing. Nat. Methods 10, 999–1002. doi: 10.1038/nmeth.2634
Marinho, A. M., Rodrigues-Filho, E., Moitinho, M. D. L. R., and Santos, L. S. (2005). Biologically active polyketides produced by Penicillium janthinellum isolated as an endophytic fungus from fruits of Melia azedarach. J. Braz. Chem. Soc. 16, 280–283. doi: 10.1590/s0103-50532005000200023
Mauch-Mani, B., Baccelli, I., Luna, E., and Flors, V. (2017). Defense priming: an adaptive part of induced resistance. Ann. Rev. Plant Biol. 68, 485–512. doi: 10.1146/annurev-arplant-042916-041132
Medvecky, M., Cejkova, D., Polansky, O., Karasova, D., Kubasova, T., Cizek, A., et al. (2018). Whole genome sequencing and function prediction of 133 gut anaerobes isolated from chicken caecum in pure cultures. BMC Genomics 19:561. doi: 10.1186/s12864-018-4959-4
Morone, F., Del Ferraro, G., and Makse, H. A. (2019). The k-core as a predictor of structural collapse in mutualistic ecosystems. Nat. Phys. 15, 95–102. doi: 10.1038/s41567-018-0304-8
Morris, C. E., and Monier, J.-M. (2003). The ecological significance of biofilm formation by plant-associated bacteria. Ann. Rev. Phytopathol. 41, 429–453. doi: 10.1146/annurev.phyto.41.022103.134521
Nardi, R., Santoro, M., Oliveira, J., Pimenta, A., Ferraz, V., Benchetrit, L., et al. (2005). Purification and molecular characterization of antibacterial compounds produced by Lactobacillus murinus strain L1. J. Appl. Microbiol. 99, 649–656. doi: 10.1111/j.1365-2672.2005.02632.x
Nguyen, N. H., Song, Z., Bates, S. T., Branco, S., Tedersoo, L., Menke, J., et al. (2016). FUNGuild: an open annotation tool for parsing fungal community datasets by ecological guild. Fungal Ecol. 20, 241–248. doi: 10.1016/j.funeco.2015.06.006
Ohgushi, T. (2005). Indirect interaction webs: herbivore-induced effects through trait change in plants. Annu. Rev. Ecol. Evol. Syst. 36, 81–105. doi: 10.1146/annurev.ecolsys.36.091704.175523
Osaka, T., Moriyama, E., Arai, S., Yagi, J., Kikuchi, J., and Tsuneda, S. (2017). Meta-analysis of fecal microbiota and metabolites in experimental colitic mice during the inflammatory and healing phases. Nutrients 9:1329. doi: 10.3390/nu9121329
Paredes, S. H., Gao, T., Law, T. F., Finkel, O. M., Mucyn, T., Teixeira, P. J. P. L., et al. (2018). Design of synthetic bacterial communities for predictable plant phenotypes. PLoS Biol. 16:e2003962. doi: 10.1371/journal.pbio.2003962
Perelmuter, K., Fraga, M., and Zunino, P. (2008). In vitro activity of potential probiotic Lactobacillus murinus isolated from the dog. J. Appl. Microbiol. 104, 1718–1725. doi: 10.1111/j.1365-2672.2007.03702.x
Peterson, G., Allen, C. R., and Holling, C. S. (1998). Ecological resilience, biodiversity, and scale. Ecosystems 1, 6–18. doi: 10.1007/s100219900002
Pettit, R. K. (2009). Mixed fermentation for natural product drug discovery. Appl. Microbiol. Biotechnol. 83, 19–25. doi: 10.1007/s00253-009-1916-9
Pham, V. H., and Kim, J. (2012). Cultivation of unculturable soil bacteria. Trends Biotechnol. 30, 475–484. doi: 10.1016/j.tibtech.2012.05.007
Pieterse, C. M., Zamioudis, C., Berendsen, R. L., Weller, D. M., Van Wees, S. C., and Bakker, P. A. (2014). Induced systemic resistance by beneficial microbes. Ann. Rev. Phytopathol. 52, 347–375. doi: 10.1146/annurev-phyto-082712-102340
Qin, J., Li, R., Raes, J., Arumugam, M., Burgdorf, K. S., Manichanh, C., et al. (2010). A human gut microbial gene catalogue established by metagenomic sequencing. Nature 464, 59–65. doi: 10.1038/nature08821
Quast, C., Pruesse, E., Yilmaz, P., Gerken, J., Schweer, T., Yarza, P., et al. (2012). The SILVA ribosomal RNA gene database project: improved data processing and web-based tools. Nucleic Acids Res. 41, D590–D596. doi: 10.1093/nar/gks1219
Ratner, M. (2015). Microbial cocktails join fecal transplants in IBD treatment trials. Nat. Biotechnol. 33, 787–788. doi: 10.1038/nbt0815-787
Ritze, Y., Bárdos, G., Claus, A., Ehrmann, V., Bergheim, I., Schwiertz, A., et al. (2014). Lactobacillus rhamnosus GG protects against non-alcoholic fatty liver disease in mice. PLoS One 9:e80169. doi: 10.1371/journal.pone.0080169
Rosenfeld, J. S. (2002). Functional redundancy in ecology and conservation. Oikos 98, 156–162. doi: 10.1034/j.1600-0706.2002.980116.x
Sadoudi, M., Tourdot-Maréchal, R., Rousseaux, S., Steyer, D., Gallardo-Chacón, J.-J., Ballester, J., et al. (2012). Yeast–yeast interactions revealed by aromatic profile analysis of Sauvignon Blanc wine fermented by single or co-culture of non-Saccharomyces and Saccharomyces yeasts. Food Microbiol. 32, 243–253. doi: 10.1016/j.fm.2012.06.006
Scheffer, M., Bascompte, J., Brock, W. A., Brovkin, V., Carpenter, S. R., Dakos, V., et al. (2009). Early-warning signals for critical transitions. Nature 461, 53–59. doi: 10.1038/nature08227
Scheffer, M., Carpenter, S., Foley, J. A., Folke, C., and Walker, B. (2001). Catastrophic shifts in ecosystems. Nature 413, 591–596. doi: 10.1038/35098000
Schreiber, T. (2000). Measuring information transfer. Phys. Rev. Lett. 85, 461–464. doi: 10.1103/PhysRevLett.85.461
Shoresh, M., Yedidia, I., and Chet, I. (2005). Involvement of jasmonic acid/ethylene signaling pathway in the systemic resistance induced in cucumber by Trichoderma asperellum T203. Phytopathology 95, 76–84. doi: 10.1094/phyto-95-0076
Shou, W. Y., Ram, S., and Vilar, J. M. G. (2007). Synthetic cooperation in engineered yeast populations. Proc. Natl. Acad. Sci. U.S.A. 104, 1877–1882. doi: 10.1073/pnas.0610575104
Sivan, A. (1984). Biological control effects of a new isolate of Trichoderma harzianum on Pythium aphanidermatum. Phytopathology 74, 498–501. doi: 10.1094/Phyto-74-498
Sprockett, D., Fukami, T., and Relman, D. A. (2018). Role of priority effects in the early-life assembly of the gut microbiota. Nat. Rev. Gastroenterol. Hepatol. 15, 197–205. doi: 10.1038/nrgastro.2017.173
Stachowicz, J. J., Whitlatch, R. B., and Osman, R. W. (1999). Species diversity and invasion resistance in a marine ecosystem. Science 286, 1577–1579. doi: 10.1126/science.286.5444.1577
Stenuit, B., and Agathos, S. N. (2015). Deciphering microbial community robustness through synthetic ecology and molecular systems synecology. Curr. Opin. Biotechnol. 33, 305–317. doi: 10.1016/j.copbio.2015.03.012
Stevens, J. L., Jackson, R. L., and Olson, J. B. (2013). Slowing PCR ramp speed reduces chimera formation from environmental samples. J. Microbiol. Methods 93, 203–205. doi: 10.1016/j.mimet.2013.03.013
Stewart, E. J. (2012). Growing unculturable bacteria. J. Bacteriol. 194, 4151–4160. doi: 10.1128/JB.00345-12
Studholme, D. J., Harris, B., Le Cocq, K., Winsbury, R., Perera, V., Ryder, L., et al. (2013). Investigating the beneficial traits of Trichoderma hamatum GD12 for sustainable agriculture—insights from genomics. Front. Plant Sci. 4:258. doi: 10.3389/fpls.2013.00258
Suding, K. N., Gross, K. L., and Houseman, G. R. (2004). Alternative states and positive feedbacks in restoration ecology. Trends Ecol. Evol. 19, 46–53. doi: 10.1016/j.tree.2003.10.005
Sugihara, G., May, R., Ye, H., Hsieh, C.-H., Deyle, E., Fogarty, M., et al. (2012). Detecting causality in complex ecosystems. Science 338, 496–500. doi: 10.1126/science.1227079
Suzuki, K., Nakaoka, S., Fukuda, S., and Masuya, H. (2019). Energy landscape analysis of ecological communities elucidates the phase space of community assembly dynamics. bioRxiv [Preprint] doi: 10.1101/709956
Suzuki, K., Yoshida, K., Nakanishi, Y., and Fukuda, S. (2017). An equation-free method reveals the ecological interaction networks within complex microbial ecosystems. Methods Ecol. Evol. 8, 1774–1785. doi: 10.1111/2041-210x.12814
Tanabe, A. S. (2018). Claident v0.2.2018.05.29, a Software Distributed by Author. Available at: http://www.fifthdimension.jp/ (accessed May 29, 2018).
Toju, H., Guimarães, P. R., Olesen, J. M., and Thompson, J. N. (2014). Assembly of complex plant–fungus networks. Nat. Commun. 5:5273. doi: 10.1038/ncomms6273
Toju, H., Okayasu, K., and Notaguchi, M. (2019). Leaf-associated microbiomes of grafted tomato plants. Sci. Rep. 9:1787. doi: 10.1038/s41598-018-38344-2
Toju, H., Peay, K. G., Yamamichi, M., Narisawa, K., Hiruma, K., Naito, K., et al. (2018). Core microbiomes for sustainable agroecosystems. Nat. Plants 4, 247–257. doi: 10.1038/s41477-018-0139-4
Toju, H., Tanabe, A., and Ishii, H. (2016a). Ericaceous plant–fungus network in a harsh alpine–subalpine environment. Mol. Ecol. 25, 3242–3257. doi: 10.1111/mec.13680
Toju, H., Yamamoto, S., Tanabe, A. S., Hayakawa, T., and Ishii, H. S. (2016b). Network modules and hubs in plant-root fungal biomes. J. R. Soc. Interface 13:20151097. doi: 10.1098/rsif.2015.1097
Toju, H., and Tanaka, Y. (2019). Consortia of anti-nematode fungi and bacteria in the rhizosphere of soybean plants attacked by root-knot nematodes. R. Soc. Open Sci. 6:181693. doi: 10.1098/rsos.181693
Toju, H., Yamamichi, M., Guimarães, P. R. Jr., Olesen, J. M., Mougi, A., Yoshida, T., et al. (2017). Species-rich networks and eco-evolutionary synthesis at the metacommunity level. Nat. Ecol. Evol. 1:0024. doi: 10.1038/s41559-016-0024
Ushio, M., Hsieh, C.-H., Masuda, R., Deyle, E. R., Ye, H., Chang, C.-W., et al. (2018). Fluctuating interaction network and time-varying stability of a natural fish community. Nature 554, 360–363. doi: 10.1038/nature25504
van der Heijden, M. G., and Hartmann, M. (2016). Networking in the plant microbiome. PLoS Biol. 14:e1002378. doi: 10.1371/journal.pbio.1002378
Vázquez, D. P., Melián, C. J., Williams, N. M., Blüthgen, N., Krasnov, B. R., and Poulin, R. (2007). Species abundance and asymmetric interaction strength in ecological networks. Oikos 116, 1120–1127. doi: 10.1111/j.0030-1299.2007.15828.x
Venturelli, O. S., Carr, A. V., Fisher, G., Hsu, R. H., Lau, R., Bowen, B. P., et al. (2018). Deciphering microbial interactions in synthetic human gut microbiome communities. Mol. Syst. Biol. 14:e8157. doi: 10.15252/msb.20178157
Wang, L., York, S. W., Ingram, L. O., and Shanmugam, K. (2019). Simultaneous fermentation of biomass-derived sugars to ethanol by a co-culture of an engineered Escherichia coli and Saccharomyces cerevisiae. Bioresour. Technol. 273, 269–276. doi: 10.1016/j.biortech.2018.11.016
Werner, G. D., and Kiers, E. T. (2015). Order of arrival structures arbuscular mycorrhizal colonization of plants. New Phytol. 205, 1515–1524. doi: 10.1111/nph.13092
Wu, Q., Sun, R. Y., Ni, M., Yu, J., Li, Y. Q., Yu, C. J., et al. (2017). Identification of a novel fungus, Trichoderma asperellum GDFS1009, and comprehensive evaluation of its biocontrol efficacy. PLoS One 12: e0179957. doi: 10.1371/journal.pone.0179957
Yedidia, I., Shoresh, M., Kerem, Z., Benhamou, N., Kapulnik, Y., and Chet, I. (2003). Concomitant induction of systemic resistance to Pseudomonas spingae pv. lachrymans in cucumber by Trichoderma asperellum (T-203) and accumulation of phytoalexins. Appl. Environ. Microbiol. 69, 7343–7353. doi: 10.1128/aem.69.12.7343-7353.2003
Keywords: biodiversity, biospheres, metagenomes, keystone species, hub species, network theory, synthetic biology, species interactions
Citation: Toju H, Abe MS, Ishii C, Hori Y, Fujita H and Fukuda S (2020) Scoring Species for Synthetic Community Design: Network Analyses of Functional Core Microbiomes. Front. Microbiol. 11:1361. doi: 10.3389/fmicb.2020.01361
Received: 16 March 2020; Accepted: 27 May 2020;
Published: 25 June 2020.
Edited by:
Jessy L. Labbé, Oak Ridge National Laboratory (DOE), United StatesReviewed by:
Tomasz Wilmanski, Institute for Systems Biology, United StatesSuleyman Yildirim, Istanbul Medipol University, Turkey
Copyright © 2020 Toju, Abe, Ishii, Hori, Fujita and Fukuda. This is an open-access article distributed under the terms of the Creative Commons Attribution License (CC BY). The use, distribution or reproduction in other forums is permitted, provided the original author(s) and the copyright owner(s) are credited and that the original publication in this journal is cited, in accordance with accepted academic practice. No use, distribution or reproduction is permitted which does not comply with these terms.
*Correspondence: Hirokazu Toju, dG9qdS5oaXJva2F6dS40Y0BreW90by11LmFjLmpw