- 1Fralin Biomedical Research Institute at VTC, Roanoke, VA, United States
- 2Department of Internal Medicine, Virginia Tech Carilion School of Medicine, Roanoke, VA, United States
- 3Virginia Tech Center for Drug Discovery, Virginia Tech, Blacksburg, VA, United States
- 4Wake Forest Baptist Comprehensive Cancer Center, Winston-Salem, NC, United States
- 5Faculty of Health Science, Virginia Tech, Blacksburg, VA, United States
- 6Basic Science Education, Virginia Tech Carilion School of Medicine, Roanoke, VA, United States
- 7Department of Biological Sciences, College of Science, Virginia Tech, Blacksburg, VA, United States
- 8Department of Biological Affairs and Research, Edward Via College of Osteopathic Medicine, Blacksburg, VA, United States
Glioblastoma, the most common malignant tumor in the brain, lacks effective treatments and is currently incurable. To identify novel drug targets for this deadly cancer, the publicly available results of RNA interference screens from the Project Achilles database were analyzed. Ten candidate genes were identified as survival genes in 15 glioblastoma cell lines. RAN, member RAS oncogene family (RAN) was expressed in glioblastoma at the highest level among all candidates based upon cDNA microarray data. However, Kaplan-Meier survival analysis did not show any correlation between RAN mRNA levels and patient survival. Because RAN is a small GTPase that regulates nuclear transport controlled by karyopherin subunit beta 1 (KPNB1), RAN was further analyzed together with KPNB1. Indeed, GBM patients with high levels of RAN also had more KPNB1 and levels of KPNB1 alone did not relate to patient prognosis. Through a Cox multivariate analysis, GBM patients with high levels of RAN and KPNB1 showed significantly shorter life expectancy when temozolomide and promoter methylation of O6-methylguanine DNA methyltransferase were used as covariates. These results indicate that RAN and KPNB1 together are associated with drug resistance and GBM poor prognosis. Furthermore, the functional blockade of RAN and KPNB1 by importazole remarkably suppressed cell viability and activated apoptosis in GBM cells expressing high levels of RAN, while having a limited effect on astrocytes and GBM cells with undetectable RAN. Together, our results demonstrate that RAN activity is important for GBM survival and the functional blockade of RAN/KPNB1 is an appealing therapeutic approach.
Introduction
Glioblastoma (GBM) is an aggressive tumor generally found in the cerebral hemispheres of the brain. Spanning 16% of the cases of all primary tumors in the brain and ~50% of all malignant brain tumors, GBM is the most common malignant type in the central nervous system (1, 2). The average length of survival for GBM patients is ~15 months, and only about 5.5% of patients will live longer than 5 years after diagnosis and aggressive treatments, such as chemotherapy, radiation therapy, and surgical resection (1, 3–5). However, surgery is not sufficient for a clean and complete resection of the tumor mass due to the infiltration of tumor cells into the normal brain parenchyma. The remaining tumor cells are often refractory to chemo drugs and radiation, thereby contributing to the high incidence of tumor recurrence that is robustly associated with a poor prognosis of GBM (6–9). Therefore, more effective treatments are needed.
To identify novel therapeutic targets for GBM, we and other research groups used RNA interference (RNAi) screening, a technique that allows a simultaneous analysis of genes in a genome for their functions in a particular setting. For example, we performed a genome-wide RNAi screen using a diphtheria toxin negative selection approach (10) and uncovered a molecular pathway that controls the transcription of activating transcription factor 5, a key survival factor for GBM (11). Identification of this molecular survival pathway has led to a phase I clinical trial, in which a combination of radiation and sorafenib, an inhibitor of RAF kinase that suppresses the expression of activating transcription factor 5, was used to treat GBM patients (12). In another study, we carried out a kinome RNAi drop-out screen, through which 20 kinases were identified as survival kinase genes (7). Among these candidates, casein kinase 1 epsilon (13) and phosphatidylinositol-4,5-bisphosphate 3-kinase catalytic subunit beta (14, 15) were further verified as essential survival factors and appealing drug targets for GBM. Studies from other groups have also revealed possible therapeutic targets (e.g., PFKFB4, PLK1, SGK1, NLK, etc.) for GBM using RNAi screens (16–19). Hence, RNAi screening is a proven, useful tool for identifying novel drug targets for GBM.
Recently, the Broad Institute initiated a program termed Project Achilles (20–23). This project aims to complete genome-wide RNAi or CRISPR-Cas9 screens in more than 1,000 different cancer cell lines in order to unveil survival genes in cancer cells and to provide a comprehensive cancer dependency map, allowing for the elimination of tedious and repetitive work of RNAi screens in different laboratories so researchers can further analyze the RNAi screen results to uncover cancer survival genes and develop effective cancer treatments. The principle of these so-called “drop-out” screens is based on the hypothesis that short hairpin RNAs (shRNAs) or guide RNAs (gRNAs) of genes that are essential for cancer cell survival induce cell death; hence, cells with these shRNAs or gRNAs will be depleted over time. By comparing the sequencing reads of shRNAs or gRNAs in cells at the initial and end time point, shRNAs or gRNAs that are lost or under-represented (due to the depletion of cells) will be identified. Results of RNAi screens in more than 500 cancer cell lines, including 15 GBM cell lines, have recently been made available to the public, offering us an opportunity to search for more survival genes in GBM.
In this report, we analyzed RNAi screen results in 15 GBM cell lines and identified 10 candidate genes that are important for the survival of GBM cells. Further comprehensive analyses revealed one gene, RAN (RAN, member RAS oncogene family), as the top candidate because this gene was highly expressed in GBM and its activity was robustly associated with drug resistance and poor prognosis in GBM. RAN is a small GTPase protein that provides energy for nucleocytoplasmic transport and mitotic spindle assembly by hydrolyzing guanosine triphosphate into guanosine diphosphate (24–28). Through this released energy, RAN regulates the activities of the importin protein complexes that mediate nuclear import and export (27–29). Hence, this protein has been implicated in the genesis and disease progression of numerous different types of cancer (30–38). However, the role of RAN in GBM has not yet been extensively explored, despite being shown in two studies as a regulator of apoptosis through blocking Bcl-2-associated X protein and activating survival pathways in GBM cells (39, 40). Directly and selectively targeting RAN is difficult and has not been very successful so far (40, 41). It has been recently shown that importazole, a small molecule inhibitor of RAN and KPNB1, blocks the interactions between RAN and KPNB1 based upon results from fluorescence resonance energy transfer, nuclear localization of fluorescent proteins, and co-immunoprecipitation. The disruption of RAN/KPNB1 complexes represses RAN/KPNB1-mediated nuclear transport (42). We therefore chose importazole to test whether a blockade of RAN activity would inhibit GBM cell viability. While importazole has been tested in different types of cancer, this drug (43–46) has not yet been applied to GBM. We found that blocking the activity of this candidate gene activated cell death and induced a potent inhibition of cell growth in GBM cell lines as well as primary GBM cells, presenting a possibility as an effective drug target for GBM.
Methods and Materials
Materials
GBM cell lines, primary GBM cells, and normal human astrocytes were cultured as described previously (7, 14, 47). In brief, GBM cell lines A172, LN-18, SF-268, SF-295, T98MG, U251, and U87MG were maintained in Dulbecco's Modified Eagle Medium (DMEM, Life Technologies) supplemented with 10% EquaFETAL® bovine serum (Atlas Biologicals, Inc.) and 100 μg/ml streptomycin and 100 IU/ml penicillin (Gibco). Primary cells VTC-001, VTC-002, VTC-004, VTC-037, VTC-056, VTC-058, VTC-084, and VTC-103 were cultured in DMEM supplemented with 15% fetal bovine serum (Peak Serum, Inc.) and penicillin/streptomycin. Normal human astrocytes were cultured in MCDB-131 Medium (Sigma) containing 3% fetal bovine serum (Peak Serum, Inc.), 10 X G-5 Supplement (Gibco), and penicillin/streptomycin. Cell lines have been authenticated by the ATCC authentication service utilizing Short Tandem Repeat (STR) profiling. Primary GBM cells were kept at low passages (no more than 10). Antibodies of RAN and GAPDH were purchased from Santa Cruz Biotechnology, Inc. Importazole was purchased from Cayman Chemicals, Inc. Stock solution of importazole was prepared at 50 mM using dimethyl sulfoxide (DMSO). Working solution was further diluted using cell culture media.
Analysis of RNAi Screen Results From the Project Achilles
RNAi screen results (Achilles_v2.4.6.rnai.gct) were retrieved from the Project Achilles database at the following website: https://portals.broadinstitute.org/achilles. The screen contains more than 50,000 short hairpin RNAs (shRNAs) that target the human genome and the results were presented as fold changes of shRNA loss (log2). The lower the fold change of a particular shRNA, the stronger the depletion of the shRNA in GBM cells. This shRNA depletion is, as hypothesized, due to the loss of cells over time. Results of these shRNAs in 15 GBM cell lines (A172, DBTRG05MG, DKMG, GB1, LN229, LN340, LN382, LN428, LN443, LN464, SF172, SNU1105, U343, U87MG, and YKG1) were first sorted by two or more shRNAs targeting one single gene. More than 4,000 genes were targeted by two or more shRNAs. Next, the fold changes of shRNA loss were averaged. Candidate shRNAs with an average of fold change <-4.0 and a fold change <-3.0 in all 15 GBM cell lines were selected.
Gene Expression Analyses Using Online Databases
cDNA microarray data were retrieved from BioGPS (http://biogps.org/#goto=welcome), Oncomine (https://www.oncomine.org/resource/login.html), Glioblastoma Bio Discovery Portal (https://gbm-biodp.nci.nih.gov), and The Cancer Genome Atlas (TCGA) database (http://www.cbioportal.org/index.do). Data from BioGPS were reanalyzed. The arbitrary units of mRNAs of candidate genes in GBM cell lines were divided by those in astrocytes, yielding fold changes (GBM/Astrocytes). Regarding data from the Oncomine database, fold changes of candidate gene mRNAs in GBM tissues normalized with those in normal brain tissues from three different studies (Shai Brain, Murat Brain, and Brendel Brain 2) were recorded and summarized in Table 1. P-values that determine the statistical significance of fold changes were included as well. mRNA levels of candidate genes in different subtypes of GBM were retrieved from Glioblastoma Bio Discovery Portal. Patient numbers of classical, mesenchymal, and proneural GBM subtypes were 199, 166, and 163, respectively. Levels of candidate gene mRNAs in GBM subtypes were then averaged. RAN levels and MGMT promoter methylation status in GBM patients were retrieved from the TCGA database and were re-analyzed using JMP software.
Kaplan-Meier Survival Analysis
Kaplan-Meier survival analyses of GBM patients from the TCGA database have been reported in GlioVis (http://gliovis.bioinfo.cnio.es), Glioblastoma Bio Discovery Portal, and The Human Protein Atlas (http://www.proteinatlas.org). The survival results were retrieved from these databases and presented together with the Log-rank P-values.
Cox Univariate and Multivariate Analysis
Gene expression data and clinical information of GBM patients were retrieved from the TCGA database (http://www.cbioportal.org/index.do). The correlation between mRNA levels and GBM patient survival was determined by Cox univariate or multivariate analysis using the JMP software as previously described (7). Hazard ratios (HR, chance of death) with P-values determining HR probabilities larger than Chi-squares were shown. The lower and upper 95% confidence intervals were plotted as well.
MTS Cell Viability Assay
The MTS cell viability assay was described previously (14, 47, 48). In brief, GBM cell lines, primary GBM cells, and astrocytes were dissociated as single cells and then plated at 500, 1,000, or 4,000 cells per well, respectively, in 100 μl of culture media in a 96-well plate. Next day, cells were treated with importazole at 12.5 μM or at various concentrations (3.125, 6.25, 12.5, 25, or 50 μM, respectively) for 3 or 6 days. A 0.1% DMSO solution was used as the control. At the end point, stock MTS reagent (Promega) was diluted in culture media at 1:10 and added to each well. Two hours later, the absorbance at 490 nm (detecting the color change of MTS in live cells) was measured using a microplate reader (Molecular Devices). Percentages of cell viability were obtained by dividing the MTS readings in importazole-treated cells with those in DMSO-treated cells. P-values were determined using the two-way ANOVA.
Caspase 3/7 Activity Assay
Apoptosis was determined using the caspase 3/7 activity assay as described previously (14, 47, 48). GBM cells and astrocytes were dissociated to single cells and plated at 500 or 4, 000 cells per well in 100 μl of culture media in a 96-well plate. Next day, cells were treated with either a 0.1% DMSO solution or 12.5 μM of importazole. After 3 days, caspase 3/7 activity assay reagent (Promega) was diluted in culture media at 1:1 and added to each well. After 1 h incubation, the luminescence of caspase 3/7 activity reagent was recorded using a microplate reader. Fold changes of caspase 3/7 activity were obtained by dividing luminescence readings in cells treated with importazole with those in cells treated with DMSO. P-values were determined using the student t-test.
Immunoblotting
Protein levels were determined using immunoblotting as described in detail previously (14, 47, 49, 50). Briefly, 25–50 μg of total protein was loaded onto an SDS-PAGE gel and then transferred onto a PVDF membrane. Antibodies were diluted as follows: anti-RAN antibody (1:500; Santa Cruz Biotechnology, Inc.), and anti-GAPDH antibody (1:200; Santa Cruz Biotechnology, Inc.).
Statistical Analyses
Significance of difference in means among different treatment groups was determined using either student t-test or two-way ANOVA. The software Prism 7 was used.
Results
Analysis of Loss-of Function Screens in GBM Cell Lines
As described earlier, Broad Institute has published drop-out RNAi screens in more than 500 cancer cell lines including 15 GBM cell lines. To identify survival genes from RNAi screens in 15 GBM cell lines, we followed the following criteria: (1) Candidate genes should be targeted by two different shRNAs; (2) The fold change of shRNA loss (log2) should be <−3.0 in every GBM cell line tested (Figure 1A, red line); and (3) The average fold change of shRNA loss (log2) across the 15 cell lines should be below −4.0 (Figure 1B, red line). From more than 50,000 shRNAs, we identified 10 survival genes in GBM.
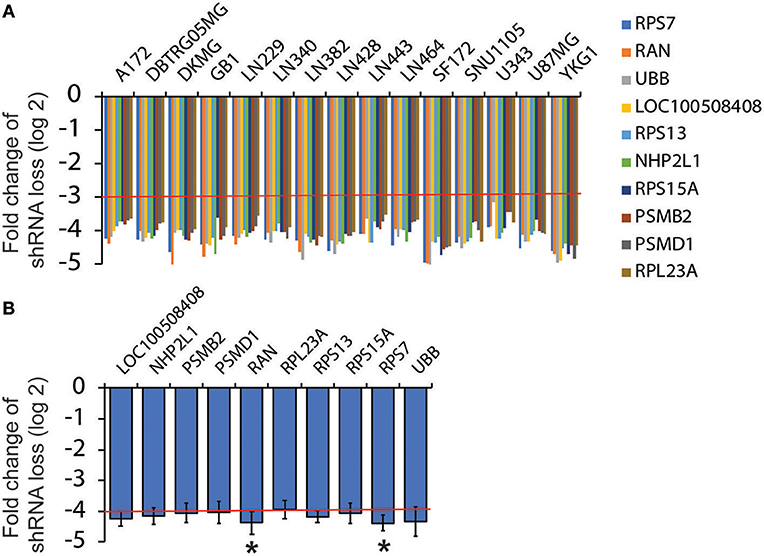
Figure 1. Analysis of RNAi screen results from Project Achilles. RNAi screen results were retrieved from Project Achilles. Candidate genes were selected based on the following criteria: (1) Genes are targeted by two or more different shRNAs; (2) The fold changes of shRNA loss (log2) are lower than −3; (3) The average fold changes of shRNA loss (log2) are lower than −4. Ten candidates were selected. The fold changes of shRNA loss (log2) in each GBM cell lines are shown in (A) and the average fold changes of shRNA loss are shown in (B). Red lines indicate cut-off numbers *P < 0.05.
Expression of Survival Genes in GBM
Because these genes are important for cell survival, it is likely that they are highly enriched in GBM. To test this possibility, we retrieved cDNA microarray data for GBM cell lines and astrocytes from the online database BioGPS (51–53). By comparing mRNA levels of candidate genes in GBM cell lines and in astrocytes, we found that levels of PSMB2, RPL23A, RPS13, and RPS15A in GBM were lower than those in astrocytes, whereas LOC100508408 was not detected in both GBM and astrocytes. In contrast, mRNA levels of NHP2L1, PSMD1, RAN, RPS7, and UBB in GBM cells were higher than those in astrocytes (Figure 2A, fold change >1.0 as indicated by the red line). Levels of RAN (RAN, member RAS oncogene family) in GBM were the highest among these candidates. We next inquired another online database, Oncomine (54, 55), where tissue microarray results were collected. In three different studies (Shai Brain, Murat Brain, and Brendel Brain 2), fold changes (GBM/normal brain) of RAN, PSMB2, RPS13, and RPS15A were >1 with P-values lower than 0.05 (Table 1). In contrast, levels of other candidate genes were not significantly high in GBM. To corroborate the above results, we measured protein levels of RAN in multiple GBM cell lines or primary GBM cells derived from patient specimens (14, 47) using immunoblotting (Figures 2B,C). RAN was detected in SF-295, U87MG, A172, U251, VTC-103, and VTC-058 cells (RAN/GAPDH >0.15; designated as RAN-positive cells), whereas LN-18, SF-268, T98G, VTC-002, VTC-004, VTC-037, VTC-056, VTC-084, and VTC-001 cells did not express RAN or expressed RAN at a very low level (RAN/GAPDH < 0.15; designated as RAN-negative cells). Our results validate the detectability of RAN protein levels in GBM and reveal variations in RAN protein levels amongst GBM cell lines. We have also shown that primary GBM cells proliferate at different rates [see Supplementary Data in (14) for details]. For example, VTC-002 and VTC-103 grew much faster than VTC-056. Intriguingly and consistent with our results shown in Figure 2C, RAN was detectable in VTC-103 and VTC-002, but not in VTC-056. These results suggest that GBM cells expressing RAN have a high-index of proliferation, indicative of a detectable activity of RAN.
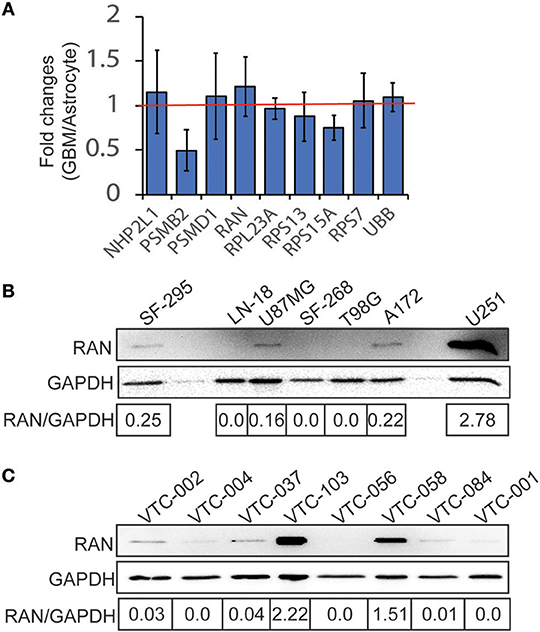
Figure 2. Expression of candidate genes in GBM. (A) cDNA microarray data were retrieved from the BioGPS database. The intensities of probes detecting candidate mRNAs in GBM cell lines were divided by those in astrocytes, yielding fold changes (GBM/Astrocyte). Fold changes of NHP2L1, PSMD1, RAN, RPS7, and UBB were above 1 (red line), suggesting that levels of these candidate mRNAs were high in GBM cell lines. Among these candidates, RAN levels in GBM are the highest. Error bars represent standard deviations from six GBM cell lines. (B) RAN protein levels in GBM cell lines. RAN and GAPDH proteins were detected in 7 GBM cell lines as indicated using immunoblotting. Band intensities were quantified using Image J. Fold changes (RAN/GAPDH) were obtained by dividing intensities of RAN with those of GAPDH. (C) RAN protein levels in primary GBM cells.
RAN and GBM Prognosis
The role of RAN in GBM has not yet been extensively explored. To address this, we tested the hypothesis that RAN, as a survival gene, correlates with GBM patient survival. By querying the TCGA GBM data using The Human Protein Atlas and the Glioblastoma Bio Discovery Portal, we found that RAN levels did not correlate with patient survival (Figure 3A, P = 0.909). We further looked into the correlation between RAN and the survival of GBM subtypes and found no statistically significant trend between RAN mRNA levels and the prognosis of classical, mesenchymal, or proneural GBM subtypes (Figures 3B–D, P = 0.427, 0.505, or 0.688, respectively).
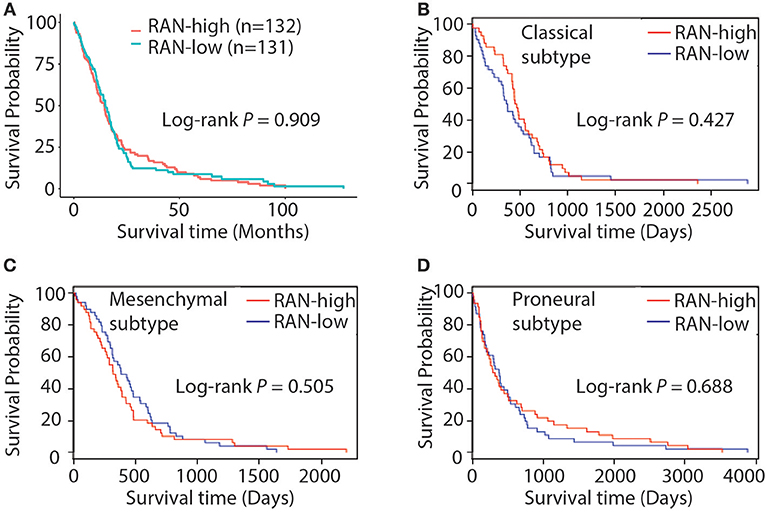
Figure 3. Kaplan-Meier analysis of RAN expression and GBM patient survival. (A) Survival curve of GBM patients with different levels of RAN. Results were retrieved from the GlioVis database. Survival curves of classical (B), mesenchymal (C), and proneural (D) GBM patients with different levels of RAN were retrieved from the Glioblastoma Bio Discovery Portal. Log-rank P-values are shown.
These results indicate that mRNA levels of RAN are not associated with GBM prognosis. However, given that nuclear transport is more active in cancer cells due to the high proliferation index (31, 33, 56), the activity of RAN and its functional partners may be more important for GBM survival. RAN regulates nuclear transportation through interacting with importin α, encoded by karyopherin subunit alpha 2 (KPNA2), and importin β1, encoded by karyopherin subunit beta 1 (KPNB1) (57–62). We therefore examined the levels of RAN together with KPNA2 and KPNB1 in GBM cell lines and tissues. Similar to RAN (Figure 4A), mRNA levels of KPNA2 (Figure 4B) and KPNB1 (Figure 4C) were elevated in LN-18, SF-268, SF-295, and U87MG cells. Linear regression analysis revealed that there was a strong trend between levels of RAN and KPNA2 (Figure 4D, R2 = 0.4798) and levels of RAN and KPNB1 (Figure 4E, R2 = 0.7591). Congruently, KPNA2 and KPNB1 were also enriched in GBM tissues compared to normal brain tissues (Table 1). In addition, results from the TCGA database showed that levels of RAN, KPNA2, or KPNB1 did not vary among different GBM subtypes (Figure S1).
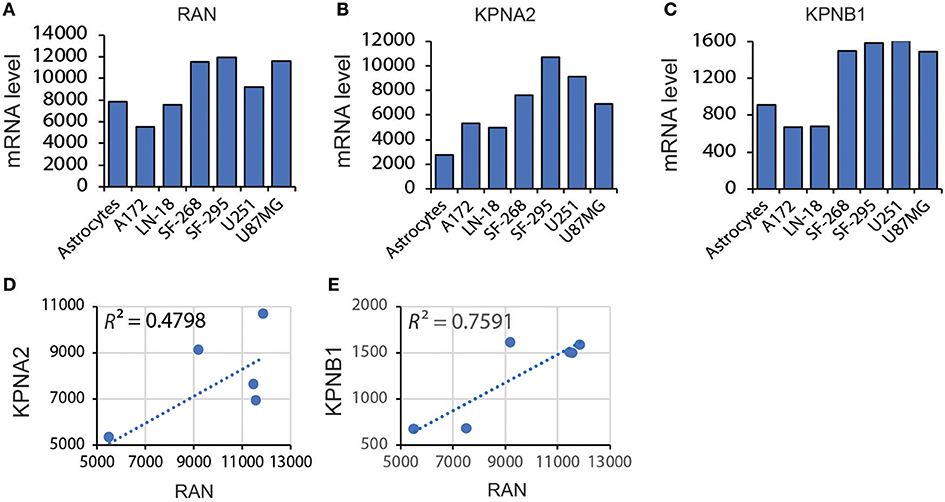
Figure 4. Correlation between levels of RAN and KPNA2 or KPNB1 in GBM. (A) Levels of RAN mRNA in astrocytes and GBM cell lines. Data were retrieved from the BioGPS database. The intensities of probes that detect RAN mRNA are shown. (B) KPNA2 mRNA levels in astrocytes and GBM cell lines. (C) KPNB1 mRNA levels in astrocytes and GBM cell lines. (D) Correlation between mRNA levels of RAN and KPNA2 in GBM cell lines. (E) Correlation between mRNA levels of RAN and KPNB1. A linear regression model was used. R square (R2) is the coefficient of determination.
Next, we determined the relationship between KPNA2 or KPNB1 and GBM prognosis. Based on the TCGA data analyzed using The Human Protein Atlas, we found that levels of KPNA2 (Figure 5A) or KPNB1 (Figure 5B) alone were not significantly correlated with patient survival (P = 0.134 and 0.106, respectively), consistent with the results for RAN (Figure 3A). Because levels of RAN and KPNB1 were more closely correlated with each other (Figure 4E), we next interrogated the relationship between levels of RAN and KPNB1 and GBM patient survival. The Cox univariate analysis showed that GBM patients with high levels of RAN or high levels of KPNB1 had a hazard ratio (HR, risk of death) of 0.942 or 1.031, respectively (Figure 5C and Table S1, panel RAN and KPNB1). In contrast, the HR of GBM patients with high levels of both RAN and KPNB1 increased to 1.315 with a P-value of 0.425 (Figure 5C and Table S1, panel RAN/KPNB1). To further understand whether this increase suggests a possible link between levels of RAN/KPNB1 and GBM prognosis, we introduced drug resistance into this study as a covariate. Temozolomide (TMZ) is a front-line chemo drug for GBM; however, patients often develop TMZ resistance due primarily to the consequences of promoter unmethylation of O-6-methylguanine DNA methyltransferase (MGMT), an enzyme that repairs TMZ-induced DNA damage (63–65). The poor prognosis of GBM patients is, therefore, closely associated with MGMT promoter methylation. Indeed, GBM patients with high levels of RAN often had an unmethylated MGMT promoter (Figure 5D). We therefore used TMZ treatment (TMZ) and/or MGMT promoter methylation as covariates in a Cox multivariate analysis model. When TMZ was used as a covariate, HRs of GBM patients with high levels of RAN, KPNB1, or RAN/KPNB1 were 1.167, 1.397, or 2.108 with a P-value of 0.545, 0.235, or 0.186, respectively (Figure 5C and Table S1, panel RAN+TMZ, KPNB1+TMZ, and RAN/KPNB1+TMZ). By adding MGMT promoter methylation (MGMT) as an additional covariate, HRs of GBM patients with high levels of RAN or high levels of KPNB1 were 1.502 or 1.380 with a P-value of 0.178 or 0.322 (Figure 5C and Table S1, panel RAN+TMZ+MGMT and KPNB1+TMZ+MGMT). In contrast, the HR of GBM patients with high levels of RAN and KPNB1 was elevated to 4.099 with a P-value of 0.042 (Figure 5C and Table S1, highlighted in red). These results indicate an inverse correlation, associated with MGMT-dependent TMZ resistance, between high levels of RAN and KPNB1 and poor prognosis of GBM patients. Our results together demonstrate that the activity, rather than the expression levels, of RAN is strongly linked to GBM patient survival.
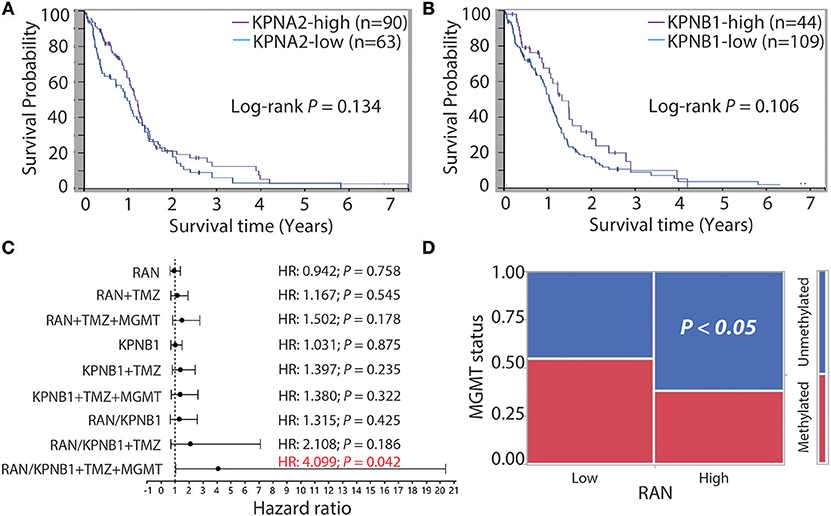
Figure 5. GBM patients with more RAN and KPNB1 exhibit MGMT-dependent TMZ resistance and have shorter life expectancies. Survival curves of GBM patients with different levels of KPNA2 (A) or patients with different levels of KPNB1 (B) were retrieved from the Human Protein Atlas. Log-rank P-values are shown. (C) Cox univariate and multivariate analyses of GBM patients with different levels of RAN and/or KPNB1. Data were retrieved from the TCGA database and re-analyzed using JMP software. Hazard ratios (HRs) that determine chances of death are shown. P-values indicate the statistical significance of HRs. TMZ treatment (TMZ) and promoter methylation status of MGMT (MGMT) were used as covariates. (D) MGMT promoter methylation in GBM patients expressing different levels of RAN. P < 0.05 indicates that GBM patients with high levels of RAN often have an unmethylated MGMT promoter.
Functional Blockade of RAN Using Importazole
The results shown above suggest that targeting RAN is a potentially appealing approach to impeding GBM disease progression. Our results also indicate that RAN activity in nuclear transport is important for GBM patient survival. We therefore chose importazole to test whether a blockade of RAN activity would inhibit GBM cell viability. We first treated astrocytes and GBM cell lines with 12.5 μM of importazole and monitored cell viability using the MTS cell viability assay. As shown in Figure 6A, importazole decreased the viability of the RAN-positive GBM cell lines A172, U87MG, U251, and SF-295 by >3-fold with a P < 0.0001, whereas the RAN-positive GBM cell lines LN-18, SF268, and T98G were much less sensitive to importazole (<3-fold) with a P < 0.001. Hence, the statistical analysis shows that the significance of importazole-induced growth inhibition is much stronger in RAN-positive cells (P < 0.0001) than in RAN-negative cells (P < 0.001). More importantly, importazole only decreased the viability of astrocytes by 15% with no statistical significance (P > 0.05). These results suggest that targeting RAN activity is an appealing approach with potentially low side effects. To corroborate these results, we treated RAN-positive or RAN-negative primary GBM cells with importazole at different doses. While both RAN-positive VTC-103 and RAN-negative VTC-037 cells showed a dose-dependent response, VTC-103 cells were more robustly sensitive to importazole than VTC-037 cells (Figure 6B; red line vs. blue line; P < 0.05), particularly when cells were treated with importazole at 12.5 or 25 μM. These results were consistent with those obtained from cell lines.
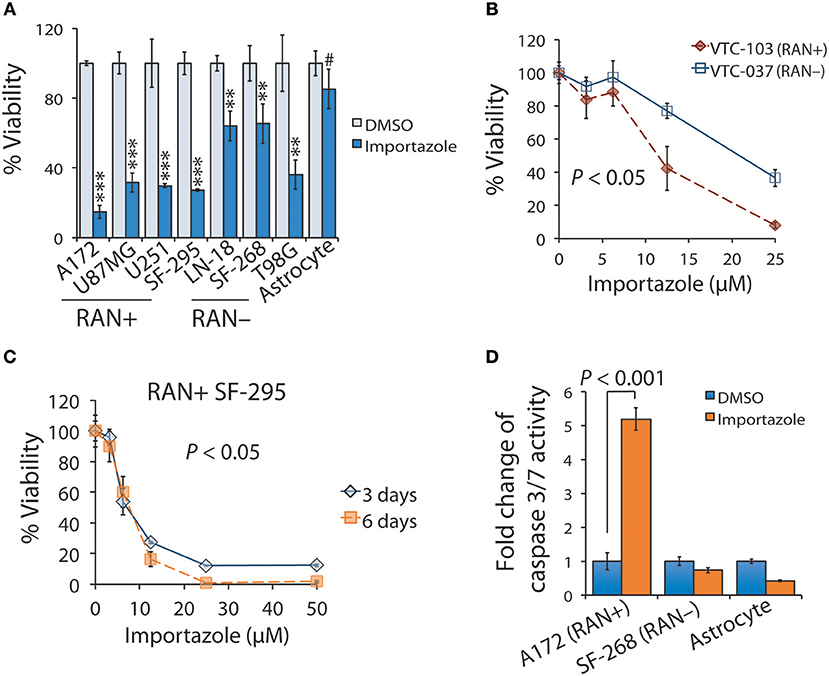
Figure 6. Functional blockade of RAN by importazole induces growth inhibition and activates apoptosis in RAN-expressing GBM cells. (A) Viability of GBM cells expressing different levels of RAN and astrocytes when treated with importazole. Cells were incubated with DMSO (light blue bars) or 12.5 μM importazole (dark blue bars) for 3 days. Cell viability was determined using the MTS viability assay. Percentages of viability were obtained by dividing the MTS absorbances of importazole-treated cells with those of DMSO-treated cells. RAN+: RAN-positive; RAN–: RAN-negative. Statistical significance between DMSO and importazole in each cell line was determined using a student t test. #P > 0.05; **P < 0.01; ***P < 0.001. (B) Viability of primary GBM cells when treated with importazole. Primary GBM cell lines VTC-103 (RAN+; red line) and VTC-037 (RAN–; blue line) were incubated with importazole at different concentrations ranging from 0 to 25 μM. P value that determines the statistical significance between responses of VTC-103 and VTC-037 to importazole at different doses was obtained using a two-way ANOVA analysis. (C) Viability of RAN+ SF-295 cells when treated with importazole at different time points. RAN+ SF-295 cells were treated with importazole at different doses ranging from 0 to 50 μM for 3 or 6 days. P-value that determines the statistical significance between different time points was obtained using a two-way ANOVA analysis. (D) Importazole-induced apoptosis in astrocytes and GBM cells expressing different levels of RAN. Cells were incubated with DMSO or 12.5 μM importazole for 3 days. Apoptosis was assessed using the caspase 3/7 activity assay. Fold changes of caspase 3/7 activity were obtained by dividing luminescence intensities of importazole-treated cells with those of DMSO-treated cells. P-value was obtained using the student t-test. Standard deviations (error bars) were derived from three independent experiments.
To determine whether importazole response is also time-dependent, we treated RAN-positive SF-295 with importazole at different doses and treatment lengths. We found that the cell viability of a 6-day treatment of importazole was lower than the cell viability of a 3-day treatment, particularly at high doses (Figure 6C). Two-way ANOVA analysis revealed a statistically significant difference between two time points (P < 0.05). Hence, the cytotoxic effect of importazole is also time-dependent. Finally, we tested whether the inhibition of cell viability by importazole is due primarily to cell death such as apoptosis. By using the caspase 3/7 activity assay, we found that importazole activated apoptosis, as manifested by the remarkable increase of caspase 3/7 activity in RAN-positive A172 cells, while failing to activate apoptosis in RAN-negative SF-268 cells and astrocytes (Figure 6D). Our results suggest that importazole induces apoptosis in RAN-expressing cells, thereby suppressing cell viability. Taken together, our results demonstrate that a functional blockade of RAN by importazole activates apoptosis in RAN-expressing GBM cells and suppresses GBM cell growth via a time/dose-dependent manner.
Discussion
In this report, we re-analyzed RNAi screen results from Project Achilles and identified RAN as an important survival factor for GBM. Our further investigation of GBM patient data revealed a robust correlation between levels of RAN/KPNB1 and GBM poor prognosis associated with MGMT-dependent TMZ resistance. Moreover, the application of importazole, an inhibitor of RAN/KPNB1 activity, substantially induced cell death and growth inhibition in RAN-expressing GBM cells. Based upon our results together with results from other research groups (31, 33, 56–62), we proposed a model illustrating the mode of action of RAN in glioblastoma (Figure 7). RAN and its partner KPNB1 regulate nuclear import of their cargos to promote glioblastoma cell survival and to induce drug resistance in patients (Figure 7, left panel). Importazole blocks interactions between RAN and KPNB1, thereby inhibiting nuclear import. The consequences of this blockade are the induction of cell death and inhibition of growth in glioblastoma (Figure 7, right panel).
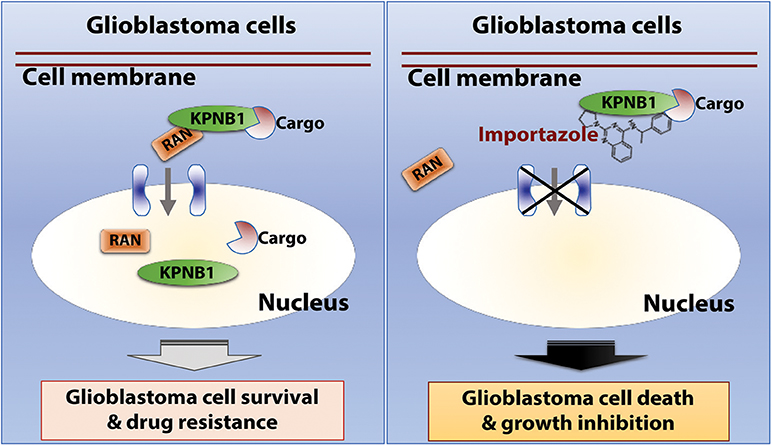
Figure 7. The model of action of RAN in glioblastoma. RAN and its partner KPNB1 regulate nuclear import to promote glioblastoma cell survival and to induce drug resistance in patients (Left). Importazole blocks interactions between RAN and KPNB1, thereby inhibiting nuclear import. The consequences of this blockade are induction of cell death and growth inhibition in glioblastoma (Right).
RAN GTPase and proteins involved in nuclear transport have been implicated in cancer progression, drug resistance, and cancer therapeutic development (30, 33, 38, 66). Deng et al., found that RAN was highly expressed in pancreatic cancers with high risk of metastasis (67). Furthermore, depletion of RAN substantially inhibited the migration of metastatic pancreatic cancer cells and the capability of these cells to metastasize to the liver. Congruently, ectopic expression of RAN activates PI3K/AKT signaling and promotes the invasive potential of non-small cell lung cancer cells (36). In a different study, Yuen et al. inactivated RAN in breast cancer cells and significantly increased the sensitivity of these cells to gefitinib (68). The role of RAN and nuclear transport mediated by RAN has not yet been widely explored in glioblastoma. In particular, whether RAN mediates TMZ resistance is not clear. Guvenc et al. examined the expression of RAN and survivin in primary GBM specimens and found that GBM patients with high levels of RAN and survivin were resistant to TMZ (40). They further developed a small chemical compound LLP-3 that disrupted the interaction between RAN and survivin. Incubation of TMZ-resistant GBM cells with LLP-3 diminished TMZ resistance.
These results are consistent with our findings presented above. Our results that demonstrate a strong link between high levels of RAN/KPNB1 and MGMT-dependent TMZ resistance (Figure 5C) are of particular interest. As we described earlier, TMZ is a front-line GBM treatment, but patients often become relapsed despite the reception of TMZ treatment due to the presence of MGMT proteins that repair TMZ-induced DNA damage (63, 69–78). Given that ~45% of GBM patients express MGMT (63), it is therefore critical to overcome MGMT-dependent TMZ resistance. Recent development of MGMT inhibitors has shown modest effect on restoring TMZ sensitivity in MGMT positive GBM patients (79–82). Our findings demonstrate that blocking the activity of RAN/KPNB1 is perhaps an effective approach to enhancing the responsiveness of GBM patients to TMZ, thereby providing a better and more promising therapeutic option for TMZ-resistant GBM patients.
Importazole has also been used in treating other cancers before. Multiple myeloma cell lines RPMI 8226 and NCI-H929 exhibited a strong response to importazole with a 50% inhibitory concentration (IC50) of 4.43 and 4.78 μM, respectively (45). As a comparison, importazole also displayed IC50s at similar range in GBM cell lines and primary tumor cells (Table S2). Given that most cancers, including GBM, demonstrate a hyper-dependency on nuclear transport (31, 44), a selective inhibition of RAN/KPNB1 activity by importazole may represent an innovative and effective treatment for GBM.
While our study unveils the crucial role of RAN in GBM cell survival, important questions remain to be addressed to establish that targeting RAN is an effective treatment option for GBM, particularly those with TMZ resistance. Future studies will reveal whether RAN is a biomarker that predicts MGMT-dependent TMZ resistance in GBM, elucidate how RAN contributes to TMZ resistance, and determine whether importazole or functional blockade of RAN circumvents TMZ resistance and inhibits GBM progression.
Author Contributions
RTV, SL, and ZS conceived the project and wrote the manuscript. KLS and KJP performed all experiments.
Funding
This work was supported by a research fund from the Roanoke Valley Governor School to KLS and SL.
Conflict of Interest Statement
The authors declare that the research was conducted in the absence of any commercial or financial relationships that could be construed as a potential conflict of interest.
Supplementary Material
The Supplementary Material for this article can be found online at: https://www.frontiersin.org/articles/10.3389/fonc.2018.00662/full#supplementary-material
References
1. Ostrom QT, Gittleman H, Xu J, Kromer C, Wolinsky Y, Kruchko C, et al. CBTRUS statistical report: primary brain and other central nervous system tumors diagnosed in the United States in 2009-2013. Neuro Oncol. (2016) 18(Suppl. 5):v1–75. doi: 10.1093/neuonc/now207
2. Siegel RL, Miller KD, Jemal A. Cancer statistics, 2018. CA Cancer J Clin. (2018) 68:7–30. doi: 10.3322/caac.21442
3. Wick W, Osswald M, Wick A, Winkler F. Treatment of glioblastoma in adults. Ther Adv Neurol Disord. (2018) 11:1–13. doi: 10.1177/1756286418790452
4. Mann J, Ramakrishna R, Magge R, Wernicke AG. Advances in radiotherapy for glioblastoma. Front Neurol. (2017) 8:748. doi: 10.3389/fneur.2017.00748
5. Ostrom QT, Bauchet L, Davis FG, Deltour I, Fisher JL, Langer CE, et al. The epidemiology of glioma in adults: a “state of the science” review. Neuro Oncol. (2014) 16:896–913. doi: 10.1093/neuonc/nou087
6. Barney C, Shukla G, Bhamidipati D, Palmer JD. Re-irradiation for recurrent glioblastoma multiforme. Chin Clin Oncol. (2017) 6:36. doi: 10.21037/cco.2017.06.18
7. Varghese RT, Liang Y, Guan T, Franck CT, Kelly DF, Sheng Z. Survival kinase genes present prognostic significance in glioblastoma. Oncotarget (2016) 7:20140–51. doi: 10.18632/oncotarget.7917
8. Steffens R, Semrau S, Lahmer G, Putz F, Lettmaier S, Eyupoglu I, et al. Recurrent glioblastoma: who receives tumor specific treatment and how often? J Neurooncol. (2016) 128:85–92. doi: 10.1007/s11060-016-2079-z
9. Kim HR, Kim KH, Kong DS, Seol HJ, Nam DH, Lim do H, et al. Outcome of salvage treatment for recurrent glioblastoma. J Clin Neurosci. (2015) 22:468–73. doi: 10.1016/j.jocn.2014.09.018
10. Sheng Z, Murphy SF, Guo S, Green MR. A diphtheria toxin negative selection in RNA interference screening. Methods Mol Biol. (2014) 1176:59–72. doi: 10.1007/978-1-4939-0992-6_6
11. Sheng Z, Li L, Zhu LJ, Smith TW, Demers A, Ross AH, et al. A genome-wide RNA interference screen reveals an essential CREB3L2-ATF5-MCL1 survival pathway in malignant glioma with therapeutic implications. Nat Med. (2010) 16:671–7. doi: 10.1038/nm.2158
12. Den RB, Kamrava M, Sheng Z, Werner-Wasik M, Dougherty E, Marinucchi M, et al. A phase I study of the combination of sorafenib with temozolomide and radiation therapy for the treatment of primary and recurrent high-grade gliomas. Int J Radiat Oncol Biol Phys. (2013) 85:321–8. doi: 10.1016/j.ijrobp.2012.04.017
13. Varghese RT, Young S, Pham L. Liang Y, Pridham KJ, Guo S, et al. Casein Kinase 1 epsilon regulates glioblastoma cell survival. Sci Rep. (2018) 8:13621. doi: 10.1038/s41598-018-31864-x
14. Pridham KJ, Le L, Guo S, Varghese RT, Algino S, Liang Y, et al. PIK3CB/p110beta is a selective survival factor for glioblastoma. Neuro Oncol. (2018) 20:494–505. doi: 10.1093/neuonc/nox181
15. Pridham KJ, Varghese RT, Sheng Z. The role of class IA phosphatidylinositol-4,5-bisphosphate 3-kinase catalytic subunits in glioblastoma. Front Oncol. (2017) 7:312. doi: 10.3389/fonc.2017.00312
16. Goidts V, Bageritz J, Puccio L, Nakata S, Zapatka M, Barbus S, et al. RNAi screening in glioma stem-like cells identifies PFKFB4 as a key molecule important for cancer cell survival. Oncogene (2012) 31:3235–43. doi: 10.1038/onc.2011.490
17. Tandle AT, Kramp T, Kil WJ, Halthore A, Gehlhaus K, Shankavaram U, et al. Inhibition of polo-like kinase 1 in glioblastoma multiforme induces mitotic catastrophe and enhances radiosensitisation. Eur J Cancer (2013) 49:3020–8. doi: 10.1016/j.ejca.2013.05.013
18. Sa JK, Yoon Y, Kim M, Kim Y, Cho HJ, Lee JK, et al. In vivo RNAi screen identifies NLK as a negative regulator of mesenchymal activity in glioblastoma. Oncotarget (2015) 6:20145–59. doi: 10.18632/oncotarget.3980
19. Kulkarni S, Goel-Bhattacharya S, Sengupta S, Cochran BH. A large-scale RNAi screen identifies SGK1 as a key survival kinase for GBM stem cells. Mol Cancer Res. (2018) 16:103–14. doi: 10.1158/1541-7786.MCR-17-0146
20. Cowley GS, Weir BA, Vazquez F, Tamayo P, Scott JA, Rusin S, et al. Parallel genome-scale loss of function screens in 216 cancer cell lines for the identification of context-specific genetic dependencies. Sci Data (2014) 1:140035. doi: 10.1038/sdata.2014.35
21. Aguirre AJ, Meyers RM, Weir BA, Vazquez F, Zhang CZ, Ben-David U, et al. Genomic copy number dictates a gene-independent cell response to CRISPR/Cas9 targeting. Cancer Discov. (2016) 6:914–29. doi: 10.1158/2159-8290.CD-16-0154
22. Meyers RM, Bryan JG, McFarland JM, Weir BA, Sizemore AE, Xu H, et al. Computational correction of copy number effect improves specificity of CRISPR-Cas9 essentiality screens in cancer cells. Nat Genet. (2017) 49:1779–84. doi: 10.1038/ng.3984
23. Tsherniak A, Vazquez F, Montgomery PG, Weir BA, Kryukov G, Cowley GS, et al. Defining a cancer dependency map. Cell (2017) 170:564–76.e16. doi: 10.1016/j.cell.2017.06.010
24. Cavazza T, Vernos I. The RanGTP pathway: from nucleo-cytoplasmic transport to spindle assembly and beyond. Front Cell Dev Biol. (2015) 3:82. doi: 10.3389/fcell.2015.00082
25. Khuperkar D, Helen M, Magre I, Joseph J. Inter-cellular transport of ran GTPase. PLoS ONE (2015) 10:e0125506. doi: 10.1371/journal.pone.0125506
26. Lavia P. The GTPase RAN regulates multiple steps of the centrosome life cycle. Chromosome Res. (2016) 24:53–65. doi: 10.1007/s10577-015-9514-4
27. Matsuura Y. Mechanistic insights from structural analyses of Ran-GTPase-driven nuclear export of proteins and RNAs. J Mol Biol. (2016) 428(10 Pt A):2025–39. doi: 10.1016/j.jmb.2015.09.025
28. Vaidyanathan S, Thangavelu PU, Duijf PH. Overexpression of Ran GTPase components regulating nuclear export, but not mitotic spindle assembly, marks chromosome instability and poor prognosis in breast cancer. Target Oncol. (2016) 11:677–86. doi: 10.1007/s11523-016-0432-y
29. Nagai M, Yoneda Y. Small GTPase Ran and Ran-binding proteins. Biomol Concepts (2012) 3:307–18. doi: 10.1515/bmc-2011-0068
30. Sanderson HS, Clarke PR. Cell biology: Ran, mitosis and the cancer connection. Curr Biol. (2006) 16:R466–8. doi: 10.1016/j.cub.2006.05.032
31. Xia F, Lee CW, Altieri DC. Tumor cell dependence on Ran-GTP-directed mitosis. Cancer Res. (2008) 68:1826–33. doi: 10.1158/0008-5472.CAN-07-5279
32. Barres V, Ouellet V, Lafontaine J, Tonin PN, Provencher DM, Mes-Masson AM. An essential role for Ran GTPase in epithelial ovarian cancer cell survival. Mol Cancer (2010) 9:272. doi: 10.1186/1476-4598-9-272
33. Doherty KJ, McKay C, Chan KK, El-Tanani MK. RAN GTPase as a target for cancer therapy: Ran binding proteins. Curr Mol Med. (2011) 11:686–95. doi: 10.2174/156652411797536688
34. Yuen HF, Chan KK, Grills C, Murray JT, Platt-Higgins A, Eldin OS, et al. Ran is a potential therapeutic target for cancer cells with molecular changes associated with activation of the PI3K/Akt/mTORC1 and Ras/MEK/ERK pathways. Clin Cancer Res. (2012) 18:380–91. doi: 10.1158/1078-0432.CCR-11-2035
35. Deng L, Lu Y, Zhao X, Sun Y, Shi Y, Fan H, et al. Ran GTPase protein promotes human pancreatic cancer proliferation by deregulating the expression of Survivin and cell cycle proteins. Biochem Biophys Res Commun. (2013) 440:322–9. doi: 10.1016/j.bbrc.2013.09.079
36. Ning J, Liu W, Zhang J, Lang Y, Xu S. Ran GTPase induces EMT and enhances invasion in non-small cell lung cancer cells through activation of PI3K-AKT pathway. Oncol Res. (2013) 21:67–72. doi: 10.3727/096504013X13747716581417
37. Saxena S, Gandhi A, Lim PW, Relles D, Sarosiek K, Kang C, et al. RAN GTPase and osteopontin in pancreatic cancer. Pancreat Disord Ther. (2013) 3:113. doi: 10.4172/2165-7092.1000113
38. Matchett KB, McFarlane S, Hamilton SE, Eltuhamy YS, Davidson MA, Murray JT, et al. Ran GTPase in nuclear envelope formation and cancer metastasis. Adv Exp Med Biol. (2014) 773:323–51. doi: 10.1007/978-1-4899-8032-8_15
39. Woo IS, Jang HS, Eun SY, Kim HJ, Ham SA, Kim HJ, et al. Ran suppresses paclitaxel-induced apoptosis in human glioblastoma cells. Apoptosis (2008) 13:1223–31. doi: 10.1007/s10495-008-0247-0
40. Guvenc H, Pavlyukov MS, Joshi K, Kurt H, Banasavadi-Siddegowda YK, Mao P, et al. Impairment of glioma stem cell survival and growth by a novel inhibitor for Survivin-Ran protein complex. Clin Cancer Res. (2013) 19:631–42. doi: 10.1158/1078-0432.CCR-12-0647
41. Haggag YA, Matchett KB, Dakir El H, Buchanan P, Osman MA, Elgizawy SA, et al. Nano-encapsulation of a novel anti-Ran-GTPase peptide for blockade of regulator of chromosome condensation 1 (RCC1) function in MDA-MB-231 breast cancer cells. Int J Pharm. (2017) 521:40–53. doi: 10.1016/j.ijpharm.2017.02.006
42. Soderholm JF, Bird SL, Kalab P, Sampathkumar Y, Hasegawa K, Uehara-Bingen M, et al. Importazole, a small molecule inhibitor of the transport receptor importin-beta. ACS Chem Biol. (2011) 6:700–8. doi: 10.1021/cb2000296
43. Kodama M, Kodama T, Newberg JY, Katayama H, Kobayashi M, Hanash SM, et al. In vivo loss-of-function screens identify KPNB1 as a new druggable oncogene in epithelial ovarian cancer. Proc Natl Acad Sci USA. (2017) 114:E7301–10. doi: 10.1073/pnas.1705441114
44. Kuusisto HV, Jans DA. Hyper-dependence of breast cancer cell types on the nuclear transporter Importin beta1. Biochim Biophys Acta. (2015) 1853:1870–8. doi: 10.1016/j.bbamcr.2015.05.002
45. Yan WQ, Du J, Jiang H, Hou J. [Effect of nuclear receptor inhibitor importazole on the proliferation and apoptosis of multiple myeloma cells]. Zhonghua Xue Ye Xue Za Zhi. (2013) 34:323–6. doi: 10.3760/cma.j.issn.0253-2727.2013.04.016
46. van der Watt PJ, Chi A, Stelma T, Stowell C, Strydom E, Carden S, et al. Targeting the nuclear import receptor Kpnbeta1 as an anticancer therapeutic. Mol Cancer Ther. (2016) 15:560–73. doi: 10.1158/1535-7163.MCT-15-0052
47. Kanabur P, Guo S, Simonds GR, Kelly DF, Gourdie RG, Verbridge SS, et al. Patient-derived glioblastoma stem cells respond differentially to targeted therapies. Oncotarget (2016) 7:86406–19. doi: 10.18632/oncotarget.13415
48. Murphy SF, Varghese RT, Lamouille S, Guo S, Pridham KJ, Kanabur P, et al. Connexin 43 inhibition sensitizes chemoresistant glioblastoma cells to temozolomide. Cancer Res. (2016) 76:139–49. doi: 10.1158/0008-5472.CAN-15-1286
49. Guo S, Liang Y, Murphy SF, Huang A, Shen H, Kelly DF, et al. A rapid and high content assay that measures cyto-ID-stained autophagic compartments and estimates autophagy flux with potential clinical applications. Autophagy (2015) 11:560–72. doi: 10.1080/15548627.2015.1017181
50. Guo S, Pridham KJ, Virbasius CM, He B, Zhang L, Varmark H, et al. A large-scale RNA interference screen identifies genes that regulate autophagy at different stages. Sci Rep. (2018) 8:2822. doi: 10.1038/s41598-018-21106-5
51. Wu C, Jin X, Tsueng G, Afrasiabi C, Su AI. BioGPS: building your own mash-up of gene annotations and expression profiles. Nucleic Acids Res. (2016) 44:D313–6. doi: 10.1093/nar/gkv1104
52. Wu C, Macleod I, Su AI. BioGPS and MyGene.info: organizing online, gene-centric information. Nucleic Acids Res. (2013) 41:D561–5. doi: 10.1093/nar/gks1114
53. Wu C, Orozco C, Boyer J, Leglise M, Goodale J, Batalov S, et al. BioGPS: an extensible and customizable portal for querying and organizing gene annotation resources. Genome Biol. (2009) 10:R130. doi: 10.1186/gb-2009-10-11-r130
54. Rhodes DR, Kalyana-Sundaram S, Mahavisno V, Varambally R, Yu J, Briggs BB, et al. Oncomine 3.0: genes, pathways, and networks in a collection of 18,000 cancer gene expression profiles. Neoplasia (2007) 9:166–80. doi: 10.1593/neo.07112
55. Rhodes DR, Yu J, Shanker K, Deshpande N, Varambally R, Ghosh D, et al. ONCOMINE: a cancer microarray database and integrated data-mining platform. Neoplasia (2004) 6:1–6. doi: 10.1016/S1476-5586(04)80047-2
56. Ly TK, Wang J, Pereira R, Rojas KS, Peng X, Feng Q, et al. Activation of the Ran GTPase is subject to growth factor regulation and can give rise to cellular transformation. J Biol Chem. (2010) 285:5815–26. doi: 10.1074/jbc.M109.071886
57. Lindsay ME, Plafker K, Smith AE, Clurman BE, Macara IG. Npap60/Nup50 is a tri-stable switch that stimulates importin-alpha:beta-mediated nuclear protein import. Cell (2002) 110:349–60. doi: 10.1016/S0092-8674(02)00836-X
58. Nevo R, Stroh C, Kienberger F, Kaftan D, Brumfeld V, Elbaum M, et al. A molecular switch between alternative conformational states in the complex of Ran and importin beta1. Nat Struct Biol. (2003) 10:553–7. doi: 10.1038/nsb940
59. Cimica V, Chen HC, Iyer JK, Reich NC. Dynamics of the STAT3 transcription factor: nuclear import dependent on Ran and importin-beta1. PLoS ONE (2011) 6:e20188. doi: 10.1371/journal.pone.0020188
60. Kose S, Imamoto N, Tachibana T, Yoshida M, Yoneda Y. beta-subunit of nuclear pore-targeting complex (importin-beta) can be exported from the nucleus in a Ran-independent manner. J Biol Chem. (1999) 274:3946–52. doi: 10.1074/jbc.274.7.3946
61. Liang P, Zhang H, Wang G, Li S, Cong S, Luo Y, et al. KPNB1, XPO7 and IPO8 mediate the translocation ofNF-kappaB/p65 into the nucleus. Traffic (2013) 14:1132–43. doi: 10.1111/tra.12097
62. Lieu KG, Shim EH, Wang J, Lokareddy RK, Tao T, Cingolani G, et al. The p53-induced factor Ei24 inhibits nuclear import through an importin beta-binding-like domain. J Cell Biol. (2014) 205:301–12. doi: 10.1083/jcb.201304055
63. Hegi ME, Diserens AC, Gorlia T, Hamou MF, de Tribolet N, Weller M, et al. MGMT gene silencing and benefit from temozolomide in glioblastoma. N Engl J Med. (2005) 352:997–1003. doi: 10.1056/NEJMoa043331
64. Hegi ME, Liu L, Herman JG, Stupp R, Wick W, Weller M, et al. Correlation of O6-methylguanine methyltransferase (MGMT) promoter methylation with clinical outcomes in glioblastoma and clinical strategies to modulate MGMT activity. J Clin Oncol. (2008) 26:4189–99. doi: 10.1200/JCO.2007.11.5964
65. Stupp R, Mason WP, van den Bent MJ, Weller M, Fisher B, Taphoorn MJ, et al. Radiotherapy plus concomitant and adjuvant temozolomide for glioblastoma. N Engl J Med. (2005) 352:987–96. doi: 10.1056/NEJMoa043330
66. Hill R, Cautain B, de Pedro N, Link W. Targeting nucleocytoplasmic transport in cancer therapy. Oncotarget (2014) 5:11–28. doi: 10.18632/oncotarget.1457
67. Deng L, Shang Y, Guo S, Liu C, Zhou L, Sun Y, et al. Ran GTPase protein promotes metastasis and invasion in pancreatic cancer by deregulating the expression of AR and CXCR4. Cancer Biol Ther. (2014) 15:1087–93. doi: 10.4161/cbt.29217
68. Yuen HF, Chan KK, Platt-Higgins A, Dakir el H, Matchett KB, Haggag YA, et al. Ran GTPase promotes cancer progression via Met recepto-rmediated downstream signaling. Oncotarget (2016) 7:75854–64. doi: 10.18632/oncotarget.12420
69. Gunther W, Pawlak E, Damasceno R, Arnold H, Terzis AJ. Temozolomide induces apoptosis and senescence in glioma cells cultured as multicellular spheroids. Br J Cancer (2003) 88:463–9. doi: 10.1038/sj.bjc.6600711
70. Plowman J, Waud WR, Koutsoukos AD, Rubinstein LV, Moore TD, Grever MR. Preclinical antitumor activity of temozolomide in mice: efficacy against human brain tumor xenografts and synergism with 1,3-bis(2-chloroethyl)-1-nitrosourea. Cancer Res. (1994) 54:3793–9.
71. Roos WP, Batista LF, Naumann SC, Wick W, Weller M, Menck CF, et al. Apoptosis in malignant glioma cells triggered by the temozolomide-induced DNA lesion O6-methylguanine. Oncogene (2007) 26:186–97. doi: 10.1038/sj.onc.1209785
72. Chahal M, Xu Y, Lesniak D, Graham K, Famulski K, Christensen JG, et al. MGMT modulates glioblastoma angiogenesis and response to the tyrosine kinase inhibitor sunitinib. Neuro Oncol. (2010) 12:822–33. doi: 10.1093/neuonc/noq017
73. Villalva C, Cortes U, Wager M, Tourani JM, Rivet P, Marquant C, et al. O6-Methylguanine-Methyltransferase (MGMT) promoter methylation status in glioma stem-like cells is correlated to temozolomide sensitivity under differentiation-promoting conditions. Int J Mol Sci. (2012) 13:6983–94. doi: 10.3390/ijms13066983
74. Kokkinakis DM, Bocangel DB, Schold SC, Moschel RC, Pegg AE. Thresholds of O6-alkylguanine-DNA alkyltransferase which confer significant resistance of human glial tumor xenografts to treatment with 1,3-bis(2-chloroethyl)-1-nitrosourea or temozolomide. Clin Cancer Res. (2001) 7:421–8.
75. Donson AM, Addo-Yobo SO, Handler MH, Gore L, Foreman NK. MGMT promoter methylation correlates with survival benefit and sensitivity to temozolomide in pediatric glioblastoma. Pediatr Blood Cancer (2007) 48:403–7. doi: 10.1002/pbc.20803
76. Wiewrodt D, Nagel G, Dreimuller N, Hundsberger T, Perneczky A, Kaina B. MGMT in primary and recurrent human glioblastomas after radiation and chemotherapy and comparison with p53 status and clinical outcome. Int J Cancer (2008) 122:1391–9. doi: 10.1002/ijc.23219
77. Kreth S, Thon N, Eigenbrod S, Lutz J, Ledderose C, Egensperger R, et al. O-methylguanine-DNA methyltransferase (MGMT) mRNA expression predicts outcome in malignant glioma independent of MGMT promoter methylation. PLoS ONE (2011) 6:e17156. doi: 10.1371/journal.pone.0017156
78. Silber JR, Bobola MS, Blank A, Chamberlain MC. O(6)-Methylguanine-DNA methyltransferase in glioma therapy: promise and problems. Biochim Biophys Acta (2012) 1826:71–82. doi: 10.1016/j.bbcan.2011.12.004
79. Wedge SR, Porteus JK, May BL, Newlands ES. Potentiation of temozolomide and BCNU cytotoxicity by O(6)-benzylguanine: a comparative study in vitro. Br J Cancer (1996) 73:482–90. doi: 10.1038/bjc.1996.85
80. Griffin RJ, Arris CE, Bleasdale C, Boyle FT, Calvert AH, Curtin NJ, et al. Resistance-modifying agents. 8. Inhibition of O(6)-alkylguanine-DNA alkyltransferase by O(6)-alkenyl-, O(6)-cycloalkenyl-, and O(6)-(2-oxoalkyl)guanines and potentiation of temozolomide cytotoxicity in vitro by O(6)-(1-cyclopentenylmethyl)guanine. J Med Chem. (2000) 43:4071–83. doi: 10.1021/jm000961o
81. Quinn JA, Desjardins A, Weingart J, Brem H, Dolan ME, Delaney SM, et al. Phase I trial of temozolomide plus O6-benzylguanine for patients with recurrent or progressive malignant glioma. J Clin Oncol. (2005) 23:7178–87. doi: 10.1200/JCO.2005.06.502
Keywords: RAN, glioblastoma, importazole, cell survival, glioblastoma prognosis, KPNB1, glioblastoma treatment
Citation: Sheng KL, Pridham KJ, Sheng Z, Lamouille S and Varghese RT (2019) Functional Blockade of Small GTPase RAN Inhibits Glioblastoma Cell Viability. Front. Oncol. 8:662. doi: 10.3389/fonc.2018.00662
Received: 03 September 2018; Accepted: 13 December 2018;
Published: 08 January 2019.
Edited by:
Shiv K. Gupta, Mayo Clinic, United StatesReviewed by:
Fabrizio Martelli, Istituto Superiore di Sanità (ISS), ItalyThomas Daubon, Institut National de la Santé et de la Recherche Médicale (INSERM), France
Copyright © 2019 Sheng, Pridham, Sheng, Lamouille and Varghese. This is an open-access article distributed under the terms of the Creative Commons Attribution License (CC BY). The use, distribution or reproduction in other forums is permitted, provided the original author(s) and the copyright owner(s) are credited and that the original publication in this journal is cited, in accordance with accepted academic practice. No use, distribution or reproduction is permitted which does not comply with these terms.
*Correspondence: Zhi Sheng, emhpc2hlbmdAdnRjLnZ0LmVkdQ==
Samy Lamouille, bGFtb3VpbHNAdnRjLnZ0LmVkdQ==
Robin T. Varghese, cnZhcmdoZXNlQHZjb20udnQuZWR1
†These authors have contributed equally to this work