- 1Hematology Division, University of Pisa, Pisa, Italy
- 2Department of Medical Biotechnologies, University of Siena, Siena, Italy
- 3Department of Surgical, Medical and Molecular Pathology and Critical Care, University of Pisa, Pisa, Italy
- 4Department of Clinical and Experimental Medicine, University of Pisa, Pisa, Italy
- 5National Research Council of Italy, Milan, Italy
- 6Department of Clinical and Experimental Medicine, Pharmacology Division, University of Pisa, Pisa, Italy
- 7Department of Clinical and Experimental Medicine, Rheumatology Division, University of Pisa, Pisa, Italy
Notwithstanding the introduction of Tyrosine Kinase Inhibitors (TKIs) revolutionized the outcome of Chronic Myeloid Leukemia (CML), one third of patients still suspends treatment for failure response. Recent research demonstrated that several BCR/ABL1-independent mechanisms can sustain resistance, but the relationship between these mechanisms and the outcome has not yet been fully understood. This study was designed to evaluate in a “real-life” setting if a change of expression of several genes involved in the WNT/BETA-CATENIN, JAK-STAT, and POLYCOMB pathways might condition the outcome of CML patients receiving TKIs. Thus, the expression of 255 genes, related to the aforementioned pathways, was measured by quantitative PCR after 6 months of therapy and compared with levels observed at diagnosis in 11 CML patients, in order to find possible correlations with quality of response to treatment and event-free-survival (EFS). These results were then re-analyzed by the principal component method (PCA) for tempting to better cluster resistant cases. After 12 months of therapy, 6 patients achieved an optimal response and 5 were “resistant;” after application of both statistical methods, it was evident that in all pathways a significant overall up-regulation occurred, and that WNT was the pathway mostly responsible for the TKIs resistance. Indeed, 100% of patients with a “low” up-regulation of this pathway achieved an optimal response vs. 33% of those who showed a “high” gene over-expression (p = 0.016). Analogously, the 24-months EFS resulted significantly influenced by the degree of up-regulation of the WNT signaling: all patients with a “low” up-regulation were event-free vs. 33% of those who presented a “high” gene expression (p = 0.05). In particular, the PCA analysis confirmed the role of WNT pathway and showed that the most significantly up-regulated genes with negative prognostic value were DKK, WNT6, WISP1, and FZD8. In conclusion, our results sustain the need of a wide and multitasking approach in order to understand the resistance mechanisms in CML.
Introduction
Chronic myeloid leukemia (CML) is a clonal myeloproliferative disorder originated from hematopoietic stem cell progenitors characterized by the presence of the Philadelphia chromosome (Ph) and the BCR/ABL1 fusion gene. The BCR/ABL1 oncoprotein is a constitutively active tyrosine kinase that promotes the activation of different transduction pathways involved in cell growth and differentiation (RAS, RAF, JUN, MYC, STAT, AKT), with the consequent transformation of the hematopoietic stem cell in a neoplastic clone. Ph'+ cells are genetically unstable and prone to have multiple heterogeneous genetic aberrations that could induce the transformation into acute leukemia, leading to the transformation from one chronic disease to an accelerated or a blastic phase (1).
A crucial event in this transition seems to be the acquisition of mutations in the kinase domain of the ABL1 gene, with the consequent resistance to the tyrosine kinase inhibitors (TKIs). Resistance, during therapy, is frequently linked to the inability of TKIs to arrange in the ATPase pocket of the fusion protein due to point mutations in the ATP-binding task. About 4% of failing cases presents ABL1 mutations, even at the low level (sub-clonal), detectable only by NGS whose sensitivity is higher than offered by the Sanger sequencing (2). The T315I mutation confers resistance to the TKIs imatinb, dasatinib, nilotinib, and bosutinib, whereas it is sensitive to ponatinib (3). Nevertheless, combination with other mutations (compound mutations), also ponatinib is ineffective, and other therapeutical approaches are necessary, such as asciminib (4).
For the majority of cases where the resistance to TKIs is not related to the ABL1 mutations different mechanisms have been already hypothesized:
1) The protective action exerted by the bone marrow niche, where hypoxia and glucose deprivation block the production of the fusion protein in the CML leukemic stem cell (LSC), with the consequent ineffective action of TKIs that cannot reach their target (5, 6);
2) An aberrant expression of some genes involved in the epigenetic control, such as those belonging to the Polycomb family; for example, over-expression of BMI1 resulted to be a negative prognostic molecular marker in terms of event-free survival (EFS) (7). The negative role of BMI1 oncogenic protein can be suggested by the fact that it is co-localized in the cytoplasm of LSC with the BCR-ABL1 P210 protein and with CD26 (8), the dipeptidil peptidase IV (DPPIV) that has been shown to represent a specific marker of CML progenitors (9, 10);
3) The hyper-activation of the JAK-STAT signaling. This pathway is involved in the transduction of signals mediated by cytokines, interferon and growth factors, with consequent support of neoplastic cell growth and invasion in many types of cancer. Additional implications in inflammation and immunity have been also recently attributed to this pathway, thus supporting the idea that it could sustain the CML LSC maintenance (11, 12): indeed, the persistent STAT3 activation seems to confer resistance to TKIs by controlling the LSC self-renewal and by favoring its hiding in the bone marrow niche. Inhibition of this pathway might represent a potential way to ameliorate the molecular response in warning or failed CML patients or to sustain deep molecular responses in cases tempting the discontinuation of therapy (13).
4) The WNT/Beta-catenin pathway, that is necessary to the self-renewal of normal cells, but whose deregulation causes leukemogenesis and progression in several neoplasias (14) seems to be relevant in CML also. After linking to its receptors, WNT ligand induces the inhibition of beta-catenin phosphorylation and its cytoplasmic accumulation, with consequent activation of transcription and expansion of the leukemic progenitors. LSCs have aberrant regulation of beta-catenin, and in a CML murine model BCR/ABL1 seems to stimulate beta-catenin through the phosphoinositide 3-kinase (PI3K/AKT) signaling, then increasing the leukemic progression (15).
5) Polymorphisms of several transmembrane drug transporters. They can be predictive of response or intolerance in CML patients: indeed, it has been demonstrated that single nucleotide polymorphisms (SNP) of hOCT1, ABCB1, and ABCG2 conditioned the imatinib pharmacokinetics and disposition whereas they did not impact on Nilotinib efficacy or toxicity (16–20). This could be relevant in the decision of which kind of TKI has to be prescribed in the first line.
In this complex scenario, we decided to investigate the expression levels of some genes reported to sustain the BCR-ABL1-independent resistance in CML: in particular, we focused on JAK-STAT, WNT/beta-catenin, and Polycomb pathways, evaluating their de-regulation after 6 months of treatment from diagnosis. In addition to the “classical” supervised statistical analysis, we adopted also The Principal Component Analysis (PCA) method, that allows an unsupervised clustering analysis, in order to detect some otherwise not easily identifiable correlation between different gene expression profiles.
Patients and Methods
Patients
Patients included in the study were a small part of those already enrolled in two our previous studies designed to investigate the role of polymorphisms during treatment with TKIs [16, 20].
All cases were observed at the Hematology Unit of the University of Pisa (Italy) and consecutively enrolled on the basis of their acceptance to sign an informed consent to donate the leftover of samples harvested for routine diagnostic tests for every further no-profit scientific purpose. The informed consent was previously approved by the Azienda Ospedaliero-Universitaria Pisana (AOUP) Ethical Committee.
Clinical characteristics of the enrolled patients are reported in Table 1.
Methods
BCR/ABL1 Quantification
Buffy coats obtained from 10 to 20 mL of peripheral blood anti-coagulated with EDTA have been used for the extraction of total RNA by using the phenol/chlorophorm method. To remove contaminating phenol, RNAs were cleaned up using silica-membrane technology (RNeasy Kit, QIAGEN, Valencia, CA, USA), and to avoid DNA-contamination RNAs were treated with DNase (New England Biolabs, Ipswich, MA, USA).
Total RNA concentrations was evaluated by using a ND-1000 spectrophotometer (NanoDrop, Wilmington, DE, USA), and the RNA quality assessed by BioAnalyzer (Agilent, Santa Clara, CA, USA).
Total RNA was then reverted into cDNA by the Ipsogen® RT Kit (Ipsogen, Milan, Italy), according to the manufacturer's instructions.
The molecular response, assessed by quantitative PCR (qPCR) for both P210 fusion transcripts, was calculated after alignment of PCR results to the international scale, as previously described in the ELN guidelines (21) and according to the rules edited by the Italian molecular network LabNet. Patients were classified as “responders,” “warning,” or “failing” according to the ELN guidelines (22).
Considering the small number of cases involved in the present studies, for a more powerful statistical analysis, patients were re-classified as “optimal” (#1, #4, #5, #6, #7, #9) or “failing” (#2, #3, #8, #10, #11) including in this latest category even those “warning,” in accordance to BCR-ABL1/ABL1 levels measured at 12 months. The quality of responses was assessed after 3, 6, and 12 month of treatment, and scored as reported in Supplementary Table 4.
The patients that did not achieve clinical response at fixed time points had been screened for the ABL1 mutational status and they did not harbor ABL1 mutations.
Gene Expression Analysis
For measuring the expression of 255 genes from the WNT, JAK-STAT and Polycomb pathways the commercial PrimePCRTM SYBR® Green assay plates (BioRad) were used in a Real Time PCR apparatus (CFX, ConnectTM, BioRad), according to the manufacturer's instructions (for a detailed list of genes see Supplementary Tables 1–3).
We analyzed samples at diagnosis and after 6 months of therapy. Expression values were calculated by the Vandesompele method using four internal housekeeping genes (ABCTB GAPDH, HPRT, and TBP). Data have been also evaluated with the “Gene Study PrimePCR analysis” software and expressed as fold changes in respect of diagnosis.
Statistical Analysis
The statistical analysis of clinical outcome was performed by using chi-square test for the dicotomic variables or the ROC analysis in order to test a possible correlation between the value of gene expression de-regulation and quality of response. For the survival, the Kaplan-Meier method was employed. The SPSS version 25.0 software was used for the conventional above listed statistical analysis. For all tests, the level of significance was set at p ≤ 0.05.
The PCA analysis was carried out using the MatLab (matrix laboratory) software; MatLab is a multi-paradigm-numerical computing platform and matrix-based language developed by MathWorks. MatLab allows to analyze data, develop algorithms, create of user models, and applications, interfacing with programs written in other languages. In the study the PCAs were performed according to Liberati et al. (23).
Results
Clinical Outcome
All patients were diagnosed in chronic phase, their median age was 69 years (range, 56–79), 64% were men, and 36% women. Patients received imatinib (8), or second-generation TKIs (3) as first line of therapy and during the median follow-up of 24 months; 4 switched therapy, 3 for unsatisfactory response and one for grade-4 toxicity (Supplementary Table 5).
There was no correlation between type of therapy and clinical response, outcome or EFS, probably due to the small number of patients receiving second-generation TKIs enrolled in the study.
The early molecular response (BCR-ABL1/ABL1 ratio IS% <10% at 3 months) (EMR) was achieved by 70% of patients (8 cases); at 6 months of treatment one patient already failed response, two were warning and the remaining eight achieved an optimal response (BCR-ABL1/ABL1 ratio IS% <1%). At 12 months, 6 patients (54.5%) achieved an optimal response (BCR-ABL1/ABL1 ratio IS% <0.1%), whereas 2 were warning and 3 failed treatment (BCR-ABL1/ABL1 ratio IS% >1%).
At 18 and 24 months, the molecular responses were superimposable with those observed at month 12th (Supplementary Table 4).
The EFS was defined as the time elapsed from treatment beginning and the occurrence of one of the following events: failure at 12 months, drug discontinuation for any significant toxicity (WHO grade 4 or lower degree but persistent), progression, loss of complete cytogenetic response (CCyR), and/or of MR3 (BCR-ABL1/ABL1 ratio IS% <0.1%), or death for any cause.
Overall 24 months-EFS in our series was 55%; as expected, it was significantly influenced by the quality of the last molecular response (80% for optimal responders vs. 20% of failing cases; p = 0.018), by the EMR (75% for who achieved EMR vs. 0% for who not achieved EMR; p = 0.004), and by the permanent discontinuation of treatment for any cause (75% for responsive cases vs. 0% for the failing ones; p = 0.001%).
Gene Expression Analysis
After 6 months of therapy a significant de-regulation of the majority of tested genes was observed: de-regulation was considered as significant if a fold change ≥2 (either for increased or decreased expression) (24) was detected (Table 2). To test whether the overall “expression burden” could play a prognostic role, the mean de-regulation value was calculated for each of the 3 considered pathways, and two categories were defined: (1) with “low” expression (when the overall number of de-regulated genes was lower than the respective mean value), and (2) with “high” expression (when the overall number of de-regulated genes was higher than the respective mean value). In all the 3 analyzed pathways an overall gene up-regulation was observed, especially for the JAK/STAT pathway (Table 3).
When we tested the correlation between the overall gene over-expression of the JAK/STAT pathway and the quality of response to TKIs, we observed that 80% of cases in optimal response belonged to the category with lower up-regulation; nevertheless, this correlation was not statistically significant (p = 0.189). Analogously, also the EFS was not significantly conditioned by the expression levels of the genes belonging to this pathway. Further, some de-regulated genes, when individually analyzed, showed a significantly correlation with clinical response and outcome (Figure 1). The 24 month-EFS was correlated to the up-regulation of: IRF1 (p = 0.008; 71% up-regulated vs. 0%), STAT 2: (p = 0.002; 71% up-regulated vs. 0%), PIAS1 (p = 0.033; 80% down–regulated vs. 20%).
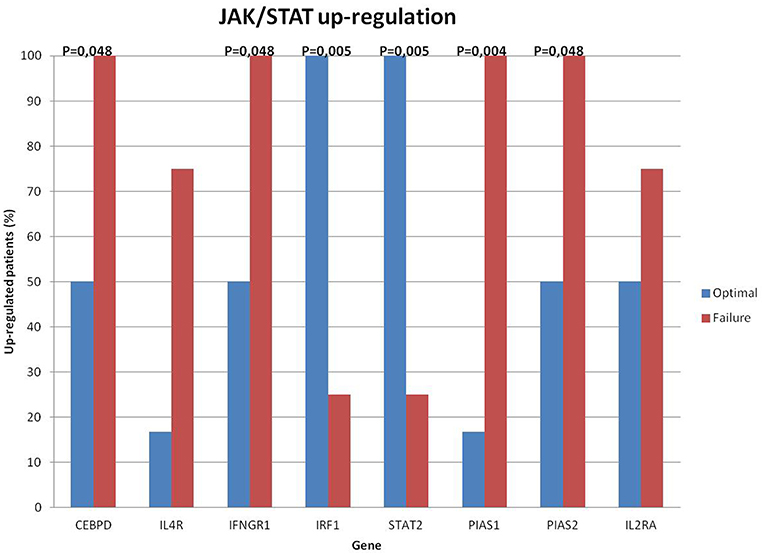
Figure 1. JAK/STAT significantly de-regulated genes. Optimal responders in blue columns and failing patients in red. Up-regulation of some JAK/STAT genes was significantly correlated to patients clinical response (p ≤ 0.05).
Then, we tested if the gene expression could be correlated to the quality of response in the remaining pathways: we observed that 100% of patients with a lower up-regulation (activation) of the WNT pathway achieved an optimal response vs. only 33% of subjects who showed a higher up-regulation of the WNT pathway (p = 0.016). Analogously, also the 24 months EFS resulted significantly influenced by the degree of up-regulation of this pathway: all patients with a “low” up-regulation were event-free vs. 33% of those who presented a “high” up-regulation (p = 0.05) (Figure 2).
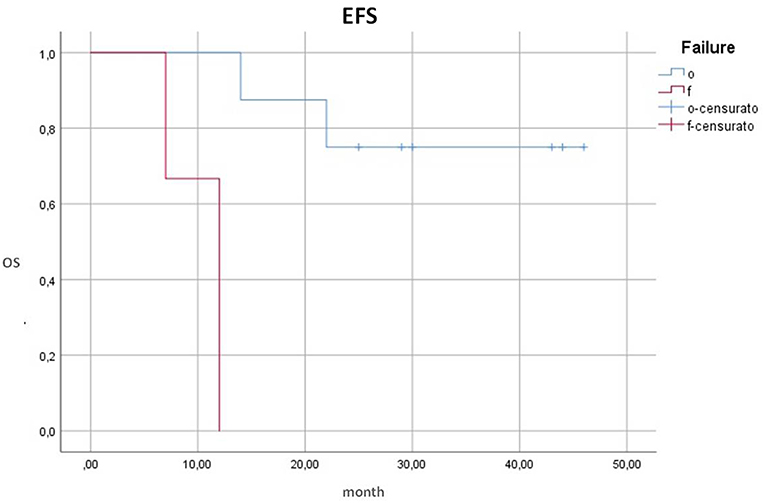
Figure 2. EFS and up-regulation of WNT pathway. EFS, event-free survival; OS, overall survival; WNT up, overexpression of WNT genes; 38, low expression; 39, high expression. 100% of patients with a “low” up-regulation were event-free vs. 33% of those who presented a “high” up-regulation (p = 0.05).
About the de- and up-regulation of the genes belonging to the POLYCOMB pathway, the measured expression levels did not influence either the quality of response or EFS. Anyway, we observed a significant down-regulation of CBX2 in optimal responder patients: this gene has been reported to play an oncogenic role, with its up-regulation being able to predict progression and shorter OS in multiple cancer types (25). Thus, its reduced expression found in optimal responders could be a proof of its role also in CML. Moreover, the up-regulation of PHC3 was significantly correlated with a worse response (p = 0.03) and a shorter EFS (p = 0.025) (Figure 3).
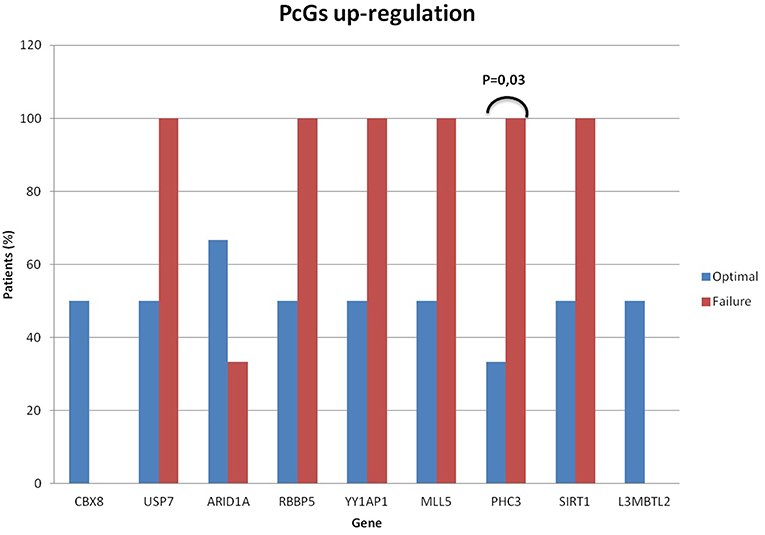
Figure 3. PcGs significantly de-regulation. Optimal responders in blue columns and failing patients in red. De-regulation of some polycomb genes was related to the clinical response, with a significantly up-regulation of PHC3 and worse response to TKIs (p = 0.03).
Finally, another field of interest was the effect in term of gene de-regulation exerted by the different TKIs; nevertheless, the small number of patients receiving second-generation TKIs did not allow the observation of any TKI-specific signature.
Principal Component Analysis Prediction Model
PCA is a multivariate analysis designed to select the linear combination of variables with higher inter-subject covariance, resulting in a good tool for gene ranking. In more detail, PCA returns a new group of orthogonal coordinates of the data space where genes are ordered in decreasing list of covariances. An experimental limitation in the present study was the difficulty in collecting a satisfying number of homogeneous patients, notwithstanding the huge number of variables (255). The principal analyzed components are usually two or three (allowing 2D or 3D plot), and the variables on each component can cluster the subjects in the matrix, thus allowing us to identify which genes would affect the principal component (and so to be significant).
Initially, we clustered in an unsupervised way the 255 tested genes and we observed that two patients stand out, respectively, for the first and the second principal component. These cases corresponded to a resistant (#8) and to a sensitive case (#6), respectively (Figure 4A). Interestingly, the genes characterizing this sample position in the matrix belonged to the WNT pathway: in particular, the first component was characterized by expression of DKK1, WNT6, DKK3, PRICKLE, WISP, and FZD8, while the second one by EP300, SFRP1, and GRB2 (Table 4).
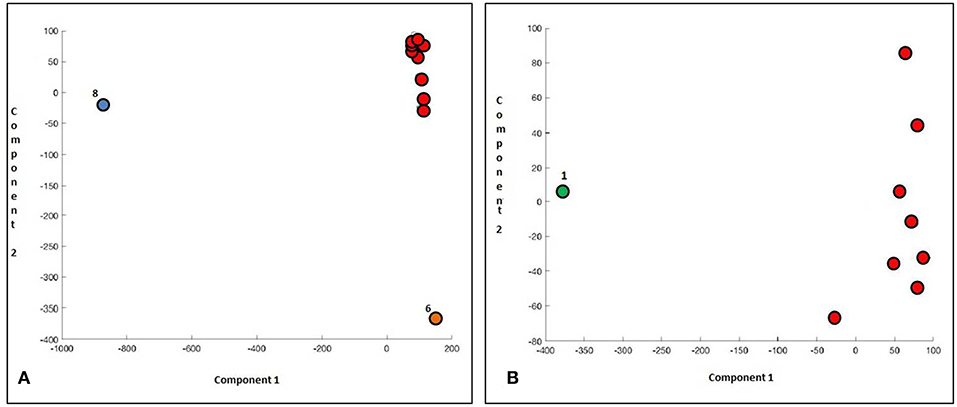
Figure 4. Principal Component Analysis scores represented in a 2D scatter plot. One point per sample is shown. Different colors represent separation between PCA classes. (A) Analysis of 255 genes in all CML patients. (B) Patients #8 and #6 were excluded.
In our second statistical evaluation, the two patients who previously clustered were removed, and the PCA was then applied to the remaining 9 cases: this time, a different expression profile was observed in one responsive patient (#1) who separately clustered. In this case, SFRP1 and CBX2 resulted the most significantly correlated genes: to note that the first gene belongs to the WNT pathway, and the second one to the PcGs. The values of the various components were 2.1, 0.22, and 0.16, respectively; interestingly, the plot differences were significantly related to the first component only (Figure 4B).
Then, we eliminated the case #8 and we repeated the PCA analysis: this time, two patients clustered together outside from the group: cases #6 and #1, both optimal responders. In this occasion, the latent values were 9.7, 1.9, and 0.2.
When the 3 pathways were separately analyzed by PCA we found that: (1) in the PcGs, the case #8 (resistant) deviated from the rest of the population predominantly on the component 1; patients #1 and #7 (optimal responders) were sited in an intermediate zone, while the remaining ones were grouped together, except for the patient #2 (suboptimal) which deviated on the component 2 (Figure 5A). The respective latent values (component 1 and 2) were: 9 and 3. The genes characterizing the component 1 were: CBX2, CBX3, and DNMT3B, while the component 2 was characterized by CBX3, HLTF, SMARCA1, PHC1, ZBTB16, and CBX2.
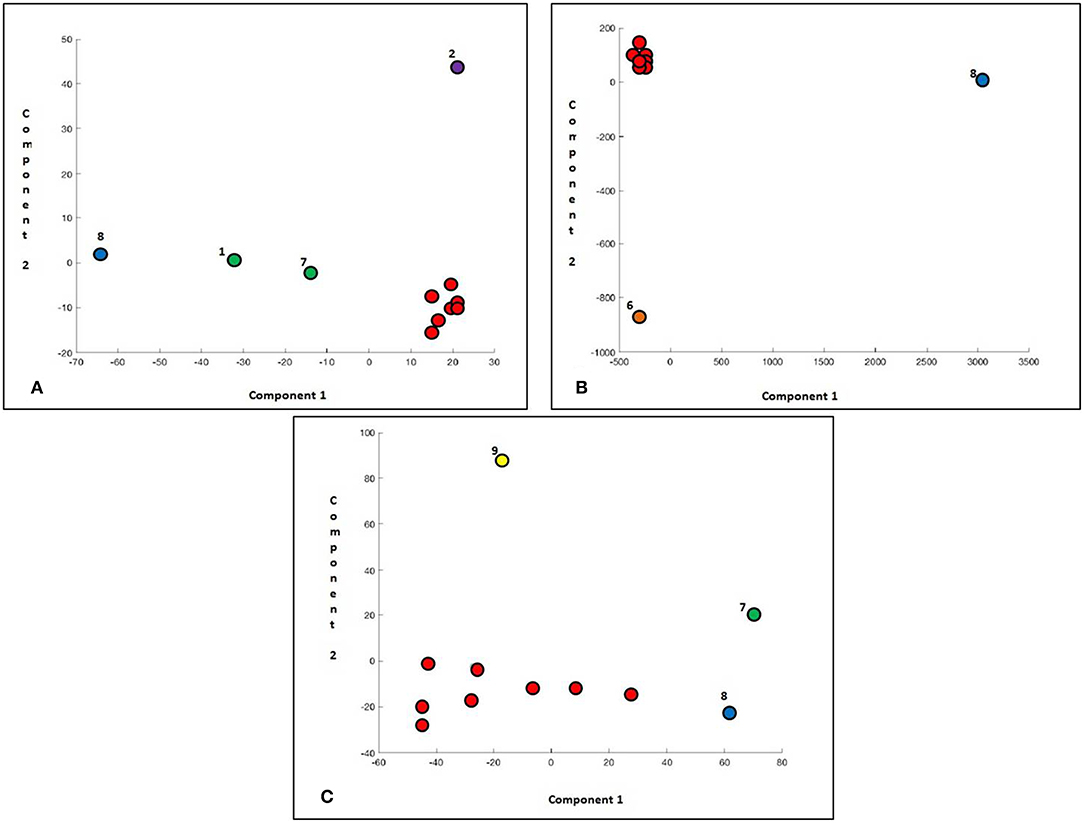
Figure 5. Two-dimensional scatter plot PCA scored for different pathways. (A) Polycomb; (B) WNT; and (C) JAK/STAT. Resistant patients #8 always scored in a PCA different class.
(2) About the WNT pathway, the main component caused a removal from the group of patient #8 whereas the case #6 differing on the component 2 (Figure 5B). The genes characterizing the component 1 were: DKK1, DKK3, WNT6, PRICKLE1, WISP1, and FZD8, while those resulting significant on the component 2 were: EP300 and SFRP1, with latent values of 1 and 0.09, respectively.
3) About the JAK-STAT pathway, two patients (#7 and #8) resulted distant on the first component, whereas #9 (sensitive) was discriminated by the second component (Figure 5C). The genes that impacted on the first component were: STAT2, IL6ST, IL10RA, FCER2, and IL2RA, whereas the genes that weighed on the second component were: SMAD3, IL6ST, and FCR2. We also found that other two genes (FCR2 and IL2RA) characterized the third component. In this analysis, the latent values were: 1.7, 1.1, and 0.8.
In a second phase of our PCA analysis, a different strategy has been adopted: we removed from the analysis the genes that were over-expressed only in one patient or never expressed, obtaining latent values on the three principal components of 2.7, 1, and 0.7. Interestingly, we observed that the sample #8 (a resistant patient) clustered on the first component and that the #7 (an optimal responder) clustered on the second component, while the remaining cases were homogenously grouped (Figure 6A).
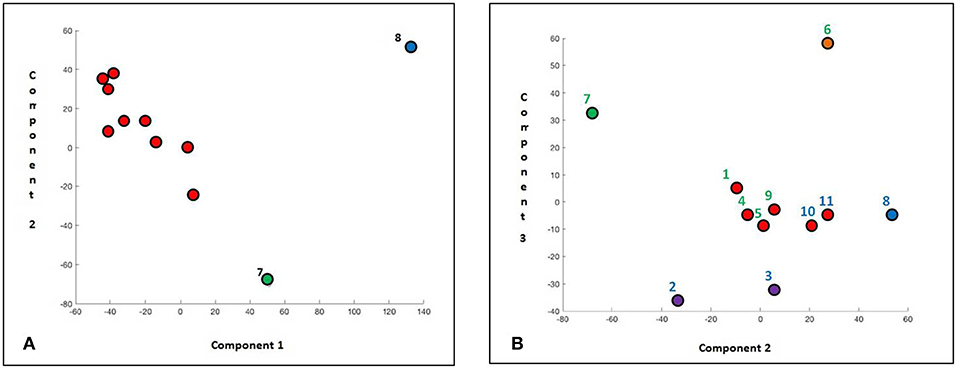
Figure 6. PCA 2D scatter plot of scored genes without largest fold-change. (A) Scatter plot on component 1 and 2. (B) Scatter plot on component 2 and 3.
When the PCA analysis was performed only on the second and third component, the patient #7 clustered near to the zero on the second component, in opposition to the case #8 who was positioned at the opposite site of the graph (Figure 6B). On the third component, we observed patient #2 and the #3 (both suboptimal patients) at one side of the graph, and the case #6 (optimal responder) at the other side. In the center appeared #1, #4, #5 (optimal responders), and in their proximity cases #10 and #11 (suboptimal). Overall, the genes that more significantly impacted on the latest analyzed main component were: TCF7, FZD7, IFNG, SOCS3, GATA3, WNT7A, AXIN2, FZD2, GR2B, and DNMT3B, and those that conditioned the second component were FZD7, TCF7, GRB2, FZD9, FZD2, VANGL2, SOCS3, CDKN1A, CXXC4, F2R, GATA3, IL10RA, and CBX3.
A larger number of analyzed samples could permit a better score separation of samples and nevertheless our PCA analysis was able to clearly distinguish, by clustering, 2 resistant (#2 and #8) and 3 sensitive patients (#1, #6, and #7); thank to this innovative approach, we were able to detect in an unsupervised way putative genes that significantly could condition the quality of response to TKIs.
Discussion
TKIs are widely used to treat CML, from more than 15 years, with a chance of surviving at 5 and 10 years higher than 90% (26, 27). However, TKIs fail to really cure leukemia, because the CML LSC survives and additional genetic abnormalities are believed to contribute to its resistance. Moreover, considering that only a minority of resistant cases present ABL1 mutations, the majority of causes of resistance to TKIs are probably BCR/ABL1-independent.
As above discussed, the bone marrow niche seems to have a relevant role in maintaining CML LSC: obviously, it is not easy to introduce the study of the niche in the clinical practice, so the identification of some simple laboratory approaches still represent an unmet clinical need.
In this line, some surrogate approaches have been identified, that include flow cytometry or molecular techniques: (1) the assessment by flow cytometry of the CD34+/CD38–/CD26+ cells in bone marrow but also in peripheral blood; (2) the measure of the expression level (or their change during treatment) of some genes candidate to explain the BCR/ABL1-independent resistance; (3) the evaluation of several pharmacokinetic and pharmacogenetic aspects that, influencing the TKIs plasma levels and their influx/efflux, can explain efficacy and toxicity of TKIs (drug transporters and cytochrome polymorphisms, drug interactions etc).
Because the role of CD34+/CD38–/CD26+ leukemic cells and pharmacogenetic/pharmacogenomic aspects have been already discussed in some of our previous works (7–20), in this study we focused on the gene expression profiling, in order to evaluate if de-regulation of some candidate genes at early time-points of therapy could impact on the clinical outcome.
As previously reported, gene expression profiling has been used to identify BCR/ABL1-independent mechanisms of resistance and quality of response in CML patients (28). Frank et al. in 2006 performing microarray (Affymetrix HGU133A DNA chip) gene expression analysis found that oxidative stress, DNA repair, centrosomal genes and those linked to apoptosis were associated to resistance (29).
Moreover, as demonstrated by Radich et al., deregulation of the WNT/β-catenin pathway is potentially relevant in the progression of disease. They defined gene signatures of chronic, accelerated, and blast phases comparing the gene expression in 91 CML cases (30). Of course, we do not have the same sample size, that's why our results confirmed that WNT/β- catenin involvement is a significant responsible factor of resistance more powerful than we expected.
Indeed, we compared the expression of 255 genes involved in the WNT, JAK-STAT, and POLYCOMB pathways at 6 months of treatment vs. diagnosis in peripheral blood from 11 patients, we observed that the pathway whose activation resulted more significantly correlated either with the quality of response or with the clinical outcome was the WNT/beta-CATENIN. These results have been validated in unsupervised analysis (PCA) that showed the same significantly de-regulation of WNT pathway.
The WNT/β-CATENIN pathway is known to be crucial for LSC self-renewal, perhaps because the BCR-ABL1 protein can modulate the β-catenin levels; in line with this hypothesis, in a murine model the disruption of β-catenin significantly reduced the probability of leukemic transformation (31). Interestingly, in our study, after PCA analysis, DKK1, DKK3, WNT6, and FZD8 resulted to be the most significantly de-regulated genes during treatment with TKIs. This results are perfectly in line with those already published from in vitro models: DKK1 and DKK3 have been shown to inhibit cancer cell proliferation (32) high levels of WNT6 inversely correlated with the response to chemotherapy in gastric cancer (33) WISP1 has been associated with a poor prognosis in glioblastoma (34, 35), and FZD8 up-regulation sustained resistance in breast cancer, prostate, and colorectal cancer (36–38).
In 2007 an Italian study demonstrated a significant gene de-regulation in the resistant patients compared to the responders ones. After a statistical analysis, the authors found a significant overexpression of 26 genes in non-responders. These genes are involved in signal transduction and transcription factors, apoptosis, cell cycle, and adhesion (39). In line with our study, the power of the proposed gene set was evaluated by two different statistical method but, to our knowledge, no studies in literature have never used both a supervised analysis and PCA at the same time in CML.
To validate results, as we decided, principal component analyses is not often used. It has been used as a biostatistical method for global approach aiming at classifying acute leukemia results of an extensive microarray study (40), in a mantle cell lymphoma study applied to a B-cell receptor-related gene signature (41), and to collect gene expression data taken from a public repository (23).
All these works and our study indicate that PCA is a feasible system that could improve validation of gene expression profiling results, by extracting significant and directly understandable information in an easy and fast way from a great amount of measures.
Translated into the clinical setting, in vitro studies provided several evidences on the role of WNT pathway inhibitors: Hu and colleagues used three drugs (IM, LY294002, and AKTi IV) to inhibit the BCR-ABL1/PI3K/AKT pathway in K562 cells, showing that the inhibition of these kinases induced a decrease of the β-catenin protein (but not the mRNA) and suppressed proliferation of CML progenitors (31). Zhou and colleagues demonstrated that β-catenin inhibition reverses TKI resistance in the advanced phases of disease, independently from the presence of ABL1 mutations, especially when WNT inhibitors are combined with nilotinib (42). Moreover, it has been demonstrated the involvement of the WNT/β-catenin signaling pathway in advanced phase of disease, and FZD8 seems to sustain the LSC survival (43).
WNT inhibitors (PRI-724, LGK-974, and BC-2059) are now used in advanced solid tumors, pancreatic cancer failing first-line therapy and metastatic colorectal cancer, in phase I or II clinical trial studies (https//clinicaltrial.gov), also in advanced myeloid malignancies. In our opinion, this could be a relevant item, as translated into the clinics the combination of WNT/β-catenin inhibitors and TKIs for overcoming resistance or progression in CML.
In conclusion, we found a significant de-regulation of WNT pathway and we demonstrated, even if in a small cohort of CML patients, that its activation could affect the sensitivity to TKIs and the clinical outcome.
Obviously, the aim of this study would be the possible transfer of these findings from the laboratory to the clinical real life; further studies focused on gene expression profiling analysis involving a higher number of patients could soon confirm our results.
Ethics Statement
This study was carried out in accordance with the recommendations of Good Clinical Practice. All subjects gave written informed consent to leave leftover from the routine diagnostic samples for further no-profit studies, in accordance with the Declaration of Helsinki. The study has been performed using samples that all patients left for research purposes after signed informed consent previously approved by the Azienda Ospedaliero-Universitaria Pisana (AOUP, Pisa, Italy) Ethical Committee.
Author Contributions
SuG, SaG, AD, and MP contributed conception and design of the study. EC, FR, SS, SB, and GE collected samples and organized databases. DL, SuG, and SaG performed statistical analysis. SaP, VM, and FGu performed gene expression analysis. ClB, GB, LI, FM, and FGh enrolled and treated patients. AC, ChB, LM, and SiP contributed to manuscript revision.
Funding
This work was partially supported by: the Italian Leukemia Association (AIL) section of Pisa, the University of Pisa (grant PRA 2015 to AD) and a 2018 SIES-Incyte scholarship.
Conflict of Interest Statement
SaG, MP, and AD received grant for speaking from Novartis, BMS, Pfizer, Incyte, MEDAC. SuG got a scholarship 2018 SIES-Incyte. All the authors declare that the research was conducted in the absence of any commercial or financial relationships that could be construed as a potential conflict of interest.
The handling editor declared a shared affiliation, though no other collaboration, with one of the authors SuG.
Acknowledgments
Authors thank all patients who participated to the study, the AIL Pisa for its financial support and all colleagues of Molecular and Cellular Biology Laboratory of Hematology Unit of Pisa Hospital who aided the efforts of the authors.
Supplementary Material
The Supplementary Material for this article can be found online at: https://www.frontiersin.org/articles/10.3389/fonc.2019.00532/full#supplementary-material
Abbreviations
ABCB1, ATP Binding Cassette subfamily B member 1; ABCG2, ATP Binding Cassette super-family G member 2; BCR/ABL1, Breakpoint Cluster Region/Abelson 1; BMI1, B cell-specific Moloney murine leukemia virus Integration site 1; CCyR, Complete Cytogenetic Response; CD26, Cluster of Differentiation 26; CML, Chronic Myeloid Leukemia; DKK, Dickkopf; DPPIV, dipeptidil-peptidasi IV; EFS, Event Free Survival; ELN, European Leukemia Network; EMR, Early Molecular Response; FZD, Frizzled; hOCT1, Human Organic Cation Transporter type 1; JAK/STAT, Janus Kinase/ Signal Transducer and Activator of Transcription; LabNet, Laboratory Network; LSC, Leukemic Stem Cell; MR3, Molecular Response with 3 log reduction; NGS, Next Generation Sequencing; PCA, Principal Component Analysis; PcGs, Polycomb Genes; SNP, single nucleotide polymorphism; TKI, Tyrosine Kinase Inhibitor; WISP, WNT Inducible Signaling Pathway; WNT, Wingless-related integration site.
References
1. Hehlmann R, Hochhaus A, Baccarani M European Leukemia Net. Chronic myeloid leukaemia. Lancet. (2007) 370:342–50. doi: 10.1016/S0140-6736(07)61165-9
2. Soverini S, De Benedittis C, Castagnetti F, Gugliotta G, Mancini M, Bavaro L, et al. In chronic myeloid leukemia patients on second-line tyrosine kinase inhibitor therapy, deep sequencing of BCR-ABL1 at the time of warning may allow sensitive detection of emerging drug-resistant mutants. BMC Cancer. (2016) 16:572 doi: 10.1186/s12885-016-2635-0
3. Cerveira N, Ferreira RB, Bizarro S, Correia C, Torres L, Lisboa S, et al. Ponatinib induces a sustained deep molecular response in a chronic myeloid leukaemia patient with an early relapse with a T315I mutation following allogeneic hematopoietic stem cell transplantation: a case report. BMC Cancer. (2018) 18:1229. doi: 10.1186/s12885-018-5100-4
4. Schoepfer J, Jahnke W, Berellini G, Buonamici S, Cotesta S, Cowan-Jacob SW, et al. Discovery of asciminib (ABL001), an allosteric inhibitor of the tyrosine kinase activity of BCR-ABL1. J Med Chem. (2018) 61:8120–35. doi: 10.1021/acs.jmedchem.8b01040
5. Rovida E, Marzi I, Cipolleschi M, Dello Sbarba P. One more stem cell niche: how the sensitivity of chronic myeloid leukemia cells to imatinib mesylate is modulated within a “hypoxic” enviroment. Hypoxia. (2017) 2:1–10. doi: 10.2147/HP.S51812
6. Rovida E, Peppicelli S, Bono S, Bianchini F, Tusa I, Cheloni G, et al. The metabolicall-modulated stem cell niche: a dynamic scenario regulating cancer cell phenotype and resistance to therapy. Cell Cycle. (2014) 13:3169–75. doi: 10.4161/15384101.2014.964107
7. Crea F, Di Paolo A, Liu HH, Polillo M, Clermont PL, Guerrini F, et al. Polycomb genes are associated with response to imatinib in chronic myeloid leukemia. Epigenomics. (2015) 7:757–65. doi: 10.2217/epi.15.35
8. Galimberti S, Grassi S, Baratè C, Guerrini F, Ciabatti E, Perutelli F, et al. The polycomb BMI1 protein is co-expressed with CD26+ in leukemic stem cells of chronic myeloid leukemia. Front Oncol. (2018) 8:555. doi: 10.3389/fonc.2018.00555
9. Valent P, Sadovnik I, Racil Z. DPPIV (CD26) as a novel stem cell marker in Ph+ chronic myeloid leukemia. Eur J Clin Investig. (2014) 44: 1239–45. doi: 10.1111/eci.12368
10. Bocchia M, Sicuranza A, Abruzzese E, Iurlo A, Sirianni S, Gozzini A, et al. Residual peripheral blood CD26+ leukemic stem cells in chronic myeloid leukemia patients during TKI therapy and during treatment-free remission. Front Oncol. (2018) 8:194. doi: 10.3389/fonc.2018.00194
11. Groner B, von Manstein V. Jak Stat signaling and cancer: opportunities, benefits and side effects of targeted inhibition. Mol Cell Endocrinol. (2017) 451:1–14. doi: 10.1016/j.mce.2017.05.033
12. Stella S, Tirrò E, Conte E, Stagno F, Di Raimondo F, Manzella L, et al. Cancer therapeutics insights, suppression of survivin induced by a BCR-ABL/JAK2/STAT3, pathway sensitizes imatinib-resistant CML cells to different cytotoxic drugs. Mol Cancer Ther. (2013) 12:1085–98. doi: 10.1158/1535-7163.MCT-12-0550
13. Nair RR, Tolentino JH, Hazlehurst LA. Role of STAT3 in transformation and drug resistance in CML. Front Oncol. (2012) 2:30 doi: 10.3389/fonc.2012.00030
14. Zhao C, Blum J, Chen A, Kwon HY, Jung SH, Cook JM, et al. Loss of b-catenin impairs the renewal of normal and CML cell. Cancer Cell.(2007) 12:528–41. doi: 10.1016/j.ccr.2007.11.003
15. Arrigoni E, Del Re M, Galimberti S, Restante G, Rofi E, Crucitta S, et al. Concise review: chronic myeloid leukemia: stem cell niche and response to pharmacologic treatment. Stem Cells Transl Med. (2018) 7:305–14. doi: 10.1002/sctm.17-0175
16. Di Paolo A, Polillo M, Capecchi M, Cervetti G, Baratè C, Angelini S, et al. The c.480C>G polymorphism of hOCT1 influences imatinib clearance in patients affected by chronic myeloid leukemia. Pharmacogenomics J. (2014) 14:328–35. doi: 10.1038/tpj.2014.7
17. Polillo M, Galimberti S, Baratè C, Petrini M, Danesi R, Di Paolo A. Pharmacogenetics of BCR/ABL inhibitors in chronic myeloid leukemia. Int J Mol Sci. (2015) 16:22811–29. doi: 10.3390/ijms160922811
18. Arrigoni E, Galimberti S, Petrini M, Danesi R, Di Paolo A. ATP-binding cassette transmembrane transporters and their epigenetic control in cancer: an overview. Expert Opin Drug Metab Toxicol. (2016) 12:1419–32. doi: 10.1080/17425255.2016.1215423
19. Galeotti L, Ceccherini F, Doming D, Laurino M, Polillo M, Di Paolo A, et al. Association of the hOCT1/ABCB1 genotype with efficacy and tolerability of imatinib in patients affected by chronic myeloid leukemia. Cancer Chemother Pharmacol. (2017) 79:767–73. doi: 10.1007/s00280-017-3271-3
20. Galimberti S, Bucelli C, Arrigoni E, Baratè C, Grassi S, Ricci F, et al. The hOCT1 and ABCB1 polymorphisms do not influence the pharmacodynamics of nilotinib in chronic myeloid leukemia. Oncotarget. (2017) 8:88021–33. doi: 10.18632/oncotarget.21406
21. Hughes T, Deininger M, Hochhaus A, Branford S, Radich J, Kaeda J, et al. Monitoring CML patients responding to treatment with tyrosine kinase inhibitors: review and recommendations for harmonizing current methodology for detecting BCR-ABL transcripts and kinase domain mutations and for expressing results. Blood. (2006) 108:28–37. doi: 10.1182/blood-2006-01-0092
22. Baccarani M, Deininger MW, Rosti G, Hochhaus A, Soverini S, Apperley JF, et al. European Leukemia Net recommendations for the management of chronic myeloid leukemia. Blood. (2013) 122:872–84. doi: 10.1182/blood-2013-05-501569
23. Liberati D, Bittani S, Garatti S. Unsupervised mining of genes classifying leukemia. In: Encyclopedia of Data Warehousing and Mining. Idea Group Inc. (2006). doi: 10.4018/978-1-59140-557-3.ch217
24. Savli H, Galimberti S, Sünnetçi D, Canestraro M, Palumbo G, Nagy B, et al. Bortezomib and arsenic trioxide activity on a myelodysplastic cell line (P39): a gene expression study. Turk J Haematol. (2015) 32:206–12. doi: 10.4274/Tjh.2014.0058
25. Clermont PL, Sun L, Crea F, Thu KL, Zhang A, Parolia A, et al. Genotranscriptomic meta-analysis of the Polycomb gene CBX2 in human cancers: initial evidence of an oncogenic role. Br J Cancer. (2014) 111:1663–72. doi: 10.1038/bjc.2014.474
26. Hochhaus A, Larson RA, Guilhot F, Radich JP, Branford S, Hughes TP, et al. Long-term outcomes of imatinib treatment for chronic myeloid leukemia. N Engl J Med. (2017) 376:917–27. doi: 10.1056/NEJMoa1609324
27. Nicolini FE, Alcazer V, Cony-Makhoul P, Heiblig M, Morisset S, Fossard G. Long-term follow-up of de novo chronic phase chronic myelogenous leukemia patients on imatinib first-line. Exp Hematol. (2018) 64:97–105. doi: 10.1016/j.exphem.2018.05.003
28. Cha K, Li Y, Yi GS. Discovering gene expression signatures responding to tyrosine kinase inhibitor treatment in chronic myeloid leukemia. BMC Med Genom. (2016) 9(Suppl 1):29. doi: 10.1186/s12920-016-0194-5
29. Frank O, Brors B, Fabarius A, Li L, Haak M, Merk S, et al. Gene expression signature of primary imatinib-resistant chronic myeloid leukemia patients. Leukemia. (2006) 20:1400–7. doi: 10.1038/sj.leu.2404270
30. Radich JP, Dai H, Mao M, Oehler V, Schelter J, Druker B, et al. Gene expression changes associated with progression and response in chronic myeloid leukemia. Proc Natl Acad Sci USA. (2006) 103:2794–9. doi: 10.1073/pnas.0510423103
31. Hu J, Feng M, Liu ZL, Liu Y, Huang ZL, Li H, et al. Potential role of wnt/b-catenin signaling in blastic transformation of chronic myeloid leukemia: cross talk between b-catenin and BCR/ABL. Tumor Biol. (2016).37:15859–72. doi: 10.1007/s13277-016-5413-3
32. Firtina S, Hatirnaz Ng Ö, Erbïlgïn Y, Özbek U, Sayïtoglu M. Dysregulation of the DKK1 gene in pediatric B-cell acute lymphoblastic leukemia. Turk J Med Sci. (2017) 47:357–63. doi: 10.3906/sag-1507-106
33. Yuan G, Regel I, Lian F, Friedrich T, Hitkova I, Hofheinz RD, et al. WNT6 is a novel target gene of caveolin-1 promoting chemoresistance to epirubicin in human gastric cancer cells. Oncogene. (2013) 32:375–87. doi: 10.1038/onc.2012.40
34. Jing D, Zhang Q, Yu H, Zhao Y, Shen L. Identification of WISP1 as a novel oncogene in glioblastoma. Int J Oncol. (2017) 51:1261–70. doi: 10.3892/ijo.2017.4119
35. Zhang X, Chen X, Liu J, Dong X, Jin Y, Tian Y, et al. Knockdown of WISP1 inhibit proliferation and induce apoptosis in ALL Jurkat cells. Int J Clin Exp Pathol. (2015) 8:15489–96.
36. Yin S, Xu L, Bonfil RD, Banerjee S, Sarkar FH, Sethi S, et al. Tumor initiating cells and FZD8 play a major role in drug resistance in triple-negative breast cancer. Mol Cancer Ther. (2013) 12:491–8. doi: 10.1158/1535-7163.MCT-12-1090
37. Li Q, Ye L, Zhang X, Wang M, Lin C, Huang S, et al. FZD8, a target of p53, promotes bone metastasis in prostate cancer by activating canonical Wnt/β-catenin signaling. Cancer Lett. (2017) 402:166–76. doi: 10.1016/j.canlet.2017.05.029
38. Xu L, Wen T, Liu Z, Xu F, Yang L, Liu J, et al. MicroRNA-375 suppresses human colorectal cancer metastasis by targeting Frizzled. Oncotarget. (2016) 7:40644–56. doi: 10.18632/oncotarget.9811
39. Cammarata G, Augugliaro L, La Rosa M, Turri D, Rizzo V, Marfia A, et al. Gene expression profile of chronic myeloid leukemia innately resistant to imatinib. Clin Leuk. (2007) 1:234–42. doi: 10.3816/CLK.2007.n.014
40. Haferlach T, Kohlmann A, Schnittger S, Dugas M, Hiddemann W, Kern W, et al. Global approach to the diagnosis of leukemia using gene expression profiling. Blood. (2005) 106:1189–98. doi: 10.1182/blood-2004-12-4938
41. Bomben R, Ferrero S, D'Agaro T, Dal Bo M, Re A, Evangelista A, et al. A B-cell receptor-related gene signature predicts survival in mantle cell lymphoma: results from the Fondazione Italiana Linfomi MCL-0208 trial. Haematologica. (2018) 103:849–56. doi: 10.3324/haematol.2017.184325
42. Zhou H, Mak PY, Mu H, Mak DH, Zeng Z, Cortes J, et al. Combined inhibition of β-catenin and Bcr–Abl synergistically targets tyrosine kinase inhibitor-resistant blast crisis chronic myeloid leukemia blasts and progenitors in vitro and in vivo. Leukemia. (2017) 31:2065–74. doi: 10.1038/leu.2017.87
Keywords: WNT/β-catenin, PcGs, JAK/STAT, CML, BCR/ABL1-independent resistance, PCA
Citation: Grassi S, Palumbo S, Mariotti V, Liberati D, Guerrini F, Ciabatti E, Salehzadeh S, Baratè C, Balducci S, Ricci F, Buda G, Iovino L, Mazziotta F, Ghio F, Ercolano G, Di Paolo A, Cecchettini A, Baldini C, Mattii L, Pellegrini S, Petrini M and Galimberti S (2019) The WNT Pathway Is Relevant for the BCR-ABL1-Independent Resistance in Chronic Myeloid Leukemia. Front. Oncol. 9:532. doi: 10.3389/fonc.2019.00532
Received: 25 January 2019; Accepted: 31 May 2019;
Published: 24 June 2019.
Edited by:
Alessandro Gozzetti, University of Siena, ItalyReviewed by:
Vemika Chandra, Children's Hospital of Philadelphia, United StatesAhmet Emre Eskazan, Istanbul University Cerrahpasa Faculty of Medicine, Turkey
Copyright © 2019 Grassi, Palumbo, Mariotti, Liberati, Guerrini, Ciabatti, Salehzadeh, Baratè, Balducci, Ricci, Buda, Iovino, Mazziotta, Ghio, Ercolano, Di Paolo, Cecchettini, Baldini, Mattii, Pellegrini, Petrini and Galimberti. This is an open-access article distributed under the terms of the Creative Commons Attribution License (CC BY). The use, distribution or reproduction in other forums is permitted, provided the original author(s) and the copyright owner(s) are credited and that the original publication in this journal is cited, in accordance with accepted academic practice. No use, distribution or reproduction is permitted which does not comply with these terms.
*Correspondence: Susanna Grassi, Z3Jhc3Npc3VzYW5uYUB2aXJnaWxpby5pdA==; Sara Galimberti, c2FyYS5nYWxpbWJlcnRpQG1lZC51bmlwaS5pdA==