- 1Department of Neurosurgery, Xiangya Hospital, Central South University, Changsha, China
- 2Department of Neurosurgery, Peking University Third Hospital, Peking University, Beijing, China
- 3Sun Yat-sen University Cancer Center, State Key Laboratory of Oncology in South China, Collaborative Innovation Center for Cancer Medicine, Guangzhou, China
- 4Institute of Skull Base Surgery & Neuro-oncology at Hunan, Changsha, China
Immune evasion in glioma strongly correlates with clinical outcomes; however, the molecular mechanisms driving the maintenance of immunosuppression remain largely unknown. Recently studies demonstrate that Klothos are aberrantly expressed in several cancers and are potential therapeutic targets in cancers. However, their roles are still unclear in glioma. Here, we show that LCTL is highly expressed in gliomas and that its expression is regulated by DNA methylation status at the promoter. LCTL expression is also found to be significantly associated with high tumor aggressiveness and poor outcomes for glioma patients. Mechanistically, results suggested that LCTL might play an important immunosuppressive role by recruiting immunosuppressive cells and regulating tumor-associated macrophages polarization, T cell exhaustion, and epithelial–mesenchymal transition through FGF signaling in glioma. Our results establish LCTL as a key biomarker for prognosis that could be considered a potential epigenetic and immunotherapeutic target for treatment.
Introduction
Gliomas are the most common primary tumors of the central nervous system and account for nearly 75% of malignant brain tumors in adults, of which glioblastoma multiform (GBM) is categorized as the most malignant subtype (1, 2). Despite current standard multimodal treatment, including maximal safe resection followed by combined radio-chemotherapy, the median survival time for patients with glioma is still <2 years (3, 4). Glioma resistant to advanced therapeutic strategies has been widely reported as a consequence of distinct metabolic mechanisms and the complicated immunosuppressive microenvironment that surrounds the tumor niche (5, 6). With advancements in molecular biology, a number of significant genetic alterations (IDH mutation, 1p/19q codeletion, H3ys27Met, and RELA-fusion) are now associated with heterogeneous tumor histology and are clinically significant based on the revised 2016 World Health Organization (WHO) classification (7). Strengthening the knowledge of such molecular alterations will ultimately contribute to a more comprehensive understanding of the diagnosis, classification, and treatment of gliomas. From this perspective, it is urgent to identify novel molecular targets and biomarkers to develop efficient therapeutic strategies.
Klotho was originally identified as an anti-aging gene in 1997 (8). The Klotho family comprises three classic members, namely Klotho (KL), βKlotho (KLB), and γKlotho (also referred as LCTL) (9). All of these three genes encode transmembrane proteins, belonging to glycosidase family 1 and sharing structural similarities to β-glycosidases. However, the critical residues required for enzymatic activity are not conserved in any of the Klotho proteins (10), which indicates that their biological function is independent of glycosidase activity. Klothos proteins are known as essential cofactors that participate in interactions between fibroblast growth factors (FGFs) and fibroblast growth factor receptors (FGFRs) (9, 11). FGF and FGFRs regulate a wide range of biological functions including cell fate, angiogenesis, immunity, and metabolism, and are aberrantly activated during carcinogenesis (12, 13). In addition, recent studies have demonstrated aberrant expression of Klothos in several cancers including breast cancer, lung cancer, and hepatocellular carcinoma (9, 14–17). Most studies indicate that KL functions as a tumor suppressor and modulates several signaling pathways such as insulin-like growth factor-1 (IGF-1), FGF, and Wnt/β-Catenin (18, 19). However, the role of KLB in cancer is controversial. Poh et al. (17) reported that it is up-regulated and associated with FGFR4 signaling in hepatocellular carcinoma. In contrast, Ye et al. (20) reported KLB is down-regulated in this disease and regulates Akt/GSK-3β/cyclin D1 signaling. Although mounting evidence suggests potential connections between the klotho family and tumor development, the role of LCTL in tumorigenesis is still uncertain. Recently, Trost et al. (9) reported that LCTL is a potential oncogene in triple negative breast cancer and that it is necessary for resistance to increased oxidative damage. LCTL was also reported to be associated with cell proliferation, apoptosis, and epithelial-mesenchymal transition (EMT) in urothelial carcinoma of the bladder (21). However, the role and clinical importance of Klothos is still unclear with respect to glioma.
In this study, we first performed differential gene expression analysis of Klothos comparing the TCGA databases for glioma and normal brain tissues via a bioinformatics approach. We found of Klotho genes, only LCTL met the preset thresholds of differential expression. Further analysis revealed that the expression of LCTL is significantly elevated in gliomas compared to that in normal brain tissues, and that it is strictly associated with pathologic and molecular characteristics of different mutants. Moreover, by analyzing certain prognostic outcomes, LCTL was found to be associated with overall survival time in patients with glioma. Similar to that with KL, DNA methylation of the LCTL promoter can silence its endogenous expression. After identifying that high LCTL expression might lead to glioma progression, we further investigated its potential biological role in glioma based on gene ontology (GO) analysis and observed that it is markedly involved in tumor-associated immune responses in this disease. Further analysis revealed that increased LCTL expression is particularly associated with various immunosuppressive behaviors such as the recruitment of suppressive immune cells, secretion of cytokines, and transformation to tumor-promoting phenotype via FGF signaling, which in turn impairs normal immunosurveillance and leads to disease progression.
Materials and Methods
Data Sets and Human Tissue Samples
The Patient clinical annotation and gene expression data used in this study were obtained from publicly available databases. The TCGA lower grade glioma and glioblastoma (GBMLGG) dataset, which included genomic data and phenotypic data, was obtained from the University of California, Santa Cruz, Xena browser (https://xenabrowser.net/). The genomic data set contained DNA methylation and gene expression RNAseq (IlluminaHiSeq) data, whereas the phenotype dataset contained demographic, clinical, pathological, and IDH status. An additional data set was obtained from the Gene Expression Omnibus (GEO) GSE16011 (n = 284), which includes lower grade glioma and glioblastoma; and the data are also available on the R2: Genomics Analysis and Visualization Platform (http://r2.amc.nl).
In addition, we obtained glioma tissue samples from 45 patients (15 grade II, 15 grade III, and 15 grade IV glioma) and 15 normal brain tissue samples from the Department of Neurosurgery, Xiangya Hospital, Hunan, China. This study was approved by the Ethics Committee of Xiangya Hospital, Central South University and informed consent was obtained from all the patients. Tissues were frozen in RNAlater (Ambion) in liquid nitrogen and stored until total RNAs were extracted.
Differential Expression Analysis
Gene Differential gene expression analysis was performed using the online database Gene Expression Profiling Interactive Analysis (GEPIA). GEPIA (22) is an interactive web platform for gene expression analysis, which includes 9,736 tumors and 8,587 normal samples from TCGA and GTEx databases and its gene expression data have been re-computed from raw RNA-Seq data based on the UCSC Xena project and a uniform pipeline for solving the imbalance between tumor and normal data. The preset differential thresholds were p-value < 0.05 and |log2FC| > 1. Based on differential analysis, the expression data are first log2(TPM + 1)-transformed and the log2FC is defined as median (tumor)—median (normal). Only genes that meet the preset thresholds are considered differentially expressed.
Gene Expression Analysis
Gene expression data from TCGA were generated using the Illumina HiSeq 2000 RNA Sequencing platform, and this dataset shows gene-level transcription estimates, as log2(x + 1)-transformed RSEM normalized counts. Gene expression data from GSE16011 was generated using an Affymetrix Gene Chip Human Genome U133 Plus 2.0 Array. In addition, LCTL expression in diverse cancers was analyzed via Tumor Immune Estimation Resource (TIMER, http://cistrome.org/TIMER/) (23).
Analysis of Genetic Alterations and DNA Methylation
The Genetic alterations analysis was performed using The cBioPortal for Cancer Genomics (http://cbioportal.org), which provides a web resource to explore, visualize, and analyze multidimensional cancer genomics data (24). DNA promoter methylation data (Methylation 450k), similar to gene expression data, were also downloaded from UCSC Xena browser. Pearson correlation analysis of gene expression and DNA methylation was performed and evaluated via R language.
Survival Analysis
Kaplan–Meier survival analysis and the Cox proportional hazard model were used to estimate the prognostic value of LCTL based on TCGA GBMLGG data using R language packages (survival and survminer). Kaplan–Meier survival analysis for the GSE16011 data set was generated by the R2: Genomics Analysis and Visualization Platform.
Gene Ontology (GO) Enrichment Analysis
Gene ontology enrichment analysis was performed by The Database for Annotation, Visualization and Integrated Discovery (DAVID, https://david.ncifcrf.gov/), an online software, to identify GO categories by their biological processes, molecular functions, and cellular components (25). Enriched ontological terms with P < 0.05 were regarded as statistical significance.
Analysis of Stromal and Immune Infiltration
ESTIMATE (Estimation of STromal and Immune cells in MAlignant Tumor tissues using Expression) algorithm was described by Yoshihara (26) to assess the presence of stromal cells and the infiltration of immune cells in tumor samples, and its predictive ability has been validated in large and independent data sets. The ESTIMATE algorithm generates three scores as follows: stromal score (positively correlating with the presence of stroma in tumor tissue), immune score (positively correlating with the level of immune cells infiltrations in tumor tissue), and estimate score (which infers and negatively correlates with tumor purity). xCell, reported by Aran (27) to estimate the enrichment of cell types, can estimate 64 cell types, spanning multiple adaptive and innate immune cells, hematopoietic progenitors, epithelial cells, and extracellular matrix cells derived from expression profiles. The scores, calculated by the ESTIMATE algorithm, were downloaded from https://bioinformatics.mdanderson.org/estimate/. The pre-calculated TCGA data based on xCell was downloaded from http://xcell.ucsf.edu/. Then the correlation between LCTL expression and ESTIMATE scores and 64 cell types from the TCGA glioma dataset were analyzed using R language.
Protein-Protein Interaction (PPI) Analysis
The Search Tool for the Retrieval of Interacting Genes (STRING), an online database, was used to identify proteins that can interact with LCTL and construct PPI networks for this protein, immunosuppressive cell recruitment factors, immunosuppressive factors, factors promoting M2 differentiation, and markers of T cell exhaustion and EMT.
Cell Lines and Culture
The human glioma cell lines (U87, U251, SF126, SF767, A172, and SHG-44) and the normal glial cell line HEB were obtained from Sun Yat-Sen University Cancer Center. All the cell lines were cultured in DMEM with 10% FBS and antibiotics (100 μg/ml penicillin and 100 μg/ml streptomycin), and maintained in standard culture condition.
RNA Extraction and Real-Time PCR
Total RNA was extracted from cell lines or human tissues by Trizol reagent (Invitrogen) according to the manufacturer's protocol. Then, total RNA was quantified and 1 μg of RNA was reverse-transcribed with the Reverse Transcription Kit (Thermo Fisher Scientific). Q-PCR was performed using SYBR Premix Ex Taq II (Takara Bio). β-Actin mRNA was used to normalize the expression of genes. Primers are described in Supplementary Table S1.
Statistical Analysis
Statistical computations and the creation of figures were performed with several packages (ggplot2, survival, survminer, corrplot) in the statistical software environment R, version 3.5.3 (http://www.r-project.org).
Results
LCTL mRNA Expression Levels in Gliomas and Other Cancers
To assess the differential expression of Klotho genes in gliomas and normal brain tissues, the online database GEPIA was used. Results showed that there was no significant difference in the expression of KL between gliomas and normal brain tissues (p > 0.05, Supplementary Figure S1A). Even though the expression of KLB was significantly downregulated in glioma compared to levels in normal brain tissue (p < 0.05, Supplementary Figure S1B), it was still not a differentially expressed, as the |log2FC| value was <1. Interestingly, among Klotho genes, only LCTL met the preset criterion and was significantly upregulated in both brain lower grade glioma (LGG) and GBM, as compared to levels in normal tissue (p < 0.05, Figure 1A). We further analyzed the expression of this gene in different grades of glioma and found that its level significantly increases with WHO grades based on the TCGA dataset (Figure 1B, left). Further, this result was validated by the GSE16011 dataset (Figure 1B, right). In addition, LCTL expression was significantly upregulated in the mesenchymal subtype compared with other three respective molecular subtypes in the TCGA dataset (Figure 1C). To further validate these findings, Q-PCR was performed in cell lines and our 45 gliomas samples. The results showed that LCTL was upregulated in glioma cell lines compared with that in normal glial cell line (Figure 1D), its expression level was higher in glioma tissues than that in normal brain tissues (Figure 1E), and the expression level of LCTL positively correlated with WHO grade of gliomas (Figure 1F). Moreover, an analysis of LCTL expression in multiple human cancer types via TIMER showed that the expression levels were highest in GBM, following by skin cutaneous melanoma (SKCM) and LGG (Figure 1G). These results indicated that LCTL is significantly up regulated in glioma and several other cancers.
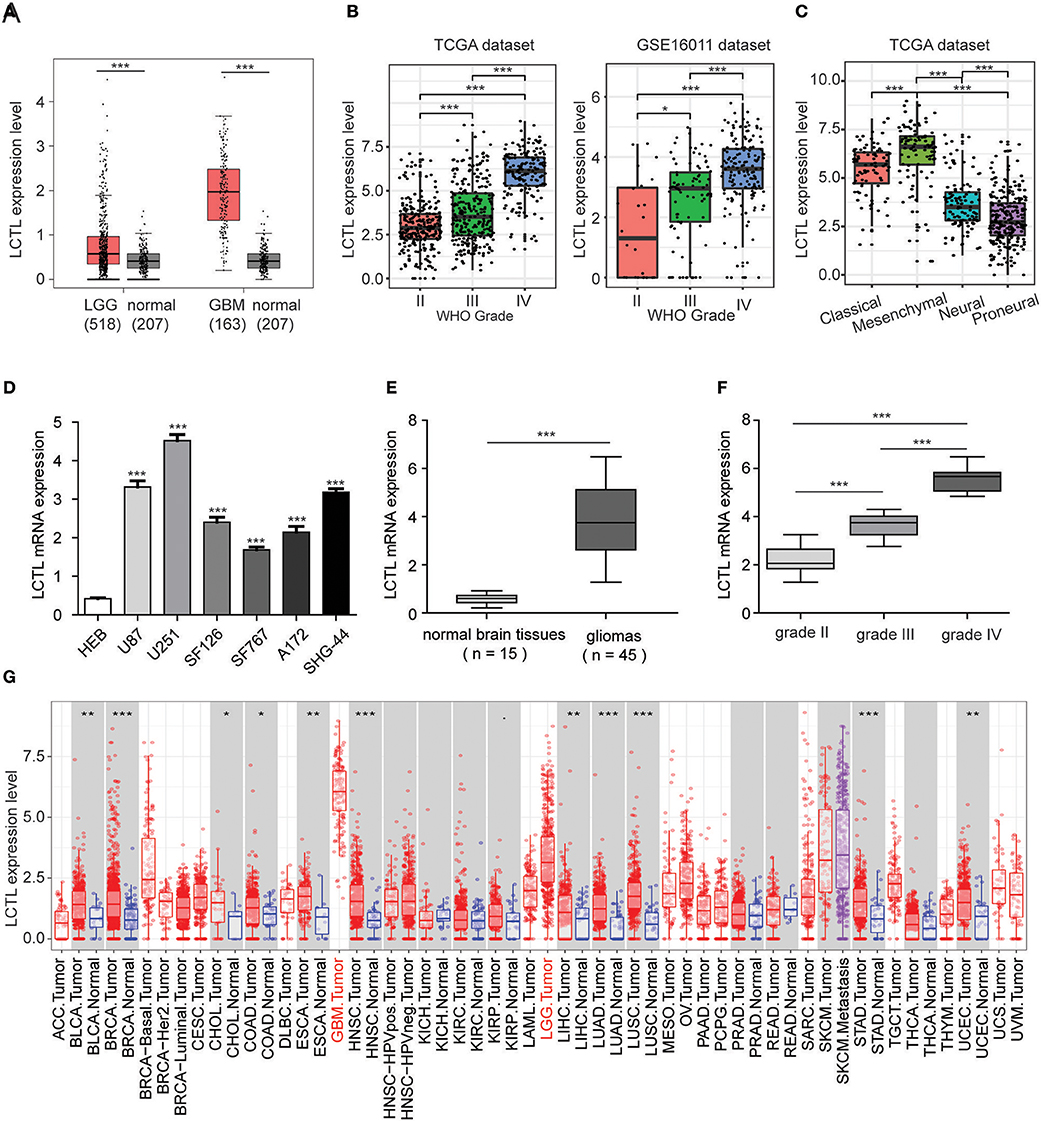
Figure 1. LCTL is highly expressed in gliomas and significantly associated with tumor aggressiveness. (A) Differential expression of LCTL in brain lower grade glioma (LGG) and glioblastoma (GBM) compared to levels in normal brain tissues. (B) LCTL expression in glioma of WHO grade II–IV based on both TCGA and GSE16011 datasets. (C) LCTL expression pattern in different molecular subtypes of glioma (classical, mesenchymal, neural, proneural) in the TCGA dataset. (D) The mRNA expression level of LCTL in glioma cell lines and the normal glial cell line HEB by Q-PCR. (E) Validation of aberrant mRNA expression of LCTL in 45 gliomas compared to 15 normal brain tissues via Q-PCR. (F) LCTL expression in glioma of WHO grade II–IV based on our patient samples. (G) Expression of LCTL in multiple cancers as determined by TIMER analysis. ACC, Adrenocortical carcinoma; BLCA, Bladder Urothelial Carcinoma; BRCA, Breast invasive carcinoma; CESC, Cervical squamous cell carcinoma and endocervical adenocarcinoma; CHOL, Cholangiocarcinoma; COAD, Colon adenocarcinoma; DLBC, Lymphoid Neoplasm Diffuse Large B-cell Lymphoma; ESCA, Esophageal carcinoma; GBM, Glioblastoma multiforme; HNSC, Head and Neck squamous cell carcinoma; KICH, Kidney Chromophobe; KIRC, Kidney renal clear cell carcinoma; KIRP, Kidney renal papillary cell carcinoma; LAML, Acute Myeloid Leukemia; LGG, Brain Lower Grade Glioma; LIHC, Liver hepatocellular carcinoma; LUAD, Lung adenocarcinoma; LUSC, Lung squamous cell carcinoma; MESO, Mesothelioma; OV, Ovarian serous cystadenocarcinoma; PAAD, Pancreatic adenocarcinoma; PCPG, Pheochromocytoma and Paraganglioma; PRAD, Prostate adenocarcinoma; READ, Rectum adenocarcinoma; SARC, Sarcoma; SKCM, Skin Cutaneous Melanoma; STAD, Stomach adenocarcinoma; TGCT, Testicular Germ Cell Tumors; THCA, Thyroid carcinoma; THYM, Thymoma; UCEC, Uterine Corpus Endometrial Carcinoma; UCS, Uterine Carcinosarcoma; UVM, Uveal Melanoma. **P < 0.01, ***P < 0.001.
LCTL Expression Is Associated With Glioma Patient Outcomes
Since the expression of LCTL was found to be aberrantly expressed in gliomas and to correlate with histological grade and molecular subtype, we further studied its prognostic value. For this, Kaplan–Meier survival analysis was performed to evaluate the predictive effects of this gene using both TCGA and GSE16011 datasets. Results indicated that high LCTL expression is not only significantly associated with poor prognosis in glioma patients (Figures 2A,B), but also in patients with high grade glioma (Figures 2C,D). Recently, increasing evidence has suggested recurrent point mutations in isocitrate dehydrogenase genes (IDH1 and IDH2) occur in specific types of glioma (28). Although tumors exhibit identical histologies, the outcome for IDH-mutant (IDH-Mut) diffuse gliomas is better than that with IDH-wildtype (IDH-Wt) disease (29). Further, in the latest version of the WHO Classification of Central Nervous System Tumors published in 2016, IDH mutations were adopted as a decisive marker for glioma classification (30). Currently, this is widely used clinically as a strong prognostic marker for glioma patients. Interestingly, we also found that LCTL expression in IDH-Wt gliomas was significantly higher than that in IDH-Mut tumors based on TCGA dataset (Figure 2E), consistent with results obtained from the GSE16011 dataset (Figure 2F). Simultaneously, we wondered whether LCTL could be an independent prognostic marker for glioma and performed univariate and multivariate Cox regression analysis based on the TCGA dataset. Univariate analysis revealed that LCTL expression, patient age at diagnosis, WHO grade, and IDH status, were significantly associated with overall survival. Based on multivariate analysis, the expression of LCTL was also a significant predictive factor after adjusting for the aforementioned clinical factors (Table 1). Taken together, LCTL is an independent prognostic factor, and high expression indicates poor clinical outcome for glioma patients.
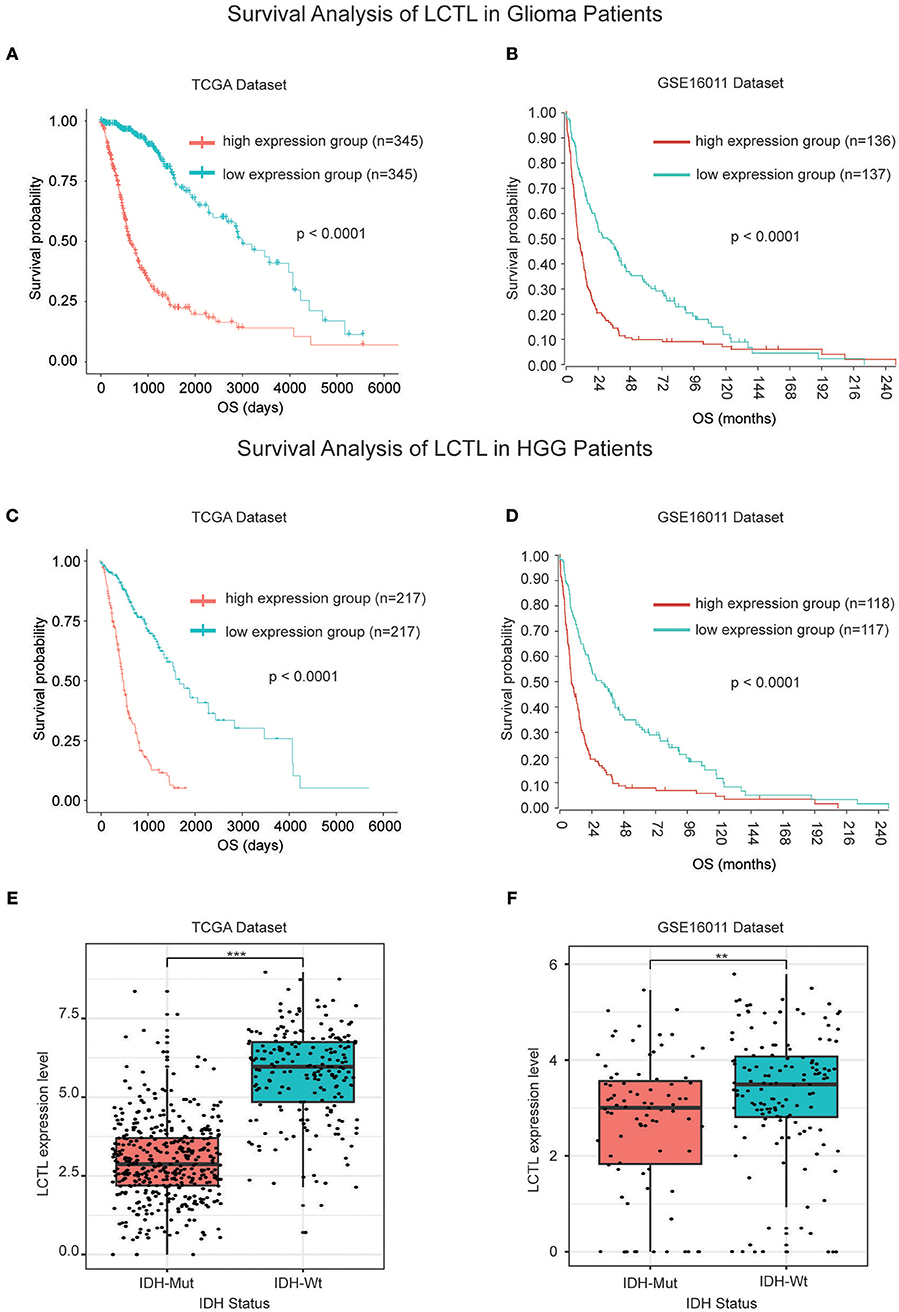
Figure 2. LCTL is a prognostic factor for glioma patients. (A,B) Kaplan–Meier survival analysis showing that high LCTL expression predicts poor prognosis for glioma patients based on both the TCGA and GSE16011 datasets. (C,D) Kaplan–Meier survival analysis showing that high LCTL expression predicts poor prognosis for high grade glioma (HGG) patients in both the TCGA and GSE16011 datasets. (E,F) The expression of LCTL is significantly higher in IDH-Wt gliomas than that in IDH-Mut disease based on both the TCGA and GSE16011 datasets. **P < 0.01, ***P < 0.001, t-test.
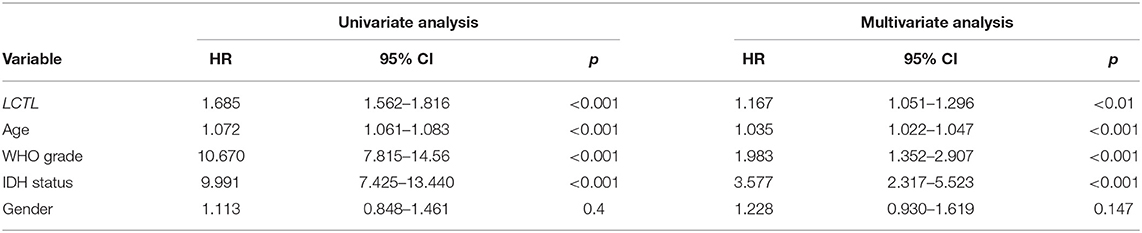
Table 1. Univariate and multivariate analysis of clinical prognostic parameters based on the TCGA Dataset.
Promoter DNA Methylation Regulates LCTL mRNA Expression
It is widely recognized that both genetic alterations (mutations, loss of heterozygosity, deletions, insertions, aneuploidy, etc.) and epigenetic alterations (DNA methylation, non-coding RNAs, transcription factors, etc.) equally contribute to carcinogenesis (31). Whereas, the former perturb normal patterns of gene expression, which is dependent on changes to normal DNA sequences, the latter result in the inappropriate silencing or activation of cancer-associated genes without changing DNA sequences (32). Interestingly, after the analysis of genetic alterations by cBioPortal, we found no genetic alterations including mutations and putative copy-number alterations in LCTL in TCGA Merged Cohort of LGG and GBM (Supplementary Table S2). Next, we investigated epigenetic alterations of LCTL, and especially promoter DNA methylation. After integrating TCGA glioma datasets, we identified 593 patients with both LCTL expression and DNA methylation data. The LCTL DNA methylation beta values at cg00686404 were significantly higher in the low LCTL expression group than in the high LCTL expression group (Figure 3A). Meanwhile, the beta values at cg00686404 were negatively correlated with LCTL mRNA expression (Pearson's r = −0.682, p < 2.2e-16; Figure 3B). Consistently, an analysis of DNA methylation at cg25923629 generated similar results (Figures 3C,D). To further validate our previous results, we next analyzed the predictive value of LCTL promoter DNA methylation for glioma patients and found that patients with high levels had significantly better prognosis than those with low levels (Figures 3E,F, p < 0.0001). In summary, these results indicate that the expression of LCTL is likely regulated by DNA methylation of its promoter, and that this epigenetic modification might also represent a potential prognostic marker for glioma patients.
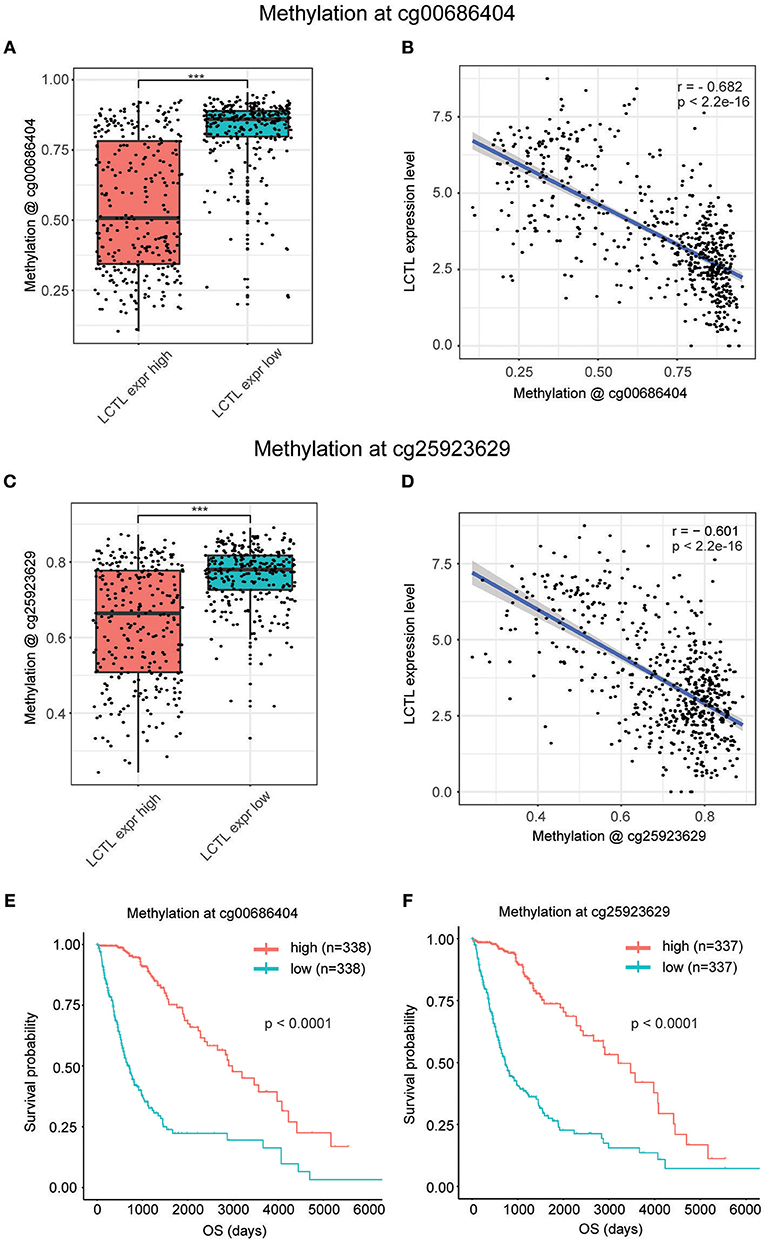
Figure 3. Promoter DNA methylation regulates LCTL mRNA expression and correlates with glioma patient prognosis. (A) Comparison of DNA methylation beta values at cg0686404. (B) Correlation between LCTL mRNA expression and promoter DNA methylation at the probe cg00686404. (C) Comparison of DNA methylation beta values at cg25923629. (D) Correlation between LCTL mRNA expression and promoter DNA methylation at the probe cg25923629. (E) Association between the promoter DNA methylation value of LCTL at cg0686404 overall survival time for glioma patients. (F) Association between the promoter DNA methylation value of LCTL at cg25923629 and overall survival time for glioma patients. ***P < 0.001, t-test.
LCTL Related GO Functional Enrichment in Glioma
To further clarify the biologic role of LCTL in glioma, GO enrichment analysis was performed. First, we analyzed the correlation between LCTL and all other genes in the TCGA dataset by Pearson correlation analysis and in the GSE16011 dataset by R2. Genes with |R| > 0.5 in both data sets were chosen for further analysis. Finally, we obtained 2091 genes and 161 genes from TCGA and GSE16011 gene lists, respectively (Supplementary Table S3). Then, we probed the bio-function of these genes by GO analysis in DAVID Bioinformatics Resources 6.8 (Supplementary Tables S4, S5) and found that genes that were tightly correlated with LCTL expression in TCGA and GSE16011 datasets were enriched in 66 biological processes terms (the top 15 terms are shown in Figure 4A left, p-value < 0.01) and nine terms (Figure 4A right, p-value < 0.01), respectively. When comparing the two lists of GO terms, we found that genes closely related with LCTL were involved in the immune response and inflammatory response for both TCGA and GSE16011 datasets. Regarding molecular function, these genes were mainly enriched in protein binding (Supplementary Figures S1C,D). Moreover, cell component analysis indicated enrichment predominantly occurred at the extracellular region (Supplementary Figures S1E,F), which indicated that these genes might play a vital role in the tumor microenvironment (TME) of glioma. Importantly, the majority of genes that were enriched in immune response and inflammatory response in the TCGA dataset were significantly positively correlated with LCTL expression (Figure 4B and Supplementary Figure S2). Collectively, these findings suggest that LCTL participates in regulating the tumor immune environment.
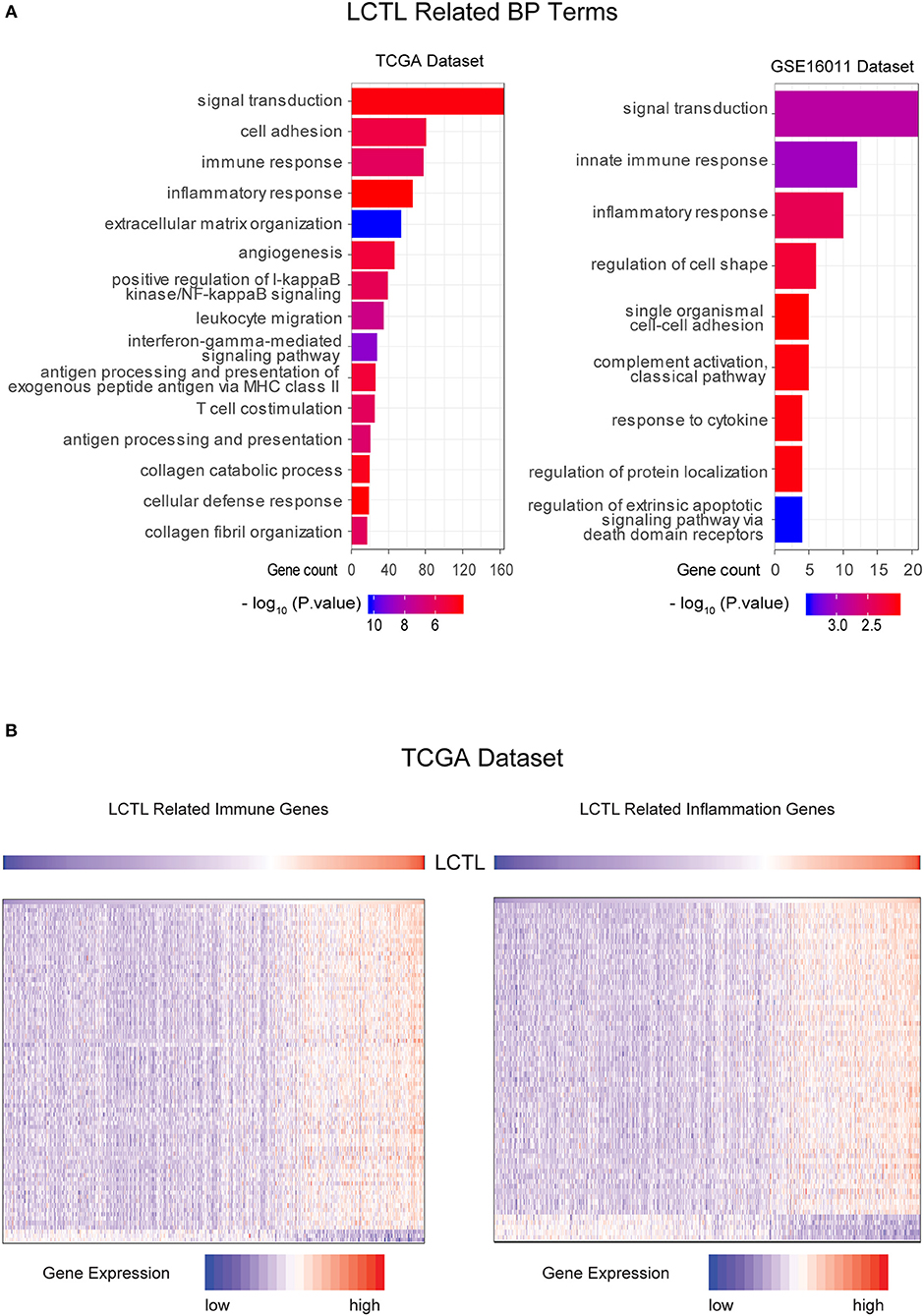
Figure 4. LCTL is related to specific gene ontology (GO) terms in glioma. (A) Results of BF (biological function) terms analyzed by DAVID based on the TCGA and GSE16011datasets. (B) Most immune response and inflammatory response related genes were significantly positively correlated with LCTL.
LCTL Expression Is Correlated With Stromal and Immune Cell Infiltration in Gliomas
Infiltrating stromal and immunes cells, which form the major normal cells component of tumors, play an important role in cancer biology and perturb tumor signaling based on molecular studies (26). Considering the results of GO analysis, we next explored whether LCTL expression is associated with immune infiltration in glioma. First, we examined the association between LCTL expression and ESTIMATE scores. Results revealed that LCTL expression was significantly positively correlated with stromal score, immune score, and ESTIMATE score in both LGG (Figure 5A) and GBM patients (Figure 5B), suggesting that it has a marked influence on stromal and immune cell infiltration. Since the immune and stromal scores were, respectively, generated based on their gene signatures (26), we therefor performed Q-PCR to investigate the association between mRNA expression of LCTL and typical genes of immune and stromal scores in our 45 glioma samples. The results demonstrated that mRNA expression of LCTL significantly correlated with that of selected typical genes (top genes correlated with immune and stromal scores in TCGA GBMLGG cohort, Supplementary Figure S3). To further determine which cell types play a predominant role in this process, we next analyzed the correlation between LCTL expression and 64 non-cancerous cell types, as estimated by xcells, based on TCGA glioma patients. The results showed that there were 39 cell types that significantly correlated with LCTL expression (Figure 5C and Table 2, Spearman's r, BH-adjust p-value < 0.05), among which, 25 types were positively correlated, whereas 14 types were negatively correlated. These cells types comprised seven lymphoid, 11 myeloid cells, eight stromal, five stem, and eight other cell types. Notably, the majority of myeloid cells, stromal cells, stem cells, and other cells were positively correlated with LCTL expression; however, most lymphoid cells were negatively correlated. These findings strongly indicate that LCTL plays a specific role in stromal and immune cell infiltration in gliomas.
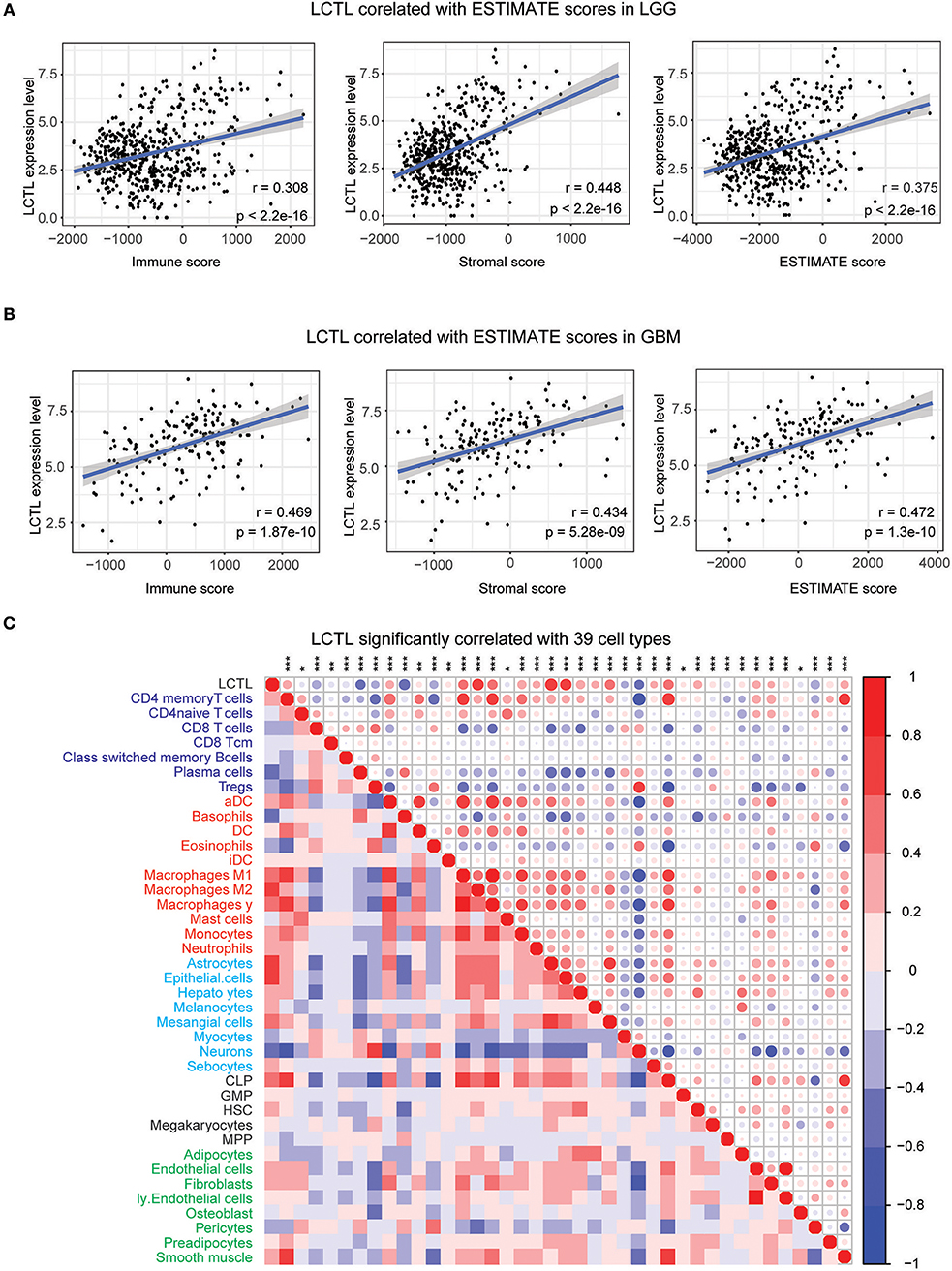
Figure 5. Correlation between LCTL expression and ESTIMATE algorithm/xcells scores in glioma. (A) LCTL expression was positively correlated with immune score, stromal score and ESTIMATE score in lower grade glioma (LGG) patients. (B) LCTL expression was positively correlated with immune score, stromal score and ESTIMATE score in glioblastoma (GBM) patients. (C) LCTL expression was significantly correlated with 39 cell types, as calculated by xcells in glioma. *P < 0.05, **P <0.01, ***P < 0.001.
LCTL Correlates With Immunosuppressive Properties
Since LCTL was found to be positively related to immunosuppressive cells, such as M2 macrophages and neutrophils, among others, but was negatively correlated with anti-tumor immune cells such as CD8+ T cells, we hypothesized that this gene could be involved in the immunosuppressive properties of glioma. To confirm this, we performed correlation analysis of LCTL expression and critical factors that recruit myeloid-derived suppressor cells, tumor-associated macrophages (TAMs), and tumor-associated neutrophils, as well as the immunosuppressive factors secreted by these cells (Figures 6A,B). LCTL was found to be significantly positively correlated with the majority of immunosuppressive cell recruitment factors [reviewed in (5)] and immunosuppressive factors [reviewed in (5, 33)]. TAMs can be divided into the “classically activated” M1 phenotype and the “alternatively activated” M2 phenotype (34). It is the M2 phenotype of TAMs that contributes to the immunosuppressive tumor environment (35). Indeed, key factors [CSF-1,CCL2, IL-4, IL-6, IL-10, TGFβ, reviewed in (5)] that drive M2 phenotype differentiation were found to be closely related to LCTL expression (Figure 6C). It is well-established that T cell exhaustion occurs in humans with cancer and that exhausted T cells in the TME lead to cancer immune evasion (36). Exhausted T cells express high levels of inhibitory receptors including PD-1, CTLA-4, TIM-3, LAG-3, BTLA, and TIGIT [reviewed in (36)]. To further substantiate these findings, we next analyzed the correlation between LCTL and inhibitory receptors of exhausted T cells (Figure 6D). Our GO enrichment analysis suggested that LCTL might be involved in TME, from which various pro-invasion signals can control EMT (37). Further, LCTL can promote EMT, as recently reported for bladder cancer (21). Consistently, we found significant correlations between LCTL and common EMT biomarkers, except CDH1 and KRT1 (Figure 6E). Specifically, LCTL expression was positively related to mesenchymal cell markers and negatively correlated with epithelial cell markers. Furthermore, we selected 14 genes, which showed the most correlation with LCTL in the TCGA dataset, from abovementioned immunosuppressive factors to validate the correlation between LCTL and immunosuppressive properties in our 45 glioma samples by Q-PCR. The results show that LCTL expression significantly correlated with the expression of these 14 genes at mRNA level (Supplementary Figure S4). Importantly, we found that LCTL can directly interact with several proteins, especially FGFRs and FGFs, via PPI analysis (Supplementary Figure S5A). Interestingly, via associations with FGFs and FGFRs, LCTL could indirectly interact with immunosuppressive factors, recruitment factors, M2 phenotype-driving factors, and markers of exhausted T cells and EMT (Figure 6F and Supplementary Figure S5B). Therefore, our study identifies LCTL as a regular of the TME in glioma, which functions by recruiting and promoting immunosuppressive cells to secrete immunosuppressive factors, in addition to regulating M2 transformation, T cell exhaustion, and EMT via the FGF signaling pathway; this is thought to ultimately facilitate tumor immune evasion, resulting in poor outcomes for glioma patients.
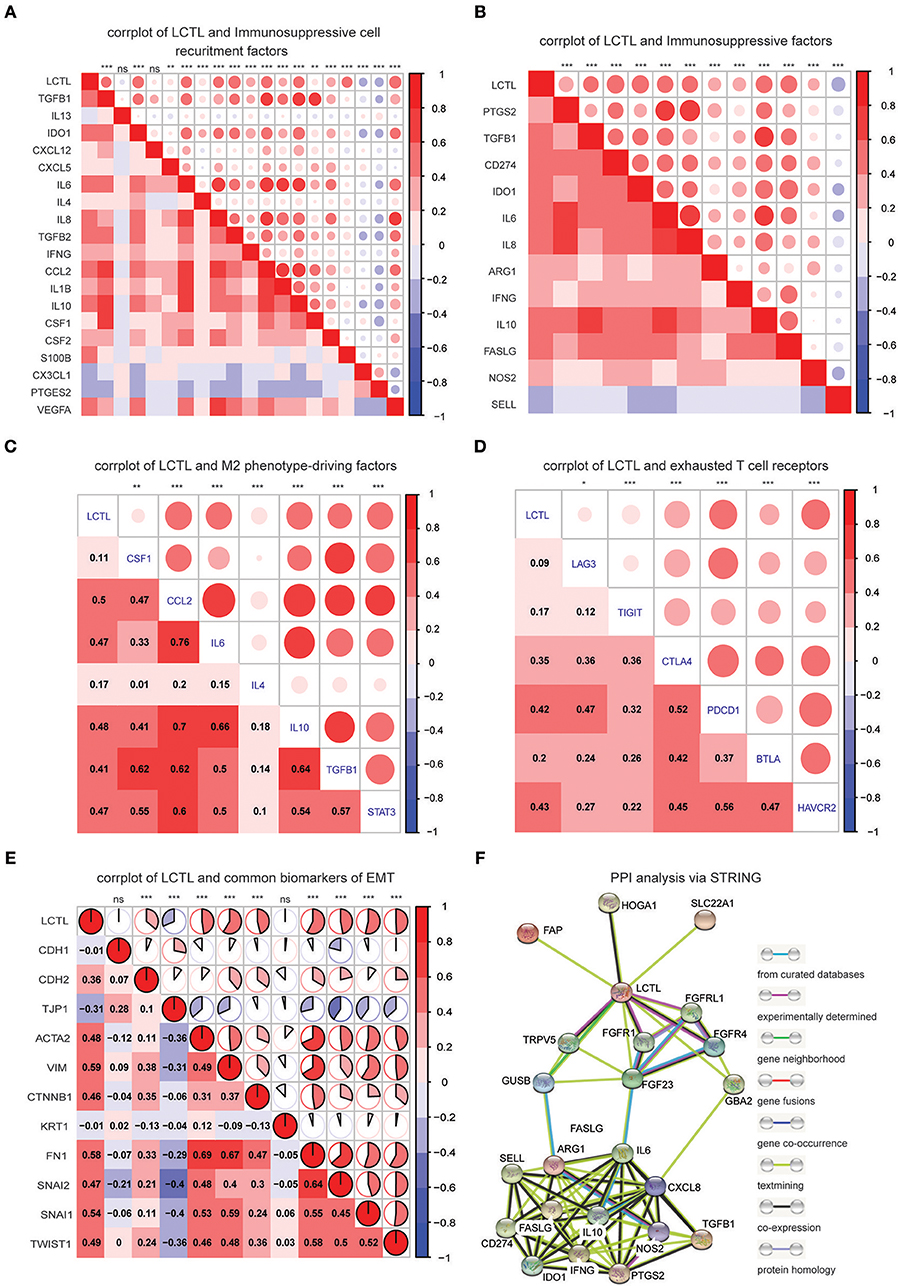
Figure 6. LCTL correlates with immunosuppressive properties and promotes epithelial-mesenchymal transition (EMT). (A) Correlation between LCTL and immunosuppressive cell recruitment factors. (B) Correlation between LCTL and immunosuppressive factors secreted by myeloid-derived suppressor cells (MDSCs), tumor-associated macrophages (TAMs) and tumor-associated neutrophils (TANs). (C) Correlation between LCTL and M2 phenotype-driving factors. (D) Correlation between LCTL and exhausted T cell receptors. (E) Correlation between LCTL and common biomarkers of epichelial-mesenchymal transition (EMT). (F) Protein-protein interaction (PPI) analysis of the network linking LCTL and immunosuppressive factors. *P < 0.05, **P <0.01, ***P < 0.001, nsP > 0.05.
Discussion
Glioma patient prognosis remains poor, which is clinically frustrating (3, 4). Even with intensive therapies, most GBM patients relapse very soon due to the highly aggressive nature of this disease. Recently, immunotherapy has shown promise for the treatment of many cancers including glioma. However, the widespread application of immunotherapy is difficult, which is attributed to autoimmune-like side effects (38, 39). One potential therapeutic strategy is to develop immunotherapy against glioma by targeting overexpressed proteins that play an essential role in immunosuppression. Our present study first identified a novel function for LCTL in promoting aggressive behavior and leading to immune evasion.
Originally, Klotho, the first identified member of the Klotho family, was discovered as an anti-aging gene in 1997 (8). Recently, both Klotho and βKlotho have been reported to be aberrantly expressed in several cancers (40). Although some studies have demonstrated the abnormal expression of LCTL in different cancers, its function was previously unclear. In this study, we found that the expression of LCTL is not only abnormally upregulated, but also significantly associated with WHO grade, molecular subtype, and IDH status in glioma. Furthermore, our study showed that LCTL is an independent prognostic marker for glioma patients and that high levels of LCTL expression predict poor outcome. DNA methylation, the main epigenetic modification, is also involved in the pathogenesis of cancer (41, 42). Accordingly, the methylation of promoters of certain genes involved in key biological pathways in glioma has been widely reported (43). For example, promoter methylation of the O6-methylguanine-DNA methyltransferase (MGMT) gene occurs in ~40% of glioma patients and is clinically used as a biomarker of response to alkylating agents (44). Because DNA methylation is potentially reversible, it is a potential target for cancer treatment. Actually, the Food and Drug Administration (FDA) has approved DNA methylation inhibitors and histone deacetylase inhibitors for cancer monotherapy (45). Here, we found that the endogenous expression of LCTL is regulated by promoter DNA methylation rather than genetic alterations in glioma. Moreover, LCTL promoter methylation levels were found to have predictive value for glioma patients, which is similar to results found for mRNA levels. Taken together, LCTL is a good candidate prognostic and therapeutic target for glioma patient.
Immunotherapy is rapidly becoming the newest and the most promising pillar of malignancy treatment, with the potential to harness the potency of and active the host immune system. However, the TME including stromal cells, inflammatory cells, vasculature, and ECM, usually prevents effective lymphocyte initiation, reduces their infiltration, and suppresses infiltrating effector cells, which contributes to host failure in rejecting tumors (46). It is thus of great importance to explore novel molecular biomarkers and targets that play critical roles in the TME. Here, we conducted GO analysis with two different large datasets, and the results strongly suggested that LCTL is involved in the regimenting immunity and the TME in glioma. Furthermore, we found that LCTL expression significantly correlates with non-cancerous cells, mainly stromal and immune cells, in glioma via two methods, namely the ESTIMATE algorithm and xcell. Interestingly, these infiltratimg cells such as M2 macrophages and neutrophils, among others, are mainly considered immunosuppressive cells based on previous studies. Moreover, the correlation between LCTL expression and marker genes encoding immunoregulatory factors, as well as exhausted T cells, imply a role for LCTL in regulating tumor immunology in glioma. On the one hand, LCTL expression was significantly positively correlated with cytokines or chemokines that recruit immunosuppressive cells, such as myeloid-derived suppressor cells, TAMs, and tumor-associated neutrophils, as well as immunosuppressive factors secreted by these cells. On the other hand, LCTL was found to be positively associated with factors that drive M2 phenotype differentiation, which suggests a potential regulatory role for LCTL in TAMs polarization. In addition, our results indicate that LCTL can induce T cell exhaustion. Recently, accumulating evidence has demonstrated that cross-talk between EMT-associated factors and the TME might facilitate tumor immune escape (47). Accordingly, LCTL was found to promote bladder cancer EMT (21). In glioma, we also found that LCTL expression is positively related to mesenchymal cell markers and negatively correlated with epithelial cell markers. Thus, we propose that this gene might be involved in regulation of the immunosuppressive microenvironment by recruiting and promoting immunosuppressive cells to secrete immunosuppressive factors, regulating M2 polarization, promoting EMT, and inducing T cell exhaustion in glioma, finally facilitating tumor immune evasion.
Mechanistically, LCTL might regulate the immunity in glioma through FGF signaling pathway. FGFR dysfunction is widely found in cancers and FGF biology is involved in many effects in a myriad of cell types, and is a key component of the tumor environment (48). In addition, increasing evidence suggests that FGF signaling can influence the recruitment and activity of TAMs. For example, the activation of inducible FGFR1 in mammary cells causes their transformation and induces the secretion of factors to recruit TAMs (49, 50). Furthermore, FGF2 secreted from esophageal cancer cells promotes macrophage migration and survival through FGFR1 signaling (51). Moreover, by cooperating with proinflammatory cytokines, FGF2 can also promote the expression of adhesion molecules by endothelial cells, thus contributing to the recruitment of circulating immune cells to the tumor (52). Indeed, a recent study demonstrated that Klotho regulates immune invasion in the cenral nervous system and inhibits thioredoxin-interacting protein-dependent activation of the NLRP3 inflammasome in macrophages via FGF23 signaling (53). It has also been determined that LCTL can interact efficiently with FGFR1b, FGFR-1c, FGFR2c, and FGFR-4 (11). In our study, we found that LCTL can interact with FGFR1, FGFRL1, FGFR4, and FGF23 through PPI analysis. Interestingly, this analysis also revealed that LCTL plays an immunoregulatory role that is dependent on FGF signaling.
Based on our findings, we propose a working modal wherein LCTL, which is highly expressed in glioma and regulated by DNA methylation at its promoter, facilitates the recruitment of and immunosuppressive cells and promote the secretion of immunosuppressive factors by these cells. This further regulates M2 transformation, T cell exhaustion in glioma, and EMT, finally augmenting tumor immune evasion (Figure 7). Our findings provide opportunities to explore novel therapeutic approaches based on the epigenetic targeting of LCTL. Further, this gene might be an ideal target for immunotherapy against gliomas.
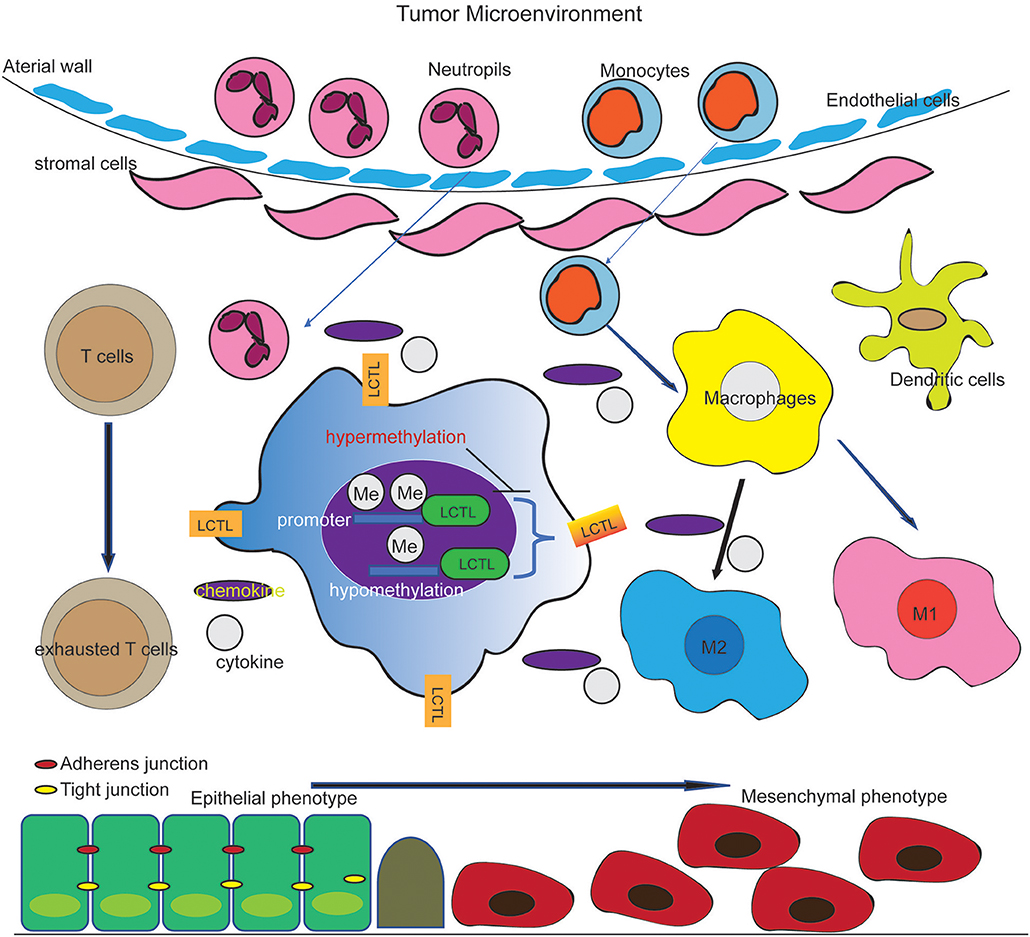
Figure 7. Working model of the effect of LCTL in the glioma microenvironment. LCTL is highly expressed in glioma, regulated by DNA promotor methylation and acts an immunosuppressive role via interacting with some immunosuppressive factors in glioma TME.
Data Availability Statement
Publicly available datasets were analyzed in this study. This data can be found here: https://xenabrowser.net/datapages/?cohort=TCGA%20lower%20grade%20glioma%20and%20glioblastoma%20(GBMLGG)&removeHub=https%3A%2F%2Fxena.treehouse.gi.ucsc.edu%3A443,GSE16011.
Ethics Statement
The studies involving human participants were reviewed and approved by Ethics Committee of Xiangya Hospital. The patients/participants provided their written informed consent to participate in this study.
Author Contributions
JS analyzed the data, performed computational coding, and wrote the manuscript. QM and WL involved in design of study, manuscript editing. HT performed the Q-PCR experiments and analyzed the results. CW, ML, XW, KX, YL, QX, CZ, and HL collected the data and samples. QL involved in design of study, manuscript review and editing, supervision of the entire work. All authors had approved the final manuscript.
Funding
This study was supported by grants from the National Natural Science Foundation of China (No. 81802974).
Conflict of Interest
The authors declare that the research was conducted in the absence of any commercial or financial relationships that could be construed as a potential conflict of interest.
Acknowledgments
We gratefully thank China Scholarship Council for supporting JS (201706370050).
Supplementary Material
The Supplementary Material for this article can be found online at: https://www.frontiersin.org/articles/10.3389/fonc.2019.01083/full#supplementary-material
References
1. Ostrom QT, Gittleman H, Liao P, Vecchione-Koval T, Wolinsky Y, Kruchko C, et al. CBTRUS Statistical Report: Primary brain and other central nervous system tumors diagnosed in the United States in 2010-2014. Neuro Oncol. (2017) 19(suppl_5), v1–88. doi: 10.1093/neuonc/nox158
2. Yi X, Cao H, Tang H, Gong G, Hu Z, Liao W, et al. Gliosarcoma: a clinical and radiological analysis of 48 cases. Eur Radiol. (2019) 29:429–38. doi: 10.1007/s00330-018-5398-y
3. Wen PY, Kesari S. Malignant gliomas in adults. N Engl J Med. (2008) 359:492–507. doi: 10.1056/NEJMra0708126
4. Stupp R, Hegi ME, Mason WP, van den Bent MJ, Taphoorn MJ, Janzer RC, et al. Effects of radiotherapy with concomitant and adjuvant temozolomide versus radiotherapy alone on survival in glioblastoma in a randomised phase III study: 5-year analysis of the EORTC-NCIC trial. Lancet Oncol. (2009) 10:459–66. doi: 10.1016/S1470-2045(09)70025-7
5. Ma Q, Long W, Xing C, Chu J, Luo M, Wang HY, et al. Cancer stem cells and immunosuppressive microenvironment in glioma. Front Immunol. (2018) 9:2924. doi: 10.3389/fimmu.2018.02924
6. Huang J, Liu F, Liu Z, Tang H, Wu H, Gong Q, et al. Immune checkpoint in glioblastoma: promising and challenging. Front Pharmacol. (2017) 8:242. doi: 10.3389/fphar.2017.00242
7. Lapointe S, Perry A, Butowski NA. Primary brain tumours in adults. Lancet. (2018) 392:432–46. doi: 10.1016/S0140-6736(18)30990-5
8. Kuro-o M, Matsumura Y, Aizawa H, Kawaguchi H, Suga T, Utsugi T, et al. Mutation of the mouse klotho gene leads to a syndrome resembling ageing. Nature. (1997) 390:45–51. doi: 10.1038/36285
9. Trost N, Pena-Llopis S, Koirala S, Stojan J, Potts PR, Fon Tacer K, et al. gammaKlotho is a novel marker and cell survival factor in a subset of triple negative breast cancers. Oncotarget. (2016) 7:2611–28. doi: 10.18632/oncotarget.6006
10. Ito S, Fujimori T, Hayashizaki Y, Nabeshima Y. Identification of a novel mouse membrane-bound family 1 glycosidase-like protein, which carries an atypical active site structure. Biochim Biophys Acta. (2002) 1576:341–5. doi: 10.1016/S0167-4781(02)00281-6
11. Fon Tacer K, Bookout AL, Ding X, Kurosu H, John GB, Wang L, et al. Research resource: Comprehensive expression atlas of the fibroblast growth factor system in adult mouse. Mol Endocrinol. (2010) 24:2050–64. doi: 10.1210/me.2010-0142
12. Tanner Y, Grose RP. Dysregulated FGF signalling in neoplastic disorders. Semin Cell Dev Biol. (2016) 53:126–35. doi: 10.1016/j.semcdb.2015.10.012
13. Katoh M. Therapeutics targeting fgf signaling network in human diseases. Trends Pharmacol Sci. (2016) 37:1081–96. doi: 10.1016/j.tips.2016.10.003
14. Ligumsky H, Rubinek T, Merenbakh-Lamin K, Yeheskel A, Sertchook R, Shahmoon S, et al. Tumor suppressor activity of klotho in breast cancer is revealed by structure-function analysis. Mol Cancer Res. (2015) 13:1398–407. doi: 10.1158/1541-7786.MCR-15-0141
15. Chen B, Wang X, Zhao W, Wu J. Klotho inhibits growth and promotes apoptosis in human lung cancer cell line A549. J Exp Clin Cancer Res. (2010) 29:99. doi: 10.1186/1756-9966-29-99
16. Shu G, Xie B, Ren F, Liu DC, Zhou J, Li Q, et al. Restoration of klotho expression induces apoptosis and autophagy in hepatocellular carcinoma cells. Cell Oncol. (2013) 36:121–9. doi: 10.1007/s13402-012-0118-0
17. Poh W, Wong W, Ong H, Aung MO, Lim SG, Chua BT, et al. Klotho-beta overexpression as a novel target for suppressing proliferation and fibroblast growth factor receptor-4 signaling in hepatocellular carcinoma. Mol Cancer. (2012) 11:14. doi: 10.1186/1476-4598-11-14
18. Wolf I, Levanon-Cohen S, Bose S, Ligumsky H, Sredni B, Kanety H, et al. Klotho: a tumor suppressor and a modulator of the IGF-1 and FGF pathways in human breast cancer. Oncogene. (2008) 27:7094–105. doi: 10.1038/onc.2008.292
19. Liu H, Fergusson MM, Castilho RM, Liu J, Cao L, Chen J, et al. Augmented Wnt signaling in a mammalian model of accelerated aging. Science. (2007) 317:803–6. doi: 10.1126/science.1143578
20. Ye X, Guo Y, Zhang Q, Chen W, Hua X, Liu W, et al. betaKlotho suppresses tumor growth in hepatocellular carcinoma by regulating Akt/GSK-3beta/cyclin D1 signaling pathway. PLoS ONE. (2013) 8:e55615. doi: 10.1371/journal.pone.0055615
21. Hori S, Miyake M, Tatsumi Y, Morizawa Y, Nakai Y, Onishi S, et al. Gamma-Klotho exhibits multiple roles in tumor growth of human bladder cancer. Oncotarget. (2018) 9:19508–24. doi: 10.18632/oncotarget.24628
22. Tang Z, Li C, Kang B, Gao G, Li C, Zhang Z. GEPIA: a web server for cancer and normal gene expression profiling and interactive analyses. Nucleic Acids Res. (2017) 45:W98–102. doi: 10.1093/nar/gkx247
23. Li B, Severson E, Pignon JC, Zhao H, Li T, Novak J, et al. Comprehensive analyses of tumor immunity: implications for cancer immunotherapy. Genome Biol. (2016) 17:174. doi: 10.1186/s13059-016-1028-7
24. Gao J, Aksoy BA, Dogrusoz U, Dresdner G, Gross B, Sumer SO, et al. Integrative analysis of complex cancer genomics and clinical profiles using the cBioPortal. Sci Signal. (2013) 6:pl1. doi: 10.1126/scisignal.2004088
25. Jiao X, Sherman BT, Huang da W, Stephens R, Baseler MW, Lane HC, et al. DAVID-WS: a stateful web service to facilitate gene/protein list analysis. Bioinformatics. (2012) 28:1805–6. doi: 10.1093/bioinformatics/bts251
26. Yoshihara K, Shahmoradgoli M, Martinez E, Vegesna R, Kim H, Torres-Garcia W, et al. Inferring tumour purity and stromal and immune cell admixture from expression data. Nat Commun. (2013) 4:2612. doi: 10.1038/ncomms3612
27. Aran D, Hu Z, Butte AJ. xCell: digitally portraying the tissue cellular heterogeneity landscape. Genome Biol. (2017) 18:220. doi: 10.1186/s13059-017-1349-1
28. Yan H, Parsons DW, Jin G, McLendon R, Rasheed BA, Yuan W, et al. IDH1 and IDH2 mutations in gliomas. N Engl J Med. (2009) 360:765–73. doi: 10.1056/NEJMoa0808710
29. Hartmann C, Hentschel B, Tatagiba M, Schramm J, Schnell O, Seidel C, et al. Molecular markers in low-grade gliomas: predictive or prognostic? Clin Cancer Res. (2011) 17:4588–99. doi: 10.1158/1078-0432.CCR-10-3194
30. Komori T. The 2016 WHO classification of tumours of the central nervous system: the major points of revision. Neurol Med Chir. (2017) 57:301–11. doi: 10.2176/nmc.ra.2017-0010
31. Kagohara LT, Stein-O'Brien GL, Kelley D, Flam E, Wick HC, Danilova LV, et al. Epigenetic regulation of gene expression in cancer: techniques, resources and analysis. Brief Funct Genomics. (2018) 17:49–63. doi: 10.1093/bfgp/elx018
32. Herceg Z, Hainaut P. Genetic and epigenetic alterations as biomarkers for cancer detection, diagnosis and prognosis. Mol Oncol. (2007) 1:26–41. doi: 10.1016/j.molonc.2007.01.004
33. Motz GT, Coukos G. Deciphering and reversing tumor immune suppression. Immunity. (2013) 39:61–73. doi: 10.1016/j.immuni.2013.07.005
34. Mantovani A, Locati M. Tumor-associated macrophages as a paradigm of macrophage plasticity, diversity, and polarization: lessons and open questions. Arterioscler Thromb Vasc Biol. (2013) 33:1478–83. doi: 10.1161/ATVBAHA.113.300168
35. Hambardzumyan D, Gutmann DH, Kettenmann H. The role of microglia and macrophages in glioma maintenance and progression. Nat Neurosci. (2016) 19:20–7. doi: 10.1038/nn.4185
36. Jiang Y, Li Y, Zhu B. T-cell exhaustion in the tumor microenvironment. Cell Death Dis. (2015) 6:e1792. doi: 10.1038/cddis.2015.162
37. Jung HY, Fattet L, Yang J. Molecular pathways: linking tumor microenvironment to epithelial-mesenchymal transition in metastasis. Clin Cancer Res. (2015) 21:962–8. doi: 10.1158/1078-0432.CCR-13-3173
38. Han SJ, Zygourakis C, Lim M, Parsa AT Immunotherapy for glioma: promises and challenges. Neurosurg Clin N Am. (2012) 23:357–70. doi: 10.1016/j.nec.2012.05.001
39. Anderson AC. Tim-3: an emerging target in the cancer immunotherapy landscape. Cancer Immunol Res. (2014) 2:393–8. doi: 10.1158/2326-6066.CIR-14-0039
40. Zhou X, Wang X. Klotho: a novel biomarker for cancer. J Cancer Res Clin Oncol. (2015) 141:961–9. doi: 10.1007/s00432-014-1788-y
41. Zeng WJ, Yang YL, Liu ZZ, Wen ZP, Chen YH, Hu XL, et al. Integrative analysis of DNA methylation and gene expression identify a three-gene signature for predicting prognosis in lower-grade gliomas. Cell Physiol Biochem. (2018) 47:428–39. doi: 10.1159/000489954
42. Baylin SB, Jones PA. A decade of exploring the cancer epigenome - biological and translational implications. Nat Rev Cancer. (2011) 11:726–34. doi: 10.1038/nrc3130
43. Feng J, Zhang Y, She X, Sun Y, Fan L, Ren X, et al. Hypermethylated gene ANKDD1A is a candidate tumor suppressor that interacts with FIH1 and decreases HIF1alpha stability to inhibit cell autophagy in the glioblastoma multiforme hypoxia microenvironment. Oncogene. (2019) 38:103–19. doi: 10.1038/s41388-018-0423-9
44. Esteller M, Garcia-Foncillas J, Andion E, Goodman SN, Hidalgo OF, Vanaclocha V, et al. Inactivation of the DNA-repair gene MGMT and the clinical response of gliomas to alkylating agents. N Engl J Med. (2000) 343:1350–4. doi: 10.1056/NEJM200011093431901
45. Raynal NJ, Da Costa EM, Lee JT, Gharibyan V, Ahmed S, Zhang H, et al. Repositioning FDA-approved drugs in combination with epigenetic drugs to reprogram colon cancer epigenome. Mol Cancer Ther. (2017) 16:397–407. doi: 10.1158/1535-7163.MCT-16-0588
46. Tang H, Qiao J, Fu YX. Immunotherapy and tumor microenvironment. Cancer Lett. (2016) 370:85–90. doi: 10.1016/j.canlet.2015.10.009
47. Terry S, Savagner P, Ortiz-Cuaran S, Mahjoubi L, Saintigny P, Thiery JP, et al. New insights into the role of EMT in tumor immune escape. Mol Oncol. (2017) 11:824–46. doi: 10.1002/1878-0261.12093
48. Clayton NS, Wilson AS, Laurent EP, Grose RP, Carter EP. Fibroblast growth factor-mediated crosstalk in cancer etiology and treatment. Dev Dyn. (2017) 246:493–501. doi: 10.1002/dvdy.24514
49. Schwertfeger KL, Xian W, Kaplan AM, Burnett SH, Cohen DA, Rosen JM. A critical role for the inflammatory response in a mouse model of preneoplastic progression. Cancer Res. (2006) 66:5676–85. doi: 10.1158/0008-5472.CAN-05-3781
50. Bohrer LR, Schwertfeger KL. Macrophages promote fibroblast growth factor receptor-driven tumor cell migration and invasion in a CXCR2-dependent manner. Mol Cancer Res. (2012) 10:1294–305. doi: 10.1158/1541-7786.MCR-12-0275
51. Takase N, Koma Y, Urakawa N, Nishio M, Arai N, Akiyama H, et al. NCAM- and FGF-2-mediated FGFR1 signaling in the tumor microenvironment of esophageal cancer regulates the survival and migration of tumor-associated macrophages and cancer cells. Cancer Lett. (2016) 380:47–58. doi: 10.1016/j.canlet.2016.06.009
52. Zittermann SI, Issekutz AC. Basic fibroblast growth factor (bFGF, FGF-2) potentiates leukocyte recruitment to inflammation by enhancing endothelial adhesion molecule expression. Am J Pathol. (2006) 168:835–46. doi: 10.2353/ajpath.2006.050479
Keywords: Klotho, LCTL, immune infiltration, methylation, FGF signaling, glioma
Citation: Su J, Ma Q, Long W, Tang H, Wu C, Luo M, Wang X, Xiao K, Li Y, Xiao Q, Zhang C, Li H and Liu Q (2019) LCTL Is a Prognostic Biomarker and Correlates With Stromal and Immune Infiltration in Gliomas. Front. Oncol. 9:1083. doi: 10.3389/fonc.2019.01083
Received: 09 July 2019; Accepted: 01 October 2019;
Published: 15 October 2019.
Edited by:
Liam Chen, Johns Hopkins University, United StatesReviewed by:
Jun Wei, University of Texas MD Anderson Cancer Center, United StatesHan Shen, Westmead Institute for Medical Research, Australia
Copyright © 2019 Su, Ma, Long, Tang, Wu, Luo, Wang, Xiao, Li, Xiao, Zhang, Li and Liu. This is an open-access article distributed under the terms of the Creative Commons Attribution License (CC BY). The use, distribution or reproduction in other forums is permitted, provided the original author(s) and the copyright owner(s) are credited and that the original publication in this journal is cited, in accordance with accepted academic practice. No use, distribution or reproduction is permitted which does not comply with these terms.
*Correspondence: Qing Liu, bGl1cWluZ2RyQGNzdS5lZHUuY24=