- 1Department of Cancer Control Research, British Columbia Cancer Research Centre, Vancouver, BC, Canada
- 2Faculty of Dentistry, University of British Columbia, Vancouver, BC, Canada
- 3Department of Integrative Oncology, British Columbia Cancer Research Centre, Vancouver, BC, Canada
- 4Faculty of Dentistry, Dalhousie University, Halifax, NS, Canada
- 5Department of Pulmonology and CIC-CRB 1404, Rouen University Hospital, Rouen, France
- 6International Research Center—A.C.Camargo Cancer Center, São Paulo, Brazil
Head and neck squamous cell carcinoma (HNSCC) has a poor survival rate mainly due to late stage diagnosis and recurrence. Despite genomic efforts to identify driver mutations and changes in protein-coding gene expression, developing effective diagnostic and prognostic biomarkers remains a priority to guide disease management and improve patient outcome. Recent reports of previously-unannotated microRNAs (miRNAs) from multiple somatic tissues have raised the possibility of HNSCC-specific miRNAs. In this study, we applied a customized in-silico analysis pipeline to identify novel miRNAs from raw small-RNA sequencing datasets from public repositories. We discovered 146 previously-unannotated sequences expressed in head and neck samples that share structural properties highly characteristic of miRNAs. The combined expression of the novel miRNAs revealed tissue and context-specific patterns. Furthermore, comparison of tumor with non-malignant tissue samples (n = 43 pairs) revealed 135 of these miRNAs as differentially expressed, most of which were overexpressed or exclusively found in tumor samples. Additionally, a subset of novel miRNAs was significantly associated with HPV infection status and patient outcome. A prognostic-model combining novel and known miRNA was developed (multivariate Cox regression analysis) leading to an improved death and relapse risk stratification (log rank p < 1e-7). The presence of these miRNAs was corroborated both in an independent dataset and by RT-qPCR analysis, supporting their potential involvement in HNSCC. In this study, we report the discovery of 146 novel miRNAs in head and neck tissues and demonstrate their potential biological significance and clinical relevance to head and neck cancer, providing a new resource for the study of HNSCC.
Introduction
Head and neck squamous cell carcinoma (HNSCC) is the eighth most common cancer worldwide (1) and has a poor survival rate, mainly due to late stage diagnosis, and frequent disease recurrence (2). Despite advances in surgical techniques, chemotherapy, radiation therapy, and targeted therapy, the 5-years survival rate of patients remains at 50% (3). Hence there is a need to expand the repertoire of head and neck specific diagnostic and prognostic biomarkers. Furthermore, in order to improve patient outcome a better understanding of the genetic and epigenetic events associated with disease progression are needed.
MicroRNAs (miRNAs) are a class of single-stranded small non-coding RNAs (sncRNAs) ~21–23 nucleotides in length, which act as regulators of gene expression by binding to complementary sequences within mRNAs (4). A single miRNA transcript can act on multiple mRNA targets, and therefore, miRNAs are involved in many biological and pathological processes. In fact, miRNA dysregulation has been shown as a frequent and important event across all stages of cancer (5–8), as well as in many different cancer types (9–15). Their stability in biofluids and tissue biopsies presents opportunities for biomarker discovery (4, 16) and subsequently drug target detection (17–19). Among the dysregulated miRNAs in HNSCC, miR-21, miR-34, miR-93, miR-155, miR-196, and miR-211 are the most studied (20). Functional assays and target prediction have demonstrated that these miRNAs play important roles in regulation of cell proliferation, immune invasion, and resistance to cell death (21–24), corroborating their role as regulators in HNSCC (20, 25). Furthermore, miRNAs have demonstrated utility as biomarkers in the diagnosis and prognostication of HNSCC. For example, under-expression of let-7d and miR-205 are associated with poor survival in HNSCC (26), and circulating miR-142, miR-186, miR-195, miR-374b, and miR-574 have been shown to be promising markers for monitoring therapy in HNSCC patients (27).
While current miRNA repositories contain ~2,500 unique miRNA sequences, they are primarily comprised of those that are either conserved across several tissues or abundantly expressed, for the most part discounting lineage- and tissue-specific miRNAs (28). However, recent studies show that numerous miRNAs may be expressed only in specific tissues or contexts (29–33), and may have utility as clinical markers of disease (8, 34).
Mining of large-scale datasets using bioinformatic algorithms has become an important tool for expanding the current annotation of miRNA repositories and discovering these tissue/context-specific miRNAs, particularly due to the data's high coverage depth and sample size. The discovery of novel miRNAs not only provides a novel resource for the research community, but may also guide future clinical efforts on the design of new drug targets and disease biomarkers. Thus, we hypothesize the existence of previously-unannotated and tissue-specific miRNAs in head and neck samples, which may have been overlooked due to their tissue/context specificity. In this study, we use a large-scale analysis of high-throughput sequencing data to uncover these novel miRNAs and explore their relevance to HNSCC tumourigenesis.
Materials and Methods
Clinical Data Sets
A discovery cohort consisting of publicly available high-throughput raw small-RNA sequencing data from 523 tumors along with 43 paired non-malignant samples was retrieved from The Cancer Genome Atlas (TCGA) on the cgHUB data repository (dbgap Project ID: 6208), available at: https://cancergenome.nih.gov/ (accessed October 2018). Clinical information on the cases, summarized in Table 1, was obtained from the University of California Santa Cruz Xena Browser, available at: https://xenabrowser.net/ (accessed August 2018). HPV status was obtained from the Cancer Genome Atlas Network (35).
Publicly available small-RNA sequencing data from an independent cohort (n = 20) of oral squamous cell carcinoma samples were obtained from the Gene Expression Omnibus (GEO) repository (Accession GSE52663) (36).
Validation was carried out using formalin-fixed paraffin-embedded (FFPE) tissue from 25 oral squamous cell carcinoma (OSCC) tumors and 5 non-malignant oral tissue samples.
Data Processing and Novel MicroRNA Discovery
The data were analyzed using a customized in-silico analysis pipeline. The study design is summarized in Figure 1, and the data subsets used for the step-wise comparisons that were conducted are summarized in Table 2.
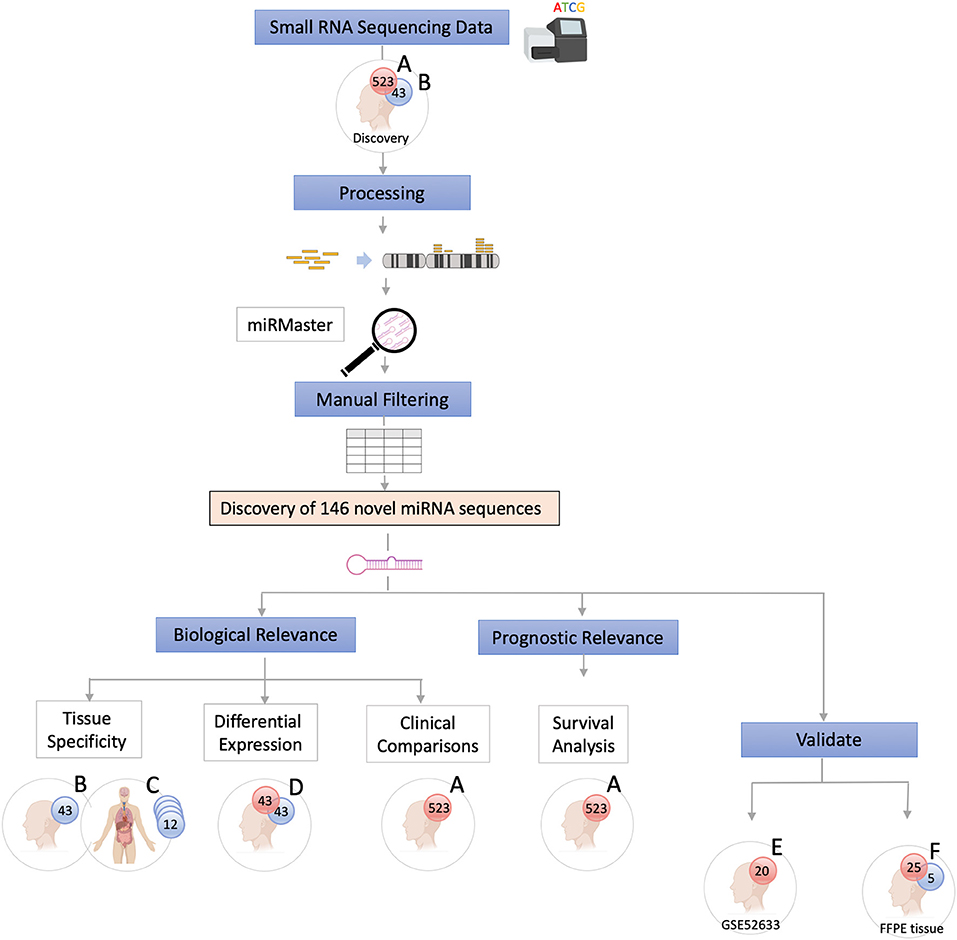
Figure 1. Study Flow Chart. High throughput small RNA-sequencing data from head and neck squamous cell carcinoma (HNSCC) (n = 523, dataset A) and matched non-malignant tissue (n = 43, dataset B) were obtained from The Cancer Genome Atlas (TCGA). Raw sequence data (BAM files) were converted into unaligned reads (FASTQ) and inputted into miRMaster for miRNA detection and quantification. A threshold criteria of ≥1 read per million (RPM) in ≥10% of samples per group was employed. To determine whether these novel sequences have potential biological relevance group comparison and association analyses were performed. Tissue specificity of the novel candidate sequences was assessed by comparing non-malignant samples (dataset B) with those from 12 other non-malignant tissue types from TCGA Pan-Cancer Atlas (dataset C) using non-linear t-Distributed Stochastic Neighbor Embedding. Differentially expressed novel miRNAs were detected by comparing tumor and matched non-malignant samples (dataset D). Clinicopathological features of the novel miRNA transcripts (n = 130) that were found to be expressed exclusively in tumor samples (dataset A) were compared. Survival analysis was performed to further characterize the novel sequences. Cox regression analysis showed that candidate novel miRNA sequences behave similarly to known miRNAs and may have prognostic value. Validation was performed on an independent dataset (Gene Expression Omnibus GSE52633) (dataset E) and by performing RT-qPCR of the most relevant miRNA candidates in formalin-fixed paraffin-embedded (FFPE) tissues (dataset F).
Raw sequence data from both HNSCC tumors and non-malignant head and neck tissue samples (Table 2, datasets A and B) obtained from TCGA in the form of BAM files were converted into unaligned (FASTQ) files using Partek Flow® (http://www.partek.com/partek-flow/). FASTQ files were then analyzed for novel miRNA expression using the online analysis platform miRMaster (https://ccb-compute.cs.uni-saarland.de/mirmaster) (accessed October 2018). This platform predicts novel miRNAs based on the miRDeep2 algorithm, a well-established novel miRNA discovery tool which identifies miRNA-like configurations by considering relative free-energy and the probability of random folding (37). Default parameters were used to perform quality filtering and read collapsing. The adapters were trimmed (Illumina TruSeq small RNA 3p), followed by the alignment of the reads to the hg38 build of the human genome (38). Sequences previously annotated in miRBase v.22 were excluded. The list of candidate novel miRNA transcripts was then further curated to include only sequences with a detectable expression of ≥1 read per million (RPM) in at least 10% of samples, for each group. Those miRNA candidates that remained after filtering were considered putative novel miRNAs.
To verify their designation as true miRNA sequences, we assessed whether these novel miRNA candidates shared structural properties and sequence features with known miRNA sequences. Nucleotide composition of the seed sequence and guanine-cytosine (GC) content were compared between the novel candidates and currently-annotated miRNAs, as well as their distribution across the genome.
Group Comparison and Association Studies
To determine the tissue-specificity of these novel miRNA candidates, normalized expression levels of the 146 candidate novel miRNA sequences from the non-malignant head and neck tissues (Table 2, datasets B and D) were queried against non-malignant samples from 12 different organ sites from TCGA Pan-Cancer Atlas using non-linear t-Distributed Stochastic Neighbor Embedding (t-SNE) dimensionality reduction. The tissues investigated included bile duct (n = 9), bladder (n = 19), brain (n = 5), cervix (n = 3), colon (n = 9), kidney (n = 71), liver (n = 47), lung (n = 91), pancreas (n = 4), prostate (n = 52), stomach (n = 45), thyroid (n = 59) and head & neck (n = 43).
To assess their involvement in HNSCC development, we sought to determine whether these novel transcripts are dysregulated in corresponding tumor samples.
An unsupervised hierarchal clustering analysis (Pearson correlation and complete linkage) was performed including novel miRNAs present in both tumor and non-malignant sample groups (Table 2, dataset D). Paired sample t-test (Benjamini-Hochberg [BH] adjusted p < 0.05 and fold change [FC] > 1.5) was applied to compare the novel miRNA expression between malignant and non-malignant samples (n = 43 pairs).
Clinical-pathological associations, examining anatomical site (oral cavity, pharynx, and larynx), smoking status (lifelong non-smoker versus continuing smoker) and HPV status (negative vs. positive), were observed for the novel miRNAs (n = 130) expressed exclusively in tumor samples (Table 2, dataset A) (t-test BH adjusted p < 0.05 and FC > 1.5).
To explore a potential prognostic relevance of the sequences discovered, the miRNA expression was associated with overall (OS) and recurrence-free survival (RFS) using the TCGA tumor samples (Table 2, dataset A). MicroRNAs associated with survival (p < 0.01) in a univariate log-rank test were included in a multivariate Cox proportional hazard model.
Target Prediction and Pathway Enrichment
To investigate the possible genes targeted by our recently discovered miRNAs and their biological roles, we performed target prediction and pathway enrichment analysis. Target prediction was performed using the miRanda v 3.3a algorithm, against all human genes 3′ UTR sequences acquired from Ensembl through Biomart tool (https://www.ensembl.org/) (39). The prediction algorithm was executed using strict alignment, alignment score ≥180 and energy threshold ≤ -20 kcal/mol parametrizations. Next, to gain further functional insights into the pathways these targets may be involved, we submitted the gene symbols identified to a comprehensive pathway enrichment analysis using pathDIP, which includes 15 distinct pathways resources (Extended pathway associations. Experimental plus orthologs plus FpClass – High Confidence; Minimum confidence level for predicted associations: 0.99) (40).
Confirmation Using an Independent Cohort
Publicly available small-RNA sequencing data from a second cohort (n = 20) (Table 2, dataset E) of oral squamous cell carcinoma (OSCC) tissue samples were downloaded from GEO (Accession GSE52663) (36). SRA files were converted to FASTQ and mapped to human genome build 38 using the STAR aligner in Partek Flow® (41). Novel miRNA candidates were then quantified by their genomic loci. Expression values were averaged to create an average expression value per sample. A detection threshold ≥10 reads across the averaged samples was employed.
Confirmation by RT-qPCR
To further confirm the presence of these miRNAs in HNSCC, we selected five of the most highly-expressed HNnov-miRNAs and confirmed their expression by PCR in an independent cohort of OSCC. Formalin-fixed paraffin-embedded (FFPE) tissue blocks (n = 25 OSCC and 5 normal oral tissue from the buccal mucosa) (Table 2, dataset F) were obtained from the British Columbia Oral Biopsy Service using written informed consent and a study protocol approved by the University of British Columbia—BC Cancer Research Ethics Board. Five 10 μm sections were cut from each block, and immediately placed into clean 1.5 mL microtubes. Deparaffinization was performed in xylene, and extraction was performed using the miRNeasy FFPE kit (QIAGEN, Hilden Germany) following manufacturer's guidelines.
Custom reverse-transcription and PCR primers were designed using the Custom TaqMan® Small RNA Assay Design Tool from Thermo Fisher. Primers were designed specific to the mature miRNA sequences for five of the highest-expressing novel HNnov-miRNAs, including HNnov-miR-59-5p (UGAGUUCUGGGCUGUAGUGUGCU), HNnov-miR-3-5p (AAUUACAGAUUGUCUCAGAGA), HNnov-miR-45-5p (GGGGGUGUAGCUCAGUGGUAGA), HNnov-miR-19-5p (CCCUGAUGAGCUUGACUCUAG), and HNnov-miR-48-3p (AAGUUUCUCUGAACGUGUAGAGC), according to Table S1. Reverse transcription of miRNA species was performed using the TaqMan™ MicroRNA Reverse Transcription Kit (Applied Biosystems™, Cat#4366596) and RT-qPCR in TaqMan™ Universal Master Mix II, with UNG (Applied Biosystems™, Cat#4440044) according to protocols established by the manufacturer. RT-qPCR was performed in an Applied Biosystems® 7500 Real-Time PCR System, and expression of mature miRNA transcripts in tumors was calculated in reference to normal oral epithelium using the 2(−ΔΔCt) method and normalized to U6 (TaqMan Cat#4427975, Assay ID: 001973).
Results
Discovery of Novel miRNA Sequences in Head and Neck Samples
In order to identify novel miRNAs in HNSCC non-malignant and tumor tissues, we submitted the raw HNSCC sncRNA sequence data from TCGA (Table 2, datasets A and B) to the online platform miRMaster and applied a miRNA-discovery algorithm as described in Materials and Methods. This initial analysis resulted in a list of miRNA candidates that were curated to exclude sequences highly homologous to those previously annotated in miRBase v.22. After curation, 146 previously unannotated miRNAs were identified (Table S1). These novel miRNA sequences are herein referred to as HNnov-miRs. The discovery of these 146 miRNAs represents a 5.5% increase to the total number of 2,656 currently-annotated miRNAs quantified by miRMaster, and an outstanding increase of 25% to the 583 currently-annotated miRNAs that were also found to be expressed at our threshold levels (1 RPM in 10% of the samples) in the TCGA HNSCC cohort (Figure 2A). Like currently-annotated miRNAs, the HNnov-miRs where shown to be widely distributed across the genome (Figure 2C). Additionally, they were found to have similar overall molecular features compared to annotated miRNAs, further supporting their identity as miRNA sequences (Table S1).
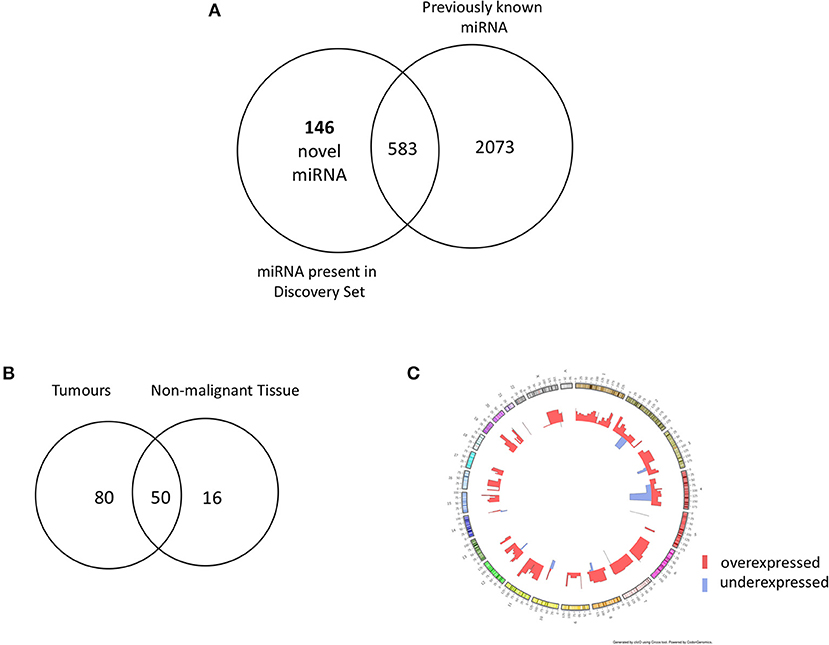
Figure 2. (A) Venn diagram summarizing the relative proportion of novel vs. previously identified miRNAs expressed to the same levels in the TCGA cohort compared to the current annotation of miRNA repositories. An addition of 146 novel miRNAs to 583 previously annotated sequences expressed to the same d level in the TCGA increases the transcriptome head and neck tissues substantially. (B) Venn diagram of novel miRNAs identified in head and neck squamous cell carcinoma tumor tissue (n = 523) and non-malignant (n = 43) tissue. Our results revealed 146 novel miRNA candidates; 80 and 16 were observed exclusively in non- malignant and tumor tissues, respectively, with 50 miRNA candidates detected in both groups. (C) Circos plot displaying the genomic localization of the novel miRNAs. The outermost circle displays the human autosomal chromosomes, and the inner layers show the expression fold changes (logged) of the novel miRNAs in head and neck squamous cell carcinoma tumors in relation to matched non-malignant tissue [created by ClicO FS: An interactive web-based service of Circos (42)].
Tissue- and Context-Specific Expression Patterns of the Novel miRNAs
Next, we sought to investigate the tissue-specificity of the HNnov-miRs by comparing their combined expression patterns in head and neck against other tissue types. This analysis showed that the HNnov-miRs are indeed head and neck-specific and their combined expression patterns were able to clearly distinguish non-malignant head and neck samples from other types of non-malignant tissue (bile duct, bladder, brain, cervix, colon, kidney, liver, lung, pancreas, prostate, stomach, and thyroid), as evidenced by t-Distributed Stochastic Neighbor Embedding (t-SNE) analysis (Figure 3). This tissue-specific nature highlights their potential relevance to head and neck biology.
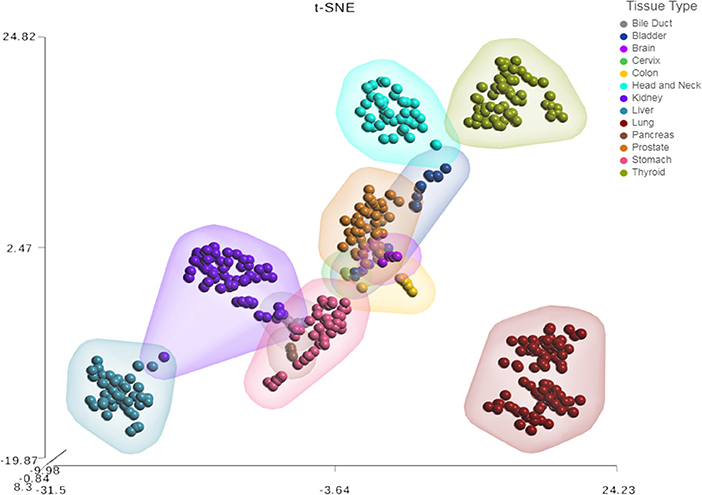
Figure 3. Tissue-specific expression patterns of unannotated miRNA transcripts. t-Distributed Stochastic Neighbor Embedding (t-SNE) analysis T-SNE shows tissue specificity of head and neck non-malignant tissue compared to other non-malignant tissue from The Cancer Genome Atlas (TCGA); bile duct (n = 9), bladder (n = 19), brain (n = 5), cervix (n = 3), colon (n = 9), were compared to head & neck (n = 43), kidney (n = 71), liver (n = 47), lung (n = 91), pancreas (n = 4), prostate (n = 52), stomach (n = 45), and thyroid (n = 59).
Differential Expression in HNSCC Tumor and Non-malignant Head and Neck Tissue
From our curated list of 146 HNnov-miRs, a total of 16 HNnov-miR sequences were exclusively expressed in non-malignant samples, 80 in tumors only, and 50 shared between both sample types (Figure 2B, Table S2). Of the 50 HNnov-miRs detected in both matched tumor and non-malignant tissue samples (n = 43 pairs), 39 were differentially expressed (BH-p < 0.05). Most sequences (n = 38) were found to be significantly over-expressed in HNSCC, while only one was under-expressed in tumors compared to non-malignant tissue (Table S2). Hierarchical clustering analysis of the HNnov-miRs detected in both tumor and matched non-malignant tissue samples demonstrated a clear difference in expression patterns between the two groups (Figure 4), which highlights that the HNnov-miRs are not only tissue-specific but also context-specific.
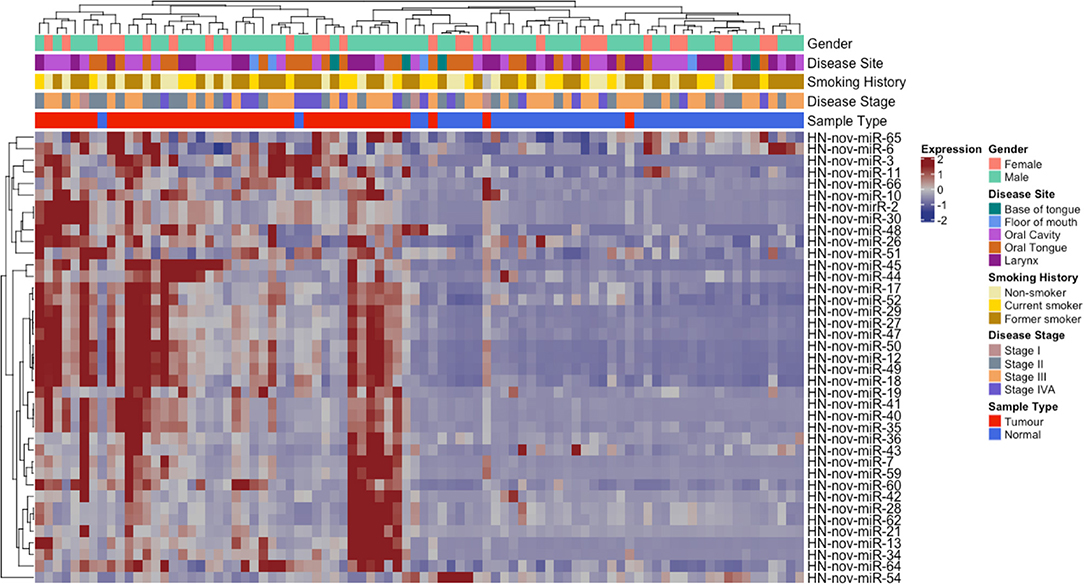
Figure 4. Unsupervised hierarchal clustering analysis comprising 39 HNnov-miR expressed in both tumors and non-malignant tissue. The dendogram shows two clusters, the first enriched by non-neoplastic samples (novel miRNA expression predominantly low) and the second by tumor samples (novel miRNA expression predominantly high). Heatmap annotation bars show some of the clinical parameters associated with each tissue sample, including gender, disease site and stage, smoking history, and tissue type.
To further explore the role of these 39 HNnov-miRNAs found to be significantly over-expressed in HNSCC, we performed target prediction analysis. This analysis revealed a total of 10,221 possible unique protein-coding gene targets (Table S2), in which 3,273 were targeted by at least 10% of the 39 miRNAs. We also performed pathway enrichment analysis on the 10,221 gene targets to investigate the biological pathways they may be involved and reported the top 20 enriched pathways (Table S6). In this analysis, none of the pathways were found to be significantly enriched after Benjamini-Hochberg correction, however it suggests the target genes to be involved mainly with interleukin signaling.
We also investigated if HNnov-miRs expression patterns differed according to different clinical parameters. Expression patterns of the novel miRNAs did not differ significantly between oral cavity and pharynx/larynx subsites. Likewise, expression between smokers and non-smokers did not differ significantly. Interestingly, three of the predicted novel miRNAs (HNnov-miR-2, HNnov-miR-30, and HNnov-miR-125) were significantly associated with HPV status (BH-p < 0.05 and fold change>1.5), where their downregulation was associated with the presence of HPV infection (Table S3).
Potential Prognostic Relevance of the Novel miRNAs
The prognostic impact of novel and known miRNAs was assessed in the TCGA cohort (n = 523) (dataset A in Table 2). Three predicted novel miRNAs were significantly associated with overall survival (OS; HNnov-miR-104, HNnov-miR-120, and HNnov-miR-136) and three were significantly associated with recurrence free survival (RFS; HNnov-miR-3, HNnov-miR-87, and HNnov-miR-135) in univariate analyses (Table S4, Figure S1). In a multivariate Cox proportional hazard model including both novel and known miRNAs, one novel miRNA remained independently associated with OS (HNnov-miR-120), and two with RFS (HNnov-miR-3 and HNnov-miR-135). We then established scores for OS and RFS using either known miRNAs alone or both novel and known miRNAs. Scores using novel and known miRNAs were more powerful in the segregation of patients within prognostic groups (Table S4, Figure S2).
Confirmation of the Novel miRNAs in an Independent Cohort
To confirm the existence of our novel miRNAs, we also investigated their presence in an additional RNA-sequencing dataset using the same analysis and filtering criteria performed in our discovery cohort. In the validation dataset (Table 2, dataset E), 102 of the 146 HNnov-miRs were detected (Table S5, Figure S3), including all three of the HNnov-miRs that were found to be overexpressed in HPV negative samples and all six of the HNnov-miRs that were associated with OS or RFS.
Validation by RT-qPCR
For this verification, we found that, compared to normal tissues, the 5 miRNA selected were all more highly expressed in OSCC, confirming not only their existence within the tumor, but their importance to tumor biology (Figure S4).
Discussion
In this study, we report a comprehensive analysis of undiscovered miRNAs that has led to the expansion of the head and neck transcriptome. By analyzing raw small-RNA sequencing data for both quantity and secondary RNA structure, we discovered 146 HNnov-miRs previously undescribed in head and neck tissues. Our characterization of these novel transcripts has revealed not only their tissue-specific nature and their context-specific expression patterns relevance to head and neck cancer biology, but also their diagnostic and prognostic potential.
The current annotation of the human miRNA transcriptome mainly contains miRNA sequences that are abundant and conserved. Therefore, cell lineage- and tissue-specific miRNAs, especially those that are less abundant, may not be included in current miRBase annotations (29). This study, like several recent studies of other organs, has shown that re-analyses of high-throughput sequencing data, can lead to large-scale discoveries of novel miRNAs that are expressed in a tissue-specific manner, thus expanding the human miRNA transcriptome (29–33).
In order to validate the expression of the 146 HNnov-miRs, we analyzed an independent dataset of HNSCC (n = 20). High throughput sequencing data of small-RNAs are scarce, and despite the limited sample size of this validation set, 102 of our HNnov-miRNAs were detected in this independent cohort. To provide an additional layer of verification, experimental validation of the miRNAs was carried out by performing RT-qPCR of the most relevant miRNA candidates in OSCC tissues, thereby strengthening the position that these novel miRNAs may serve as a new resource for the exploration of head and neck cancer specific transcripts in future investigations.
Interestingly, our study did not show a difference in expression pattern of HNnov-miRNA between HNSCC tumors from smokers and non-smokers. These observations are sustained by similar studies. Kolokythas et al. have reported similar miRNA expression in oral squamous cell carcinoma in never-smokers and ever-smokers (43). Similarly, a study that looked at genome wide analysis in 30 oral potentially malignant lesions that progressed to cancer and a study that examined loss of heterozygosity at 9p, 17p, and 4q in 455 lesions with oral epithelial dysplasia showed similar genetic alterations between smokers and nonsmokers (44, 45). However, Irimie et al. have reported that the overall variation in gene expression profiles was different for patients who smoked compared to those who have never smoked. The interaction between genetics and exposure to non-tobacco environmental carcinogens complicates the identification of a single effect, such as smoking, related to HNSCC.
Our results showed that three of the predicted novel miRNAs (HNnov-miR-2, HNnov-miR-30, and HNnov-miR-125) were significantly associated with HPV status. Interestingly, all of these novel genes map to chromosome 12, and both HNnov-miR-2 and HNnov-miR30 lie within the genes KRT6C and KRT6B, respectively. This is interesting as both KRT6C, and B, have previously described to have roles in various cancers, and are included in a gene signature separating lung adenocarcinoma, from lung squamous cell carcinoma (46, 47). Further, we also find expression of these genes to be associated with HPV status. Additional studies will be needed to determine if these novel miRNAs work in conjunction with, or have specific functions independent of these cancer associated protein coding genes (Figure 5).
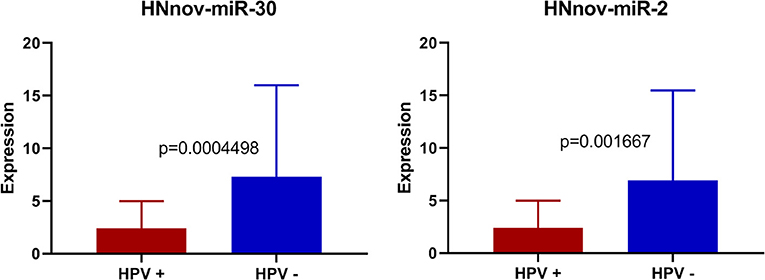
Figure 5. Expression of HNnov-miR-2 and HNnov-miR-30 is significantly associated with negative HPV status in tumors (Mann Whitney U-test).
The potential utility of the HNnov-miRNAs is highlighted by our observations that a subset of these transcripts is significantly associated with patient outcome (Figure S1), and that combining novel and known miRNAs improved the prognostic signature (Figure S2). The expression of HNnov-miR-120, HNnov-miR-3 and HNnov-miR-135 have prognostic relevance regarding recurrence-free and overall survival in patients with HNSCC and may improve the current prognostic risk stratification of HNSCC.
Here, to investigate if the unannotated miRNAs discovered in head and neck tissue were tissue specific, we assessed a number of non-malignant datasets generated by TCGA, including some cohorts with low sample numbers. In general, the more samples of a tissue type analyzed, the greater likelihood of discovering additional unannotated miRNA transcripts, especially those with non-constitutive or low expression levels. Therefore, a caveat of this analysis is that some of the HNnov-miRs may have not been detected in the additional tissues analyzed because of the low sample numbers, particularly in the cohorts such as brain and cervix. However, it can indicate that they if present in these other tissues, they display different expression levels and their combined patter of in head and neck are quite tissue specific. While this study represents the first-generation analysis of these unannotated miRNAs, and focuses on head and neck tissue, future studies with additional samples will be needed to comprehensively catalog these species across human tissues.
Although we cannot weigh the HNnov-miRNAs newly discovered in this study against literature, we can assess whether the expression and function of the known miRNA observed within our custom pipeline are consistent with what is found in the literature. Our findings are consistent with a systematic review of 21 studies by Jamali et al. which indicated that overexpression of miR-18a, miR-19a, miR-21, miR-134a, and miR-155, miR-181a, miR-210, were associated with poor survival, and that significantly decreased expression of let-7d, let-7g, miR-17, miR-34a, and miR-125b, miR-126a, miR-153, miR-200c, miR-203, miR-205, miR 218, miR-363, miR-375, miR-491-p5, miR-451, were associated with poor prognosis (48). In our study, we analyzed miRNA expression in the TCGA dataset (n = 523, dataset A), and found that among the abovementioned miRNAs, miR-134a, miR-153, miR-200c, miR-205, and miR-125b were significantly associated with overall survival in univariate analysis. After controlling for heterogeneity, Jamali's fixed model meta-analysis indicated that a significantly increased expression of miR-21 is associated with poor survival (Pooled HR = 1.57–95% CI: 1.22–2.02, P < 0.05) (48). In multivariate analysis, we found that only miR-205 remained significantly associated with overall survival. These findings add weight to the relevance and legitimacy of the novel miRNA discovered within our pipeline.
In conclusion, annotated miRNAs represent only a fraction of all the miRNAs encoded by the human genome. Here we identified 146 HNnov-miRs expressed in head and neck tissues with potential relevance to HNSCC biology, as well as diagnostic and prognostic potential. While our study was performed on a predictive platform and mainly relied on small-RNA sequencing data, the validation of 5 of these novel miRNAs by RT-qPCR supported their existence. Likewise, to understand their biological role and potential clinical utility, further functional assays will be required. An important next step would be to query the presence of these HNnov-miRNAs in liquid biopsies, such as serum samples. Here, we expand the current repertoire of head and neck miRNAs and provide an important new resource for the exploration of organ and disease specific transcripts that may guide future discoveries in head and neck cancers.
Data Availability Statement
All data analyzed in this study are publicly available: TCGA consortium/NIH GDC (https://gdc.cancer.gov/); and GEO database accession number: GSE52663.
Ethics Statement
The studies involving human participants were reviewed and approved by University of British Columbia Research Ethics Board. The patients/participants provided their written informed consent to participate in this study.
Author Contributions
LR and BM were responsible for the project design. LR, BM, EM, FG, AS, MB-F, and GS contributed to data acquisition, data analysis, interpretation of results, and manuscript preparation. CG and WL were principle investigators of this project. All authors have read, edited and approved the final manuscript, and agree to be accountable for the content of the work.
Funding
This work was supported by grants from the Canadian Institutes for Health Research [CIHR FDN-143345]. LR was supported by the BC Cancer Foundation and University of British Columbia Faculty of Dentistry. EM was a Vanier Canada Graduate Scholar. FG was supported by the Ligue nationale contre le cancer, the Fonds de Recherche en Santé Respiratoire (appel d'offres 2018 emis en commun avec la Fondation du Souffle), the Fondation Charles Nicolle. MB-F was supported by the São Paulo Research Foundation (2018/06138-8).
Conflict of Interest
The authors declare that the research was conducted in the absence of any commercial or financial relationships that could be construed as a potential conflict of interest.
Acknowledgments
The authors wish to thank Dr. Miriam P. Rosin (Department of Cancer Control Research, BC Cancer) and the British Columbia Oral Cancer Prevention Program for their assistance with the processing of tissue samples.
Supplementary Material
The Supplementary Material for this article can be found online at: https://www.frontiersin.org/articles/10.3389/fonc.2019.01305/full#supplementary-material
References
1. Bray F, Ferlay J, Soerjomataram I, Siegel RL, Torre LA, Jemal A. Global cancer statistics 2018: GLOBOCAN estimates of incidence and mortality worldwide for 36 cancers in 185 countries. CA Cancer J Clin. (2018) 68:394–424. doi: 10.3322/caac.21492
2. Warnakulasuriya S. Living with oral cancer: epidemiology with particular reference to prevalence and life-style changes that influence survival. Oral Oncol. (2010) 46:407–10. doi: 10.1016/j.oraloncology.2010.02.015
3. Lozano R, Naghavi M, Foreman K, Lim S, Shibuya K, Aboyans V, et al. Global and regional mortality from 235 causes of death for 20 age groups in 1990 and 2010: a systematic analysis for the Global Burden of Disease Study 2010. Lancet. (2012) 380:2095–128. doi: 10.1016/S0140-6736(12)61728-0
4. Gebert LFR, MacRae IJ. Regulation of microRNA function in animals. Nat Rev Mol Cell Biol. (2019) 20:21–37. doi: 10.1038/s41580-018-0045-7
5. Vickers MM, Bar J, Gorn-Hondermann I, Yarom N, Daneshmand M, Hanson JE, et al. Stage-dependent differential expression of microRNAs in colorectal cancer: potential role as markers of metastatic disease. Clin Exp Metastasis. (2012) 29:123–32. doi: 10.1007/s10585-011-9435-3
6. Hayes J, Peruzzi PP, Lawler S. MicroRNAs in cancer: biomarkers, functions and therapy. Trends Mol Med. (2014) 20:460–9. doi: 10.1016/j.molmed.2014.06.005
7. Becker-Santos DD, Thu KL, English JC, Pikor LA, Martinez VD, Zhang M, et al. Developmental transcription factor NFIB is a putative target of oncofetal miRNAs and is associated with tumour aggressiveness in lung adenocarcinoma. J Pathol. (2016) 240:161–72. doi: 10.1002/path.4765
8. Avissar M, McClean MD, Kelsey KT, Marsit CJ. MicroRNA expression in head and neck cancer associates with alcohol consumption and survival. Carcinogenesis. (2009) 30:2059–63. doi: 10.1093/carcin/bgp277
9. Iorio MV, Ferracin M, Liu CG, Veronese A, Spizzo R, Sabbioni S, et al. MicroRNA gene expression deregulation in human breast cancer. Cancer Res. (2005) 65:7065–70. doi: 10.1158/0008-5472.CAN-05-1783
10. Lu J, Getz G, Miska EA, Alvarez-Saavedra E, Lamb J, Peck D, et al. MicroRNA expression profiles classify human cancers. Nature. (2005) 435:834–8. doi: 10.1038/nature03702
11. Murakami Y, Yasuda T, Saigo K, Urashima T, Toyoda H, Okanoue T, et al. Comprehensive analysis of microRNA expression patterns in hepatocellular carcinoma and non-tumorous tissues. Oncogene. (2006) 25:2537–45. doi: 10.1038/sj.onc.1209283
12. Roldo C, Missiaglia E, Hagan JP, Falconi M, Capelli P, Bersani S, et al. MicroRNA expression abnormalities in pancreatic endocrine and acinar tumors are associated with distinctive pathologic features and clinical behavior. J Clin Oncol. (2006) 24:4677–84. doi: 10.1200/JCO.2005.05.5194
13. Enfield KS, Pikor LA, Martinez VD, Lam WL. Mechanistic roles of non-coding RNAs in lung cancer biology and their clinical implications. Genet Res Int. (2012) 2012:737416. doi: 10.1155/2012/737416
14. Mazeh H, Deutch T, Karas A, Bogardus KA, Mizrahi I, Gur-Wahnon D, et al. Next-generation sequencing identifies a highly accurate miRNA panel that distinguishes well-differentiated thyroid cancer from benign thyroid nodules. Cancer Epidemiol Biomarkers Prev. (2018) 27:858–63. doi: 10.1158/1055-9965.EPI-18-0055
15. Tokar T, Pastrello C, Ramnarine VR, Zhu CQ, Craddock KJ, Pikor LA, et al. Differentially expressed microRNAs in lung adenocarcinoma invert effects of copy number aberrations of prognostic genes. Oncotarget. (2018) 9:9137–55. doi: 10.18632/oncotarget.24070
16. Mazumder S, Datta S, Ray JG, Chaudhuri K, Chatterjee R. Liquid biopsy: miRNA as a potential biomarker in oral cancer. Cancer Epidemiol. (2019) 58:137–45. doi: 10.1016/j.canep.2018.12.008
17. Vucic EA, Thu KL, Pikor LA, Enfield KS, Yee J, English JC, et al. Smoking status impacts microRNA mediated prognosis and lung adenocarcinoma biology. BMC Cancer. (2014) 14:778. doi: 10.1186/1471-2407-14-778
18. Rupaimoole R, Slack FJ. MicroRNA therapeutics: towards a new era for the management of cancer and other diseases. Nat Rev Drug Discov. (2017) 16:203–22. doi: 10.1038/nrd.2016.246
19. Tokar T, Pastrello C, Rossos AEM, Abovsky M, Hauschild AC, Tsay M, et al. mirDIP 4.1-integrative database of human microRNA target predictions. Nucleic Acids Res. (2018) 46:D360–70. doi: 10.1093/nar/gkx1144
20. Lubov J, Maschietto M, Ibrahim I, Mlynarek A, Hier M, Kowalski LP, et al. Meta-analysis of microRNAs expression in head and neck cancer: uncovering association with outcome and mechanisms. Oncotarget. (2017) 8:55511–24. doi: 10.18632/oncotarget.19224
21. Corra F, Agnoletto C, Minotti L, Baldassari F, Volinia S. The network of non-coding RNAs in cancer drug resistance. Front Oncol. (2018) 8:327. doi: 10.3389/fonc.2018.00327
22. Felix TF, Lopez Lapa RM, de Carvalho M, Bertoni N, Tokar T, Oliveira RA, et al. MicroRNA modulated networks of adaptive and innate immune response in pancreatic ductal adenocarcinoma. PLoS ONE. (2019) 14:e0217421. doi: 10.1371/journal.pone.0217421
23. Macharia LW, Wanjiru CM, Mureithi MW, Pereira CM, Ferrer VP, Moura-Neto V. MicroRNAs, hypoxia and the stem-like state as contributors to cancer aggressiveness. Front Genet. (2019) 10:125. doi: 10.3389/fgene.2019.00125
24. Yang X, Li Y, Zou L, Zhu Z. Role of exosomes in crosstalk between cancer-associated fibroblasts and cancer cells. Front Oncol. (2019) 9:356. doi: 10.3389/fonc.2019.00356
25. Yang CX, Sedhom W, Song J, Lu SL. The role of MicroRNAs in recurrence and metastasis of head and neck squamous cell carcinoma. Cancers. (2019) 11:E395. doi: 10.3390/cancers11030395
26. Childs G, Fazzari M, Kung G, Kawachi N, Brandwein-Gensler M, McLemore M, et al. Low-level expression of microRNAs let-7d and miR-205 are prognostic markers of head and neck squamous cell carcinoma. Am J Pathol. (2009) 174:736–45. doi: 10.2353/ajpath.2009.080731
27. Summerer I, Unger K, Braselmann H, Schuettrumpf L, Maihoefer C, Baumeister P, et al. Circulating microRNAs as prognostic therapy biomarkers in head and neck cancer patients. Br J Cancer. (2015) 113:76–82. doi: 10.1038/bjc.2015.111
28. Ludwig N, Leidinger P, Becker K, Backes C, Fehlmann T, Pallasch C, et al. Distribution of miRNA expression across human tissues. Nucleic Acids Res. (2016) 44:3865–77. doi: 10.1093/nar/gkw116
29. Londin E, Loher P, Telonis AG, Quann K, Clark P, Jing Y, et al. Analysis of 13 cell types reveals evidence for the expression of numerous novel primate- and tissue-specific microRNAs. Proc Natl Acad Sci USA. (2015) 112:E1106–15. doi: 10.1073/pnas.1420955112
30. Marshall EA, Sage AP, Ng KW, Martinez VD, Firmino NS, Bennewith KL, et al. Small non-coding RNA transcriptome of the NCI-60 cell line panel. Sci Data. (2017) 4:170157. doi: 10.1038/sdata.2017.157
31. Minatel BC, Martinez VD, Ng KW, Sage AP, Tokar T, Marshall EA, et al. Large-scale discovery of previously undetected microRNAs specific to human liver. Hum Genomics. (2018) 12:16. doi: 10.1186/s40246-018-0148-4
32. Sage AP, Minatel BC, Marshall EA, Martinez VD, Stewart GL, Enfield KSS, et al. Expanding the miRNA transcriptome of human kidney and renal cell carcinoma. Int J Genomics. (2018) 2018:6972397. doi: 10.1155/2018/6972397
33. Barros-Filho MC, Pewarchuk M, Minatel BC, Sage AP, Marshall EA, Martinez VD, et al. Previously undescribed thyroid-specific miRNA sequences in papillary thyroid carcinoma. J Hum Genet. (2019) 64:505–8. doi: 10.1038/s10038-019-0583-7
34. Martinez VD, Marshall EA, Anderson C, Ng KW, Minatel BC, Sage AP, et al. Discovery of previously undetected mircoRNAs in mesothelioma and their use as tissue-of-origin markers. Am J Respir Cell Mol Biol. (2019) 61:266–8. doi: 10.1165/rcmb.2018-0204LE
35. The Cancer Genome Atlas Network. Comprehensive genomic characterization of head and neck squamous cell carcinomas. Nature. (2015) 517:576–82. doi: 10.1038/nature14129
36. Yoon AJ, Wang S, Shen J, Robine N, Philipone E, Oster MW, et al. Prognostic value of miR-375 and miR-214-3p in early stage oral squamous cell carcinoma. Am J Transl Res. (2014) 6:580–92.
37. Friedlander MR, Mackowiak SD, Li N, Chen W, Rajewsky N. miRDeep2 accurately identifies known and hundreds of novel microRNA genes in seven animal clades. Nucleic Acids Res. (2012) 40:37–52. doi: 10.1093/nar/gkr688
38. Fehlmann T, Backes C, Kahraman M, Haas J, Ludwig N, Posch AE, et al. Web-based NGS data analysis using miRMaster: a large-scale meta-analysis of human miRNAs. Nucleic Acids Res. (2017) 45:8731–44. doi: 10.1093/nar/gkx595
39. Enright AJ, John B, Gaul U, Tuschi T, Sander C, Mark DS. MicroRNA targets in drosophila. Genome Biol. (2003) 5:R1. doi: 10.1186/gb-2003-5-1-r1
40. Rahmati S, Abovsky M, Pastrello C, Jurisica I. pathDIP: an annotated resource for known and predicted human gene-pathway associations and pathway enrichment analysis. Nucleic Acids Res. (2017) 45(D1):D419–26. doi: 10.1093/nar/gkw1082
41. Dobin A, Davis CA, Schlesinger F, Drenkow J, Zaleski C, Jha S, et al. STAR: ultrafast universal RNA-seq aligner. Bioinformatics. (2013) 29:15–21. doi: 10.1093/bioinformatics/bts635
42. Cheong WH, Tan YC, Yap SJ, Ng KP. ClicO FS: an interactive web-based service of Circos. Bioinformatics. (2015) 31:3685–7. doi: 10.1093/bioinformatics/btv433
43. Kolokythas A, Zhou Y, Schwartz JL, Adami GR. Similar squamous cell carcinoma epithelium microRNA expression in never smokers and ever smokers. PLoS ONE. (2015) 10:e0141695. doi: 10.1371/journal.pone.0141695
44. Rock LD, Rosin MP, Zhang L, Chan B, Shariati B, Laronde DM. Characterization of epithelial oral dysplasia in non-smokers: First steps towards precision medicine. Oral Oncol. (2018) 78:119–25. doi: 10.1016/j.oraloncology.2018.01.028
45. de la Oliva J, Larque AB, Marti C, Bodalo-Torruella M, Nonell L, Nadal A, et al. Oral premalignant lesions of smokers and non-smokers show similar carcinogenic pathways and outcomes. A clinicopathological and molecular comparative analysis. J Oral Pathol Med. doi: 10.1111/jop.12864. [Epub ahead of print].
46. Chang HH, Dreyfuss JM, Ramoni MF. A transcriptional network signature characterizes lung cancer subtypes. Cancer. (2011) 117:353–60. doi: 10.1002/cncr.25592
47. Hu J, Zhang LC, Song X, Lu JR, Jin Z. KRT6 interacting with notch1 contributes to progression of renal cell carcinoma, and aliskiren inhibits renal carcinoma cell lines proliferation in vitro. Int J Clin Exp Pathol. (2015) 8:9182–8.
Keywords: microRNAs, non-coding RNA, gene expression profiling, head and neck cancer, computational biology
Citation: Rock LD, Minatel BC, Marshall EA, Guisier F, Sage AP, Barros-Filho MC, Stewart GL, Garnis C and Lam WL (2019) Expanding the Transcriptome of Head and Neck Squamous Cell Carcinoma Through Novel MicroRNA Discovery. Front. Oncol. 9:1305. doi: 10.3389/fonc.2019.01305
Received: 12 July 2019; Accepted: 11 November 2019;
Published: 27 November 2019.
Edited by:
Luisa Lanfrancone, European Institute of Oncology (IEO), ItalyReviewed by:
Luigi Fattore, Sapienza University of Rome, ItalyMarco Sciacovelli, University of Cambridge, United Kingdom
Copyright © 2019 Rock, Minatel, Marshall, Guisier, Sage, Barros-Filho, Stewart, Garnis and Lam. This is an open-access article distributed under the terms of the Creative Commons Attribution License (CC BY). The use, distribution or reproduction in other forums is permitted, provided the original author(s) and the copyright owner(s) are credited and that the original publication in this journal is cited, in accordance with accepted academic practice. No use, distribution or reproduction is permitted which does not comply with these terms.
*Correspondence: Leigha D. Rock, bGVpZ2hhLnJvY2tAZGFsLmNh