- 1School of Artificial Intelligence, University of Chinese Academy of Sciences, Beijing, China
- 2CAS Key Laboratory of Molecular Imaging, Institute of Automation, Chinese Academy of Sciences, Beijing, China
- 3Cancer Hospital of China Medical University, Shenyang, China
- 4Liaoning Cancer Hospital & Institute, Shenyang, China
- 5Beijing Advanced Innovation Center for Big Data-Based Precision Medicine, School of Medicine, Beihang University, Beijing, China
Objectives: To develop a radiomic model based on multiparametric magnetic resonance imaging (MRI) for predicting treatment response prior to commencing concurrent chemotherapy and radiation therapy (CCRT) for locally advanced cervical cancer.
Materials and methods: The retrospective study enrolled 120 patients (allocated to a training or a test set) with locally advanced cervical cancer who underwent CCRT between December 2014 and June 2017. All patients enrolled underwent MRI with nine sequences before treatment and again at the end of the fourth week of treatment. Responses were evaluated by MRI according to RECIST standards, and patients were divided into a responder group or non-responder group. For every MRI sequence, a total of 114 radiomic features were extracted from the outlined tumor habitat. On the training set, the least absolute shrinkage and selection operator method was used to select key features and to construct nine habitat signatures. Then, three kinds of machine learning models were compared and applied to integrate these predictive signatures and the clinical characteristics into a radiomic model. The discrimination ability, reliability, and calibration of our radiomic model were evaluated.
Results: The radiomic model, which consisted of three habitat signatures from sagittal T2 image, axial T1 enhanced-MRI image, and ADC image, respectively, has shown good predictive performance, with area under the curve of 0.820 (95% CI: 0.713–0.927) in the training set and 0.798 (95% CI: 0.678–0.917) in the test set. Meanwhile, the model proved to perform better than each single signature or clinical characteristic.
Conclusions: A radiomic model employing features from multiple tumor habitats held the ability for predicting treatment response in patients with locally advanced cervical cancer before commencing CCRT. These results illustrated a potential new tool for improving medical decision-making and therapeutic strategies.
Introduction
Cervical cancer is one of the leading causes of cancer-related mortality in women, among which locally advanced cervical cancer has poor prognosis (1, 2). According to National Comprehensive Cancer Network (NCCN) guidelines, concurrent chemotherapy and radiation therapy (CCRT) with cisplatin-based chemotherapy is a primary treatment for patients with locally advanced cervical cancer (3); the 5-years survival rate can reach 60–80% (4). However, if first-line CCRT fails, the extended CCRT treatment period inevitably delays the commencement of other potentially effective treatments (5). In addition, CCRT has many side effects. Extra-pelvic irradiation affects the bones that contain more than half the body's proliferative active bone marrow volume and may cause myelosuppression. Platinum-based CCRT can aggravate myelosuppression, but treatment delay or interruption affects its efficacy (6). Therefore, the prediction of response to CCRT before treatment commences may help us to decide whether to choose CCRT as first-line treatment. Moreover, response prediction can guide personalized medicine by selecting patients who are most sensitive to CCRT.
In clinical practice, assessing response to CCRT is often based on tumor biopsy histology during or after treatment, which provides no value in predicting the outcome for a CCRT decision. Magnetic resonance imaging (MRI) is routinely used for non-invasive diagnosis, treatment planning, and assessing treatment response of locally advanced cervical cancer (7). There are numerous MRI sequences, such as T1 and T2, dynamic contrast enhanced MRI, and diffusion-weighted MRI (DWI). Although these MRI sequences could assess the likely response based on morphological changes after treatment (8), this is still a lagging indicator (9). Pretreatment methods for predicting the response of CCRT are still lacking.
Radiomics is an emerging field that aims to extract large amounts of quantitative information from medical images that may not be easily obtained or quantified by traditional means (10–13). This kind of technique is able to capture features that reflect intratumoral heterogeneity, which is thought to be related to sensitivity to chemotherapy, radiotherapy, and other treatments (14). Therefore, it has been widely used to predict treatment response in nasopharyngeal carcinoma (15), rectal carcinoma (16), and lung cancer (17).
In this study, we aimed to develop and validate a radiomic model for predicting treatment response to CCRT in locally advanced cervical cancer using pre-treatment MRI data (Figure 1).
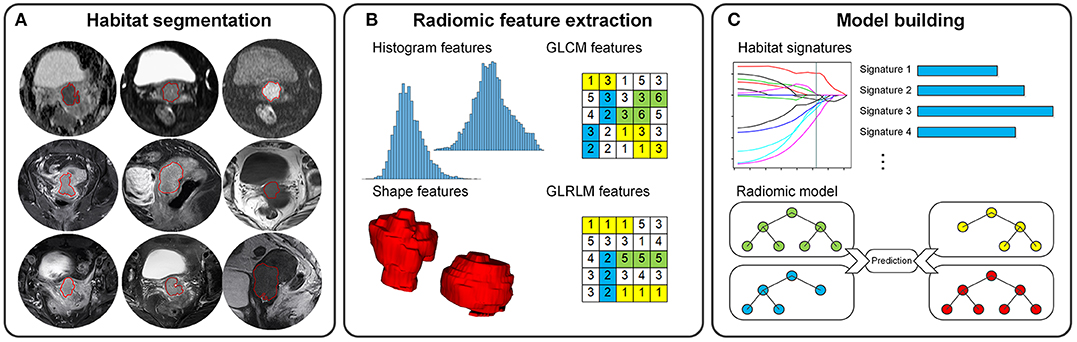
Figure 1. Radiomics pipeline for the prediction of CCRT response in locally advanced cervical cancer. (A) Nine tumor habitat segmentations. (B) 3D radiomic feature extraction. (C) Development of habitat signatures and radiomic model.
Materials and Methods
Ethical approval for this retrospective study was granted by the ethics committee of the Cancer Hospital of China Medical University, and the informed consent for patients was waived.
Patients
One hundred and forty consecutive patients with cervical carcinoma, treated between December 2014 and June 2017, were enrolled according to the following inclusion criteria: (1) histologically proven (biopsy) cervical carcinoma and clinical diagnosis of locally advanced cervical cancer according to the International Federation of Gynecology and Obstetrics (FIGO) classification (FIGO stage IB2-IIB) (18); (2) no medical treatment or CCRT prior to the pre-treatment MRI; (3) no other malignancies during the study; (4) CCRT administered based on the NCCN Clinical Practice Guidelines in Oncology-Cervical Cancer Guideline) (3); and (5) MRI performed again at the end of the fourth week of treatment for response evaluation. 20 patients were excluded from the study according to the exclusion criteria: (1) missing clinical data (i.e., the clinical characteristics listed in Table 1; n = 5); (2) image artifacts (n = 3); (3) >3 slices of tumor volume (the slice thickness = 4 mm; n = 4); and (4) more than 1 week between performing the pre-treatment MRI and commencing CCRT (n = 8). Therefore, a total of 120 patients met these criteria. Computer random number generation was used to split half of patients into the training set [n = 60, mean age, 51.5 ± 9.2 (range, 27–76) years], and the rest patients into the test set [n = 60, mean age, 53.7 ± 9.0 (range, 34–75) years].
All patients underwent standard CCRT according to NCCN guidelines (3). The detailed treatment schemes are shown in Supplementary Material.
MRI Protocols
All patients underwent the pre-treatment multiparametric MRI examinations before CCRT (within 1 week) and had a follow-up MRI. The MRI images were acquired using a 3.0T MR system (Siemens Magnetom Verio, Erlangen, Germany). The scanning range was set to cover the whole pelvis, and the scanning positioning line was consistent. The detailed MRI scanning protocols are shown in Supplementary Material and Table S1. Patients were scanned with sagittal T2, axial T1, axial T2-FS, DWI with b = 0, and b = 800, apparent diffusion coefficient (ADC), and enhanced-MRI (sagittal, axial, and coronal directions). The follow-up MRI scans used the same parameters with the pre-treatment MRI. In this study, we retrieved the pre-treatment MRI images from a picture archiving and communication system (PACS; Neusoft, Shenyang, China) for segmentation and analysis.
Evaluation of Treatment Outcome
Referring to Response Evaluation Criteria in Solid Tumors standards, two highly experienced radiologists in gynecological MRI jointly analyzed treatment response (19). Responses can be classified into groups of CR (Complete response, absence of residual tumor), PR (partial response, the longest diameter of the tumor was <70% of the original size), PD (progressive disease, at least a 20% increase in the longest tumor diameter compared to the original size), and SD (stable disease, neither sufficient shrinkage for PR nor sufficient increase for PD). Patients were thus divided into a responder group, including CR and PR, or a non-responder group, including PD and SD in this study. The pre-treatment MRI before CCRT was used to predict responder or non-responder in this study.
Tumor Habitat Segmentation and Feature Extraction
Habitats are the tumor regions/subregions in different image sequences (11). In this study, the highlighted tumor volume in one MRI sequence was defined as a tumor habitat. Three-dimensional (3D) regions of interest (ROI) of tumor habitat was segmented manually by a radiologist with 10 years' experience (radiologist 1) using ITK-SNAP software (version 3.6.0; http://www.itk-snap.org). In details, the ROI were drawn on each of the 2D slices to include the entire tumor volume. Nine tumor habitats were delineated on the nine pre-treatment MRI sequences. In addition, we randomly chose 25 patients from the training set and asked another radiologist (radiologist 2) with 15 years' experience to segment habitats for analyzing the reproducibility of features.
We normalized image intensity and calculated quantitative radiomic features from these habitats. The radiomic features contained 114 3D descriptors for each habitat, including shape features, histogram features, gray-level co-occurrence matrix (GLCM) features, and gray-level run-length matrix (GLRLM) features. Referring to the Image Biomarker Standardization Initiative (IBSI) (20), the features were extracted using an in-house developed toolbox performed in MATLAB (version 2017a; MathWorks, Natick, MA, USA). The details of radiomic feature extraction are described in Supplementary Material.
Habitat Signature and Radiomic Model Building
Based on the training set, we built nine habitat signatures and a radiomic model in this study. For each habitat, a three-step feature selection procedure was used to remove the non-reproducible or redundant features and find the most predictive ones in the training set. First, we analyzed the stability of radiomic features from the re-segmentation data using inter-class correlation coefficient (ICC). Features with ICCs <0.8 were removed. Second, Pearson correlation coefficients between every pair of features were calculated to measure the linear correlations. To reduce redundancy, for the pairs that yielded correlation coefficients > 0.6, we only retained the more stable one (i.e., with high ICC). Then, the least absolute shrinkage and selection operator (LASSO) logistic regression model (21) was utilized to assess the importance of features and obtain the most predictive combination as the habitat signature. Therefore, we obtained nine habitat signatures from the nine MRI sequences.
To build the radiomic model, we compared three kinds of machine learning models, including support vector machine, random forest, and logistic regression model, with the habitat signatures and clinical characteristics as input variables. To select the best model as well as the optimized hype-parameters, and to remove the meaningless or redundant variables, multiple three-fold cross-validation with the grid search was implemented on the training set. The average accuracy was used as the evaluation criteria. We then obtained the final radiomic model by re-training the selected model with the whole training set.
Statistical Analysis and Performance Evaluation
Clinical characteristics with potential prognostic outcomes were identified in univariate analysis on the basis of the chi-square test or Fisher's exact test for categorical variables and the t-test or Mann-Whitney U test for continuous variables. Multivariable logistic regression analysis was implemented to identify the independent predictors. The statistical significance used P < 0.05.
The quantitative discrimination performance was assessed using the receiver operator characteristics (ROC) analysis. The area under the curve (AUC) with 95% confidence interval (95% CI) was calculated. The point on the ROC curve of the training set yielding the highest Youden's index was selected as the cut-off value. Then, the accuracy of the model was assessed. We implemented calibration curve analysis with the Hosmer-Lemeshow test to measure the agreement between the predicted probability of treatment response and the observed results. The consistency test for the radiomic model was conducted by comparing its outputs based on the segmentation of the two radiologists (i.e., the re-segmentation data). Furthermore, to fully investigate the performance and robustness of the proposed multi-habitat based radiomics, we randomly split the 120 patients into training sets or test sets 20 times. Then, the model was built and validated repeatedly. The methods of statistical analysis were conducted using the R software (version 3.5.0; http://www.rproject.org).
Results
Clinical Characteristics
The clinical characteristics of patients are summarized in Table 1. There were no significant differences between the responder and non-responder groups in the training or test sets in terms of any of the clinical characteristics, based on univariate analysis (P > 0.05).
Feature Selection and Modeling
Among the 114 original radiomic features normalized by z-score transformation in each habitat, key features were obtained after the three-step feature selection methodology (Table 2). The selected features captured the intensity, shape, and texture pattern of the tumors. Then, nine habitat signatures were built via LASSO logistic regression, respectively. All the signatures showed significant associations with the treatment response, yielding AUCs ranging from 0.611 to 0.741 in the training set. Beginning with the habitat signatures and the clinical characteristics, grid search was implemented on three machine learning models, respectively. The random forest combining the habitat signatures from sagittal T2 image, axial T1 enhanced-MRI image, and ADC image yielded the highest average accuracy in multiple cross-validations, and therefore was selected to construct the radiomic model. The radiomic scores, which represented the predicted possibility of response to CCRT, were calculated via radiomic model for all the patients.
Performance Evaluation of Radiomic Model
In the ROC analysis, the radiomic model yielded satisfactory prediction performance in the training set (AUC = 0.820, 95% CI: 0.713–0.927) and test set (AUC = 0.798, 95% CI: 0.678–0.917). It had better predictive performance than any single habitat signature in both training and test sets, which are listed in Table 3 in detail. The radiomic model was the only factor that reached statistical significance for predicting treatment response in the multivariable analysis including the clinical characteristics. The distribution of radiomic model score and the treatment response for each patient, as well as the ROC curves, are shown in Figure 2. With the cut-off value of 0.520, the classification accuracies were 0.750 in both the training and test sets. The calibration curves of the radiomic model showed good agreement between the predicted and actual probabilities of treatment response in the two sets (Figure 3). The Hosmer-Lemeshow test suggests that there is no significant departure (P = 0.368 and 0.437, respectively). The radiomic scores of the re-segmentation data demonstrated that there was substantial inter-radiologist agreement for the model (ICC = 0.809). Meanwhile, the model's performances were relatively consistent on the segmentations of the two radiologists (AUCs = 0.903 and 0.803, respectively; Delong test P = 0.116). Moreover, we repeatedly randomly split the whole data into paired training and test sets (n = 60 in both sets), and used each of the training/test sets to completely rebuild and evaluate a model. In 20 cross-validation experiments, the AUCs on the holdout test set ranged from 0.776 to 0.849 with a mean value of 0.804.
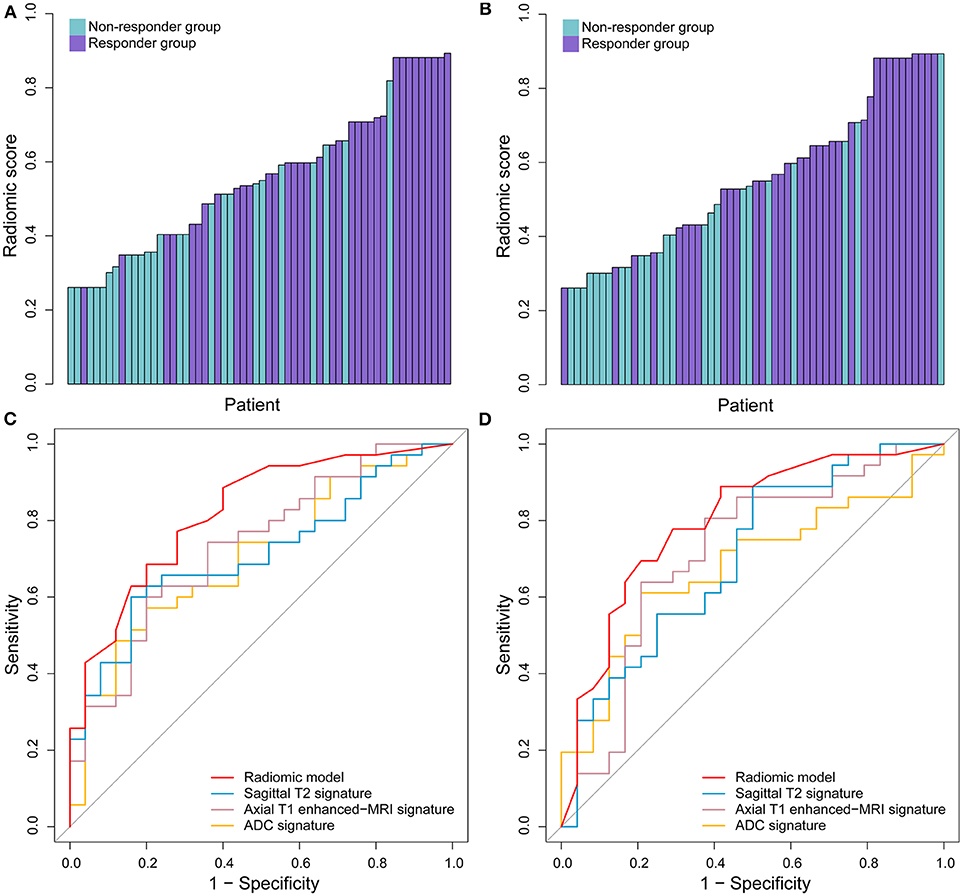
Figure 2. The performance of radiomic model in predicting the response to treatment. The radiomic model scores in the training set (A) and test set. (B) The ROC curves of radiomic model and selected three single signatures in the training set (C) and test set (D).
Discussion
In this study, a MRI radiomic model was built based on multi-habitat signatures to predict response to CCRT in locally advanced cervical cancer. The radiomic model, which consisted of three habitat signatures, had good predictive performance in both training and test sets. Moreover, our model performed better than those with single habitat signature or clinical characteristics.
We used multi-habitat signatures from different MRI sequences. These sequences reflected different characteristics of tumors, including morphological, physiological, and metabolic characteristics. DWI MRI sequence uses ADC for quantification and comparison in functional imaging (22), which may reflect the biological heterogeneity of tumors. DWI-ADC changes early during radiotherapy for cervical cancer, predicting tumor responses on MRI (23). The signatures from DWI scans apparently conflict with the observations on routine MRI. This could be the result of DWI scans being assessed only qualitatively (e.g., signal intensity, texture), as there is no established ADC value that is a diagnostic for lesion tissue. T2 MRI images can detect tumor intensity, and T1 enhanced-MRI images may reflect intratumoral heterogeneity (15).
Intratumor heterogeneity was thought to influence the CCRT response (14, 24). Most locally advanced cervical tumors present with high intratumoral heterogeneity in virtually all distinguishable phenotypes, such as proliferation, vascularity, metabolism, oxygenation, etc., which directly suggests tumor resistance to therapy (25). Although histopathological samples can be acquired by surgery or biopsy, tumor heterogeneity may lead to sampling errors. Such data are available only after completion of all pre-operative treatment and surgery and cannot be used as guidance for adjusting the therapeutic approach before or during treatment. Identification of a biomarker that could illuminate the individual's biological behavior and aid in identifying those who are less likely to benefit from CCRT and should thus undergo alternative treatment or intensive follow-up regimens would help with further classifications of locally advanced cervical cancers. Predicting tumor response to CCRT accurately before treatment has been a major challenge in the era of personalized medicine, and no generally accepted biomarker for cervical cancer has been reported (26). Being a non-invasive and low-cost method, radiomics may offer an innovative solution to this problem.
In this study, we explored 1,026 quantitative features to uncover the tumor characteristics (27, 28). A main challenge of this study is to reduce high dimensional feature set to a representative subset that is most closely associated with the responses. For each habitat, we reduced the 114 radiomic features to a signature with a few key features. Multiple cross-validation with three machine learning methods was then applied to the nine signatures and three signatures were selected for model building. These steps could reduce the complexity of the model and avoid overfitting.
The incidence of cervical carcinoma is related to certain risk factors, such as early sexual intercourse, frequent pregnancies, abortion, births, and a family history of cancer (29). We included these factors into the model, but they proved to be less significant (P > 0.05).
This study had some limitations. First of all, our study may carry some degree of selection bias. All data were obtained from a single research center, and patients may not have been randomized strictly. Further validation of the method based on external centers and large-scale cohorts is needed. Secondly, the selection of patients in the advanced clinical stage may limit the generalizability of our findings to other stages. Additionally, to ensure the reproducibility and stability of the radiomic model, the same scanning sequences and parameters were used for all patients. Therefore, the model needs to be tested on data with different MRI machines and scanning parameters.
In summary, we have identified an association between the habitat signatures and responses to CCRT in locally advanced cervical cancer. This association may have a clinical impact on selecting individualized therapies for patients with a rather reliable predicted outcome, before or during treatment.
Data Availability Statement
The datasets for this article are not publicly available because of patient information privacy. Requests to access the datasets should be directed to JT, amllLnRpYW5AaWEuYWMuY24=.
Ethics Statement
Ethical approval for this retrospective study was granted by the ethics committee of the Cancer Hospital of China Medical University, and the informed consent for patients was waived.
Author Contributions
MF, YK, and DD contributed equally to this work. JT and YL conceived and launched this study. MF, DD, and CH designed the medical and statistical analysis. YK, TY, NZ, and WJ collected cases and implemented the control of image quality and clinical diagnosis. MF and DD analyzed the data and carried out statistical experiments. MF, YK, DD, and LZ provided result interpretation. MF, YK, and DD wrote the first draft of this manuscript. LZ, JT, and YL revised and edited the final version. All authors reviewed and approved the manuscript.
Funding
This work was supported by the National Key R&D Program of China (2017YFC1308700, 2017YFA0205200, and 2017YFC1309100), National Natural Science Foundation of China (91959130, 81971776, 81771924, 81930053, 81671851, and 81527805), the Beijing Natural Science Foundation (L182061), and the Youth Innovation Promotion Association CAS (2017175).
Conflict of Interest
The authors declare that the research was conducted in the absence of any commercial or financial relationships that could be construed as a potential conflict of interest.
Supplementary Material
The Supplementary Material for this article can be found online at: https://www.frontiersin.org/articles/10.3389/fonc.2020.00563/full#supplementary-material
Abbreviations
ADC, apparent diffusion coefficient; AIC, Akaike's information criterion; AUC, area under the curve; CCRT, concurrent chemotherapy and radiation therapy; CI, confidence interval; CR, complete response; DWI, diffusion-weighted MRI; FIGO, International Federation of Gynecology and Obstetrics; GLCM, gray-level co-occurrence matrix; GLRLM, gray-level run-length matrix; IBSI, Image Biomarker Standardization Initiative; ICC, inter-class correlation coefficient; LASSO, least absolute shrinkage and selection operator; MRI, magnetic resonance imaging; NCCN, National Comprehensive Cancer Network; PD, progressive disease; PR, partial response; ROC, receiver operator characteristics; SD, stable disease; VIF, variance inflation factor.
References
1. Siegel RL, Miller KD, Jemal A. Cancer statistics, 2015 CA Cancer J Clin. (2014) 65:5–29. doi: 10.3322/caac.21254
2. Chen W, Zheng R, Baade PD, Zhang S, Zeng H, Bray F, et al. Cancer statistics in China, 2015. CA: Cancer J Clin. (2016) 66:115–32. doi: 10.3322/caac.21338
3. National Comprehensive Cancer Network (NCCN) guidelines. Available online: http://www.nccn.org/.
4. Markman M. Chemoradiation in the management of cervix cancer: current status and future directions. Oncology. (2013) 84:246–50. doi: 10.1159/000346804
5. Kastritis E, Bamias A, Efstathiou E, Gika D, Bozas G, Zorzou P, et al. The outcome of advanced or recurrent non-squamous carcinoma of the uterine cervix after platinum-based combination chemotherapy. Gynecol Oncol. (2006) 99:376–82. doi: 10.1016/j.ygyno.2005.06.024
6. Schwarz JK, Wahab S, Grigsby PW. Prospective phase I-II trial of helical tomotherapy with or without chemotherapy for postoperative cervical cancer patients. Int J Radiat Oncol Biol Phys. (2011) 81:1258–63. doi: 10.1016/j.ijrobp.2010.07.038
7. Balleyguier C, Sala E, Da Cunha T, Bergman A, Brkljacic B, Danza F, et al. Staging of uterine cervical cancer with MRI: guidelines of the european society of urogenital radiology. Eur Radiol. (2011) 21:1102–10. doi: 10.1007/s00330-010-1998-x
8. Dimopoulos JC, Schirl G, Baldinger A, Helbich TH, Pötter R. MRI assessment of cervical cancer for adaptive radiotherapy. Strahlentherapie Onkologie. (2009) 185:282–7. doi: 10.1007/s00066-009-1918-7
9. Davnall F, Yip CS, Ljungqvist G, Selmi M, Ng F, Sanghera B, et al. Assessment of tumor heterogeneity: an emerging imaging tool for clinical practice?. Insights Imaging. (2012) 3:573–89. doi: 10.1007/s13244-012-0196-6
10. Lambin P, Rios-Velazquez E, Leijenaar R, Carvalho S, van Stiphout RG, Granton P, et al. Radiomics: extracting more information from medical images using advanced feature analysis. Eur J Cancer. (2012) 48:441–6. doi: 10.1016/j.ejca.2011.11.036
11. Gillies RJ, Kinahan PE, Hricak H. Radiomics: images are more than pictures, they are data. Radiology. (2016). 278:563–77. doi: 10.1148/radiol.2015151169
12. Dong D, Tang L, Li ZY, Fang MJ, Gao JB, Shan XH, et al. Development and validation of an individualized nomogram to identify occult peritoneal metastasis in patients with advanced gastric cancer, Ann Oncol. (2019). 30:431–8. doi: 10.1093/annonc/mdz001
13. Lambin P, Leijenaar RTH, Deist TM, Peerlings J, de Jong EEC, van Timmeren J, et al. Radiomics: the bridge between medical imaging and personalized medicine. Nat Rev Clin Oncol. (2017) 14:749–62. doi: 10.1038/nrclinonc.2017.141
14. Junttila MR, de Sauvage FJ. Influence of tumour micro-environment heterogeneity on therapeutic response. Nature. (2013) 501:346–54. doi: 10.1038/nature12626
15. Zhang B, Tian J, Dong D, Gu D, Dong Y, Zhang L, et al. Radiomics features of Multiparametric MRI as novel prognostic factors in advanced nasopharyngeal carcinoma. Clin Cancer Res. (2017) 23:4259–69. doi: 10.1158/1078-0432.CCR-16-2910
16. Van Griethuysen JJM, Trebeschi S, Beets-Tan R, Lambregts DMJ, Lahaye M, Bakers FCH, et al. Radiomics signature of primary diffusion MR for treatment response prediction in rectal carcinoma. Eur J Cancer. (2017) 72:S58–8. doi: 10.1016/S0959-8049(17)30268-X
17. Song J, Shi J, Dong D, Fang M, Zhong W, Wang K, et al. A new approach to predict progression-free survival in stage IV EGFR-mutant NSCLC patients with EGFR-TKI therapy, Clin Cancer Res. (2018). 24:3583–92. doi: 10.1158/1078-0432.CCR-17-2507
18. Minig L, Patrono MG, Romero N, Rodríguez Moreno JF, Garcia-Donas J. Different strategies of treatment for uterine cervical carcinoma stage IB2-IIB. World J Clin Oncol. (2014) 5:86–92. doi: 10.5306/wjco.v5.i2.86
19. Eisenhauer EA, Therasse P, Bogaerts J, Schwartz LH, Sargent D, Ford R, et al. New response evaluation criteria in solid tumours: revised RECIST guideline (version 1.1). Eur J Cancer. (2009) 45:228–47. doi: 10.1016/j.ejca.2008.10.026
20. Zwanenburg A, Leger S, Vallières M, Löck S. Results from the image biomarker standardisation initiative. Radiother Oncol. (2016) 127:S543–4. doi: 10.1016/S0167-8140(18)31291-X
21. Sauerbrei W, Royston P, Binder H. Selection of important variables and determination of functional form for continuous predictors in multivariable model building. Stat Med. (2007) 26:5512–28. doi: 10.1002/sim.3148
22. Wang YT, Li YC, Yin LL, Pu H. Can diffusion-weighted magnetic resonance imaging predict survival in patients with cervical cancer? A meta-analysis. Eur J Radiol. (2016) 85:2174–81. doi: 10.1016/j.ejrad.2016.10.011
23. Chen J, Zhang Y, Liang B, Yang Z. The utility of diffusion-weighted MR imaging in cervical cancer. Eur J Radiol. (2010) 74:e101–6. doi: 10.1016/j.ejrad.2009.04.025
24. Dong D, Zhang F, Zhong LZ, Fang MJ, Huang CL, Yao JJ, et al. Development and validation of a novel MR imaging predictor of response to induction chemotherapy in locoregionally advanced nasopharyngeal cancer: a randomized controlled trial substudy (NCT01245959), BMC Med. (2019). 17:190. doi: 10.1186/s12916-019-1422-6
25. Yang F, Young L, Grigsby P. Predictive value of standardized intratumoral metabolic heterogeneity in locally advanced cervical cancer treated with chemoradiation. Int J Gynecol Cancer. (2016) 26:777–84. doi: 10.1097/IGC.0000000000000616
26. Bachtiary B, Boutros PC, Pintilie M, Shi W, Bastianutto C, Li JH, et al. Gene expression profiling in cervical cancer: an exploration of intratumor heterogeneity. Clin Cancer Res. (2006) 12:5632–40. doi: 10.1158/1078-0432.CCR-06-0357
27. Aerts HJ, Velazquez ER, Leijenaar RT, Parmar C, Grossmann P, Carvalho S, et al. Decoding tumour phenotype by noninvasive imaging using a quantitative radiomics approach. Nat Commun. (2014) 5:4006. doi: 10.1038/ncomms5644
28. Huang YQ, Liang CH, He L, Tian J, Liang CS, Chen X, et al. Development and validation of a radiomics nomogram for preoperative prediction of lymph node metastasis in colorectal cancer. J Clin Oncol. (2016) 34:2157–64. doi: 10.1200/JCO.2015.65.9128
Keywords: cervical cancer, MRI, radiomics, treatment response prediction, concurrent chemotherapy and radiation therapy, precision medicine
Citation: Fang M, Kan Y, Dong D, Yu T, Zhao N, Jiang W, Zhong L, Hu C, Luo Y and Tian J (2020) Multi-Habitat Based Radiomics for the Prediction of Treatment Response to Concurrent Chemotherapy and Radiation Therapy in Locally Advanced Cervical Cancer. Front. Oncol. 10:563. doi: 10.3389/fonc.2020.00563
Received: 01 November 2019; Accepted: 30 March 2020;
Published: 05 May 2020.
Edited by:
Natalie Julie Serkova, University of Colorado, United StatesReviewed by:
Xudong Shen, Guizhou Medical University, ChinaChuanming Li, Chongqing Medical University, China
Copyright © 2020 Fang, Kan, Dong, Yu, Zhao, Jiang, Zhong, Hu, Luo and Tian. This is an open-access article distributed under the terms of the Creative Commons Attribution License (CC BY). The use, distribution or reproduction in other forums is permitted, provided the original author(s) and the copyright owner(s) are credited and that the original publication in this journal is cited, in accordance with accepted academic practice. No use, distribution or reproduction is permitted which does not comply with these terms.
*Correspondence: Yahong Luo, bHVveWFob25nODg4OEBob3RtYWlsLmNvbQ==; Jie Tian, amllLnRpYW5AaWEuYWMuY24=
†These authors have contributed equally to this work