- 1Department of Pathology, Fudan University Shanghai Cancer Center, Shanghai, China
- 2Department of Oncology, Shanghai Medical School, Fudan University, Shanghai, China
- 3Canhelp Genomics Research Center, Hangzhou, Zhejiang Province, China
- 4Department of Medical Oncology, Fudan University Shanghai Cancer Center, Shanghai, China
The well-established cell-of-origin (COO) algorithm categorizes diffuse large B-cell lymphoma (DLBCL) into activated B-cell-like (ABC) and germinal center B-cell-like (GCB) subgroups through gene expression profiling. We aimed to develop and validate a qPCR-based gene expression assay to determine the COO subgroups of DLBCL with formalin-fixed paraffin-embedded (FFPE) tissue. We first established a DLBCL transcriptome database of 1,016 samples retrieved from three published datasets (GSE10846, GSE22470, and GSE31312). With this database, we identified a qPCR-based 32-gene expression signature (DLBCL-COO assay) that is significantly associated with the COO subgroups. The DLBCL-COO assay was further validated in a cohort of 160 Chinese DLBCL patients. Biopsy samples from DLBCL patients with paired FFPE and fresh frozen tissue were collected to assign COO subtypes based on the immunohistochemistry (IHC) algorithm (Han's algorithm), DLBCL-COO assay, and global gene expression profiling with RNA-seq. For 111 paired FFPE and fresh DLBCL samples, the concordance between the IHC, qPCR, and RNA-seq methods was 77.5% and 91.9%, respectively. The DLBCL-COO assay demonstrated a significantly superior concordance of COO determination with the “gold standard” RNA-seq compared with the IHC assignment with Han's algorithm (P = 0.005). Furthermore, the overall survival of GCB patients defined by the DLBCL-COO assay was significantly superior to that of ABC patients (P = 0.023). This effect was not seen when the tumors were classified by the IHC algorithm. The DLBCL-COO assay provides flexibility and accuracy in DLBCL subtype characterization. These findings demonstrated that the DLBCL-COO assay might serve as a useful tool for guiding prognostic and therapeutic options for DLBCL patients.
Introduction
Diffuse large B-cell lymphoma (DLBCL) is the most common subtype of malignant lymphomas, accounting for more than 40% of newly diagnosed cases. Although DLBCL is potentially curable with standard treatment, there is an urgent need for new therapies since most refractory or relapsed patients will eventually die from the disease. DLBCL has been recognized as a group of heterogeneous diseases with diverse genetic features and variable clinical outcomes. Almost two decades ago, Alizadeh et al. (1) performed gene expression profiling (GEP) with cDNA microarrays to explore unrecognized molecular heterogeneity in DLBCL. Using hierarchical clustering, there were at least two distinct groups within DLBCL: the germinal center B-cell-like (GCB) group and the activated B-cell-like (ABC) group. This method is widely recognized as the cell-of-origin (COO) classification algorithm. In a series of large randomized clinical studies following the establishment of COO classification, DLBCL patients with the ABC subtype showed significantly inferior characteristics compared with those with the GCB subtype, even in the clinical study evaluating the efficacy of immune chemotherapy (2).
In recent years, COO classification has been not only recognized as a prognostic factor but also used to tailor therapies for DLBCL patients (3). Additionally, COO classification or its surrogates are widely incorporated into the clinical development of state-of-the-art therapies for de novo and refractory/relapsed DLBCL patients (4). Thus, the World Health Organization (WHO) Classification for Lymphoid Malignancies required the determination of COO for every newly diagnosed DLBCL case. However, COO classification using cDNA microarrays or RNA-seq is not economical or flexible for surgical pathology laboratories and is not compatible with formalin-fixed, paraffin-embedded (FFPE) samples. Immunohistochemistry (IHC) panels, such as the Hans algorithm, may be applied as surrogates and are widely used, but there is low concordance with cDNA microarray or RNA-seq classification, and intraobserver and interobserver variation may undermine their accuracy (5). Although medium-throughput assays, such as NanoString, may be applied to FFPE samples and may be accurate compared with the “gold standard” assay (6), the integrated and enclosed platform, high price, and sophisticated workflow may limit their routine application.
In the current study, we developed a novel gene expression assay (DLBCL-COO assay) that allows differentiation between the GCB and ABC DLBCL subtypes in FFPE specimens using a quantitative reverse transcription polymerase chain reaction (qPCR) platform and evaluated the DLBCL-COO assay against RNA-seq and IHC assays. We further discussed its potential application in routine clinical practice as well as the clinical development of novel therapies for DLBCL patients.
Materials and Methods
Gene Expression Database Curation
The DLBCL gene expression datasets with confirmed COO subtypes were collected from a public data repository, the National Center for Biotechnology Information (NCBI) Gene Expression Omnibus (GEO) database, and curated to form a comprehensive DLBCL transcriptome database. The gene expression datasets retrieved from three GEO series (GSE10846, GSE22470, and GSE31312) were mainly conducted on two different Affymetrix oligonucleotide microarray platforms, including the GeneChip Human Genome U133A Array and the U133 Plus 2.0 Array. Detailed descriptions of the specimen characteristics and clinical features are provided in the original studies.
Gene Expression Data Analysis
Normalization and analysis of gene expression data were performed using R software and packages available from the Bioconductor project (www.bioconductor.org). The single-channel array normalization (SCAN) approach from the SCAN-UPC package was used to process the Affymetrix microarray data (7, 8). Upon normalizing each raw CEL file, SCAN outputs probe-level expression values. We further used custom mapping files from the BrainArray resource to summarize the probe-level intensities directly to gene-level expression values (9). Thus, probe mapping to multiple genes and other problems associated with older generations of Affymetrix probe designs were avoided. After normalization, we applied the ComBat approach to adjust for batch effects (10). To identify a gene expression signature, we used the recursive feature elimination–support vector machine (RFE-SVM) algorithm for feature selection and classification modeling (11). A linear SVM classifier was derived using the training samples with known ABC or GCB labels and applied to the test samples. When the probability predicted by the DLBCL-COO assay that a sample belongs to the ABC or GCB subgroup is >75%, the specimen is classified as the ABC or GCB subtype accordingly. Otherwise, specimens with a probability lower than 75% were considered unclassified. The Database for Annotation, Visualization and Integrated Discovery (DAVID) bioinformatics resource was used to integrate functional genomic annotations (12). A biological network was constructed by NetworkAnalyst software (www.networkanalyst.cn, version 3.0) (13, 14). Protein–protein interactions were retrieved from the IMEx Interactome Database (15).
Development of the DLBCL-COO Assay
The DLBCL-COO assay was developed on the Applied Biosystems 7500 Real-Time PCR system (Applied Biosystems, Foster City, CA, USA), targeting 32 candidate markers and three housekeeping genes identified with microarray analysis. To support clinical applications using FFPE samples with poor RNA quality, primers were designed to amplify short template mRNA regions of exon-spanning junctions. In addition, the TaqMan MGB probes incorporate a 5′ fluorescent reporter dye and a 3′ nonfluorescent quencher, which offers the advantage of lower background signal, resulting in better precision in quantitation.
Case Selection
The study was approved by the ethical committee of Fudan University Shanghai Cancer Center (Approval case number: 1904199-18). Specimens between June 1, 2012, and December 19, 2018, that were maintained at the Department of Pathology, Fudan University Shanghai Cancer Center, were retrospectively archived in the current study. All cases were reviewed by two independent pathologists (X-NJ and X-QL) in compliance with WHO classification and were histologically confirmed as de novo DLBCL, not otherwise specified (DLBCL, NOS).
Morphology and Immunohistochemistry
The following antibodies (Ventana Medical Systems, Tucson, Arizona, USA) were applied on a BenchMark XT automated immunostainer (Ventana Medical Systems, Tucson, Arizona, USA) with Cell Conditioning 1 heat retrieval solution (Ventana Medical Systems, Tucson, Arizona, USA): anti-CD10, anti-BCL6, and anti-MUM1. For all staining procedures, tonsils with reactive hyperplasia served as external controls and reactive lymphocytes as internal controls. The cut-off value for tumor positivity was set at 30% of tumor cell staining for CD10, BCL6, and MUM1. Cases were designated as GCB or non-GCB using the algorithm specified by Hans et al. (16). The morphological and IHC results were independently evaluated by two pathologists (W-HY and X-QL).
Sample Processing and qPCR Analysis
Total RNA was isolated from FFPE tissue and fresh tissue using the RecoverAll Total Nucleic Acid Isolation Kit (Thermo Fisher Scientific, Waltham, MA, USA) per the manufacturer's guidelines. The concentration of total RNA was quantified by a Qubit 3.0 Fluorometer (Thermo Fisher Scientific, Waltham, MA, USA), while RNA integrity and quality were further appraised using agarose gel electrophoresis. For each sample, reverse transcription was performed on isolated total RNA using the High-Capacity cDNA Reverse Transcription Kit with RNase Inhibitor (Applied Biosystems, Foster City, CA, USA). The PCR program consisted of an initiation step at 95°C for 10 min, followed by 40 cycles at 95°C for 15 s and 60°C for 1 min. All measurements were taken in triplicate. The melting curves of each measurement were checked; only the coordinate results were included in the subsequent analysis. Three genes (IPO8, PGK1, and TFRC) that have been reported to be consistently expressed in DLBCL cells were selected as housekeeping genes. First, qPCR results of housekeeping genes with various sample storage duration and RNA quality were investigated, and then, the average Ct value of each target gene minus the mean of three housekeeping genes was calculated as ΔCt. The –ΔCt value of each gene was applied for downstream analysis.
RNA Sequencing and Data Analyses
RNA-seq was performed on the NovaSeq 6000 system (Illumina, San Diego, CA, USA) using 1 μg of RNA extracted from fresh tumor tissue according to the manufacturer's instructions. The raw sequencing data were preprocessed using the BRB-SeqTools suite (https://github.com/DeplanckeLab/BRB-seqTools). The GEP-based classification method was performed to determine the COO molecular subtype of each specimen as described in Wright et al. (17) and Reddy et al. (18).
Statistical Analysis
For comparison with the Hans-based IHC method, all COO subtypes of samples from the GEP methods were categorized as either “GCB” or “non-GCB.” All GCB predictions remained GCB, and any “ABC” or “UNC” subtype predictions from the RNA sequencing and qPCR assays were converted to the “non-GCB” subtype. The concordance between any pair of assays was calculated using only the total number of samples that could be called by both of those assays. The overall percent agreement and asymptotic 95% confidence intervals (CIs) are presented. To determine the positive percent agreement (PPA) and negative percent agreement (NPA), the global GEP-based subtyping method served as a standard reference in each comparison. Overall survival (OS) was defined as the time from diagnosis until death or the last follow-up date. Kaplan–Meier survival curves were constructed for OS analysis. A value of p = 0.05 was determined as the level of statistical significance. All statistical analyses were performed using SPSS Statistics version 23 software (SPSS Inc., Chicago, USA).
Results
Establishment of the DLBCL Transcriptome Database
To create a DLBCL transcriptome database for COO subtype classification, we performed a systematic search of major biological data repositories [e.g., ArrayExpress, GEO, and The Cancer Genome Atlas (TCGA)] to collect gene expression data sets of DLBCL samples with a confirmed COO subtype status. Overall, we accumulated the gene expression profiles of 1,016 samples to form a comprehensive DLBCL transcriptome database. We further adopted a training–testing–validation approach to identify and validate a reliable gene expression signature in this study. First, the gene expression profiles of 167 ABC samples and 183 GCB samples were retrieved from the database and curated to form a training set. Second, two independent cohorts were used as in silico test sets to evaluate the classification performance: one was composed of the gene expression profiles of 215 fresh tumor samples (Test Set 1, 71 ABC samples and 144 GCB samples), and the other was composed of the gene expression profiles of 451 FFPE samples (Test Set 2, 214 ABC samples and 237 GCB samples). Third, the developed qPCR assay was clinically validated against the RNA-seq and IHC assays on paired fresh and FFPE samples of de novo DLBCL patients treated at Fudan University Shanghai Cancer Center (Validation Set). Figure 1 depicts the three distinct phases of our study design, and Tables 1, 2 summarize the clinical characteristics of the samples in the study.
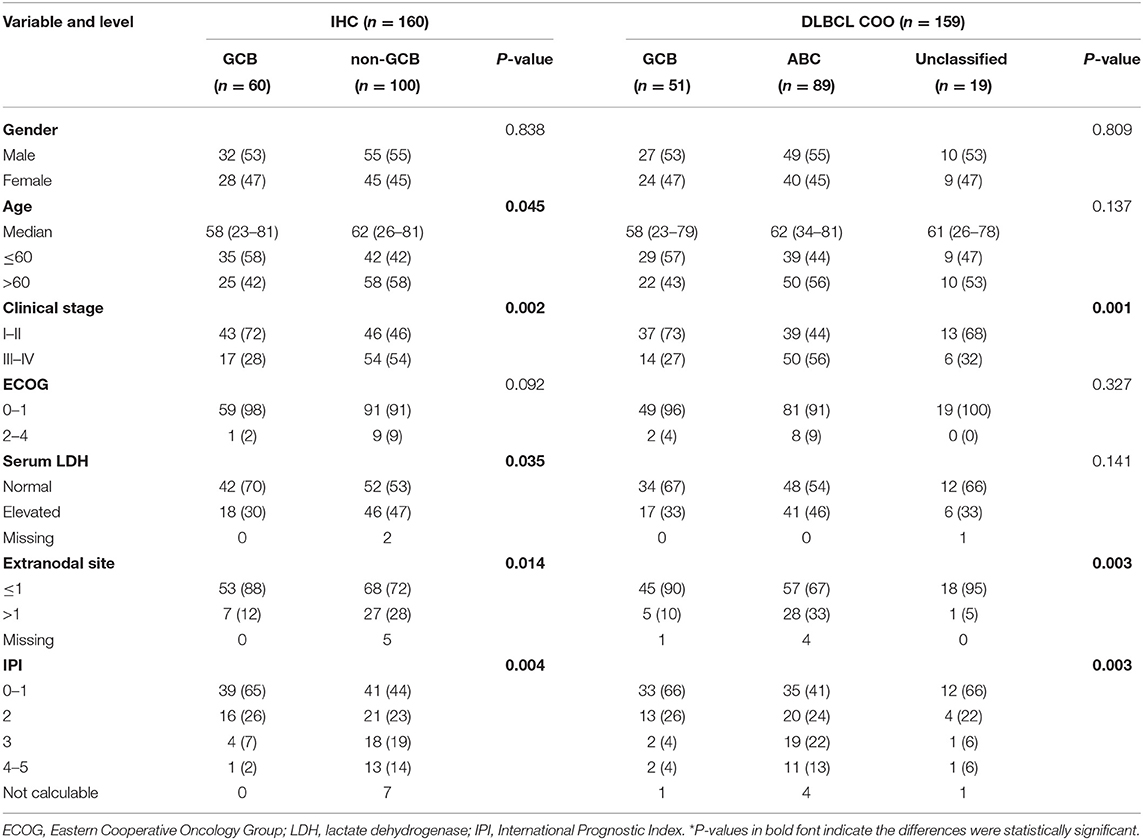
Table 2. Clinical characteristics of diffuse large B-cell lymphoma (DLBCL) patients stratify by immunohistochemistry (IHC) and DLBCL-COO.
Identification of a 32-Gene Expression Signature in the Training Set
The training set consisted of 167 ABC and 183 GCB samples. After the data normalization and annotation steps, a matrix of 20,342 unique genes in 350 samples (≈7.12 million data points) was prepared for downstream bioinformatics analyses. Extracting a subset of informative genes from high-dimension genomic data is a critical step for gene expression signature identification. Here, we deployed the RFE-SVM algorithm with the linear SVM classification model and the parameter C equal to 1. The algorithm identified a compact panel of 32 genes that are significantly associated with the two molecular subtypes. As listed in Table 3, 16 genes were overexpressed in the ABC subtype, and 16 genes were overexpressed in the GCB subtype. We further investigated whether the 32 candidate genes exhibited biological features relevant to the DLBCL molecular subtypes. As shown in Table 4, the most significantly enriched gene categories are involved in B-cell differentiation, B-cell activation, humoral immune response, and hemopoiesis. We also explored the underlying biological networks of the selected candidate genes. We used the 32 genes as seeds to generate a minimum protein–protein interaction network. The network comprised 21 genes of the 32-gene set and was centered on essential nodes such as BCL6, UBC, AICDA, LMO2, UCHL1, and MME (Figure S1).
Independent Validation in Fresh and FFPE DLBCL Samples
The classification model comprising 32 subtype-specific genes was established using the entire training set and then applied to Test Set 1, which was composed of 71 ABC and 144 GCB fresh frozen samples. With the 32-gene expression signature, 69 samples were classified as ABC and 146 as GCB. The overall agreement between the 32-gene expression signature classification and the reference diagnosis reached 97.2% (209 of 215; 95% CI: 0.94–0.99). The PPA was 98.6% (142/144, 95% CI: 0.95–1.00), and the NPA was 94.4% (67/71, 95% CI: 0.85–0.98), considering GCB as positive (Table 5). It was of interest to evaluate the classification performance of the gene expression signature in FFPE samples. We further applied the 32-gene expression signature to Test Set 2, which was composed of 214 ABC and 237 GCB FFPE samples. Of the 451 samples, the 32-gene expression signature classified 239 as ABC and 212 as GCB. The agreement between the gene expression-based assignments and the reference diagnoses reached 93.6% (422 of 451; 95% CI: 0.909–0.957). The PPA was 88.6% (210/237, 95% CI: 0.84–0.92), and the NPA was 99.1% (212/214, 95% CI: 0.96–1.00), considering GCB as positive (Table 5).
Clinical Validation of the 32-Gene Expression Signature by qPCR Analysis
A total of 160 DLBCL patients with confirmed COO subtypes based on IHC assignment were enrolled in the current study. Han's algorithm assigned 60 cases (37.5%) as GCB and 100 cases (62.5%) as non-GCB. One hundred fifty-nine of 160 FFPE specimens met all criteria and were successfully assayed by the DLBCL-COO assay. We first evaluated the hierarchical clustering of the 32 genes and 159 samples based on the qPCR data. Complete linkage hierarchical clustering analysis was performed where the metric of similarity was Pearson's correlation between the 32-gene expression profiles of the samples. As shown in Figure 2, the samples were clustered into distinct groups that followed the COO subtypes. Among the three subtypes, most GCB samples clustered together, whereas the unclassified samples were more similar to ABC samples. According to the predictions by the 32-gene signature, 89 cases (56.0%) were classified as ABC, 51 cases (32.1%) as GCB, and 19 cases (11.9%) as unclassified. In addition, 113 DLBCL patients had paired fresh frozen tissue, and 111 cases passed stringent quality control for RNA-seq analysis. The gold standard RNA-seq method defined 34 cases (30.6%) as GCB, 50 cases (45.1%) as ABC, and 27 cases (24.3%) as unclassified. The concordance between DLBCL-COO and RNA-seq and the concordance between IHC Han's algorithm and RNA-seq are summarized in Table 6. The DLBCL-COO assay demonstrated a significantly superior concordance of COO determination with the gold standard RNA-seq compared with the IHC assignment with Han's algorithm (91.9 vs. 77.5%; P = 0.005). Additionally, the PPA and NPA of the DLBCL-COO assay assigning GCB/non-GCB were 88.2% (30 of 34, 95% CI: 0.72–0.96) and 93.5% (72 of 77, 95% CI: 0.85–0.98), respectively.
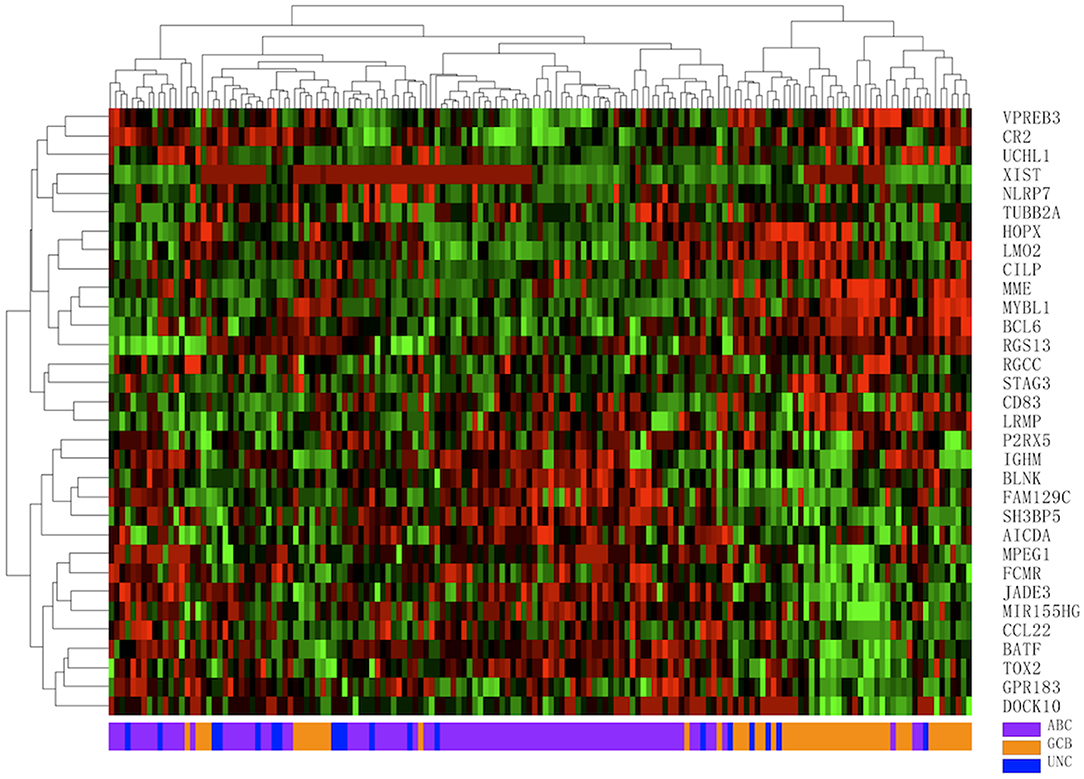
Figure 2. Hierarchical clustering analysis of 32 gene expression profiles in 159 diffuse large B-cell lymphoma (DLBCL) specimens. Colored pixels capture the magnitude of the expression for each gene, where shades of red and green represent over-expression and under-expression, respectively. Right: the official symbol of 32 genes; left: a dendrogram of hierarchical clustering of these genes. Bottom: COO subgroups including germinal center B-cell-like (GCB, orange), activated B-cell-like (ABC, purple), unclassified (UNC, blue); top: a dendrogram of hierarchical clustering of all samples.
One hundred twenty-nine DLBCL cases with survival information, IHC assignment results, and DLBCL-COO assay results were identified. The clinical information related to the IHC and DLBCL-COO assignment results is summarized in Table 2. Han's algorithm failed to stratify DLBCL patients, mostly treated with the R-CHOP (rituximab, cyclophosphamide, doxorubicin, vincristine, and prednisone) regimen, into different prognostic groups (Figure 3A) (P = 0.091). However, the OS of GCB patients defined by the DLBCL-COO assay was significantly superior to that of ABC patients (Figure 3B) (P = 0.023). The patients assigned as unclassified DLBCL had an intermediate OS (Figure 3B).
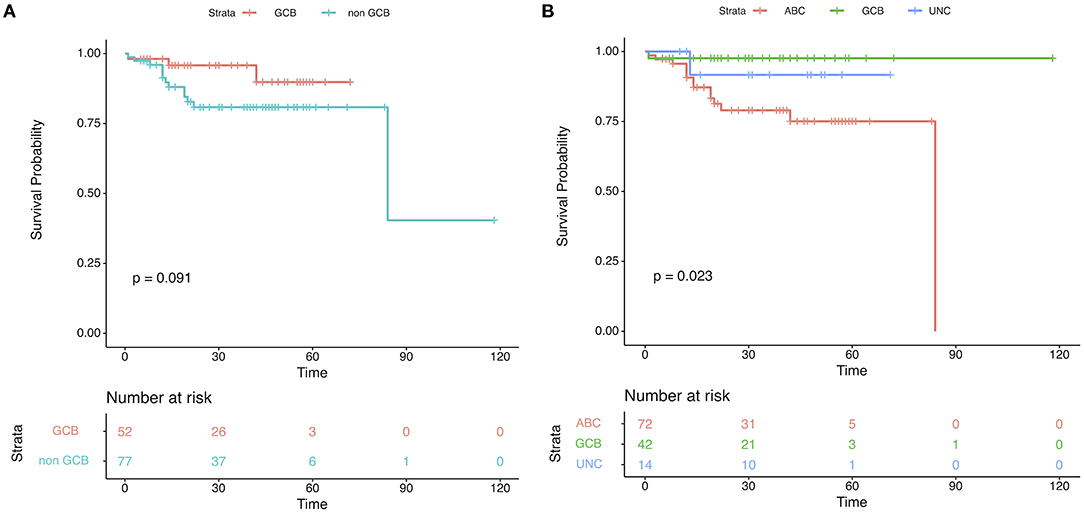
Figure 3. Patient outcomes according to cell-of-origin (COO) in the validation set. Kaplan–Meier plots of overall survival based on molecular subgroups stratified by (A) immunohistochemistry (IHC) and (B) DLBCL-COO assay. P-values were obtained using the log-rank test. UNC, Unclassified.
Discussion
As DLBCL is a heterogeneous disease in genetic, biological, and clinical behavior, precise classification is critical for predicting prognosis or the efficacy of therapies. Characterizing DLBCL into GCB and ABC based on COO represents a milestone in the heterogeneity delineation of DLBCL. These COO classification results successfully correlated with the patient outcome, even in the era of immunochemotherapy with rituximab (2). The COO classification system demonstrated the different cancer biology and etiologies in DLBCL, making it possible to tailor therapies to different subgroups of patients. The most exciting application of COO classification may be the efficacy prediction of BTK inhibitors and lenalidomide in treating refractory or relapsed DLBCL patients (19, 20). Although randomized phase three clinical studies evaluating the efficacy of BTK inhibitors and lenalidomide in treating treatment-naive DLBCL based on the COO classification failed (21, 22), COO determination for newly diagnosed DLBCL patients is still mandatory. Several novel classification systems based on the DLBCL genetic landscape have been proposed recently, like four genetic subtypes based on the status of MYD88 L265P, CD79b mutations, NOTCH1 mutations, BCL6 fusion, NOTCH2 mutations, BCL2 translocations, and EZH2 mutations (23). However, these systems highly interacted with the COO classification (23, 24), indicating that COO classification may be the backbone of other state-of-the-art classification algorithms.
As the gold standard of COO determination is global GEP based on cDNA microarray or RNA-seq, which is inaccessible for routine testing, the most widely used and flexible COO surrogate is IHC. However, the interobserver and intraobserver reproducibility of IHC COO assignment are not satisfactory, and the concordance across different IHC COO algorithms is quite low (25). The IHC COO assignment failed to predict the outcome of DLBCL patients treated with immunochemotherapy (26) and failed to predict the efficacy of the BTK inhibitor ibrutinib in treating DLBCL (27). Several medium through-put assays compatible with FFPE samples have been developed in recent years, demonstrating high concordance with global GEP based on cDNA microarray or RNA-seq (6). Nonetheless, the complexity of the assay based on a specific platform (NanoString) or the Illumina sequencer and the high cost may potentially limit its wide application in routine practice, especially in poor resource areas.
Therefore, it is necessary to establish a COO determination assay with appropriate cost, comparable accuracy with the gold standard assay, and compatibility with FFPE samples. The qPCR technology is generally considered the “gold standard” procedure for measuring individual gene expression and is often used to confirm the findings of DNA microarray and RNA-seq analyses. Furthermore, the qPCR technology can be easily applied to FFPE specimens, and thus, it is widely applicable in clinical practice. Recently, Tekin et al. reported a successful validation of a qPCR-based six-gene predictor for DLBCL prognosis in an international clinical study (28). Herein, the DLBCL-COO assay is a qPCR assay that detects a 32-gene expression profile for DLBCL molecular classification. The DLBCL-COO assay was trained against the so-called gold standard of COO assignment using GEP on fresh frozen tissue, tested, and then validated in multiple independent cohorts. Although a slight loss in signal intensities was observed when FFPE sample storage duration increased (Figure S2), the qPCR-based TaqMan assays remained accurate and robust for gene expression profiling. The overall successful rate of the DLBCL-COO assay is satisfactory (159/160, 99%), even for the FFPE samples archived 5 years ago, indicating satisfactory compatibility with FFPE samples. This may be critical for relapsed or refractory DLBCL, as biopsied samples may be archived for several years. Regarding accuracy, the concordance of the DLBCL-COO assay with the gold standard RNA-seq assay was 91.9%, which was comparable to the NanoString and HTG assays, even though there is a lack of head-to-head studies, suggesting that the COO assignment by DLBCL-COO is precise.
In addition to routine clinical practice, the clinical development of novel therapies for DLBCL also requires a COO assignment assay with high accuracy and consistency, and short turnover duration. In the PHOENIX study, which is a randomized, double-blind, placebo-controlled, multicenter, phase 3 study comparing the efficacy and safety of ibrutinib in combination with R-CHOP vs. placebo in combination with R-CHOP in patients with the newly diagnosed non-GCB subtype of DLBCL, GEP showed that 75.9% of patients with non-GCB DLBCL assigned by IHC had ABC DLBCL (23). As central pathology COO assignment and review were applied in this well-controlled study, the concordance between the IHC COO assignment and GEP assignment may be much lower. In another phase 3 study evaluating the efficacy of R-CHOP plus lenalidomide in previously untreated ABC DLBCL (ROBUST study), the NanoString Lymph2Cx GEP assay was applied to assign COO, demonstrating 15% failure in the test (29). As the samples from previously untreated DLBCL patients were recently biopsied in the ROBUST study, the failure rate may be higher for the long-archived samples of relapsed and refractory DLBCL patients. In these settings, a more compatible assay beyond GEP as well as a more accurate assay beyond IHC will be more effectively incorporated into clinical development.
Conclusion
In conclusion, the DLBCL-COO assay provides flexibility and accuracy in DLBCL subtype characterization into GCB and ABC. These subtype distinctions should help guide disease prognosis and treatment options in DLBCL clinical practice. Further prospective studies including incorporation into prospective interventional studies will be needed to evaluate the performance in detail.
Data Availability Statement
Publicly available datasets were analyzed in this study, these can be found in the NCBI Gene Expression Omnibus (GSE10846, GSE22470, GSE147986, and GSE31312).
Ethics Statement
The studies involving human participants were reviewed and approved by the Clinical Research Ethics Committee of Fudan University Shanghai Cancer Center. The patients/participants provided their written informed consent to participate in this study.
Author Contributions
W-HY, X-NJ, W-GW, and X-QL designed the study. W-HY, X-NJ, W-GW, Y-FS, Y-XW, and Z-ZL performed the experiments. W-HY, Y-FS, and Q-HX analyzed all data. W-HY, X-NJ, W-GW, and Y-FS wrote the initial manuscript draft. Q-HX, X-YZ, J-NC, X-NH, and X-QL critically revised the manuscript and gave valuable insight to the study concept. All authors revised the manuscript and read and approved the final manuscript.
Funding
This work was partially supported by research funding from the Guide Project of Science and Technology Commission of Shanghai Municipality (134119a5000) and Canhelp Genomics Co., Ltd.
Conflict of Interest
Y-FS, Y-XW, Z-ZL and Q-HX are employed by Canhelp Genomics Co., Ltd. The authors declare that this study received funding from Canhelp Genomics Co., Ltd. The funder had the following involvement with the study: data collection and analysis, and preparation of the manuscript.
The remaining authors declare that the research was conducted in the absence of any commercial or financial relationships that could be construed as a potential conflict of interest.
Supplementary Material
The Supplementary Material for this article can be found online at: https://www.frontiersin.org/articles/10.3389/fonc.2020.00803/full#supplementary-material
References
1. Alizadeh AA, Eisen MB, Davis RE, Ma C, Lossos IS, Rosenwald A, et al. Distinct types of diffuse large B-cell lymphoma identified by gene expression profiling. Nature. (2000) 403:503–11. doi: 10.1038/35000501
2. Gutierrez-Garcia G, Cardesa-Salzmann T, Climent F, Gonzalez-Barca E, Mercadal S, Mate JL, et al. Gene-expression profiling and not immunophenotypic algorithms predicts prognosis in patients with diffuse large B-cell lymphoma treated with immunochemotherapy. Blood. (2011) 117:4836–43. doi: 10.1182/blood-2010-12-322362
3. Rutherford SC, Leonard JP. DLBCL cell of origin: what role should it play in care today? Oncology. (2018) 32:445–9.
4. Hernandez-Ilizaliturri FJ, Deeb G, Zinzani PL, Pileri SA, Malik F, Macon WR, et al. Higher response to lenalidomide in relapsed/refractory diffuse large B-cell lymphoma in nongerminal center B-cell-like than in germinal center B-cell-like phenotype. Cancer. (2011) 117:5058–66. doi: 10.1002/cncr.26135
5. Saad AG, Grada Z, Bishop B, Abulsayen H, Hassan M, Firpo-Betancourt A, et al. nCounter nanostring assay shows variable concordance with immunohistochemistry-based algorithms in classifying cases of diffuse large B-cell lymphoma according to the cell-of-origin. Appl Immunohistochem Mol Morphol. (2019) 27:644–8. doi: 10.1097/PAI.0000000000000696
6. Scott DW, Wright GW, Williams PM, Lih CJ, Walsh W, Jaffe ES, et al. Determining cell-of-origin subtypes of diffuse large B-cell lymphoma using gene expression in formalin-fixed paraffin-embedded tissue. Blood. (2014) 123:1214–7. doi: 10.1182/blood-2013-11-536433
7. Piccolo SR, Sun Y, Campbell JD, Lenburg ME, Bild AH, Johnson WE. A single-sample microarray normalization method to facilitate personalized-medicine workflows. Genomics. (2012) 100:337–44. doi: 10.1016/j.ygeno.2012.08.003
8. Piccolo SR, Withers MR, Francis OE, Bild AH, Johnson WE. Multiplatform single-sample estimates of transcriptional activation. Proc Natl Acad Sci USA. (2013) 110:17778–83. doi: 10.1073/pnas.1305823110
9. Dai M, Wang P, Boyd AD, Kostov G, Athey B, Jones EG, et al. Evolving gene/transcript definitions significantly alter the interpretation of GeneChip data. Nucleic Acids Res. (2005) 33:e175. doi: 10.1093/nar/gni179
10. Johnson WE, Li C, Rabinovic A. Adjusting batch effects in microarray expression data using empirical Bayes methods. Biostatistics. (2007) 8:118–27. doi: 10.1093/biostatistics/kxj037
11. Guyon I, Weston J, Barnhill S, Vapnik V. Gene selection for cancer classification using support vector machines. Mach Learn. (2002) 46:389–422. doi: 10.1023/A:1012487302797
12. Huang da W, Sherman BT, Lempicki RA. Systematic and integrative analysis of large gene lists using DAVID bioinformatics resources. Nat Protoc. (2009) 4:44–57. doi: 10.1038/nprot.2008.211
13. Xia J, Benner MJ, Hancock RE. NetworkAnalyst–integrative approaches for protein-protein interaction network analysis and visual exploration. Nucleic Acids Res. (2014) 42:W167–74. doi: 10.1093/nar/gku443
14. Xia J, Gill EE, Hancock RE. NetworkAnalyst for statistical, visual and network-based meta-analysis of gene expression data. Nat Protoc. (2015) 10:823–44. doi: 10.1038/nprot.2015.052
15. Breuer K, Foroushani AK, Laird MR, Chen C, Sribnaia A, Lo R, et al. InnateDB: systems biology of innate immunity and beyond–recent updates and continuing curation. Nucleic Acids Res. (2013) 41:D1228–33. doi: 10.1093/nar/gks1147
16. Hans CP, Weisenburger DD, Greiner TC, Gascoyne RD, Delabie J, Ott G, et al. Confirmation of the molecular classification of diffuse large B-cell lymphoma by immunohistochemistry using a tissue microarray. Blood. (2004) 103:275–82. doi: 10.1182/blood-2003-05-1545
17. Wright G, Tan B, Rosenwald A, Hurt EH, Wiestner A, Staudt LM. A gene expression-based method to diagnose clinically distinct subgroups of diffuse large B cell lymphoma. Proc Natl Acad Sci USA. (2003) 100:9991–6. doi: 10.1073/pnas.1732008100
18. Reddy A, Zhang J, Davis NS, Moffitt AB, Love CL, Waldrop A, et al. Genetic and functional drivers of diffuse large B cell lymphoma. Cell. (2017) 171:481–94.e415. doi: 10.1016/j.cell.2017.09.027
19. Wilson WH, Young RM, Schmitz R, Yang Y, Pittaluga S, Wright G, et al. Targeting B cell receptor signaling with ibrutinib in diffuse large B cell lymphoma. Nat Med. (2015) 21:922–6. doi: 10.1038/nm.3884
20. Thieblemont C, Tilly H, Gomes da Silva M, Casasnovas RO, Fruchart C, Morschhauser F, et al. Lenalidomide maintenance compared with placebo in responding elderly patients with diffuse large B-cell lymphoma treated with first-line rituximab plus cyclophosphamide, doxorubicin, vincristine, and prednisone. J Clin Oncol. (2017) 35:2473–81. doi: 10.1200/JCO.2017.72.6984
21. Vitolo U, Chiappella A, Witzig TE, Spina M, Gascoyne RD, Zhang L, et al. Real-time cell-of-origin subtype identification by gene expression profile in the phase 3 robust trial of lenalidomide plus R-CHOP vs PLACEBO plus R-CHOP in previously untreated ABC-type DLBCL. Haematologica. (2016) 101:392.
22. Younes A, Sehn LH, Johnson P, Zinzani PL, Hong X, Zhu J, et al. Randomized phase III trial of ibrutinib and rituximab plus cyclophosphamide, doxorubicin, vincristine, and prednisone in non-germinal center B-cell diffuse large B-cell lymphoma. J Clin Oncol. (2019) 37:1285–95. doi: 10.1200/JCO.18.02403
23. Schmitz R, Wright GW, Huang DW, Johnson CA, Phelan JD, Wang JQ, et al. Genetics and pathogenesis of diffuse large B-cell lymphoma. N Engl J Med. (2018) 378:1396–407. doi: 10.1056/NEJMoa1801445
24. Chapuy B, Stewart C, Dunford AJ, Kim J, Kamburov A, Redd RA, et al. Molecular subtypes of diffuse large B cell lymphoma are associated with distinct pathogenic mechanisms and outcomes. Nat Med. (2018) 24:679–90. doi: 10.1038/s41591-018-0016-8
25. Coutinho R, Clear AJ, Owen A, Wilson A, Matthews J, Lee A, et al. Poor concordance among nine immunohistochemistry classifiers of cell-of-origin for diffuse large B-cell lymphoma: implications for therapeutic strategies. Clin Cancer Res. (2013) 19:6686–95. doi: 10.1158/1078-0432.CCR-13-1482
26. Barraclough A, Alzahrani M, Ettrup MS, Bishton M, van Vliet C, Farinha P, et al. COO and MYC/BCL2 status do not predict outcome among patients with stage I/II DLBCL: a retrospective multicenter study. Blood Adv. (2019) 3:2013–21. doi: 10.1182/bloodadvances.2019000251
27. Winter AM, Landsburg DJ, Mato AR, Isaac K, Hernandez-Ilizaliturri FJ, Reddy N, et al. A multi-institutional outcomes analysis of patients with relapsed or refractory DLBCL treated with ibrutinib. Blood. (2017) 130:1676–9. doi: 10.1182/blood-2017-05-786988
28. Tekin N, Omidvar N, Morris TP, Conget P, Bruna F, Timar B, et al. Protocol for qRT-PCR analysis from formalin fixed paraffin embedded tissue sections from diffuse large b-cell lymphoma: validation of the six-gene predictor score. Oncotarget. (2016) 7:83319–29. doi: 10.18632/oncotarget.13066
Keywords: diffuse large B-cell lymphoma, cell-of-origin, gene expression profiling, immunohistochemistry, quantitative polymerase reaction (PCR), formalin-fixed paraffin-embedded tissue
Citation: Yan W-H, Jiang X-N, Wang W-G, Sun Y-F, Wo Y-X, Luo Z-Z, Xu Q-H, Zhou X-Y, Cao J-N, Hong X-N and Li X-Q (2020) Cell-of-Origin Subtyping of Diffuse Large B-Cell Lymphoma by Using a qPCR-based Gene Expression Assay on Formalin-Fixed Paraffin-Embedded Tissues. Front. Oncol. 10:803. doi: 10.3389/fonc.2020.00803
Received: 22 February 2020; Accepted: 23 April 2020;
Published: 05 June 2020.
Edited by:
Shimin Hu, University of Texas MD Anderson Cancer Center, United StatesReviewed by:
Hui Liu, Affiliated Hospital of Xuzhou Medical University, ChinaMariko Yabe, Cornell University, United States
Copyright © 2020 Yan, Jiang, Wang, Sun, Wo, Luo, Xu, Zhou, Cao, Hong and Li. This is an open-access article distributed under the terms of the Creative Commons Attribution License (CC BY). The use, distribution or reproduction in other forums is permitted, provided the original author(s) and the copyright owner(s) are credited and that the original publication in this journal is cited, in accordance with accepted academic practice. No use, distribution or reproduction is permitted which does not comply with these terms.
*Correspondence: Xiao-Qiu Li, bGVleGlhb3FpdUBob3RtYWlsLmNvbQ==
†These authors have contributed equally to this work
‡Present address: Wei-Ge Wang, BeiGene Shanghai, Ltd., Shanghai, China