- 1Department of Biliary Tract Surgery I, Eastern Hepatobiliary Surgery Hospital, Shanghai, China
- 2Winchester School of Art, University of Southampton, Southampton, United Kingdom
- 3Department of Medicine, Beijing Medicinovo Technology Co., Ltd., Beijing, China
- 4School of Computer Science, University of Sydney, Sydney, NSW, Australia
- 5Department of Medicine, Dalian Medicinovo Technology Co., Ltd., Dalian, China
Background and Objectives: Currently, the prognostic performance of the staging systems proposed by the 8th edition of the American Joint Committee on Cancer (AJCC 8th) and the Liver Cancer Study Group of Japan (LCSGJ) in resectable intrahepatic cholangiocarcinoma (ICC) remains controversial. The aim of this study was to use machine learning techniques to modify existing ICC staging strategies based on clinical data and to demonstrate the accuracy and discrimination capacity in prognostic prediction.
Patients and Methods: This is a retrospective study based on 1,390 patients who underwent surgical resection for ICC at Eastern Hepatobiliary Surgery Hospital from 2007 to 2015. External validation was performed for patients from 2015 to 2017. The ensemble of three machine learning algorithms was used to select the most important prognostic factors and stepwise Cox regression was employed to derive a modified scoring system. The discriminative ability and predictive accuracy were assessed using the Concordance Index (C-index) and Brier Score (BS). The results were externally validated through a cohort of 42 patients operated on from the same institution.
Results: Six independent prognosis factors were selected and incorporated in the modified scoring system, including carcinoembryonic antigen, carbohydrate antigen 19-9, alpha-fetoprotein, prealbumin, T and N of ICC staging category in 8th edition of AJCC. The proposed scoring system showed a more favorable discriminatory ability and model performance than the AJCC 8th and LCSGJ staging systems, with a higher C-index of 0.693 (95% CI, 0.663–0.723) in the internal validation cohort and 0.671 (95% CI, 0.602–0.740) in the external validation cohort, which was then confirmed with lower BS (0.103 in internal validation cohort and 0.169 in external validation cohort). Meanwhile, machine learning techniques for variable selection together with stepwise Cox regression for survival analysis shows a better prognostic accuracy than using stepwise Cox regression method only.
Conclusions: This study put forward a modified ICC scoring system based on prognosis factors selection incorporated with machine learning, for individualized prognosis evaluation in patients with ICC.
Introduction
Intrahepatic cholangiocarcinoma (ICC) is a malignant neoplasm originating from the epithelial cells of bile ducts located above the secondary bile duct branch (1). It is the second most common primary malignancy of liver and its incidence has been increasing in recent years (2–4). Surgical resection is the main potentially curative for ICC, the 5-year overall survival (OS) rates after hepatectomy and lymphadenectomy is 15 to 35% (5–9). Appropriate staging for ICC patients can be used to describe the severity and range of involvement of malignant tumors, thus prompting clinicians to understand the prognosis of the disease.
Now the eighth edition of American Joint Committee on Cancer (AJCC 8th) staging system and the Liver Cancer Study Group of Japan (LCSGJ) staging system are widely used in clinical practice (10–13). Although studies have demonstrated that the modified AJCC staging system improves stratifying ability, it remains controversial (14, 15). The LCSGJ staging system focuses on the hepatocellular carcinoma (HCC) which has distinct differences in biological behaviors and postoperative outcomes (16). Some new stratification strategies begin to incorporate readily available clinical parameters, such as carbohydrate antigen 19-9 (CA19-9), alkaline phosphatase (ALP) and alpha-fetoprotein (AFP) (17–19). To more effectively utilize these clinical parameters, not just on surgical-pathological factors, we combined the robust machine learning methods to analyze the high-dimension data in clinical practice.
Meanwhile, the selection of variables which involved in the outcome imputation was significant for staging performance. In similar studies, multivariate analysis using Cox regression to identify the independent prognostic factors for survival was a common method, such as the ICC prognostic staging systems performed by Zhou et al. (19), the modified staging system for mass-forming ICC (16), the Fudan score (17), and in nomogram predicting strategies (18). In present study, we attempted to improve the conventional survival analysis by combining with machine learning algorithms for variable selection, since in the real-world studies, variables are not always independent to each other and they are closely related in the non-linear way. The normal used multivariate analysis methods or linear models cannot capture the complex relationships of variables, which are machine learning methods skilled in, especially we used decision tree-based ensemble methods, i.e., eXtreme Gradient Boosting (XGBoost), random forest (RF), and gradient boosted decision tree (GBDT). The three methods are able to divide and re-aggregate the variables to achieve the minimum prediction error when growing sub-trees. Through this way, the non-linear relationship between variables can be well captured. In addition, they are all with the ability of learning from data with missing values directly, that can better adapt to the data situation in the real world. To confirm their effectiveness, we performed the three variable selection methods for comparison and our proposed method outperforms others by a significant margin. Moreover, our study also incorporated the prognostic factors for TNM staging as an improvement of traditional strategy.
The objective of the current study is to integrate pathological factors and clinical parameters to construct a useful and personalized scoring system with machine learning methods, which can accurately predict the survival outcomes of ICC patients under surgical resection.
Materials and Methods
Patients Cohort
The cohort comprised 1,390 pathologically confirmed ICC patients who underwent hepatectomy between January 2007 and October 2015 at the Eastern Hepatobiliary Surgery Hospital (EHBH) in Shanghai, China, which is a high-volume medical center. The data collection was cut-off on November, 2018. Patients diagnosed with Perihilar (Klatskin) tumors and mixed with hepatocellular carcinoma tumors were excluded. All deaths were confirmed to have occurred after ICC recurrence to avoid the interference of competing mortality. The data collection and tumor staging processes were supervised and examined by two pathologists. The patients in external validation cohort (n=42, January 2016 to June 2017) were screened with the same criteria of the internal cohort. The data collection was cut-off on June, 2020. Variable characteristic statistics of the training cohort and external validation cohort were summarized in Supplemental Table and Supplementary Data of Entire Cohort. The protocol of this study has been approved by the Ethics Committee of the EHBH, and the informed consent has been exempted in the Ethical approval documents.
We collected data of 27 clinical independent variables including provided basic clinical information (age, gender, jaundice, history of stone, history of tumor, and smoking), laboratory results [blood type, hepatitis B virus (HBV), CA19-9, γ-glutamyltranspeptidase (γ-GT), albumin (Alb), alanine aminotransferase (ALT), ALP, prealbumin (PA), aspartate aminotransferase (AST), carcinoembryonic antigen (CEA), AFP, direct bilirubin (DBIL), and total bilirubin (TBIL)], and perioperative data (T/N/M or TNM stage in AJCC 8th, T or TNM stage in LCSGJ, resection type, and tumor size). All laboratory examinations were performed within 1 week before resection or intervention. To be applicable to machine learning, all relevant variables were cleansed and converted into numerical codes.
Study Design
The aim of this research was to construct a more accurate and simple ICC scoring system for predicting the prognosis after resection based on the clinical factors and stages. Overall Survival for 3 years after resection was the end point in our study. We enriched many types of variables in the initial cohort, and variable selection was implemented via three machine learning methods, i.e., XGBoost, RF, and GBDT. The algorithms calculated the contribution of each independent variable to the target variable and obtained the importance score (IS). We combined the intersection variables with the highest IS for further analysis.
Cox proportional hazard models with backward stepwise regression were used to evaluate the impacts of intersection variables on survival, and the prognostic scoring equation was obtained. Overall, the predictive accuracy and discrimination ability between models were compared. In addition, for validating the advantages of the research methods, we compared survival predictions with/without machine learning screening. Since the data collection and research were implemented in the Eastern Hepatobiliary Surgery Hospital (Shanghai, China), this scoring strategy we proposed is simply called EHBH-ICC in the later section. The overall study process is illustrated in Figure 1.
Tumor, Node, Metastasis Stage
The 8th edition of AJCC and the LCSGJ staging manual in patients who underwent operations were adopted as baseline models for performance comparison (1, 20).
Machine Learning
In the process of machine learning modeling, we chose the XGBoost, RF, and GBDT for the variable selection, which are capable of dealing with missing values under certain assumptions and do not require data imputation. Since our data was derived from real-world settings with a small number of missing values, machine learning methods with incomplete data learning ability are necessary. We performed these three algorithms using Scikit-learn: a machine learning framework (https://www.scikit-learn.org/stable/) in Python 3.6.8. In order to achieve their best performance, the AutoML (https://github.com/ClimbsRocks/auto_ml) method was adopted to automatically select their hyperparameters.
Statistical and Survival Analysis
Data statistics were characterized as quantity (%) or median (interquartile range, IQR). Mann-Whitney U test and chi-square were used on continuous variables and categorical variables respectively, and p<0.05 was considered statistically significant. Relevant prognostic predictors were evaluated by the Cox proportional hazard model using backward stepwise regression (Wald-test, p<0.05 represents a significant difference). We ensured comparability of the training and internal validation cohorts, a random distribution was applied in a ratio of 8:2. To estimate the influence of prognostic factors, the hazard ratio (HR) was calculated. Kaplan-Meier analysis was used in survival analysis and log-rank test was adopted to compare significant differences. The Concordance Index (C-index) and Brier Score (BS) were utilized to evaluate the discrimination ability and predictive performance of the staging methods. The higher C-index indicates, the better discrimination ability of the model. BS was an important measure of model calibration, i.e., the mean squared difference between the predicted probability and the actual outcome. The lower BS value indicates the higher prediction accuracy of the model. Statistical analysis and modeling were performed using Python (version 3.6.8) and R Studio (version 1.1.463).
Results
Clinicopathologic Characteristics of Patients
A total of 1,390 patients underwent surgical resection for ICC during the study period. Twenty-seven types of variables included in the primary entire cohort were sorted out and input into the models, patients’ demographic information, medical history, tumor information, and examination information were contained in modeling and reported in Table 1. The median survival time was 15.5 months (IQR 7.7 to 27.7 months). Of all ICC patients in this study, there were 560 of them (40.3%) having a survival of less than 1 year, 576 patients (41.4%) died between 1 and 3 years after surgery, while 254 (18.2%) died after 3 years. There were 939 females (67.6%) and 451 males (32.4%) enrolled in the study, with a male-to-female ratio of 1:2.1. Among study population, 316 patients (22.7%) had HBV infection. TNM staging and T classification of AJCC 8th and LCSGJ were evaluated. The T classification (AJCC 8th) includes the extents or existence of tumor diameter, vascular invasion, solitary or multiple tumors, perforation of the visceral peritoneum, and direct invasion of local extrahepatic structures. Nodal and metastasis categories’ conditions between the two staging systems were similar, so we counted them together. Only one patient was diagnosed with T1b, that is, had a tumor size larger than 5 cm and without vascular invasion, T1a and T1b tumors were combined in the following study.
Selection and Comparison of Prognostic Factors
The IS of variables, most relevant to patient OS for 3 years were calculated by XGBoost, RF, and GBDT, the top 20 important variables selected from which were assembled in Table 2. Then we extracted the intersection of the above variables, and the retained 15 important variables were ALP, γ-GT, N, T, Alb, tumor size, AST, DBIL, TBIL, PA, ALT, AFP, CEA, CA19-9, and age. Among the variables, IS of T staging of AJCC 8th were higher than that of LCSGJ staging system, therefore T (AJCC 8th) was adopted and used “T” as a general name in the following analysis. Variables screened by machine learning participated in developing the Cox proportional hazard regression model. Table 3 counted the variables in training cohort (n=1,112) used for modeling and the internal validation cohort (n=278) used for verification. The median survival time (months) of training cohort and internal validation cohort was 15.6 (IQR: 7.9–27.7) and 15.3 (IQR: 7.1–27.4), respectively. The data distribution among all factors in cohorts had relative equilibrium (p>0.05).
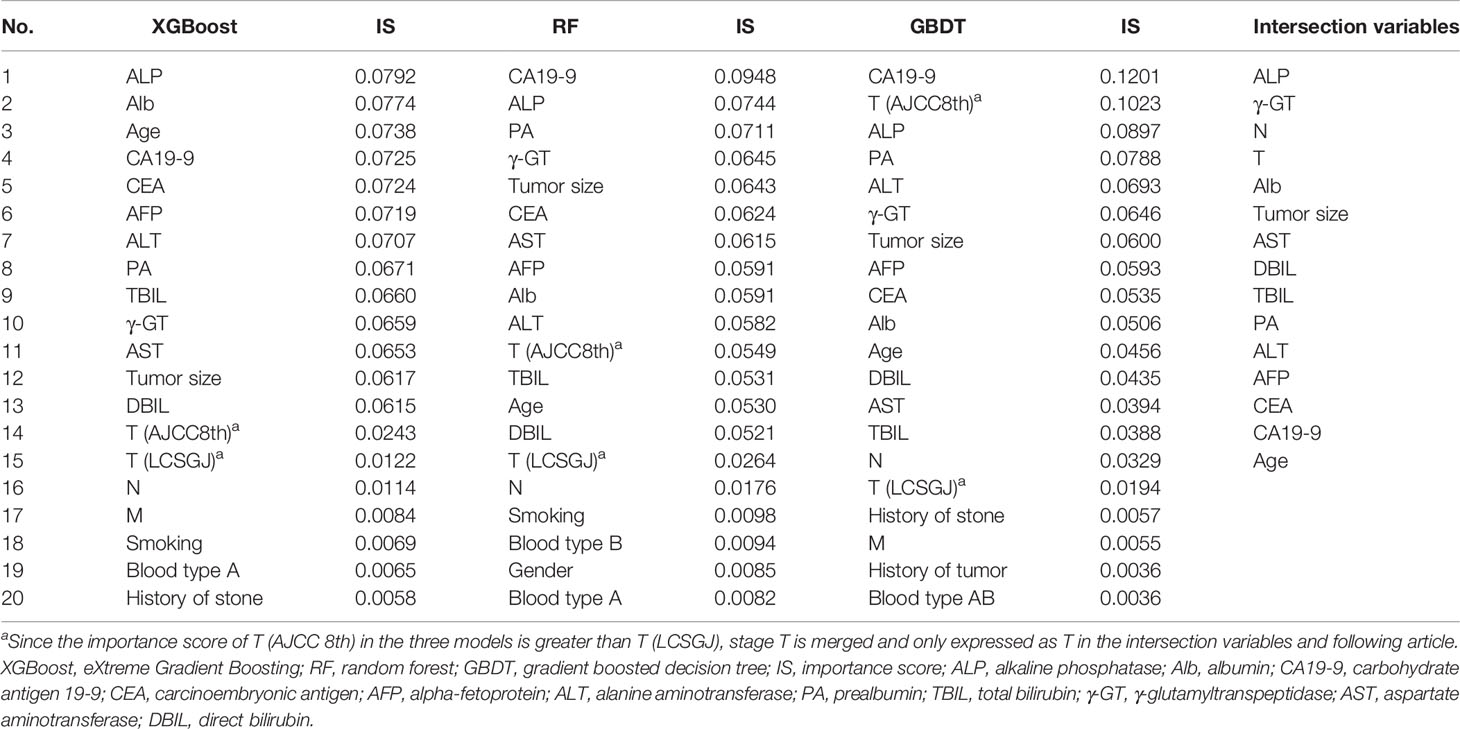
Table 2 The important variables calculated by XGBoost, random forest (RF), and gradient boosted decision tree (GBDT), and their intersection variables.
The data sets in Table 3 were used to perform the Cox regression model, and further screened through backward stepwise regression (p<0.05). The results of backward stepwise regression are demonstrated in Table 4. The natural logarithmic transformation was applied on the continuous variables to avoid deviation of data distribution. Multivariate analysis by stepwise regression revealed that T classification of AJCC 8th (HR, 1.204; 95% CI, 1.142–1.270), N (HR, 1.927; 95% CI, 1.655–2.243), ln (CEA) (HR, 1.158; 95% CI, 1.098–1.221), ln (CA19-9) (HR, 1.127; 95% CI, 1.085–1.171), ln (AFP) (HR, 1.057; 95% CI, 1.019–1.096), and ln (PA) (HR, 0.830; 95% CI, 0.714–0.964) were determined to be independent predictors of 3-year OS in ICC patients.
Variable Selection Methods Comparison
The Cox regression models with stepwise selection were commonly used in similar studies to select variables, which significantly associated with the prognostic outcome after ICC resection. To verify whether the variable selection incorporated machine learning algorithms can improve the model accuracy or not, we performed three approaches for comparison: only by Cox proportional hazards model with backward stepwise regression (namely SR), only by machine learning (namely ML), and combining both methods (SR+ML) (Figure 2). By establishing the survival prediction models, the C-index (Figure 2A) and BS (Figure 2B) of the above three approaches were obtained, and the results demonstrated that SR+ML (C-index, 0.693; BS, 0.115) had better performance in the most of survival time than only ML and only SR. Therefore, machine learning was proven to capture the prognostic predictors of postoperative outcome more accurately during variable processing, consequently improving the prediction performance of the model. The influenced factors selected via only SR including: sex, age, history of stone, smoking habit, HBV, T, N, M, CA19-9, PA, CEA, DBIL, TBIL, excision, and the blood type A. The variables screening results of SR via Cox analysis were summarized in Supplemental Table 2.
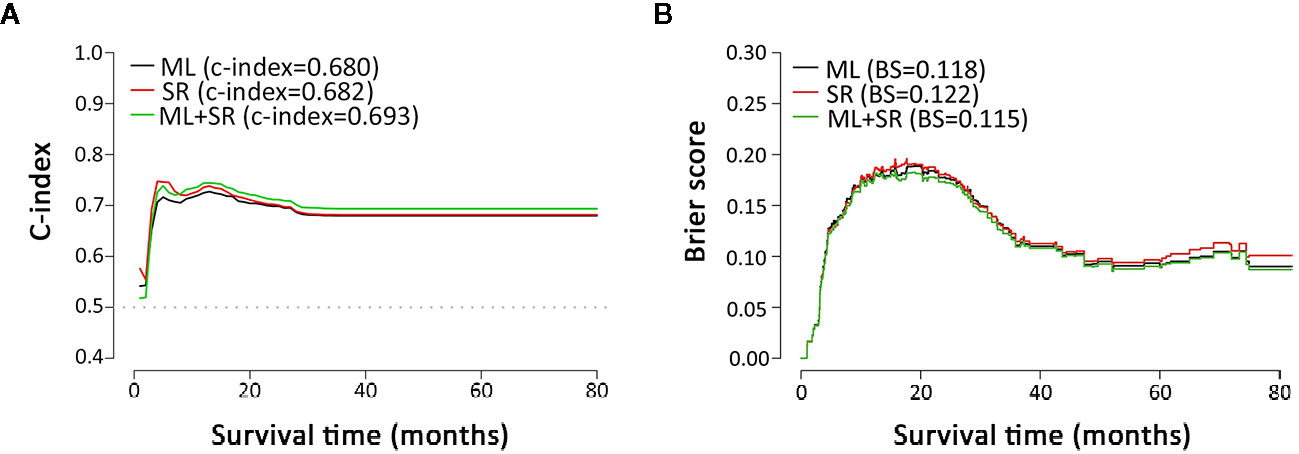
Figure 2 Metrics comparison of models based on different multivariate analysis approaches. (A, B) are C-index and brier score comparisons of models based on multivariate analysis by ML, SR, and ML+SR, respectively. ML, machine learning; SR, stepwise regression.
Establishment and Evaluation of Eastern Hepatobiliary Surgery Hospital-Intrahepatic Cholangiocarcinoma Scoring System
Based on the Cox regression, the range of the prognostic index for each individual is from −1.2 to 2.4. In order to adjust the score in our proposed scoring system into positive, we obtained the EHBH-ICC scoring formula as follows:
Histograms of survival risk score distribution for training cohort and internal validation cohort were built based on our EHBH-ICC score (Figures 3A, B). According to the score distribution, we divided patients into four risk groups: low (0–10), moderate (11–20), high (21–30), and extremely high (>30). The median risk scores in training and internal validation cohorts were 16.3 and 17.0, respectively. Figure 4A displays the good prognostic stratification for patients between stages in internal validation cohort (log rank p<0.001).
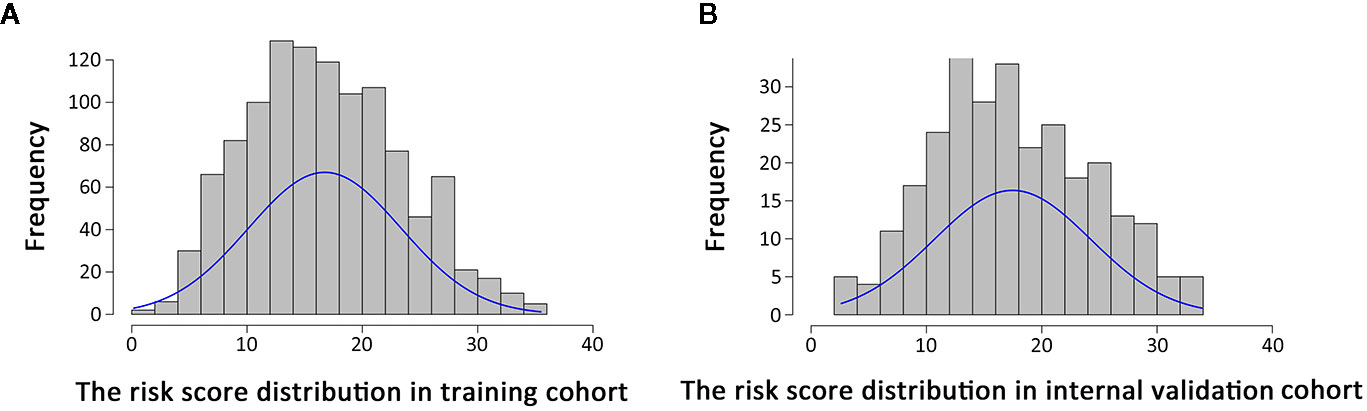
Figure 3 Distribution of risk scores in patients using Eastern Hepatobiliary Surgery Hospital-intrahepatic cholangiocarcinoma (EHBH-ICC) scoring system. (A, B) are risk score distributions in training cohort (n=1,112, median=16.3) and internal validation cohort (n=278, median=17.0), respectively.
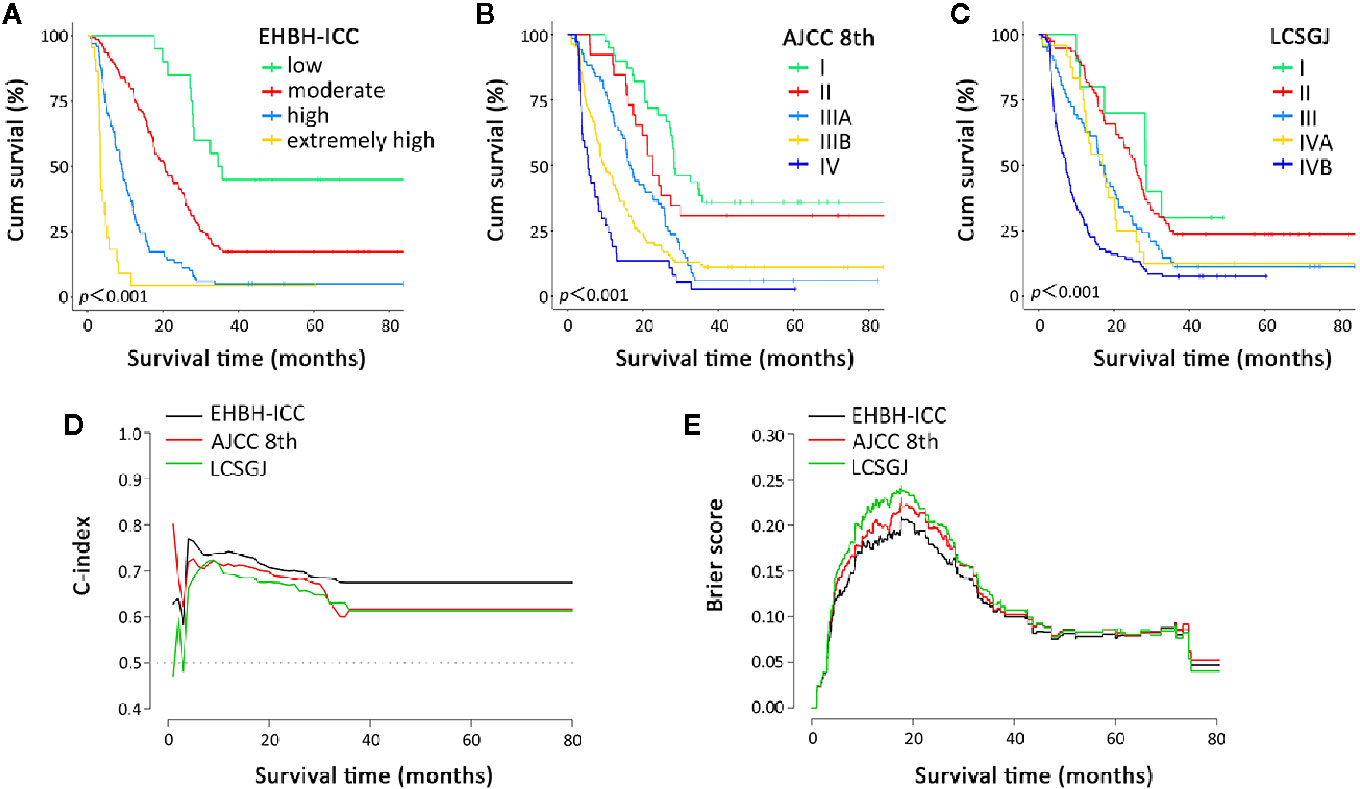
Figure 4 Overall survival curves and prognostic performance indicator curves in the Eastern Hepatobiliary Surgery Hospital-intrahepatic cholangiocarcinoma (EHBH-ICC), American Joint Committee on Cancer (AJCC) 8th, and the Liver Cancer Study Group of Japan (LCSGJ) staging systems. (A–C) depict the overall survival according to the three staging systems in internal validation cohort, all log rank p<0.001. (D, E) present the C-index and brier score change in long-term survival, respectively.
Comparison of Predictive Accuracy for Overall Survival in Eastern Hepatobiliary Surgery Hospital-Intrahepatic Cholangiocarcinoma, American Joint Committee on Cancer 8th and the Liver Cancer Study Group of Japan Staging System
Further, we made a comparison of the EHBH-ICC staging system with AJCC 8th and the LCSGJ staging systems. Since time-to-mortality and time-to-event were crucial to interpret the results, Figures 4A–C depict the Kaplan-Meier curves of the three different staging systems. All of three systems in our study appeared a progressive decrease in OS during the study period. The log-rank test proved that all these staging methods have p<0.001.
The discrimination ability and prediction performance of EHBH-ICC score model in internal validation cohort and external validation cohort were respectively indicated with higher C-index of 0.693 (95% CI, 0.663–0.723) and 0.671 (95% CI, 0.602–0.740) than the AJCC 8th and LCSGJ staging systems, which were then confirmed with lower probability calibration of BS (0.103 in internal validation cohort and 0.169 external validation cohort). Detailed C-index and BS results are presented in Table 5 and Figures 4D, E. The model evaluation results show that the EHBH-ICC score was the most precise in predicting the survival after resection in this study.
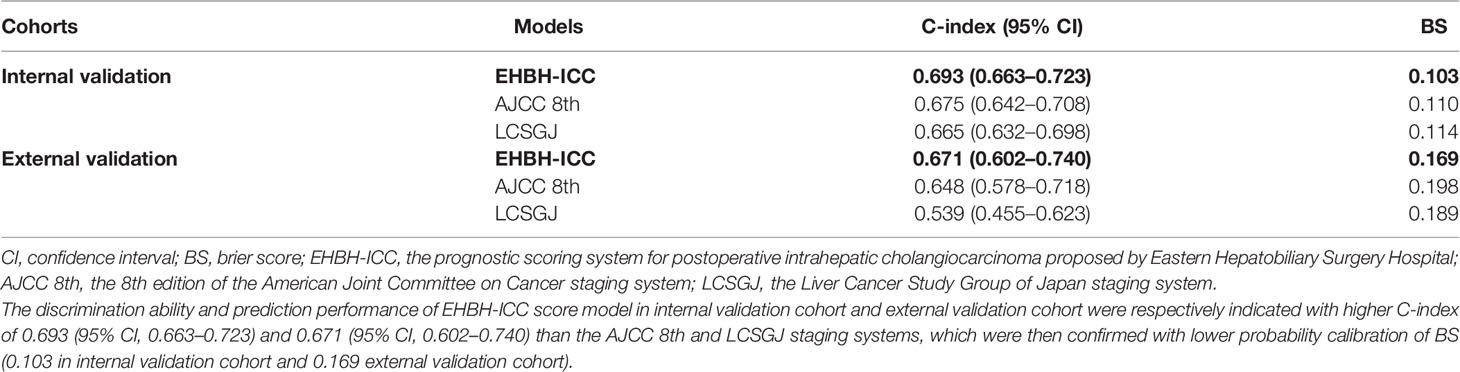
Table 5 The comparison of Eastern Hepatobiliary Surgery Hospital (EHBH)-intrahepatic cholangiocarcinoma (ICC), American Joint Committee on Cancer (AJCC) 8th and the Liver Cancer Study Group of Japan (LCSGJ) staging system in internal and external validation cohorts.
Discussion
ICC is the second most common primary hepatic malignancies after HCC with increasing incidence and mortality worldwide (21, 22). Hepatectomy is considered as the mainstay of curative option for ICC (23). Accurate tumor staging provides the prognostic details, evaluates the risk level appropriately, as well as assists the choice of adjuvant therapeutic options.
At present, the most commonly used staging systems for ICC are the TNM classification systems, among which, the AJCC 8th and LCSGJ are widely approbatory. With relentless efforts of AJCC to improve the prognostic staging of ICC, there are still research evidences that it is inadequate. T1b with single lesion larger than 5 cm without vascular invasion in AJCC was often rare in clinical treatments. And some recent studies indicated that stage II and stage IIIA for ICC patients in AJCC edition failed to show significant prognostic differentiation. Survival time for intrahepatic metastases was sometimes lower than in patients with serous membrane protruding tumors; however, these patients were only at T2 stage. Some recent studies assessed the prognostic performance of the 7th and 8th edition versions of AJCC staging system, proving that there was no remarkable improvement in overall prognostic discrimination, especially in the staging of T3 category (14, 24, 25). While the LCSGJ focuses on the HCC which has distinct differences in biological behaviors and postoperative outcomes. Some modified staging systems for resectable ICC reserved the prognostic factors in TNM classification or combined these two systems as one of the predictors (19, 26). In our investigation, we analyzed the diagnoses of both staging systems above as separate independent variables. We hypothesized that pathology factors are important prognostic factors for postoperative ICC patients but are only partially relevant. Our study was based on multi-dimensional clinical real-world data in relatively larger population, thus we could seek factors affecting postoperative survival of ICC patients with a wider perspective.
We derived 15 important factors by three algorithms concurrently (Table 2), and further identified T (AJCC 8th) and N classifications, CEA, CA19-9, AFP, PA as the prognostic predictive factors. Multiple potential tumor biomarkers have been used in evaluating the prognosis of ICC (27–29). For now, many researches have constructed some new assessment systems with diagnostic biomarkers to predict the survival of patients, such as CA19-9, AFP, CEA, ALP, and PA (17, 19, 30). These factors were confirmed by our results and were involved in the outcome scoring of ICC patients. Serum CA 19-9 and CEA were most investigated in prognosis of ICC (17, 18, 31). Jaklitsch et al. had proven that the inclusion of preoperative CA 19-9 and CEA in AJCC and LCSGJ staging systems improved the prognostic survival prediction after resection for ICC (32). Serum AFP is a widely used tumor marker of HCC (33), and the positive serum AFP (>20 ng/ml) is seen in approximately 19% of ICC patients (34). Zhou et al. showed that the lymph node metastasis rate was low in ICC patients with positive AFP (35). PA generated by liver is commonly regarded as a sensitive marker of nutritional status. A study reported that patients with lower PA have poorer outcomes in ICC (19), which is consistent with our result that PA level is negatively associated with the score. Compared with pathological factors, clinical parameters are easier to obtain and can also provide valuable reference. In our EHBH-ICC scoring system, the diagnosis of T and N and the laboratory results can be directly substituted into the calculation to obtain the corresponding risk level scores.
To our knowledge, our report is the first ICC staging method developed based on machine learning models. In recent years, machine learning-based methods are widely used in diagnosis, treatment and outcome prediction such as prostate cancer (36), renal cancer (37), non-small cell lung cancer (38), and cardiovascular event prediction (39). Machine learning can deal with different data types even if data are incomplete or incoherent comparing with traditional statistics. Many studies have demonstrated the advantages of machine learning algorithms over traditional statistical methods (40).
According to the EHBH-ICC scoring system, patients are divided into four survival risk grades (low to extremely high). This is a scoring approach to predict the outcome of resectable ICC in Chinese population. The other scoring approach, for instance, the Fudan scoring system was only conducted for 344 patients with multivariate Cox regression. Compared with the Fudan scoring system, the EHBH-ICC has different calculation methods and key prognostic factors. A similarity between Fudan scoring system and our system was the discovery and application of the prognostic value of readily available clinical parameters. Our ultimate validation methods of discrimination ability and performance were C-index and BS. The EHBH-ICC scoring system (C-index, 0.693; BS, 0.103) has more accurate prognostic prediction for ICC patients via comparison with the AJCC 8th and LCSGJ edition (Figures 4D, E).
In our study, patients’ tumor diversity was well reflected. With the continuously increasing sample size, the evaluation system will be more optimized to predict the prognosis of patients more accurately to make decision of the treatment. We cannot only obtain the proportion of risk factors in the prognosis of patients, but also accurately predict the prognosis of patients with the increasing score via machine learning.
However, there are limitations in our study. Our study is a retrospective study in one single center. More medical centers and samples could be added to optimize our evaluation system and solve the limitation. In conclusion, the EHBH-ICC scoring system shows good predictive ability for ICC patients who underwent surgical operation via evaluation and comparison with existing staging systems (the AJCC 8th and LCSGJ). The machine learning-based EHBH-ICC scoring system can effectively evaluate the ICC prognosis after resections and be used in clinical practice.
Data Availability Statement
The original contributions presented in the study are included in the article/Supplementary Material; further inquiries can be directed to the corresponding authors.
Ethics Statement
The protocol of this study has been approved by the Ethics Committee of the Eastern Hepatobiliary Surgery Hospital, and the informed consent has been exempted in the Ethical approval documents.
Author Contributions
ZL and LY conceptualized the study. JS and ZW contributed to the methodology. JS and CZ conducted the formal analysis and investigation. ZL, YW, and XH wrote and prepared the original draft. FG and XJ provided the resources and supervised the study. All authors contributed to the article and approved the submitted version.
Conflict of Interest
JS and FG were employed by company Beijing Medicinovo Technology Co., Ltd. YW and XH were employed by company Dalian Medicinovo Technology Co., Ltd.
The remaining authors declare that the research was conducted in the absence of any commercial or financial relationships that could be construed as a potential conflict of interest.
Supplementary Material
The Supplementary Material for this article can be found online at: https://www.frontiersin.org/articles/10.3389/fonc.2020.576901/full#supplementary-material.
References
1. Liver Cancer Study Group of Japan. General rules for the clinical and pathological study of primary liver cancer. Frist English edition. Tokyo: Kanehara & Co Ltd (1997).
2. Nathan H, Pawlik TM, Wolfgang CL, Choti MA, Cameron JL, Schulick RD. Trends in survival after surgery for cholangiocarcinoma: a 30 -year population based SEER database analysis. J Gastrointest Surg (2007) 11:1488–96. doi: 10.1007/s11605-007-0282-0
3. Njei B. Changing pattern of epidemiology in intrahepatic cholangiocarcinoma. Hepatology (2014) 60:1107–8. doi: 10.1002/hep.26958
4. Saha SK, Zhu AX, Fuchs CS, Brooks GA. Forty-year trends in cholangiocarcinoma incidence in the U.S.: Intrahepatic disease on the rise. Oncologist (2016) 21:594–9. doi: 10.1634/theoncologist.2015-0446
5. Bridgewater J, Galle PR, Khan SA, Park JW, Patel T, Pawlik TM, et al. Guidelines for the diagnosis and management of intrahepatic cholangiocarcinoma. J Hepatol (2014) 60:1268–89. doi: 10.1016/j.jhep.2014.01.021
6. Doussot A, Groot-Koerkamp B, Wiggers JK, Chou J, Gonen M, DeMatteo RP, et al. Outcomes after resection of intrahepatic cholangiocarcinoma: External validation and comparison of prognostic models. J Am Coll Surg (2015) 221:452–61. doi: 10.1016/j.jamcollsurg.2015.04.009
7. Guglielmi A, Ruzzenente A, Campagnaro T, Pachera S, Valdegamberi A, Nicoli P, et al. Intrahepatic cholangiocarcinoma: prognostic factorsafter surgical resection. World J Surg (2009) 33:1247–54. doi: 10.1007/s00268-009-9970-0
8. Lang H, Sotiropoulos GC, Sgourakis G, Schmitz KJ, Paul A, Hilgard P, et al. Operations for intrahepatic cholangiocarcinoma: single-institution experience of 158 patients. J Am Coll Surg (2009) 208:218–28. doi: 10.1016/j.jamcollsurg.2008.10.017
9. Lin XH, Luo JC. The risk factors and prognostic factors of intrahepatic cholangiocarcinoma. J Chin Med Assoc (2017) 80:121–2. doi: 10.1016/j.jcma.2016.12.001
10. Farges O, Fuks D. Clinical presentation andmanagement of intrahepatic cholangiocarcinoma. Gastroenterol Clin Biol (2010) 34:191–9. doi: 10.1016/j.gcb.2010.01.006
11. Ohtsuka M, Ito H, Kimura F, Shimizu H, Togawa A, Yoshidome H, et al. Results ofsurgical treatment for intrahepatic cholangiocarcinoma and clinicopathological factors influencing survival. Br J Surg (2002) 89:1525–31. doi: 10.1046/j.1365-2168.2002.02268.x
12. Amin MB, Greene FL, Edge SB, Compton CC, Gershenwald JE, Brookland RK, et al. The Eighth Edition AJCC Cancer Staging Manual: continuing to build a bridge from a population -based to a more “personalized” approach to cancer staging. CA Cancer J Clin (2017) 67:93–9. doi: 10.3322/caac.21388
13. Kudo M, Matsui O, Izumi N, Iijima H, Kadoya M, Imai Y, et al. Surveillance and diagnostic algorithm for hepatocellular carcinoma proposed by the Liver Cancer Study Group of Japan: 2014 Update. Oncology (2014) 87 Suppl 1:7–21. doi: 10.1159/000368141
14. Spolverato G, Bagante F, Weiss M, Alexandrescu S, Marques HP, Aldrighetti L, et al. Comparative performances of the 7th and the8th editions of the American Joint Committee on Cancer staging systems for intrahepatic cholangiocarcinoma. J Surg Oncol (2017) 115:696–703. doi: 10.1002/jso.24569
15. Lee AJ, Chun YS. Intrahepatic cholangiocarcinoma: the AJCC/UICC 8th edition updates. Chin Clin Oncol (2018) 7:52. doi: 10.21037/cco.2018.07.03
16. Uenishi T, Ariizumi S, Aoki T, Ebata T, Ohtsuka M, Tanaka E, et al. Proposal of a new staging system for mass-forming intrahepatic cholangiocarcinoma: a multicenter analysis by the Study Group for Hepatic Surgery of the Japanese Society of Hepato-Biliary-Pancreatic Surgery. J Hepatobiliary Pancreat Sci (2014) 21:499–508. doi: 10.1002/jhbp.92
17. Jiang W, Zeng ZC, Tang ZY, Fan J, Sun HC, Zhou J, et al. A prognostic scoring system based on clinical features of intrahepatic cholangiocarcinoma: the Fudan score. Ann Oncol (2011) 22:1644–52. doi: 10.1093/annonc/mdq650
18. Wang Y, Li J, Xia Y, Gong R, Wang K, Yan Z, et al. Prognostic nomogram for intrahepatic cholangiocarcinoma after hepatectomy. J Clin Oncol (2013) 31:1188–95. doi: 10.1200/JCO.2012.41.5984
19. Zhou H, Jiang X, Li Q, Hu J, Zhong Z, Wang H, et al. A simple and effective prognostic staging system based on clinicopathologic features of intrahepatic cholangiocarcinoma. Am J Cancer Res (2015) 5:1831–43.
20. American Joint Committee on Cancer. AJCC Cancer Staging Manual. 8th Edition. Amin MB, Edge S, Greene F, Byrd DR, editors. New York: Springer (2017).
21. Global Burden of Disease Cancer Collaboration, Fitzmaurice C, Dicker D, Pain A, Hamavid H, Moradi-Lakeh M, et al. The global burden of cancer 2013. JAMA Oncol (2015) 1:505–27. doi: 10.1001/jamaoncol.2015.0735
22. Aljiffry M, Abdulelah A, Walsh M, Peltekian K, Alwayn I, Molinari M. Evidence-based approach to cholangiocarcinoma: a systematic review of thecurrent literature. J Am Coll Surg (2009) 208:134–47. doi: 10.1016/j.jamcollsurg.2008.09.007
23. Dodson RM, Weiss MJ, Cosgrove D, Herman JM, Kamel I, Anders R, et al. Intrahepatic cholangiocarcinoma: management options and emerging therapies. J Am Coll Surg (2013) 217:736–50.e4. doi: 10.1016/j.jamcollsurg.2013.05.021
24. Kang SH, Hwang S, Lee YJ, Kim KH, Ahn CS, Moon DB, et al. Prognostic comparison of the 7th and 8th editions of the American Joint Committee on Cancer staging system for intrahepatic cholangiocarcinoma. J Hepatobiliary Pancreat Sci (2018) 25:240–8. doi: 10.1002/jhbp.543
25. Kim Y, Moris DP, Zhang XF, Bagante F, Spolverato G, Schmidt C, et al. Evaluation of the 8th edition American Joint Commission on Cancer (AJCC) staging system for patients with intrahepatic cholangiocarcinoma: A surveillance, epidemiology, and end results (SEER) analysis. J Surg Oncol (2017) 116:643–50. doi: 10.1002/jso.24720
26. Meng ZW, Pan W, Hong HJ, Chen JZ, Chen YL. Macroscopic types of intrahepatic cholangiocarcinoma and the eighth edition of AJCC/UICC TNM staging system. Oncotarget (2017) 8:101165–74. doi: 10.18632/oncotarget.20932
27. Endo I, Gonen M, Yopp AC, Dalal KM, Zhou Q, Klimstra D, et al. Intrahepatic cholangiocarcinoma: rising frequency, improved survival, and determinants of outcome after resection. Ann Surg (2008) 248:84–96. doi: 10.1097/SLA.0b013e318176c4d3
28. Paik KY, Jung JC, Heo JS, Choi SH, Choi DW, Kim YI. What prognostic factors are important for resected intrahepatic cholangiocarcinoma? J Gastroenterol Hepatol (2008) 23:766–70. doi: 10.1111/j.1440-1746.2007.05040.x
29. Rahnemai-Azar AA, Weisbrod A, Dillhoff M, Schmidt C, Pawlik TM. Intrahepatic cholangiocarcinoma: Molecular markers for diagnosis and prognosis. Surg Oncol (2017) 26:125–37. doi: 10.1016/j.suronc.2016.12.009
30. Sasaki K, Margonis GA, Andreatos N, Chen Q, Barbon C, Bagante F, et al. Serum tumor markers enhance the predictive power of the AJCC and LCSGJ staging systems in resectable intrahepatic cholangiocarcinoma. HPB (Oxford) (2018) 20:956–65. doi: 10.1016/j.hpb.2018.04.005
31. Cho SY, Park SJ, Kim SH, Han SS, Kim YK, Lee KW, et al. Survival analysis of intrahepatic cholangiocarcinoma after resection. Ann Surg Oncol (2010) 17:1823–30. doi: 10.1245/s10434-010-0938-y
32. Jaklitsch M, Petrowsky H. The power to predict with biomarkers: carbohydrate antigen 19-9 (CA 19-9) and carcinoembryonic antigen (CEA) serum markers in intrahepatic cholangiocarcinoma. Transl Gastroenterol Hepatol (2019) 4:23. doi: 10.21037/tgh.2019.03.06
33. Kudo M, Kitano M, Sakurai T, Nishida N. General rules for the clinical and pathological study of primary liver cancer, nationwide follow-up survey and clinical practice guidelines: The outstanding achievements of the Liver Cancer Study Group of Japan. Dig Dis (2015) 33:765–70. doi: 10.1159/000439101
34. Primary liver cancer in Japan. Clinicopathologic features and results of surgical treatment. Liver Cancer Study Group of Japan. Ann Surg (1990) 211:277–87.
35. Zhou YM, Yang JM, Li B, Yin ZF, Xu F, Wang B, et al. Clinicopathologic characteristics of intrahepatic cholangiocarcinoma in patients with positive serum a-fetoprotein. World J Gastroenterol (2008) 14:2251–4. doi: 10.3748/wjg.14.2251
36. Kim JK, Yook IH, Choi MJ, Lee JS, Park YH, Lee JY, et al. A performance comparison on the machine learning classifiers in predictive pathology staging of prostate cancer. Stud Health Technol Inform (2017) 245:1273.
37. Zheng H, Ji J, Zhao L, Chen M, Shi A, Pan L, et al. Prediction and diagnosis of renal cell carcinoma using nuclear magnetic resonance-based serum metabolomics and self-organizing maps. Oncotarget (2016) 7:59189–98. doi: 10.18632/oncotarget.10830
38. Yu KH, Zhang C, Berry GJ, Altman RB, Ré C, Rubin DL, et al. Predicting non-small cell lung cancer prognosis by fully automated microscopic pathology image features. Nat Commun (2016) 7:12474. doi: 10.1038/ncomms12474
39. Ambale-Venkatesh B, Yang X, Wu CO, Liu K, Hundley WG, McClelland R, et al. Cardiovascular event prediction by machine learning: The multi-ethnic study of atherosclerosis. Circ Res (2017) 121:1092–101. doi: 10.1161/CIRCRESAHA.117.311312
Keywords: intrahepatic cholangiocarcinoma, prognosis, staging system, machine learning, overall survival
Citation: Li Z, Yuan L, Zhang C, Sun J, Wang Z, Wang Y, Hao X, Gao F and Jiang X (2021) A Novel Prognostic Scoring System of Intrahepatic Cholangiocarcinoma With Machine Learning Basing on Real-World Data. Front. Oncol. 10:576901. doi: 10.3389/fonc.2020.576901
Received: 27 June 2020; Accepted: 07 December 2020;
Published: 20 January 2021.
Edited by:
Jiankun Hu, Sichuan University, ChinaReviewed by:
Qing Wang, Tsinghua University, ChinaYingbin Liu, Shanghai Jiaotong University, China
Guohao Wu, Fudan University, China
Copyright © 2021 Li, Yuan, Zhang, Sun, Wang, Wang, Hao, Gao and Jiang. This is an open-access article distributed under the terms of the Creative Commons Attribution License (CC BY). The use, distribution or reproduction in other forums is permitted, provided the original author(s) and the copyright owner(s) are credited and that the original publication in this journal is cited, in accordance with accepted academic practice. No use, distribution or reproduction is permitted which does not comply with these terms.
*Correspondence: Fei Gao, Z2FvZmVpOTAwMEAxNjMuY29t; Xiaoqing Jiang, amlhbmd4aWFvcWluZ3Byb0BzaW5hLmNvbQ==
†These authors have contributed equally to this work and share first authorship