- 1Department of Pediatric Respiratory Medicine, Tianjin Children’s Hospital (Tianjin University Children’s Hospital), Tianjin, China
- 2Department of Science and Education, Tianjin Children’s Hospital (Tianjin University Children’s Hospital), Tianjin, China
- 3Department of Clinical Lab, Tianjin Children’s Hospital (Tianjin University Children’s Hospital), Tianjin, China
- 4Department of Pediatric Endocrinology, Tianjin Children’s Hospital (Tianjin University Children’s Hospital), Tianjin, China
- 5Tianjin Institute of Pediatrics (Tianjin Key Laboratory of Birth Defects for Prevention and Treatment), Tianjin Children’s Hospital (Tianjin University Children’s Hospital), Tianjin, China
Background: Acute myelogenous leukemia (AML) is a common pediatric malignancy in children younger than 15 years old. Although the overall survival (OS) has been improved in recent years, the mechanisms of AML remain largely unknown. Hence, the purpose of this study is to explore the differentially methylated genes and to investigate the underlying mechanism in AML initiation and progression based on the bioinformatic analysis.
Methods: Methylation array data and gene expression data were obtained from TARGET Data Matrix. The consensus clustering analysis was performed using ConsensusClusterPlus R package. The global DNA methylation was analyzed using methylationArrayAnalysis R package and differentially methylated genes (DMGs), and differentially expressed genes (DEGs) were identified using Limma R package. Besides, the biological function was analyzed using clusterProfiler R package. The correlation between DMGs and DEGs was determined using psych R package. Moreover, the correlation between DMGs and AML was assessed using varElect online tool. And the overall survival and progression-free survival were analyzed using survival R package.
Results: All AML samples in this study were divided into three clusters at k = 3. Based on consensus clustering, we identified 1,146 CpGs, including 40 hypermethylated and 1,106 hypomethylated CpGs in AML. Besides, a total 529 DEGs were identified, including 270 upregulated and 259 downregulated DEGs in AML. The function analysis showed that DEGs significantly enriched in AML related biological process. Moreover, the correlation between DMGs and DEGs indicated that seven DMGs directly interacted with AML. CD34, HOXA7, and CD96 showed the strongest correlation with AML. Further, we explored three CpG sites cg03583857, cg26511321, cg04039397 of CD34, HOXA7, and CD96 which acted as the clinical prognostic biomarkers.
Conclusion: Our study identified three novel methylated genes in AML and also explored the mechanism of methylated genes in AML. Our finding may provide novel potential prognostic markers for AML.
Introduction
Acute myelogenous leukemia (AML) is a common pediatric hematologic cancer in children younger than 15 years (1, 2). In the past several years, the survival rate for AML has increased because of the improvement of therapeutic strategies, risk stratification, and supportive care (3, 4). It has been reported that the long-term survival rate of AML is about 70% in children (5). Although the antitumor treatment for AML exhibits the optimistic outcome, the initiation and development of AML remain unclear.
Recent researches have demonstrated epigenetic modifications act a critical role in AML through altering the transcriptional activation of oncogenes or tumor suppressors (6–8). DNA methylation is a common epigenetic modification in biological process and pathological processes in multiple tumors, such as regulation of tumorigenesis and development in breast cancer and lung cancer (9, 10). Previous study has found that there is abnormal DNA methylation before cancer cell mutation (11). In an experiment on breast cancer cells, some scholars have found that inhibition of DNA methylation can accelerate the spread and metastasis of breast cancer cells (12). Some scholars have also found that the changes of DNA methylation and transcriptome are related to the sensitivity of chemotherapeutic drugs (13, 14). Furthermore, scholars have found that cisplatin resistance is related to the hypermethylation deletion of several CpG sites mainly located in the intergenomic region, which directly affects the efficacy of treatment, thus affecting the survival time of patients (15). Moreover, increasing evidence has demonstrated that DNA methylated genes might act as the biomarkers in AML (16, 17). Multi-omics of AML has indicated that high frequent methylation events occurs in children than adults (18). Besides, it has been revealed that hypermethylation of the ASNS promoter inhibits ASNS expression to increase sensitive to L-asparaginase and Decitabine (19). Furthermore, previous studies found that azacitidine and decitabine act as the inhibitors of DNA methylation and have been used in chemotherapy in patients with AML (20, 21). Inhibiting the expression of DNA methylation can effectively improve the effect of chemotherapy and prolong the survival time of AML patients (22). Previous studies have found that DNA methylation is a high sensitivity and specificity biomarker of AML in distinguishing it from other leukemia (9). Besides DNA methylation being strongly associated with prognosis of AML patients, it has been reported that different methylation types have different clinical outcomes for AML patients (23). And DNA methylated genes can be used as therapeutic targets in AML (24).
Although numerous studies have illustrated the regulatory effects of DNA methylation in AML, research for prognostic biomarkers based on DNA methylated genes is still lacking. Hence, we conduct an integrative analysis of methylation and transcription in AML based on bioinformatics and identify differentially methylated genes in AML. Furthermore, we analyzed the correlation between differentially methylated genes and AM and explored the DNA methylation-related prognostic biomarkers for AML patients.
Methods
Data Collecting and Processing
DNA methylation data and gene expression profilers were obtained from 219 paired AML samples and normal samples in TARGET Data Matrix. DNA methylation data was carried out with Illumina HumanMethylation27K array, and gene expression profiles were carried out with Affymetrix Gene ST. The methylation levels were assessed using beta (β)-value which ranged from 0 to 1. β-value represented the ratio of the methylated allele frequency and unmethylated allele frequency. And the expression microarray data was normalized using quantile normalization methods.
Unsupervised Consensus Clustering Analysis
The ConsensusClusterPlus R package was used for unsupervised consensus analysis, and the clustering was performed using pam method with sampling proportion 0.8. Importantly, the consensus clustering included the following parts. The consistent matrix (CM) plots were illustrated based on k-value. And empirical cumulative distribution function (CDF) plots revealed the consensus distributions for each k. The k at approximate maximum distribution indicated maximum stability cluster structure. The delta area plot showed the delta area score (y-axis); the delta area score determines the stability of clustering. Moreover, the item tracking plot exhibited the consensus cluster of items (columns) at each k (rows) to identify the stability of clustering. Cluster-consensus plot showed the cluster-consensus value at different k values; high cluster-consensus value indicated low stability of clustering. Item-consensus plot is the average consensus value between an item and members of a consensus cluster. An item corresponded to multiple item-consensus values with different ks.
Differential DNA Methylation Analysis
The principal component analysis (PCA) was used to analysis the sample bias in this study. And then, DNA methylation analysis was performed using methylationArrayAnalysis R package. The probes containing single nucleotide polymorphisms (SNPs), probes located in chromosome X, and more than 10% missing values in the probes were excluded in this study. The differentially DNA methylation probes (DMPs) were predicated with a cutoff value |Δβ| ≥0.2 and adjusted P-value <0.05. And DNA methylation regions (DMRs) were analyzed with a cutoff value no. CpGs ≥3, |meandiff| ≥0.2, and fisher (P) >0.05.
Differentially Expressed Gene Analysis
Limma R package was used to determine the DEGs between cluster 2 and cluster 3 in AML. The cutoff value was |log2 (fold change) | ≥ 1 and P-value < 0.05. The volcano plot of DEGs was drawn using ggplot R package, and heatmap of DEGs was drawn using pheatmap R package.
Gene Ontology Annotation and Kyoto Encyclopedia of Genes and Genomes Enrichment Analysis
The biological function was analyzed by GO and KEGG pathway enrichment analysis using clusterProfiler R package with a threshold P-value <0.05. GO annotation included biological process (BP), molecular function (MF), and cellular component (CC).
Correlation Between DMGs and DEGs
Correlation between DMGs and DEGs was calculated by Pearson correlation analysis using psych R package with a cutoff value correlation ≥0.5 and adjusted P-value <0.05. Moreover, the protein–protein interaction (PPI) network of hypomethylated-high expression genes and hypermethylated-low expression genes was constructed and visualized using Cytoscape.
Correlation Between DMGs and AML
The correlation between DMGs and AML was analyzed using varElect online tool, and high score indicated the strong correlation.
Survival Analysis
The overall survival (OS) and progression-free survival (PFS) were analyzed by Kaplan–Meier (KM) survival plots using survival R package based on β-value.
Results
Construction of Three Unsupervised Clusters in AML
The DNA methylation array data of 219 AML samples was obtained from TARGET Data Matrix and normalized using exclusion criteria. Then, the consensus clustering was performed using ConsensusClusterPlus R package; the CDF showed the lowest rangeability at consensus index 0.2–0.6 with k = 3 (Figure 1A). The delta area scores 2.5 was the highest at k = 3 (Figure 1B). Besides, the CM plot also showed the highest consistency at k = 3 (Figure 1C, Supplementary Figure 1A). Besides, the results of the tracking plot (Supplementary Figure 1B), cluster consensus plot (Supplementary Figure 1C), item consensus plot (Supplementary Figure 1D) also indicated the cluster stability at k = 3. Thus, consensus clustering of AML samples identified three clusters, and the clusters 2 and 3 showed the maximum sample number could be used for subsequent analysis. We further assess the correlation between three clusters and survival rates; we found the differential DNA methylation has strong correlation with OS and PFS of patients (Figures 1D, E). Our finding suggested that potential DNA methylated-related biomarkers were used to distinguish the different clusters of AML samples.
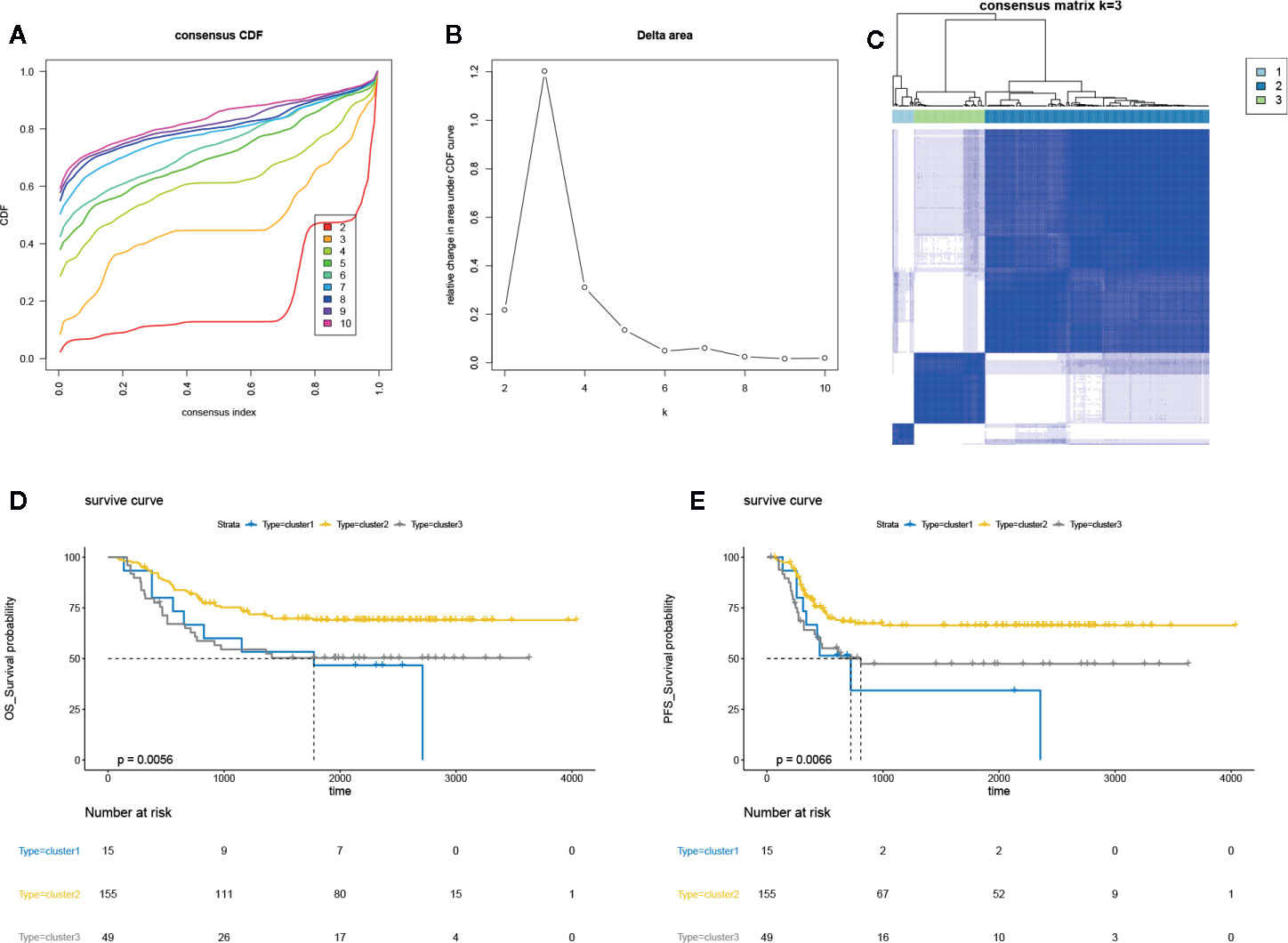
Figure 1 Unsupervised clustering analysis in AML. (A) The empirical cumulative distribution function (CDF) plots revealed the consensus distributions for each k. (B) The delta area score displayed the relative growth in cluster stability. (C) The circular manhattan (CM) plot exhibited the clusters at k = 3. (D) The overall survival (OS) plot for the AML patients of clusters 1, 2, 3 using survival R package. (B) progression-free survival (PFS) plot for the AML patients of clusters 1, 2, 3 using survival R package.
Global Methylation Analysis in AML
The DNA methylation in three clusters was analyzed. Above all, the PCA results showed that the data among three clusters exhibited the distinct group-bias clustering and individual difference (Figure 2A), and the methylation distribution density plot showed the β-value DMPs were concentrated distribution at β-value <0.2 and β-value >0.8 (Figure 2B). A total 1,146 CpG sites were identified between cluster 3 and cluster 2, which included 40 hypermethylated and 1,106 hypomethylated CpG sites in AML (Figure 2C). Then, we analyzed the distribution of differentially methylated CpG sites in chromosomes; the results showed the differentially methylated CpG sites mainly were distributed in chromosomes 1, 2, 3, 7, 8, 9, 11, 15, 18, 22 (Table 1). We further explored the distribution of differentially methylated CpGs in function regions, including promoters, enhancer, TSS1500, TSS200, 1stExon, 5′UTR, 3′UTR (Table 2). Moreover, we assessed the distribution of differentially methylated CpGs in whole genome; we observed that low level of hypermethylated and high levels of hypomethylated CpGs in N-shore, S-shore, island, OpenSea, and 1,108 methylated genes were screened based on the DMPs in whole genome (Table 2). Next, we examined the distribution of differentially methylated CpGs in chromosomes; 2,856 DMRs were screened, and seven significant DMRs were filtered with a cutoff value no.cpgs ≥3, |meandiff| ≥0.2, Fisher (P value) >0.05 (Table 3). Importantly, seven significant DMRs included six hypomethylated CpGs (meandiff ≤ −0.2) and one hypermethylated CpGs (meandiff ≥ 0.2); a total 45 genes were involved in these regions, such as WT1, CCND1, RUNX3 (Table 3). Further, to analyze the DMRs in WT1, CCND1, RUNX3, we found that CpGs sites of WT1 were observed in the gene body at chromosome 11; the regions included N-shore, S-shore, and Island (Figure 2C). CpGs sites of RUNX3 were identified in the gene body, TSS1500, 1stExon, 5′UTR; the regions included OpenSea (Figure 2D). And the CpGs of CCND1 were observed in gene body, TSS1500, and 3′UTR (Figures 2E, F). Our results indicated there were obvious differentially methylated CpGs in AML.
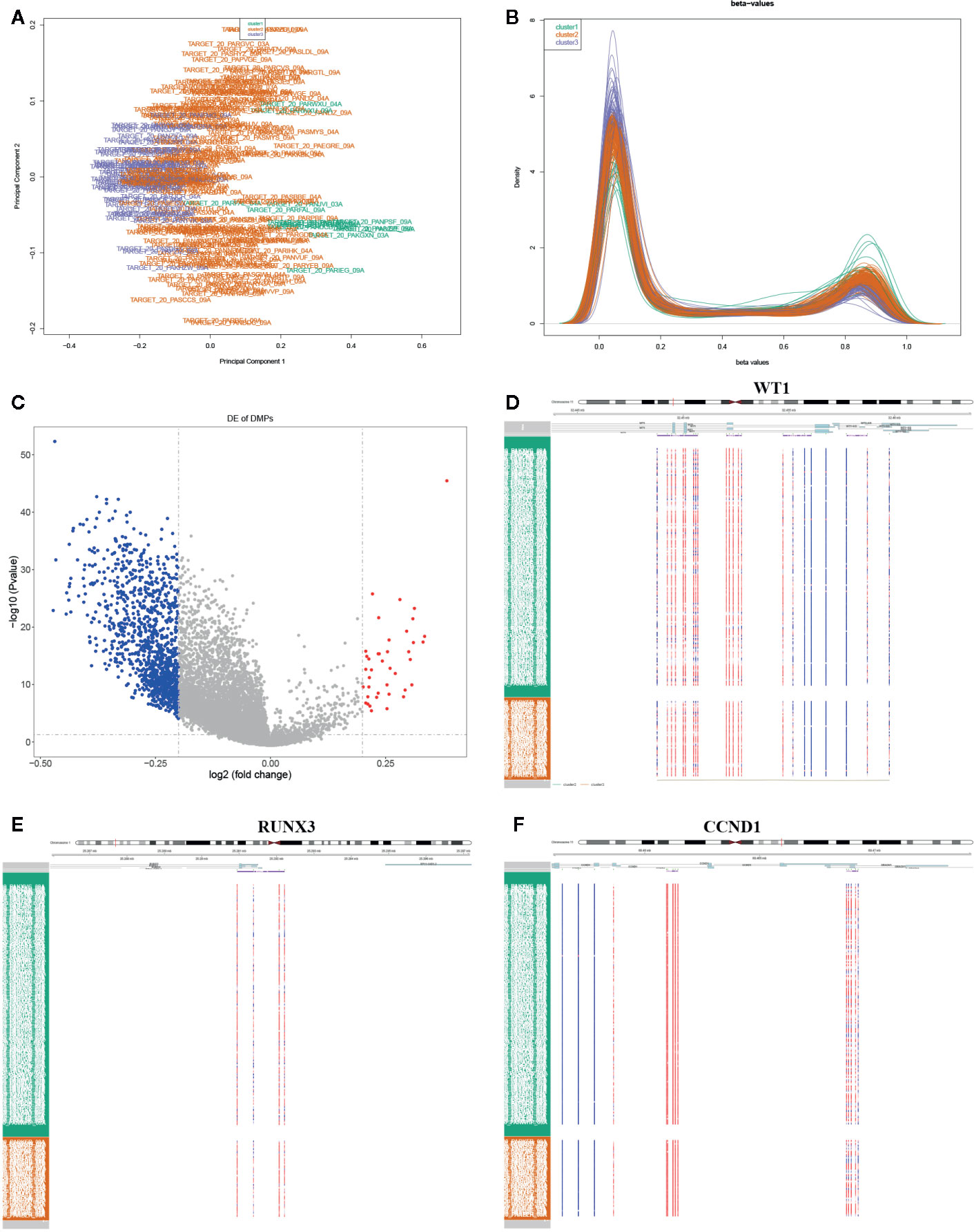
Figure 2 Global methylation analysis. (A) The principal component analysis (PCA) for the sample clustering. (B) The methylation density distribution plot showed the sample distribution trend and repeatability. (C) The volcano plot displayed the differential CpG sites between cluster 3 and cluster 2. (D–F) The methylated heatmap of the WT1, RUNX3, and CCND1.
Identification of the DEGs in AML
We further identified the DEGs of AML samples: the mRNA expression profiles were grouped into three clusters based on the consensus clustering. The multi-dimensional scaling (MDS) results showed the clustering exhibited unbiased in the group; it indicated gene expression of samples was consistent and repeatable (Figure 3A). A total 529 DEGs were screened between cluster 3 and cluster 2 using Limma R package, including 270 upregulated DEGs and 259 downregulated DEGs with a threshold | log2 (fold change) | ≥1 and P-value ≤0.05 (Figure 3B). Besides, the top 100 genes containing 50 upregulated and 50 downregulated DEGs were identified using pheatmap R package (Figure 3C). Our data suggested that DEGs significantly distinguished between cluster 3 and cluster 2.
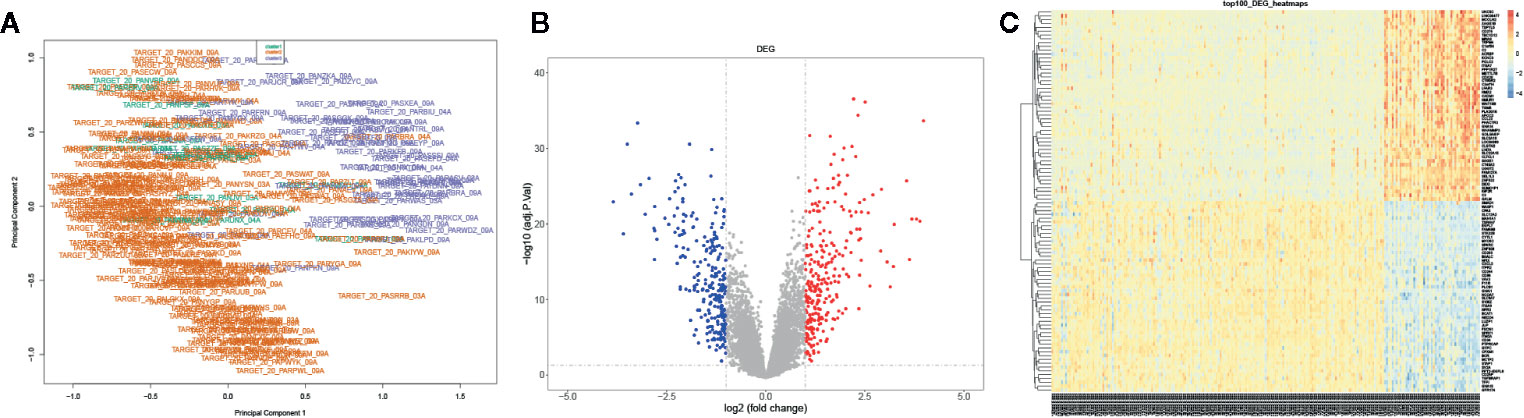
Figure 3 Identification of the DEGs in AML. (A) The PCA for the sample clustering. (B) The volcano plot displayed the DEGs between cluster 3 and cluster 2. (C) The heatmap exhibited the DEGs between cluster 3 and cluster 2.
Identification of the Biological Function of DEGs
We further investigated the biological function of DEGs by GO and KEGG pathway enrichment analysis using clusterProfiler R package. The biological processes of upregulated DEGs significantly enriched in AML related biological processes, such as leukocyte migration, positive regulation of myeloid leukocyte mediated immunity, regulation of myeloid leukocyte mediated immunity, regulation of leukocyte mediated immunity (Figure 4A), and cellular component enriched in integrin complex, vacuolar lumen. protein complex involved in cell adhesion, etc. (Figure 4B). Moreover, KEGG pathways were enriched in chemokine signaling pathway, cell adhesion molecules (CAMs), etc. (Figure 4C). Besides, the biological processes of downregulated DEGs were enriched in AML-associated biological processes, such as leukocyte migration, leukocyte proliferation, positive regulation of leukocyte proliferation, negative regulation of leukocyte activation (Figure 4D). Cellular component was enriched in the cytoplasmic side of plasma membrane and cytoplasmic side of membrane (Figure 4E). Molecular function was enriched in prostanoid receptor activity, SH3/SH2 adaptor activity, signaling adaptor activity, and icosanoid receptor activity (Figure 4F). And the KEGG pathways was enriched in transcriptional misregulation in cancer, acute myeloid leukemia, leukocyte transendothelial migration, etc. (Figure 4G). Taken together, the transcriptional misregulation in cancer and acute myeloid leukemia pathways might participate in AML progression; HOXA 10, HOXA9, HOXA11, RXRA, MEIS1, PBX3, ITGB7, ITGAM, FCGR1A, JUP, MPO, KIT, ZBTB16, RUNX1T1, CCNA1, SMAD1, TSPAN7, ERG, MYCN, WT1, CCND2, NTRK1, HPGD, PROM1 were involved in this pathway (Figures 4H, I).
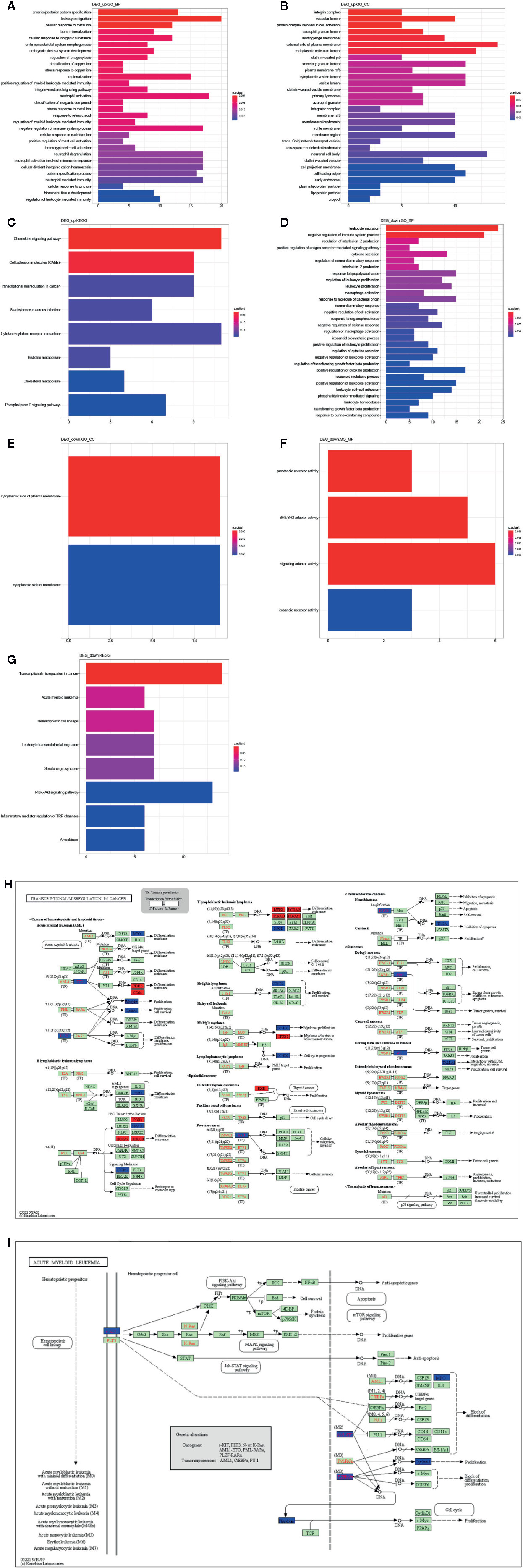
Figure 4 Function analysis of DEGs. GO annotation (A) and (D) BP, (B) and (E) CC, (F) MF, and (C) and (G) KEGG enrichment analysis the enriched pathways of upregulated/downregulated DEGs. (H) The transcriptional misregulation in cancer pathway (hsa052202) was enriched by KEGG analysis. (I) The acute myeloid leukemia pathways (hsa05221) were enriched by KEGG analysis.
Identification of the MDGs in AML
To determine the effects of DNA methylation on the gene expression, we examined the correlation between DMGs and DEGs by Pearson correlation analysis using psych R package. A total of 37,402 CpG-gene pairs were identified with a threshold |r| > 0.05 and adjusted P-value < 0.05, and there were 26,558 CpG-gene pairs which were related to downregulated genes (Table 4). The correlation between DMPs and DEGs was analyzed using STRING and visualized using Cytoscape (Figure 5). We found 18 DMGs containing 18 methylated regions in promoters, including three hypermethylated and 15 hypomethylated regions (Table 5). Moreover, correlation between 18 DMGs and AML was analyzed using varElect online tool; we found seven DMGs directly related to AML, and CD34, HOXA7, and CD96 exhibited the strongest correlation with AML (Table 6).
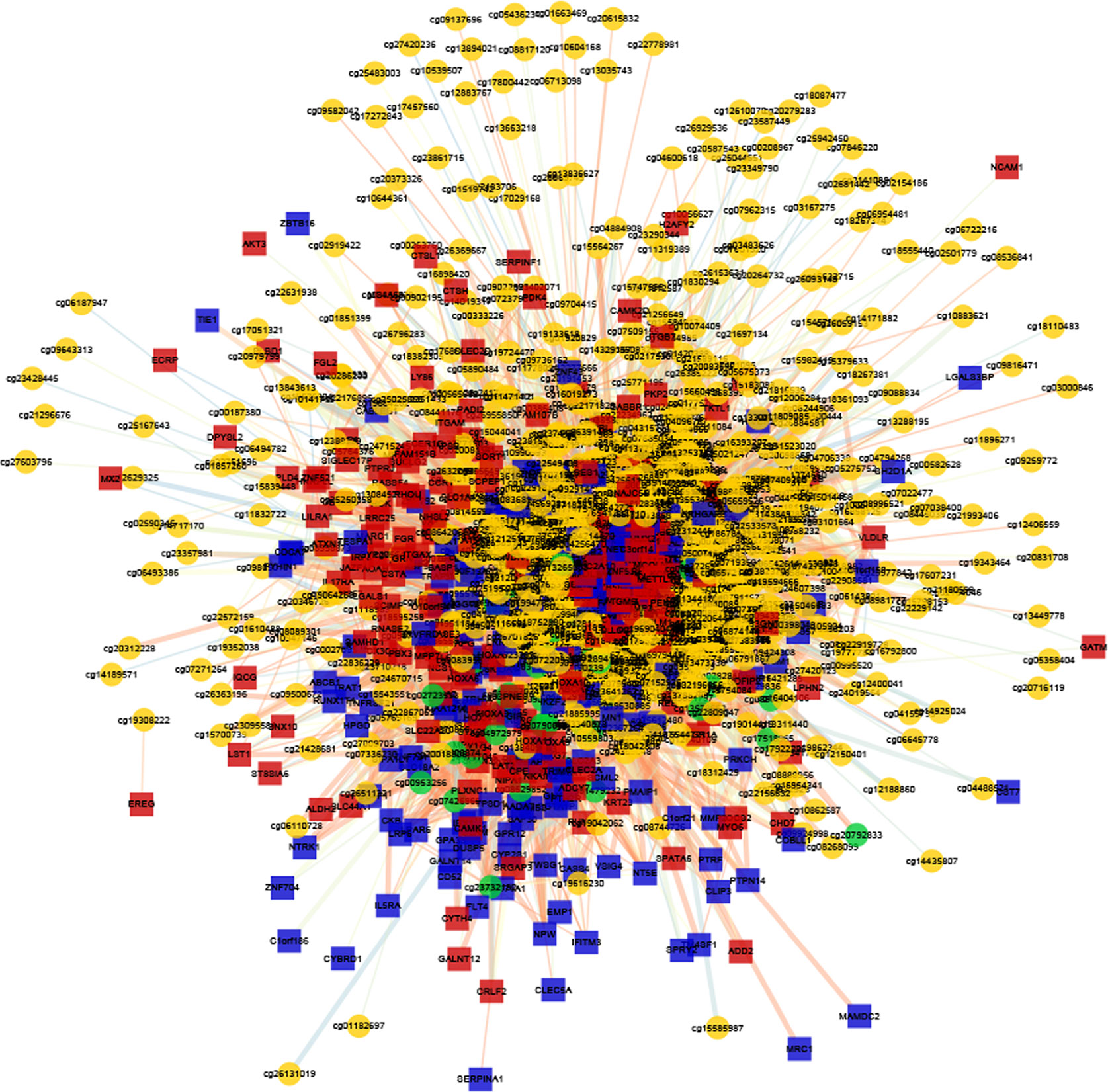
Figure 5 Correlation between DMG sites and DEG analysis. The co-expression network of DMGs and DEGs.
Construction of Three-MEGs Clinical Prognostic Biomarkers for AML
The Cox regression analysis was used to explore the role of methylation on AML. The clinical significance of three CpG sites (cg03583857, cg26511321, cg04039397) of candidate methylated genes was investigated by Cox regression analysis. The results showed AUC values of cg03583857 is 0.775 (Figure 6A), AUC value of cg04039397 is 0.901 (Figure 6B), and AUC value of cg26511321 is 0.815 (Figure 6C). The methylated regions were illustrated in Figure 6D. Therefore, three CpG sites cg03583857, cg26511321, cg04039397 of CD34, HOXA7, and CD96 might be use as the clinical prognostic biomarkers.
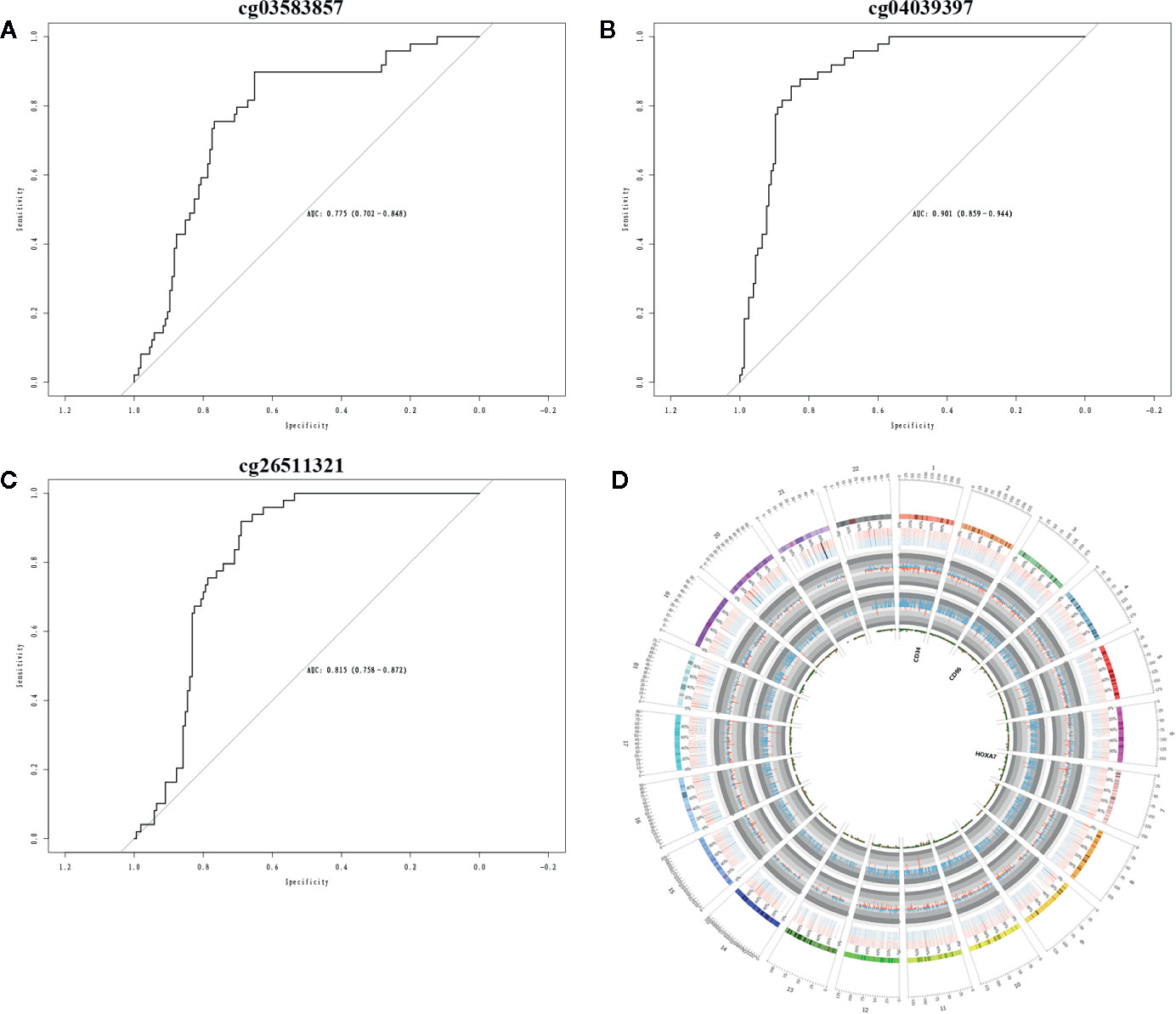
Figure 6 Clinical prognostic biomarker analysis. (A–C) The ROC plots of CD34, CD96, and HOXA7 for the consensus clusters. (D) The circular plot of CpGs.
Discussion
The overall survival of pediatric AML has increased in recent years with the development of chemotherapy, supportive care, and savage therapy (4); the overall survival increased from 49 ± 3% to 76 ± 4% between 1987 and 2012 (5). However, chemotherapy alone is the main treatment. Some recurrent and refractory AMLs are insensitive to chemotherapeutic drugs, which seriously restrict the improvement of survival rate in patients with AML. It has been reported that epigenetic aberrations are important reasons of leukemogenesis (17), such as the DNA methylated miR-150-5p which is mediated by DNA methyltransferase 1 that promotes the FGFR1-drived leukemogenesis (25). DNA methylation plays an important role in the regulation of the occurrence and development of AML (26). Some scholars have added DNA methylation inhibitors to refractory AML patients and found that the vast majority of AML patients can significantly prolong the survival time (24). Besides, the methylated GGH promoter associates with the variability of methotrexate responses in AML patients (27). Methotrexate as a first-line chemotherapy drug for clinical treatment of AML; DNA methylation can reduce the response of the human body to methotrexate and then directly affect the therapeutic effect. Inhibition of DNA methylation can increase the sensitivity of the human body to methotrexate (28). Clinical studies have found that the combined use of anti-DNA methylation drugs can significantly improve the effect of chemotherapy and prolong survival time (22).
In this study, we clustered AML samples into three consensus clusters at k = 3, and the DMGs were identified and validated in AML, including CD34, HOXA7, and CD96. CD34 plays as a role in response to iodine-131 radiotherapy in thyroid carcinoma (29). HOXA7 is a member of the HOX family which was involved in the development of multiple cancer types (30). CD96 has been reported as a novel immune checkpoint in glioma and associates with immune cell infiltration (31). High expression of CD34 and DNA methylation has been found in children with neuroepithelial tumors. Further studies have found that there are frequent genetic abnormalities involving B-Raf proto-oncogene (BRAF) or fibroblast growth factor receptors 2 and 3 (FGFR2, FGFR3), which can induce the occurrence and development of neuroepithelial tumors (32). In a methylation study on the early diagnosis of non-small cell lung cancer, it has found that the expression of HOXA7 methylation had high sensitivity and specificity in the diagnosis of non-small cell lung cancer (33). The low expression of HOXA7 methylation also exists in breast cancer cells, while the normal expression of HOXA7 methylation in normal tissues adjacent to breast cancer, ndicating that HOXA methylation expression plays an important role in the occurrence and development of breast cancer (33). CD96 is related to TIGIT, CD226, CRTAM, TIM-3, PD-L1, CTLA-4, and STAT3. These checkpoint proteins have additive antitumor effects, and CD96 plays an important role in immune response (34); azacytidine and decitabine have become inhibitors of DNA methylation combined with chemotherapeutic drugs, which can prolong the survival time of AML patients and improve the prognosis of AML patients (22).
Three key targets of DNA methylation on AML disease from multiple angles, which can provide genetic theoretical evidence for the early diagnosis of AML were further analyzed to provide clinical evidence for the inhibition of DNA methylation to improve the therapeutic effect and to provide ideas for the diagnosis and treatment of refractory and recurrent AML. It provides hope for AML patients to prolong their survival time and enter the clinical remission stage as soon as possible.
Here, we performed an integrated analysis of DNA methylation and gene expression; the abnormal expression genes involved in AML were identified. Moreover, our finding suggested that DNA methylated promoter of genes showed low mRNA expression, which is related to the overall survival of AML patients in cluster 3 which is significantly shorter than AML patients in cluster 2. Hypermethylation of CD34 and CD96 at cg03583857 and cg04039397 significantly inhibited mRNA expression, and hypomethylation of HOXA7 at cg26511321 obviously accelerated mRNA expression. CD34 is one of the members of the cluster of differentiation 3 (CD34) family, which is a type-I transmembrane sialomucins and acts as the marker of hematopoietic stem cells and vascular (35). In gastrointestinal stromal tumor (GIST) patients, a strong inverse correlation between DNA methylation degree and CD34 expression has been evaluated by immunohistochemical staining. The expression of CD34 has been modulated by DNA methylation in a site-dependent manner in GISTs.
Abnormal expression of HOX gene is a common feature in AML (36). However, the molecular mechanism of HOX gene in AML remains unclear. Previous study demonstrated that CCCTC binding factor (CTCF) mediated aberrant expression of HOX in AML. Di Vinci, A. et al. have shown that HOXA7 is a methylation target associated with aggressive behavior in meningiomas. The above studies have confirmed that the methylation of HOXA7 is closely related to the occurrence and development of tumors.
CD96 is an immune related gene which acts as an immune related marker in AML (34), high expression of CD96 associated with poor prognosis in de novo AML (37). It has been indicated that CD96 is regulated by differential DNA methylation to change the mRNA and protein expression. The above studies proved that CD96 methylation interferes its mRNA expression, which indirectly affects the occurrence and metastasis of cancer.
In summary, we performed an integrative analysis of multi-omics in AML and identified the differential DNA methylated genes such as CD34, HOXA7, and CD96 and explored DNA methylation and clinical implication of CD34, HOXA7, and CD96 in AML.
Data Availability Statement
Publicly available datasets were analyzed in this study. This data can be found here: https://ocg.cancer.gov/programs/target/data-matrix.
Author Contributions
TZ and JZ contributed to the conception of the study. YX and CC contributed significantly to analysis and manuscript preparation. JZ performed the data analyses and wrote the manuscript. WG, CZ, XC, LG, CC, and YX helped perform the analysis with constructive discussions. All authors contributed to the article and approved the submitted version.
Funding
This work was supported by National Natural Science Foundation of China [Grant number 81771589], the Key Project of Tianjin Health Care Professionals [Grant number 16KG166] and the Program of Tianjin Science and Technology Plan [Grant number 18ZXDBSY00170].
Conflict of Interest
The authors declare that the research was conducted in the absence of any commercial or financial relationships that could be construed as a potential conflict of interest.
Supplementary Material
The Supplementary Material for this article can be found online at: https://www.frontiersin.org/articles/10.3389/fonc.2020.591937/full#supplementary-material
Supplementary Figure 1 | Unsupervised clustering analysis in AML. (A) The CM plot exhibited the clusters at k = 1, 2, 4, 5, 6. (B) The Tracking plot indicated the consensus cluster of items at each k. (C) The cluster-consensus plot showed the consensus value of each k. (D) The item-consensus plot exhibited the multiple item-consensus values for an item at the k clusters.
References
1. Schapira MM, Stevens EM, Sharpe JE, Hochman L, Reiter JG, Calhoun SR, et al. Outcomes among pediatric patients with cancer who are treated on trial versus off trial: A matched cohort study. Cancer (2020) 126:3471–82. doi: 10.1002/cncr.32947
2. Cammarata-Scalisi F, Girardi K, Strocchio L, Merli P, Bernardin AG, Galeotti A, et al. Oral Manifestations and Complications in Childhood Acute Myeloid Leukemia. Cancers (2020) 12:1634. doi: 10.3390/cancers12061634
3. Pelland-Marcotte MC, Pole JD, Hwee J, Sutradhar R, Science M, Nathan P C, et al. Long-Term Risk of Infections After Treatment of Childhood Leukemia: A Population-Based Cohort Study Using Administrative Health Data. J Clin Oncol (2019) 37:2651–60. doi: 10.1200/JCO.19.00570
4. Pession A, Masetti R, Rizzari C, Putti MC, Casale F, Fagioli F, et al. Results of the AIEOP AML 2002/01 multicenter prospective trial for the treatment of children with acute myeloid leukemia. Blood (2013) 122:170–8. doi: 10.1182/blood-2013-03-491621
5. Rasche M, Zimmermann M, Borschel L, Bourquin JP, Dworzak M, Klingebiel T, et al. Successes and challenges in the treatment of pediatric acute myeloid leukemia: a retrospective analysis of the AML-BFM trials from 1987 to 2012. Leukemia (2018) 32:2167–77. doi: 10.1038/s41375-018-0071-7
6. Jost E, do ON, Wilop S, Herman JG, Osieka R, Galm O. Aberrant DNA methylation of the transcription factor C/EBPalpha in acute myelogenous leukemia. Leuk Res (2009) 33:443–9. doi: 10.1016/j.leukres.2008.07.027
7. Galm O, Wilop S, Lüders C, Jost E, Gehbauer G, Herman J G, et al. Clinical implications of aberrant DNA methylation patterns in acute myelogenous leukemia. Ann Hematol (2005) 84:39–46. doi: 10.1007/s00277-005-0005-0
8. Hoang NM, Rui L. DNA methyltransferases in hematological malignancies. J Genet Genomics (2020) 20:1673–8527. doi: 10.1016/j.jgg.2020.04.006
9. Jiang H, Ou Z, He Y, Yu M, Wu S, Li G, et al. DNA methylation markers in the diagnosis and prognosis of common leukemias. Signal Transduct Target Ther (2020) 5:3. doi: 10.1038/s41392-019-0090-5
10. Hurkmans DP, Tamminga M, van Es B, Peters T, Karman W, van Wijck RTA, et al. Molecular data show conserved DNA locations distinguishing lung cancer subtypes and regulation of immune genes. Lung Cancer (Amsterdam Netherlands) (2020) 146:341–9. doi: 10.1016/j.lungcan.2020.06.008
11. Klutstein M, Nejman D, Greenfield R, Cedar H. DNA Methylation in Cancer and Aging. Cancer Res (2016) 12:34467–3450. doi: 10.1158/0008-5472.CAN-15-3278
12. Gkountela S, Castro-Giner F, Szczerba BM, Vetter M, Landin J, Scherrer R, et al. Circulating Tumor Cell Clustering Shapes DNA Methylation to Enable Metastasis Seeding. Cell (2019) 176:98–112. doi: 10.1016/j.cell.2018.11.046
13. Xu H, Wu S, Shen X, Shi Z, Wu D, Yuan Y, et al. Methylation-mediated miR-214 regulates proliferation and drug sensitivity of renal cell carcinoma cells through targeting LIVIN. J Cell Mol Med (2020) 24:6410–25. doi: 10.1111/jcmm.15287
14. Kazim N, Adhikari A, Oh T J, Davie J. The transcription elongation factor TCEA3 induces apoptosis in rhabdomyosarcoma. Cell Death Dis (2020) 11:67. doi: 10.1038/s41419-020-2258-x
15. Lund RJ, Huhtinen K, Salmi J, Rantala J, Nguyen EV, Moulder R, et al. DNA methylation and Transcriptome Changes Associated with Cisplatin Resistance in Ovarian Cancer. Sci Rep (2017) 7:1469. doi: 10.1038/s41598-017-01624-4
16. Rothenburger T, McLaughlin K-M, Herold T, Schneider C, Oellerich T, Rothweiler F, et al. SAMHD1 is a key regulator of the lineage-specific response of acute lymphoblastic leukaemias to nelarabine. Commun Biol (2020) 3:324. doi: 10.1038/s42003-020-1052-8
17. Friedemann M, Gutewort K, Thiem D, Nacke B, Jandeck C, Lange BS, et al. Methylation of the Phospholipase A2 Receptor 1 Promoter Region in Childhood B Cell Acute Lymphoblastic Leukaemia. Sci Rep (2020) 10:9058. doi: 10.1038/s41598-020-65825-0
18. Bolouri H, Farrar JE, Triche T Jr., Ries RE, Lim EL, Alonzo TA, et al. Erratum: The molecular landscape of pediatric acute myeloid leukemia reveals recurrent structural alterations and age-specific mutational interactions. Nat Med (2018) 24:526. doi: 10.1038/nm0418-526b
19. Serravalle S, Bertuccio SN, Astolfi A, Melchionda F, Pession A. Synergistic Cytotoxic Effect of L-Asparaginase Combined with Decitabine as a Demethylating Agent in Pediatric T-ALL, with Specific Epigenetic Signature. BioMed Res Int (2016) 2016:1985750. doi: 10.1155/2016/1985750
20. Martín I, Navarro B, Serrano A, Villamón E, Calabuig M, Solano C, et al. Impact of clinical features, cytogenetics, genetic mutations, and methylation dynamics of CDKN2B and DLC-1 promoters on treatment response to azacitidine. Ann Hematol (2020) 99:527–37. doi: 10.1007/s00277-020-03932-8
21. You L, Han Q, Zhu L, Zhu Y, Bao C, Yang C, et al. Decitabine-Mediated Epigenetic Reprograming Enhances Anti-leukemia Efficacy of CD123-Targeted Chimeric Antigen Receptor T-Cells. Front Immunol (2020) 11:1787. doi: 10.3389/fimmu.2020.01787
22. Bewersdorf JA-O, Shallis R, Stahl M, Zeidan AM. Epigenetic therapy combinations in acute myeloid leukemia: what are the options? Ther Adv Hematol (2019) 11:10. doi: 10.1177/2040620718816698
23. Gao H, He X, Li Q, Wang Y, Tian Y, Chen X, et al. Genome-wide DNA methylome analysis reveals methylation subtypes with different clinical outcomes for acute myeloid leukemia patients. Cancer Med (2020) 9:6296–305 doi: 10.1002/cam4.3291
24. Carraway HE, Malkaram SA, Cen Y, Shatnawi A, Fan J, Ali HEA, et al. Activation of SIRT6 by DNA hypomethylating agents and clinical consequences on combination therapy in leukemia. Sci Rep (2020) 10:10325. doi: 10.1038/s41598-020-67170-8
25. Hu T, Chong Y, Cai B, Liu Y, Lu S, Cowell JK. DNA methyltransferase 1-mediated CpG methylation of the miR-150-5p promoter contributes to fibroblast growth factor receptor 1-driven leukemogenesis. J Biol Chem (2019) 294:18122–30. doi: 10.1074/jbc.RA119.010144
26. Benetatos L, Vartholomatos G. Enhancer DNA methylation in acute myeloid leukemia and myelodysplastic syndromes. Cell Mol Life Sci (2018) 75:1999–2009. doi: 10.1007/s00018-018-2783-2
27. Wang S-M, Kong X-Y, Li M, Sun L-L, Yan D. Association of GGH Promoter Methylation Levels with Methotrexate Concentrations in Chinese Children with Acute Lymphoblastic Leukemia. Pharmacotherapy (2020) 40(7):614–22. doi: 10.1002/phar.2430
28. Li Y, Liu S, Wang H, Mai H, Yuan X, Li C, et al. Methylation level of CpG islands in GGH gene promoter in pediatric acute leukemia. PLoS One (2017) 12:e0173472. doi: 10.1371/journal.pone.0173472
29. Shuwen H, Xi Y, Miao D, Jiamin X, Jing Z, Weili G. Nine Genes Mediate the Therapeutic Effects of Iodine-131 Radiotherapy in Thyroid Carcinoma Patients. Dis Markers (2020) 2020:9369341. doi: 10.1155/2020/9369341
30. Cui Y, Yan M, Zhang C, Xue J, Zhang Q, Ma S, et al. Comprehensive analysis of the HOXA gene family identifies HOXA13 as a novel oncogenic gene in kidney renal clear cell carcinoma. J Cancer Res Clin Oncol (2020) 146:1993–2006. doi: 10.1007/s00432-020-03259-x
31. Liu F, Huang J, He F, Ma X, Fan F, Meng M, et al. CD96, a new immune checkpoint, correlates with immune profile and clinical outcome of glioma. Sci Rep (2020) 10:10768. doi: 10.1038/s41598-020-66806-z
32. Huse JA-O, Snuderl M, Jones DT, Brathwaite CD, Altman N, Lavi E, et al. Polymorphous low-grade neuroepithelial tumor of the young (PLNTY): an epileptogenic neoplasm with oligodendroglioma-like components, aberrant CD34 expression, and genetic alterations involving the MAP kinase pathway. Acta Neuropathol (2017) 133(3):417–29. doi: 10.1007/s00401-016-1639-9
33. Chen CA-O, Huang X, Yin W, Peng M, Wu F, Wu X, et al. Ultrasensitive DNA hypermethylation detection using plasma for early detection of NSCLC: a study in Chinese patients with very small nodules. Clin Epigenetics (2020) 12:39. doi: 10.1186/s13148-020-00828-2
34. Coustan-Smith E, Song G, Shurtleff S, Yeoh AE, Chng WJ, Chen SP, et al. Universal monitoring of minimal residual disease in acute myeloid leukemia. (2018) 3:98561. JCI Insight doi: 10.1172/jci.insight.98561
35. Lin YY, Wang CY, Phan NN, Chiao CC, Li CY, Sun Z, et al. PODXL2 maintains cellular stemness and promotes breast cancer development through the Rac1/Akt pathway. Int J Med Sci (2020) 17:1639–51. doi: 10.7150/ijms.46125
36. Wang H, Lin SY, Hu FF, Guo AA-O, Hu H. The expression and regulation of HOX genes and membrane proteins among different cytogenetic groups of acute myeloid leukemia. Mol Genet Genomic Med (2020) 8:1665. doi: 10.1002/mgg3.1365
Keywords: multi-omics, prognostic, acute myelogenous leukemia, children, methylation
Citation: Zheng J, Zhang T, Guo W, Zhou C, Cui X, Gao L, Cai C and Xu Y (2020) Integrative Analysis of Multi-Omics Identified the Prognostic Biomarkers in Acute Myelogenous Leukemia. Front. Oncol. 10:591937. doi: 10.3389/fonc.2020.591937
Received: 05 August 2020; Accepted: 28 October 2020;
Published: 10 December 2020.
Edited by:
Simone Cesaro, Integrated University Hospital Verona, ItalyCopyright © 2020 Zheng, Zhang, Guo, Zhou, Cui, Gao, Cai and Xu. This is an open-access article distributed under the terms of the Creative Commons Attribution License (CC BY). The use, distribution or reproduction in other forums is permitted, provided the original author(s) and the copyright owner(s) are credited and that the original publication in this journal is cited, in accordance with accepted academic practice. No use, distribution or reproduction is permitted which does not comply with these terms.
*Correspondence: Chunquan Cai, Y3FjbnM2QDEyNi5jb20=; Yongsheng Xu, eHh5eXNzQDEyNi5jb20=