- Department of Thyroid and Breast Surgery, The First People’s Hospital of Shangqiu, Shangqiu, China
Background: Triple-negative breast cancer (TNBC) is one of the most aggressive subtypes of breast cancer with poorest clinical outcomes. Patients of childbearing age have a higher probability of TNBC diagnosis, with more demands on maintenance and restoration of physical and psychosocial function. This study aimed to design effective and comprehensive nomograms to predict survival in these patients.
Methods: We used the SEER database to identify patients with TNBC aged between 18 and 45 and randomly classified these patients into a training (n=2,296) and a validation (n=2,297) cohort. Nomograms for estimating overall survival (OS) and breast cancer-specific survival (BCSS) were generated based on multivariate Cox proportional hazards models and competing-risk models in the training cohort. The performances of the nomograms were quantified in the validation cohort using calibration curves, time-dependent receiver operating characteristic (ROC) curves and Harrell’s concordance index (C-index).
Results: A total of 4,593 TNBC patients of childbearing age were enrolled. Four prognostic factors for OS and six for BCSS were identified and incorporated to construct nomograms. In the validation cohort, calibration curves showed excellent agreement between nomogram-predicted and actual survival data. The nomograms also achieved relatively high Harrell’s C-indexes and areas under the time-dependent ROC curves for estimating OS and BCSS in both training and validation cohorts.
Conclusions: Independent prognostic factors were identified, and used to develop nomograms to predict OS and BCSS in childbearing-age patients with TNBC. These models could enable individualized risk estimation and risk-adapted treatment for these patients.
Introduction
Breast cancer is the most prevalent malignancy among females, which ranks first in new cases and second in deaths according to estimation from the American Cancer Society in 2019 (1). And as of 2019, there were more than 3.8 million women with a history of invasive breast cancer in the United States (2). Triple-negative breast cancer (TNBC) is a subset of breast cancer that lacks expression of estrogen receptor (ER), progesterone receptor (PR), and human epidermal growth factor receptor 2 (HER2). TNBC represents one of the most aggressive subtypes of breast cancer and remains the most challenging subtype to treat (3). The proportion of patients of childbearing age was higher in TNBC than in other breast cancer subtypes (4), and childbearing females were also more likely to be diagnosed with TNBC than with the other subtypes (5). In addition, childbearing-age patients have relatively different demographics and clinicopathologic characteristics and treatment strategies compared with patients in other physiological stages (6–8). Childbearing age refers to nearly 30 years after a woman reaches the age of 18. In this period, the reproductive and endocrine functions of the ovaries attained full growth or maturity, and the mammary glands undergo periodic changes under the regulation of ovarian hormones. The ovarian dysfunction, delayed childbearing, inability to breastfeed, and job changes, which may result from cancer treatment, have a tremendous impact on the physical, psychosocial well-being of these patients, resulting a reduction of disability adjusted life years (DALYs) (9–12). Therefore, it is of great significance to differentiate these patients with different risk of death, especially breast cancer specific death, and implement different treatment strategies.
With the increased use of neoadjuvant chemotherapy in TNBC patients, pathologic response has been recognized as an important prognostic factor (13). However, neoadjuvant has adverse therapeutic effects and takes time, which have an impact on the childbearing patients’ willingness to treatment (14). Even worse, some non-pathologic responding patients do not benefit from it and may delay prompt treatment. So, if the level of risk can be identified based on other characteristics, it will facilitate the identification and implementation of a tailored treatment. Our previous study described the molecular characteristics of TNBC patients of childbearing age, which provide a rationale for clinical management (15). However, in clinical practice, clinicopathologic characteristics are more accessible for clinicians than molecular profiling. Thus, the urgent clinical need for risk estimation prompted us to construct a clinicopathological information-based model for predicting survival in childbearing-age TNBC patients.
Nomograms are reliable and effective tools to quantify individual risk by incorporating and illustrating multiple important prognostic factors. They performed well in predicting survival in a variety of cancer types (16). In addition to nomogram for all four subtypes of breast cancer (17), researchers established specific nomograms for different histological subtypes (18–23), clinical subtypes (24–26), metastatic status (27–30), and age group (31–35) of breast cancer. However, to the best of our knowledge, nomograms for predicting the survival of childbearing-age patients with TNBC have not been reported. In this study, we aimed to formulate comprehensive nomograms based on complete clinical data selected from the Surveillance, Epidemiology, and End Results (SEER) database to estimate survival in TNBC patients of childbearing age.
Materials and Methods
Data Source and Patient Screening
Data were extracted from the SEER*Stat version 8.3.6.1, SEER 18 Cancer Registry [1976-2016] (with additional treatment fields) of the National Cancer Institute. The following criteria were used to identify eligible patients: female gender; age of 18–45 years at diagnosis; diagnosed between 2010 and 2015; pathologically confirmed invasive ductal carcinoma of the breast (ICD-O-3 8500/3); diagnosis confirmed by positive histology and not by autopsy or a death certificate; breast cancer as the first and only primary tumor; unilateral breast cancer; adjusted AJCC stage I-III; histological grade I-III; known tumor size; known regional lymph nodes status; and ER, PR, and HER2 negativity. Since the HER2 status was not recorded in the database until 2010, patients diagnosed before 2010 were not included. Patients diagnosed after 2015 were also excluded to ensure adequate follow-up time. Exclusion criteria were as follows: no record of regional lymph node status or tumor size; Paget’s disease and inflammatory breast cancer; incomplete survival data and unspecified tumor laterality or location information; survival month less than 1. Eventually, 4,593 patients were included after the screening. The following data were collected and transformed into categorical variables: age, race, marital status, laterality, grade, location, tumor size, positive lymph nodes, breast surgery, radiation, and chemotherapy.
Identification of Prognostic Factors in the Training Cohort
Patients were randomly classified into a training and a validation cohort at a 1:1 ratio (36). The primary endpoint were overall survival (OS) and breast cancer-specific survival (BCSS). OS was defined as the interval from breast cancer diagnosis to the last follow-up or death from any cause. BCSS was define as the interval from the time of diagnosis to last follow-up or death from breast cancer. Independent prognostic factors for OS were identified by multivariate Cox proportional hazards models, and the results were reported using hazard ratios (HRs) and 95% confidence intervals (CIs). The cumulative incidence rates of breast cancer specific death (CIBCSD) were calculated based on competing-risk models, and differences among groups were assessed by the Gray’s test (37, 38). In the competing-risk regression model, deaths from non-breast cancer specific causes were considered as competing risks.
Model Construction in the Training Cohort
Two nomograms were constructed to predict survival in the training cohort (39). Independent prognostic factors in multivariate Cox proportional hazards models were used to construct the nomogram for 3- and 5-year OS. Factors associated with CIBCSD in the competing-risk models were used to build the BCSS nomogram. The BCSS nomogram was also constructed based on the Cox regression model, in which patients succumbing to non-breast cancer specific causes were considered to be censored.
Model Validation in Both Cohorts
The nomograms were validated in both training and validation cohorts. First, the predictive accuracies of the nomograms were validated by bootstrapping with 1000 repetitions, and the discriminative ability was quantified by the concordance index (C-index). The C-index ranges from 0.5 (occurring by random chance) to 1.0 (perfectly correct discrimination). Second, calibration curves were generated to obtain nomogram-predicted survival, which is then compared with the corresponding Kaplan-Meier estimates. Third, according to the nomogram, we calculated the total points for all patients (40). The predictive precision of the risk score as a continuous variable was evaluated by time-dependent receiver operating characteristic (ROC) curves, and areas under the curves (AUCs) were used as the criterion (41). The ROC curves plotted the predictive sensitivity and specificity; a larger AUC (range 0.5~1.0) reflected a more accurate prediction. Finally, to demonstrate the clinical values of the nomograms that included all meaningful variables, two normal TNBC patients were assessed as examples.
Statistical Analysis
All statistical analyses were performed with STATA (version 14.1) and R (version 3.6.1). The R packages including caret, rms, cmprsk, survivalROC, and nomogramFormula were used. Statistical significance was defined as P < 0.05.
Results
Patient Characteristics
A total of 4,593 patients from the SEER program were enrolled in our study. The demographics and clinicopathologic characteristics of these patients are listed in Table 1. Among these patients, median follow-up months were 37 months (25%–75%, 22–58 months). Nearly half of them were aged between 40 and 45 (45.8%), while those between 35 and 40 (29.0%), and less than 35 (25.2%) composed the remaining half. Most of the patients were white (69.4%) and more than half of the patients were unmarried (58.8%). All assessed factors showed similar distribution between the training and validation cohorts.
Factors Associated With Overall Survival in the Training Cohort
In univariate Cox analysis, race, marital status, tumor location, tumor size, number of positive lymph nodes and breast surgery type were significantly correlated with OS (all P < 0.001 except for race and breast surgery, with P = 0.009 and P = 0.003, respectively). These prognostic factors were included in multivariate Cox analysis. The results confirmed that unmarried status, overlapped tumor location, large tumor, and more positive lymph nodes were independent adverse prognostic factors (Table 2). These variables were included in a weighted scoring system to estimate 3- and 5-year OS.
Factors Associated With Breast Cancer-Specific Survival in the Training Cohort
To identify prognostic factors associated with BCSS, we determined CIBCSD and cumulative incidence of non-breast cancer specific death (CINBCSD) based on the developed competing-risk models. At 3 and 5 years after diagnosis, CIBCSD rates in the training cohort were 0.144 and 0.195, respectively, while CINBCSD rates were 0.011 and 0.020, respectively. Estimates of CIBCSD and other causes according to the clinicopathological variables are shown in Table 3. Black patients had the highest CIBCSD (0.192 and 0.246 for 3 and 5 years, respectively), while white and patients of other race had lower CIBCSD (white, 0.133 and 0.187 for 3 and 5 years, respectively; other race, 0.122 and 0.142 for 3 and 5 years, respectively; P = 0.006). Other factors significantly associated with CIBCSD are marital status, tumor size, tumor location, lymph node status, and surgery type (all P < 0.001). All these factors were used to construct a nomogram to predict 3- and 5-year BCSS.
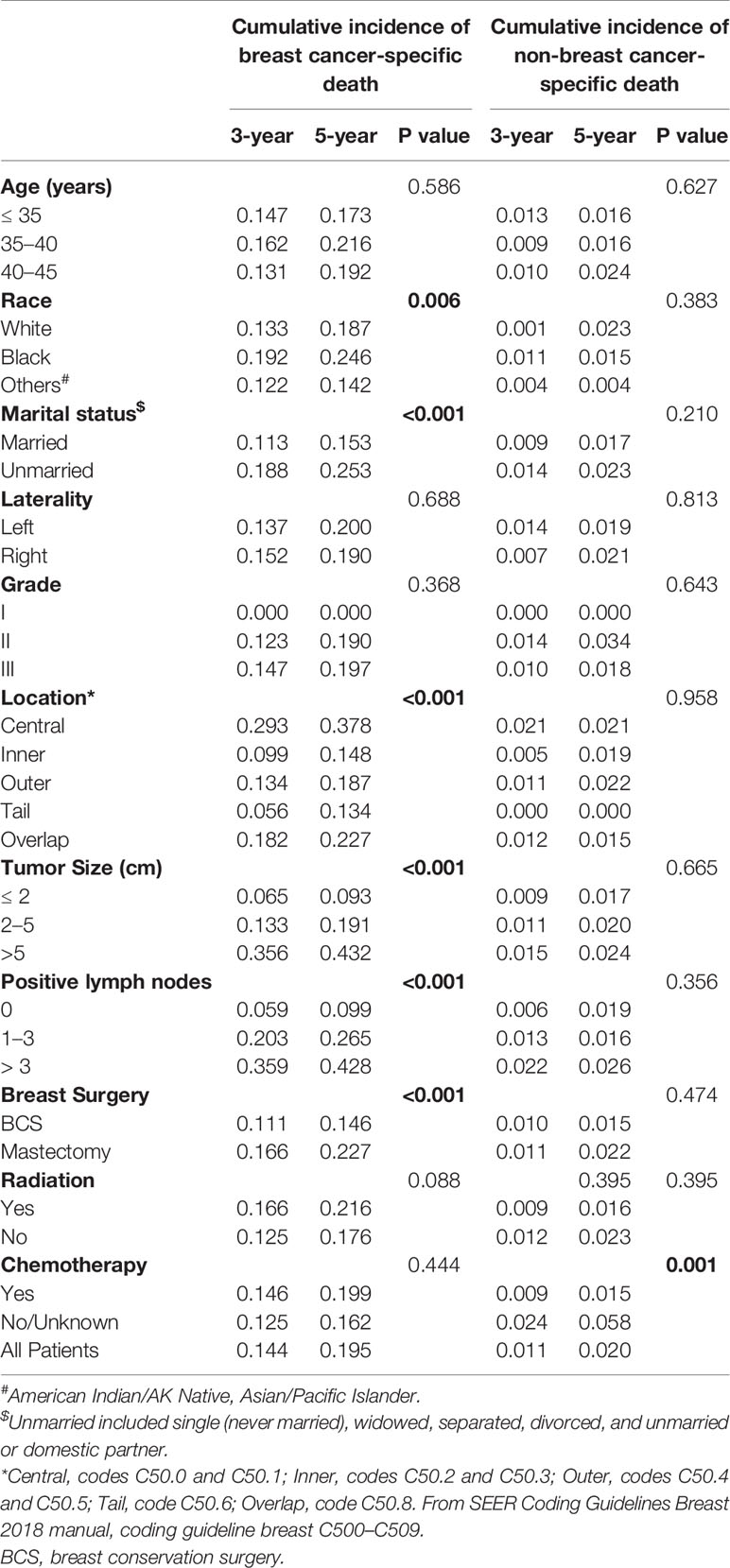
Table 3 Three- and 5-year cumulative incidence rates of death among patients in the training cohort.
Nomograms
Based on the prognostic factors identified in the training cohort, nomograms were formulated to predict 3- and 5-year OS and BCSS (Figure 1). To clarify the applications of these nomograms, two representative TNBC patients were assessed. Both patients were married, underwent surgery, and diagnosed with grade III, invasive ductal carcinoma with outer location. The first patient was a 36-year-old white patient diagnosed with a tumor of 1.5 cm in diameter and without positive lymph node, while the second was a 37-year-old patient of other race (American Indian/AK Native, Asian/Pacific Islander) diagnosed with a tumor of 5.5 cm in diameter and with 5 positive lymph nodes. According to the nomograms, the first patient scored 59.1 and 47.9 in the OS and BCSS nomograms, respectively, which indicated that her odds of 3- and 5-year OS were greater than 0.85, and those of 3- and 5-year BCSS were greater than 0.95. Scores of the second patient in OS and BCSS nomograms were 214.3 and 205.1, respectively, which indicated that the odds of both 3- and 5-year OS and BCSS were less than 0.7. These results can help to guide individualized treatment for these two patients.
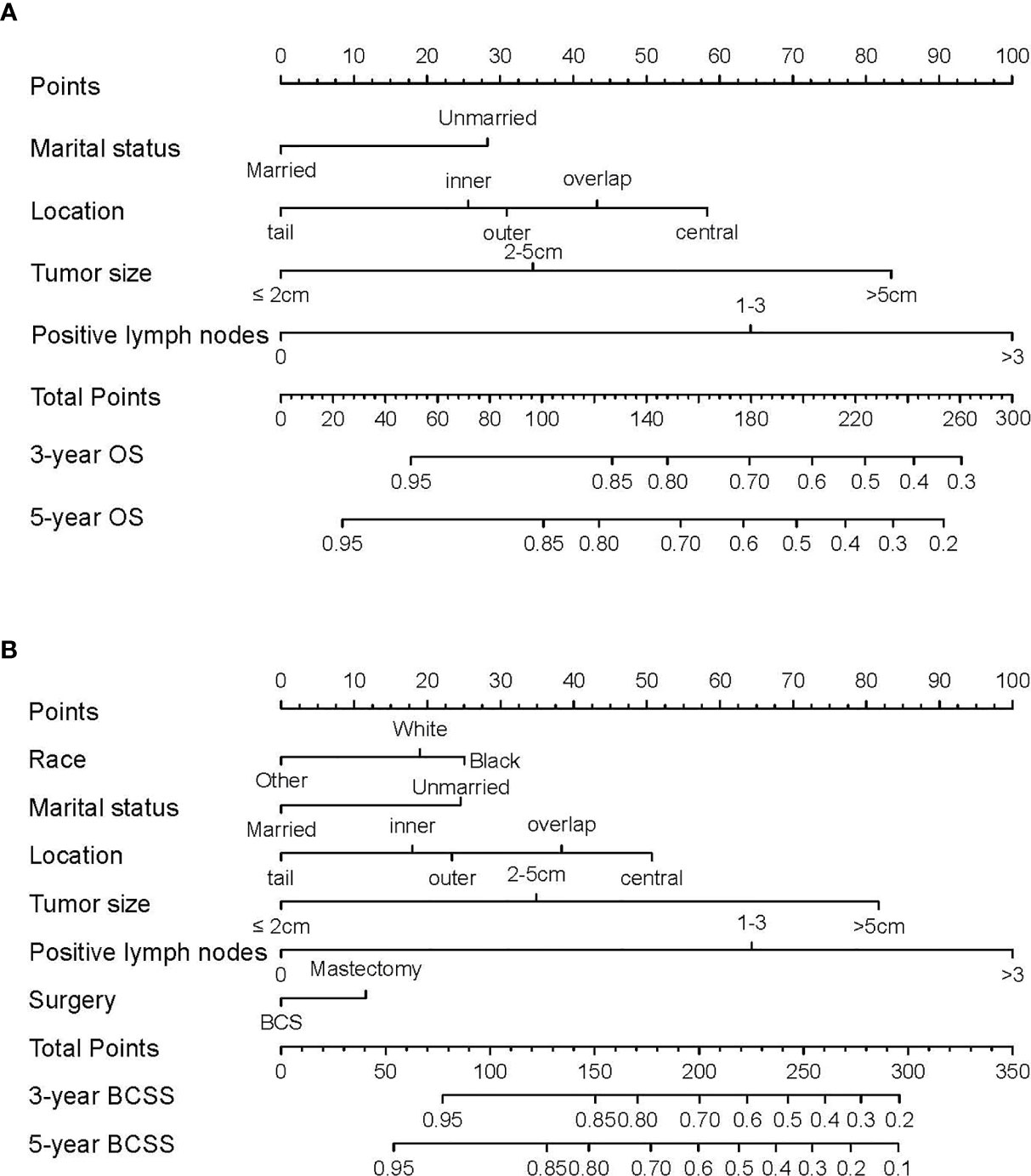
Figure 1 Nomograms predicting 3- and 5-year (A) overall survival (OS) and (B) breast cancer-specific survival (BCSS) in TNBC patients of childbearing age. Instructions for nomogram use were as follows. First, assign points to each characteristic for a given patient by drawing a vertical line from that variable to the points scale. Then, sum all the points and draw a vertical line from the total points scale to the 3- and 5-year OS or BCSS to obtain the likelihood of 3- or 5-year survival.
Model Validation
Nomogram validation was processed in both the training and validation cohorts. In the training cohort, the Harrell’s C-indexes for the nomograms for predicting OS and BCSS were 0.766 and 0.776, respectively. In the validation cohort, the C-indexes were slightly lower, i.e., 0.763 and 0.765, respectively (Table S1). The external and internal calibration curves were shown in Figure 2 and Figure S1, which demonstrated an excellent agreement between the actual and nomogram-predicted survival rates. The time-dependent ROC curves for predicting 3- and 5-year OS and BCSS in the training and validation cohorts were presented in Figure 3. With the risk score as a continuous variable, the AUCs for 3- and 5-year OS and BCSS predictions were all above 0.74. These results demonstrated that the nomograms were useful tools for the prediction of survival in TNBC patients of childbearing age.
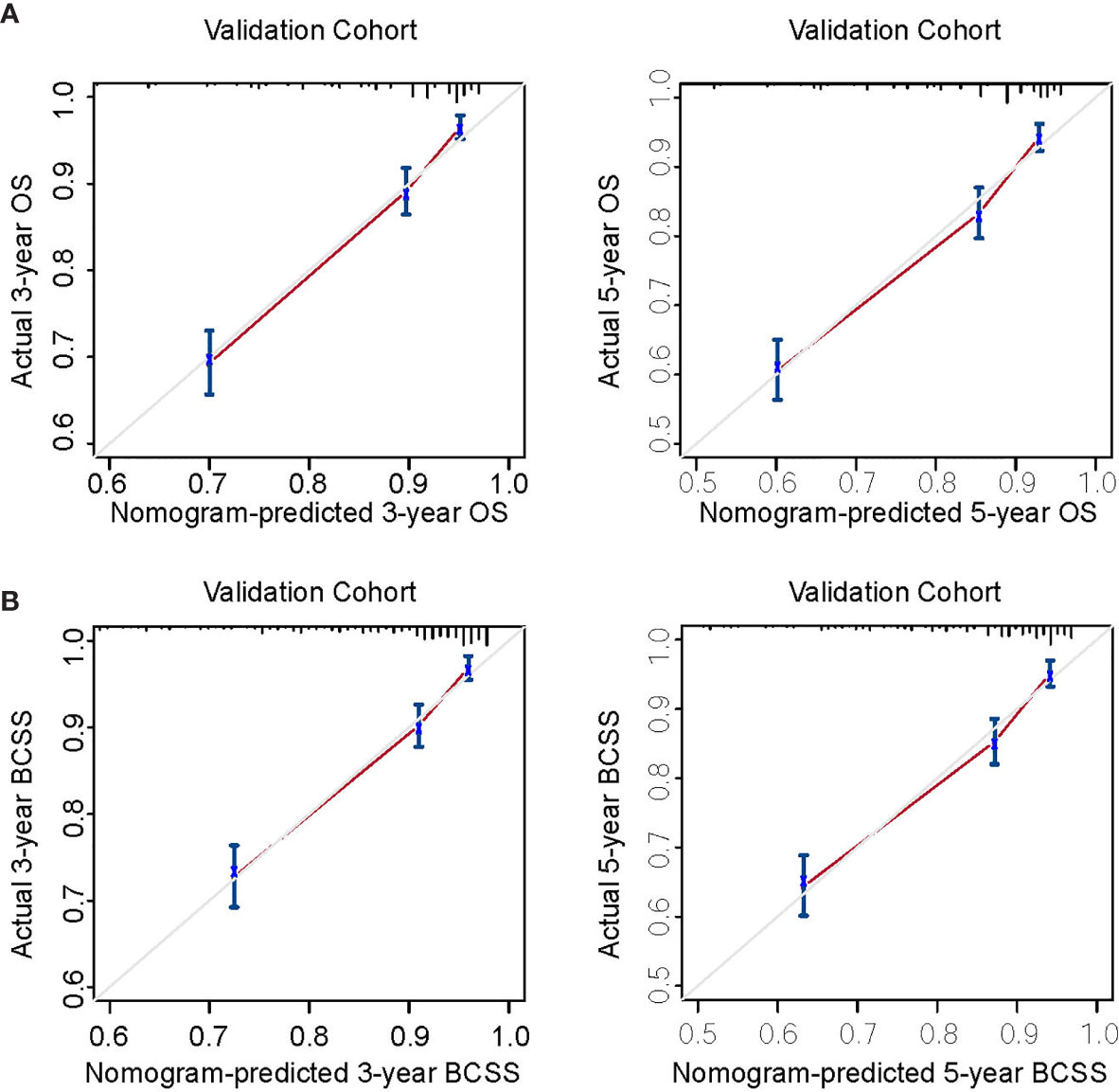
Figure 2 Calibration curves for external validation. (A) Nomogram calibration curves for 3- and 5-year overall survival (OS). (B) Nomogram calibration curves for 3- and 5-year breast cancer-specific survival (BCSS). X-axis, nomogram-predicted survival; Y-axis, actual survival.
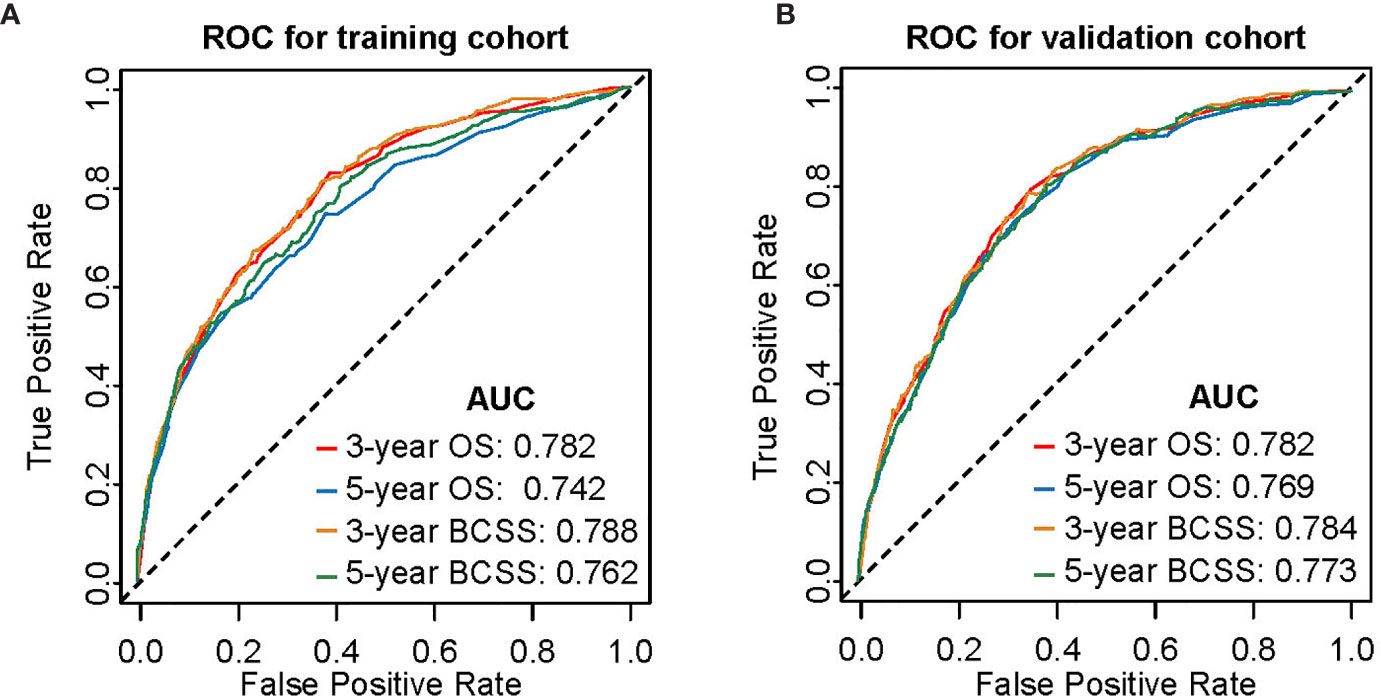
Figure 3 Time-dependent receiver operating characteristic (ROC) curves for predicting 3- and 5-year OS and BCSS. (A) Internal validation in the training cohort. (B) External validation in the validation cohort. AUC, area under curve; OS, overall survival; BCSS, breast cancer-specific survival.
Discussion
In the light of the relatively high mobility and invasiveness of tumor, strong desire to preserve and restore physiological and social functions, high requirements for quality of life, and unique clinicopathological features of TNBC patients of childbearing age, a brief nomogram based on follow-up data of a large cohort for predicting OS and BCSS should be quite practical in clinic. Although nomograms predicting survival of patients with TNBC or of a specific age group have been reported (20, 22, 31–33, 35), there is no nomogram for TNBC patients of childbearing age, a period of highly active physiological and social function. Using data from the SEER program, we established reliable nomograms to predict the 3- and 5-year OS and BCSS of these patients based on Cox regression and competing-risk models. The calibration curves, time-dependent ROC analysis and Harrell’s C-indexes demonstrated satisfactory performances of our nomograms. Therefore, our nomograms can be used for personalized risk prediction and to guide treatment for TNBC patients of childbearing age.
In the current study, several demographics and clinicopathologic characteristics were shown to be prognosis factors of 3- and 5-year OS and BCSS, including marital status, tumor location, tumor size, number of positive lymph nodes, race and surgery, corroborating previous studies of TNBC patients (42–46). The primary tumor site is considered as an important independent prognostic factor of breast cancer, and several studies have shown that tumor location in lower inner zone suggests a poor prognosis (47, 48). In the univariate analysis of OS in the training cohort, OS was significantly different among the five groups of tumor location. In multivariate analysis, though only the “overlap” site was significantly associated with poor prognosis, the hazard ratios of various groups confirmed the impact of tumor location on OS. Therefore, this factor was incorporated in the nomogram for predicting OS. As for BCSS, different groups of location were significantly different in CIBCSD and showed no differences in CINBCSD, indicating that tumor location is a significant prognosis factor of breast cancer-specific death. According to the CIBCSD of each group, we developed a scoring system to qualify the risk caused by tumor location. Previous studies have shown higher incidence of TNBC among black women compared with other races, which is determined by biological differences and socioeconomic factors (45, 49). In addition, African ethnicity is a significant and independent predictor of poor outcome (50). In this study, race was a significant but not independent prognosis factor of OS. However, regarding BCSS, black women had higher CIBCSD compared with white and “other” patients. Therefore, race was included in our scoring system for BCSS prediction but not for OS prediction.
Despite the above strengths, there were some limitations in this study. First, the undetailed data of adjuvant chemotherapy and radiotherapy in the SEER database cannot distinguish “no treatment” and “unknown if patients received treatment”, which were combined into a group in our study. Moreover, the lack of information on neoadjuvant therapy in SEER database made it impossible to evaluate the relationship between neoadjuvant therapy and survival in this paper. Some other proven prognostic factors of breast cancer in childbearing age, including breastfeeding, adiposity, and oral contraceptive use (51), were also not available in the SEER database. Although more detailed treatment information is available in other databases, i.e., the National Cancer Database (NCDB) database, we chose SEER as our data source because NCDB is a hospital-based rather than population-based database without available BCSS data (52–54). Second, breast cancer that occurs in patients before the age of 45 has a higher potential to result from hereditary causes. Patients with hereditary breast cancer have a higher risk of recurrence and death. However, because of the lack of genetic data in SEER datasets, we cannot incorporate this important factor into our nomograms, which may lead to predictive bias. Third, patients with incomplete clinical information or survival data were excluded from this study, which could result in selection bias. Further, limited by its single data source and retrospective nature, the nomograms should be further validated in other cohorts, and a prospective research should be performed before its clinical application.
Conclusion
We developed nomograms to predict OS and BCSS in TNBC patients of childbearing age based on a relatively large cohort with detailed follow-up. The nomograms had excellent performances in both training and validation cohorts. It may serve as an efficient tool for clinicians to predict the prognosis of these patients and to guide individualized treatment.
Data Availability Statement
The original contributions presented in the study are included in the article/Supplementary Materials; further inquiries can be directed to the corresponding author.
Ethics Statement
Since the data were from the SEER database, informed patient consent and ethical approval were not required.
Author Contributions
XC conceptualized the work, collected, analyzed, and interpreted the date, drafted the article, critically revised the article, and gave the final approval of the version to be published. DS and XL conceptualized the work, critically revised the article, and gave the final approval of the version to be published. All authors contributed to the article and approved the submitted version.
Funding
This work was supported by grants from the Training Plan of Excellent Talents of The First People’s Hospital of Shangqiu (SQFPH2019). The funder had no role in the study design, data collection and analysis, decision to publish, or preparation of the manuscript.
Conflict of Interest
The authors declare that the research was conducted in the absence of any commercial or financial relationships that could be construed as a potential conflict of interest.
Supplementary Material
The Supplementary Material for this article can be found online at: https://www.frontiersin.org/articles/10.3389/fonc.2020.636549/full#supplementary-material
References
1. Siegel RL, Miller KD, Jemal A. Cancer statistics, 2019. CA: A Cancer J Clin (2019) 69(1):7–34. doi: 10.3322/caac.21551
2. DeSantis CE, Ma J, Gaudet MM, Newman LA, Miller KD, Goding Sauer A, et al. Breast cancer statistics, 2019. CA: Cancer J Clin (2019) 69(6):438–51. doi: 10.3322/caac.21583
3. Garrido-Castro AC, Lin NU, Polyak K. Insights into Molecular Classifications of Triple-Negative Breast Cancer: Improving Patient Selection for Treatment. Cancer Discov (2019) 9(2):176–98. doi: 10.1158/2159-8290.Cd-18-1177
4. Sajid MT, Ahmed M, Azhar M, Mustafa QU, Shukr I, Ahmed M, et al. Age-related frequency of triple negative breast cancer in women. J Coll Physicians Surgeons–Pakistan JCPSP (2014) 24(6):400–3.
5. Scott LC, Mobley LR, Kuo T-M, Il’yasova D. Update on triple-negative breast cancer disparities for the United States: A population-based study from the United States Cancer Statistics database, 2010 through 2014. Cancer (2019) 125(19):3412–7. doi: 10.1002/cncr.32207
6. Aapro M, Wildiers H. Triple-negative breast cancer in the older population. Ann Oncol (2012) Suppl 6:vi52–5. doi: 10.1093/annonc/mds189
7. Liedtke C, Hess KR, Karn T, Rody A, Kiesel L, Hortobagyi GN, et al. The prognostic impact of age in patients with triple-negative breast cancer. Breast Cancer Res Treat (2013) 138(2):591–9. doi: 10.1007/s10549-013-2461-x
8. Plichta JK, Thomas SM, Vernon R, Fayanju OM, Rosenberger LH, Hyslop T, et al. Breast cancer tumor histopathology, stage at presentation, and treatment in the extremes of age. Breast Cancer Res Treat (2020) 180(1):227–35. doi: 10.1007/s10549-020-05542-4
9. Lambertini M, Goldrat O, Clatot F, Demeestere I, Awada A. Controversies about fertility and pregnancy issues in young breast cancer patients: current state of the art. Curr Opin Oncol (2017) 29(4):243–52. doi: 10.1097/cco.0000000000000380
10. Paluch-Shimon S, Pagani O, Partridge AH, Abulkhair O, Cardoso MJ, Dent RA, et al. ESO-ESMO 3rd international consensus guidelines for breast cancer in young women (BCY3). Breast (2017) 35:203–17. doi: 10.1016/j.breast.2017.07.017
11. Oktay K, Harvey BE, Partridge AH, Quinn GP, Reinecke J, Taylor HS, et al. Fertility Preservation in Patients With Cancer: ASCO Clinical Practice Guideline Update. J Clin Oncol (2018) 36(19):1994–2001. doi: 10.1200/jco.2018.78.1914
12. Christinat A, Pagani O. Fertility after breast cancer. Maturitas (2012) 73(3):191–6. doi: 10.1016/j.maturitas.2012.07.013
13. Murphy BL, Day CN, Hoskin TL, Habermann EB, Boughey JC. Neoadjuvant Chemotherapy Use in Breast Cancer is Greatest in Excellent Responders: Triple-Negative and HER2+ Subtypes. Ann Surg Oncol (2018) 25(8):2241–8. doi: 10.1245/s10434-018-6531-5
14. Rosenberg SM, Newman LA, Partridge AH. Breast Cancer in Young Women: Rare Disease or Public Health Problem? JAMA Oncol (2015) 1(7):877–8. doi: 10.1001/jamaoncol.2015.2112
15. Ma D, Jiang YZ, Xiao Y, Xie MD, Zhao S, Jin X, et al. Integrated molecular profiling of young and elderly patients with triple-negative breast cancer indicates different biological bases and clinical management strategies. Cancer (2020) 126(14):3209–18. doi: 10.1002/cncr.32922
16. Balachandran VP, Gonen M, Smith JJ, DeMatteo RP. Nomograms in oncology: more than meets the eye. Lancet Oncol (2015) 16(4):e173–80. doi: 10.1016/s1470-2045(14)71116-7
17. Chu JL, Yang DH, Wang L, Xia JL. Nomograms predicting survival for all four subtypes of breast cancer: a SEER-based population study. Ann Trans Med (2020) 8(8):544. doi: 10.21037/atm-20-2808
18. Luo CX, Zhong XR, Wang Z, Wang Y, Wang YP, He P, et al. Prognostic nomogram for patients with non-metastatic HER2 positive breast cancer in a prospective cohort. Int J Biol Markers (2019) 34(1):41–6. doi: 10.1177/1724600818824786
19. Li D, Zhong CH, Cheng Y, Zhu N, Tan YN, Zhu LZ, et al. A Competing Nomogram to Predict Survival Outcomes in Invasive Micropapillary Breast Cancer. J Cancer (2019) 10(27):6801–12. doi: 10.7150/jca.27955
20. Guo LW, Jiang LM, Gong Y, Zhang HH, Li XG, He M, et al. Development and validation of nomograms for predicting overall and breast cancer-specific survival among patients with triple-negative breast cancer. Cancer Manage Res (2018) 10:5881–94. doi: 10.2147/cmar.S178859
21. Ye FG, Xia C, Ma D, Lin PY, Hu X, Shao ZM. Nomogram for predicting preoperative lymph node involvement in patients with invasive micropapillary carcinoma of breast: a SEER population-based study. BMC Cancer (2018) 18(1):1085. doi: 10.1186/s12885-018-4982-5
22. Dai DJ, Jin HC, Wang X. Nomogram for predicting survival in triple-negative breast cancer patients with histology of infiltrating duct carcinoma: a population-based study. Am J Cancer Res (2018) 8(8):1576–85.
23. Sun W, Jiang YZ, Liu YR, Ma D, Shao ZM. Nomograms to estimate long-term overall survival and breast cancer-specific survival of patients with luminal breast cancer. Oncotarget (2016) 7(15):20496–506. doi: 10.18632/oncotarget.7975
24. Diao JD, Ma LX, Sun MY, Wu CJ, Wang LJ, Liu YL, et al. Construction and validation of a nomogram to predict overall survival in patients with inflammatory breast cancer. Cancer Med (2019) 8(12):5600–8. doi: 10.1002/cam4.2470
25. Zhang HG, Ma GF, Du SS, Sun J, Zhang Q, Yuan BY, et al. Nomogram for predicting cancer specific survival in inflammatory breast carcinoma: a SEER population-based study. Peerj (2019) 7:e7659. doi: 10.7717/peerj.7659
26. Pan XX, Yang W, Chen YF, Tong LH, Li CR, Li H. Nomogram for predicting the overall survival of patients with inflammatory breast cancer: A SEER-based study. Breast (2019) 47:56–61. doi: 10.1016/j.breast.2019.05.015
27. Zhao W, Wu L, Zhao AD, Zhang M, Tian Q, Shen YW, et al. A nomogram for predicting survival in patients with de novo metastatic breast cancer: a population-based study. BMC Cancer (2020) 20(1):982. doi: 10.1186/s12885-020-07449-1
28. Xiong Y, Cao H, Zhang YQ, Pan Z, Dong SY, Wang GSY, et al. Nomogram-Predicted Survival of Breast Cancer Brain Metastasis: a SEER-Based Population Study. World Neurosurg (2019) 128:E823–E34. doi: 10.1016/j.wneu.2019.04.262
29. Wang Z, Cheng YG, Chen S, Shao HY, Chen XW, Wang ZN, et al. Novel prognostic nomograms for female patients with breast cancer and bone metastasis at presentation. Ann Trans Med (2020) 8(5):197. doi: 10.21037/atm.2020.01.37
30. Lin ZH, Yan SC, Zhang JY, Pan Q. A Nomogram for Distinction and Potential Prediction of Liver Metastasis in Breast Cancer Patients. J Cancer (2018) 9(12):2098–106. doi: 10.7150/jca.24445
31. Gong Y, Ji P, Sun W, Jiang YZ, Hu X, Shao ZM. Development and Validation of Nomograms for Predicting Overall and Breast Cancer-Specific Survival in Young Women with Breast Cancer: A Population-Based Study. Transl Oncol (2018) 11(6):1334–42. doi: 10.1016/j.tranon.2018.08.008
32. Lu X, Li X, Ling H, Gong Y, Guo L, He M, et al. Nomogram for Predicting Breast Cancer-Specific Mortality of Elderly Women with Breast Cancer. Med Sci Monit (2020) 26:e925210. doi: 10.12659/MSM.925210
33. Sun Y, Li Y, Wu J, Tian H, Liu H, Fang Y, et al. Nomograms for prediction of overall and cancer-specific survival in young breast cancer. Breast Cancer Res Treat (2020) 184(2):597–613. doi: 10.1007/s10549-020-05870-5
34. Li X, Dai D, Chen B, He S, Zhang J, Wen C, et al. Prognostic Values Of Preoperative Serum CEA And CA125 Levels And Nomograms For Young Breast Cancer Patients. Onco Targets Ther (2019) 12:8789–800. doi: 10.2147/OTT.S221335
35. Lin H, Zhang F, Wang L, Zeng. Use of clinical nomograms for predicting survival outcomes in young women with breast cancer. Oncol Lett (2019) 17(2):1505–16. doi: 10.3892/ol.2018.9772
36. Kuhn M. caret: Classification and Regression Training. (2020). Available at: https://cran.r-project.org/web/packages/caret/index.html.
37. Gray R. A Class of $K$-Sample Tests for Comparing the Cumulative Incidence of a Competing Risk. Ann Stat (1988) 16:1141–54. doi: 10.1214/aos/1176350951
38. Gary B. cmprsk: Subdistribution Analysis of Competing Risk. (2014). Available at: https://cran.r-project.org/web/packages/cmprsk/index.html.
39. Harrell Jr FE. rms: Regression Modeling Strategies. (2017). Available at: https://cran.r-project.org/web/packages/rms/index.html.
40. Zhang J, Jin Z. nomogramFormula: Calculate Total Points and Probabilities for Nomogram. (2020). Available at: https://cran.r-project.org/web/packages/nomogramFormula/index.html.
41. Patrick J. Heagerty and packaging by Paramita Saha-Chaudhuri. survivalROC: Time-dependent ROC curve estimation from censored survival data. (2013). Available at: https://cran.r-project.org/web/packages/survivalROC/index.html.
42. Yu KD, Jiang YZ, Chen S, Cao ZG, Wu J, Shen ZZ, et al. Effect of large tumor size on cancer-specific mortality in node-negative breast cancer. Mayo Clinic Proc (2012) 87(12):1171–80. doi: 10.1016/j.mayocp.2012.07.023
43. Falagas ME, Zarkadoulia EA, Ioannidou EN, Peppas G, Christodoulou C, Rafailidis PI. The effect of psychosocial factors on breast cancer outcome: a systematic review. Breast Cancer Res BCR (2007) 9(4):R44. doi: 10.1186/bcr1744
44. Carter CL, Allen C, Henson DE. Relation of tumor size, lymph node status, and survival in 24,740 breast cancer cases. Cancer (1989) 63(1):181–7. doi: 10.1002/1097-0142(19890101)63:1<181::aid-cncr2820630129>3.0.co;2-h
45. Jemal A, Robbins AS, Lin CC, Flanders WD, DeSantis CE, Ward EM, et al. Factors That Contributed to Black-White Disparities in Survival Among Nonelderly Women With Breast Cancer Between 2004 and 2013. J Clin Oncol (2018) 36(1):14–24. doi: 10.1200/jco.2017.73.7932
46. Orecchia R. Breast cancer: post-mastectomy radiotherapy reduces recurrence and mortality. Nat Rev Clin Oncol (2014) 11(7):382–4. doi: 10.1038/nrclinonc.2014.95
47. Yang J, Tang S, Zhou Y, Qiu J, Zhang J, Zhu S, et al. Prognostic implication of the primary tumor location in early-stage breast cancer: focus on lower inner zone. Breast Cancer (Tokyo Japan) (2018) 25(1):100–7. doi: 10.1007/s12282-017-0797-5
48. Wu S, Zhou J, Ren Y, Sun J, Li F, Lin Q, et al. Tumor location is a prognostic factor for survival of Chinese women with T1-2N0M0 breast cancer. Int J Surg (London England) (2014) 12(5):394–8. doi: 10.1016/j.ijsu.2014.03.011
49. Jatoi I, Anderson WF, Rao SR, Devesa SS. Breast cancer trends among black and white women in the United States. J Clin Oncol (2005) 23(31):7836–41. doi: 10.1200/jco.2004.01.0421
50. Newman LA, Griffith KA, Jatoi I, Simon MS, Crowe JP, Colditz GA. Meta-analysis of survival in African American and white American patients with breast cancer: ethnicity compared with socioeconomic status. J Clin Oncol (2006) 24(9):1342–9. doi: 10.1200/jco.2005.03.3472
51. Chollet-Hinton L, Olshan AF, Nichols HB, Anders CK, Lund JL, Allott EH, et al. Biology and Etiology of Young-Onset Breast Cancers among Premenopausal African American Women: Results from the AMBER Consortium. Cancer Epidemiol Biomarkers Prev Publ Am Assoc Cancer Research Cosponsored by Am Soc Prevent Oncol (2017) 26(12):1722–9. doi: 10.1158/1055-9965.Epi-17-0450
52. Mettlin CJ, Menck HR, Winchester DP, Murphy GP. A comparison of breast, colorectal, lung, and prostate cancers reported to the National Cancer Data Base and the Surveillance, Epidemiology, and End Results Program. Cancer (1997) 79(10):2052–61. doi: 10.1002/(sici)1097-0142(19970515)79:10<2052::aid-cncr29>3.0.co;2-s
53. Lin CC, Virgo KS, Robbins AS, Jemal A, Ward EM. Comparison of Comorbid Medical Conditions in the National Cancer Database and the SEER-Medicare Database. Ann Surg Oncol (2016) 23(13):4139–48. doi: 10.1245/s10434-016-5508-5
Keywords: nomogram, triple-negative breast cancer, overall survival, breast cancer-specific survival, SEER, prognosis, prediction, childbearing age
Citation: Cui X, Song D and Li X (2021) Construction and Validation of Nomograms Predicting Survival in Triple-Negative Breast Cancer Patients of Childbearing Age. Front. Oncol. 10:636549. doi: 10.3389/fonc.2020.636549
Received: 01 December 2020; Accepted: 21 December 2020;
Published: 08 February 2021.
Edited by:
Yi-Zhou Jiang, Fudan University, ChinaCopyright © 2021 Cui, Song and Li. This is an open-access article distributed under the terms of the Creative Commons Attribution License (CC BY). The use, distribution or reproduction in other forums is permitted, provided the original author(s) and the copyright owner(s) are credited and that the original publication in this journal is cited, in accordance with accepted academic practice. No use, distribution or reproduction is permitted which does not comply with these terms.
*Correspondence: Xiang Cui, eGlhbmdjdWkyMjNAMTYzLmNvbQ==