- 1Department of Radiology, University of Turku, Turku, Finland
- 2Turku Brain and Mind Center, University of Turku, Turku, Finland
- 3Department of Biotechnology and Molecular Medicine, A.I. Virtanen Institute for Molecular Sciences, Kuopio, Finland
- 4Turku PET Centre, University of Turku and Turku University Hospital, Turku, Finland
- 5Turku Center for Disease Modeling, University of Turku, Turku, Finland
- 6Medical Imaging Centre of Southwest Finland, Turku University Hospital, Turku, Finland
- 7Department of Oncology and Radiotherapy, Turku University Hospital, Turku, Finland
- 8Research Unit of Medical Imaging, Physics and Technology, University of Oulu, Oulu, Finland
- 9Department of Clinical Radiology, Oulu University Hospital, Oulu, Finland
- 10Department of Radiology, University of Oulu, Oulu, Finland
Purpose: To evaluate fitting quality and repeatability of four mathematical models for diffusion weighted imaging (DWI) during tumor progression in mouse xenograft model of prostate cancer.
Methods: Human prostate cancer cells (PC-3) were implanted subcutaneously in right hind limbs of 11 immunodeficient mice. Tumor growth was followed by weekly DWI examinations using a 7T MR scanner. Additional DWI examination was performed after repositioning following the fourth DWI examination to evaluate short term repeatability. DWI was performed using 15 and 12 b-values in the ranges of 0-500 and 0-2000 s/mm2, respectively. Corrected Akaike information criteria and F-ratio were used to evaluate fitting quality of each model (mono-exponential, stretched exponential, kurtosis, and bi-exponential).
Results: Significant changes were observed in DWI data during the tumor growth, indicated by ADCm, ADCs, and ADCk. Similar results were obtained using low as well as high b-values. No marked changes in model preference were present between the weeks 1−4. The parameters of the mono-exponential, stretched exponential, and kurtosis models had smaller confidence interval and coefficient of repeatability values than the parameters of the bi-exponential model.
Conclusion: Stretched exponential and kurtosis models showed better fit to DWI data than the mono-exponential model and presented with good repeatability.
Introduction
Diffusion weighed imaging (DWI) has extensively been used for cancer characterization in both pre-clinical (1, 2) and clinical settings (3) during the last decade. Furthermore, DWI is increasingly being used for monitoring cancer therapy responses (4). In biological tissue, DWI contrast is predominantly affected by microscopic motion of water molecules and water interactions with surroundings. The most recognized DWI imaging acquisition method is the Stejskal–Tanner pulsed field gradient method. With this method, motion caused by self diffusion of a proton is acquired by applying a pair of motion-encoding gradients. The first gradient dephases and second one rephrases stationary protons, while moving water protons stays dephased resulting to decreased signal intensity. The signal attenuation depends on water diffusion coefficient (D [mm2/s]) as well as direction of the self diffusion of water (5).
Several different mathematical models have been proposed to describe the DWI signal decay. The mono-exponential model is the simplest and widely used, in which one parameter D (or often the apparent diffusion coefficient, ADC) describes the diffusion. This model fits well to DWI data measured from pure water without any restrictions. At low b-values DWI signal decay deviates from the mono-exponential function due to presence of intra-voxel incoherent motion (IVIM), as originally proposed by Le Bihan and co-workers (6, 7). The search for new non-invasive imaging markers has led to increased interests in IVIM-derived parameters, which have demonstrated correlation with microvessel density in colorectal cancer (HT29) model (8). Nevertheless, IVIM-derived parameters are not directly related to tissue perfusion (6, 7), but perfusion and IVIM-derived parameters are rather related to the capillary structure (9, 10). Similarly to low b-values, DWI signal decay at high b-values deviates from the mono-exponential function, and it is better described by non-Gaussian mathematical models (11–14).
In general, a single mono-exponential decay provides oversimplified description of the complicated water motion in the tissue. However, the modeling with several free parameters could lead to “over-fitting” of data, and poor repeatability of the fitted parameters. Optimal model would have the highest information content and provides independent parameters, which are related to physical quantities (e.g. cell and/or vessel density) while still retain high repeatability/reliability of fitted parameters.
The Akaike information criteria (AIC) has been widely used for model selection in previous studies (15–17). A model with smaller AIC value would be a preferred model due to less information loss as compared with a model presenting with higher AIC. Similarly to AIC, F-ratio is commonly being applied for model selection (18). Model selection based on F-ratio tends to prefer a more simplified model (19) in contrast to AIC.
In the current study, we evaluated four different mathematical models for DWI within a study applying PC-3 prostate cancer cells grown in immunodeficient mice using both low (0-500 s/mm2) and high b-values (0-2000 s/mm2). The tumor growth was followed for four weeks with repeated MR examinations performed once a week. Corrected Akaike information criteria (AICc) and F-ratio were used to evaluate information content of the models. Non-Gaussian DWI models provided better fit to DWI data obtained using both low and high b-values. However, non-Gaussian DWI models were not clearly preferred over the mono-exponential model for DWI data obtained using low b-values in contrast to DWI data obtained using high b-values. Furthermore, DWI fitted parameters changed significantly during tumor progression.
Material and Methods
Animal Tumor Model
One million PC-3 (Anticancer Inc., USA) human prostate cancer cells were inoculated subcutaneously in immunodeficient mice (n=11, HSD: Athymic Nude Foxn 1nu, Harlan Laboratories, Indianapolis, IN, USA). The cells also expressed red florescent protein, while this property was not applied in the present study. Mice were housed in individually ventilated cages under controlled conditions of light (12h light/12h dark), temperature (21 ± 3°C), and humidity (55% ± 15%) in specific pathogen-free conditions at the Central Animal Laboratory, University of Turku for the first 5 days, and thereafter in similar conditions at the University of Eastern Finland Kuopio campus. Mice were provided with irradiated soy-free natural-ingredient feed (RM3 (E), Special Diets Services, Essex, UK) and autoclaved tap water ad libitum, and were housed complying with international guidelines on the care and use of laboratory animals. All animal handling was conducted in accordance with the Finnish Committee for the use and care of laboratory animals and the institutional animal care policies, which fully meet the requirements as defined in the U.S. National Institutes of Health guidelines on animal experimentation.
MR Imaging
The first MR examination was performed 8 days after cell implantation. Tumor growth was followed for four weeks with repeated MR examinations once a week. Immediately following the fourth MR examination, six and seven mice had repeated DWI scan performed using low and high b-values, respectively. The second repetition was performed following animal and coil repositioning approximately 60 minutes after the first set of DWI. The repeated DWI examinations were used to evaluate short term repeatability of the measured parameters. The anesthetized mice (1.5% isoflurane in 70%N2/30%O2) were imaged using a 7T animal MR scanner (7T Pharmascan, Bruker GmbH, Ettlingen, Germany) with 72 mm volume transmitter (Bruker GmbH) and 10 mm surface receiver coil (Bruker GmbH). Multislice T2-weighted anatomical images covering the whole tumor area were obtained (TR/TE 2500 ms/33 ms, field of view (FOV) = 30 × 30 mm2, matrix size 256 × 256, 15 slices) to localize a slice with maximum tumor diameter for DWI measurements. Diffusion weighted single shot spin-echo echo planar imaging was applied with the parameters: TR/TE 3750/25.3 (low b-value set) 3000/30 ms (high b-value set), FOV 3 × 1.5 cm2, matrix 128 ×64, slice thickness 1 mm, three orthogonal diffusion directions, and two different sets of b-values: low b-value set (15 b-values in total): 0, 2, 4, 6, 9, 12, 14, 18, 23, 25, 28, 50, 100, 300, 500 s/mm2, and high b-value set (12 b-values in total): 0, 100, 300, 500, 700, 900, 1100, 1300, 1500, 1700, 1900, 2000 s/mm2. For further analysis, the mean value of the signal from three directions was calculated.
Data Modeling
The following four mathematical models were applied to the DWI signal obtained using low and high b-values:
1. Mono-exponential model:
where b is the b-value, S(0) is the signal intensity at b-value of 0 s/mm2, and ADCm is the apparent diffusion coefficient calculated using the mono-exponential model.
2. Stretched exponential model also known as Kohlrausch-Williams-Watts model (20):
where ADCs is the apparent diffusion coefficient calculated using the stretched exponential model, and α is the heterogeneity index. The dimensionless α parameter varies from 0 to 1. During the fitting procedure, α parameter was constrained to be in the range of 0 to 1.
3. Kurtosis model:
where ADCk is the apparent diffusion coefficient calculated using the kurtosis model, and K is the kurtosis. Jensen at al. (21) originally developed the kurtosis model to fit deviation of diffusion tensor signal from the mono-exponential function. The dimensionless positive K parameter characterizes the deviation from the mono-exponential signal decay.
4a. Bi-exponential model for low b-values:
where fp is the “pseudodiffusion” fraction, Df is the fast diffusion coefficient, and Dp is the “pseudodiffusion” coefficient. The intravoxel incoherent motion (IVIM) theory is an advanced method to separate diffusion and perfusion effects using DWI (6) at low b-values. According to the IVIM theory, the blood flow in the capillaries causes a dephasing of the magnetization when motion-encoding gradients are applied. This means that the motion of water molecules due to microcirculation of blood in the capillaries has a similar effect on the resulting DWI signal as their motion due to molecular diffusion.
4b. Bi-exponential model for high b-values:
where ff is the fraction of fast diffusion, Df is the fast diffusion coefficient, and Ds is the slow diffusion coefficient.
The DWI signal decay of each individual voxel has been fitted using four mathematical models, as described above, to generate parametric maps of the parameters. The fitting procedure has been performed using in-house written C++ code utilizing Broyden–Fletcher–Goldfarb–Shanno (BFGS) algorithm (22) in dlib library (23).
Following multiple initializations values were used to prevent local minima in the fitting procedure in order to avoid local minima in the fitting procedure (initializations values for high b-values data are in brackets):
1. Mono-exponential:
2. Stretched exponential:
3. Kurtosis:
4a (b). Biexponential:
Image Analysis
The tumor area was manually delineated on T2-weighted anatomical images and the regions of interest (ROIs) were defined to the corresponding parametric images. Voxels with ADCm values higher than 8.0^10-3 s2/mm were discarded as those voxels were considered to represent necrosis. Median values of the fitted parameters of each ROI between repeated scans were compared using one-way analysis of variance with Bonferroni test (p<0.05 statistically significant).
Evaluation of Fitting Quality
Corrected Akaike information criteria difference (ΔAICc) (15) was used to evaluate model fit to DWI data of each individual voxel:
where N is the sample size, P is the number of parameters, SS is the sum of squares between data points and fitted curve, A subscript represents the simpler model, B subscripts represents the more complex model.
In addition to ΔAICc, F-ratio (F) with 1% level of significance was used to evaluate model fit to data:
where DF (= number of data points − number of parameters) is the degree of freedom, A subscript represents the simpler model, B subscripts represents the more complex model.
Repeatability of the Fitted Parameters
Repeatability of the fitted parameters was evaluated using the same methodology as in a previous human DWI drug intervention study (24). The difference (d) in median values per ROI between two repeated scans performed 4 weeks after the initial scan was calculated for a subset of mice (six mice for low b-values and seven for high b-values). Mean squared difference (msd) was calculated as follows:
where d is the difference between two repeated scans, n is the number of mice with repeated scan. Subsequently, 95% confidence interval (CI) for changes in the study cohort was calculated:
where msd is the mean squared difference, n is the number of mice with repeated scan.Finally, coefficient of repeatability (r) was calculated as follows:
Results
PC-3 cancer cell growth was followed for 4 weeks with repeated MR examinations performed once a week. The changes in diffusion parameters are visualized in parametric maps on top of T2-weighted images for a representative case (Figures 1 and 2) while the rest of data are shown in Supplementary material (Figures S1–S20). Median signal intensity of tumor ROI and the correcting fitted curves of week 1 and week 4 are shown in Figure 3. The data is shown for the same representative tumor as shown in Figure 1.
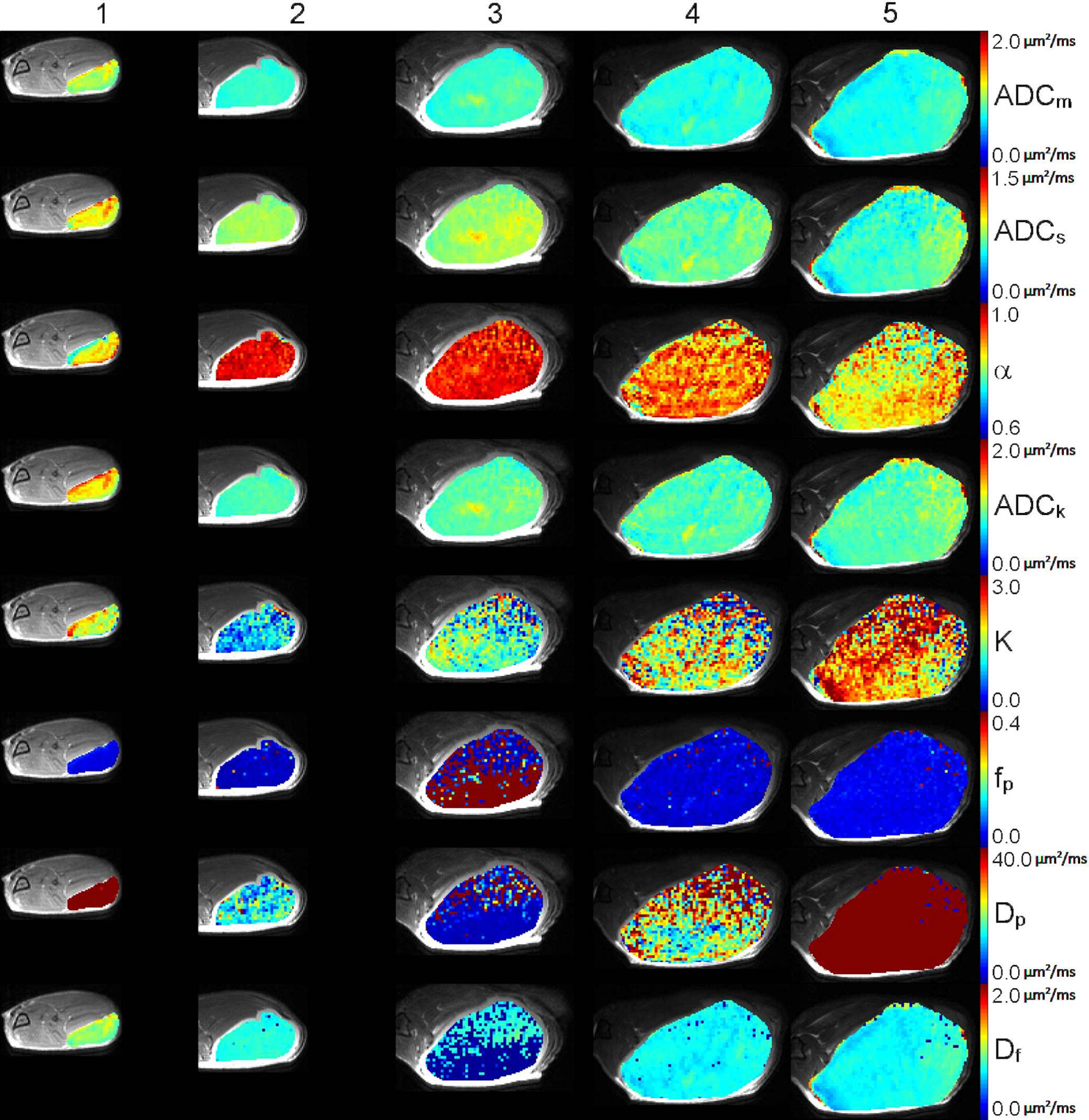
Figure 1 Low b-value DWI data of a representative tumor: T2-weighted image fused with parametric maps for ADCm, ADCs, α, ADCk, K, fp, Dp, and Df parameters are shown, and represent different degree of tumor homogeneity between week 1 (column 1), week 2 (column 2), week 3 (column 4) and week 4 (column 1). Furthermore, the second repeated imaging on week 4 is shown (column 5). The parametric maps are scaled as follows: ADCm, min−max: 0−2.0 µm2/ms; ADCs, min−max: 0−1.5 µm2/ms; α, min−max: 0.6−1.0; ADCk, min−max: 0−2.0 µm2/ms; K, min−max: 0−3.0; fp, min−max: 0−0.4; Dp, min−max: 0−40.0 µm2/ms; Df, min−max: 0−2.0 µm2/ms.
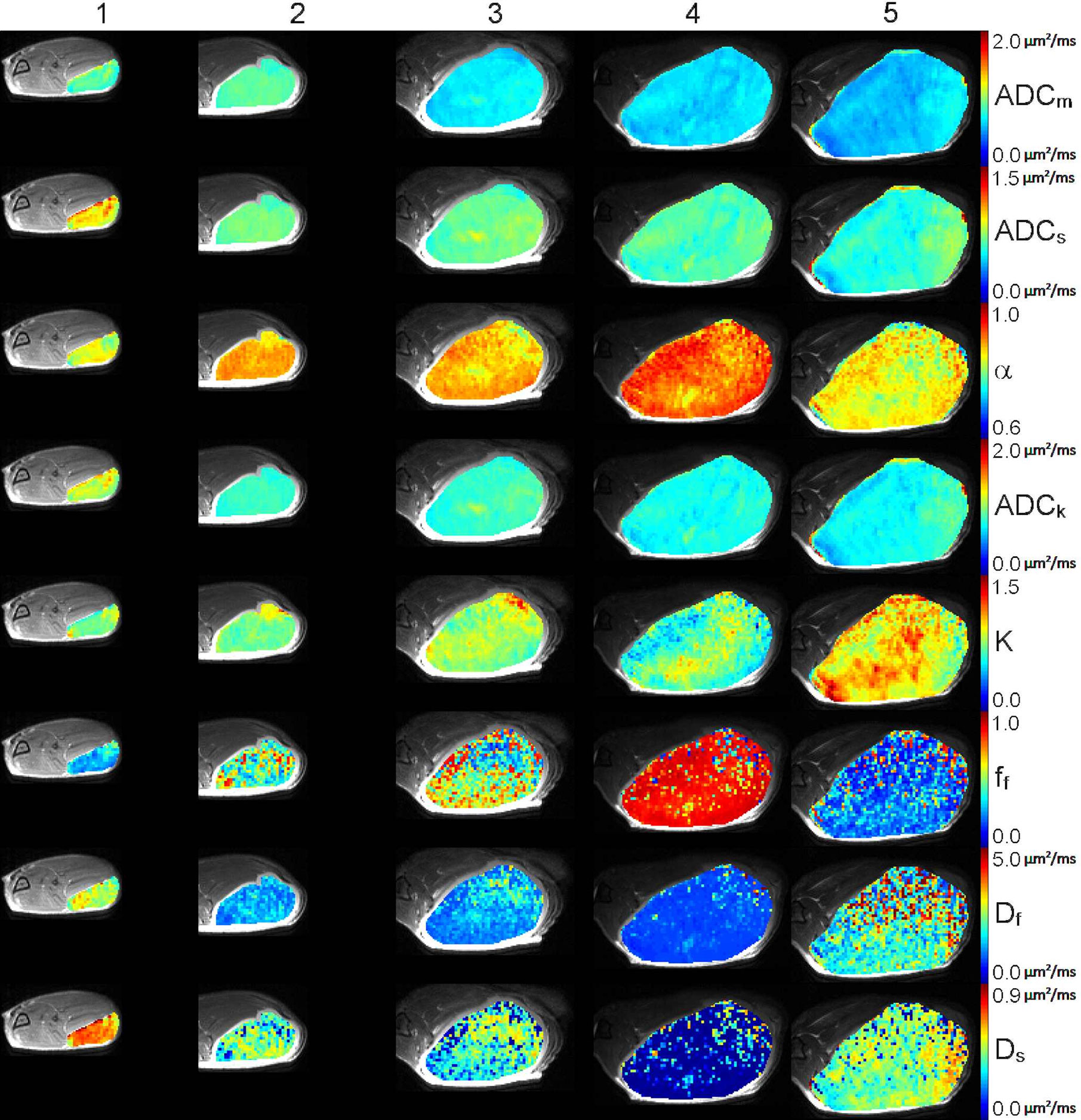
Figure 2 High b-value DWI data of a representative tumor: T2-weighted image fused with parametric maps for ADCm, ADCs, α, ADCk, K, ff, Df, and Ds parameters are shown, and represent different degree of tumor homogeneity between week 1 (column 1), week 2 (column 2), week 3 (column 3), week 4 (column 4). Furthermore, the second repetition done on week 4 is shown (column 5). The parametric maps are scaled as follows: ADCm (A1−5) min−max: 0−2.0 µm2/ms, ADCs (B1−5) min−max: 0−1.5 µm2/ms, α (C1-5) min−max: 0.6−1.0, ADCk (D1−5) min−max: 0−2.0 µm2/ms, K (E1−5), min−max: 0−1.5, ff (F1−5), min−max: 0−1.0, Df (G1−5) min−max: 0−5.0 µm2/ms, Ds (H1−5) min−max: 0−0.9 µm2/ms.
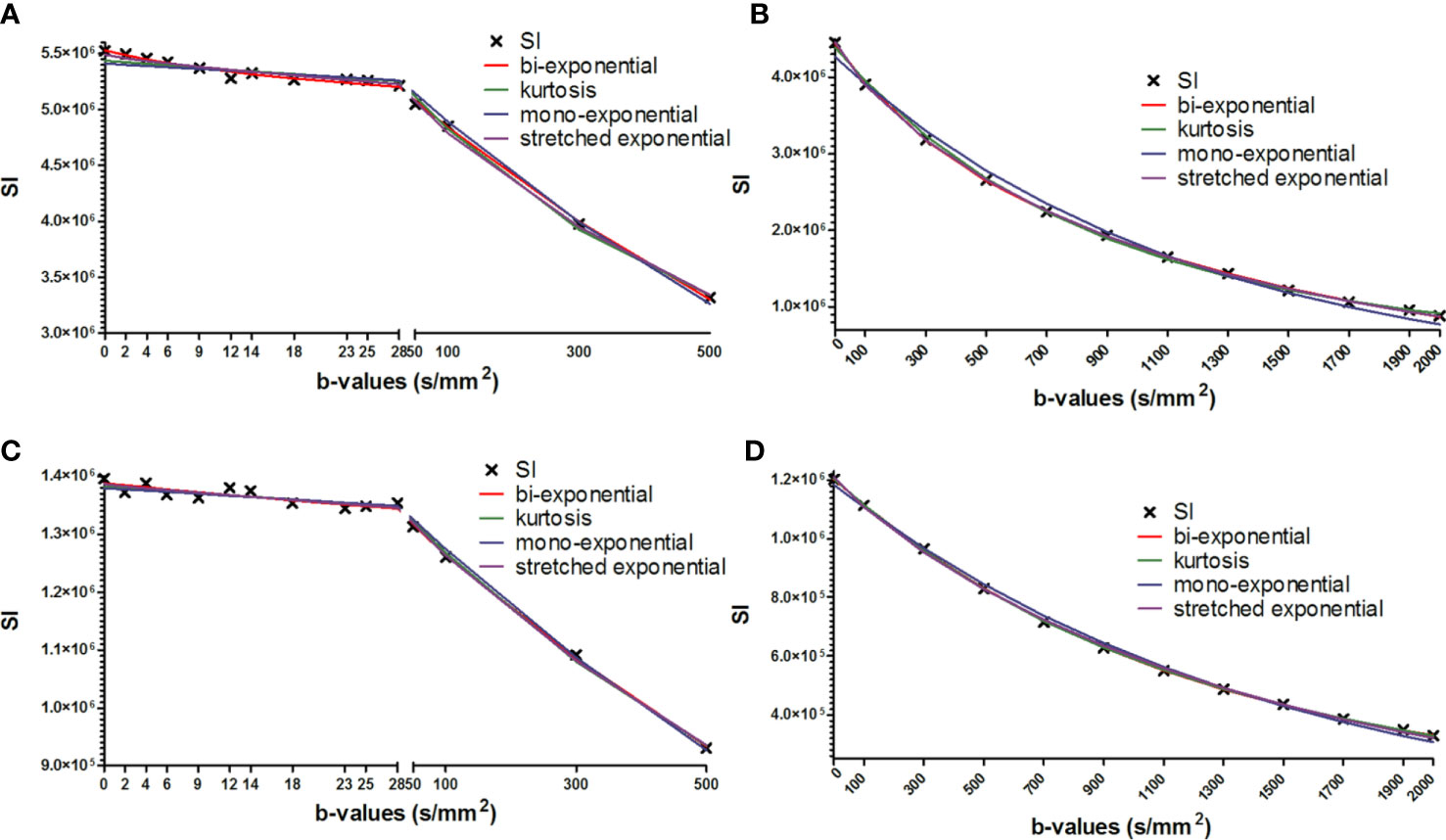
Figure 3 Mean signal intensity as a function of b-values (x-axis) fitted using all four models. The data is shown for the same representative tumor as shown in Figures 1 and 2. (A); week 1, low b-value DWI data. (B); week 1, high b-value DWI data. (C); week 4, low b-value DWI data. (D); week 4 high b-value DWI data. Bi-exponential, kurtosis and stretched exponential models provide better fit to the DWI decay curve than the mono-exponential model especially at high b-value DWI data.
Median values of the fitted parameters ADCm, ADCs, ADCk, and Ds between week 1 and those measured at weeks 2, 3 and 4 differed significantly while the differences between weeks 2, 3 and 4 were not significant using the low b-value data. Similarly, median values of K parameter increased significantly between week 1 and week 3 and 4. In contrast, no significant differences were present between median values of different weeks for α, Dp, and ff parameters (Figure 4). Using high b-values, significant changes were present in median values of ADCm, ADCs, α, and ADCk between week 1 and weeks 2, 3 and 4, while differences between week 2, 3 and 4 were not significant. The changes in K parameter were significant only between week 1 and week 4, while differences between week 1 and weeks 3 and 4 were significant for Df and Ds parameters (Figure 5).
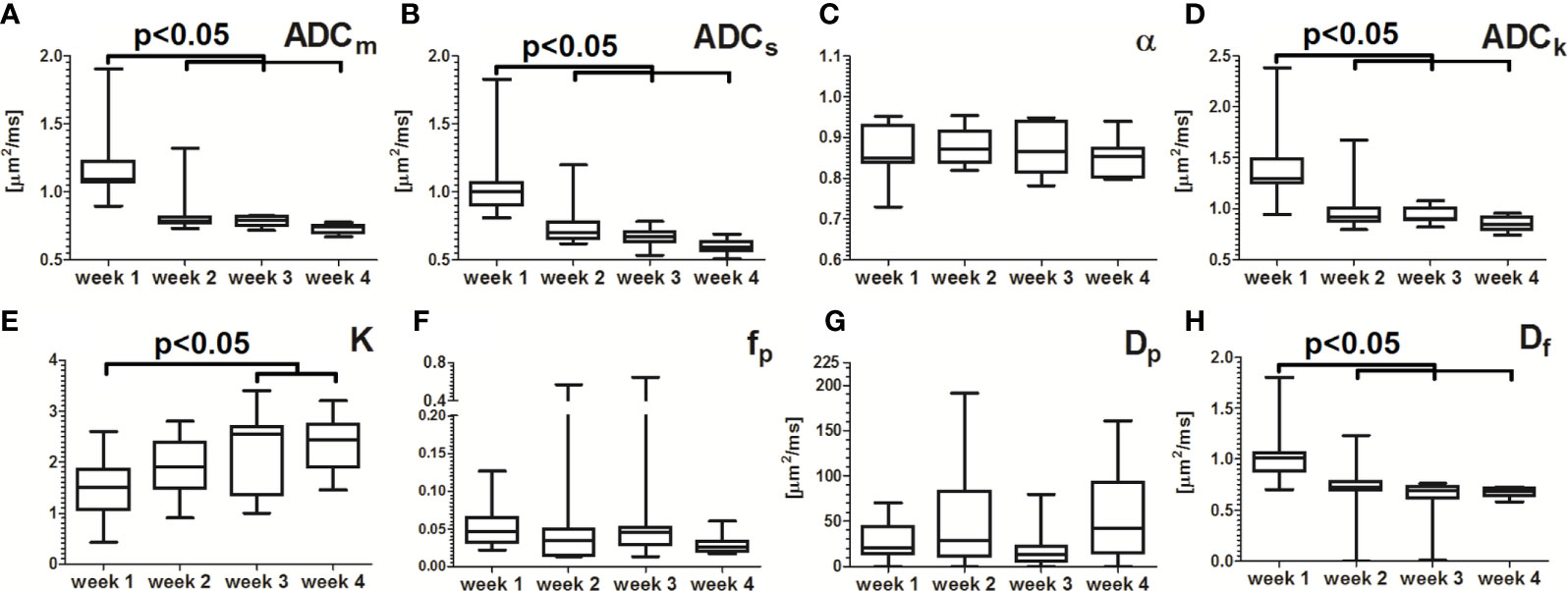
Figure 4 Median values of regions of interest (n=11) derived from DWI data obtained using low b-values. Significant changes (p<0.05) were present in ADCm (part A), ADCs (part B), ADCk (part D), and Df (part H) values between week 1 and those from the weeks 2, 3 and 4. K (part E) parameter differed significantly (p<0.05) between week 1 and weeks 3 and 4. The differences between weeks 2, 3 and 4 were not significant. The remaining differences in the fitted values (alpha, part C; Fp, part F; Dp part G) between weeks did not reach the level of statistical significance. The box extends from the 25th to 75th percentiles while the error bars extend from minimal to maximal values.
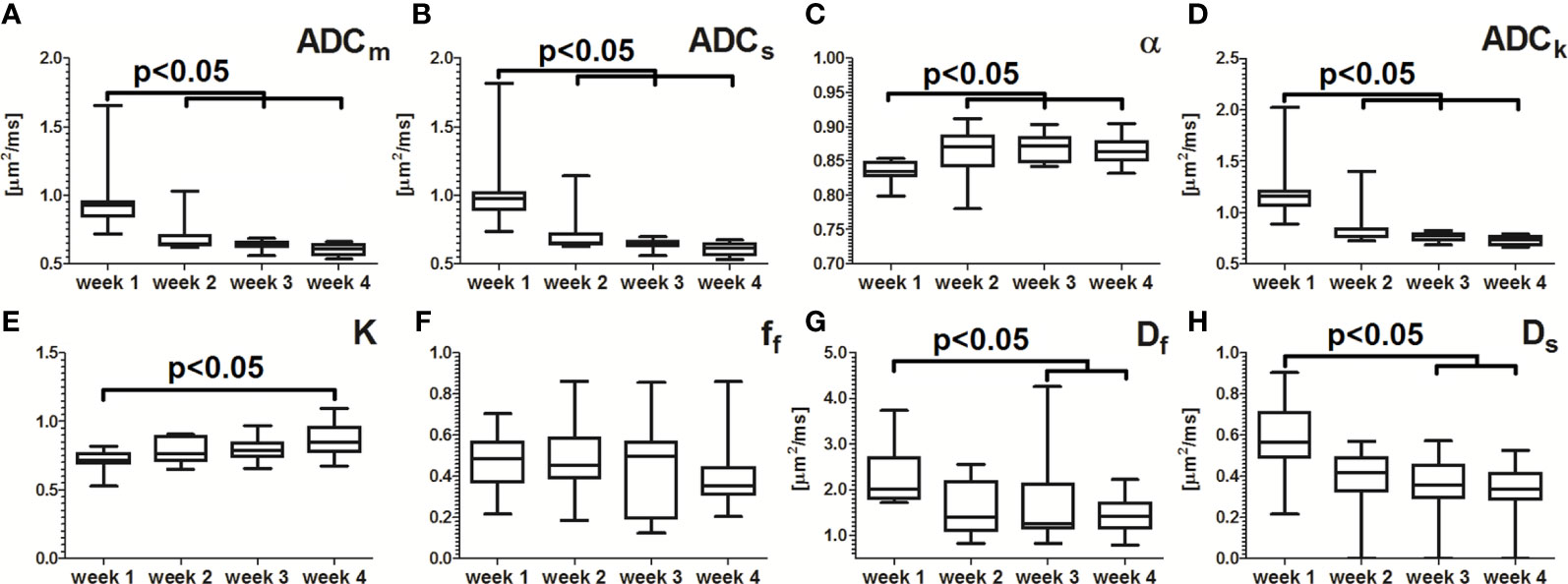
Figure 5 Median values of regions of interest (n=11) derived from DWI data obtained using high b-values. Significant changes (p<0.05) were present in ADCm (part A), ADCs (part B), alpha (part C), and ADCk (part D) values between week 1 and weeks 2, 3 and 4. K (part E) parameter differed significantly (p<0.05) between week 1 and week 4. The differences between weeks 2, 3 and 4 were not significant. Values of Df (part G) and Ds (part H) parameters differed significantly between week 1 and weeks 3 and 4. The remaining differences in the fitted values between weeks did not reach the level of statistical significance (Ff, part F). The box extends from the 25th to 75th percentiles while the error bars extend from minimal to maximal values.
Model Selection
In general, DWI data obtained using low b-values fitted better by the stretched exponential model as compared with mono-exponential, kurtosis and bi-exponential models based on AICc and F-ratio. In more than 50% of voxels the kurtosis model had lower AICc values than the mono-exponential model. However, the kurtosis model did not provide significantly better fit to data than the mono-exponential model in more than 50% of voxels (averaged medians of 11 mice) based on F-test. Similarly, the bi-exponential model was not preferred over mono-exponential in more than 50% of voxels (averaged medians of 11 mice). No dramatic changes in model preference were present between different time points (Tables 1 and 2).
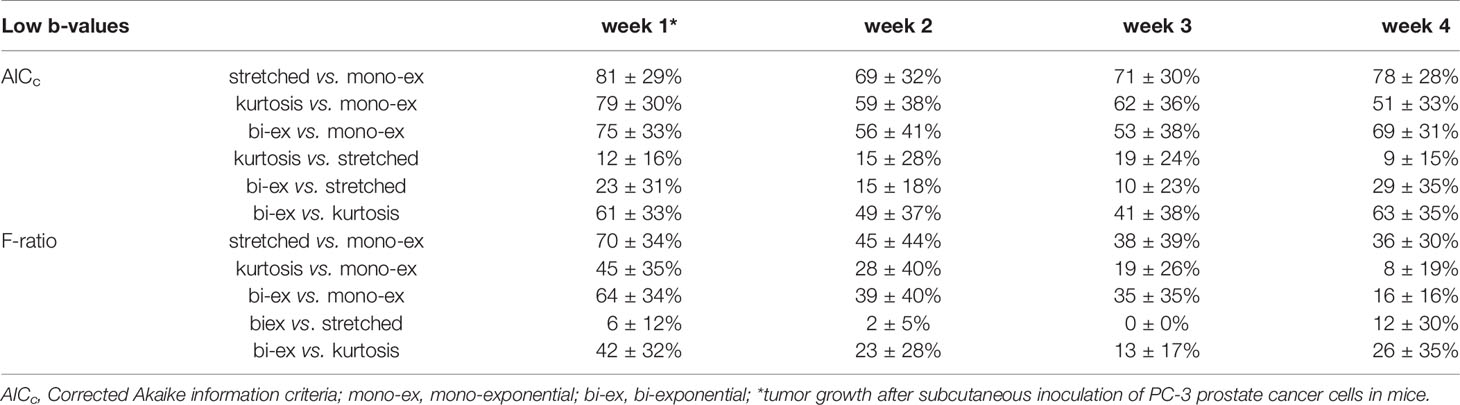
Table 1 Mean ± standard deviation of median percentage values per mouse described better by the first model of the comparison is shown in the table for DWI data obtained using low b-values.
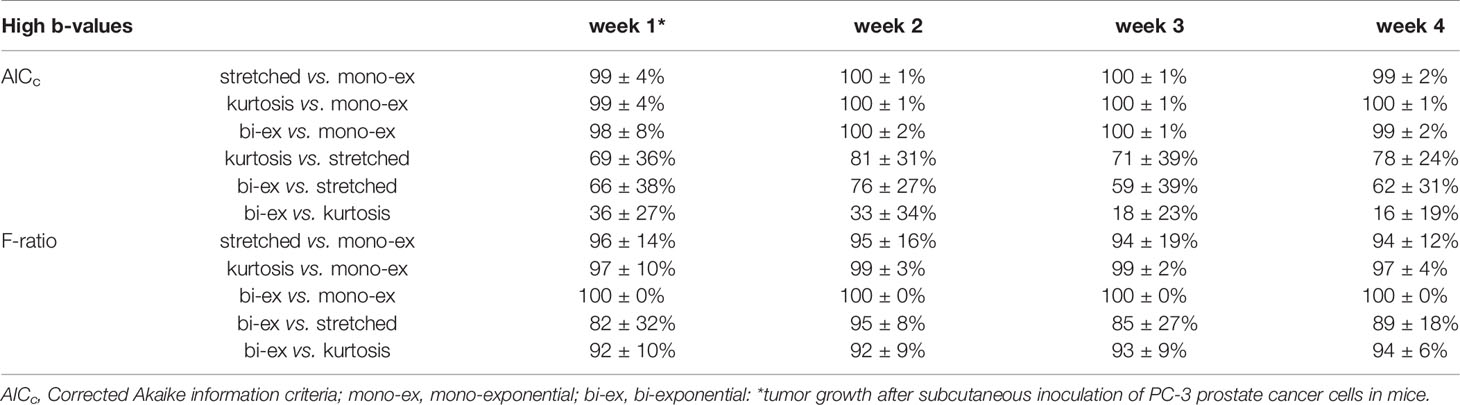
Table 2 Mean ± standard deviation of median percentage values per mouse described better by the first model of the comparison is shown in the table for DWI data obtained using high b-values.
In contrast to low b-values, in vast majority of voxels stretched exponential, kurtosis and bi-exponential models fitted DWI data obtained using high b-values better than the mono-exponential model based on AICc and F-test. The kurtosis model was preferred over the stretched exponential model in average in ~75% of voxels based on AICc. The bi-exponential models still provided significantly better fit to data than the stretched exponential and kurtosis models based on F-test.
Repeatability of the Fitted Parameters
The parameters of mono-exponential, stretched exponential and kurtosis models had confidence interval values smaller than 25% of the corresponding averaged median values (Table 3) for DWI data obtained using both low as well as high b-values. Similarly, coefficients of repeatability were smaller than 45% of the corresponding averaged median values (Table 3), with the exception of K parameter for low b-value DWI data (r% 59.7%). In contrast, the parameters of the bi-exponential model had much larger confidence interval and coefficient of repeatability values, especially for low b-value DWI data. Large confidence interval and coefficient of repeatability values for the parameters of bi-exponential model implicate poor measurement repeatability. Confidence interval and coefficient of repeatability values for mono-exponential, stretched exponential and kurtosis models we similar for DWI data acquired using low and high b-values. However, K parameter of kurtosis models had approximately 2-times higher relative coefficient of repeatability values than the ADCm, ADCs, ADCk parameters for low as well as high b-values.
Signal intensities at the second repeated DWI examination differed systematically from those measured at the first DWI examination, and the median values of ADCm, ADCs, and ADCk parameters were lower in the repeated DWI in all mice.
Discussion
The use of DWI for cancer detection, characterization and cancer therapy response monitoring continues to increase in both pre-clinical and clinical settings. Despite wide use of DWI, accurate and robust modeling of DWI signal decay remains a challenge. In the current study, we have evaluated four different mathematical models for DWI data (low and high b-values) of PC-3-cell derived human prostate cancer xenografts in mice, in terms of fitting quality and repeatability. Significant changes were observed in median values of ADC parameters detected between week 1 and those measured 1-3 weeks later, while the difference between the values obtained at weeks 2-4 were not significant. In previous studies cell density was shown to correlate with ADCm parameter (25, 26). In the light of these previous studies, our findings indicated lower cell density of the tumors at the first time point measured.
According to our finding, non-Gaussian DWI models provided better fit to DWI data than the most commonly applied mono-exponential model, which is in line with previous findings (6, 16, 21, 27). The use of high b-values and non-Gaussian DWI models for early therapy response evaluation has demonstrated promising results in human brain tumor (28), colon cancer mouse model (29), and glioma mouse model (30). In a study by Hoff and co-workers (30), fast diffusion component of the bi-exponential model had the largest percent change from baseline in glioma mouse model, suggesting a role of non-Gaussian DWI models for prediction of early therapy response.
Several recent pre-clinical (31–33) and clinical (34–36) studies demonstrated promising results for IVIM derived parameters especially in organs with high perfusion, such as liver or kidney. In these organs the intravoxel incoherent motion present with relatively larger contribution to signal decay. The perfusion fraction was shown to be in the range of > 20-30%, being substantially more than that observed in brain, for example (37). In the current study, the averaged median perfusion fraction value was 9%, thus, being similar to human brain. Due to relatively low contribution of the intravoxel incoherent motion fraction to the measured signal, it is questionable how accurately a least square fitting procedure performed independently for each voxel (in a presence of measurement and physiological noise) can evaluated such small exponential component of the bi-exponential model. Despite preventing local minima in the fitting procedure, the repeatability of IVIM derived parameters (bi-exponential model for low b-values) was low. Coefficient of repeatability (expressed in % of averaged median values) for fp, Dp and Df were 491.8%, 399.1% and 163.2%, respectively. Similar to our study, IVIM derived parameters using least square fitting have shown low reproducibility in human liver (38). The small contribution of “pseudodiffusion” component (fp, Dp) to the final fitting residuals during least square fitting procedure, calls into question the validity of IVIM parameters that are estimated using least square fitting procedure for organs with small “pseudodiffusion” component (39–41). Efforts to increase fitting robustness of the bi-exponential model resulted into wider use of “segmented analysis” (32, 42) where each exponential component is being fitted individually in subsequent fashion. However, it should be noted that the resulting fitting residual is likely to be higher for “segmented analysis” than for “simultaneous” least square fitting of the bi-exponential model. Information content and bias of “segmented analysis” in comparison with other mathematical models remains to be established. Orton and the co-workers (43) have proposed the use of a Bayesian approach for improved estimation accuracy of IVIM parameters. Bayesian approach shrinks the distribution of parameters and “moves” outliers closer to the central distribution. Despite very promising results (36), this approach might not be applicable for cases with limited number of fitting voxels with large physiological voxel heterogeneity. Bayesian approach is a balance between improving quality of parametric maps and suppressing heterogeneity. Other possible approach is the use of neighborhood information during the fitting procedure (44).
Deviation of the DWI signal from mono-exponential decay at low b-values is, according to IVIM theory (6), due to intravoxel incoherent motion associated with capillary perfusion. “Perfusion fraction” (fp in eq. 4) was proposed to reflex fractional volume (%) of capillary blood flow while “pseudodiffusion” (Dp in eq. 4) probably relates to blood velocity. Biological reasons for non-Gaussian DWI signal decay at high b-values remains open despite several proposed theories (7, 27, 45, 46). In the current study, the highest used b-value was 2000 s/mm2, which could have resulted in a less accurate estimation of slow diffusion component. Nevertheless, in vast majority of voxels non-Gaussian models provided better fit to DWI obtained using b-values up to 2000 s/mm2. As shown in prior studies (47, 48) of DWI models functions for PCa, that b-value distribution and DWI acquisition parameters may contribute to the fitting performance, and exploration of these factors is left for future studies.
Our study is limited by relatively small sample size. Furthermore, no attempts to histologically validate our findings with cell density have been made. Thus, further studies are needed to better investigate correlation of parameters derived from non-Gaussian DWI models with histopathological markers. Signal intensities differed systematically in the second DWI examination of the same tumor performed approximately 60 minutes after the first examination. This systematic bias in repeated DWI examinations has an effect on the estimation of repeatability. The confidence interval and coefficient of repeatability values between the repeated DWI examinations performed on week 4 are likely worse than those between weeks 1−4. Thus, the presented repeatability values should be regarded as the worst estimates due to systematic bias caused by DWI signal differences between the repeated DWI examinations. A potential cause of the bias is a temperature drop of the tumor (not mouse core temperature) in the second repeated DWI examination. Nevertheless, it is beyond the scope of the current study to fully explore an effect of temperature and anesthesia (26) on DWI decay curve derived parameters.
In conclusion, we have evaluated four different mathematical models for DWI of PC-3 prostate cancer cell derived xenografts in mice. Significant changes in the fitted parameters were present during tumor progression potentially due to increased cell density in later stages. The “pseudodiffusion” component in the analyzed tumors was shown to be less than 10% of the bi-exponential model. Due to low repeatability of the bi-exponential model parameters derived from low and high b-values DWI data using independent least square fitting on a voxel level, a degree of caution should be applied if these parameters are used for cancer characterization and therapy response monitoring. On the other hand, mono-exponential, stretched exponential, and kurtosis models shown high information content and robust repeatability.
Data Availability Statement
The original contributions presented in the study are included in the article/Supplementary Material. Further inquiries can be directed to the corresponding author.
Ethics Statement
The animal study was reviewed and approved by Turku Center for Disease Modeling, University of Turku, Turku, Finland.
Author Contributions
All authors listed have made a substantial, direct, and intellectual contribution to the work and approved it for publication. Specifically, Conceptualization: HaM, AR, TL, IJ. Data curation: All authors. Funding acquisition: HA, TL, IJ. Investigation: HaL, HeL, HV, MP, TL. Methodology: All authors. Project administration: AR, TL, IJ. Resources: AR, MP, TL, IJ. Software and statistical analyses: HaM, IJ. Supervision: TL, IJ. Validation: HaM, IJ, Visualization: HaM, IJ. Writing – original draft: HaM, IJ. Writing – review & editing: HaM, AR, TL, IJ.
Funding
This study was financially supported by grants Sigrid Jusélius Foundation (HaM, HA, and IJ), Finnish Cultural Foundation (HM and IJ), Finnish Cancer Society (IJ), Orion Research Foundation (HaM), Instrumentarium Research Foundation (IJ), Academy of Finland (AR and TL), Turku University Hospital (HA and IJ), TYKS-SAPA research fund (HA and IJ), Turku University Foundation (HaM and IJ), and University of Eastern Finland strategic funding (TL and HL).
Conflict of Interest
The authors declare that the research was conducted in the absence of any commercial or financial relationships that could be construed as a potential conflict of interest.
Supplementary Material
The Supplementary Material for this article can be found online at: https://www.frontiersin.org/articles/10.3389/fonc.2021.583921/full#supplementary-material
References
1. Moffat BA, Hall DE, Stojanovska J, McConville PJ, Moody JB, Chenevert TL, et al. Diffusion Imaging for Evaluation of Tumor Therapies in Preclinical Animal Models. MAGMA (2004) 17:249–59. doi: 10.1007/s10334-004-0079-z
2. Liimatainen T, Hakumaki JM, Kauppinen RA, Ala-Korpela M. Monitoring of Gliomas in Vivo by Diffusion MRI and (1)H MRS During Gene Therapy-Induced Apoptosis: Interrelationships Between Water Diffusion and Mobile Lipids. NMR Biomed (2009) 22:272–9. doi: 10.1002/nbm.1320
3. Padhani AR, Liu G, Koh DM, Chenevert TL, Thoeny HC, Takahara TT, et al. Diffusion-Weighted Magnetic Resonance Imaging as a Cancer Biomarker: Consensus and Recommendations. Neoplasia (2009) 11:102–25. doi: 10.1593/neo.81328
4. Padhani AR, Makris A, Gall P, Collins DJ, Tunariu N, de Bono JS. Therapy Monitoring of Skeletal Metastases With Whole-Body Diffusion MRI. J Magn Reson Imaging (2014) 39:1049–78. doi: 10.1002/jmri.24548
5. Bammer R. Basic Principles of Diffusion-Weighted Imaging. Eur J Radiol (2003) 45:169–84. doi: 10.1016/S0720-048X(02)00303-0
6. LeBihan D, Breton E, Lallemand D, Aubin ML, Vignaud J, Laval-Jeantet M. Separation of Diffusion and Perfusion in Intravoxel Incoherent Motion MR Imaging. Radiology (1988) 168:497–505. doi: 10.1148/radiology.168.2.3393671
7. LeBihan D. The ‘Wet Mind’: Water and Functional Neuroimaging. Phys Med Biol (2007) 52:R57–90. doi: 10.1088/0031-9155/52/7/R02
8. Lee HJ, Rha SY, Chung YE, Shim HS, Kim YJ, Hur J, et al. Tumor Perfusion-Rrelated Parameter of Diffusion-Weighted Magnetic Resonance Imaging: Correlation With Histological Microvessel Density. Magn Reson Med (2014) 71:1554–8. doi: 10.1002/mrm.24810
9. LeBihan D, Turner R. The Capillary Network: A Link Between IVIM and Classical Perfusion. Magn Reson Med (1992) 27:171–8. doi: 10.1002/mrm.1910270116
10. LeBihan D. Intravoxel Incoherent Motion Perfusion MR Imaging: A Wake-Up Call. Radiology (2008) 249:748–52. doi: 10.1148/radiol.2493081301
11. Niendorf T, Dijkhuizen RM, Norris DG, van Lookeren CM, Nicolay K. Biexponential Diffusion Attenuation in Various States of Brain Tissue: Implications for Diffusion-Weighted Imaging. Magn Reson Med (1996) 36:847–57. doi: 10.1002/mrm.1910360607
12. Bennett KM, Schmainda KM, Bennett RT, Rowe DB, Lu H, Hyde JS. Characterization of Continuously Distributed Cortical Water Diffusion Rates with a Stretched-Exponential Model. Magn Reson Med (2003) 50:727–34. doi: 10.1002/mrm.10581
13. Clark CA, Le BD. Water Diffusion Compartmentation and Anisotropy at High B Values in the Human Brain. Magn Reson Med (2000) 44:852–9. doi: 10.1002/1522-2594(200012)44:6<852::AID-MRM5>3.0.CO;2-A
14. Yablonskiy DA, Bretthorst GL, Ackerman JJ. Statistical Model for Diffusion Attenuated MR Signal. Magn Reson Med (2003) 50:664–9. doi: 10.1002/mrm.10578
15. Schuster DM, Votaw JR, Nieh PT, Weiping W, Jonathon JA, Viraj VM, et al. Initial Experience with the Radiotracer Anti-1-Amino-3-18F-Fluorocyclobutane-1-Carboxylic Acid with PET/CT in Prostate Carcinoma. J Nucl Med (2007) 48:56–63.
16. Bourne RM, Panagiotaki E, Bongers A, Sved P, Watson G, Alexander DC. Information Theoretic Ranking of Four Models of Diffusion Attenuation in Fresh and Fixed Prostate Tissue Ex Vivo. Magn Reson Med (2014) 72:1418–26. doi: 10.1002/mrm.25032
17. Wittsack HJ, Lanzman RS, Mathys C, Janssen H, Modder U, Blondin D. Statistical Evaluation of Diffusion-Weighted Imaging of the Human Kidney. Magn Reson Med (2010) 64:616–22. doi: 10.1002/mrm.22436
18. Boxenbaum HG, Riegelman S, Elashoff RM. Statistical Estimations in Pharmacokinetics. J Pharmacokinet Biopharm (1974) 2:123–48. doi: 10.1007/BF01061504
19. Ludden TM, Beal SL, Sheiner LB. Comparison of the Akaike Information Criterion, the Schwarz Criterion and the F Test as Guides to Model Selection. J Pharmacokinet Biopharm (1994) 22:431–45. doi: 10.1007/BF02353864
20. Kopf M, Corinth C, Haferkamp O, Nonnenmacher TF. Anomalous Diffusion of Water in Biological Tissues. Biophys J (1996) 70:2950–8. doi: 10.1016/S0006-3495(96)79865-X
21. Jensen JH, Helpern JA, Ramani A, Lu H, Kaczynski K. Diffusional Kurtosis Imaging: The Quantification of Non-Gaussian Water Diffusion by Means of Magnetic Resonance Imaging. Magn Reson Med (2005) 53:1432–40. doi: 10.1002/mrm.20508
22. Jensen RT, Battey JF, Spindel ER, Benya RV. International Union of Pharmacology. LXVIII. Mammalian Bombesin Receptors: Nomenclature, Distribution, Pharmacology, Signaling, and Functions in Normal and Disease States. Pharmacol Rev (2008) 60:1–42. doi: 10.1124/pr.107.07108
23. Cescato R, Maina T, Nock B, Nikolopoulou A, Charalambidis D, Piccand V, et al. Bombesin Receptor Antagonists may be Preferable to Agonists for Tumor targeting. J Nucl Med (2008) 49:318–26. doi: 10.2967/jnumed.107.045054
24. Koh DM, Blackledge M, Collins DJ, Padhani AR, Wallace T, Wilton B, et al. Reproducibility and Changes in the Apparent Diffusion Coefficients of Solid Tumours Treated With Combretastatin A4 Phosphate and Bevacizumab in a Two-Centre Phase I Clinical Trial. Eur Radiol (2009) 19:2728–38. doi: 10.1007/s00330-009-1469-4
25. Zelhof B, Pickles M, Liney G, Gibbs P, Rodrigues G, Kraus S, et al. Correlation of Diffusion-Weighted Magnetic Resonance Data with Cellularity in Prostate Cancer. BJU Int (2009) 103:883–8. doi: 10.1111/j.1464-410X.2008.08130.x
26. Valette J, Guillermier M, Besret L, Hantraye P, Bloch G, Lebon V. Isoflurane Strongly Affects The Diffusion of Intracellular Metabolites, as Shown by 1H Nuclear Magnetic Resonance Spectroscopy of the Monkey Brain. J Cereb Blood Flow Metab (2007) 27:588–96. doi: 10.1148/radiol.10091343
27. Mulkern RV, Gudbjartsson H, Westin CF, Zengingonul HP, Gartner W, Guttmann CR, et al. Multi-Component Apparent Diffusion Coefficients in Human Brain. NMR Biomed (1999) 12:51–62. doi: 10.1002/(SICI)1099-1492(199902)12:1<51::AID-NBM546>3.0.CO;2-E
28. Mardor Y, Pfeffer R, Spiegelmann R, Roth Y, Maier SE, Nissim O, et al. Early Detection of Response to Radiation Therapy in Patients with Brain Malignancies Using Conventional and High B-Value Diffusion-Weighted Magnetic Resonance imaging. J Clin Oncol (2003) 21:1094–100. doi: 10.1200/JCO.2003.05.069
29. Roth Y, Tichler T, Kostenich G, Ruiz-Cabello J, Maier SE, Cohen JS, et al. High-B-Value Diffusion-Weighted MR Imaging for Pretreatment Prediction and Early Monitoring of Tumor Response to Therapy in Mice. Radiology (2004) 232:685–92. doi: 10.1148/radiol.2322030778
30. Hoff BA, Chenevert TL, Bhojani MS, Kwee TC, Rehemtulla A, Le Bihan D, et al. Assessment of Multiexponential Diffusion Features as MRI Cancer Therapy Response Metrics. Magn Reson Med (2010) 64:1499–509. doi: 10.1002/mrm.22507
31. Zhang Y, Jin N, Deng J, Guo Y, White SB, Yang GY, et al. Intra-Voxel Incoherent Motion MRI in Rodent Model of Diethylnitrosamine-Induced Liver Fibrosis. Magn Reson Imaging (2013) 31:1017–21. doi: 10.1016/j.mri.2013.03.007
32. Kim S, Decarlo L, Cho GY, Jensen JH, Sodickson DK, Moy L, et al. Interstitial Fluid Pressure Correlates with Intravoxel Incoherent Motion Imaging Metrics in a Mouse Mammary Carcinoma Model. NMR Biomed (2012) 25:787–94. doi: 10.1002/nbm.1793
33. Chow AM, Gao DS, Fan SJ, Qiao Z, Lee FY, Yang J, et al. Liver Fibrosis: An Intravoxel Incoherent Motion (IVIM) Study. J Magn Reson Imaging (2012) 36:159–67. doi: 10.1002/jmri.23607
34. Penner AH, Sprinkart AM, Kukuk GM, Gütgemann I, Gieseke J, Schild HH, et al. Intravoxel Incoherent Motion Model-Based Liver Lesion Characterisation From Three B-Value Diffusion-Weighted MRI. Eur Radiol (2013) 23:2773–83. doi: 10.1007/s00330-013-2869-z
35. Jerome NP, Orton MR, d’Arcy JA, Collins DJ, Koh DM, Leach MO. Comparison of Free-Breathing with Navigator-Controlled Acquisition Regimes in Abdominal Diffusion-Weighted Magnetic Resonance Images: Effect on ADC and IVIM Statistics. J Magn Reson Imaging (2014) 39:235–40. doi: 10.1002/jmri.24140
36. Dyvorne HA, Galea N, Nevers T, Fiel MI, Carpenter D, Wong E, et al. Diffusion-Weighted Imaging of the Liver with Multiple B Values: Effect of Diffusion Gradient Polarity and Breathing Acquisition on Image Quality and Intravoxel Incoherent Motion Parameters–A Pilot Study. Radiology (2013) 266:920–9. doi: 10.1148/radiol.12120686
37. Pekar J, Moonen CT, van Zijl PC. On the Precision of Diffusion/Perfusion Imaging by Gradient Sensitization. Magn Reson Med (1992) 23:122–9. doi: 10.1002/mrm.1910230113
38. Andreou A, Koh DM, Collins DJ, Blackledge M, Wallace T, Leach MO, et al. Measurement Reproducibility of Perfusion Fraction and Pseudodiffusion Coefficient Derived by Intravoxel Incoherent Motion Diffusion-Weighted MR Imaging in Normal Liver and Metastases. Eur Radiol (2013) 23:428–34. doi: 10.1007/s00330-012-2604-1
39. Federau C, Maeder P, O’Brien K, Browaeys P, Meuli R, Hagmann P. Quantitative Measurement of Brain Perfusion with Intravoxel Incoherent Motion MR Imaging. Radiology (2012) 265:874–81. doi: 10.1148/radiol.12120584
40. Federau C, Meuli R, O’Brien K, Maeder P, Hagmann P. Perfusion Measurement in Brain Gliomas with Intravoxel Incoherent Motion MRI. AJNR Am J Neuroradiol (2014) 35:256–62. doi: 10.3174/ajnr.A3686
41. Bisdas S, Koh TS, Roder C, Braun C, Schittenhelm J, Ernemann U, et al. Intravoxel Incoherent Motion Diffusion-Weighted MR Imaging of Gliomas: Feasibility of the Method and Initial Results. Neuroradiology (2013) 55:1189–96. doi: 10.1007/s00234-013-1229-7
42. Luciani A, Vignaud A, Cavet M, Van Nhieu JT, Mallat A, Ruel L, et al. Liver Cirrhosis: Intravoxel Incoherent Motion MR Imaging–Pilot Study. Radiology (2008) 249:891–9. doi: 10.1148/radiol.2493080080
43. Orton MR, Collins DJ, Koh DM, Leach MO. Improved intravoxel incoherent Motion Analysis of Diffusion Weighted Imaging by Data Driven Bayesian Modeling. Magn Reson Med (2014) 71:411–20. doi: 10.1002/mrm.24649
44. Freiman M, Perez-Rossello JM, Callahan MJ, Voss SD, Ecklund K, Mulkern R, et al. Reliable Estimation of Incoherent Motion Parametric Maps from Diffusion-Weighted MRI Using Fusion Bootstrap Moves. Med Image Anal (2013) 17:325–36. doi: 10.1016/j.media.2012.12.001
45. Schwarcz A, Bogner P, Meric P, Correze JL, Berente Z, Pál J, et al. The Existence of Biexponential Signal Decay in Magnetic Resonance Diffusion-Weighted Imaging Appears to be Independent of Compartmentalization. Magn Reson Med (2004) 51:278–85. doi: 10.1002/mrm.10702
46. Ababneh Z, Beloeil H, Berde CB, Gambarota G, Maier SE, Mulkern RV. Biexponential Parameterization of Diffusion and T2 Relaxation Decay Curves in a Rat Muscle Edema Model: Decay Curve Components and Water Compartments. Magn Reson Med (2005) 54:524–31. doi: 10.1002/mrm.20610
47. Meisaari H, Toivonen J, Pesola M, Taimen P, Boström PJ, Pahikkala T, et al. Diffusion-Weighted Imaging of Prostate Cancer: Effect of B-Value Distribution on Repeatability and Cancer Characterization. Magn Reson Imaging (2015) 33:1212–8. doi: 10.1016/j.mri.2015.07.004
Keywords: diffusion weighted imaging, PC-3 xenograft prostate tumors, prostate cancer mouse model, repeatability, Akaike information criteria (AIC), F-ratio
Citation: Merisaari H, Laakso H, Liljenbäck H, Virtanen H, Aronen HJ, Minn H, Poutanen M, Roivainen A, Liimatainen T and Jambor I (2021) Statistical Evaluation of Different Mathematical Models for Diffusion Weighted Imaging of Prostate Cancer Xenografts in Mice. Front. Oncol. 11:583921. doi: 10.3389/fonc.2021.583921
Received: 22 July 2020; Accepted: 23 March 2021;
Published: 26 May 2021.
Edited by:
Concetto Spampinato, University of Catania, ItalyReviewed by:
Chris Albanese, Georgetown University, United StatesCarmelo Pino, University of Catania, Italy
Copyright © 2021 Merisaari, Laakso, Liljenbäck, Virtanen, Aronen, Minn, Poutanen, Roivainen, Liimatainen and Jambor. This is an open-access article distributed under the terms of the Creative Commons Attribution License (CC BY). The use, distribution or reproduction in other forums is permitted, provided the original author(s) and the copyright owner(s) are credited and that the original publication in this journal is cited, in accordance with accepted academic practice. No use, distribution or reproduction is permitted which does not comply with these terms.
*Correspondence: Ivan Jambor, aXZqYW1iQHV0dS5maQ==