- Department of Radiation Oncology, The First Affiliated Hospital of Guangxi Medical University, Nanning, China
Pelvic intensity-modulated radiation therapy (IMRT) combined with concurrent chemotherapy is an effective treatment for cervical cancer; however, radiation resistance impairs its clinical benefit. The vaginal microbiome plays an important but poorly understood role in cancer radiochemotherapy. In this study, we investigated the effects of treatment on the overall composition and alteration of the vaginal microbiome in patients receiving pelvic IMRT with concurrent cisplatin-based chemotherapy. We collected samples from twenty patients with cervical cancer and six healthy controls and performed 16S rRNA sequencing. Vaginal microbial composition analysis revealed significant differences between the two groups, but no significant differences between radiation treatment time points. However, the relative abundances of Gammaproteobacteria, Gemmatimonadetes, Gemmatimonadales, Pseudomonadales, Gemmatimonadaceae, Rikenellaceae, Acinetobacter, Desulfovibrio, Prevotella 9, Rikenellaceae RC9 gut group, Turicibacter, and the metagenome increased with time. The results encourage further study into the effects of the vaginal microbiome on cervical cancer treatment strategies, especially radiochemotherapy. Better understanding of these effects could inform new therapeutic approaches to enhance the efficacy of radiochemotherapy.
Introduction
Cervical cancer, the most common gynecological malignancy, occurs in the epithelial lining of the cervix (1). For patients with locally advanced cervical cancer, radiotherapy combined with chemotherapy has become the mainstream treatment, usually involving intensity-modulated radiation therapy (IMRT) (2, 3). Although IMRT provides high dose conformity and spares organs at risk, resistance to treatment is an obstacle for patients with cervical cancer (4). Therefore, strategies to enhance the effects of radiation and chemotherapy are required to obtain better clinical outcomes.
The human microbiome is the collection of microorganisms that inhabit the mucosal surfaces of the body, including the vagina (5). In recent years, sequencing technology has made great progress in cataloguing these populations, with 16S ribosomal RNA (rRNA) sequencing most commonly used. Changes in the vaginal microbiome (VM) are associated with cervical cancer development (6–8), and evidence is rapidly mounting that it can affect cancer treatment outcomes through diverse mechanisms (9, 10). The VM can therefore be considered a novel target to improve the treatment sensitivity of cervical cancer. However, the role of the VM in patients with cervical cancer who receive pelvic IMRT combined with chemotherapy is not well understood. To assess the effects of pelvic radiochemotherapy on the VM, we compared the VM profiles of patients with cervical cancer and healthy controls, and then tracked the changes to the VM in patients with cervical cancer during pelvic IMRT combined with concurrent cisplatin-based chemotherapy by bacterial 16S rRNA gene sequencing.
Methods
Patients and Treatment
A prospective study was conducted by enrolling 20 patients with cervical cancer who received radical radiochemotherapy at the First Affiliated Hospital of Guangxi Medical University from April 2016 to May 2017. The inclusion criteria were: 1) patients were scheduled for pelvic IMRT at a dose delivered to a planning clinical target volume (PCTV) of 50 Gy in combination with concomitant cisplatin-based chemotherapy, 2) availability for vaginal sampling using a sterile swab stick, and 3) willingness to participate. The exclusion criteria were: 1) recent treatment with antibiotics, steroids, or immune-suppressants, 2) distant metastasis, and 3) previous pelvic radiotherapy for another tumor or with palliative intent. In addition, six healthy individuals were also enrolled from April 2016 to May 2017. Their inclusion criteria were 1) no history of malignancy, 2) a Karnofsky performance status ≥ 90, and 3) willingness to undergo vaginal sampling using a sterile swab stick. Written informed consent was obtained from all subjects, and the research protocol was approved by the Ethical Review Committee of the First Affiliated Hospital of Guangxi Medical University. Information that can be used to identify individual participants during or after the data collection is available and can be accessed. We confirm that all methods were performed in accordance with relevant guidelines and regulations.
All patients underwent a contrast-enhanced CT scan in the supine position with an immobilization device. The images datasets were imported to the treatment planning system (TPS). The gross tumor volume (GTV) and clinical target volume (CTV) was determined by CT and MRI. The CTV of the tumor bed (CTV-T) included the range of 10 mm from GTV as well as the entire uterus, cervix, parametria, and at least 3 cm proximal of the vagina. The nodal CTV (CTV-N) was delineated to include bilateral iliac, obturator, and presacral lymphatic drainage region with an expansion of the blood vessels by 7 mm. The planning clinical target volume (PCTV) was generated by a uniform expansion of 10 mm from CTV-T and 7 mm from CTV-N. The PCTV was prescribed such that > 95% was received at ≥ 50 Gy in 25 fractions, five times weekly for 5 weeks. The pelvic IMRT plan was generated using seven-field beam. In addition, all patients received weekly brachytherapy at a dose of 30 to 35 Gy following the pelvic IMRT. The constraints for organs at risk (OARs) were defined according to the institutional guidelines. Concurrent cisplatin-based chemotherapy was adopted in conjunction with IMRT as part of the treatment protocol. The concurrent chemotherapy regimen comprised the administration of cisplatin alone (80–100 mg/m2) every 3 weeks for two cycles.
Sampling and DNA Extraction
A sterile swab stick was used to obtain a specimen from the cervical lesion using aseptic technique. When possible, four sequential samples were collected based on radiation treatment time points: before starting pelvic radiotherapy (the first time point sample, T1), after the fifth radiotherapy session (the second time point sample, T2), after the 15th radiotherapy session (the third time point sample, T3), and after the 25th radiotherapy session (the fourth time point sample, T4). T1 samples were obtained 1 week before starting radiotherapy. The swab tops were placed in 2-ml sterile DNAase/RNase-free cryovials containing phosphate-buffered saline (400 μl), and stored at −80°C until further processing. A cell lysis procedure including enzymatic lysis and bead beating was used prior to DNA extraction using a QIAamp DNA Mini Kit (Qiagen, Hilden, Germany) and amplification by polymerase chain reaction (PCR).
Library Preparation and Sequencing
In total, 71 vaginal swabs with sufficiently high-quality DNA were collected. The V3-V4 hypervariable regions of the 16S rRNA gene were amplified with the primers 338F (5′-ACTCCTACGGGAGGCAGCA-3′) and 806R (5′-GGACTACHVGGGTWTCTAAT-3′) on a 2720 Thermal Cycler (Applied Biosystems, USA) (11, 12). PCR was conducted using the following program: 2 min at 98°C, then 20 cycles of 30 s at 98°C, 30 s at 50°C, and 1 min at 72°C, followed by a final incubation at 72°C for 5 min. Reactions were performed in triplicate. The reaction mix (50 μl total) consisted of 2×TransStart FastPfu Fly PCR SuperMix (25 μl), each primer (1 μl of 10 μM), nuclease-free water (20 μl), and template DNA (10 ng). The resultant PCR products were purified using VAHTS DNA Clean Beads. Secondary PCR was performed under different conditions using special index primers. The PCR program was: 30 s at 98°C, then 10 cycles of 10 s at 98°C, 30 s at 65°C, and 30 s at 72°C, then a final 5 min incubation at 72°C. PCR reactions were performed in triplicate. The reaction mix (50 μl total) consisted of Phusion DNA Polymerase (25 μl), i5/i7 index primers (1 μl of 2.5 μM), UltraPure water (13 μl), and purified PCR product (10 μl). Reactions were analyzed on 1.8% agarose gels to ensure successful amplification. Unsuccessful reactions were repeated after a 10× dilution of the initial template concentration, and removed from the experiment if unsuccessful again. PCR products were extracted from the agarose gels, further purified using an AxyPrep DNA Gel Extraction Kit (Axygen Biosciences, USA), and eluted with Tris-HCl. ImageJ (National Institutes of Health, Bethesda, MD, USA) was used to quantify the electrophoresis results. The library was pooled at equimolar concentrations and resolved on a 1.8% agarose gel, and the 600 bp band was extracted. The purified library was paired-end sequenced (2 × 250) on an Illumina HiSeq platform (Illumina, San Diego, CA, USA) according to the manufacturer’s instructions.
Bioinformatic Analysis
FLASH (1.2.11) was used to merge paired end reads with a minimum overlap of 10 bp (13). Primer and barcode sequences were trimmed using cutadapt (v1.18) (14); and chimeric sequences were detected and removed with VSEARCH (v2.13.6) (15). The trimmed data were processed to form OTUs at 97% identity using VSEARCH, and a representative OTU was selected from each cluster (15, 16). Using the Silva_132 16S rRNA database as a reference, Ribosomal Database Project classifiers were used to assign taxonomic ranks to each OTU using Qiime (v1.9.1) (16, 17). The alpha-diversity and beta-diversity indices were calculated based on the rarefied OTU counts. Alpha-diversity was performed in Mothur (v1.38.1) (18, 19), and represents an analysis of the diversity in a single sample, reflected by parameters including the Sobs, Chao1, Ace, Shannon, and Simpson indices. The Wilcoxon rank-sum test was used to compare alpha-diversity indices. Beta-diversity is a measure of the microbiota structure between groups. Both weighted and unweighted UniFrac distance matrices were plotted in the PCA, and ANOSIMs were performed using the R package “ade4” (20). For taxa with a prevalence > 10%, differential abundance analysis was performed using the Wilcoxon rank-sum test at the phylum, class, order, family, and genus levels. For multiple comparisons of bacterial counts, the false discovery rate was calculated using the Benjamini-Hochberg method (21). Microorganism features used to distinguish gut microbiotas specific to cervical cancer were identified using the linear discriminant analysis effect size method, with an alpha cutoff of 0.05 and an effect size cutoff of 2.0 (22). Phylogenetic Investigation of Communities by Reconstruction of Unobserved States was used to predict the abundances of functional categories in Kyoto Encyclopedia of Genes and Genomes (KEGG) orthologs (23). Graphing of KEGG pathways at levels 2 (41 pathways) and 3 (328 pathways) was performed with STAMP, and p values were calculated using White’s non-parametric t-test (24).
Statistics
R software (ver. 3.5.1, the R Project for Statistical Computing) was used for statistical analysis. In descriptive analyses, the mean ± standard deviation (s.d.) was used for normally distributed continuous variables and the median ± interquartile range (IQR) was used for continuous variables with skewed distributions. Comparisons of the relative abundance of detected genera between groups were conducted using the Wilcoxon rank-sum test. The Sobs, ACE, Simpson, Shannon, and Chao1 indices were compared using Student’s t-test. P<0.05 was considered statistically significant.
Results
Patient Characteristics
We analyzed 71 vaginal swab samples from 20 patients with cervical cancer and six healthy controls. Table 1 shows the clinical characteristics of the patients with cervical cancer. Their median age was 54 years (range: 44–73). All patients received pelvic IMRT plus brachytherapy combined with cisplatin-based chemotherapy. Each radiation plan met the prescribed dose requirements, and the mean dose of the planning clinical target volume (PCTV) was calculated as 54 Gy. All 20 patients provided samples at T1, and 15/20 permitted the collection of samples at all four timepoints (T1–T4).
VM Diversity Estimations in Patients With Cervical Cancer and Healthy Controls
After quality control processes and the removal of chimeric sequences, we obtained 2,983 operational taxonomic units (OTUs) in total. Table S1 summarizes the numbers of unique sequences and OTUs in each normalized sample. Of these, 612 OTUs (20.5%) were detected in healthy controls, 2,500 (83.8%) were detected in patients with cervical cancer, and 483 OTUs (16.2%) were detected in both groups (Figure 1A). Abundance comparisons of individual OTUs through principal component analysis (PCA) revealed differences in the VM composition of healthy controls and patients with cervical cancer (Figure 1B). Furthermore, species diversity and richness were also higher in cervical cancer samples than in control samples. The Chao, Ace, Shannon, and Simpson indices (p = 0.016, = 0.002, <0.001, <0.003, and <0.006, respectively) are shown in Figure 1C and Table 2. Beta diversity analysis revealed statistically significant differences between the two groups (p = 0.005; weighted UniFrac and analysis of similarity (ANOSIM); Figure 1D).
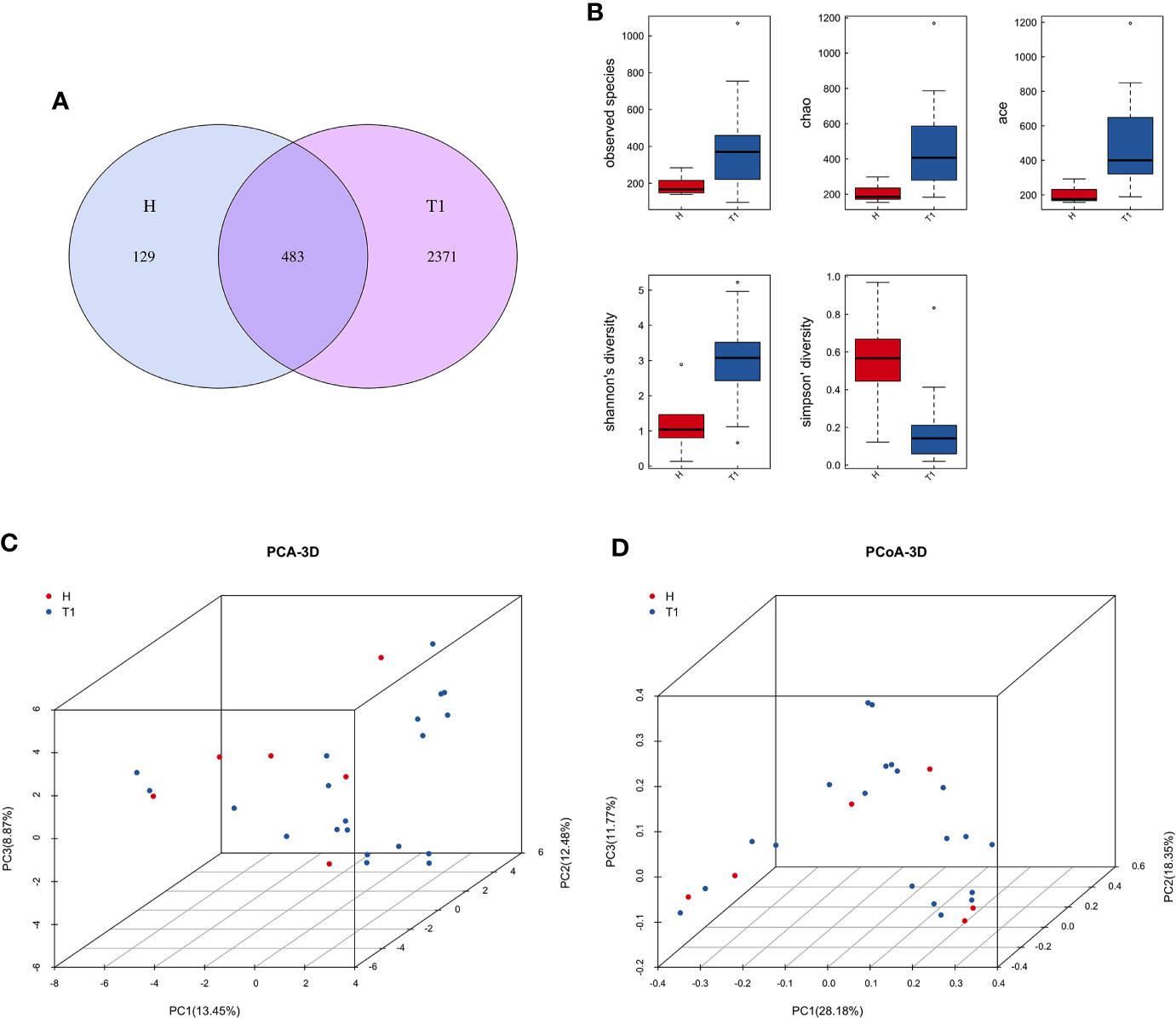
Figure 1 (A) Venn diagram for the integration between healthy controls and cervix cancer patients OTUs. (B) Principal component analysis (PCA) of vaginal microbiome of 20 patients and 6 healthy controls. (C) The comparison between patients and healthy controls by alpha diversity analysis. (D) The comparison between patients and healthy controls by beta diversity analysis. H, Healthy controls; T1, the first time point sample of patients.

Table 2 The comparison between patients with cervical cancer and healthy controls by alpha diversity analysis.
VM Diversity Estimations in Patients With Cervical Cancer During Treatment
To explore the effects of radiation on the VM during the treatment process, we first analyzed VM richness and diversity at four time points (T1–T4). In total, 6,109 OTUs were obtained, and 1,382 were common among all timepoints. Then, the bacterial compositions at each timepoint were compared using overlap analysis (Figure 2A) and 3D-PCA (Figure 2B). Interestingly, in comparisons of T1 vs T2, T3 vs T2, T4 vs T3 and T4 vs T1, the timepoints were not spatially distinct, nor were they significantly different in VM richness and diversity according to alpha (Figures 3A–D) and beta diversity analyses (data not shown).
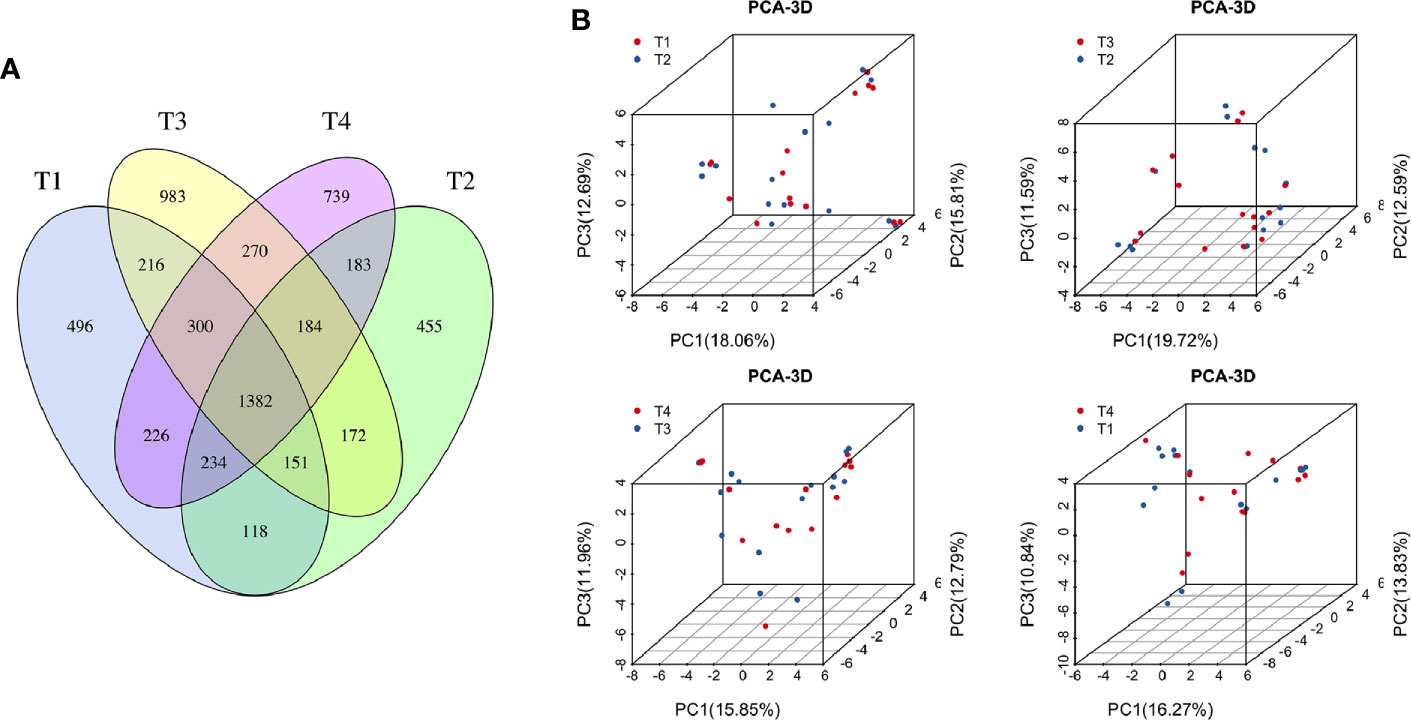
Figure 2 (A) Venn diagram for the integration among the four time points of patient samples. (B) 3D scatter plot of PCA results for comparison among four time points of patient samples (T1 vs T2, T3 vs T2, T4 vs T3, and T4 vs T1). T1, the first time point sample; T2, the second time point sample; T3, the third time point sample; T4, the fourth time point sample.
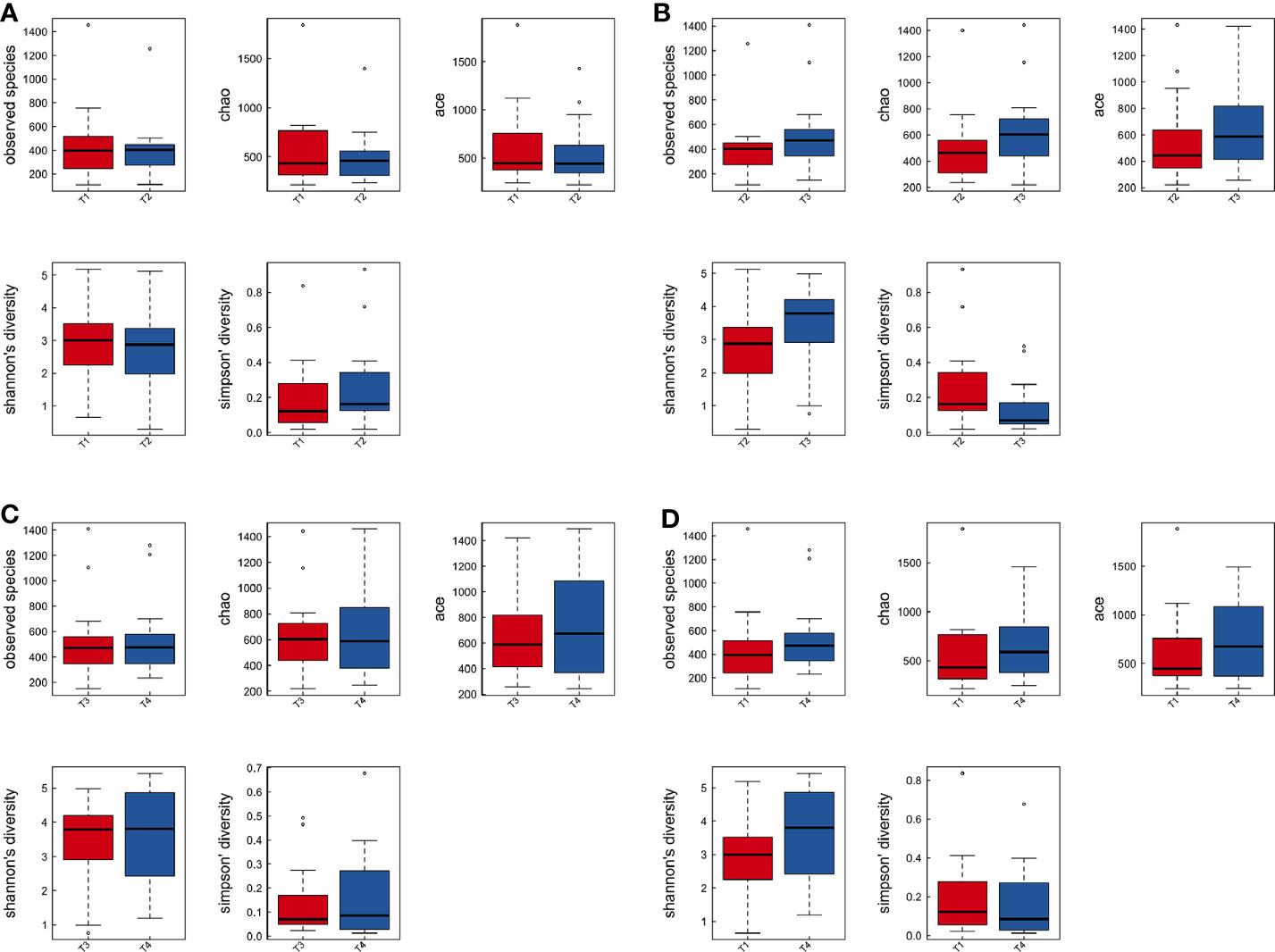
Figure 3 The comparison among the four time points of patient samples by alpha diversity analysis. Boxplots of alpha diversity in T1 vs T2 (A), T3 vs T2 (B), T4 vs T3 (C), and T4 vs T1 (D) using different metrics (observed OTUs, the Shannon index, and the Simpson index). T1, the first time point sample; T2, the second time point sample; T3, the third time point sample; T4, the fourth time point sample.
Impact of Radiation Therapy on the VM Composition of Patients With Cervical Cancer
Annotation analysis revealed the distribution of the microbiota at the phylum, class, order, family, genus, and species levels over time (Figures 4A–F). Figure 5 shows changes in the relative abundances of major phylum-level taxa during radiation therapy. The relative abundances of Gammaproteobacteria, Gemmatimonadetes, Gemmatimonadales, Pseudomonadales, Gemmatimonadaceae, Rikenellaceae, Acinetobacter, Desulfovibrio, Prevotella 9, the Rikenellaceae RC9 gut group, Turicibacter, and the metagenome increased with radiation time.
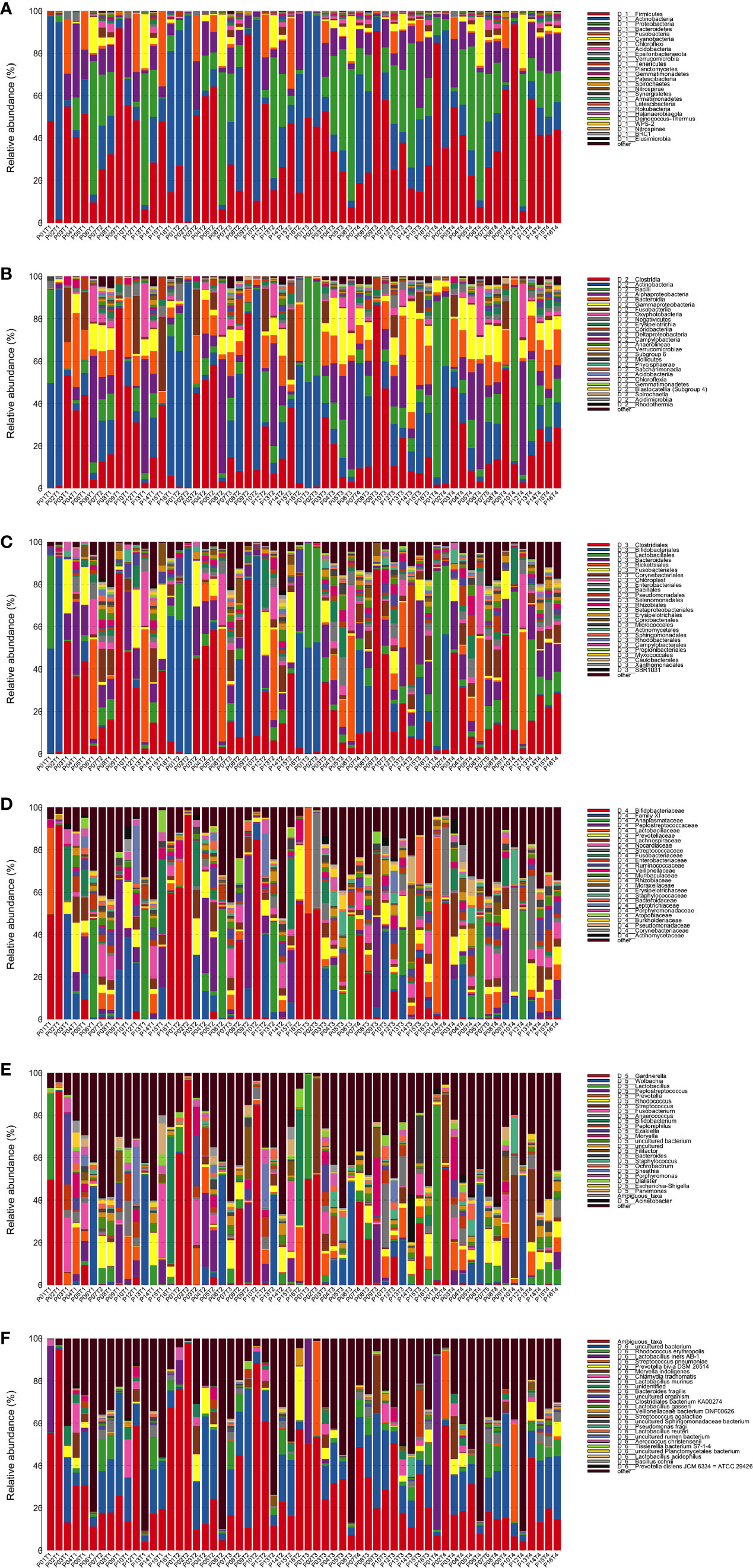
Figure 4 Distribution of bacterial phyla (A), classes (B), orders (C), families (D), genera (E), and species (F) obtained by next-generation sequencing of samples from 15 patients at four time points.
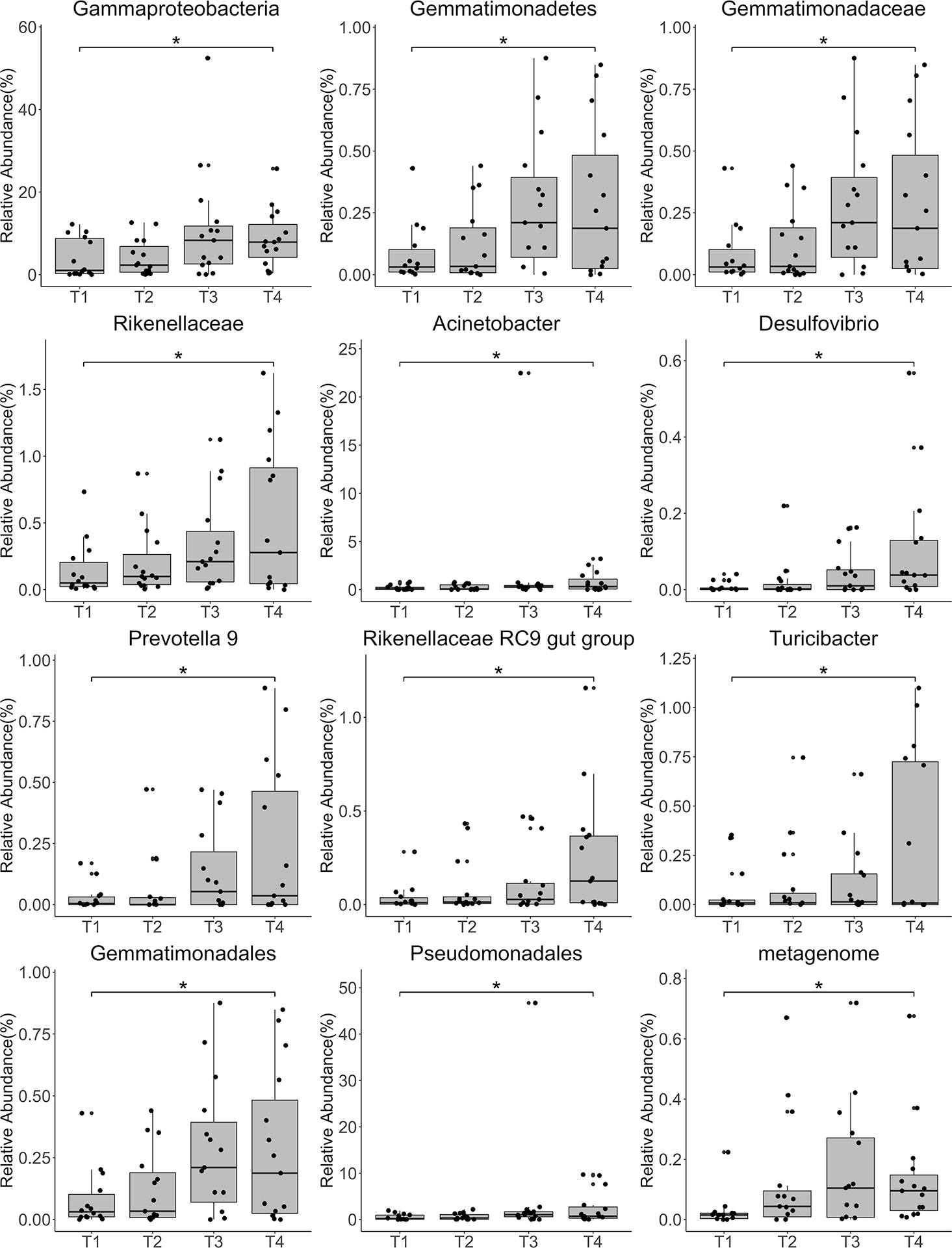
Figure 5 Relative abundances of the top 12 most abundant genera at each time point. Each bar represents the mean abundance ± the standard error of the mean. *P<0.05.
Discussion
Cervical cancer often results from persistent infection with human papillomavirus (HPV), which induces cervical epithelial cells to become cancerous (25). However, the process of cervical carcinogenesis can be affected by the VM (8), and increasing evidence indicates that VM changes play important roles in the process (26, 27). Conversely, cervical cancer disrupts the ratio between commensal and pathogenic microbiome species, resulting in microenvironmental changes (28). However, studies investigating the role of the VM in patients with cervical cancer who receive pelvic radiochemotherapy are scarce. The aim of our study was to examine associations between the use of pelvic radiochemotherapy and VM changes.
Currently, radiochemotherapy is a common treatment strategy for cervical cancer. The National Comprehensive Cancer Network guidelines (V1.2020) for cervical cancer recommend a dose of 45–50 Gy in standard fractionation with IMRT (29). In this study, all patients were prescribed a PCTV of 50 Gy. However, due to limitations in the radiation technique, the dose distribution in the target area is not absolutely uniform. To ensure quality radiation therapy, the patient received at least 50 Gy in 25 fractions, meaning that > 50 Gy was present in the PCTV. Dose data were recorded using a Varian Eclipse V8.0 treatment planning system. IMRT protects organs at risk better than two-dimensional radiation techniques; therefore, our results are based on pelvic IMRT combined with cisplatin-based concurrent chemotherapy.
In our study, there were more OTUs in patients with cervical cancer than in healthy controls, and the abundances of individual OTUs were significantly different between the two groups. When compared at different radiation treatment time points, there are no significant differences found in VM richness and diversity by alpha and beta diversity analysis. Nevertheless, changes in the relative abundances of several taxa were observed during radiation therapy. The dose distribution of radiation is closely associated to the volume and the location of tumor, which might affect distribution of vaginal microbiome during the radiation treatment. The research endpoint may be insufficient to prove the vaginal microbiome as a key clinical index for cervical cancer patients, so a larger cohort is needed to determine the correlation between VM and cervical cancer patients in future study.
It is well established that VM imbalance is strongly correlated with cervical cancer. Vaginal dysbiosis (characterized by a non-Lactobacillus-dominant composition) and inflammation have been associated with HPV persistence and progression to cervical cancer (30). Compared to patients diagnosed with low− and high-grade squamous intraepithelial lesions, increased levels of Lactobacillus crispatus, Lactobacillus iners, and Lactobacillus taiwanensis were observed in the vaginal swabs of healthy women, while Gardnerella vaginalis and Lactobacillus acidophilus were absent. The bacterial dysbiosis observed in these patients, which featured a predominance of G. vaginalis and a concomitant paucity of L. crispatus, L. iners, and L. taiwanensis, may be associated with the development of HPV-dependent cervical cancer (31). However, whether pelvic IMRT affects the vaginal microbiome remains unknown.
A previous study compared differences in the proportions of bacteria isolated before and after radiotherapy using aerobic culture. The results showed no significant changes in the positive cultures of pathogens; however, the normal flora significantly increased after external beam irradiation (32). However, methods based on next-generation sequencing (NGS) were not used in this study. Using 16S rRNA sequencing, we observed no significant changes in overall diversity before, during, and after radiotherapy, consistent with the above study. However, the relative abundances of several taxa, including Gammaproteobacteria, Pseudomonadales, Gemmatimonadaceae, and Prevotella 9, increased significantly with irradiation time.
The tumor microenvironment can have great impact on radioresistance and tumor recurrence (33). The microbiome can also affect cancer by triggering DNA damage, modulating inflammation, and generating metabolites (10). Several previous studies have shown that patients receiving radiotherapy display obvious changes in the microbiomes of the irradiated areas as well as changes in the microenvironment, indicating that the microbiome may serve as an aberrant proinflammatory factor. A study by Wang et al. showed that Gammaproteobacteria, Pseudomonadales, and Prevotella 9, which showed increased abundance with radiation time in this study, were more abundant in the fecal microbiome after pelvic radiation, and were strongly associated with diagnoses of radiation enteritis. In vitro experiments indicated that radiation-induced microbiome dysbiosis results in epithelial cell damage, promoting inflammatory responses in the local mucosa by activating nuclear factor κB (NFκB) signaling and cytokine secretion (34). Radiation can reduce resistance to commonly used antibiotics, and vancomycin pretreatment can enhance the antitumor effects of radiation in vivo by increasing antigen presentation and cytotoxic T cell infiltration into the tumor, through modulation of the gut microbiota (32, 35). It has been suggested that microbiome superantigens might promote radiotherapy-induced inflammation by activating T cells and attenuating epithelial cell recovery (36). Increasing evidence indicates that inflammatory signaling pathways such as the toll-like receptor/myeloid differentiation primary response 88, proinflammatory cytokine, NFκB, and cyclooxygenase-2 pathways are bridging factors between the microbiome and cancer (37). The detection of Gemmatimonadaceae DNA in the blood has been associated with tumor progression in patients treated with nivolumab (38). While some studies have found no benefit to probiotic use (39), a meta-analysis demonstrated a beneficial effect of probiotics in reducing the incidence of diarrhea (40). Furthermore, one study reported that the anaerobic bacteria Clostridium novyi-NT, which is missing its major toxin gene, is able to selectively destroy the hypoxic regions of tumors and improve the efficacy of radiation in mouse tumor models (41).
In conclusion, we conducted a bioinformatic analysis of the VM in patients with cervical cancer receiving pelvic radiochemotherapy and healthy controls by 16S rRNA sequencing. We firstly examined microbiome differences between the cervical cancer patients and health controls, and then investigated the impact of pelvic radiochemotherapy on the VM in patients with cervical cancer. Our data indicated some changes in the relative abundances of the microbiome, which might have critical effects on the efficacy of radiochemotherapy. Future studies will be required to understand the relationship between the VM and radiochemotherapy in cervical cancer.
Data Availability Statement
The data sets presented in this study can be found in online repositories. The names of the repository/repositories and accession number(s) can be found below: 16S rRNA squencing data to SRA (https://www.ncbi.nlm.nih.gov/sra/). The SRA ID is PRJNA687644.
Ethics Statement
The studies involving human participants were reviewed and approved by The Ethical Review Committee of the First Affiliated Hospital of Guangxi Medical University. The patients/participants provided their written informed consent to participate in this study.
Author Contributions
RW and TH designed the study. YZ and SM collected samples and followed up the patients. BL, FL, and CL performed the experiments. LJ and ZW performed the data analyses. LJ wrote the main manuscript text and BL prepared all figures and tables. RW and TH critically inspected the manuscript and participated in its revision. LJ and BL contributed equally to this study and are the co-first authors of this paper. All authors contributed to the article and approved the submitted version.
Funding
This study was supported by the Youth Science Foundation of Guangxi Medical University (GXMUYSF201505), the Basic Ability Enhancement Project for Young Teachers in Guangxi Zhuang Autonomous Region (2018KY0134), the Guangxi Zhuang Autonomous Region Health and Wellness Committee Science and Technology Project (S2017017), the Key Research and Development Program of Guangxi (Guike AB18281003), the “139” Program for high-level medical talents in Guangxi, Innovation Team of the First Affiliated Hospital of Guangxi Medical University, and the Guangxi Science and Technology Program Project (GK AD17129013). The funders had no role in study design, data collection and analysis, decision to publish, or preparation of the manuscript.
Conflict of Interest
The authors declare that the research was conducted in the absence of any commercial or financial relationships that could be construed as a potential conflict of interest.
Acknowledgments
We thank Mr. Jindong Zhu and Mr. Lucheng Zhang (Anrenxin Biotechnology Co., Ltd at Nanning, China) for their helpful discussions and bioinformatics analysis.
Supplementary Material
The Supplementary Material for this article can be found online at: https://www.frontiersin.org/articles/10.3389/fonc.2021.615439/full#supplementary-material
Supplementary Table 1 | The OTUs of cervical cancer patients and healthy controls. CV, cervical cancer patients; HC, healthy controls.
References
1. Bray F, Ferlay J, Soerjomataram I, Siegel RL, Torre LA, Jemal A. Global cancer statistics 2018: GLOBOCAN estimates of incidence and mortality worldwide for 36 cancers in 185 countries. CA Cancer J Clin (2018) 68:394–424. doi: 10.3322/caac.21492
2. Vordermark D. Radiotherapy of Cervical Cancer. Oncol Res Treat (2016) 39:516–20. doi: 10.1159/000448902
3. Berger T, Seppenwoolde Y, Potter R, Assenholt MS, Lindegaard JC, Nout RA, et al. Importance of Technique, Target Selection, Contouring, Dose Prescription, and Dose-Planning in External Beam Radiation Therapy for Cervical Cancer: Evolution of Practice From EMBRACE-I to II. Int J Radiat Oncol Biol Phys (2019) 104:885–94. doi: 10.1016/j.ijrobp.2019.03.020
4. Kitahara O, Katagiri T, Tsunoda T, Harima Y, Nakamura Y. Classification of sensitivity or resistance of cervical cancers to ionizing radiation according to expression profiles of 62 genes selected by cDNA microarray analysis. Neoplasia (2002) 4:295–303. doi: 10.1038/sj.neo.7900251
5. Babu G, Singaravelu BG, Srikumar R, Reddy SV, Kokan A. Comparative Study on the Vaginal Flora and Incidence of Asymptomatic Vaginosis among Healthy Women and in Women with Infertility Problems of Reproductive Age. J Clin Diagn Res (2017) 11:Dc18–dc22. doi: 10.7860/JCDR/2017/28296.10417
6. Njoku K, Crosbie EJ. Does the vaginal microbiome drive cervical carcinogenesis? BJOG (2020) 127(2):181.
7. Champer M, Wong AM, Champer J, Brito IL, Messer PW, Hou JY, et al. The role of the vaginal microbiome in gynaecological cancer. BJOG (2018) 125:309–15. doi: 10.1111/1471-0528.14631
8. Kyrgiou M, Mitra A, Moscicki AB. Does the vaginal microbiota play a role in the development of cervical cancer? Transl Res (2017) 179:168–82. doi: 10.1016/j.trsl.2016.07.004
9. Zhu XX, Yang XJ, Chao YL, Zheng HM, Sheng HF, Liu HY, et al. The Potential Effect of Oral Microbiota in the Prediction of Mucositis During Radiotherapy for Nasopharyngeal Carcinoma. EBioMedicine (2017) 18:23–31. doi: 10.1016/j.ebiom.2017.02.002
10. Bhatt AP, Redinbo MR, Bultman SJ. The role of the microbiome in cancer development and therapy. CA Cancer J Clin (2017) 67:326–44. doi: 10.3322/caac.21398
11. Zakrzewski M, Goesmann A, Jaenicke S, Junemann S, Eikmeyer F, Szczepanowski R, et al. Profiling of the metabolically active community from a production-scale biogas plant by means of high-throughput metatranscriptome sequencing. J Biotechnol (2012) 158:248–58. doi: 10.1016/j.jbiotec.2012.01.020
12. Klindworth A, Pruesse E, Schweer T, Peplies J, Quast C, Horn M, et al. Evaluation of general 16S ribosomal RNA gene PCR primers for classical and next-generation sequencing-based diversity studies. Nucleic Acids Res (2013) 41:e1. doi: 10.1093/nar/gks808
13. Magoc T, Salzberg SL. FLASH: fast length adjustment of short reads to improve genome assemblies. Bioinformatics (2011) 27:2957–63. doi: 10.1093/bioinformatics/btr507
14. Park CH, Yeo HJ, Park YE, Baek SA, Kim JK, Park SU. Transcriptome Analysis and Metabolic Profiling of Lycoris Radiata. Biol (Basel) (2019) 8(3):63. doi: 10.3390/biology8030063
15. Rognes T, Flouri T, Nichols B, Quince C, Mahe F. VSEARCH: a versatile open source tool for metagenomics. PeerJ (2016) 4:e2584. doi: 10.7717/peerj.2584
16. Quast C, Pruesse E, Yilmaz P, Gerken J, Schweer T, Yarza P, et al. The SILVA ribosomal RNA gene database project: improved data processing and web-based tools. Nucleic Acids Res (2013) 41:D590–6. doi: 10.1093/nar/gks1219
17. Caporaso JG, Kuczynski J, Stombaugh J, Bittinger K, Bushman FD, Costello EK, et al. QIIME allows analysis of high-throughput community sequencing data. Nat Methods (2010) 7:335–6. doi: 10.1038/nmeth.f.303
18. Schloss PD, Gevers D, Westcott SL. Reducing the effects of PCR amplification and sequencing artifacts on 16S rRNA-based studies. PLoS One (2011) 6:e27310. doi: 10.1371/journal.pone.0027310
19. Paul D, Kumbhare SV, Mhatre SS, Chowdhury SP, Shetty SA, Marathe NP, et al. Exploration of Microbial Diversity and Community Structure of Lonar Lake: The Only Hypersaline Meteorite Crater Lake within Basalt Rock. Front Microbiol (2015) 6:1553. doi: 10.3389/fmicb.2015.01553
20. Sun CH, Liu HY, Liu B, Yuan BD, Lu CH. Analysis of the Gut Microbiome of Wild and Captive Pere David’s Deer. Front Microbiol (2019) 10:2331. doi: 10.3389/fmicb.2019.02331
21. Keich U, Noble WS. Controlling the FDR in imperfect matches to an incomplete database. J Am Stat Assoc (2018) 113:973–82. doi: 10.1080/01621459.2017.1375931
22. Segata N, Izard J, Waldron L, Gevers D, Miropolsky L, Garrett WS, et al. Metagenomic biomarker discovery and explanation. Genome Biol (2011) 12:R60. doi: 10.1186/gb-2011-12-6-r60
23. Langille MG, Zaneveld J, Caporaso JG, McDonald D, Knights D, Reyes JA, et al. Predictive functional profiling of microbial communities using 16S rRNA marker gene sequences. Nat Biotechnol (2013) 31:814–21. doi: 10.1038/nbt.2676
24. Abel S, Abel zur Wiesch P, Chang HH, Davis BM, Lipsitch M, Waldor MK. Sequence tag-based analysis of microbial population dynamics. Nat Methods (2015) 12:223–6. 3 p following 226. doi: 10.1038/nmeth.3253
25. Cohen PA, Jhingran A, Oaknin A, Denny L. Cervical cancer. Lancet (2019) 393:169–82. doi: 10.1016/S0140-6736(18)32470-X
26. Mitra A, MacIntyre DA, Lee YS, Smith A, Marchesi JR, Lehne B, et al. Cervical intraepithelial neoplasia disease progression is associated with increased vaginal microbiome diversity. Sci Rep (2015) 5:16865. doi: 10.1038/srep16865
27. Zhang C, Liu Y, Gao W, Pan Y, Gao Y, Shen J, et al. The direct and indirect association of cervical microbiota with the risk of cervical intraepithelial neoplasia. Cancer Med (2018) 7:2172–9. doi: 10.1002/cam4.1471
28. Mitra A, MacIntyre DA, Marchesi JR, Lee YS, Bennett PR, Kyrgiou M. The vaginal microbiota, human papillomavirus infection and cervical intraepithelial neoplasia: what do we know and where are we going next? Microbiome (2016) 4:58. doi: 10.1186/s40168-016-0203-0
29. Howe HL, Wu X, Ries LA, Cokkinides V, Ahmed F, Jemal A, et al. Annual report to the nation on the status of cancer, 1975-2003, featuring cancer among U.S. Hispanic/Latino Popul Cancer (2006) 107:1711–42. doi: 10.1002/cncr.22193
30. Laniewski P, Cui H, Roe DJ, Barnes D, Goulder A, Monk BJ, et al. Features of the cervicovaginal microenvironment drive cancer biomarker signatures in patients across cervical carcinogenesis. Sci Rep (2019) 9:7333. doi: 10.1038/s41598-019-43849-5
31. Kwasniewski W, Wolun-Cholewa M, Kotarski J, Warchol W, Kuzma D, Kwasniewska A, et al. Microbiota dysbiosis is associated with HPV-induced cervical carcinogenesis. Oncol Lett (2018) 16:7035–47. doi: 10.3892/ol.2018.9509
32. Mubangizi L, Namusoke F, Mutyaba T. Aerobic cervical bacteriology and antibiotic sensitivity patterns in patients with advanced cervical cancer before and after radiotherapy at a national referral hospital in Uganda. Int J Gynaecol Obstet (2014) 126:37–40. doi: 10.1016/j.ijgo.2014.01.013
33. Barker HE, Paget JT, Khan AA, Harrington KJ. The tumour microenvironment after radiotherapy: mechanisms of resistance and recurrence. Nat Rev Cancer (2015) 15:409–25. doi: 10.1038/nrc3958
34. Wang Z, Wang Q, Wang X, Zhu L, Chen J, Zhang B, et al. Gut microbial dysbiosis is associated with development and progression of radiation enteritis during pelvic radiotherapy. J Cell Mol Med (2019) 23:3747–56. doi: 10.1111/jcmm.14289
35. Uribe-Herranz M, Rafail S, Beghi S, Gil-de-Gomez L, Verginadis I, Bittinger K, et al. Gut microbiota modulate dendritic cell antigen presentation and radiotherapy-induced antitumor immune response. J Clin Invest (2020) 130:466–79. doi: 10.1172/JCI124332
36. Hill A, Hanson M, Bogle MA, Duvic M. Severe radiation dermatitis is related to Staphylococcus aureus. Am J Clin Oncol (2004) 27:361–3. doi: 10.1097/01.COC.0000071418.12121.C2
37. Kipanyula MJ, Seke Etet PF, Vecchio L, Farahna M, Nukenine EN, Nwabo Kamdje AH. Signaling pathways bridging microbial-triggered inflammation and cancer. Cell Signal (2013) 25:403–16. doi: 10.1016/j.cellsig.2012.10.014
38. Ouaknine Krief J, Helly de Tauriers P, Dumenil C, Neveux N, Dumoulin J, Giraud V, et al. Role of antibiotic use, plasma citrulline and blood microbiome in advanced non-small cell lung cancer patients treated with nivolumab. J Immunother Cancer (2019) 7:176. doi: 10.1186/s40425-019-0658-1
39. Blanarova C, Galovicova A, Petrasova D. Use of probiotics for prevention of radiation-induced diarrhea. Bratisl Lek Listy (2009) 110:98–104.
40. Liu MM, Li ST, Shu Y, Zhan HQ. Probiotics for prevention of radiation-induced diarrhea: A meta-analysis of randomized controlled trials. PLoS One (2017) 12:e0178870. doi: 10.1371/journal.pone.0178870
Keywords: vaginal microbiome, cervical cancer, intensity-modulated radiation therapy, radiochemotherapy, 16S rRNA sequencing
Citation: Jiang L, Li B, Zhang Y, Ma S, Liu C, Liang F, Wei Z, Huang T and Wang R (2021) Influence of Pelvic Intensity-Modulated Radiation Therapy With Concurrent Cisplatin-Based Chemotherapy of Cervical Cancer on the Vaginal Microbiome. Front. Oncol. 11:615439. doi: 10.3389/fonc.2021.615439
Received: 09 October 2020; Accepted: 06 January 2021;
Published: 23 February 2021.
Edited by:
Xi Yang, Fudan University, ChinaReviewed by:
Qing Guo, Jiangsu Taizhou People’s Hospital, ChinaMengying Shi, University of Massachusetts, Lowell, United States
Copyright © 2021 Jiang, Li, Zhang, Ma, Liu, Liang, Wei, Huang and Wang. This is an open-access article distributed under the terms of the Creative Commons Attribution License (CC BY). The use, distribution or reproduction in other forums is permitted, provided the original author(s) and the copyright owner(s) are credited and that the original publication in this journal is cited, in accordance with accepted academic practice. No use, distribution or reproduction is permitted which does not comply with these terms.
*Correspondence: Rensheng Wang, MTM4MDc4MDYwMDhAMTYzLmNvbQ==; Tingting Huang, aHR0Z3htdUAxNjMuY29t
†These authors have contributed equally to this work and share first authorship