- 1Department of Oncology, KU Leuven, Leuven, Belgium
- 2Laboratory for Translational Cell and Tissue Research, Department of Imaging and Pathology, KU Leuven, Leuven, Belgium
- 3Laboratory for Precision Cancer Medicine, Translational Cell and Tissue Research Unit, Department of Imaging and Pathology, KU Leuven, Leuven, Belgium
The state-of-the-art for melanoma treatment has recently witnessed an enormous revolution, evolving from a chemotherapeutic, “one-drug-for-all” approach, to a tailored molecular- and immunological-based approach with the potential to make personalized therapy a reality. Nevertheless, methods still have to improve a lot before these can reliably characterize all the tumoral features that make each patient unique. While the clinical introduction of next-generation sequencing has made it possible to match mutational profiles to specific targeted therapies, improving response rates to immunotherapy will similarly require a deep understanding of the immune microenvironment and the specific contribution of each component in a patient-specific way. Recent advancements in artificial intelligence and single-cell profiling of resected tumor samples are paving the way for this challenging task. In this review, we provide an overview of the state-of-the-art in artificial intelligence and multiplexed immunohistochemistry in pathology, and how these bear the potential to improve diagnostics and therapy matching in melanoma. A major asset of in-situ single-cell profiling methods is that these preserve the spatial distribution of the cells in the tissue, allowing researchers to not only determine the cellular composition of the tumoral microenvironment, but also study tissue sociology, making inferences about specific cell-cell interactions and visualizing distinctive cellular architectures - all features that have an impact on anti-tumoral response rates. Despite the many advantages, the introduction of these approaches requires the digitization of tissue slides and the development of standardized analysis pipelines which pose substantial challenges that need to be addressed before these can enter clinical routine.
Introduction
Next-Generation Pathology and Personalized Medicine in Melanoma
The oncological treatment of melanoma has radically changed over the past 10 years: it evolved from a “one-fits-all” chemotherapeutic treatment with DTIC (1) to a more tailored setting where therapies are only given when patient- and tumor-specific features are present. This evolution toward personalized therapy was ignited by the observation that specific drugs were only clinically effective in the presence of a specific mutation (2–10). In addition, following the first successes with IL-2 therapy (11–13), immunotherapy was re-evaluated leading to the identification and implementation of checkpoint inhibitor therapy, a type of immunotherapy based on blocking the breaks that normally prevent the immune system from becoming hyperactivated (14–17). While oncology is gradually moving toward personalized treatments, also pathological assessments need to progress to cope with the need for in-depth characterizations of tumor tissues from individual patients. Salto-Tellez et al. have previously discussed how pathology, a discipline originally based on the evaluation of tissue morphology by hematoxylin-eosin (HE) staining, witnessed 3 main revolutions: first, the introduction of immunohistochemistry (IHC) in the 80s; second, the adoption of molecular techniques in pathology (molecular pathology, MP; mostly next-gen sequencing); and, most recently, the development of artificial intelligence (AI) tools to support the pathologist to evaluate and interpret the different features (18). While tools from the first two revolutions are nowadays fully embedded in routine clinical work and represent the earliest steps toward personalized medicine, the third revolution is still awaiting its breakthrough.
From the available tools, MP is the most advanced as it reached the required level of specificity to represent the state-of-the-art. It is mostly based on next-generation sequencing through which it allows the identification of genetic aberrations, either by analyzing focused gene panels or whole genome sequencing. In melanoma, the mutational profile is nowadays used to support diagnostics but also to select the most appropriate treatment. For the former, the new WHO Skin Cancer classification has identified 9 molecular pathways in which the melanocytic lesions can be classified based on the type and number of genetic alterations involved (19). Each of these pathways is further divided in 3 categories with different biological behavior (benign, intermediate and malignant) that can also be predicted according to the number of genetic alterations (≤1, 2 and >2 respectively) (19). The choice of treatment, on the other hand, is primarily based on the presence of targetable mutations, such as BRAF V600 mutations, for which specific therapies are available (2–10).
While NGS methods are constantly improving and evolving, the use of IHC hardly changed over the past 20 years. Indeed, as opposed to NGS analyses that typically cover 10-100 genes simultaneously, conventional IHC allows to stain tissue sections one marker at the time. As such, the analysis of multiple biomarkers typically requires the analysis of serial sections which may be a limiting step in small biopsies where only small amounts of materials are available. Moreover, by its inability to investigate the co-expression of several markers in the same cell, important information is systematically missed. A workaround has been to analyze marker expression patterns in serial sections, but this approach does not achieve sufficient detail to get to a robust interpretation. As a consequence, conventional IHC has become largely insufficient to cope with the required level and depth by which tumor tissues for each individual patients should be analyzed. A striking example involves the use of PD-L1 as a single-plex marker for the prediction of immunotherapy response: even though it has been implemented in routine pathological assessments, its detection suffers from significant technical hurdles making it largely insufficient as a good predictive marker. Moreover, recent research suggests that the cell types that express PD-L1 and their location in the tissue is also of major importance. However, gaining such insights cannot be addressed by old pathological practices where a semi-quantitative eye-balling interpretation of the staining is used for subjective evaluation, and therefore requires the implementation of single cell-technologies that preserve the spatial distribution of the various cell types and their original state (20). Multiplexed IHC, a technological approach that harbors the potential to collect exactly this type of data, has witnessed major progress over the past 2-3 years, but still requires several adaptations. For instance, it relies on full image digitalization and extended computational analysis, a limitation (but also opportunity) that multiplexed IHC and artificial intelligence (AI) have in common for their further implementation in a clinical setting.
Even though digital pathology-based AI tools have already been developed and have shown some diagnostic, prognostic, and predictive potential comparable to standard molecular and genomic-based tests, digital pathology (i.e. the process of digitizing whole-slide images using advanced slide-scanning techniques) has not yet been introduced in hospitals at large scale. Recent advancements in multiplexed IHC anticipate an even more important role for AI in pathology. The plethora of data generated by multiplexed IHC where tens to hundreds of markers are measured in thousands to millions of cells in their spatial context, provides the ideal setting to exploit AI and deep learning methods in particular. One of the strongest aspects of deep learning is to discover hidden features (and their combinations) otherwise invisible by purely visual inspection, and correlate them with clinical data. The parallel advancement of multiplexed IHC and AI-based computational models represent an unprecedented scenario for the introduction of next-generation pathology in clinical practice, characterized by the more widespread usage of digital images and the introduction of artificial intelligence and deep learning tools on histopathological images.
In this review we discuss the state-of-the-art, the potential and the challenges linked to the introduction of next-generation pathology to the clinical practice of melanoma patients. All the studies considered in this review are summarized in Table 1.
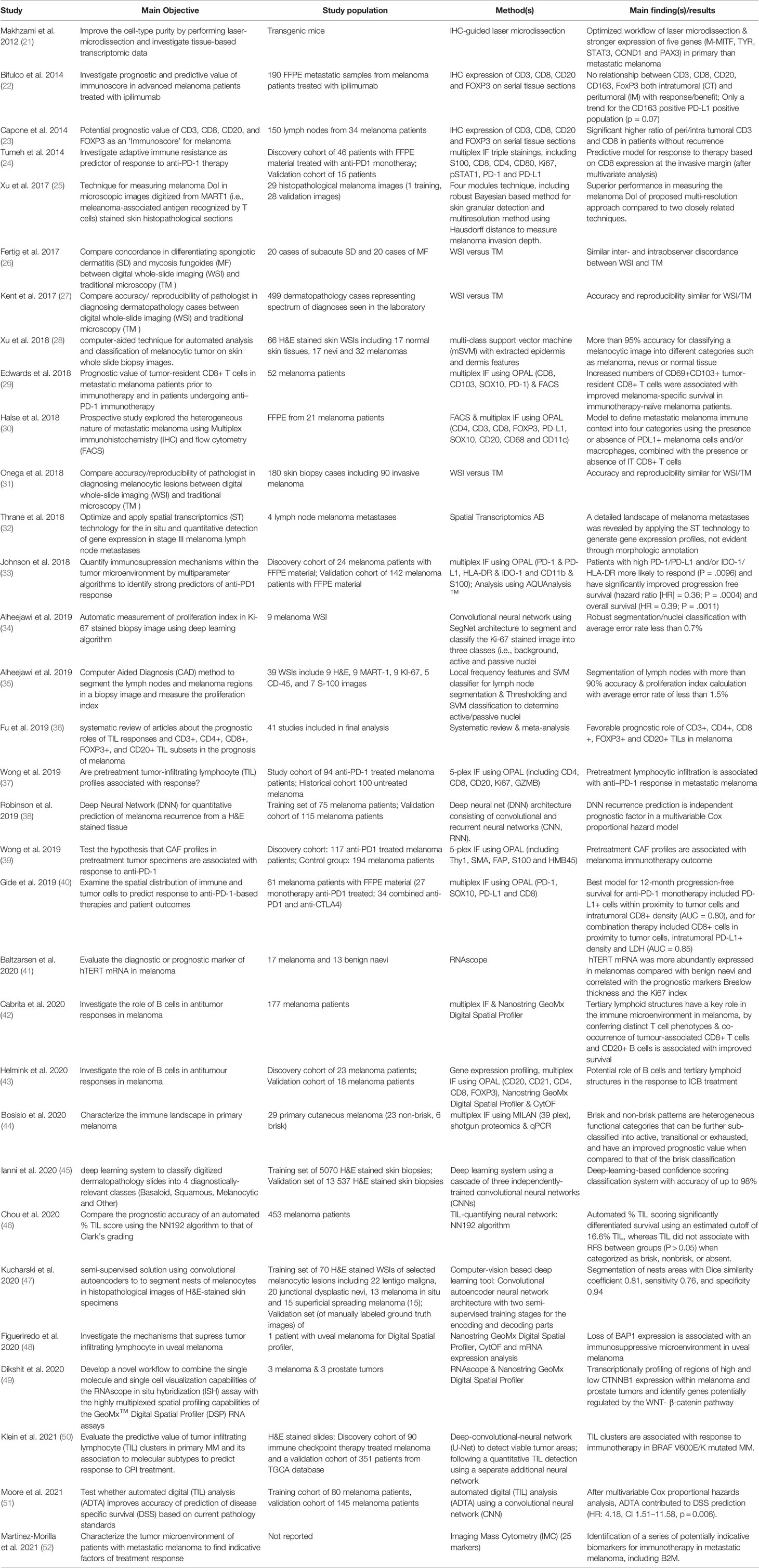
Table 1 Overview of recent studies using digital pathology in melanoma. All studies are ordered according to time of publication.
The New Morphological Evaluation: AI-Based
Historically, the role of the dermatopathologist in malignant melanoma concerned mainly 3 aspects: (i) find the right histopathological diagnosis of pigmented lesions; (ii) define the pathological staging for the primary malignant melanoma on the basis of Breslow thickness and ulceration; and (iii) list all the other relevant prognostic parameters not included in the staging process such as regression, inflammatory infiltrate, microsatellites, etc. This evaluation has always been done using a simple hematoxylin-eosin (HE) staining and a visual interpretation of the morphometric features of the tissue by the pathologist. The first task listed above is definitely the most challenging and still impossible to be performed by the machine autonomously. For the last two monotonous tasks, instead, the pathologist can be more effectively assisted by digital pathology where these parameters can be objectively quantified by the computer on digitized whole-slide images leaving more time to the pathologist for the diagnostic process.
First of all, finding the right histopathological diagnosis of pigmented lesions is known to be one of the most challenging tasks in pathology, requiring extended training and expertise. This is further highlighted by the fact that there can be a high degree of discordance when the same lesion gets evaluated by different pathologists (53). Even though discordance is still present among the more experienced dermatopathologists (54), experience and specific training in dermatopathology do improve the diagnosis of difficult cases (55). In fact, digital pathology can be used to virtually share slides between peripheral hospitals and reference centers, facilitating the process of second opinion and expert review. As such, both AI and digital pathology can provide a more standardized level of diagnostic accuracy, ensuring patients get access to the most reliable diagnostic assessments. Digitized whole scan images of a histological slide have been found to have similar effectiveness, both in terms of accuracy and diagnostic workflow, to traditional microscopy for the evaluation melanocytic lesions (26, 27, 56). Moreover, artificial intelligence can also bring its experience, namely its machine learning training, to the side of less experienced pathologists to assist them with more complex diagnostics. In this direction, even before the introduction of machine learning, feature extraction-based algorithms had already proven to be efficient to distinguish melanocytic lesions with an accuracy of 95% (28). Even more recently, a first machine learning algorithm was developed to evaluate the degree of uniformity and symmetry of melanocytic nests as a first step to discriminate between benign and malignant lesions (47). Nevertheless, it is very unlikely that the application of digital pathology and AI will replace the pathologist in the diagnostic process, especially for melanocytic lesions. Since the use of deep learning allows the mining of complex morphometric features that go beyond mere visual identification, these can be applied in the form of an augmented reality rather than of an autonomously working AI, in order to suggest elements in favor and against the diagnosis of melanoma that will necessarily need to be reviewed by the pathologist itself. The augmented reality will bring to the attention of the pathologist features that should not be missed, helping him to recognize the trivial case (all the features pointing in one direction) from the more complex one (more contrasting/ambiguous features), speeding up the work of the pathologist, thanks to a triage process but not substituting him in making the definitive diagnosis. Therefore, it is also more realistic that the role of digital pathology and machine learning will be assisting the general pathologists with less experience in melanocytic lesions rather than the experienced dermatopathologist (Figure 1).
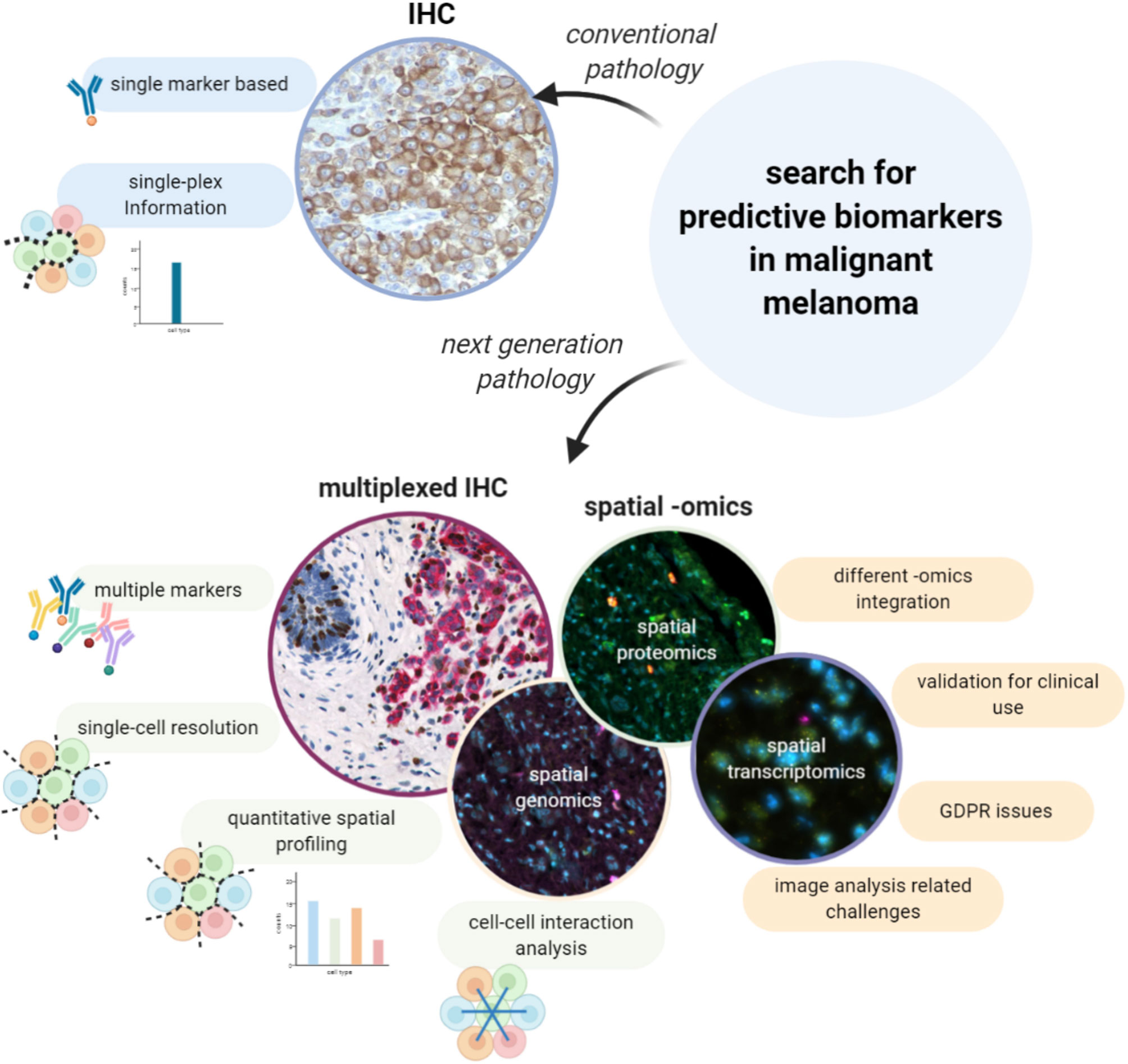
Figure 1 Digital Pathology and AI for a new morphological evaluation. The limitations related to the visual inspection-based diagnosis made by the pathologist on HE stained samples can be overcome with digital pathology and the support of AI and image analysis. Thanks to such computational tools it is possible to achieve more accurate diagnoses, based on a quantitative and more detailed analysis rather than a qualitative assessment, to support pathologists in their work, to automate time-consuming and repetitive tasks and to also improve the organization and the way cases are stored.
Interestingly, artificial intelligence could also be used to organize collections of digitized tissue slides by image similarity, and, as such, go far beyond the use of mere text-based searches. This can have various applications: (i) matching new cases to archived morphologically similar cases to propose a putative diagnosis and potentially improve the diagnostic accuracy; (ii) groups of similar images can more efficiently be retrieved from the archives for training purposes, not only to develop new or improved algorithms, but also for pathologists-in-training (57).
Software packages that are able to apply automated measurements, can also make diagnostics more efficient by automatically retrieving the required parameters and adding them to clinical reports. One of the first studies to use deep learning in histopathology allowed to recognize and count mitotic cells in breast cancer with higher accuracy compared to manual assessment (58). As manual counting mitotic nuclei is a highly time-consuming tasks, it could be easily replaced by AI in melanoma reporting as well. Other practical examples involve the measurement of the Breslow thickness (25), the evaluation of the proliferation index or the detection of lymph node metastasis (34, 35), for which deep learning algorithms are already available. In addition to this, deep learning has been proven useful as an alternative way to the most traditional pathological report to predict the risk of melanoma recurrence, on the basis of features extracted from HE images (38). Moreover, image analysis and machine learning were also applied to quantify tumor infiltrating lymphocytes (TILs) on HE, revealing to be a better tool than the actual semiquantitative classification in brisk, non-brisk and absent to estimate survival for melanoma patients (46, 50, 51) and to be associated with response to checkpoint inhibitors in BRAF V600E/K mutated malignant melanomas (50)
Finally, on top of assisting the pathologist with the diagnostic process and the definition of the prognosis, there are additional advantages to the introduction of digital pathology (Figure 1). The number of cases in dermatopathology has been rising over the last decade, and as such also the workload of the dermatopathologists (59). Most of these lesions are benign and easy to recognize, yet require dedicated time for evaluation. This reduces the available time for the more challenging/difficult cases. Software packages have recently entered the market that can assign a “class” to a skin lesion (e.g. epithelial vs melanocytic), detect easy, benign lesions, that can be prioritized and quickly diagnosed, and assign a particular flag to cases recognized as “complex”, onto which the pathologist can focus longer (45). In this way, artificial intelligence can help to optimize the flow of the daily work of the pathologist and improve the robustness and efficiency to come to a proper diagnosis.
Beyond Morphology: The Spatial Omics
As stated higher, the evolving treatment landscape in malignant melanoma has resulted in an increased demand for more and better predictive evaluations on top of the already available prognostic ones. The combination of both is a prerequisite to move toward personalized approaches in which treatments are matched to the right patients. Within metastatic melanoma, the use of checkpoint inhibitor therapy has revolutionized the outcome for patients with an objective response rate between 33.7-45%. Interestingly, the clinical efficacy of anti-PD1 antibodies as monotherapy (15, 16, 60), was slightly improved when combined with anti-CTLA-4 antibodies (up to 58%) (60, 61), but at the cost of higher toxicity rates. To avoid the biological, ethical and economical costs of administering non-effective treatments to patients, we will need to find predictive biomarkers that can guide clinicians to make informed decisions. In this light, several biomarkers have been described, such as a minimal expression of PD-L1 by conventional IHC (62), a minimal level of tumor mutational burden (TMB) (63), and gene expression profiling (GEP) using the IPRES or IMPRES signatures (64, 65), but none of these have provided the required sensitivity and/or specificity to be implemented in the clinic. This could be due to the limited amounts of information on the tumor and its microenvironment that are gathered by these assays, and which turned out to be insufficient to efficiently predict response to therapy. Indeed, understanding the conditions in which the immune system can be reinvigorated by ICB turns out to be complex and requires the integration of multiple parameters and features. Next-generation pathology using spatially resolved single-cell assessments of a tissue has the potential to shed more light on the complex role of the TME in a patient response to therapy, as it integrates functional information of each individual cell while adding information about their spatial context (Figure 2) and as such the interactions between different cell types.
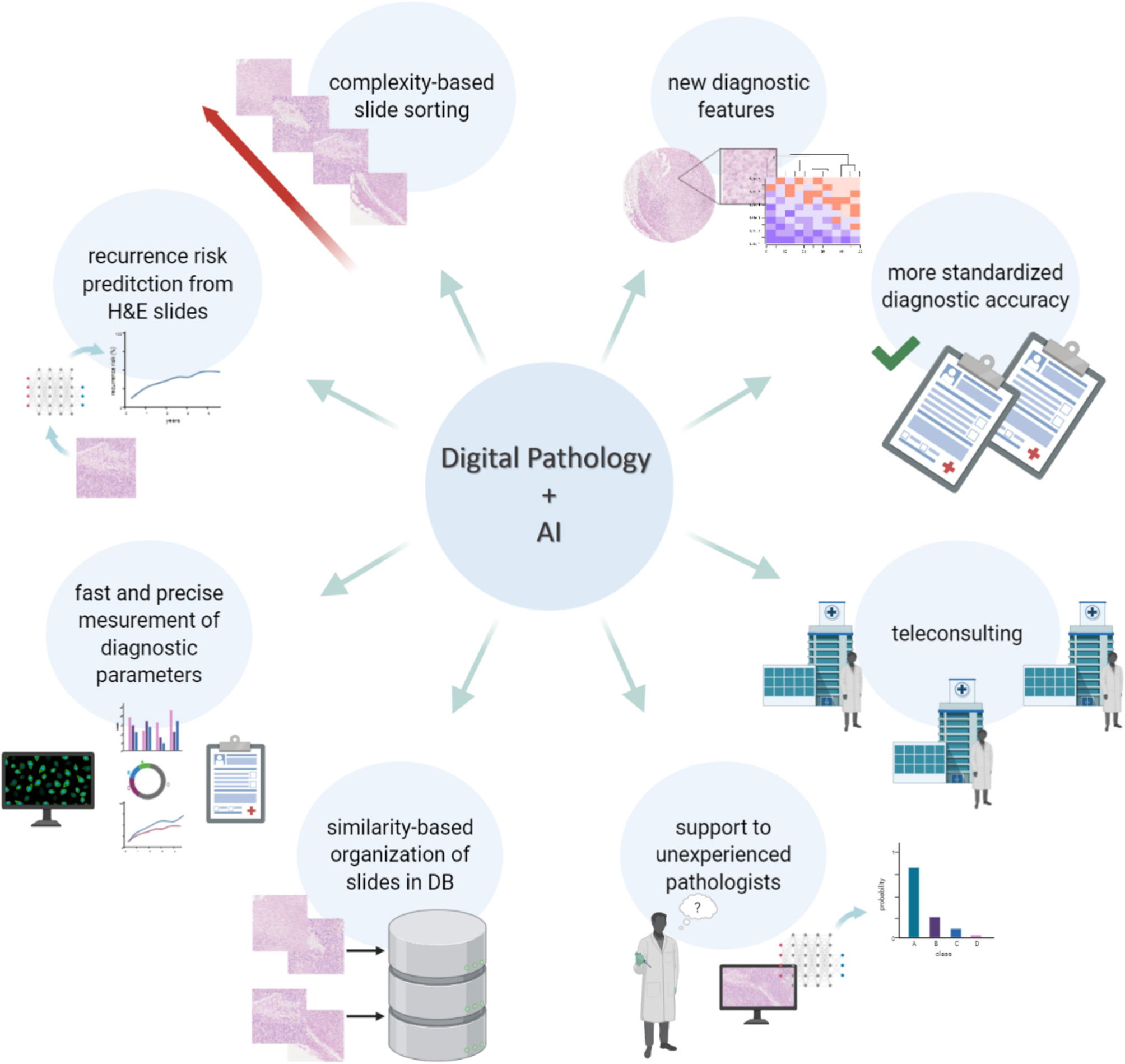
Figure 2 Searching for predictive biomarkers in malignant melanoma with spatial multiplexing techniques: advantages and challenges. Predictive evaluation of malignant melanoma is needed for a more personalized treatment plan, but predictive biomarkers must still be identified. Conventional IHC is a single-plex based method which does not provide information at single-cell level. On the other hand, multiplexed IHC and spatial -omics methods make it possible to extract information from multiple markers at single-cell resolution and to investigate cell-cell interactions. However, despite the great advantages, those techniques have not yet been validated in clinics and it is currently not possible to integrate the information from different -omics on the same section at single-cell level. Moreover, those methods are strictly dependent on computational techniques for the downstream analysis, hence they carry all the challenges related to image analysis.
As anticipated in the introduction, conventional IHC cannot provide a multiparametric in-depth characterization of the tissue at single cell level. To overcome the limitations of conventional IHC, multiple approaches have been tested. A first example involves the use of virtual multiplexing which vertically aligns digital images from serial sections. Virtual multiplexing has been made (commercially) available by VisioPharm and HistogGeneX (66) among others. An example is the Tissuealign™ analysis module from VisioPharm that has been validated for in vitro diagnostic use (CE-IVD) in Europe in combination with the CE IVD APPs from VisioPharm (67, 68). Nevertheless, vertical registration still does not allow detailed single-cell phenotyping which requires insights in the co-expression of different markers in exactly the same cell. In addition, to identify all the inflammatory subpopulations that are present in a histological sample, the evaluation of more than 20 markers is needed, ideally on the same tissue section (“high-plexing”). Nowadays, several methods for tissue multiplexing are available (69) and any technique representing a surrogate to investigate co-expression of markers at single cell level should be replaced by multiplexed IHC. First investigated in the context of colorectal cancer (70), the implementation of the concept of an ‘Immunoscore’ or immunoprofiling into a renewed cancer staging system incorporating the effects of the host immune response based on the numeration of specific lymphocyte populations alongside with the tumor cell-autonomous characteristics has been proven useful in the context of advanced melanoma as well (23). The colorectal Immunoscore, which involved a quantitative assessment of CD3+ and CD8+ T cells both at the invasive margin and bulk of the tumor, was already published in 2006 and encouraged the adoption of digital pathology tools for biomarker discovery (70, 71). Specific for melanoma, the definition of a comparable Immunoscore seems to be a more difficult challenge (72). In many patients, metastatic lymph nodes are the only available tissue samples and concerns are raised about the applicability of an Immunoscore in lymph nodes because they are constitutively rich in CD3 and CD20 lymphocytes. In a first effort, an Immunoscore constructed based on the expression of CD8, CD3, CD20 and FOXP3, was applied on a small cohort of stage III melanoma patients showing significant differences in the peri/intratumoral ratio for both CD3 and CD8, with the ratio being higher in patients without recurrence compared to patients with melanoma recurrence, with similar trends for both FOXP3 and CD20 were observed (71). In a more recently published systematic review, a favorable prognostic role of the CD3+, CD4+, CD8+, FOXP3+, and CD20+ TILs on the overall survival of melanoma patients was confirmed. In addition, in a subgroup analysis, brisk TILs were associated with overall survival, recurrence-free survival, and melanoma-specific survival (36). Likewise, the predictive performance of an alternative Immunoscore, using a digital image analysis application to characterize immune infiltrate expression of CD3, CD8, CD20, FOXP3 and CD163 and of PD-L1, was tested in a metastatic melanoma cohort of patients treated with Ipilimumab in the MISIPI trial (22, 72). Unfortunately, this trial was unable to confirm the relationship between intra/peritumoral expression of CD3, CD8, CD20, CD163, FOXP3 and a response/benefit to therapy, apart from a trend for the CD163-PD-L1 double positive population (22). Another study, using a low-plex with only 6 markers found instead that the quantity but not the activation of CD8+ TILs was associated with anti-PD-1 response in metastatic melanoma (37). In an attempt to categorize the intrinsic heterogeneous nature of metastatic melanoma, Halse and colleagues used multiplex immunohistochemistry to provide a model which defines the immune context into four categories, using the presence or absence of PD-L1+ melanoma cells and/or macrophages, and their location within or around the tumor, combined with the presence or absence of intratumoral CD8+ T cells. This model values the melanoma TME as a spectrum between tumor escape and tumor (immune) control within the space of a tissue (30), encouraging others to investigate the spatial distribution of both immune and tumoral cells when interpreting the response to immunotherapy. Confirming the latter, whereas no association with response or survival could be observed in the expression of individual biomarkers (PD-1, PD-L1, IDO-1, HLA-DR), a spatially-resolved low-plex PD-1/PD-L1 interaction score and/or IDO-1/HLA-DR co-expression was strongly associated with an anti–PD-1 response, highlighting the importance of quantitative spatial profiling for multiple features (33). Furthermore, Gide and colleagues examined the spatial distribution of immune and tumor cells using a 5-plex immunofluorescence approach in samples of patients prior to a treatment with either anti-PD-1 monotherapy or a combination of anti-CTLA-4 and anti-PD-1. In a multivariate analysis, the best predictor for a 12-month progression-free survival upon anti-PD-1 monotherapy involved the quantification of the proximity of PD-L1+ immune cells to tumor cells and the density of intratumoral CD8+ T-cell, as such achieving an AUC of 0.80. For the combination therapy, the authors identified that a correlation with the proximity of CD8+ T-cells to tumor cells, the density of intratumoral PD-L1+ cells and LDH expression (AUC = 0.85) to response to therapy (40). Similarly, others have shown that pre-treatment samples obtained from responders to anti-PD1 therapy showed that increased amounts of CD8-, PD-1- and PD-L1+ cells resided at the invasive tumor margins and within the tumor, with close proximity the ligands PD-1 and PD-L1 (24). In addition, the use of multiplexed immunofluorescence highlighted a potential predictive role for specific cancer-associated fibroblasts (39) and CD103+ tumor-resident CD8+ T cells (29) in melanoma patients treated with anti-PD-1 therapy. Also, the potential contributing role of tumor-associated B-cells has been studied using multiplexed immunofluorescence, showing an association between co-occurrence of tumor associated CD8+ T cells and CD20+ B cells with improved survival, while revealing the formation of tertiary lymphoid structures (TLS) in these CD8+CD20+ tumors (42) and their potential role in response to immunotherapy (43). Overall, according to a recently published meta-analyses, the extended information that could be extracted using multiplexed IHC/IF appears to be associated with improved relative diagnostic accuracy in predicting clinical response to anti-PD-1 therapy over the other previously mentioned biomarkers (PD-L1 conventional IHC, TMB, GEP) (73).
Strikingly, most of the research trying to map the melanoma TME were done using “low-plex” methods (less than 10 markers on the same section), and yet the majority of them added interesting insights into the anti-melanoma immune response and response to immunotherapy. The reason why even a low-plex approach can be more insightful compared to other molecular methods (e.g. NGS analysis) which often cover even more parameters, could be related to the insights gained within the spatial component. Indeed, the spatial dimension (i.e. understanding the exact position of each cell type within a tissue) could be considered as a biomarker itself. Available analysis methods are now able to generate cell density metrics for specific tissue regions, assess the distance between various cell types, among many more. Such higher-order insights grant the possibility to go beyond mere cytometric analysis of the tissue (i.e. the overall cellular composition of a tissue) and investigate “cellular sociology” in order to make assumptions about their interactions in particular niches. Moreover, already in the early days of single-cell genomics, it was clear that the success of the different single-cell technologies would depend, in part, on the extent to which researchers preserve the states of cells and the original composition of a tissue (74). After all, most of the initial single-cell methods required cells to be dissociated from the tissue, thereby losing all spatial information while potentially affecting the original cell states. To deal with this flaw, the most recent single-cell methods aim at preserving the cells in their original context and state.
Within the available spatial omics methods, we can distinguish spatial proteomics, transcriptomics and genomics (Figure 2). Most of the spatial proteomics techniques are antibody based methods that achieve their plexability from either multi-spectral imaging and/or iterative imaging of successive antibody staining cycles combined with fluorophore bleaching/inactivation/cleaving or antibody stripping (75–80). Similarly, spatial transcriptomics enable the in-situ visualization of RNA transcripts within the tissue either by measuring pre-determined targets or even global expression data (81–86). A detailed description and comparison of the different available techniques for spatial -omics, goes beyond the scope of this review.
Several of the abovementioned technologies have been applied to melanoma in an attempt to improve prognostic and predictive performance of potential biomarkers, or as a discovery tool to unravel mechanistic insights in the TME. Accordingly, our group previously used MILAN – an imaging/antibody-based single-cell proteomics method - to functionally study tissue architecture, thereby redefining the TIL infiltrate in primary melanoma into a functional classification with an improved prognostic value as compared to the dogmatic morphological classification (44) and described a higher level of interaction between melanoma cells with active CD8+ and CD4+ T cells in patients responding to anti-PD-1 as compared to the non-responding patients (87). Others have used imaging mass cytometry (IMC) with a 25-antibody panel to identify tumor and immune cell markers in melanoma patients treated with immune checkpoint blockers, revealing significant associations of MHC-I, CSF1R, IRF1, LAG-3, PD-1, MHC-II and beta2-microglobulin expression in tumor tissue with progression-free survival, whereas high levels of TIM-3 and PD-L2 in the stroma also predicted response to immunotherapy (52). Spatial transcriptomics have been used to visualize the distribution of mRNA within the melanoma TME, revealing among others the complex transcriptional spatial landscape and genetic heterogeneity in stage III cutaneous melanoma (32).
The Integration of Multi-Omics Within the Tissue
Most of the methods for spatial omics are limited by their ability to examine only one type of analyte (protein or nucleic acids). One step closer toward a complete understanding of the TME of melanoma and the drivers of response to immunotherapy will require the integration of information retrieved from several -omics approaches, each providing complementary information. The integration of information from different omics technologies and particularly those integrating information from the same section at single-cell level while preserving the spatial context represents the ultimate goal for next-generation pathology. In spite of major progress in the development of methodologies that simultaneously extract various features from the same cell, a genuine, spatially integrated multi-omics approach, enabling the simultaneous analysis of proteome, transcriptome, genome- and epigenome within the spatial coordinates is still not available. Therefore, while the development of such combinatory technologies is ongoing, other approaches are being evaluated, including the computational integration of spatially resolved assays (which typically rely on a predefined, but limited set of features), and an unbiased method, such as single-cell RNA-sequencing (scRNAseq) which requires cells to be removed from the tissue. Another simplified approach consists of performing various spatially resolved omics analyses on serial sections, and integrating the findings in a comprehensive framework. As discussed earlier, the use of multiplex immunofluorescence confirmed the importance of tumor-associated B cells and TLS in metastatic melanoma (42, 43), a finding that was further corroborated by spatial transcriptomics, indicating that T cells in TLS-negative tumors had a dysfunctional molecular phenotype (42) whereas TLS-positive tumors are associated with markers of T cell activation and B cell proliferation (43). Alternatively, combining multiple omics on serial sections can be integrated at the tissue level by making use of vertical registration. For example, digitally superimposing a melan-A classical IHC on 1 section and RNAscope probing for hTERT on the adjacent section, allowed these researchers to show a higher expression of hTERT mRNA in melanoma as compared to benign naevi (41). Yet another approach is to laser-microdissect regions from a tissue for gene-expression analysis, while being guided by classical IHC staining on the adjacent section. Such approach has been used to compare expression profiles of IHC-positive and IHC-negative areas, thereby improving cell-type purity in the different samples as compared to classical tissue-based transcriptomic data (21). With the development of Spatially-resolved Transcriptomics via Epitope Anchoring (SvEA) in pivotal work done by Govek and colleagues, this has recently been made possible (88). In this approach, the transcriptomic data acquired via CITE-seq (89) can be mapped to spatially resolved CODEX mIHC data (77), while retaining the single cell spatial resolution by making use of measurements of the same antigens in both methods (88). In spite of the obvious translational potential of this novel approach, it has not yet been applied within the melanoma field. More recently, the GeoMx® DSP (Digital Spatial Profiling) platform has been made commercially available (90). This platform allows protein or RNA quantification within user-defined regions-of-interest (ROI), with the possibility of single-cell resolution ROI selection (The UV laser can be focused as narrow as 10 µm in diameter). This ROI selection is achieved by combining regular, low-plex IF staining together with dozens of primary antibodies or mRNA hybridization probes each covalently attached to indexing oligonucleotides that can be collected for quantification using a UV-photo cleavable linker (91). Using this method, Dikshit and colleagues recently transcriptionally profiled regions with high and low Beta-catenin expression in melanoma, showing a significant correlation with several immune-regulatory targets such as CTLA-4 and PD-1 (49). Similarly, the method was used to show a specific expression profile in fibrotic areas with high macrophage and T cell infiltration in BAP-1 negative uveal melanoma, suggestive of T-cell exhaustion other than PD-1/CTLA-4 engagement, as well as mechanisms of immune exclusion, supporting the clinical observation of immunotherapeutic failure in this subgroup and the need for development of specific treatment approaches (48). Two other studies have used DSP to characterize the tumor expression profile of melanoma patients treated with immune checkpoint blockade in a neoadjuvant setting, showing that baseline immune infiltration was correlated with response to treatment (92, 93). Although offered as an easy applicable method, a detailed understanding of its composition, function and chemistry is advisable to guide experimental design and data interpretation (94).
Finally, AI will have a predominant role in the integration of all the different data types. In the previous section we have discussed how AI has already outperformed pathologists on evaluating morphological features such as mitotic counts (58), Breslow thickness (25) or at detecting lymph node metastases (34, 35) - routine tasks that could significantly enhance the throughput and efficiency of pathologists while ensuring sufficient can be spent on complex cases. However, the strongest ability of AI, and in particular deep learning, is to identify unknown patterns or features that are too complex for pathologists to merely assess by visual eyeballing but which could be of important diagnostic, prognostic, or predictive relevance. This is already true in the case of morphometric features (95). In the case of spatially-resolved, single-cell multi-omics data, the feature space is still significantly larger and the number of hidden associations that could be used as biomarkers is virtually endless. For their translatability to clinical practice however, these complex features require first to be re-engineered to simpler biomarkers or simpler algorithms that identify the specific discriminant features which might be more easily accepted by clinicians (96).
Discussion: Next-Generation Pathology and Its Challenges
Even though next-generation pathology is becoming gradually more prominent and qualifies as a necessity in research, very few of the previously discussed developments have yet been validated for clinical use. With the increasing importance of understanding the immune contexture and the possible development of panels of prognostic/predictive biomarkers across multiple diseases, we foresee that the implementation of next-generation pathology in clinical practice will be mandatory. However, there are still a lot of hurdles and challenges that need to be overcome before multiplexed IHC and digital pathology will be implementable in clinical practice.
The main difficulty of implementing multiplexed IHC is to overcome the common thinking that it is based on the repetition of multiple conventional IHC assays. Indeed, multiplexed IHC is a complex process and there are various challenges that need to be considered. The first challenge is about choosing the most appropriate method. This choice should consider several factors. First of all, the type of samples to be analyzed: some methods require FFPE materials while others can be performed on frozen samples. In a standard clinical pathology lab, FFPE remains the method of choice to preserve tissue specimen, even though multiple methods (mainly multi-omics) require the availability of frozen materials. The second choice will be to related to the actual staining procedure: this can be achieved either by (i) a cyclic method, in which slides are stained multiple times with low-plex antibody cocktails while between every cycle the signal is removed via antibody stripping or bleaching of the fluorophore; or (ii) an all-at-once acquisition, where a cocktail containing all the antibodies of choice are applied on the tissue section in a single step. In the first case the acquisition will be slower, while in the second case the increased speed of acquisition will increase technology costs. For instance, several methods require modified/engineered/conjugated antibodies (e.g. with nucleotide barcodes or metal ions) that are more expensive than conventional clones typically used in routine across clinical pathology labs. On top of this, the instruments that are used to detect the signals are often a factor 2-3 more expensive than conventional autostainers typically used for classical IHC. These machines are generally closed systems that have the advantage to be completely automated, requiring less work by the lab technician being therefore less prone to errors. Nevertheless, these methods may be limited to the acquisition of regions of interest rather than whole slides; depending on the number of antibodies to be detected the acquisition time could require even hours per square mm. A final parameter to consider on the wet-lab part is the number of samples that should be analyzed simultaneously: while some methods allow the analysis of a single slide at the time, others are compatible with batch processing.
The second big challenge of implementing multiplexed IHC in hospital routine relates to data analysis. At the moment, most of the wet-lab methods are not paired with a system to analyze the data. Importantly, multiplexed IHC can no longer be evaluated through mere visual inspection (as opposed to conventional IHC where it is common practice), but requires specific methods for quantitative, spatially resolved analysis. Therefore, until ad hoc software packages will be introduced for specific predictive/prognostic analyses, experts in image analysis and bioinformaticians will remain required for the downstream analysis. In addition, a simple panel of 10 markers will generate 100 digital images when analyzing 10 samples, an amount that will steadily increase when increasing numbers of markers and samples are processed. This is where the hurdles to implement multiplex IHC converge with those of implementing digital pathology.
As described above, digital pathology bears the potential to revolutionize dermatology and dermatopathology. To achieve its implementation, though, multiple challenges have to be overcome, and typically involve hurdles that are cultural, involve validation, available infrastructure and GDPR-related issues. From a cultural point of view, in spite of the advantages listed in the first chapter of this review, pathologists still show some reservations about the use of digital slides for diagnosis, mainly regarding the time needed to evaluate whole digital slides during routine work, with a preference to reserve the digital format for teaching, second opinions and dissemination purposes (31). Second, appropriate validation remains an absolute requirement for any new technique that get implemented for diagnostic purposes. Such validation does not solely happen at the level of a company trying to sell a diagnostic tool, but can also happen directly at a local level, for instance in a pathology department that is willing to introduce a new technique in its workflow. Validation itself will mainly require side-by-side comparisons of manually and digitally interpreted tissue slides (97). Validation is also required for deep learning algorithms, which have the danger to be based on overfitted or miscorrelating data from training sets. In particular, on one side algorithms have to be strictly disease-specific and exclude any other disease that may be encountered during analysis (e.g., algorithms developed for the analysis of melanoma should recognize and revoke the analysis of any other skin tumor). On the other side, since they are extremely dependent on their training, it is important to be aware that if important differences are introduced in time that may artificially change the features of the prospectively collected diagnostic data set, high rates of misclassification can be registered (98). Next to these first two challenges, there are also important infrastructural challenges to be considered that can hamper the implementation of digitization in a clinical institution. A standard microscopy slide, such as the ones routinely used in pathology are typically 75 mm long, 26 mm wide, and approximately 1 mm thick. As the resolution and color depth of digital detectors improve, the size of images that capture these slides keeps on increasing. For a state-of-the-art acquisition instrument with a resolution of 0.44 micrometers/px (20X) and color depth of 16 bit (~65K gray levels), and assuming a 2-dimensional slide with standard dimensions, single images achieve a size of ~20 Gigabytes (Gb). Of course, depending on the size of the scanned area and the type of image compression, whole slide images can range between 0.5 and 4 Gb (96). This is translated to hundreds of Terabytes per year (or even Petabytes when considering a large hospital) that need to be properly acquired, stored, transferred, and processed. Designing and implementing a proper infrastructure for digital pathology that deals with all these tasks is not trivial and key for a successful digital transition. Regarding image acquisition, this is an easily solvable problem, since in the 20 years since the introduction of whole-slide imaging scanners, several of them have been marketed for clinical use in the European Union and in the US (99, 100). Image storage and transfer are instead critical steps and they may require important investments on the side of the institution. There exist different types of solutions for image storage, ranging from local ones such as Direct Attached Storage (DAS), network-based solutions such as Network Attached Storage (NAS), cloud-based solutions (such as Amazon’s S3 Glacier storage for example) or external services (regional supercomputer centers). The last two examples require sending data to third parties which could have GDPR issues (see below). In most cases, several of these solutions need to be simultaneously implemented to archive the data depending on different factors, including access frequency (hot/interactive versus cold/archival storage) or intended use (101). For image transfer, solutions where data is remotely stored or/and the images are remotely analyzed, the speed in which data is transferred (network bandwidth) becomes a critical factor. If we consider a 10 Gb image and a standard Local Area Network (LAN) with a bandwidth of 100Mbit/s, it will take ~15 minutes to transfer the file. Therefore it is crucial to guarantee an environment with sufficient bandwidth prior to taking the step to digital pathology. Finally, most of the digital image analysis algorithms currently used in clinical practice are limited to traditional image analysis and can be used on ordinary computers with Central Processing Units (CPUs) (96). Deep learning algorithms, on the other hand, are heavily dependent on processing acceleration units such as Graphical Processing Units (GPUs) (102). High-end GPUs are very expensive and therefore centers implementing deep learning in digital pathology might choose for a dedicated workstation/server or even to train/run their algorithms in the cloud or in external supercomputer centers. Lately, the development of Tensor Processing Units (TPUs) are allowing the training of deep neural networks (DNN) 15-30 times faster and 30-80 times more energy efficient than contemporary CPUs or GPUs (103). Additional infrastructural challenges to be considered for implementing deep learning in digital pathology include: the number of users of the dedicated computers, the flexibility of the system to implement new algorithms or variable case-loads, implementation/running cost of the facility, cyber-security, data maintenance, etc. (96). A practical example of the implementation process of a fully digital workflow at the University Medical Centre in Utrecht can be found in Stathonikos et al. (104).
The last challenge for digital pathology is correlated with the fact that digital images, as well as patient materials, are subject to the regulation on data protection and privacy in the European Union and the European Economic Area on the protection of natural persons with regard to the processing of personal data and on the free movement of such data (General Data Protection Regulation, GDPR) (105). With respect to digital pathology, it contains several basic principles that digital slides containing human samples must comply with. These include: Purpose specification, the valid legal basis for the collection of the data including the goal for which the data is being collected (106); anonymization or pseudo-anonymization, data is only anonymous when it is impossible to track it to natural persons while pseudo-anonymous data requires extra information to map it to natural persons (107, 108); Data minimization, the collected data should be limited to what is strictly necessary for the scope of the project (107) transparency, the registration of the study and the provision of the relevant information to the subject of the study; storage limitation, the collected data should only be kept as long as needed; and security, the stored data should be processed and stored in such way that it avoids or limits the potential for unlawful processing, accidental loss, destruction or damage (107). This includes technical measures such as badge and password-mediated access control, detailed logs monitoring every ongoing process on the system, data encryption, etc. If the data is stored on the cloud or in external sources, a legal contract needs to be written between the collector and the third party which needs to be checked by legal entities (109, 110).
To conclude, the highly requested demand for a better understanding of the TME in melanoma and its use to further improve the clinical response rates to immunotherapy, the fast-moving technological advancements in machine learning and the rise of spatial omics, have pushed dermatopathology into the digital era. Although the use of digital pathology has already proven to be insightful in melanoma, its exploitation to the full potential by combining spatially resolved single-cell data with artificial intelligence for clinical purposes, is still a rather future perspective. However, such an approach possesses the capability to overcome existing limitations and bring us one step closer to personalized medicine. Nonetheless, despite the many advantages, a lot of the imaging-based methods go along with substantial challenges that need to be addressed before its implementation in daily practice will be possible.
Author Contributions
YH decided the contents, did the main literature research, wrote all the parts about the omics, reviewed the format and the structure of the paper. AA wrote the part on the dry lab challenges and reviewed the format and the structure of the paper. MA researched the topic on GDPR regulations and reviewed the paper. GM created the images and reviewed the paper. OB reviewed the paper from the oncologist point of view. FS reviewed the paper from the bioengineer point of view. FB decided the contents, structured the flow of the paper, gave the pathology insights, wrote the introduction, the part about AI beyond morphology and the part on the discussion about the wet lab challenges. All authors contributed to the article and approved the submitted version.
Funding
AA was supported by the Leuven Kankerinstituut (LKI) and the Opening The Future (OTF) foundation. This work is also supported by the Kom op tegen kanker (KOTK) foundation.
Conflict of Interest
The authors declare that the research was conducted in the absence of any commercial or financial relationships that could be construed as a potential conflict of interest.
References
1. Luikart SD, Kennealey GT, Kirkwood JM. Randomized phase III trial of vinblastine, bleomycin, and cis-dichlorodiammine-platinum versus dacarbazine in malignant melanoma. J Clin Oncol (1984) 2(3):164–8. doi: 10.1200/JCO.1984.2.3.164
2. Cancer Genome Atlas Network. Genomic Classification of Cutaneous Melanoma. Cell (2015) 161(7):1681–96. doi: 10.1016/j.cell.2015.05.044
3. Chapman PB, Hauschild A, Robert C, Haanen JB, Ascierto P, Larkin J. Improved survival with vemurafenib in melanoma with BRAF V600E mutation. N Engl J Med (2011) 364(26):2507–16. doi: 10.1056/NEJMoa1103782
4. Hauschild A, Grob JJ, Demidov LV, Jouary T, Gutzmer R, Millward, et al. Dabrafenib in BRAF-mutated metastatic melanoma: a multicentre, open-label, phase 3 randomised controlled trial. Lancet (2012) 380(9839):358–65. doi: 10.1016/S0140-6736(12)60868-X
5. Flaherty KT, Robert C, Hersey P, Nathan P, Garbe C, Milhem M, et al. Improved survival with MEK inhibition in BRAF-mutated melanoma. N Engl J Med (2012) 367(2):107–14. doi: 10.1056/NEJMoa1203421
6. Larkin J, Ascierto PA, Dréno B, Atkinson V, Liszkay G, Maio M, et al. Combined vemurafenib and cobimetinib in BRAF-mutated melanoma. N Engl J Med (2014) 371(20):1867–76. doi: 10.1056/NEJMoa1408868
7. Robert C, Karaszewska B, Schachter J, Rutkowski P, Mackiewicz A, Stroiakovski D, et al. Improved overall survival in melanoma with combined dabrafenib and trametinib. N Engl J Med (2015) 372(1):30–9. doi: 10.1056/NEJMoa1412690
8. Long GV, Stroyakovskiy D, Gogas H, Levchenko E, de Braud F, Larkin J, et al. Combined BRAF and MEK inhibition versus BRAF inhibition alone in melanoma. N Engl J Med (2014) 371(20):1877–88. doi: 10.1056/NEJMoa1406037
9. Dummer R, Ascierto PA, Gogas HJ, Arance A, Mandala M, Liszkay G, et al. Encorafenib plus binimetinib versus vemurafenib or encorafenib in patients with BRAF-mutant melanoma (COLUMBUS): a multicentre, open-label, randomised phase 3 trial. Lancet Oncol (2018) 19(5):603–15. doi: 10.1016/S1470-2045(18)30142-6
10. Hodi FS, Corless CL, Giobbie-Hurder A, Fletcher JA, Zhu M, Marino-Enriquez A, et al. Imatinib for melanomas harboring mutationally activated or amplified KIT arising on mucosal, acral, and chronically sun-damaged skin. J Clin Oncol (2013) 31(26):3182–90. doi: 10.1200/JCO.2012.47.7836
11. Atkins MB, Lotze MT, Dutcher JP, Fisher RI, Weiss G, Margolin K, et al. High-dose recombinant interleukin 2 therapy for patients with metastatic melanoma: analysis of 270 patients treated between 1985 and 1993. J Clin Oncol (1999) 17(7):2105–16. doi: 10.1200/JCO.1999.17.7.2105
12. Schwartzentruber DJ, Lawson DH, Richards JM, Conry RM, Miller DM, Treisman J, et al. gp100 peptide vaccine and interleukin-2 in patients with advanced melanoma. N Engl J Med (2011) 364(22):2119–27. doi: 10.1056/NEJMoa1012863
13. Smith FO, Downey SG, Klapper JA, Yang JC, Sherry RM, Royal RE, et al. Treatment of metastatic melanoma using interleukin-2 alone or in conjunction with vaccines. Clin Cancer Res (2008) 14(17):5610–8. doi: 10.1158/1078-0432.CCR-08-0116
14. Robert C, Thomas L, Bondarenko I, O’Day S, Weber J, Garbe C, et al. Ipilimumab plus dacarbazine for previously untreated metastatic melanoma. N Engl J Med (2011) 364(26):2517–26. doi: 10.1056/NEJMoa1104621
15. Robert C, Long GV, Brady B, Dutriaux C, Maio M, Mortier L, et al. Nivolumab in previously untreated melanoma without BRAF mutation. N Engl J Med (2015) 372(4):320–30. doi: 10.1056/NEJMoa1412082
16. Robert C, Schachter J, Long GV, Arance A, Grob JJ, Mortier L, et al. KEYNOTE-006 investigators. Pembrolizumab versus Ipilimumab in Advanced Melanoma. N Engl J Med (2015) 372(26):2521–32. doi: 10.1056/NEJMoa1503093
17. Larkin J, Chiarion-Sileni V, Gonzalez R, Grob JJ, Cowey CL, Lao CD, et al. Combined Nivolumab and Ipilimumab or Monotherapy in Untreated Melanoma. N Engl J Med (2015) 373(1):23–34. doi: 10.1056/NEJMoa1504030
18. Salto-Tellez M, Maxwell P, Hamilton P. Artificial intelligence-the third revolution in pathology. Histopathology (2019) 74(3):372–6. doi: 10.1111/his.13760
19. Elder DE, Massi D, Scolyer R, Willemze R. WHO Classification of Skin Tumours. 4th Edition. Lyon: IARC (2018).
21. Makhzami S, Rambow F, Delmas V, Larue L. Efficient gene expression profiling of laser-microdissected melanoma metastases. Pigment Cell Melanoma Res (2012) 25(6):783–91. doi: 10.1111/pcmr.12013
22. Bifulco C, Capone M, Feng Z, Madonna G, Simeone E, Curvietto M, et al. MISIPI study: Melanoma ImmunoScore evaluation in patients treated with IPIlimumab. J Transl Med (2014) 12(Suppl 1):P11. doi: 10.1186/1479-5876-12-S1-P11
23. Capone M, Madonna G, Sebastiao N, Bird J, Ayala F, Caracò C, et al. Immunoscore: a new possible approach for melanoma classification. J Immunother Cancer (2014) 2(Suppl 3):P193. doi: 10.1186/2051-1426-2-S3-P193
24. Tumeh PC, Harview CL, Yearley JH, Shintaku IP, Taylor EJ, Robert L, et al. PD-1 blockade induces responses by inhibiting adaptive immune resistance. Nature (2014) 515(7528):568–71. doi: 10.1038/nature13954
25. Xu H, Berendt R, Jha N, Mandal M. Automatic measurement of melanoma depth of invasion in skin histopathological images. Micron (2017) 97:56–67. doi: 10.1016/j.micron.2017.03.004
26. Fertig RM, Gaudi S, Cervantes J, Maddy A, Sangueza O, Vu J, et al. Feasibility study in teledermatopathology: An examination of the histopathologic features of mycosis fungoides and spongiotic dermatitis. J Cutan Pathol (2017) 44(11):919–24. doi: 10.1111/cup.13018
27. Kent MN, Olsen TG, Feeser TA, Tesno KC, Moad JC, Conroy MP, et al. Diagnostic Accuracy of Virtual Pathology vs Traditional Microscopy in a Large Dermatopathology Study. JAMA Dermatol (2017) 153(12):1285–91. doi: 10.1001/jamadermatol.2017.3284
28. Xu H, Lu C, Berendt R, Jha N, Mandal M. Automated analysis and classification of melanocytic tumor on skin whole slide images. Comput Med Imaging Graph (2018) 66:124–34. doi: 10.1016/j.compmedimag.2018.01.008
29. Edwards J, Wilmott JS, Madore J, Gide TN, Quek C, Tasker A, et al. CD103+ Tumor-Resident CD8+ T Cells Are Associated with Improved Survival in Immunotherapy-Naïve Melanoma Patients and Expand Significantly During Anti-PD-1 Treatment. Clin Cancer Res (2018) 24(13):3036–45. doi: 10.1158/1078-0432.CCR-17-2257
30. Halse H, Colebatch AJ, Petrone P, Henderson MA, Mills JK, Snow H, et al. Multiplex immunohistochemistry accurately defines the immune context of metastatic melanoma. Sci Rep (2018) 8(1):11158. doi: 10.1038/s41598-018-28944-3
31. Onega T, Reisch LM, Frederick PD, Geller BM, Nelson HD, Lott JP, et al. Use of Digital Whole Slide Imaging in Dermatopathology. J Digit Imaging (2016) 29(2):243–53. doi: 10.1007/s10278-015-9836-y
32. Thrane K, Eriksson H, Maaskola J, Hansson J, Lundeberg J. Spatially Resolved Transcriptomics Enables Dissection of Genetic Heterogeneity in Stage III Cutaneous Malignant Melanoma. Cancer Res (2018) 78(20):5970–9. doi: 10.1158/0008-5472.CAN-18-0747
33. Johnson DB, Bordeaux J, Kim JY, Vaupel C, Rimm DL, Ho TH, et al. Quantitative Spatial Profiling of PD-1/PD-L1 Interaction and HLA-DR/IDO-1 Predicts Improved Outcomes of Anti-PD-1 Therapies in Metastatic Melanoma. Clin Cancer Res (2018) 24(21):5250–60. doi: 10.1158/1078-0432.CCR-18-0309
34. Alheejawi S, Mandal M, Berendt R, Jha N. Automated Melanoma Staging in Lymph Node Biopsy Image using Deep Learning, 2019 IEEE Canadian Conference of Electrical and Computer Engineering (CCECE). Edmonton, AB, Canada (2019). p. 1–4. doi: 10.1109/CCECE.2019.8861878
35. Alheejawi S, Xu H, Berendt R, Jha N, Mandal M. Novel lymph node segmentation and proliferation index measurement for skin melanoma biopsy images. Comput Med Imaging Graph (2019) 73:19–29. doi: 10.1016/j.compmedimag.2019.01.006
36. Fu Q, Chen N, Ge C, Li R, Li Z, Zeng B, et al. Prognostic value of tumor-infiltrating lymphocytes in melanoma: a systematic review and meta-analysis. Oncoimmunology (2019) 8(7):1593806. doi: 10.1080/2162402X.2019.1593806
37. Wong PF, Wei W, Smithy JW, Acs B, Toki MI, Blenman KRM, et al. Multiplex Quantitative Analysis of Tumor-Infiltrating Lymphocytes and Immunotherapy Outcome in Metastatic Melanoma. Clin Cancer Res (2019) 25(8):2442–9. doi: 10.1158/1078-0432.CCR-18-2652
38. Robinson E, Kulkarni PM, Pradhan JS, Gartrell RD, Yang C, Rizk EM, et al. Prediction of distant melanoma recurrence from primary tumor digital H&E images using deep learning. J Clin Oncol (2019) 37(15_suppl):9577–7. doi: 10.1200/JCO.2019.37.15_suppl.9577
39. Wong PF, Wei W, Gupta S, Smithy JW, Zelterman D, Kluger HM, et al. Multiplex quantitative analysis of cancer-associated fibroblasts and immunotherapy outcome in metastatic melanoma. J Immunother Cancer (2019) 7(1):194. doi: 10.1186/s40425-019-0675-0
40. Gide TN, Silva IP, Quek C, Ahmed T, Menzies AM, Carlino MS, et al. Close proximity of immune and tumor cells underlies response to anti-PD-1 based therapies in metastatic melanoma patients. Oncoimmunology (2019) 9(1):1659093. doi: 10.1080/2162402X.2019.1659093
41. Baltzarsen PB, Georgsen JB, Nielsen PS, Steiniche T, Stougaard M. Detection of mRNA of Telomerase Protein in Benign Naevi and Melanomas Using RNAscope. Appl Immunohistochem Mol Morphol (2020) 28(1):36–41. doi: 10.1097/PAI.0000000000000690
42. Cabrita R, Lauss M, Sanna A, Donia M, Skaarup Larsen M, Mitra S, et al. Tertiary lymphoid structures improve immunotherapy and survival in melanoma. Nature (2020) 577(7791):561–5. doi: 10.1038/s41586-019-1914-8
43. Helmink BA, Reddy SM, Gao J, Zhang S, Basar R, Thakur R, et al. B cells and tertiary lymphoid structures promote immunotherapy response. Nature (2020) 577(7791):549–55. doi: 10.1038/s41586-019-1922-8
44. Bosisio FM, Antoranz A, van Herck Y, Bolognesi MM, Marcelis L, Chinello C, et al. Functional heterogeneity of lymphocytic patterns in primary melanoma dissected through single-cell multiplexing. Elife (2020) 9:e53008. doi: 10.7554/eLife.53008
45. Ianni JD, Soans RE, Sankarapandian S, Chamarthi RV, Ayyagari D, Olsen TG, et al. Tailored for Real-World: A Whole Slide Image Classification System Validated on Uncurated Multi-Site Data Emulating the Prospective Pathology Workload. Sci Rep (2020) 10(1):3217. doi: 10.1038/s41598-020-59985-2
46. Chou M, Illa-Bochaca I, Minxi B, Darvishian F, Johannet P, Moran U, et al. Optimization of an automated tumor-infiltrating lymphocyte algorithm for improved prognostication in primary melanoma. Mod Pathol (2021) 34(3):562–71. doi: 10.1038/s41379-020-00686-6
47. Kucharski D, Kleczek P, Jaworek-Korjakowska J, Dyduch G, Gorgon M. Semi-Supervised Nests of Melanocytes Segmentation Method Using Convolutional Autoencoders. Sensors (Basel) (2020) 20(6):1546. doi: 10.3390/s20061546
48. Figueiredo CR, Kalirai H, Sacco JJ, Azevedo RA, Duckworth A, Slupsky JR, et al. Loss of BAP1 expression is associated with an immunosuppressive microenvironment in uveal melanoma, with implications for immunotherapy development. J Pathol (2020) 250(4):420–39. doi: 10.1002/path.5384
49. Dikshit A, Zollinger A, Merritt C, Nguyen K, McKay-Fleisch J, Anderson C, et al. Molecularly guided highly multiplexed digital spatial analysis reveals differential gene expression profiles in the WNT-β-catenin pathway between melanoma and prostate tumors. Cancer Res (2020) 80(16 Supplement):2707. doi: 10.1158/1538-7445.AM2020-2707
50. Klein S, Mauch C, Brinker K, Noh KW, Knez S, Büttner R, et al. Tumor infiltrating lymphocyte clusters are associated with response to immune checkpoint inhibition in BRAF V600E/K mutated malignant melanomas. Sci Rep (2021) 11(1):1834. doi: 10.1038/s41598-021-81330-4
51. Moore MR, Friesner ID, Rizk EM, Fullerton BT, Mondal M, Trager MH, et al. Automated digital TIL analysis (ADTA) adds prognostic value to standard assessment of depth and ulceration in primary melanoma. Sci Rep (2021) 11(1):2809. doi: 10.1038/s41598-021-82305-1
52. Martinez-Morilla S, Villarroel-Espindola F, Wong PF, Kluger H, Toki M, Aung TN, et al. (2020). Biomarker discovery in immunotherapy-treated melanoma patients with imaging mass cytometry [abstract], in: Proceedings of the Annual Meeting of the American Association for Cancer Research 2020, Philadelphia (PA): AACR, 2020 Apr 27-28 and Jun 22-24, Vol. 80. p. Abstract nr 2001, Cancer Res.
53. Elmore JG, Barnhill RL, Elder DE, Longton GM, Pepe MS, Reisch LM, et al. Pathologists’ diagnosis of invasive melanoma and melanocytic proliferations: observer accuracy and reproducibility study. BMJ (2017) 357:j2813. doi: 10.1136/bmj.j2813
54. Farmer ER, Gonin R, Hanna MP. Discordance in the histopathologic diagnosis of melanoma and melanocytic nevi between expert pathologists. Hum Pathol (1996) 27(6):528–31. doi: 10.1016/s0046-8177(96)90157-4
55. Elder DE, Piepkorn MW, Barnhill RL, Longton GM, Nelson HD, Knezevich SR, et al. Pathologist characteristics associated with accuracy and reproducibility of melanocytic skin lesion interpretation. J Am Acad Dermatol (2018) 79(1):52–59.e5. doi: 10.1016/j.jaad.2018.02.070
56. Onega T, Barnhill RL, Piepkorn MW, Longton GM, Elder DE, Weinstock MA, et al. Accuracy of Digital Pathologic Analysis vs Traditional Microscopy in the Interpretation of Melanocytic Lesions. JAMA Dermatol (2018) 154(10):1159–66. doi: 10.1001/jamadermatol.2018.2388
57. Kalra S, Tizhoosh HR, Shah S, Choi C, Damaskinos S, Safarpoor A, et al. Pan-cancer diagnostic consensus through searching archival histopathology images using artificial intelligence. NPJ Digit Med (2020) 3:31. doi: 10.1038/s41746-020-0238-2
58. Cireşan DC, Giusti A, Gambardella LM, Schmidhuber J. Mitosis detection in breast cancer histology images with deep neural networks. Med Image Comput Comput Assist Interv (2013) 16(Pt 2):411–8. doi: 10.1007/978-3-642-40763-5_51
59. Filosa A, Filosa G. Melanoma Diagnosis: The Importance of Histopathological Report. Dermatopathol (Basel) (2018) 5(1):41–3. doi: 10.1159/000486670
60. Larkin J, Chiarion-Sileni V, Gonzalez R, Grob JJ, Rutkowski P, Lao CD, et al. Five-Year Survival with Combined Nivolumab and Ipilimumab in Advanced Melanoma. N Engl J Med (2019) 381(16):1535–46. doi: 10.1056/NEJMoa1910836
61. Rotte A. Combination of CTLA-4 and PD-1 blockers for treatment of cancer. J Exp Clin Cancer Res (2019) 38(1):255. doi: 10.1186/s13046-019-1259-z
62. Daud AI, Wolchok JD, Robert C, Hwu WJ, Weber JS, Ribas A, et al. Programmed Death-Ligand 1 Expression and Response to the Anti-Programmed Death 1 Antibody Pembrolizumab in Melanoma. J Clin Oncol (2016) 34(34):4102–9. doi: 10.1200/JCO.2016.67.2477
63. Morrison C, Pabla S, Conroy JM, Nesline MK, Glenn ST, Dressman D, et al. Predicting response to checkpoint inhibitors in melanoma beyond PD-L1 and mutational burden. J Immunother Cancer (2018) 6(1):32. doi: 10.1186/s40425-018-0344-8
64. Hugo W, Zaretsky JM, Sun L, Song C, Moreno BH, Hu-Lieskovan S, et al. Genomic and Transcriptomic Features of Response to Anti-PD-1 Therapy in Metastatic Melanoma. Cell (2016) 165(1):35–44. doi: 10.1016/j.cell.2016.02.065
65. Auslander N, Zhang G, Lee JS, Frederick DT, Miao B, Moll T, et al. Robust prediction of response to immune checkpoint blockade therapy in metastatic melanoma. Nat Med (2018) 24(10):1545–9. doi: 10.1038/s41591-018-0157-9
66. HistoGeneX. Histo Highlights (2016). Available at: https://www.histogenex.com/images/PDFs/Histo-Highlights-July-2016-HistoGeneX-Newsletter.pdf (Accessed November 25, 2020).
67. Høiberg S. Feature-based registration of sectional images. European patent specification EP2095332B1. Munchen: Germany: European Patent Office (2009).
68. Visiopharm. High-quality alignment of serial sections (2020). Available at: https://visiopharm.com/visiopharm-digital-image-analysis-software-features/tissuealign/ (Accessed November 25, 2020).
69. Shakya R, Nguyen TH, Waterhouse N, Khanna R. Immune contexture analysis in immuno-oncology: applications and challenges of multiplex fluorescent immunohistochemistry. Clin Transl Immunol (2020) 9(10):e1183. doi: 10.1002/cti2.1183
70. Galon J, Costes A, Sanchez-Cabo F, Kirilovsky A, Mlecnik B, Lagorce-Pagès C, et al. Type, density, and location of immune cells within human colorectal tumors predict clinical outcome. Science (2006) 313(5795):1960–4. doi: 10.1126/science.1129139
71. Galon J, Mlecnik B, Bindea G, Angell HK, Berger A, Lagorce C, et al. Towards the introduction of the ‘Immunoscore’ in the classification of malignant tumours. J Pathol (2014) 232(2):199–209. doi: 10.1002/path.4287
72. Galon J, Fox BA, Bifulco CB, Masucci G, Rau T, Botti, et al. Immunoscore and Immunoprofiling in cancer: an update from the melanoma and immunotherapy bridge 2015. J Transl Med (2016) 14:273. doi: 10.1186/s12967-016-1029-z
73. Lu S, Stein JE, Rimm DL, Wang DW, Bell JM, Johnson DB, et al. Comparison of Biomarker Modalities for Predicting Response to PD-1/PD-L1 Checkpoint Blockade: A Systematic Review and Meta-analysis. JAMA Oncol (2019) 5(8):1195–204. doi: 10.1001/jamaoncol.2019.1549
74. Giladi A, Amit I. Immunology, one cell at a time. Nature (2017) 547(7661):27–9. doi: 10.1038/547027a
75. Giesen C, Wang HA, Schapiro D, Zivanovic N, Jacobs A, Hattendorf B, et al. Highly multiplexed imaging of tumor tissues with subcellular resolution by mass cytometry. Nat Methods (2014) 11(4):417–22. doi: 10.1038/nmeth.2869
76. Angelo M, Bendall SC, Finck R, Hale MB, Hitzman C, Borowsky AD, et al. Multiplexed ion beam imaging of human breast tumors. Nat Med (2014) 20(4):436–42. doi: 10.1038/nm.3488
77. Goltsev Y, Samusik N, Kennedy-Darling J, Bhate S, Hale M, Vazquez G, et al. Deep Profiling of Mouse Splenic Architecture with CODEX Multiplexed Imaging. Cell (2018) 174(4):968–981.e15. doi: 10.1016/j.cell.2018.07.010
78. Lin JR, Fallahi-Sichani M, Sorger PK. Highly multiplexed imaging of single cells using a high-throughput cyclic immunofluorescence method. Nat Commun (2015) 6:8390. doi: 10.1038/ncomms9390
79. Bolognesi MM, Manzoni M, Scalia CR, Zannella S, Bosisio FM, Faretta M, et al. G. Multiplex Staining by Sequential Immunostaining and Antibody Removal on Routine Tissue Sections. J Histochem Cytochem (2017) 65(8):431–44. doi: 10.1369/0022155417719419
80. De Smet F, Antoranz Martinez A, Bosisio FM. Next-Generation Pathology by Multiplexed Immunohistochemistry. Trends Biochem Sci (2020) 20:S0968–0004(20)30245-0. doi: 10.1016/j.tibs.2020.09.009
81. Rodriques SG, Stickels RR, Goeva A, Martin CA, Murray E, Vanderburg CR, et al. Slide-seq: A scalable technology for measuring genome-wide expression at high spatial resolution. Science (2019) 363(6434):1463–7. doi: 10.1126/science.aaw1219
82. Wang F, Flanagan J, Su N, Wang LC, Bui S, Nielson A, et al. RNAscope: a novel in situ RNA analysis platform for formalin-fixed, paraffin-embedded tissues. J Mol Diagn (2012) 14(1):22–9. doi: 10.1016/j.jmoldx.2011.08.002
83. Nagendran M, Riordan DP, Harbury PB, Desai TJ. Automated cell-type classification in intact tissues by single-cell molecular profiling. Elife (2018) 7:e30510. doi: 10.7554/eLife.30510
84. Eng CL, Lawson M, Zhu Q, Dries R, Koulena N, Takei Y, et al. Transcriptome-scale super-resolved imaging in tissues by RNA seqFISH. Nature (2019) 568(7751):235–9. doi: 10.1038/s41586-019-1049-y
85. Zollinger DR, Lingle SE, Sorg K, Beechem JM, Merritt CR. GeoMx™ RNA Assay: High Multiplex, Digital, Spatial Analysis of RNA in FFPE Tissue. Methods Mol Biol (2020) 2148:331–45. doi: 10.1007/978-1-0716-0623-0_21
86. Lee JH, Daugharthy ER, Scheiman J, Kalhor R, Yang JL, Ferrante TC, et al. Highly multiplexed subcellular RNA sequencing in situ. Science (2014) 343(6177):1360–3. doi: 10.1126/science.1250212
87. Bosisio F, Antoranz A, van Herck Y, Bolognesi M, Lynch S, Rahman A, et al. (2020). High Resolution Multiplexing of Melanoma Microenvironment in Responders/Non-Responders to Checkpoint Therapy, in: MODERN PATHOLOGY: vol. 33(SUPPL 2), (457-458). Presented at the 109th Annual Meeting of the United-States-and-Canadian-Academy-of-Pathology (USCAP), Los Angeles, CA, 29 Feb 2020-05 Mar 2020.
88. Govek KW, Troisi EC, Miao Z, Woodhouse S, Camara PG. Single-Cell Transcriptomic Analysis of mIHC Images via Antigen Mapping. bioRxiv (2020), 672501. doi: 10.1101/672501
89. Stoeckius M, Hafemeister C, Stephenson W, Houck-Loomis B, Chattopadhyay PK, Swerdlow H, et al. Simultaneous epitope and transcriptome measurement in single cells. Nat Methods (2017) 14(9):865–8. doi: 10.1038/nmeth.4380
90. GeoMx Digital Spatial Profiling | NanoString Technologies. Available at: https://www.nanostring.com/products/geomx-digital-spatial-profiler/geomx-dsp, SITE.
91. Merritt CR, Ong GT, Church SE, Barker K, Danaher P, Geiss G, et al. Multiplex digital spatial profiling of proteins and RNA in fixed tissue. Nat Biotechnol (2020) 38(5):586–99. doi: 10.1038/s41587-020-0472-9
92. Amaria RN, Reddy SM, Tawbi HA, Davies MA, Ross MI, Glitza IC, et al. Neoadjuvant immune checkpoint blockade in high-risk resectable melanoma. Nat Med (2018) 24(11):1649–54. doi: 10.1038/s41591-018-0197-1
93. Blank CU, Rozeman EA, Fanchi LF, Sikorska K, van de Wiel B, Kvistborg P, et al. Neoadjuvant versus adjuvant ipilimumab plus nivolumab in macroscopic stage III melanoma. Nat Med (2018) 24(11):1655–61. doi: 10.1038/s41591-018-0198-0
94. Decalf J, Albert ML, Ziai J. New tools for pathology: a user’s review of a highly multiplexed method for in situ analysis of protein and RNA expression in tissue. J Pathol (2019) 247(5):650–61. doi: 10.1002/path.5223
95. Dong F, Irshad H, Oh EY, Lerwill MF, Brachtel EF, Jones NC, et al. Computational pathology to discriminate benign from malignant intraductal proliferations of the breast. PloS One (2014) 9(12):e114885. doi: 10.1371/journal.pone.0114885
96. Abels E, Pantanowitz L, Aeffner F, Zarella MD, van der Laak J, Bui MM, et al. Computational pathology definitions, best practices, and recommendations for regulatory guidance: a white paper from the Digital Pathology Association. J Pathol (2019) 249(3):286–94. doi: 10.1002/path.5331
97. Pantanowitz L, Sinard JH, Henricks WH, Fatheree LA, Carter AB, Contis L, et al. Validating whole slide imaging for diagnostic purposes in pathology: guideline from the College of American Pathologists Pathology and Laboratory Quality Center. Arch Pathol Lab Med (2013) 137(12):1710–22. doi: 10.5858/arpa.2013-0093-CP
98. Esteva A, Topol E. Can skin cancer diagnosis be transformed by AI? Lancet (2019) 394(10211):1795. doi: 10.1016/S0140-6736(19)32726-6
99. Pantanowitz L, Sharma A, Carter AB, Kurc T, Sussman A, Saltz J. Twenty Years of Digital Pathology: An Overview of the Road Travelled, What is on the Horizon, and the Emergence of Vendor-Neutral Archives. J Pathol Inform (2018) Nov 219:40. doi: 10.4103/jpi.jpi_69_18
100. Evans AJ, Bauer TW, Bui MM, Cornish TC, Duncan H, Glassy EF, et al. US Food and Drug Administration Approval of Whole Slide Imaging for Primary Diagnosis: A Key Milestone Is Reached and New Questions Are Raised. Arch Pathol Lab Med (2018) 142(11):1383–7. doi: 10.5858/arpa.2017-0496-CP
101. Zarella MD, Bowman D, Aeffner F, Farahani N, Xthona A, Absar SF, et al. A Practical Guide to Whole Slide Imaging: A White Paper From the Digital Pathology Association. Arch Pathol Lab Med (2019) 143(2):222–34. doi: 10.5858/arpa.2018-0343-RA
102. Tizhoosh HR, Pantanowitz L. Artificial Intelligence and Digital Pathology: Challenges and Opportunities. J Pathol Inform (2018) 9:38. doi: 10.4103/jpi.jpi_53_18
103. Jouppi N, Young C, Patil N, Patterson D. Motivation for and Evaluation of the First Tensor Processing Unit. IEEE Micro (2018) 38(3):10–9. doi: 10.1109/MM.2018.032271057
104. Stathonikos N, Nguyen TQ, Spoto CP, Verdaasdonk MAM, van Diest PJ. Being fully digital: perspective of a Dutch academic pathology laboratory. Histopathology (2019) 75(5):621–35. doi: 10.1111/his.13953
105. EUR-Lex - 32016R0679 - EN - EUR-Lex. Available at: https://eur-lex.europa.eu/eli/reg/2016/679/oj.
106. Art. 89 GDPR – Safeguards and derogations relating to processing for archiving purposes in the public interest, scientific or historical research purposes or statistical purposes | General Data Protection Regulation (GDPR). Available at: https://gdpr-info.eu/art-89-gdpr/.
107. Art. 5 GDPR – Principles relating to processing of personal data. In: . General Data Protection Regulation (GDPR). Available at: https://gdpr-info.eu/art-5-gdpr/.
108. Art. 6 GDPR – Lawfulness of processing. In: . General Data Protection Regulation (GDPR). Available at: https://gdpr-info.eu/art-6-gdpr/.
109. Art. 40 GDPR – Codes of conduct. In: . General Data Protection Regulation (GDPR). Available at: https://gdpr-info.eu/art-40-gdpr/.
110. Art. 45 GDPR – Transfers on the basis of an adequacy decision. In: . General Data Protection Regulation (GDPR). Available at: https://gdpr-info.eu/art-45-gdpr/.
Keywords: melanoma, multiplex, single cell, digital pathology, spatial proteomics
Citation: Van Herck Y, Antoranz A, Andhari MD, Milli G, Bechter O, De Smet F and Bosisio FM (2021) Multiplexed Immunohistochemistry and Digital Pathology as the Foundation for Next-Generation Pathology in Melanoma: Methodological Comparison and Future Clinical Applications. Front. Oncol. 11:636681. doi: 10.3389/fonc.2021.636681
Received: 01 December 2020; Accepted: 12 March 2021;
Published: 29 March 2021.
Edited by:
Igor Puzanov, University at Buffalo, United StatesReviewed by:
Selma Ugurel, University of Duisburg-Essen, GermanyMatthew D. Vesely, Yale University, United States
Copyright © 2021 Van Herck, Antoranz, Andhari, Milli, Bechter, De Smet and Bosisio. This is an open-access article distributed under the terms of the Creative Commons Attribution License (CC BY). The use, distribution or reproduction in other forums is permitted, provided the original author(s) and the copyright owner(s) are credited and that the original publication in this journal is cited, in accordance with accepted academic practice. No use, distribution or reproduction is permitted which does not comply with these terms.
*Correspondence: Francesca Maria Bosisio, ZnJhbmNlc2NhbWFyaWEuYm9zaXNpb0BrdWxldXZlbi5iZQ==
†These authors share first authorship
‡These authors share last authorship