- 1Department of Medical Oncology, Beijing Tuberculosis and Thoracic Tumor Research Institute, Beijing Chest Hospital, Capital Medical University, Beijing, China
- 2Department of Radiology, Beijing Tuberculosis and Thoracic Tumor Research Institute, Beijing Chest Hospital, Capital Medical University, Beijing, China
- 3Research and Development, Nanjing Geneseeq Technology Inc., Nanjing, China
- 4Cancer Research Center, Beijing Tuberculosis and Thoracic Tumor Research Institute, Beijing Chest Hospital, Capital Medical University, Beijing, China
Purpose: Circulating cell-free DNA (cfDNA) level has been demonstrated to be associated with efficacy in first generation EGFR TKIs in non-small cell lung cancer (NSCLC). However, the role of dynamic cfDNA analysis using next-generation sequencing (NGS) in patients with subsequent third-generation EGFR TKIs remains unclear.
Methods: From 2016 to 2019, 81 NSCLC patients with EGFR T790M mutation either in tissue or plasma who received third-generation EGFR TKIs treatment were enrolled. CfDNA were sequenced by NGS with a 425-gene panel. The association of clinical characteristics, pretreatment, dynamic cfDNA and T790M level with outcomes in patients treated with the third-generation TKIs were analyzed.
Results: In univariate analysis, the median PFS of patients with undetectable cfDNA level during treatment was significantly longer than those with detectable cfDNA (16.97 vs. 6.10 months; HR 0.2109; P < 0.0001). The median PFS of patients with undetectable T790M level during treatment was significantly longer than those with detectable T790M (14.1 vs. 4.4 months; HR 0.2192; P < 0.001). Cox hazard proportion model showed that cfDNA clearance was an independent predictor for longer PFS (HR 0.3085; P < 0.001) and longer OS (HR 0.499; P = 0.034). The most common resistant mutations of the third-generation TKIs were EGFR C797S (24%). CDK6 CNV, GRIN2A, BRCA2, EGFR D761N, EGFR Q791H, EGFR V843I, and ERBB4 mutation genes may possibly be new resistant mechanisms.
Conclusions: Patients with undetectable cfDNA during the third-generation EGFR TKI treatment have superior clinical outcomes, and dynamic cfDNA analysis by NGS is valuable to explore potential resistant mechanisms.
Introduction
EGFR gene mutations account for the most frequency of oncogenic mutations in advanced non-small cell lung cancer (NSCLC). The first-generation EGFR TKIs, gefitinib, erlotinib, icotinib, have been the standard-of-care as the first-line treatment in China for advanced NSCLC with EGFR-sensitizing mutations. Unfortunately, acquired resistance still occurs in the majority of patients. About 50–60% resistant mechanism of the first generation EGFR TKIs was EGFR T790M gene mutation. Third-generation EGFR TKIs, such as osimertinib, alflutinib, target the T790M mutation with outstanding clinical efficacy (1, 2).
As a viable alternative for tissue biopsy, liquid biopsy with circulating cell-free DNA (cfDNA) analysis has been used in identifying molecular target, predicting response and prognosis, and monitoring resistance during targeted treatment for lung cancer (3). cfDNA clearance during anticancer therapies has been an emerging topic in lung cancer, especially in EGFR-mutated NSCLCs. Previous studies have reported a promising role of cfDNA longitudinal monitoring using different liquid biopsy methodologies (4–7). The longitudinal cfDNA monitoring has been proposed as a follow-up tool in the identification of acquired mechanisms of resistance that can impact sequential treatment management (5, 8). Furthermore, several studies have reported cfDNA clearance as a potential predictive biomarker for NSCLC patients during immunotherapy (9).
The role of cfDNA dynamic changing in predicting the response to the third-generation EGFR TKIs has been investigated in small-scale studies with limited sample size using PCR technique (10). Due to the limitation of obtaining tissue samples at disease progression (PD), ctDNA pretreatment and at PD time point provide hints for better understanding the EGFR TKI resistant mechanisms (11, 12). Compared to conventional PCR-based testing, next-generation sequencing (NGS) is a more sensitive and comprehensive technique for detecting uncommon actionable mutations including acquired resistance alterations. Currently, there is a lack of research on dynamic detection of cfDNA by NGS in patients treated with the third-generation EGFR TKI, which limited the exploration of resistant mechanisms and the formulation of subsequent treatment regimens (13).
In this study, 81 EGFR-mutant advanced NSCLC patients who were confirmed to have acquired T790M mutation were enrolled, and the clinical implications of dynamic plasma cfDNA analysis using a 425-gene capture panel during the third-generation TKI treatment was investigated. The results implied a broad potential of cfDNA as an adjuvant tool in practical clinical management, regarding both prognosis prediction and resistant mechanism identification.
Methods
Patient Cohort
Advanced NSCLC patients with EGFR T790M identified in the tissue or plasma by NGS and prior-first-generation EGFR TKI treatment were enrolled. All patients received the third-generation TKIs sequentially. Key exclusion criteria were cases without NGS testing for tissue or plasma, or without disease evaluation. The study was approved by the ethics committee of Beijing Chest Hospital, and all patients had signed written informed consent. Clinical staging complied with the AJCC 8th staging system, and treatment response was assessed with the Response Evaluation Criteria in Solid Tumors (RECIST), version 1.1. Blood samples were collected at the point of pretreatment and during the treatment of the third-generation EGFR TKIs.
DNA Extraction and Targeted Next-Generation Sequencing
Genomic DNA from FFPE samples and white blood cells were extracted using the QIAamp DNA FFPE Tissue Kit (Qiagen). Plasma was extracted from about 10 ml whole blood in EDTA-coated tubes within 2 h of blood withdrawing, and circulating cell free DNA (cfDNA) was extracted using the QIAamp Circulating Nucleic Acid Kit (QIAGEN). Genomic DNA from white blood cells was extracted using DNeasy Blood & Tissue Kit (Qiagen, Germany) and used as normal control. All DNA concentration and purity were qualified by Nanodrop2000 (Thermo Fisher Scientific). All DNA samples were also quantified by Qubit 3.0 using the dsDNA HS Assay Kit (Life Technologies) according to the manufacturer’s protocol. The median yield for the plasma cfDNA at baseline was 15.68 ng/ml and 18.05ng/ml at disease progression.
Sequencing libraries were constructed using KAPA Hyper Prep kit (KAPA Biosystems) with an optimized manufacturer’s instructions. In brief, cfDNA or DNA was experienced with end-repairing, A-tailing, adapter ligation and size selection using Agencourt AMPure XP beads (Beckman Coulter). Libraries were then subjected to PCR amplification and purification before targeted enrichment. The size distribution of libraries was measured by Agilent Technologies 2100 Bioanalyzer (Agilent Technologies). The enriched libraries were sequenced on Illumina Hiseq 4000 NGS platforms to cover mean depths of at least 1,000×, 3,000×, and 100×, for FFPE, cfDNA, and blood, respectively. DNA extraction and NGS were performed in Geneseeq Technology Inc. A complete list of the genes included in the NGS panel was in Supplementary Table 1.
Data Processing
Trimmomatic was used for FASTQ file quality control (QC). Leading/trailing low quality (quality reading below 30) or N bases were removed. Remaining reads were mapped to the reference sequence data (Human Genome version 19) using Burrows-Wheeler Aligner (BWA-mem, v0.7.12). Indel realignment and base quality score recalibration were performed with Genome Analysis Toolkit (GATK 3.4.0). Somatic mutations were detected with VarScan2. Copy number variations (CNVs) were detected using ADTEx (http://adtex.sourceforge.net) with default parameters as reported by previous studies (14–16). To eliminate sequencing artifact, a local bioinformatics pipeline was performed. Firstly, we used a local white blood cell (WBCs) database, containing recurrent somatic alterations from WBCs of 400 patients, to eliminate the sequencing artifacts. Specifically, if a variant was detected (i.e. ≥2 mutant reads) in >10% of the samples, it was considered a likely artifact and was removed. Secondly, a background denoising strategy was performed. Briefly, we performed panel sequencing with similar sequencing depth used in this study on plasma samples of 50 healthy individuals to assemble a database of alterations at each site of the panel and build a background error model. A specific alteration at a specific site was considered sequencing noise if the allele frequency (AF) and distinct supporting reads were not significantly beyond the background error probability. Only alteration with an AF over three standard deviations from the mean AF of healthy plasma-cfDNAs pool remained and subjected for further analyze.
Bioinformatics Analyses
Trimmomatic48 was used for FASTQ file quality control (QC) (17). Sequencing data were aligned to the reference hg19 (Human Genome version 19) with the Burrows-Wheeler Aligner (BWA-mem, v0.7.12) (18). SNVs and Indels were detected using SCALPEL (http://scalpel.sourceforge.net) and Genome Analysis Toolkit (GATK). Tumor purity was estimated by ABSOLUTE (19). Purity-adjusted gene-level and segment-level copy numbers were calculated by CNVKit (20) ADTEx (http://adtex.sourceforge.net).
Statistical Analysis
Progression-free survival (PFS) was defined as the first date of third-generation EGFR TKIs until disease progression or death resulting from any cause. Overall survival (OS) was defined as the first date of third-generation EGFR TKIs until death from any cause. CfDNA level was defined as the sum of total cfDNA total mutation abundance detected by the 425-gene panel NGS. Similarly, T790M level was reflecting the mutation abundance detected by the 425-gene panel. Comparisons were made using an unpaired two-tailed t-test, and analysis of variance (one-way ANOVA) was performed using Graphpad Prism V8.0, and linear regression analysis and some survival analysis were also analyzed using Prism V8.0. Most of the statistical analyses were performed using R version 3.5.3. In particular, Survival, Survminer, and Cox analysis were used for the analysis of PFS and OS. All reported P values were two-tailed, and P values less than 0.05 were considered statistically significant.
Results
Patient Characteristics and Samples
From May 2016 to November 2019, 81 patients with a median age of 64 years old (range, 36–84) were enrolled. Twenty-six patients (32.1%) were male, 79 (97.5%) were adenocarcinoma, 16 (19.8%) were smokers, 77 (95.1%) were stage IV, 27 (33.3%) patients had central nervous system metastasis, and 57 (70.4%) were in second-line setting (Table 1). Above all, 64 patients (79.0%) were disease progression and 33 patients (40.7%) died at the last follow-up time on Nov. 30, 2019. Baseline samples were taken before the third-generation TKI treatment. Baseline tissue samples were obtained in 46 patients and identified with EGFR T790M. Baseline plasma samples were obtained in 77 patients. Sixty-six patients were cfDNA positive at baseline including 56 T790-positive cases. Fifty-nine patients had serial plasma samples available (Figure 1). Forty-one patients received osimertinib (80 mg, qd) and 40 patients received alflutinib (80 mg, qd). Clearing rates of cfDNA and EGFR T790M over the third-generation TKI treatment course were shown in Figure 2. The clearance of cfDNA as well as EGFR-T790M increased from the treatment onset and reached the maximum at three months, suggesting these patients responded to the treatment.
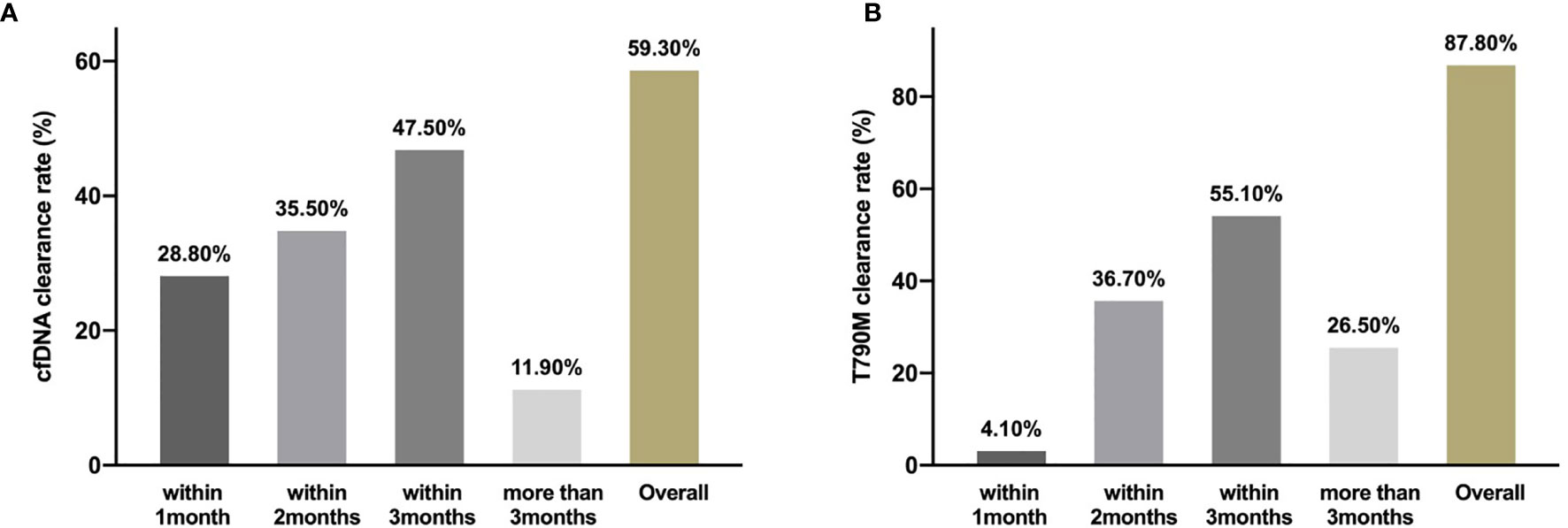
Figure 2 Clearing rate of cfDNA and EGFR T790M. The clearance rate of cfDNA (A) and EGFR T790M (B) within 1month, months and more than 3months during the third generation TKIs treatment.
Predictors of Progression-Free Survival
The median PFS of 81 patients was 14.167 months (95%CI 11.704–16.630). There was no significant correlation between sex, age, smoking history, clinical staging, CNS metastasis, or treatment-line with the PFS of the third-generation TKIs, respectively (Supplementary Table 2). Histology type was not analyzed because 79 of 81 patients were adenocarcinoma.
Interestingly, univariate analysis showed that the median PFS of the patients with pretreatment undetectable cfDNA was significantly longer than those with detectable cfDNA (18.0 vs. 12.47 months; HR 0.5140, 95%CI, 0.2749–0.9610; P = 0.0374; Figure 3A). The PFS of patients with pretreatment undetectable T790M was superior to those with detectable T790M patients (17.2 vs. 12 months; HR 0.6946, 95%CI 0.4055–1.190; P = 0.0430; Figure 3B). However, multivariate analysis showed that neither pretreatment cfDNA level (HR 0.7759, 95%CI 0.3275–1.828; P = 0.564) nor pretreatment T790M level (HR 1.0535, 95%CI 0.4927–2.253; P = 0.893) was associated with the PFS for patients who received the third-generation EGFR TKIs treatment.
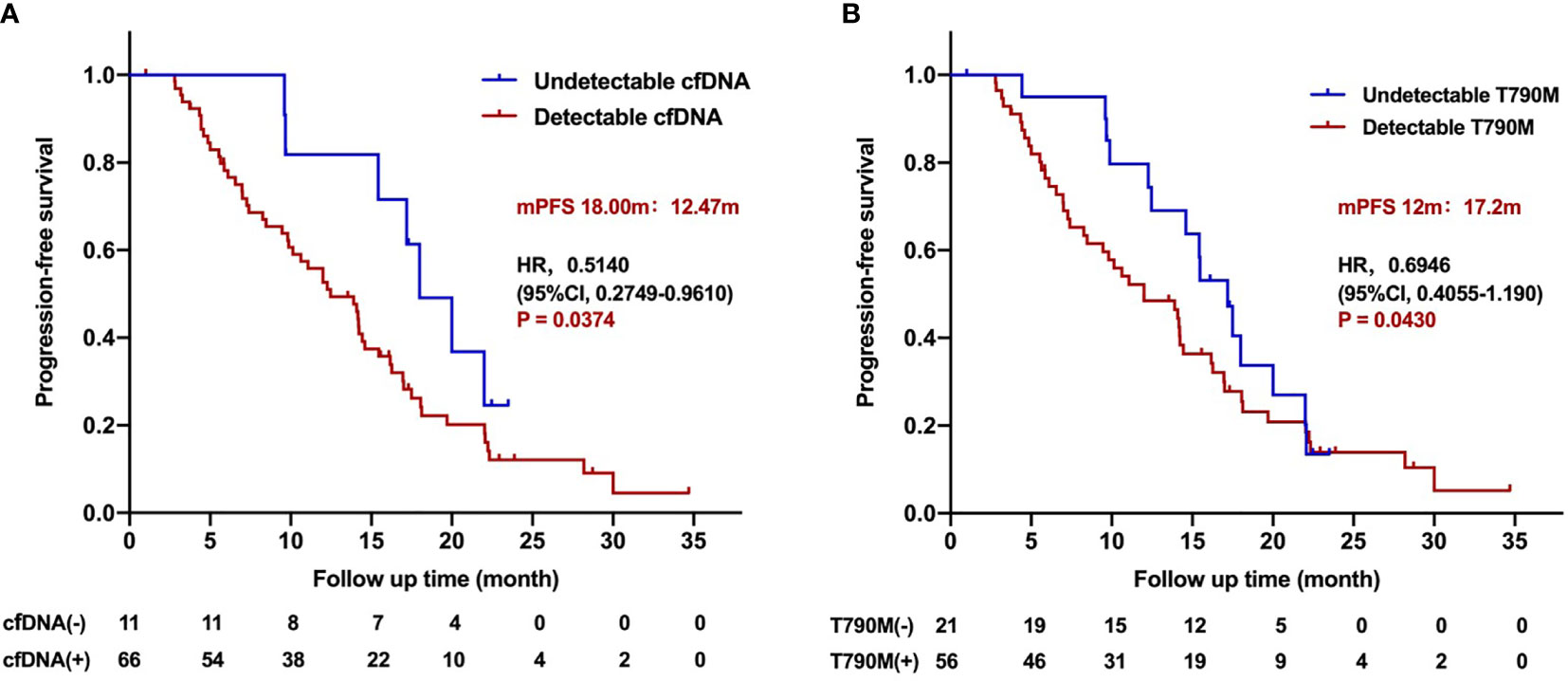
Figure 3 Progression-free survival for patients treated with the third-generation EGFR TKIs according to pretreatment cfDNA and T790M level. (A) Kaplan–Meier analysis stratified by cfDNA level. (B) Kaplan–Meier analysis stratified by T790M level.
Further analysis was carried out to study the association of dynamic changing of cfDNA with PFS. In 59 cfDNA-positive patients who had serial plasma samples, 35 patients presented with cfDNA clearance. The clearance rate of cfDNA level within 1, 2, 3, and beyond 3 months during the third-generation TKI treatment was 28.8% (17/59), 35.5% (21/59), 47.5% (28/59), and 11.9% (7/59), respectively. The PFS of patients with undetectable fDNA during treatment was significantly longer than that of patients with detectable cfDNA (16.97 vs. 6.10 months; HR 0.2109, 95%CI 0.09580–0.4643; P < 0.0001; Figure 4A). The PFS of patients with undetectable cfDNA during the entire time of treatment was significantly longer than that with detectable cfDNA within post-treatment of 1, 2, 3, or more than 3 months, respectively (Supplementary Figure 1). Nevertheless, there were no significant differences on PFS among patients with undetectable cfDNA in 1, 2, 3, or more than 3 months after the third-generation TKI treatment. The duration of undetectable cfDNA was also positively correlated with the longer PFS during the third-generation TKI treatment (R2 = 0.4428; P < 0.0001; Supplementary Figure 2).
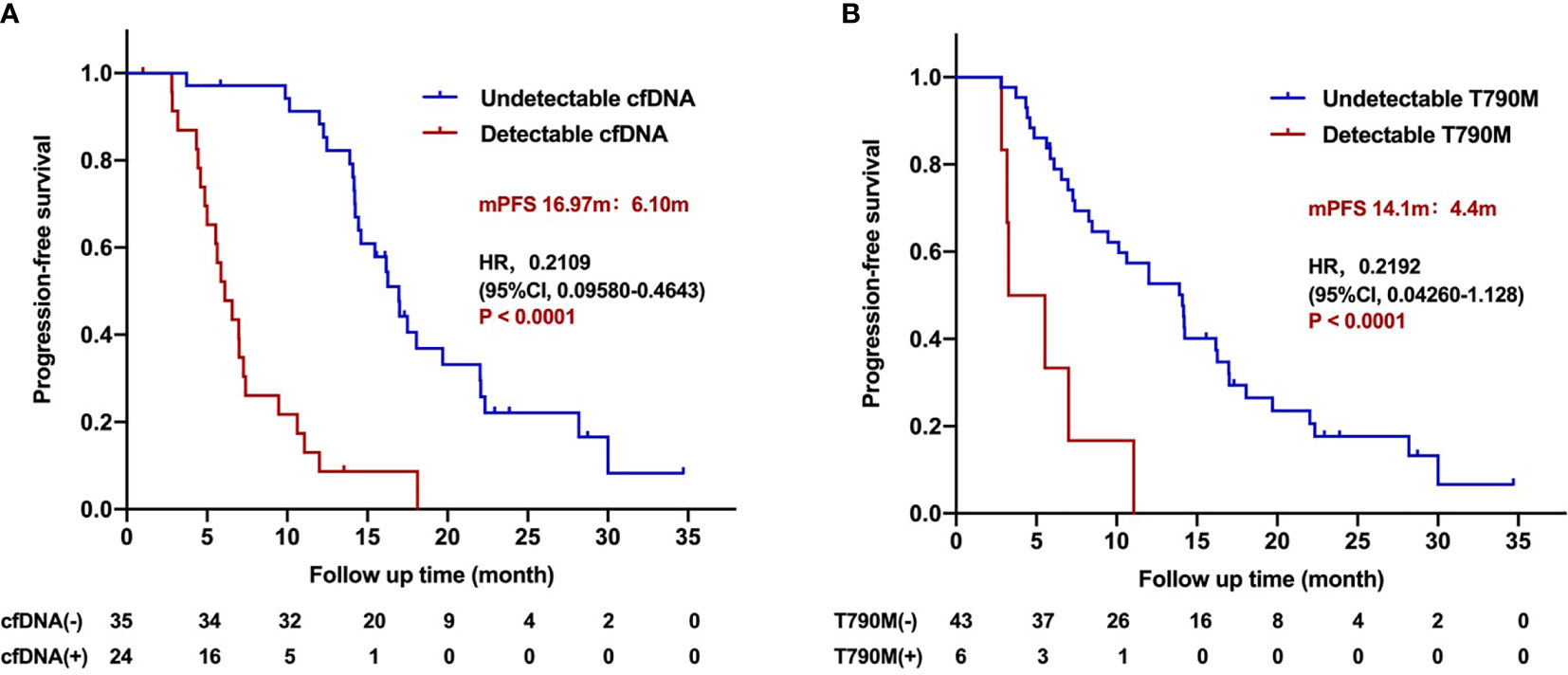
Figure 4 Progression-free survival for patients treated with the third-generation EGFR TKIs according to dynamic cfDNA and T790M level during treatment. (A) Kaplan–Meier analysis stratified by cfDNA level. (B) Kaplan–Meier analysis stratified by T790M level.
In 49 T790M-positive patients who had serial plasma samples, 43 patients developed T790M clearance. Patients with undetectable T790M during treatment benefited from the third-generation TKI treatment compared with those with detectable T790M patients (14.1 vs. 4.4 months; HR 0.2192, 95%CI 0.04260–1.128; P < 0.001; Figure 4B). The relationship between T790M clearance speed and PFS is classified within 1, 2, 3 months, and more than 3 months after the third-generation TKI treatment (Supplementary Figure 3). The clearance rate of T790M level within 1, 2, 3 and beyond 3 months during the third-generation TKI treatment was 4.1% (2/49), 36.7% (18/49), 55.1% (27/49), and 26.5% (13/49). Among them, one patient did not receive continuous dynamic monitoring, and clearance was almost 1 year after treatment, which was included in the total number of clearance, but not in the calculation of different time points. The PFS stratified by T790M level at different times of treatment was shown in (Supplementary Figure 4). The duration of undetectable T790M was also positively correlated with longer PFS of the third-generation TKIs (R2 = 0.7235; P < 0.0001; Supplementary Figure 4).
In multivariate analysis, undetectable cfDNA level or T790M level during treatment was performed by Cox analysis. The results showed that undetectable cfDNA level during treatment was an independent predictor for longer PFS of the third-generation TKIs (HR 0.3085, 95%CI 0.1617–0.5885; P = 0.000359), while undetectable T790M was not (HR 0.57, 95%CI 0.2874–1.1303; P = 0.1075). These results suggest that dynamic cfDNA level can predict the efficacy of the third-generation TKIs, and negative cfDNA during treatment is associated with better outcome.
Predictors of Overall Survival
The median overall survival was 25.533 months (95%CI 22.615–28.452). Unfortunately, there were no significant correlations between clinical characteristics and over survival time (Supplementary Table 2), and no association was observed between pretreatment cfDNA level (HR 0.3525, 95%CI 0.1361–0.9129; P = 0.1336) or T790M level (HR 0.3680, 95%CI 0.1690–0.8012; P = 0.0498) and overall survival time. The cfDNA clearance was associated with the OS (34.9 vs. 16.3 months; HR 0.3105, 95%CI 0.1301–0.7412; P = 0.0014; Figure 5). Dynamic T790M level changes were not correlated with the OS (HR 0.6472, 95%CI 0.1127–3.716; P = 0.5509). These results demonstrated that detectable cfDNA during treatment is a poor factor of overall survival.
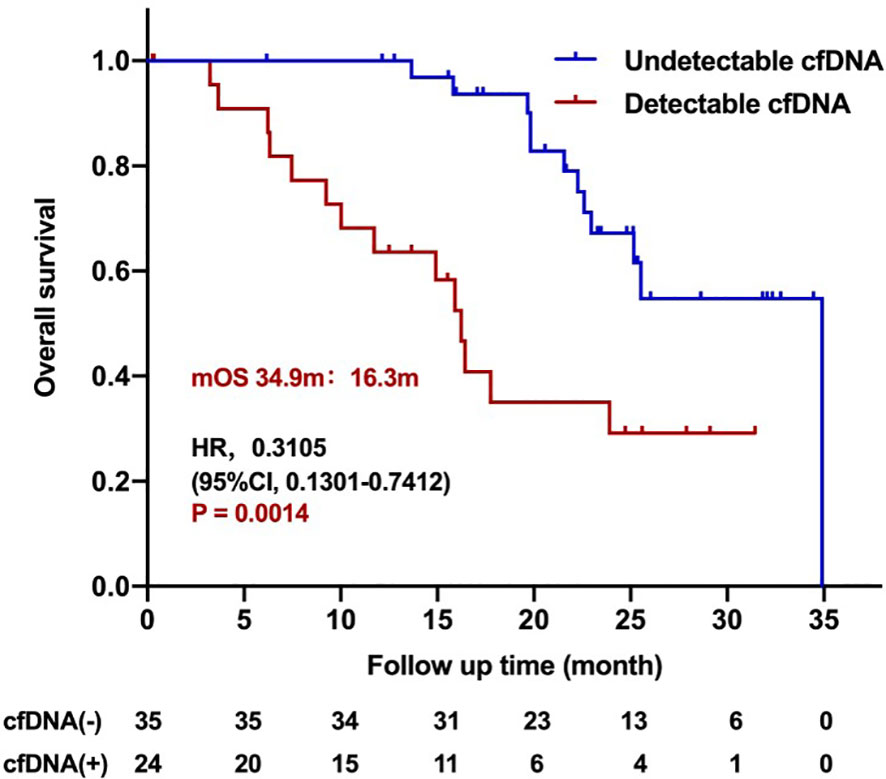
Figure 5 Kaplan–Meier analysis of overall survival for patients treated with the third-generation EGFR TKIs according to dynamic cfDNA level during treatment.
Relationship of Cell-Free DNA Changing With Imaging (Chest CT)
The association of dynamic cfDNA changes with medical imaging presentation of patients who developed resistance to the third-generation TKIs was analyzed. The result showed that re-detected or re-elevated cfDNA level occurred earlier than progression on clinical imaging in 33 patients with 4.8 months in advance (P < 0.0001; Supplementary Figure 5), which may predict disease progression and recurrence risk.
Resistance Mechanisms Detected by Next-Generation Sequencing
In this cohort, thirty-nine patients had matched plasma and tumor tissue at baseline. Overall concordance, defined as the proportion of patients for whom at least one identical genomic alteration was identified in both tissue and plasma (21), was 69.2%(27/39). For EGFR T790M, 53.8% (21/39) of patients with matched plasma and tissue had T790M identified in both samples. The gene mutation profiles at baseline and PD point during the third-generation TKI treatment were further explored. The top 30 somatic mutational genes at pretreatment were showed in Figure 6A. EGFR gene mutation (19del and L858R), TP53 gene mutation, and EGFR amplification were the most common gene variations. Other concurrent gene mutations were also detected, including BIM, CTNNB1, and PIK3CA gene mutations. Six gene mutations in pretreatment samples, including CDKN1B amplification, ERBB2 amplification, ERBB2 mutation, KMT2D mutation, PTEN mutation, and SETD2 mutation were significantly associated with PFS (Supplementary Table 3). The top 30 somatic mutations profile at PD point were listed in Figure 6B. The resistant mutation profile of the third-generation TKIs was shown in Figure 6C, in which EGFR C797S mutation was the most common resistant mutation (24%). MET CNV and ERBB2 p.S22I and p.E992K mutations were also found in 3 and 5% patients, respectively. Moreover, 18 genes detected at PD were statistically significantly correlated with PFS (Supplementary Table 4), showing the complex mechanisms of drug resistance. These results suggest that dynamic cfDNA analysis is helpful in monitoring resistance to targeted therapy.
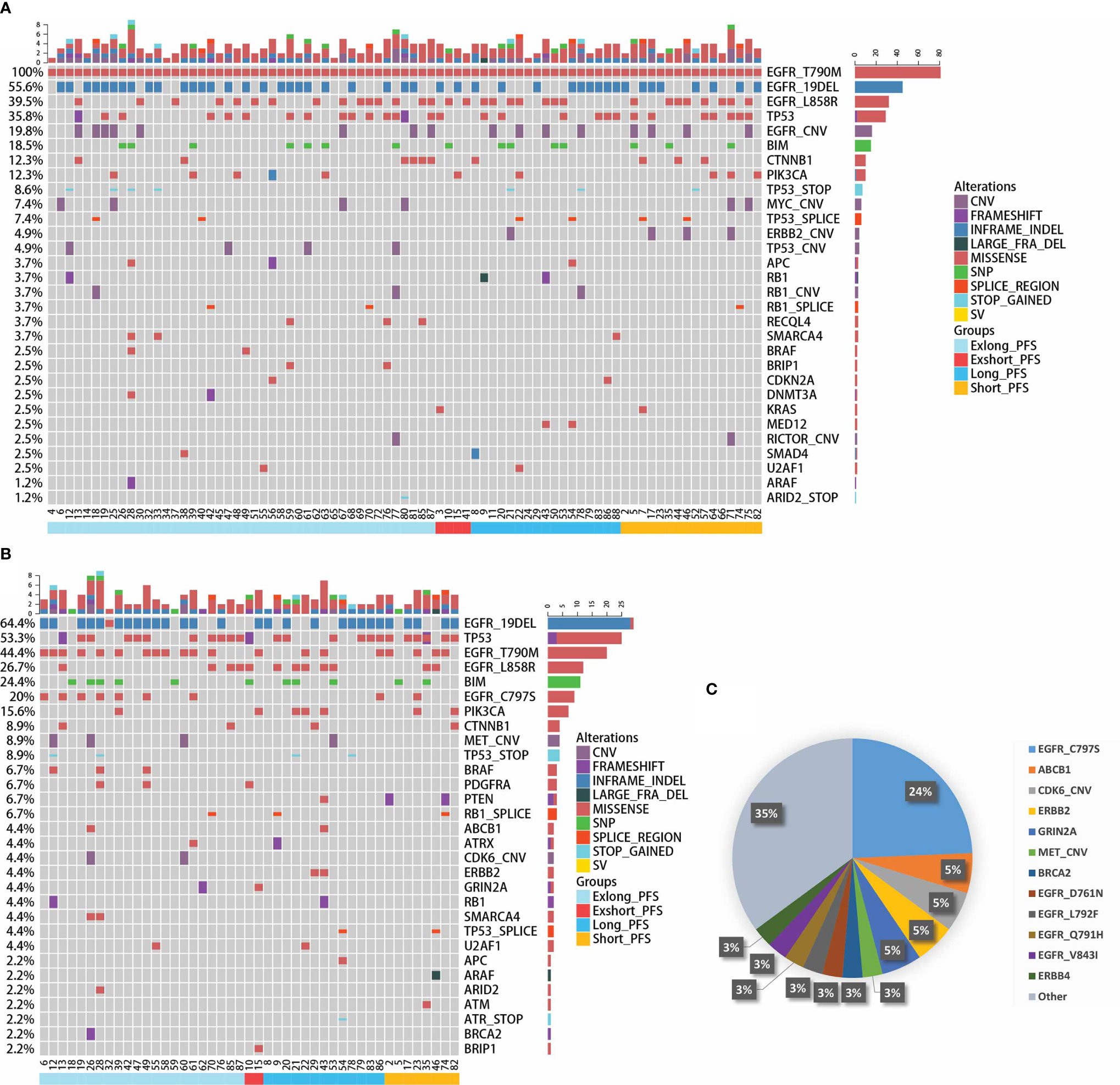
Figure 6 Gene mutation profile based on next-generation sequencing. (A) Somatic gene mutations profile containing the top 30 genes at pretreatment of the third-generation EGFR TKIs. (B) Somatic gene mutations profile containing the top 30 genes at disease progression point for patients with third-generation EGFR TKIs treatment. (C) Resistant gene mutation profile of third-generation TKIs.
Discussion
This study evaluated the role of dynamic cfDNA level based on a large-panel NGS with 425 genes in predicting outcomes and identifying possible resistant mutations in EGFR T790M-positive NSCLC patients who received the third-generation EGFR TKIs. Our results demonstrated that cfDNA clearance significantly correlated the longer PFS and OS of the third-generation EGFR TKIs. Moreover, cfDNA analysis depicted the resistant landscape during TKI treatment, providing guidance for successive treatment options.
Several studies have shown that cfDNA clearance during first-line EGFR TKI treatment predicts outcomes for first- and second-generation TKI therapy (22, 23). One of the studies showed that depletion of fDNA predicted outcome of patients with EGFR mutant NSCLC who received first-generation EGFR TKI using Cobas® EGFR mutation test v2 (24). Song et al. reported that cfDNA clearance was associated with longer PFS in a real-world NSCLC cohort involving different kinds of treatment strategies using a 168-gene panel (25). But there was a lack of studies concerning the role of dynamic cfDNA level during the third-generation TKI treatment. A case report showed that clearance of fDNA-based ddPCR can predict the response to osimertinib (26). Ebert et al. also reported that clearing of all EGFR cfDNA after osimertinib treatment predicts the efficacy of Osimertinib with significant improvement in PFS, ORR, and DCR in 82 patients with NSCLC using Cobas® EGFR mutation test v2 (27). Another study showed that cfDNA presence at 6 weeks correlated with short PFS and OS in a phase 1/2 study of osimertinib plus bevacizumab as first-line therapy for advanced EGFR-mutant NSCLC using the QX200 BioRad PCR system (28). Buder A et al. reported that cfDNA at initial and 8 weeks after osimertinib treatment in advanced EGFR-positive NSCLC was associated with shorter PFS and OS using droplet digital PCR (29). However, the limitations of the above studies were more likely due to the method of cfDNA testing, such as PCR, a non-quantitative analysis of cfDNA focusing on the limited specific mutation sites, a small sample size, or a smaller gene panel involving over 100 genes. Another important difference was that previous studies focus on cfDNA of EGFR genes, while our study analyzed the total cfDNA level detected by NGS. Our study demonstrated that clearing of cfDNA predicts the longer PFS and OS of the third-generation EGFR TKIs using a large gene NGS panel including 425 genes, and T790M level was not associated with outcomes. These results indicated that cfDNA was a positive predictor of prognosis of patients who received the third-generation TKIs.
Early clearance of plasma EGFR mutations (Ex19del, L858R and T790M) has been reported as a predictor of response to osimeritinb in both pretreated and treatment-naïve patients in different clinical trials (30–32). In this study, the correlation between EGFR T790M mutation and prognosis has been further discussed. In AURA3 trial, T790M-positive patients verified by tissue samples had a longer PFS than those verified in plasma (33), which indicated detectable plasma T790M status could be a reflection of tumor burden. The relative mutation purity of T790M (defined as the ratio of allelic frequency to maximum somatic allele frequency) was associated with significantly longer PFS of osimertinib treatment using small panel NGS (34). Bordi P et al. reported that patients with lower EGFR sensitive mutation ctDNA level (<2,200 copies/ml or allele frequency (AF) <6.1%) had a better PFS of osimertinib in plasma by ddPCR and Therascreen® (10). In our study, in univariate analysis, pretreatment T790M level and level change during treatment course were associated with the PFS of the third-generation TKIs, but both were not confirmed in multivariate analysis which might be due to the confounding factors.
In clinical practices, the diagnosis of disease progression largely relied on radiological imaging. However, whether plasma cfDNA T790M can be employed as a valuable marker for changing the treatment strategy remains unknown. Prospective clinical trials on treatment strategy based on cfDNA level are highly expected. We observed that the re-detectable or re-elevated cfDNA level occurred 4.8 months earlier than progression on medical imaging, indicating molecular events develop earlier than clinical changing, which is accord with the previous studies focusing on cfDNA detection in early cancer relapse (35–37).
The cfDNA analysis based on NGS testing was also valuable in monitoring targeted drug resistance during rebiopsy. The resistant mechanism of osimertinib in pretreated and treatment-naïve patients has been shown to be different (38–40). In pretreated patients, MET amplification was observed in 19% of patients at disease progression and/or discontinuation with concurrent EGFR C797S (7%) and EGFR C797S+HER2 amplification (1%). In those samples with MET amplification, there was an almost equal split between samples that lost T790M (43%) versus those that retained T790M (57%) at disease progression and/or discontinuation. In treatment-naïve patients, the most frequently observed Osimertinib-resistant alterations were MET amplification (15%) and EGFR C797S (7%). Here in this study, the frequency of MET amplification was relatively low (3%), whereas the incidence of EGFR C797S mutation was higher than previously reported (24%). Meanwhile, cell cycle gene alterations such as CDK6 CNV were presented at higher ratio compared to previous studies. Here ABCB1 gene mutation which has been demonstrated as one of the resistant mechanisms of Osimertinib was also identified at a ratio of 5% (41, 42). These discrepancies in the detection of resistant mechanism could be due to the different panel using in each study as well as difference in the ethnic background of patients enrolled.
It is worth mentioning that several novel resistant gene alterations are firstly reported in our study, including CDK6 CNV, GRIN2A, BRCA2, EGFR D761N, EGFR Q791H, EGFR V843I, and ERBB4 gene alteration. CDK6 CNV has been reported to correlate with first-generation EGFR TKI resistance and shorten PFS to osimeritinib (43). The clinical impact of rare and compound mutations of EGFR including EGFR D761N, EGFR Q791H, EGFR V843I has been mentioned in EGFR TKI treatment, however, without conclusions thus far (44). ERBB4 alterations have also been reported in NSCLC and found to be activated (45). Further studies are warranted to validate these potential resistant gene alterations. Besides, six gene alterations have been found at pretreatment and 18 gene alterations at PD point. The PFS of patients with these novel resistance gene alterations was shorter than that of patients without the gene alterations based on the small sample sizes, which needs validation in larger cohort and further studies.
Although plasma cfDNA-based re-biospy showed advantage in identifying off-target genetic resistance, histologic transformation which is a frequent early resistance mechanism to first-line osimertinib still might require tissue re-biopsy for further evaluation, especially for patients with co-mutation of EGFR, TP53 and RB1 (46). To overcome these emerging resistance mechanisms during osimeritinib treatment, combinational approaches have been proposed including CDK4/6 dual inhibitor plus osimertinib (47), MET-TKI plus osimertinib (48), checkpoint inhibitor plus EGFR TKI (49) in preclinical and clinical settings.
There are some limitations in our study. Firstly, not all of the patients had matched tissue and dynamic plasma samples resulting in partial data missing. The lack of matched tissue and dynamic plasma to compare the genetic background of multiple samples in the same patients partially invalidated the result obtained. Secondly, not all of the patients had the re-biopsy tumor tissue at resistant point of the third-generation EGFR TKIs. But censored data was allowed in terms of statistics. The over survival follow-up is on the way. Thirdly, the detailed function of these newly identified resistant gene alterations is unclear, which requires more functional studies.
Taken together, we observed the association of cfDNA clearance with the better PFS and OS in T790M-positive advanced NSCLC patients who received the third-generation EGFR TKIs through serial NGS testing. The dynamic plasma cfDNA level may be helpful for monitoring tumor response, predicting patient outcomes, and identifying resistance mechanisms during the third-generation EGFR TKI treatment in clinical practice. Prospective clinical trials of guiding the treatment strategy based on cfDNA level during targeted therapy are expected.
Data Availability Statement
The data presented in the study are deposited in the Genome Sequence Archive for Human (GSA) repository, accession number HRA000725. The link is https://bigd.big.ac.cn/gsa-human/.
Ethics Statement
The studies involving human participants were reviewed and approved by the ethics committee of Beijing Chest Hospital. The patients/participants provided their written informed consent to participate in this study.
Author Contributions
JW, SZ, and LM designed the study and interpreted the results. LM, HL, DW, YH, MY, QZ, NQ, XZ, XL, HZ, YW, JL, and XY enrolled the patients and collected the clinical data. JW, LM, and HL wrote the manuscript. RY contributed to the manuscript revision–manuscript editing as well as data analysis during revision. All authors contributed to the article and approved the submitted version.
Funding
This study was supported by the Capital Health Development Scientific Research Fund-the young talents program (2018-4-1043), Beijing Municipal Administration of Hospitals’ Youth program (QML20181602).
Conflict of Interest
RY is an employee of Nanjing Geneseeq Technology Inc.
The remaining authors declare that the research was conducted in the absence of any commercial or financial relationships that could be construed as a potential conflict of interest.
Acknowledgments
We thank all patients and families who participated in this study. We thank Yang Shao in Geneseeq Technology Inc. for providing the experimental process of data sequencing.
Supplementary Material
The Supplementary Material for this article can be found online at: https://www.frontiersin.org/articles/10.3389/fonc.2021.643199/full#supplementary-material
Supplementary Figure 1 | Analysis of progression-free survival of the third-generation EGFR TKIs treatment among those with undetectable cfDNA level and those with detectable cfDNA within post-treatment of 1, 2, 3, or more than 3 months. (A) PFS stratified by different cfDNA level within 1 month after treatment. (B) PFS stratified by different cfDNA level within 2 months after treatment. (C) PFS stratified by different cfDNA level within 3 months after treatment. (D) PFS stratified by different cfDNA level more than 3 months after treatment.
Supplementary Figure 2 | Correlation of cfDNA clearance duration with progression-free survival in patients treated with the third-generation EGFR TKIs.
Supplementary Figure 3 | Analysis of progression-free survival of the third-generation EGFR TKIs treatment among those with undetectable T790M level and those with detectable T790M within post-treatment of 1, 2, 3, or more than 3 months. (A) PFS stratified by different T790M level within 1 month after treatment. (B) PFS stratified by different T790M level within 2 months after treatment. (C) PFS stratified by different T790M level within 3 months after treatment. (D) PFS stratified by different T790M level more than 3 months after treatment.
Supplementary Figure 4 | Correlation of EGFR T790M clearance duration with progression-free survival in patients treated with the third-generation EGFR TKIs.
Supplementary Figure 5 | Association of dynamic cfDNA level with imaging presentation of patients who developed resistance to the third-generation EGFR TKIs.
References
1. Rajappa S, Krishna MV, Narayanan P. Integrating Osimertinib in Clinical Practice for Non-Small Cell Lung Cancer Treatment. Adv Ther (2019) 36(6):1279–90. doi: 10.1007/s12325-019-00917-6
2. Shi Y, Zhang S, Hu X, Feng J, Ma Z, Zhou J, et al. Safety, Clinical Activity, and Pharmacokinetics of Alflutinib (AST2818) in Patients With Advanced NSCLC With EGFR T790M Mutation. J Thorac Oncol (2020) 15(6):1015–26. doi: 10.1016/j.jtho.2020.01.010
3. Siravegna G, Mussolin B, Venesio T, Marsoni S, Seoane J, Dive C, et al. How liquid biopsies can change clinical practice in oncology. Ann Oncol (2019) 30(10):1580–90. doi: 10.1093/annonc/mdz227
4. Oxnard GR, Arcila ME, Sima CS, Riely GJ, Chmielecki J, Kris MG, et al. Acquired resistance to EGFR tyrosine kinase inhibitors in EGFR-mutant lung cancer: distinct natural history of patients with tumors harboring the T790M mutation. Clin Cancer Res (2011) 17(6):1616–22. doi: 10.1158/1078-0432.CCR-10-2692
5. Iwama E, Sakai K, Hidaka N, Inoue K, Fujii A, Nakagaki N, et al. Longitudinal monitoring of somatic genetic alterations in circulating cell-free DNA during treatment with epidermal growth factor receptor-tyrosine kinase inhibitors. Cancer (2020) 126(1):219–27. doi: 10.1002/cncr.32481
6. Lee JY, Qing X, Xiumin W, Yali B, Chi S, Bak SH, et al. Longitudinal monitoring of EGFR mutations in plasma predicts outcomes of NSCLC patients treated with EGFR TKIs: Korean Lung Cancer Consortium (KLCC-12-02). Oncotarget (2016) 7(6):6984–93. doi: 10.18632/oncotarget.6874
7. Tseng JS, Yang TY, Tsai CR, Chen KC, Hsu KH, Tsai MH, et al. Dynamic plasma EGFR mutation status as a predictor of EGFR-TKI efficacy in patients with EGFR-mutant lung adenocarcinoma. J Thorac Oncol (2015) 10(4):603–10. doi: 10.1097/JTO.0000000000000443
8. Malapelle U, Rolfo C. International Society of Liquid B. Liquid biopsy as a follow-up tool: Comment on longitudinal monitoring of somatic genetic alterations in circulating cell-free DNA during treatment with epidermal growth factor receptor-tyrosine kinase inhibitors. Cancer (2020) 126(1):22–5. doi: 10.1002/cncr.32482
9. Rossi G, Russo A, Tagliamento M, Tuzi A, Nigro O, Vallome G, et al. Precision Medicine for NSCLC in the Era of Immunotherapy: New Biomarkers to Select the Most Suitable Treatment or the Most Suitable Patient. Cancers (2020) 12(5):1125. doi: 10.3390/cancers12051125
10. Bordi P, Del Re M, Minari R, Rofi E, Buti S, Restante G, et al. From the beginning to resistance: Study of plasma monitoring and resistance mechanisms in a cohort of patients treated with osimertinib for advanced T790M-positive NSCLC. Lung Cancer (2019) 131:78–85. doi: 10.1016/j.lungcan.2019.03.017
11. Spence T, Perera S, Weiss J, Grenier S, Ranich L, Shepherd F, et al. Clinical implementation of circulating tumour DNA testing for EGFR T790M for detection of treatment resistance in non-small cell lung cancer. J Clin Pathol (2020) 74(2):91–7. doi: 10.1136/jclinpath-2020-206668
12. Usui K, Yokoyama T, Naka G, Ishida H, Kishi K, Uemura K, et al. Plasma ctDNA monitoring during epidermal growth factor receptor (EGFR)-tyrosine kinase inhibitor treatment in patients with EGFR-mutant non-small cell lung cancer (JP-CLEAR trial). Jpn J Clin Oncol (2019) 49(6):554–8. doi: 10.1093/jjco/hyz023
13. Piotrowska Z, Isozaki H, Lennerz JK, Gainor JF, Lennes IT, Zhu VW, et al. Landscape of Acquired Resistance to Osimertinib in EGFR-Mutant NSCLC and Clinical Validation of Combined EGFR and RET Inhibition with Osimertinib and BLU-667 for Acquired RET Fusion. Cancer Discov (2018) 8(12):1529–39. doi: 10.1158/2159-8290.CD-18-1022
14. Wang DS, Liu ZX, Lu YX, Bao H, Wu X, Zeng ZL, et al. Liquid biopsies to track trastuzumab resistance in metastatic HER2-positive gastric cancer. Gut (2019) 68(7):1152–61. doi: 10.1136/gutjnl-2018-316522
15. Yang W, You N, Jia M, Yeung SJ, Ou W, Yu M, et al. Undetectable circulating tumor DNA levels correlate with low risk of recurrence/metastasis in postoperative pathologic stage I lung adenocarcinoma patients. Lung Cancer (2020) 146:327–34. doi: 10.1016/j.lungcan.2020.06.009
16. Tong L, Ding N, Tong X, Li J, Zhang Y, Wang X, et al. Tumor-derived DNA from pleural effusion supernatant as a promising alternative to tumor tissue in genomic profiling of advanced lung cancer. Theranostics (2019) 9(19):5532–41. doi: 10.7150/thno.34070
17. Bolger AM, Lohse M, Usadel B. Trimmomatic: a flexible trimmer for Illumina sequence data. Bioinformatics (2014) 30(15):2114–20. doi: 10.1093/bioinformatics/btu170
18. Li H, Durbin R. Fast and accurate short read alignment with Burrows-Wheeler transform. Bioinformatics (2009) 25(14):1754–60. doi: 10.1093/bioinformatics/btp324
19. Carter SL, Cibulskis K, Helman E, McKenna A, Shen H, Zack T, et al. Absolute quantification of somatic DNA alterations in human cancer. Nat Biotechnol (2012) 30(5):413–21. doi: 10.1038/nbt.2203
20. Talevich E, Shain AH, Botton T, Bastian BC. CNVkit: Genome-Wide Copy Number Detection and Visualization from Targeted DNA Sequencing. PLoS Comput Biol (2016) 12(4):e1004873. doi: 10.1371/journal.pcbi.1004873
21. Sabari JK, Offin M, Stephens D, Ni A, Lee A, Pavlakis N, et al. A Prospective Study of Circulating Tumor DNA to Guide Matched Targeted Therapy in Lung Cancers. J Natl Cancer Inst (2019) 111(6):575–83. doi: 10.1093/jnci/djy156
22. Iwama E, Sakai K, Azuma K, Harada T, Okamoto I. Monitoring of somatic mutations in circulating cell-free DNA by digital PCR and next-generation sequencing during afatinib treatment in patients with lung adenocarcinoma positive for EGFR activating mutations. Ann Oncol (2017) 28(1):136–41. doi: 10.1093/annonc/mdw531
23. Mok T, Wu YL, Lee JS, Yu CJ, Sriuranpong V, Sandoval-Tan J, et al. Detection and Dynamic Changes of EGFR Mutations from Circulating Tumor DNA as a Predictor of Survival Outcomes in NSCLC Patients Treated with First-line Intercalated Erlotinib and Chemotherapy. Clin Cancer Res (2015) 21(14):3196–203. doi: 10.1158/1078-0432.CCR-14-2594
24. Ebert EBF, McCulloch T, Hansen KH, Linnet H, Sorensen B, Meldgaard P. Clearing of circulating tumour DNA predicts clinical response to first line tyrosine kinase inhibitors in advanced epidermal growth factor receptor mutated non-small cell lung cancer. Lung Cancer (2020) 141:37–43. doi: 10.1016/j.lungcan.2019.12.016
25. Song Y, Hu C, Xie Z, Wu L, Zhu Z, Rao C, et al. Circulating tumor DNA clearance predicts prognosis across treatment regimen in a large real-world longitudinally monitored advanced non-small cell lung cancer cohort. Transl Lung Cancer Res (2020) 9(2):269–79. doi: 10.21037/tlcr.2020.03.17
26. Vallee A, Audigier-Valette C, Herbreteau G, Merrien J, Tessonnier L, Theoleyre S, et al. Rapid clearance of circulating tumor DNA during treatment with AZD9291 of a lung cancer patient presenting the resistance EGFR T790M mutation. Lung Cancer (2016) 91:73–4. doi: 10.1016/j.lungcan.2015.11.008
27. Boysen Fynboe Ebert E, McCulloch T, Holmskov Hansen K, Linnet H, Sorensen B, Meldgaard P. Clearing of circulating tumour DNA predicts clinical response to osimertinib in EGFR mutated lung cancer patients. Lung Cancer (2020) 143:67–72. doi: 10.1016/j.lungcan.2020.03.020
28. Yu HA, Schoenfeld AJ, Makhnin A, Kim R, Rizvi H, Tsui D, et al. Effect of Osimertinib and Bevacizumab on Progression-Free Survival for Patients With Metastatic EGFR-Mutant Lung Cancers: A Phase 1/2 Single-Group Open-Label Trial. JAMA Oncol (2020) 6(7):1048–54. doi: 10.1001/jamaoncol.2020.1260
29. Buder A, Hochmair MJ, Setinek U, Pirker R, Filipits M. EGFR mutation tracking predicts survival in advanced EGFR-mutated non-small cell lung cancer patients treated with osimertinib. Transl Lung Cancer Res (2020) 9(2):239–45. doi: 10.21037/tlcr.2020.03.02
30. Shepherd FA, Papadimitrakopoulou V, Mok T, Wu Y-L, Han J-Y, Ahn M-J, et al. Early clearance of plasma EGFR mutations as a predictor of response to osimertinib in the AURA3 trial. J Clin Oncol (2018) 36(15_suppl):9027. doi: 10.1200/JCO.2018.36.15_suppl.9027
31. Thress KS, Markovets A, Barrett JC, Chmielecki J, Goldberg SB, Shepherd FA, et al. Complete clearance of plasma EGFR mutations as a predictor of outcome on osimertinib in the AURA trial. J Clin Oncol (2017) 35(15_suppl):9018. doi: 10.1200/JCO.2017.35.15_suppl.9018
32. Zhou C, Imamura F, Cheng Y, Okamoto I, Cho BC, Lin MC, et al. Early clearance of plasma EGFR mutations as a predictor of response to osimertinib and comparator EGFR-TKIs in the FLAURA trial. J Clin Oncol (2019) 37(15_suppl):9020. doi: 10.1200/JCO.2019.37.15_suppl.9020
33. Papadimitrakopoulou VA, Han JY, Ahn MJ, Ramalingam SS, Delmonte A, Hsia TC, et al. Epidermal growth factor receptor mutation analysis in tissue and plasma from the AURA3 trial: Osimertinib versus platinum-pemetrexed for T790M mutation-positive advanced non-small cell lung cancer. Cancer (2020) 126(2):373–80. doi: 10.1002/cncr.32503
34. Zheng Q, Hong S, Huang Y, Zhao H, Yang Y, Hou X, et al. EGFR T790M relative mutation purity predicts osimertinib treatment efficacy in non-small cell lung cancer patients. Clin Transl Med (2020) 9(1):17. doi: 10.1186/s40169-020-0269-y
35. Abbosh C, Birkbak NJ, Wilson GA, Jamalhanjani M, Constantin T, Salari R, et al. Phylogenetic ctDNA analysis depicts early-stage lung cancer evolution. Nature (2017) 545(7655):446–51. doi: 10.1038/nature22364
36. Dudley JC, Schroersmartin JG, Lazzareschi D, Shi W, Chen SB, Esfahani MS, et al. Detection and Surveillance of Bladder Cancer Using Urine Tumor DNA. Cancer Discov (2019) 9(4):500–9. doi: 10.1158/2159-8290.CD-18-0825
37. Tie J, Wang Y, Tomasetti C, Li L, Springer S, Kinde I, et al. Circulating tumor DNA analysis detects minimal residual disease and predicts recurrence in patients with stage II colon cancer. Sci Transl Med (2016) 8(346):346ra92. doi: 10.1126/scitranslmed.aaf6219
38. Leonetti A, Sharma S, Minari R, Perego P, Giovannetti E, Tiseo M. Resistance mechanisms to osimertinib in EGFR-mutated non-small cell lung cancer. Br J Cancer (2019) 121(9):725–37. doi: 10.1038/s41416-019-0573-8
39. Ramalingam SS, Cheng Y, Zhou C, Ohe Y, Imamura F, Cho BC, et al. Mechanisms of acquired resistance to first-line osimertinib: Preliminary data from the phase III FLAURA study. Ann Oncol (2018) 29:viii740. doi: 10.1093/annonc/mdy424.063
40. Papadimitrakopoulou VA, Wu YL, Han JY, Ahn MJ, Ramalingam SS, John T, et al. Analysis of resistance mechanisms to osimertinib in patients with EGFR T790M advanced NSCLC from the AURA3 study. Ann Oncol (2018) 29:viii741. doi: 10.1093/annonc/mdy424.064
41. Zhang XY, Zhang YK, Wang YJ, Gupta P, Zeng L, Xu M, et al. Osimertinib (AZD9291), a Mutant-Selective EGFR Inhibitor, Reverses ABCB1-Mediated Drug Resistance in Cancer Cells. Molecules (2016) 21(9):1236. doi: 10.3390/molecules21091236
42. Van Hoppe S, Jamalpoor A, Rood JJM, Wagenaar E, Sparidans RW, Beijnen JH, et al. Brain accumulation of osimertinib and its active metabolite AZ5104 is restricted by ABCB1 (P-glycoprotein) and ABCG2 (breast cancer resistance protein). Pharmacol Res (2019) 146:104297. doi: 10.1016/j.phrs.2019.104297
43. Blakely CM, Watkins TBK, Wu W, Gini B, Chabon JJ, McCoach CE, et al. Evolution and clinical impact of co-occurring genetic alterations in advanced-stage EGFR-mutant lung cancers. Nat Genet (2017) 49(12):1693–704. doi: 10.1038/ng.3990
44. Martin J, Lehmann A, Klauschen F, Hummel M, Lenze D, Grohe C, et al. Clinical Impact of Rare and Compound Mutations of Epidermal Growth Factor Receptor in Patients With Non-Small-Cell Lung Cancer. Clin Lung Cancer (2019) 20(5):350–62.e4. doi: 10.1016/j.cllc.2019.04.012
45. Kurppa KJ, Denessiouk K, Johnson MS, Elenius K. Activating ERBB4 mutations in non-small cell lung cancer. Oncogene (2016) 35(10):1283–91. doi: 10.1038/onc.2015.185
46. Schoenfeld AJ, Chan JM, Kubota D, Sato H, Rizvi H, Daneshbod Y, et al. Tumor Analyses Reveal Squamous Transformation and Off-Target Alterations As Early Resistance Mechanisms to First-line Osimertinib in EGFR-Mutant Lung Cancer. Clin Cancer Res (2020) 26(11):2654–63. doi: 10.1158/1078-0432.CCR-19-3563
47. La Monica S, Fumarola C, Cretella D, Bonelli M, Minari R, Cavazzoni A, et al. Efficacy of the CDK4/6 Dual Inhibitor Abemaciclib in EGFR-Mutated NSCLC Cell Lines with Different Resistance Mechanisms to Osimertinib. Cancers (2020) 13(1):6. doi: 10.3390/cancers13010006
48. Sequist LV, Han JY, Ahn MJ, Cho BC, Yu H, Kim SW, et al. Osimertinib plus savolitinib in patients with EGFR mutation-positive, MET-amplified, non-small-cell lung cancer after progression on EGFR tyrosine kinase inhibitors: interim results from a multicentre, open-label, phase 1b study. Lancet Oncol (2020) 21(3):373–86. doi: 10.1016/S1470-2045(19)30785-5
Keywords: circulating cell-free DNA (cfDNA), EGFR, T790M, third-generation EGFR TKI declarations, lung cancer
Citation: Ma L, Li H, Wang D, Hu Y, Yu M, Zhang Q, Qin N, Zhang X, Li X, Zhang H, Wu Y, Lv J, Yang X, Yu R, Zhang S and Wang J (2021) Dynamic cfDNA Analysis by NGS in EGFR T790M-Positive Advanced NSCLC Patients Failed to the First-Generation EGFR-TKIs. Front. Oncol. 11:643199. doi: 10.3389/fonc.2021.643199
Received: 17 December 2020; Accepted: 01 February 2021;
Published: 25 March 2021.
Edited by:
Pasquale Pisapia, University of Naples Federico II, ItalyReviewed by:
Alessandro Russo, A.O. Papardo, ItalyLucia Anna Muscarella, Casa Sollievo della Sofferenza (IRCCS), Italy
Copyright © 2021 Ma, Li, Wang, Hu, Yu, Zhang, Qin, Zhang, Li, Zhang, Wu, Lv, Yang, Yu, Zhang and Wang. This is an open-access article distributed under the terms of the Creative Commons Attribution License (CC BY). The use, distribution or reproduction in other forums is permitted, provided the original author(s) and the copyright owner(s) are credited and that the original publication in this journal is cited, in accordance with accepted academic practice. No use, distribution or reproduction is permitted which does not comply with these terms.
*Correspondence: Jinghui Wang, jinghuiwang2006@163.com; Shucai Zhang, sczhang6304@163.com
†ORCID: Jinghui Wang, orcid.org/0000-0002-4999-2106
‡These authors have contributed equally to this work