- 1Key Laboratory of Systems Biomedicine (Ministry of Education), Shanghai Center for Systems Biomedicine, Shanghai Jiao Tong University, Shanghai, China
- 2Shenzhen People’s Hospital, Second Clinical Medical College of Jinan University, Shenzhen, China
- 3Department of Obstetrics and Gynecology, Minhang Hospital, Fudan University, Shanghai, China
- 4Department of Clinical Laboratory, Jiangsu Health Vocational College, Nanjing, China
Cervical cancer (CC) is one of the most common gynecological malignant tumors. The 5-year survival rate remains poor for the advanced and metastatic cervical cancer for the lack of effective treatments. Immunotherapy plays an important role in clinical tumor therapy. Neoantigens derived from tumor-specific somatic mutations are prospective targets for immunotherapy. Hence, the identification of new targets is of great significance for the treatment of advanced and metastatic cervical cancer. In this study, we performed whole-exome sequencing in 70 samples, including 25 cervical intraepithelial neoplasia (CINs) with corresponding blood samples and 10 CCs along with paired adjacent tissues to identify genomic variations and to find the potential neoantigens for CC immunotherapy. Using systematic bioinformatics pipeline, we found that C>T transitions were in both CINs and CCs. In contrast, the number of somatic mutations in CCs was significantly higher than those in CINs (t-test, P = 6.60E-04). Meanwhile, mutational signatures analysis revealed that signature 6 was detected in CIN2, CIN3, and CC, but not in CIN1, while signature 2 was only observed in CCs. Furthermore, PIK3CA, ARHGAP5 and ADGRB1 were identified as potential driver genes in this report, of which ADGRB1 was firstly reported in CC. Based on the genomic variation profiling of CINs and CCs, we identified 2586 potential neoantigens in these patients, of which 45 neoantigens were found in three neoantigen-related databases (TSNAdb, IEDB, and CTDatabase). Our current findings lay a solid foundation for the study of the pathogenesis of CC and the development of neoantigen-targeted immunotherapeutic measures.
Introduction
Cervical cancer (CC) is one of the most common gynecological malignant tumors, second only to breast cancer, which occupies the second place in the incidence of women’s malignant tumors in China. There are about half a million new cases of CC every year, and more than 80% of these cases are in developing countries (1, 2). The prognosis of patients with CC is closely related to clinical stage, pathological type, treatment method, patient compliance, etc. Early detection, early diagnosis, and early treatment are the keys to the treatment of CC. Some patients with early cervical cancer can be cured through surgery, chemotherapy, etc. Platinum-based chemotherapy regimens, including cisplatin, carboplatin, fluorouracil, and paclitaxel, are often used in patients with CC (3). However, many patients with advanced cervical cancer and those with metastases to other tissues and organs have a poor prognosis. Hence, the identification of new targets for treatments of CC, especially for immunotherapy, is of great significance for the treatment of advanced and metastatic cervical cancer.
With the development of immunotherapeutic technologies, Immune Check-Point (ICP) inhibitors have been used against different human malignancies, which include metastatic melanoma, bladder cancer, stomach cancer, renal cell cancer, head, neck cancer, etc (4). Compared with the traditional cancer cell inhibition therapies, ICP inhibitors can extend the survival in advanced and metastatic cancer patients who are previously thought to be incurable (5). Up till the present moment, bevacizumab and pembrolizumab are two FDA-approved immunotherapy options for CC treatment, which are only responded in a minority of CC. Therefore, it is vitally important to discover attractive immunotherapeutic targets for CC therapy.
Derived from the somatic mutations, neoantigens only exist on cancer cells, which can have vital roles in tumor-specific T cell-mediated antitumor immunity (6–9). Neoantigens on the cell surface can bind to major histocompatibility complex (MHC) molecules, which can be recognized by T cells and then elicit immune responses (10). It was reported that the patients with high tumor mutation load were apt to benefit from immunotherapies, including PD-L1 and CTLA-4, and other immunotherapeutic technologies (11, 12). Furthermore, it is crucial that neoantigens in CCs can be identified to develop the neoantigen-targeted immunotherapies.
Recently, some studies have analyzed the genomic variations of CC in detail and proved that several mutated genes, such as PIK3CA, PTEN, EP300, TP53, FBXW7, MAPK, HLA-A, and CASP8 (13–17), which are closely related to the occurrence and development of CC. However, the use of genetic mutation information to identify neoantigens of CINs and CCs is limited. Qin et al. used two available public data sets, including The Cancer Genome Atlas cohorts of Cervical squamous cell carcinoma and endocervical adenocarcinoma (TCGA-CESC) data set and the exome and RNA sequencing data [published by Ojesina et al. (13)], to analyze the neoantigen landscape in CC (18). Li et al. investigated cancer epitope trees in early cervical cancer patients by whole-exome sequencing. Each patient displays a unique phylogenetic tree in which almost all subclones harbored neoantigens. These literatures only studied the relationship between mutations and neoantigens in CC (19). However, it is still not quite clear about the landscape of neoantigens during the stepwise process from CINs to CCs.
Herein, we systematically evaluated the genomic variations to identify the potential neoantigens and find better immunotherapeutic targets for CC treatment. We performed a whole-exome sequencing analysis (WES) of CINs and CCs. Our results showed that there were different distributions of the somatic mutated genes with different stages. Our findings could refine our knowledge concerning the occurrence and development of cervical cancer, and were intended to provide attractive immunotherapeutic targets to research and develop the new treatments for cervical cancer.
Materials and Methods
Clinical Samples
For physical examination volunteers and patients suffering from CINs and CCs, the written informed consent was obtained from each person before enrollment. The cervical exfoliated cells were sampled using cervical brushes for each enrolled patient, which were used to observe the morphological characteristics based on the liquid-based cytology (Becton Dickinson Company, New Jersey, USA). In Shenzhen People’s Hospital, cervical liquid-based cytological test was used as a routine screening test for CC. Hence, those patients with cytological abnormality were recommended to accept colposcopy examination. And then the colposcopy findings were used to determine if a biopsy is necessary. The diagnoses of CIN1, CIN2, CIN3, or CCs were made and reviewed by two pathologists independently (Smith et al., 2018). This study was approved by the ethical review board of Shenzhen People’s Hospital (SPH-2016010).
A total of 70 samples from 35 patients suffering from CINs and CCs were enrolled in this study. The blood samples from CINs and the paired adjacent paracancer tissues from CCs were used as the control. The biopsies from CINs and the surgically resected tumors and paired adjacent paracancer tissues were snap-frozen in liquid nitrogen and stored at −80°C. Blood samples were stored at −20°C. All tissues were diagnosed by two independent pathologists using hematoxylin and eosin (H&E) staining. The tumor cell content of the analyzed CCs was all more than 75% (ranging from 75% to 90%), while no tumor cells were observed in adjacent tumor tissues and CIN samples (Supplementary Figure 1). A detailed description of clinical information was found in Supplementary Table 1, which includes source, age, FIGO stage, grade, and pathological type. Besides, there were no significant differences in age among the four groups (Kruskal-Wallis chi-squared test, P = 0.06).
DNA Extraction and Whole-Exome Sequencing
Genomic DNA was extracted from the blood and tissue sample using QIAGEN Blood DNA Mini Kit (Qiagen, Hilden, Germany) and QIAGEN DNA Mini Kit (Qiagen, Hilden, Germany) according to the manufacturer’s protocol. Whole-exome capture was done with Roche SeqCap EZ exome V3.0 (Roche Sequencing, Pleasanton, CA), targeting 64 Mb sequences from exons. Captured libraries were analyzed on Agilent’s 2100 Bioanalyzer. DNA concentrations were measured using Qubit 2.9 Fluorometer (Thermo Fisher, Shanghai, CN). Seventy paired samples on the flowcells were sequenced with Illumina HiSeq X-Ten platform to generate 150-bp paired-end reads. The raw data were trimmed and assembled using Trimmomatic version 0.36 (20) to obtain clean data. Subsequently, the clean data were mapped to the reference human genome GRCh38 using Burrows-Wheeler Aligner (BWA) version 0.7.15 (21) and alignment information was stored in *.sam format. The samtools version 1.3.1 software was employed to converted SAM files into BAM files (http://samtools.sourceforge.net/SAM1.pdf) for each sample (22). Duplicate reads were removed using Picard Tools (http://broadinstitute.github.io/picard/) version 2.17.11 according to the standard data pipeline of the Broad Institute. Local realignments and base quality recalibrations were performed using the Genome Analysis Toolkit (GATK) version 3.8.0 (23).
Variation Calling Analysis
Somatic SNVs were identified using Strelka (24) algorithm and somatic small insertions or deletions (indels) were detected using VarScan2 (25) algorithm, respectively. All the software was performed for identifying mutations in matched tumor-normal samples with the default parameters. Then, this pipeline was applied as a filter criterion (based on a stringent criterion) to obtain a high-confidence somatic SNVs and Indels. In brief, a mutation was identified as a candidate somatic mutation only when (I) the depth of coverage was no less than 14 in the tumor samples and no less than 8 in the matched control samples; (II) the reads according to the altered allele in the tumor were no less than 5; (III) the fraction of reads according to the altered allele in the tissue was no less than 5% and in the matched control was less than 3%; (IV) the fraction of the reads according to the altered allele frequency in the tumor samples was four-fold or more than in the matched control samples. Finally, mutations were filtered to exclude intronic and silent changes and to retain mutations resulting in missense mutations, nonsense mutations, frameshifts, or splice site alterations. Candidate somatic mutations were further annotated using ANNOVAR (26) for information related to the location, function, previous reports and sequencing data supporting the status of the mutation. Then it converted to MAF files with vcf2maf (https://github.com/mskcc/vcf2maf). The gene functions on mutated genes by using the public database GeneCards (https://www.genecards.org/) (27).
Copy number analysis was performed using CNVkit (28). To obtain copy number alterations in CINs and CCs, genomic identification of significant targets in cancer (GISTIC) 2.0 version 6.2 with join segment size = 4; confidence level = 0.95, and q-value FDR < 0.25 was used (29). Heatmap plots were generated with the MATLAB “colormap” function in GISTIC with segmented Copy Number files.
Somatic mutation signatures were estimated by the Bioconductor package deconstructSigs (30), which identified a linear combination of pre-defined signatures that most accurately reconstructed the mutational profile of a tumor sample. These candidate signatures were compared with the COSMIC signatures. Each mutational signature was assigned a calculated weight representing its contribution to the case samples, where a higher weight value indicates a greater relative contribution of the signature.
Because of the limited numbers of each group sample available for this study cohort, we took a strict enumeration approach to identify potential driver genes. In brief, a gene was considered to be a significantly mutated gene if the following criteria were satisfied: (I) the candidate driver genes were observed in at least 3 out of 35 cases; (II) the candidate driver genes need to be observed in the catalogs of 568 mutational driver genes (31); (III) the candidate significantly mutations were deemed to be deleterious (either a stop-gain or predicted deleterious in two of the three computational approaches applied by SIFT (http://sift.jcvi.org/), Polythen2 (http://genetics.bwh.harvard.edu/pph2/) and MutationTaster (http://www.mutationtaster.org/); The remain mutated genes were considered as potential driver genes.
HLA Types and Neoantigens Analysis
HLA types for all the patients were performed computationally using POLYSOLVER (32), which used a BAM file as input and employed a Bayesian classifier to determine genotype in four-digit resolution. Likewise, each non-synonymous SNV was translated into a 22mer peptide sequence that centered on the mutated amino acid. Subsequently, the 22mer was separated into 8-11mer via a sliding window for detecting MHC class I binding, which was known to be the possible lengths for peptides presented by human MHC class I molecules. We then predicted MHC binding affinity for each peptide as described previously (33). NetMHCpan 4.0 (34) tool was used to determine the binding affinity strength of every mutated peptide to patient-specific HLA alleles for identifying exome-derived neoantigens. And then, the putative neoantigens binders were identified as those with a predicted binding affinity IC50< 500 nM and %Rank < 2%. When RNA-seq available, a neoantigen was considered to be expressed if a mutated gene TumorFPKM ≥ 0.5 and |log2(TumorFPKM/NormalFPKM)|≥0.1, FPKM, Fragments Per Kilobase per Million.
Variation Analysis of Public Data
Publicly available somatic mutation profiles were obtained from The Cancer Genome Atlas (TCGA) repositories (https://tcga-data.nci.nih.gov/tcga/), including cervical squamous cell carcinoma and endocervical adenocarcinoma (CESC), head and neck squamous cell carcinoma (HNSC), esophageal carcinoma (ESCA), lymphoid neoplasm diffuse large B-cell lymphoma (DLBC), liver hepatocellular carcinoma (LIHC), ovarian serous cystadenocarcinoma (OV), uterine carcinosarcoma (UCEC), and uveal melanoma (UCS), which were used to analyze the mutation spectrums and mutational signatures related to our results. Raw RNA-seq data that were downloaded from NCBI SRA (SRA315538) were performed to validate the expression of the predicted neoantigens (16).
Statistical Analysis
All analyses were performed in the R statistical environment version ≥3.5.1. All data were expressed as the mean ± standard deviation (SD) unless otherwise stated. A two-sided Student’s t-test was used to compare quantitative data between two groups and Kruskal-Wallis chi-squared were used to compare categorical data among more groups. Pearson’s correlation coefficient was used to evaluate the linear correlation. P-values of <0.05 were considered to be statistically significant.
Results
Genomic Alterations in CINs and CCs
First, whole-exome sequencing was performed on 35 cervical neoplasia genomes and matched control samples, including blood cells and paired adjacent paracancer, using the Illumina HiSeq X-ten platform. In total, 99.96% of the clean reads successfully mapped to the reference genome (GRCh38 release). Each sample had at least 64 Mb of target exons covered with an average depth of 95.9× (range from 79.9× to 123×). More than 90.06% of the targeted regions were covered effectively with at least 30× reads (Supplementary Figure 2 and Supplementary Table 2). A concise flowchart was schematically illustrated in Figure 1.
To identify the somatic mutations in CINs and CCs, SNVs, and Indels were called by using Strelka (24) and VarScan2 (25), respectively. In total, we detected 3,489 somatic mutations (see Materials and Methods section for details) in exons and splicing regions, including 2080 non-synonymous SNVs, 969 synonymous SNVs, 203 nonsense, 52 splicing, 139 frameshifts, 46 in-frameshifts. The average number of mutations was 330.5 in CCs (range from 27 to 1424), which was significantly higher than that in CIN1 (6.45), CIN2 (6.80), or CIN3 (9.67), respectively (Kruskal-Wallis chi-squared test, P = 8.13E-05). These results showed that the number of somatic mutations was distinctly increasing from CINs to CCs (Figure 2A, upper panel and Supplementary Table 3).
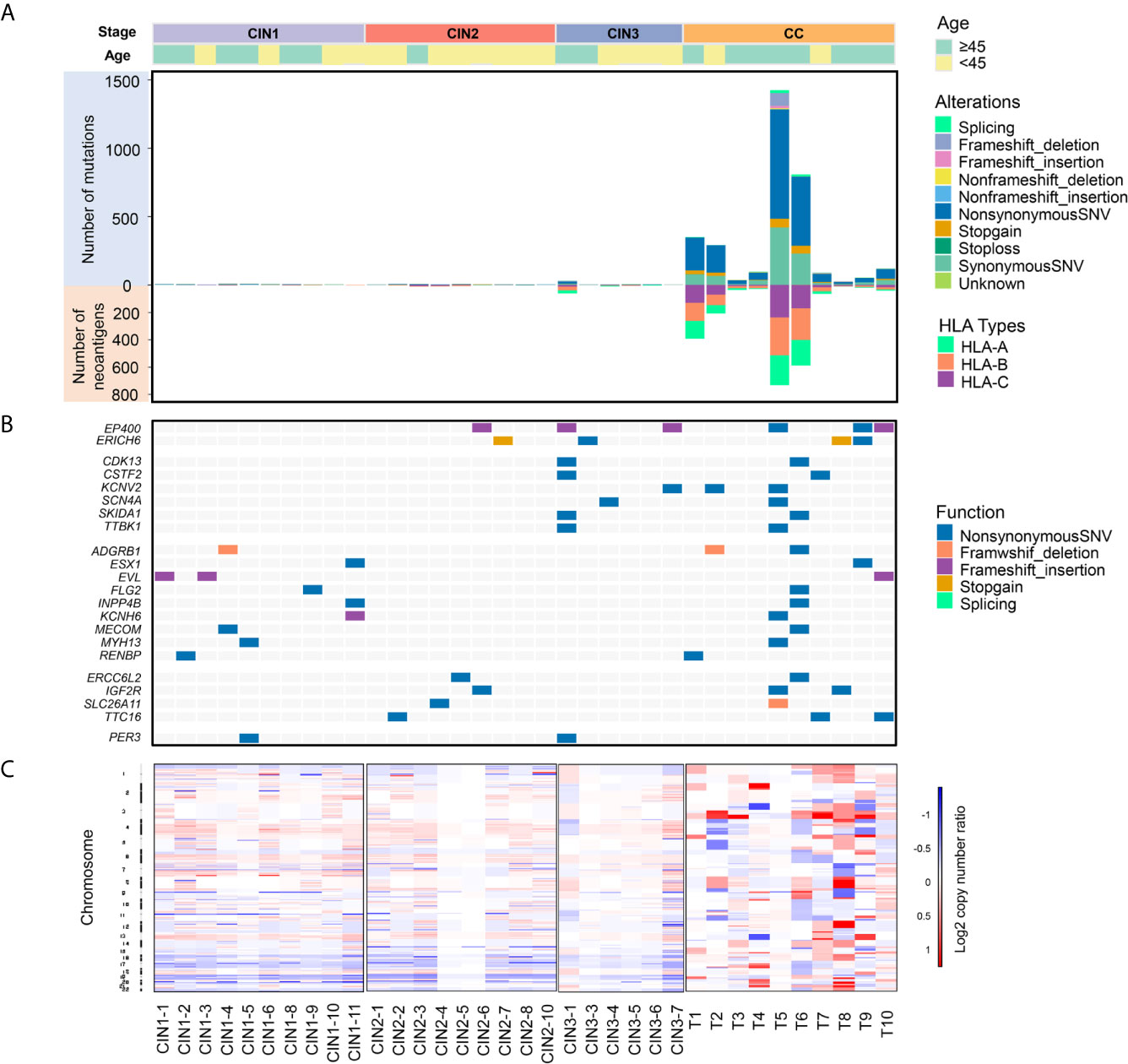
Figure 2 A comprehensive mutational feature of 35 cervical neoplasia genomes by whole-exome sequencing. (A) Upper panel: The number of all the mutations in genomes was depicted. Each bar represented an individual cervical neoplasia sample. Different colors represented different mutation types. The number of somatic mutations in CCs were significantly higher than in CINs (t-test, p = 6.60E-04). Lower panel: Predicted HLA class I neoantigens in 35 cervical neoplasia samples. Different colors represented different HLA types. The number of exome-derived neoantigens in CCs were significantly higher than in CINs (t-test, p = 3.62E-04). (B) A heatmap showed the genes were both detected in CINs and CCs. (C) DNA copy number segments of 35 cervical neoplasia patients showed that CNAs events were detected more frequently in CCs than in CINs. Red and blue boxes represented copy number amplification and deletion events, respectively (x-axis, 35 cervical neoplasia patients in each group; y-axis, chromosome number).
Furthermore, we analyzed the 2474 mutations involving in 2125 genes, including 2080 non-synonymous SNVs, 203 nonsense, 52 splicing and 139 frameshifts, 95.1% of which (2,363 mutations involving in 2,021 genes) were common with those reported in the TCGA-CESC data set. Among the mutations, 94.62% (2,341/2,474) of them were detected only in CCs, which indicated that the genomic alterations mainly occurred in CCs. Notably, 63 mutations in 22 genes were both observed in CINs and CCs. To validate our findings, we compared these mutated genes with those in other databases, such as COSMIC, TCGA-CESC data set, and COMSIC-CGC. The results showed that these mutated genes were all overlapped in COSMIC and TCGA-CESC, while only three mutated genes were in COMSIC-CGC database (Figure 2B and Supplementary Table 4). EP400(6/35) and ERICH6(4/35) were observed in CIN2, CIN3, and CCs, as well as CDK13, CSTF2, KCNV2, SCN4A, SKIDA1, and TTBK1 were observed in CIN3 and CCs. All of these genes were detected in a consecutive group, which was a novel discovery in this cohort that was never prominently reported in previous studies (13–16). These genes in CINs and CCs, such as EVL, INPP4B, IGF2R, and ADGRB1, were involved into some well-defined cancer-related pathway, including RTK/RAS/PI(3)K, Wnt/β-catenin, p53/mTOR, cell cycle, and DNA damage, suggested that these mutated genes might be novel biomarkers for the early diagnosis and treatment of CC (Supplementary Table 5). Also, there were 70 mutations involving 70 genes in CIN1 or CIN2 or CIN3, while not in CCs. IKBKB, TNFRSF10A, and IL1R1 were involved into the apoptosis-related pathways, suggesting that these mutated genes in CINs might be associated with early apoptosis events (Supplementary Table 5). The sequence quality, including all mutant sites, was carefully validated by integrative genomics viewer (IGV) (35) according to the criterion for variation calling (Supplementary Figure 3 and Supplementary Table 4).
Meanwhile, we also analyzed DNA copy number alterations (CNAs) segments by using WES data and identified 6, 6, 3, and 14 CNAs in CIN1, CIN2, CIN3, and CCs, respectively. These results also implied that the genomic instability and alterations had a stepwise increase during the development of CC and suggested that CNAs may also occur in CINs stage. In addition, we found that significant gains occurred in chromosomes 1q, 3q, 13p, and 19q, while there are significant losses in chromosomes 6p and 16q in CCs. Our results were consistent with previous reports (13, 15–17) (Figure 2C and Supplementary Figure S4).
Mutational Signatures in CINs and CCs
In this study, we found that C>T/G>A transitions and C>G/G>C transversions dominated the mutation spectrum in both CINs (CIN1: C>T/G>A-56%, C>G/G>C-6%; CIN2: C>T/G>A-56.25%, C>G/G>C-4.17%; CIN3: C>T/G>A-61.22%, C>G/G>C-6.12%) and CCs (C>T/G>A-63.47%, C>G/G>C-21.85%) (Figure 3A). Interestingly, in the development process from CINs to CCs, the frequency of C>A/G>T, C>T/G>A, and C>G/G>C displayed a trend of gradual increase, while the frequency of A>T/T>A, A>C/T>G, and A>G/T>C displayed a trend of gradual decline (Figure 3B). C>T/G>A transition was the highest-frequency substitution type in CCs. These results indicated that the occurrence and development of cervical cancer may be closely related to C or G base damage in the genome caused by some etiologies, which are supported by previous reports (13, 15, 16).
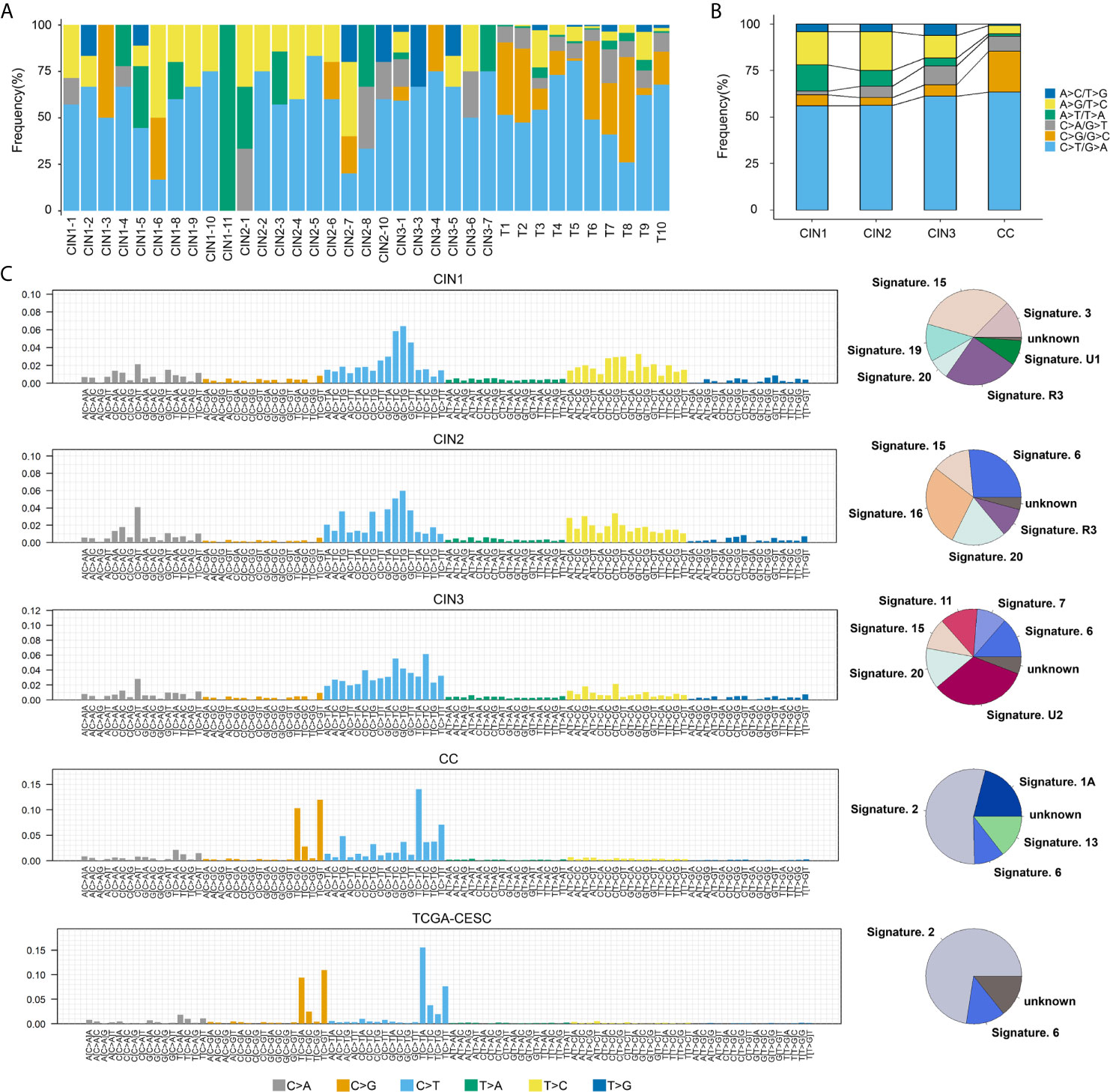
Figure 3 The mutation spectrums and mutational signatures in CINs and CCs. (A) Mutation substitution types identified the 6 substitutions classification on the basis of the nucleotide frequency of the human genome among all samples. The horizontal axes indicated the cervical neoplasia patients ID, and the vertical axes depicted the percentage of mutations categories to a specific mutation type. C>T/G>A transitions were predominant across most of cervical neoplasia patients. The horizontal axis indicated the sample ID, and the ordinate corresponds to the number of mutation types. (B) The trend of each nucleotide substitution subtype in CIN1, CIN2, CIN3, and CCs. (C) Mutational signatures in CIN1, CIN2, CIN3, CC, and TCGA-CESC, receptively. Proportion of mutation signatures distribution in each group (on the left). Inset pie chart shows the proportion of mutation signatures in each group (on the right).
It is well known that the diversity of mutational processes underlying the development of cancer, with potential implications for understanding of cancer aetiology, prevention, and therapy, and certain mutational signatures reveal the specific of mutational processes and the underlying aetiologies of cancer development (36). The identified mutational signatures were signature 15 (32.9%)/19 (12.7%), signature 6 (26.5%)/16 (28%), signature 6 (13.6%)/20(13.7%), and signature 2 (54.2%)/6 (10.2%) for CIN1, CIN2, CIN3, and CCs, respectively (Figure 3C). Signature 6 was detected in CIN2, CIN3, and CCs, while not in CIN1, suggesting that signature 6 may be of a novel biomarker for CC early warning. In contrast, the overall mutational signatures in CCs were signature 2 (54.2%) and signature 6 (10.2%), which were highly consistent with the TCGA-CESC data set [signature 2 (72.5%)/6 (13.2)] (Figure 3C). Signature 2 is due to over activity of members of the APOBEC family of cytidine deaminases, which convert cytidine to uracil, coupled to activity of the base excision repair and DNA replication machineries (36). We detected that signature 2 was observed notably in CCs, but not in CINs. Indeed, it has been reported that APOBEC cytidine deaminases displayed are strongly associated with cervical cancer in the previous reports (13, 15, 37). In all, these findings indicated that signature 2 was a predominant source of mutation signatures in CCs, which was dramatically distinct from CINs.
In addition, we compared the mutation spectrums and mutational signatures with two HPV+-associated cancers (CESC and HNSC), three virus-associated cancers (ESCA, DLBC, and LIHC), and three gynecological cancers (UCEC, UCS and OV), which were from TCGA repositories. It showed that C>T/G>A transitions (57.52%) and C>G/G>C transversions (21.88%) accounted for the highest proport in HPV+-associated cancers, C>T/G>A transitions (43.6%), C>A/G>T transversions (15.46%), and A>G/T>C transitions (15.07%) accounted for the highest proport in virus-associated cancers, and C>T/G>A transitions (42.25%) and C>A/G>T transversions (22.08%) accounted for the highest proportion in gynecological cancers (Figures 4A, B). Also, C>T/G>A transitions was the highest proportion in three different cancer types. Besides, the average proportion of C>T/G>A transitions and C>G/G>C transversions in HPV+-associated cancers were much higher than those in virus-associated cancers and gynecological cancers, whereas the proportion of C>A/G>T transversions in gynecological cancers was higher than that in HPV+-associated cancers and virus-associated cancers (Figure 4B and Supplementary Table 6). Moreover, signature 2 accounted for a relatively large proportion in HPV+-associated cancers, but not in virus-associated cancers and gynecological cancers, which may be a novel biomarker for the screening and treatment in HPV+-associated cancers. However, there were no similar signatures in virus-associated cancers or gynecological cancers (Figure 4C). These results suggested that different cancer types showed the different mutation spectrums and mutational signatures.
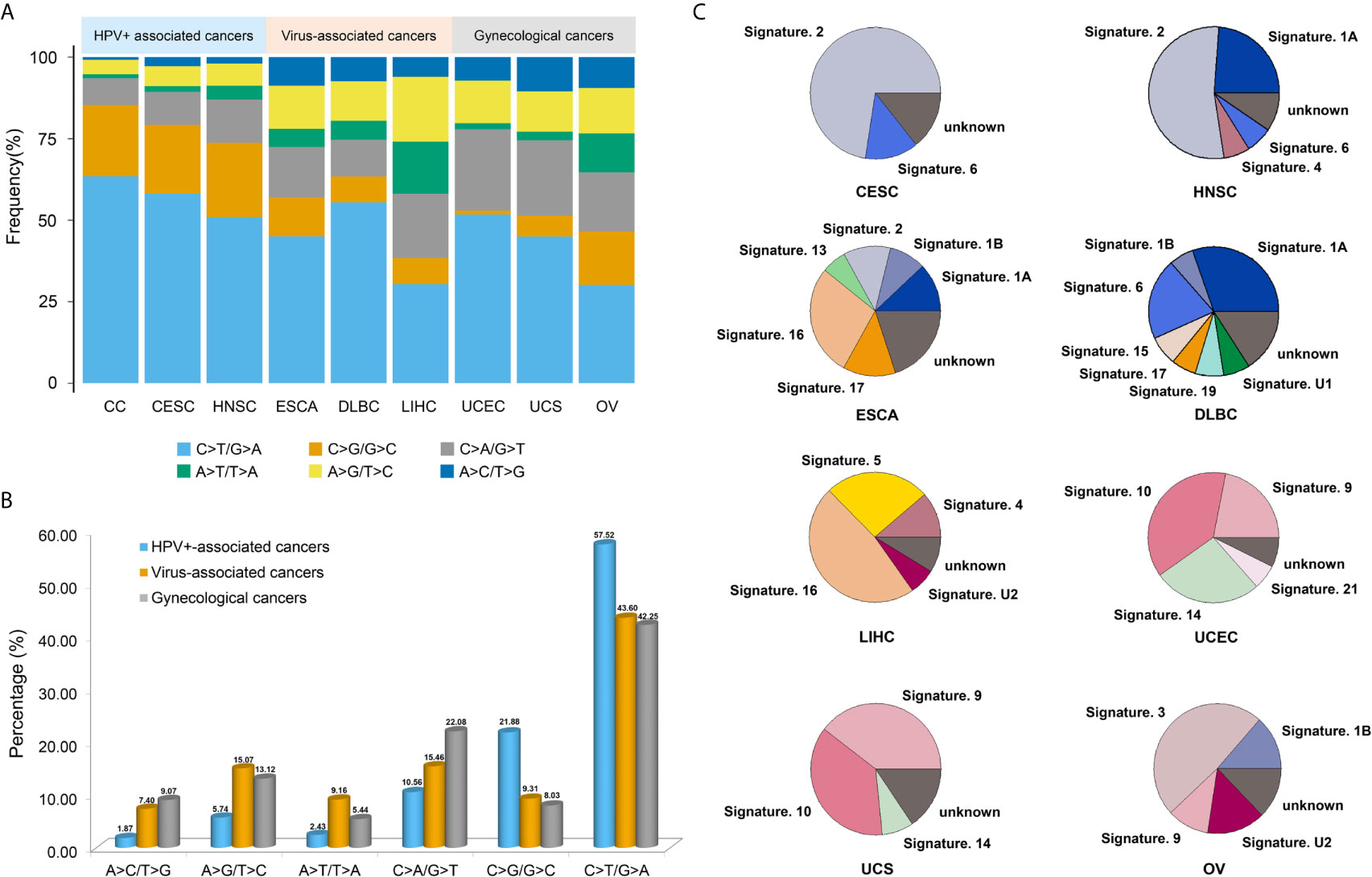
Figure 4 The mutation spectrums and mutational signatures in three different cancer types. (A) Barplots showed the proportion of each substitution subtype in HPV+-associated cancers, virus-associated cancers and gynecological cancers. (B) The average proportion of each substitution subtype in three different cancer types. (C) The proportion of each mutational signatures in HPV+-associated cancers, virus-associated cancers and gynecological cancers.
Potential driver genes in CCs
We analyzed significantly somatic mutations on 35 cervical neoplasia genomes and identified three driver genes (Table 1) according to a filtering criterion (see Materials and Methods section for details). PIK3CA and ARHGAP5 were observed in the previous reports for CC (13–16), while ADGRB1 was a novel driver gene in CC. Notably, there were no driver genes were detected in CINs.
PIK3CA (phosphatidylinositol 3-kinase catalytic subunit alpha), one of the most frequently mutated genes, was only detected in CCs. In our current study, PIK3CA point mutations were detected in 60% (6/10) CCs, which was higher than that reported in previously genome sequencing studies [27.3% in TCGA-CESC data set; 26% in Cancer Genome Atlas Research Network et al. (15); 12.6% in Ojesina et al. (13); 16.67% in Huang et al. (16); 53.33% in Chung et al. (17)]. Moreover, mutations in PIK3CA were detected at commonly observed nucleotide positions R88Q, E545Q and H1047L in three CCs (38). Expect the substitutions R38C, all of the mutations in PIK3CA had been reported in cervical adenocarcinoma in the COSMIC database_v90 (https://cancer.sanger.ac.uk/cosmic/). ARHGAP5 (Rho GTPase activating protein 5) was identified only in CCs with 30% (3/10) mutation ratio, of which all mutation sites had already been reported in COSMIC, as well as, two mutations (G1951A and G416A) were probably damaging (Table 1). ADGRB1 (adhesion G protein-coupled receptor B1), a newly recognized driver gene in CCs with 20% (2/10) mutation ratio, of which one mutation was probably damaging (Table 1). COSMIC database showed that ADGRB1 was a moderately over-expressed in 6.84% (21/307) of CCs, and there were many types of variants, including somatic mutations, CNVs and dysregulation in ADGRB1 in a quantity of cancers. Similar to the previous reporting the driver genes in CC (13, 15, 16), FBXW7, EP300, CASP8 and FAT1(10%, 20%, 10% and 10% of tumor, respectively) were also identified in our cohort with a low frequency, which may result from a small sample size in our cohort.
Discovery the Neoantigens in Both CINs and CCs
To obtain more data to identify neoantigens, we downloaded both WES data and RNA-seq data from four pairs of CC samples (16). Of which the four WES data was also used to predict the neoantigens, while the RNA-seq data were used to examine the expression of neoantigens.
Then we performed POLYSOLVER to identify the four-digit HLA class I alleles in 39 samples (4 samples plus 35 samples). We found that HLA-A*26:01and HLA-C*07:02 were the most common alleles at HLA-A and HLA-C loci in both CCs and CINs (CCs-HLA-A: 42.86%, CCs-HLA-C: 42.86%; CINs-HLA-A: 20%, CINs-HLA-C: 20%). For HLA-B, the most prevalent alleles were HLA-B*15:18 (35.71%) in CCs, while HLA-B*40:01 (12%) was the most prevalent allele in CINs (Table 2).
Moreover, we performed NetMHCpan 4.0 program to predict the neoantigens using the parameters of %Rank < 2% and IC50 <500 nM. We identified 2,586 potential neoantigens in CINs and CCs (Supplementary Table 7). CINs had a median of 3.1 non-synonymous mutations (range, from 1 to 16) with a mean of 5.3 neoantigens (range, from 1 to 61), whereas CCs had a higher mutation burden (median, 162.7; range, from 15 to 800) with a mean of 175.5 neoantigens (range, 5 to 732) (Table 2 and Figure 2A, lower panel). The number of non-synonymous mutations showed a strong linear correlation with the number of neoantigens (Pearson’s correlation coefficient r = 0.96, P = 6.19E-22) (Figure 5A) that were consistent with previous research on TCGA-CESC data set (Pearson’s correlation coefficient r = 0.9, P <0.001) (39).
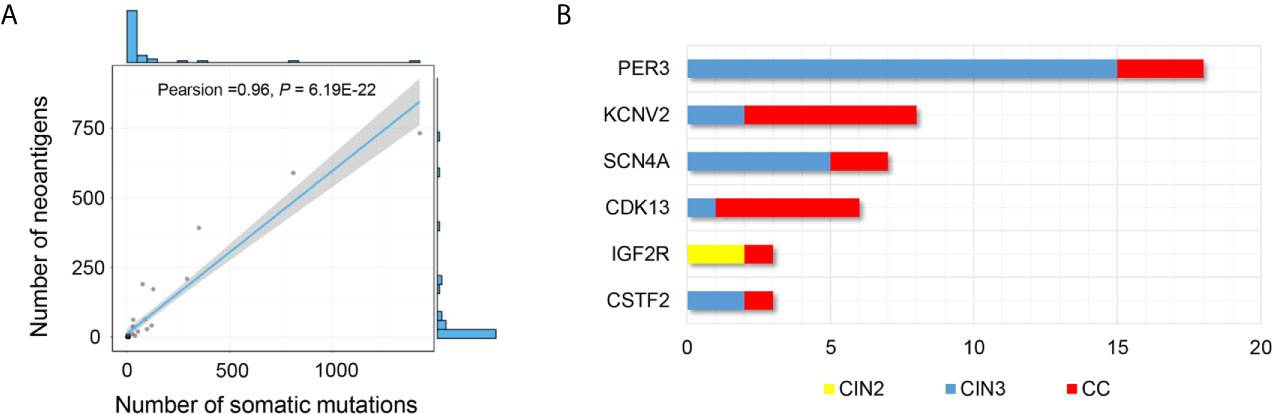
Figure 5 Distribution of potential neoepitopes genes during stepwise cervical carcinogenesis. (A) Correlation between the number of mutations and the number of exome-derived neoepitopes (Pearson’s correlation coefficient, r = 0.96, P = 6.19E-22). (B) Barplots showed the number of PNeoGs in both CINs and CCs.
In addition, we identified 6 “potential neoantigens genes” (PNeoGs) in both CINs and CCs: five PNeoGs in both CIN3 and CCs, one PNeoG in both CIN2 and CCs (Figure 5B and Supplementary Table 7). For example, IGF2R was an immune-related gene (IRGs), deposit in the Immunology Database and Analysis Portal (ImmPort). Besides, it was reported that IGF2R has an oncogenic role through transportation mannose-6-phosphate (M6P)-tagged in CCs (40). These results indicated that neoantigens could be generated in the CIN stage. If it is the case, neoantigen-based immunotherapeutic measures might be useful for prevent of CC.
It is one interesting question whether the genes corresponding to these neoantigens are expressed? To answer this question, we used the RNA-seq data to examine the expression of neoantigens. The result showed that there were 332 potential neoantigens in four pairs of samples, 50.9% (167/332, 167 neoantigens involving in 59 genes) of which were expressed (Supplementary Table 8).
Validation the Potential Neoantigens by Public Data
To verify the accuracy of our results and to discover the potential immune therapeutic targets, we compared our results with Tumor-Specific Neoantigen database (TSNAdb) (41), Immune Epitope Database (IEDB) (42), and CTDatabase (43). Out of 2586 potential neoantigens, 45 neoantigens were overlapped in the three databases. In which 10 neoantigens involving into five proteins was in TCGA-CESC data set (TSNAdb), two neoantigens involving into two proteins in IEDB, and 33 neoantigens involving into 11 proteins in CTAs. Out of 18 neoantigen-related proteins, six proteins were reported to the therapeutic targets and the corresponding drugs in Therapeutic Target Database (44). For example, PIK3CA was an inclusion criterion in one clinical trial (NCT02957266) for directing cervical carcinoma therapy (45), as well as, ERBB3 and FBXW7 were considered as biomarkers in several clinical trials for malignant solid tumor (Table 3).
Interestingly, we found that almost all of immune targets occurred only in one sample. To validate the result, we analyzed the frequency of potential neoantigens in the similar data sets, such as TCGA-CESC. The result showed that there were 7,748 neoantigens in a total of 286 samples, only 49 neoantigens appeared simultaneously in at least two samples, and nine neoantigens appeared simultaneously in three samples. These results were basically consistent with our results. In addition, only 10 of the identified neoantigens in our study overlapped with those in the TCGA-CESC database (Supplementary Table 9). It suggested that neoantigens have a large heterogeneity.
Taken together, respective public data validation results indicated a list of immune therapeutic targets, which can narrow the scope of immunological targets for cervical cancer immunotherapy. Due to individual differences, almost all patients in our cohort have found no neoantigens in common, which indicates that personalized immunotherapy maybe one of the most effective immunotherapies for cervical cancer.
Discussion
In this study, we performed a comprehensive analysis for the genomic variation profiling to identify potential neoantigens, which were taken as potential immune therapeutic targets for cervical cancer. This study is the first time to analyze the neoantigens in CINs and CCs by whole-exome sequencing.
Somatic mutations analysis revealed that genomic alterations occurred during the development from CINs to CCs, and 22 high frequently mutated genes were identified in both CINs and CCs. EP400, a known component of multiple histone acetylase complexes, was regarded as an E2-dependent regulator of human papillomavirus oncogene expression (46). PER3 was reported to be related to cancer development (47, 48). Meanwhile, we also observed that the CNA segments in the CCs were more susceptible than in CINs. Intriguingly, the mutation spectrum in CINs was the same as that in CCs, whereas mutation signatures revealed different mutational processes dominant in the progression of CC stages. Consistent with previous report (15), we identified signature 2, an APOBEC-related signature, was only observed in CCs. Notably, signature 6, in association with defective DNA mismatch repair, was detected in CIN2, CIN3, and CCs, but not in CIN1, indicating that signature 6 may be a novel biomarker for the early diagnosis and treatment of CC.
Three potential driver genes were identified in this study, including PIK3CA, ARHGAP5, and ADGRB1. Especially, ADGRB1 as a novel driver gene. PIK3CA was one of the most frequently mutated genes associated with cervical carcinoma and had been observed in our cohort. PIK3CA was only observed in CCs and was much higher than that reported in previously genome sequencing studies [14% in Ojesina et al. (13), 16.67% in Cancer Genome Atlas Research Network (15), 26% in Huang et al. (16)]. ADGRB1 identified in CCs was a newly recognized driver gene that was found in Aflatoxin-Associated Hepatocellular Carcinoma (49), suggesting that ADGRB1 play a vital role in cancer pathogenesis.
Tumor immunotherapy is a treatment method to control and eliminate tumors by restarting and maintaining the tumor-immune microenvironment and restoring the normal anti-tumor immune response. Neoantigens are important targets of immunotherapy, including monoclonal antibody immune checkpoint inhibitors, therapeutic antibodies, cancer vaccines, cell therapy, and small molecule inhibitors. In recent years, tumor immunotherapy has been gaining good news. Currently, it has demonstrated strong anti-tumor activity in the treatment of solid tumors, such as melanoma, non-small cell lung cancer, kidney cancer, and prostate cancer, and several tumor immunotherapies drugs have been approved for clinical application by the US Food and Drug Administration (FDA) (4, 50). However, the use of genetic mutation information to identify neoantigens of CINs and CCs is limited. In this study, we identified 2586 neoantigens during the stepwise process from CINs to CCs and found that the number of exome-derived neoantigens in CCs were significantly higher than that in CINs. These data also indicated that some of the neoantigens were occurred in the early stage of CCs, suggesting that the progression of cervical neoplasia genomes from CINs to CCs may affect the tumor microenvironment and lead to cancer development. One of the PNenGs, IGF2R, was a tumor suppressor gene, with a high frequency of loss-heterozygosity (LOH) and protein expression in diverse types of malignant cancer (51, 52). In particular, a recent study identified IGF2R as a poor prognostic biomarker for CC and demonstrated that it had oncogenic functions (40). We also identified that the somatic mutations at IGF2R were deemed to highly damaging and the exome-derived neoantigens were detected in both CINs and CCs. Furthermore, we also demonstrated IGF2R was correlated with a poor prognosis and was upregulated in tumor tissues compared with normal tissues in the TCGA-CESC data set (Supplementary Figure 5) (53). These results suggest that IGF2R expression or IGF2R somatic mutations and exome-derived neoantigens may be a novel biomarker for CC early warning, screening and therapy, although further extended clinical studies might be required.
Based on the present neoantigen-related public database, we identified 45 different neoantigens involving into 18 genes (Table 3), which were regarded to be candidate targets for CC immunotherapy. Based on the available public TCGA-CESC data set, we found that ERBB3 and DSG3 were significantly up-regulated at RNA levels in tumors compared to normal tissues, whereas C3orf70, SH2D3C, and SPAG9 were down-regulated in tumors. The down-regulated C3orf70 expression is closely related with a poor prognosis of cervical cancer (Supplementary Figure 5) (53). Our findings suggested that these genes could be used for neoantigen-targeted immunotherapies in CC in the future. Theoretically, the predicted neoantigens need to be synthesized and tested for reactivity by autologous T cells using various assays, such as ELISPOT, fluorescently labeled HLA tetramers, or barcode-labeled HLA multimers (54). Furthermore, we also analyzed the neoantigens derived from Indels mutations (Supplementary Table 3) (55). The results showed that 678 potential neoantigens involving into 142 indels were identified (Supplementary Table 10). Moreover, we compared these neoantigens with TSNAdb, IEDB, and CTAs database. We found that only SPAG17 protein were overlapped with CTAs, and none of them were overlapped in TSNAdb or IEDB database or the corresponding drugs in Therapeutic Target Database. In this study, because of the limited available clinical samples, we could not do the experimental verification to obtain the effective neoantigens. We will further study the mechanism of action of neoantigen-specific T cell–activating immunotherapeutic approaches in preclinical models of cervical cancer and extend the neoantigen-target immunotherapy approaches in large sample size of cervical cancer.
Up to now, the landscape of alterations and neoantigens from CINs to CCs is still unclear. One of the important reasons is clinical samples of CINs are usually small and intermixed with normal cells. By comparison with the previous study (18), our study has expanded the number of samples, especially in CIN1, to disclose genomic alterations from CIN1, CIN2, CIN3 to CCs. Furthermore, our study describes the neoantigen landscape during the stepwise processing from CINs to CCs and narrows the immune targets to provide insight for immunotherapy guidelines. Our present findings disclose an important clinical significance and cast light on the potential for early warning, diagnoses and immunotherapy in CC. Nonetheless, it is urgent to investigate a comprehensive molecular and integrative analyses for identifying attractive targets of CC immunotherapy via large-scale studies of genomic, transcriptomic, epigenomic, and proteomic data.
Conclusion
In conclusion, we systematically analyzed the comprehensive genomic variation profiling for identifying the potential exome-derived neoantigens in CINs and CCs. Our findings may yield insights into the similarities and differences in genomics between CINs and CCs and provide attractive targets to increase the effectiveness of immunotherapies in CC.
Data Availability Statement
The data sets presented in this study can be found in online repositories. The names of the repository/repositories and accession number(s) can be found below: https://www.ncbi.nlm.nih.gov/, PRJNA419579.
Ethics Statement
The studies involving human participants were reviewed and approved by SPH-2016010. The patients/participants provided their written informed consent to participate in this study.
Author Contributions
JH conceived the project and designed the experiments. CB, AN, and WH performed sequencing. CB and JH analyzed the sequencing data. WH performed the pathology experiments. JH contributed reagents, materials, and analysis tools. WH, HX, LX, JL, and BZ contributed the samples. CB and JH integrated, analyzed, and interpreted all data. JH contributed to the supervision of the work. CB and JH wrote the manuscript with the assistance and final approval of all authors. All authors contributed to the article and approved the submitted version.
Funding
This study was supported by grants from the Science and Technology Development Project of Pukou District, Nanjing (S2017-10); Health Fit Technology Project of Health Commission of Xinjiang Autonomous Region (FPSYTG-201901) and Scientific Research Project of Shanghai Health Commission (201840340).
Conflict of Interest
The authors declare that the research was conducted in the absence of any commercial or financial relationships that could be construed as a potential conflict of interest.
Supplementary Material
The Supplementary Material for this article can be found online at: https://www.frontiersin.org/articles/10.3389/fonc.2021.672386/full#supplementary-material
Supplementary Figure 1 | The result of H&E saining in 10 CC patients.
Supplementary Figure 2 | Detail information about sample data quality control (QC) of 35 cervical neoplasia genomes.
Supplementary Figure 3 | A heatmap showed the distribution of gene in CINs group. More detail information can be obtained in Supplementary Table S4.
Supplementary Figure 4 | Copy number profiles, amplification and deletion. GISTIC2.0 analyses of CNAs in 35 samples revealed significantly amplification and deletion regions peaks on several chromosomes. Red and blue lines represented copy number amplification and deletion events, respectively.
Supplementary Figure 5 | The expression of the neoantigen-related genes and the and prognosis informatics from GEPIA database. (A–F) The expression of the neoantigen-related genes. Red indicated tumor and grey indicated normal. (G, H) Kaplan–Meier analysis comparing the groups with a different expression of IGF2R and C3orf70 in TCGA-CESC.
References
1. Bray F, Ferlay J, Soerjomataram I, Siegel RL, Torre LA, Jemal A. Global Cancer Statistics 2018: GLOBOCAN Estimates of Incidence and Mortality Worldwide for 36 Cancers in 185 Countries. CA Cancer J Clin (2018) 68(6):394–424. doi: 10.3322/caac.21492
2. Cohen PA, Jhingran A, Oaknin A, Denny L. Cervical Cancer. Lancet (2019) 393(10167):169–82. doi: 10.1016/S0140-6736(18)32470-X
3. Schlecht NF, Kulaga S, Robitaille J, Ferreira S, Santos M, Miyamura RA, et al. Persistent Human Papillomavirus Infection As A Predictor of Cervical Intraepithelial Neoplasia. JAMA (2001) 286(24):3106–14. doi: 10.1001/jama.286.24.3106
4. De Felice F, Marchetti C, Palaia I, Ostuni R, Muzii L, Tombolini V, et al. Immune Check-Point in Cervical Cancer. Crit Rev Oncol Hematol (2018) 129:40–3. doi: 10.1016/j.critrevonc.2018.06.006
5. Schadendorf D, Hodi FS, Robert C, Weber JS, Margolin K, Hamid O, et al. Pooled Analysis of Long-Term Survival Data From Phase II and Phase III Trials of Ipilimumab in Unresectable or Metastatic Melanoma. J Clin Oncol (2015) 33(17):1889–94. doi: 10.1200/JCO.2014.56.2736
6. DuPage M, Mazumdar C, Schmidt LM, Cheung AF, Jacks T. Expression of Tumour-Specific Antigens Underlies Cancer Immunoediting. Nature (2012) 482(7385):405–9. doi: 10.1038/nature10803
7. Matsushita H, Vesely MD, Koboldt DC, Rickert CG, Uppaluri R, Magrini VJ, et al. Cancer Exome Analysis Reveals A T-cell-dependent Mechanism of Cancer Immunoediting. Nature (2012) 482(7385):400–4. doi: 10.1038/nature10755
8. McGranahan N, Furness AJ, Rosenthal R, Ramskov S, Lyngaa R, Saini SK, et al. Clonal Neoantigens Elicit T Cell Immunoreactivity and Sensitivity to Immune Checkpoint Blockade. Science (2016) 351(6280):1463–9. doi: 10.1126/science.aaf1490
9. Verdegaal EM, de Miranda NF, Visser M, Harryvan T, van Buuren MM, Andersen RS, et al. Neoantigen Landscape Dynamics During Human Melanoma-T Cell Interactions. Nature (2016) 536(7614):91–5. doi: 10.1038/nature18945
10. Vitiello A, Zanetti M. Neoantigen Prediction and the Need for Validation. Nat Biotechnol (2017) 35(9):815–7. doi: 10.1038/nbt.3932
11. Llosa NJ, Cruise M, Tam A, Wicks EC, Hechenbleikner EM, Taube JM, et al. The Vigorous Immune Microenvironment of Microsatellite Instable Colon Cancer Is Balanced by Multiple Counter-Inhibitory Checkpoints. Cancer Discovery (2015) 5(1):43–51. doi: 10.1158/2159-8290.CD-14-0863
12. Angelova M, Charoentong P, Hackl H, Fischer ML, Snajder R, Krogsdam AM, et al. Characterization of the Immunophenotypes and Antigenomes of Colorectal Cancers Reveals Distinct Tumor Escape Mechanisms and Novel Targets for Immunotherapy. Genome Biol (2015) 16(1):64. doi: 10.1186/s13059-015-0620-6
13. Ojesina AI, Lichtenstein L, Freeman SS, Pedamallu CS, Imaz-Rosshandler I, Pugh TJ, et al. Landscape of Genomic Alterations in Cervical Carcinomas. Nature (2014) 506(7488):371–5. doi: 10.1038/nature12881
14. Hu Z, Zhu D, Wang W, Li W, Jia W, Zeng X, et al. Genome-Wide Profiling of HPV Integration in Cervical Cancer Identifies Clustered Genomic Hot Spots and A Potential Microhomology-Mediated Integration Mechanism. Nat Genet (2015) 47(2):158–63. doi: 10.1038/ng.3178
15. Cancer Genome Atlas Research Network, Albert Einstein College of Medicine, Analytical Biological Services, Barretos Cancer Hospital, Baylor College of Medicine, Beckman Research Institute of City of Hope, et al. Integrated Genomic and Molecular Characterization of Cervical Cancer. Nature (2017) 543(7645):378–84. doi: 10.1038/nature21386
16. Huang J, Qian Z, Gong Y, Wang Y, Guan Y, Han Y, et al. Comprehensive Genomic Variation Profiling of Cervical Intraepithelial Neoplasia and Cervical Cancer Identifies Potential Targets for Cervical Cancer Early Warning. J Med Genet (2019) 56(3):186–94. doi: 10.1136/jmedgenet-2018-105745
17. Chung TK, Van Hummelen P, Chan PK, Cheung TH, Yim SF, Yu MY, et al. Genomic Aberrations in Cervical Adenocarcinomas in Hong Kong Chinese Women. Int J Cancer (2015) 137(4):776–83. doi: 10.1002/ijc.29456
18. Qin Y, Ekmekcioglu S, Forget MA, Szekvolgyi L, Hwu P, Grimm EA, et al. Cervical Cancer Neoantigen Landscape and Immune Activity Is Associated With Human Papillomavirus Master Regulators. Front Immunol (2017) 8:689. doi: 10.3389/fimmu.2017.00689
19. Li X, Huang H, Guan Y, Gong Y, He CY, Yi X, et al. Whole-Exome Sequencing Predicted Cancer Epitope Trees of 23 Early Cervical Cancers in Chinese Women. Cancer Med (2017) 6(1):207–19. doi: 10.1002/cam4.953
20. Bolger AM, Lohse M, Usadel B. Trimmomatic: A Flexible Trimmer for Illumina Sequence Data. Bioinformatics (2014) 30(15):2114–20. doi: 10.1093/bioinformatics/btu170
21. Li H, Durbin R. Fast and Accurate Long-Read Alignment With Burrows-Wheeler Transform. Bioinformatics (2010) 26(5):589–95. doi: 10.1093/bioinformatics/btp698
22. Li H, Handsaker B, Wysoker A, Fennell T, Ruan J, Homer N, et al. The Sequence Alignment/Map Format and Samtools. Bioinformatics (2009) 25(16):2078–9. doi: 10.1093/bioinformatics/btp352
23. DePristo MA, Banks E, Poplin R, Garimella KV, Maguire JR, Hartl C, et al. A Framework for Variation Discovery and Genotyping Using Next-Generation Dna Sequencing Data. Nat Genet (2011) 43(5):491–8. doi: 10.1038/ng.806
24. Saunders CT, Wong WS, Swamy S, Becq J, Murray LJ, Cheetham RK. Strelka: Accurate Somatic Small-Variant Calling From Sequenced Tumor-Normal Sample Pairs. Bioinformatics (2012) 28(14):1811–7. doi: 10.1093/bioinformatics/bts271
25. Koboldt DC, Zhang Q, Larson DE, Shen D, McLellan MD, Lin L, et al. VarScan 2: Somatic Mutation and Copy Number Alteration Discovery in Cancer by Exome Sequencing. Genome Res (2012) 22(3):568–76. doi: 10.1101/gr.129684.111
26. Wang K, Li M, Hakonarson H. Annovar: Functional Annotation of Genetic Variants From High-Throughput Sequencing Data. Nucleic Acids Res (2010) 38(16):e164. doi: 10.1093/nar/gkq603
27. Belinky F, Nativ N, Stelzer G, Zimmerman S, Iny Stein T, Safran M, et al. Pathcards: Multi-Source Consolidation of Human Biological Pathways. Database (Oxford) (2015) 2015):bav006. doi: 10.1093/database/bav006
28. Talevich E, Shain AH, Botton T, Bastian BC. Cnvkit: Genome-Wide Copy Number Detection and Visualization From Targeted DNA Sequencing. PloS Comput Biol (2016) 12(4):e1004873. doi: 10.1371/journal.pcbi.1004873
29. Mermel CH, Schumacher SE, Hill B, Meyerson ML, Beroukhim R, Getz G. Gistic2.0 Facilitates Sensitive and Confident Localization of the Targets of Focal Somatic Copy-Number Alteration in Human Cancers. Genome Biol (2011) 12(4):R41. doi: 10.1186/gb-2011-12-4-r41
30. Rosenthal R, McGranahan N, Herrero J, Taylor BS, Swanton C. Deconstructsigs: Delineating Mutational Processes in Single Tumors Distinguishes DNA Repair Deficiencies and Patterns of Carcinoma Evolution. Genome Biol (2016) 17:31. doi: 10.1186/s13059-016-0893-4
31. Martínez-Jiménez F, Muiños F, Sentís I, Deu-Pons J, Reyes-Salazar I, Arnedo-Pac C, et al. A Compendium of Mutational Cancer Driver Genes. Nat Rev Cancer (2020) 20(10):555–72. doi: 10.1038/s41568-020-0290-x
32. Shukla SA, Rooney MS, Rajasagi M, Tiao G, Dixon PM, Lawrence MS, et al. Comprehensive Analysis of Cancer-Associated Somatic Mutations in Class I Hla Genes. Nat Biotechnol (2015) 33(11):1152–8. doi: 10.1038/nbt.3344
33. Gubin MM, Zhang X, Schuster H, Caron E, Ward JP, Noguchi T, et al. Checkpoint Blockade Cancer Immunotherapy Targets Tumour-Specific Mutant Antigens. Nature (2014) 515(7528):577–81. doi: 10.1038/nature13988
34. Hoof I, Peters B, Sidney J, Pedersen LE, Sette A, Lund O, et al. NetMHCpan, a Method for MHC Class I Binding Prediction Beyond Humans. Immunogenetics (2009) 61(1):1–13. doi: 10.1007/s00251-008-0341-z
35. Thorvaldsdóttir H, Robinson JT, Mesirov JP. Integrative Genomics Viewer (Igv): High-Performance Genomics Data Visualization and Exploration. Brief Bioinform (2013) 14(2):178–92. doi: 10.1093/bib/bbs017
36. Alexandrov LB, Nik-Zainal S, Wedge DC, Aparicio SA, Behjati S, Biankin AV, et al. Signatures of Mutational Processes in Human Cancer. Nature (2013) 500(7463):415–21. doi: 10.1038/nature12477
37. Roberts SA, Lawrence MS, Klimczak LJ, Grimm SA, Fargo D, Stojanov P, et al. An APOBEC Cytidine Deaminase Mutagenesis Pattern is Widespread in Human Cancers. Nat Genet (2013) 45(9):970–6. doi: 10.1038/ng.2702
38. Burke JE, Perisic O, Masson GR, Vadas O, Williams RL. Oncogenic Mutations Mimic and Enhance Dynamic Events in the Natural Activation of Phosphoinositide 3-Kinase P110α (Pik3ca). Proc Natl Acad Sci U.S.A. (2012) 109(38):15259–64. doi: 10.1073/pnas.1205508109
39. Chen YP, Zhang Y, Lv JW, Li YQ, Wang YQ, He QM, et al. Genomic Analysis of Tumor Microenvironment Immune Types Across 14 Solid Cancer Types: Immunotherapeutic Implications. Theranostics (2017) 7(14):3585–94. doi: 10.7150/thno.21471
40. Takeda T, Komatsu M, Chiwaki F, Komatsuzaki R, Nakamura K, Tsuji K, et al. Upregulation of IGF2R Evades Lysosomal Dysfunction-Induced Apoptosis of Cervical Cancer Cells. via transport cathepsins. Cell Death Dis (2019) 10(12):876. doi: 10.1038/s41419-019-2117-9
41. Wu J, Zhao W, Zhou B, Su Z, Gu X, Zhou Z, et al. Tsnadb: A Database for Tumor-Specific Neoantigens From Immunogenomics Data Analysis. Genomics Proteomics Bioinf (2018) 16(4):276–82. doi: 10.1016/j.gpb.2018.06.003
42. Vita R, Mahajan S, Overton JA, Dhanda SK, Martini S, Cantrell JR, et al. The Immune Epitope Database (Iedb): 2018 Update. Nucleic Acids Res (2019) 47(D1):D339–43. doi: 10.1093/nar/gky1006
43. Almeida LG, Sakabe NJ, deOliveira AR, Silva MC, Mundstein AS, Cohen T, et al. Ctdatabase: A Knowledge-Base of High-Throughput and Curated Data on Cancer-Testis Antigens. Nucleic Acids Res (2009) 37(Database issue):D816–9. doi: 10.1093/nar/gkn673
44. Wang Y, Zhang S, Li F, Zhou Y, Zhang Y, Wang Z, et al. Therapeutic Target Database 2020: Enriched Resource for Facilitating Research and Early Development of Targeted Therapeutics. Nucleic Acids Res (2020) 48(D1):D1031–41. doi: 10.1093/nar/gkz981
45. Wishart DS, Feunang YD, Guo AC, Lo EJ, Marcu A, Grant JR, et al. DrugBank 5.0: A Major Update to the DrugBank Database for 2018. Nucleic Acids Res (2018) 46(D1):D1074–82. doi: 10.1093/nar/gkx1037
46. Smith JA, White EA, Sowa ME, Powell ML, Ottinger M, Harper JW, et al. Genome-Wide Sirna Screen Identifies SMCX, EP400, and Brd4 as E2-dependent Regulators of Human Papillomavirus Oncogene Expression. Proc Natl Acad Sci USA (2010) 107(8):3752–7. doi: 10.1073/pnas.0914818107
47. Climent J, Perez-Losada J, Quigley DA, Kim IJ, Delrosario R, Jen KY, et al. Deletion of the PER3 Gene on Chromosome 1p36 in Recurrent ER-Positive Breast Cancer. J Clin Oncol (2010) 28(23):3770–8. doi: 10.1200/JCO.2009.27.0215
48. Wang X, Yan D, Teng M, Fan J, Zhou C, Li D, et al. Reduced Expression of PER3 is Associated With Incidence and Development of Colon Cancer. Ann Surg Oncol (2012) 19(9):3081–8. doi: 10.1245/s10434-012-2279-5
49. Zhang W, He H, Zang M, Wu Q, Zhao H, Lu LL, et al. Genetic Features of Aflatoxin-Associated Hepatocellular Carcinoma. Gastroenterology (2017) 153(1):249–62. doi: 10.1053/j.gastro.2017.03.024
50. Zhou C, Zhu C, Liu Q. Toward in Silico Identification of Tumor Neoantigens in Immunotherapy. Trends Mol Med (2019) 25(11):980–92. doi: 10.1016/j.molmed.2019.08.001
51. Martin-Kleiner I, Gall Troselj K. Mannose-6-Phosphate/Insulin-Like Growth Factor 2 Receptor (M6P/IGF2R) in Carcinogenesis. Cancer Lett (2010) 289(1):11–22. doi: 10.1016/j.canlet.2009.06.036
52. Saito S, Sirahama S, Matsushima M, Suzuki M, Sagae S, Kudo R, et al. Definition of a Commonly Deleted Region in Ovarian Cancers to a 300-Kb Segment of Chromosome 6q27. Cancer Res (1996) 56(24):5586–9.
53. Tang Z, Kang B, Li C, Chen T, Zhang Z. Gepia2: An Enhanced Web Server for Large-Scale Expression Profiling and Interactive Analysis. Nucleic Acids Res (2019) 47(W1):W556–60. doi: 10.1093/nar/gkz430
54. Gubin MM, Artyomov MN, Mardis ER, Schreiber RD. Tumor Neoantigens: Building a Framework for Personalized Cancer Immunotherapy. J Clin Invest (2015) 125(9):3413–21. doi: 10.1172/JCI80008
Keywords: cervical cancer, cervical intraepithelial neoplasia, genome variation, neoantigens, immunotherapy
Citation: Bao C, An N, Xie H, Xu L, Zhou B, Luo J, Huang W and Huang J (2021) Identifying Potential Neoantigens for Cervical Cancer Immunotherapy Using Comprehensive Genomic Variation Profiling of Cervical Intraepithelial Neoplasia and Cervical Cancer. Front. Oncol. 11:672386. doi: 10.3389/fonc.2021.672386
Received: 28 February 2021; Accepted: 17 May 2021;
Published: 17 June 2021.
Edited by:
Qi Zhao, Sun Yat-sen University Cancer Center (SYSUCC), ChinaReviewed by:
Chao Chen, Beijing Genomics Institute (BGI), ChinaYueyuan Zheng, Cedars Sinai Medical Center, United States
Copyright © 2021 Bao, An, Xie, Xu, Zhou, Luo, Huang and Huang. This is an open-access article distributed under the terms of the Creative Commons Attribution License (CC BY). The use, distribution or reproduction in other forums is permitted, provided the original author(s) and the copyright owner(s) are credited and that the original publication in this journal is cited, in accordance with accepted academic practice. No use, distribution or reproduction is permitted which does not comply with these terms.
*Correspondence: Jian Huang, amlhbmh1YW5nQHNqdHUuZWR1LmNu