- 1Biomedical Informatics Research Laboratory, Department of Biology, Lahore University of Management Sciences, Lahore, Pakistan
- 2Department of Pathology, Shaukat Khanum Memorial Cancer Hospital and Research Centre, Lahore, Pakistan
- 3Epigenetics Laboratory, Department of Biology, Lahore University of Management Sciences, Lahore, Pakistan
- 4Cancer Therapeutics Laboratory, Department of Biology, Lahore University of Management Sciences, Lahore, Pakistan
In silico models of biomolecular regulation in cancer, annotated with patient-specific gene expression data, can aid in the development of novel personalized cancer therapeutic strategies. Drosophila melanogaster is a well-established animal model that is increasingly being employed to evaluate such preclinical personalized cancer therapies. Here, we report five Boolean network models of biomolecular regulation in cells lining the Drosophila midgut epithelium and annotate them with colorectal cancer patient-specific mutation data to develop an in silico Drosophila Patient Model (DPM). We employed cell-type-specific RNA-seq gene expression data from the FlyGut-seq database to annotate and then validate these networks. Next, we developed three literature-based colorectal cancer case studies to evaluate cell fate outcomes from the model. Results obtained from analyses of the proposed DPM help: (i) elucidate cell fate evolution in colorectal tumorigenesis, (ii) validate cytotoxicity of nine FDA-approved CRC drugs, and (iii) devise optimal personalized treatment combinations. The personalized network models helped identify synergistic combinations of paclitaxel-regorafenib, paclitaxel-bortezomib, docetaxel-bortezomib, and paclitaxel-imatinib for treating different colorectal cancer patients. Follow-on therapeutic screening of six colorectal cancer patients from cBioPortal using this drug combination demonstrated a 100% increase in apoptosis and a 100% decrease in proliferation. In conclusion, this work outlines a novel roadmap for decoding colorectal tumorigenesis along with the development of personalized combinatorial therapeutics for preclinical translational studies.
Introduction
Cancer development is a multistep process that is driven by a heterogeneous combination of somatic mutations at the genetic and epigenetic levels (1, 2). Specific mutations in oncogenes (3) and tumor suppressor genes (4), that result in their activation and inactivation, respectively, manifest themselves at the tissue level in the form of polyps, multi-layering, and metastasis (1, 5, 6). These system-level properties resulting from heterogeneous biomolecular aberrations and dysregulated cellular processes are abstracted as “hallmarks of cancer” (1, 6). The heterogeneity exhibited by cancer cells stems from factors such as genomic instability, clonal evolution, and variations in the microenvironment (7, 8). This fosters plasticity in cancer cells which lead to drug resistance – a leading impediment in the treatment of the disease (7–9). As a result, despite major research initiatives and resultant advancements in decoding the molecular basis of cancer, a comprehensive treatment for the disease still alludes researchers. The limited therapeutic regimens approved by the Food and Drug Administration (FDA) (10–12) exhibit variable efficacies across patients besides a multitude of toxic side effects and, multi-drug resistance (13). Towards designing efficacious personalized cancer therapeutics, recent advances in high-throughput omics-based approaches complemented by patient-specific gene expression data can provide significant assistance (14, 15). Several online databases and portals including cBioPortal (16), The Cancer Genome Atlas (TCGA) (17), and International Cancer Genome Consortium (ICGC) (18) amongst others (19, 20) provide such freely available datasets. However, effective and seamless utilization of such patient-specific genomic data to design personalized cancer therapies is still a fledgling area.
Researchers are increasingly employing whole-animal models (21–24) such a mouse, zebrafish, and fruit fly for preclinical in vivo validation of therapeutic hypotheses generated from personalized preclinical studies. Amongst the animal models, Drosophila melanogaster has become a popular platform for gene manipulation, investigating site-specific changes in the genome, and high-throughput whole-animal screening (14, 25). Importantly, a comparative study of the human and fly genome showed around 75% of disease-causing genes in humans are conserved in Drosophila (24, 26). Additionally, ease of handling and significantly lower genetic redundancy imparts further advantage to the employment of fly models (27). As a result, over 50 different data repositories, and tools are now available for hosting data on the fly genome, RNAi screens, and expression data including FlyGut-seq (28), and FlyAtlas (29) databases. Specifically in the case of cancer, several in vivo studies have been designed to elicit novel therapeutic targets using the Drosophila model system (30–33). One salient example is the validation of indomethacin, which is reported to enhance human Adenomatous Polyposis Coli (hAPC) induced phenotype in Drosophila eye (34) and therefore, employed for treating colorectal cancer (CRC). Vandetanib, another approved targeted therapy that was also validated by using the Drosophila system, suppressed Ret activity, and was later approved for medullary thyroid carcinoma (MTC) (30). However, a major shortcoming of using such mono-therapeutic agents for cancer treatment stems from the tumor heterogeneity which results in the selection of resistant cells (35, 36) besides acting specifically on singular pathways. To overcome these issues, multiple therapeutic agents acting on multiple pathways in synergy can significantly increase drug efficacy, besides lowering the therapeutic dosage (36). To evaluate high-efficacy synergistic drug combinations, researchers have employed the Drosophila model in preclinical studies to elicit optimal drug combinations (32, 33). The Drosophila Lung Cancer Model by Levine et al. (32) helped identify trametinib and fluvastatin as combinatorial drug therapy for lung cancer. Further, an EGFR induced lung tumor model was also designed in Drosophila which assisted in providing an alternative combination of drugs for lung cancer treatment through screening an FDA-approved compound library (33). However, combinatorial therapies pose unique challenges such as multidrug resistance in chemotherapy (13) and cross drug resistance (37, 38) besides the continuing need for higher therapeutic efficacies (39). Towards tackling these issues, researchers are now ‘personalizing’ live animal platforms for employment in preclinical studies to design efficacious therapeutic regimens. For instance, a comprehensive state-of-the-art in vivo Drosophila Patient Model (DPM) using a personalized therapeutics approach was described in flies (40). This particular study involved genetic manipulation of the fly genome to induce mutations specific to KRAS-mutant metastatic colorectal cancer. Combinatorial therapies were then given to the transgenic flies, harboring mutations that were identified in the patient, to discover high-efficacy synergistic drug combinations.
Here, we propose a novel computational framework in the form of an in silico Drosophila Patient Model (DPM), for developing personalized drug combinations for CRC patients. This framework is designed such that it can facilitate the modeling and analysis of patient-specified CRC network models along with evaluation of combinatorial therapeutic strategies (41, 42). We have constructed five biomolecular network models of cells regulating the maintenance of adult Drosophila midgut epithelium lining. These include multipotent intestinal stem cells (ISCs) (43–47), enteroblasts (EBs) (48), enterocytes (ECs), enteroendocrine cells (EEs) (49–53), and visceral muscle (VM) cells (54). Next, we evaluated each network’s ability to program cell fates under normal conditions as well as under minor perturbations. The ISCs are under the regulation of two sub-regions at the time of division; Apical and Basal (52). In our study, we have incorporated this information and analyzed ISC network under Apical and Basal regulation by changing inputs to the network. The networks including ISC’s under Apical and Basal regulation, EB, and EC, were then subjected to three types of inputs including physiological inputs (referred to as “normal”), aberrant inputs such that the fly homeostatic midgut regulation is perturbed (referred to as “stress”), and oncogenic inputs (referred to as “cancer”). The cell fate outcomes under normal and cancer conditions were validated against published literature. The individual output node propensities for the normal case were also validated against RNA-seq gene expression values taken from the FlyGut-seq (28) database. Additionally, three literature-based case studies were constructed to further validate the proposed in silico DPM. The first case study replicates colorectal tumorigenesis under progressive mutations using Martorell et al.’s CRC model (55). In the second case study, we employed Markstein et al.’s (56) model to perform therapeutic interventions to validate the cytotoxicity of nine FDA-approved drugs. Finally, in the third case study, we reproduced Bangi et al.’s KRAS-mutant CRC model (40) for evaluating optimal personalized drug treatment combinations by incorporating key patient-specific mutations into our model followed by combinatorial therapeutic screening. Building on these case studies, we devised a novel synergistic combination of a chemotherapeutic agent and a targeted therapy i.e., paclitaxel-regorafenib, paclitaxel-bortezomib, docetaxel-bortezomib, and paclitaxel-imatinib for treating six CRC patients taken from cBioPortal (16), while four patients were treated with only targeted therapy. The results obtained from combinatorial chemo- and targeted therapies show up to a 100% increase in anti-cancerous cell fates such as apoptosis and a 100% reduction in tumorigenesis promoting cell fates such as hyper-proliferation.
Taken together, we propose a computational framework in the form of an in silico DPM to provide personalized CRC therapeutics. This approach can help reduce the overall cancer treatment cost by facilitating the development of higher efficacy combinatorial therapies for colorectal cancer.
Results
Network Construction and Robustness Analysis of Regulatory Homeostasis in Drosophila melanogaster Midgut
To investigate the biomolecular signaling regulating the homeostasis in Drosophila melanogaster midgut (Supplementary Figure 1), we undertook an extensive literature survey and constructed five cell-type-specific rules-based network models (details in Supplementary Tables 1–5). Each model corresponds to one of the five cellular phenotypes lining the Drosophila midgut including intestinal stem cells (ISCs) (43–47), enteroblasts (EBs) (48), enterocytes (ECs), enteroendocrine cells (EEs) (49–53), and visceral muscle (VM) (54). The schematic of pathway integration in each network model is provided in Supplementary Figures 2–6. ISC network contains 48 nodes and 70 edges, EB consists of 45 nodes and 65 edges, EC and EE comprise 39 nodes and 55 edges, and VM contains 42 nodes and 57 edges (Figures 1A–D).
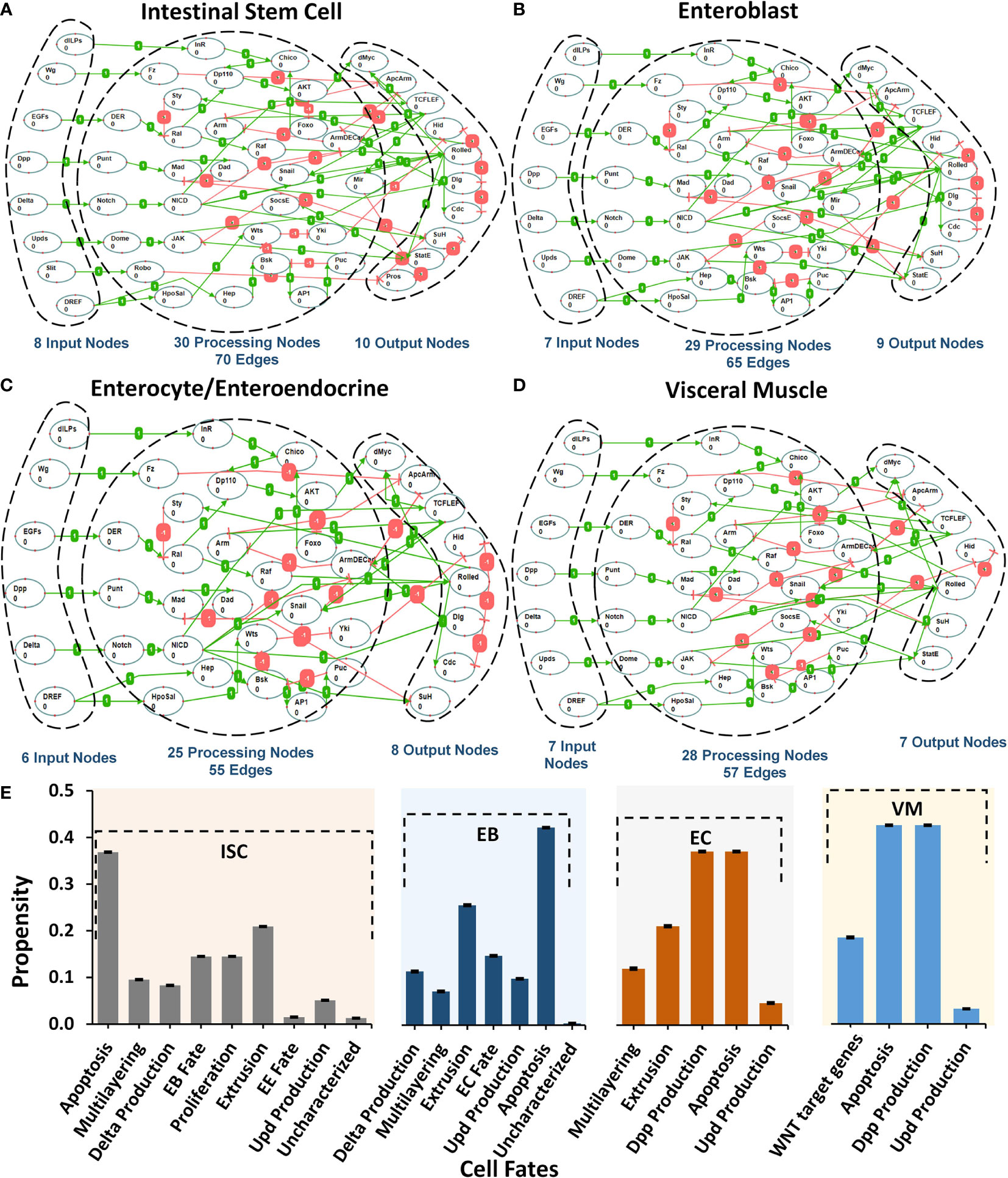
Figure 1 Regulatory schema of networks for the five cell types present in Drosophila melanogaster midgut. (A–D) The mapping between inputs, processing, and output nodes present in the biomolecular network models of five cell types i.e. ISC, EB, EC/EE, and VM. (E) Cellular fate propensities for ISC, EBs, ECs, and VM, along with their respective SEMs.
Next, to evaluate the biological plausibility of each network, we assessed the network response under normal input node values taken from the FlyGut-seq database (28) (see Materials and Methods). Our results show that the biomolecular network of ISC cells programmed apoptosis (with a propensity of 0.332), extrusion (0.188), proliferation (0.131), and differentiation/EB fate (0.131). EB network exhibited apoptosis (0.379), and extrusion (0.230). In the case of the EC network, apoptosis and dpp production were both programmed with propensities of 0.331, while for VM network apoptosis and dpp production cell fate program with 0.398 propensity (Figure 1E).
To determine the robustness of cell fate programming by each type of cell, we induced a 10% perturbation in the input stimuli and observed the network response. The highest variation in cell fates was exhibited in apoptosis (SEM 0.0006), delta production (SEM 0.0012), multilayering (0.0014), and WNT target genes (0.0009) for ISC, EB, EC, and VM, respectively (Supplementary Figure 7). The robust cell fate programming results indicate that all five networks are biologically plausible as they exhibited robustness against random perturbations and are hence feasible for employment in onward analyses (57, 58) (Supplementary Table 6).
Evaluation and Validation of Biomolecular Network Models Under Normal, Stress and Colon Cancer Conditions
To investigate and evaluate the proposed normal networks under normal, stress, and cancerous conditions (construed as a combination of inputs), Deterministic Analysis (DA) was performed (59) (Supplementary Table 7). Results from our analyses (Figure 2) revealed that under normal conditions, ISC’s Apical regulation programmed apoptosis, extrusion, proliferation, and differentiation (or EB fate) with propensities of 0.295, 0.178, 0.130, and 0.130, respectively (Supplementary Table 8) (see Materials and Methods). Under stress conditions, the propensity for proliferation, delta production, apoptosis, and differentiation increased to 0.141, 0.074 (from 0.062 in normal conditions), 0.344, and 0.141, respectively. Lastly, in cancerous conditions, propensities for multi-layering increased to 0.207, while proliferation, delta production decreased to 0.089 and 0.014, respectively. The results were again validated from the literature which supports that normal ISCs in stress conditions are known to undergo higher proliferation (60–62) and since delta is a marker for proliferation, its value increases as well (63–65). However, in the case of cancer conditions such as nutrient deprivation, etc., normal cells exhibit lowered proliferation (66, 67). Literature reports also that ISCs upon encountering extreme stress, exhibit epithelium multi-layering, augmented by overgrowth (68, 69) (Supplementary Figures 8–10).
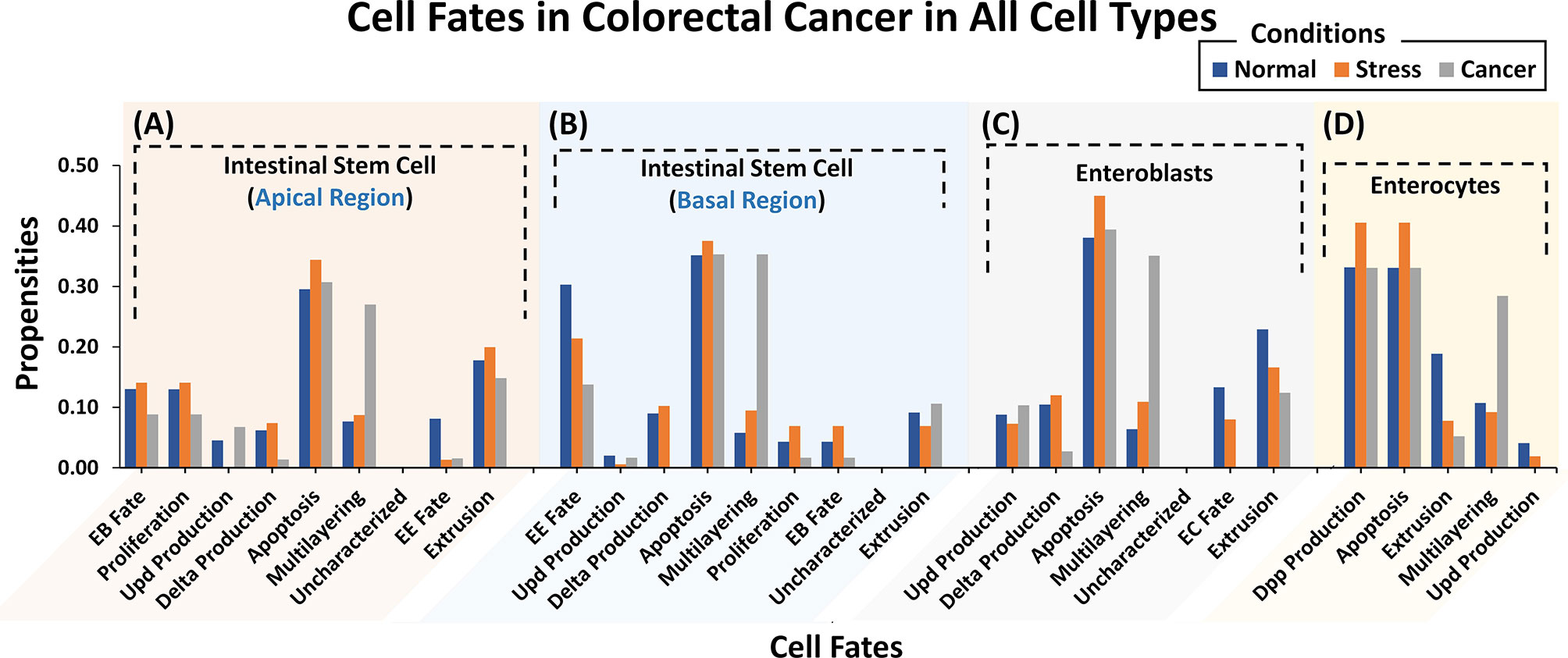
Figure 2 Cell fate propensities for intestinal stem cells (ISCs) under Apical and Basal regulation, enteroblasts (EBs), and enterocytes (ECs) in normal, stress, and cancer conditions. (A) ISC’s under Apical regulation adopt eight different cell fates in three ambient conditions. In normal conditions, the highest propensity was observed for apoptosis followed by extrusion, proliferation, and EB fate, in order. In the case of stress, the highest propensity is that of apoptosis, followed by extrusion, EB fate, and proliferation. In cancer, the highest propensity is that of apologies followed by multi-layering and extrusion. (B) ISCs under Basal regulation program eight different cell fates with the highest propensity being for apoptosis fate in normal, stress, and cancer conditions. (C) Six cellular fates in EB, with the highest propensity for apoptosis in normal, stress, and cancer conditions. (D) Five cellular fates in EC, with the highest propensity for dpp production and apoptosis in normal, stress, and cancer conditions. Uncharacterized cell fate has a 0.000 propensity in all conditions and every network.
For the ISC network under Basal regulation and in normal conditions (Supplementary Table 7), the cell fate outcomes included apoptosis, differentiation (or EE fate), and extrusion, with propensities of 0.353, 0.303, and 0.094, respectively (Supplementary Table 8). Under stress, apoptosis, proliferation, and delta production increased to 0.375, 0.069 (from 0.045 in normal conditions), and 0.102 (from 0.089 in normal conditions), respectively. For cancer conditions, the propensity of apoptosis, proliferation, and delta production decreased to 0.353, 0.017, and 0.000, respectively, whereas multi-layering increased to 0.353. Stressful cellular environments are known to increase the apoptosis rate (70–72). In absence of mutations, normal cells residing in toxic and oncogenic environments reduce their proliferation rate and delta production (63–67). Cell division rate, moreover, needs to be balanced with cell turnover and apoptosis so when proliferation slows down so does cell death (70, 71) (Supplementary Figures 11–13).
Next, we evaluated cell fate programming of the EB network under normal conditions (Supplementary Table 7). The results showed apoptosis, extrusion, and differentiation (or EC fate) cell fates with propensities of 0.381, 0.229, and 0.133, respectively (Supplementary Table 8). However, under stress conditions, the propensity for apoptosis and multi-layering increased to 0.450 and 0.109, respectively, whereas, extrusion and differentiation (or EC fate) decreased to 0.166, and 0.080, respectively. Under cancerous conditions, the salient cell fates programmed included multi-layering, apoptosis, and extrusion with propensities of 0.351, 0.394, and 0.124, respectively. Also, differentiation was suppressed to 0.000 due to toxic cellular environments. The trend in cell fate propensities under cancerous conditions also exhibited multi-layering (68, 69) along with low delta production and extrusion (Supplementary Figures 14–16). This corroborates with published literature stating that delta is a known marker for ISC and in the case of ISC proliferation, is reduced along with delta production (63–67) in cancer conditions.
Moreover, the EC network was also analyzed for response under normal conditions (Supplementary Table 7). The emergent cell fates included dpp production, apoptosis, and extrusion with propensities of 0.331, 0.331, and 0.189, respectively (Supplementary Table 8). Under stress, the extrusion rate decreased to 0.078, while dpp production and apoptosis both increased to 0.406, respectively. Dpp signaling is also known to increase under stress conditions to promote cell division (73). Under cancer conditions, however, an increase in propensities of multi-layering (0.284) was observed which is in agreement with published studies (68, 69) (Supplementary Figures 17–19).
Lastly, a comparison of output node values for ISC, EB, and EC networks under normal conditions was performed against experimental RNA-seq data from the FlyGut-seq database (28). Note that due to the paucity of regulatory dynamics in the literature on EE and VM, we could not evaluate their networks further. The output node propensities for ISC, EB, and EC were found to be comparable with values from the FlyGut-seq database (28) (Figure 3 and Supplementary Table 9). The full names of nodes in the network are mentioned in Supplementary Table 10.
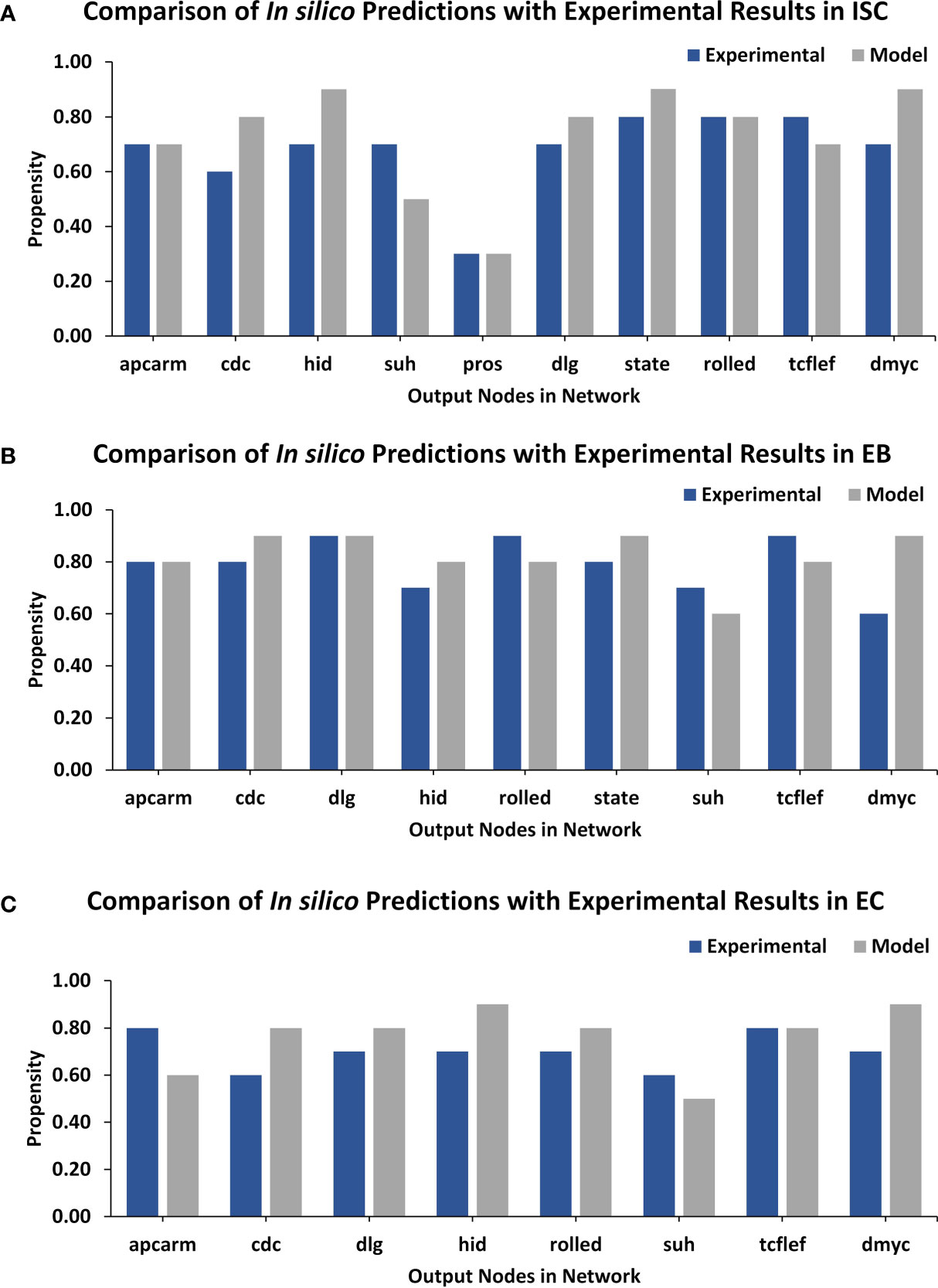
Figure 3 TISON output nodes propensities (in silico results) validation from FlyGut-seq database (in vivo results). (A) Comparison of ten output nodes propensities in ISC network: adenomatous polyposis coli (Apc2), cdc42 (Cdc42), head involution defective (hid), suppressor of hairless (Su(H)), prospero (pros), discs large 1 (dlg1), signal-transducer and activator of transcription protein at 92E (Stat92E), rolled (rl), pangolin (pan), and dMyc (myc). (B) Comparison of nine output nodes propensities in EB network: adenomatous polyposis coli (Apc2), cdc42 (Cdc42), discs large 1 (dlg1), head involution defective (hid), rolled (rl), signal-transducer, and activator of transcription protein at 92E (Stat92E), suppressor of hairless (Su(H)), pangolin (pan), and dMyc (myc). (C) Comparison of eight output nodes propensities in EC network: adenomatous polyposis coli (Apc2), cdc42 (Cdc42), discs large 1 (dlg1), head involution defective (hid), rolled (rl), suppressor of hairless (Su(H)), pangolin (pan) and dMyc (myc) (Supplementary Table 10).
Case Study 1 – Investigating Colorectal Tumorigenesis Under Progressive Mutations in Drosophila Midgut
To decode the emergent cell fates during initiation and progression of colorectal cancer (CRC) in the adult Drosophila midgut, two salient driver mutations (55) in adenomatous polyposis coli (Apc, in WNT pathway) (74) and Ras (in the EGFR pathway) (75) were incorporated into the ISC network. These mutations were initially incorporated to act individually and later simultaneously (Supplementary Figure 20). The emergent cell fates in the control case (without mutations) included apoptosis, proliferation, and differentiation, along with loss of polarity, multi-layering, and extrusion with propensities of 0.296, 0.130, 0.130, 0.00, 0.077, and 0.179, respectively. Upon incorporation of Apc mutation into the ISC network, a slight decrease in apoptosis and proliferation was observed as their propensities decreased to 0.256 and 0.112, respectively. Differentiation and extrusion also got reduced to 0.112 and 0.151, respectively, while multi-layering increased to 0.256, and loss of polarity remained unaffected. Next, upon introducing Ras mutation, a decrease in apoptosis (0.210) and an increase in proliferation (0.148) was observed, which indicated cellular overgrowth. Furthermore, in line with Martorell et al. (55), loss of polarity and extrusion increased to 0.080 and 0.210, respectively.
On the other hand, the concurrent incorporation of Apc and Ras mutations resulted in hyper-proliferation and overgrowth as apoptosis decreased to 0.173 and proliferation increased to 0.173. The differentiation rate was observed to be 0.112 and loss of polarity, multi-layering and extrusion increased to 0.061, 0.173, and 0.173, respectively. Hence, with concurrent mutations in Apc and Ras, the emergent cell fates started exhibiting the hallmarks of cancer including abnormal proliferation and loss of differentiation, etc. (76). These results were also coherent with both the experimental findings reported by Martorell et al. (55) (Figure 4 and Supplementary Table 11) and differential gene expression data (Supplementary Table 12).
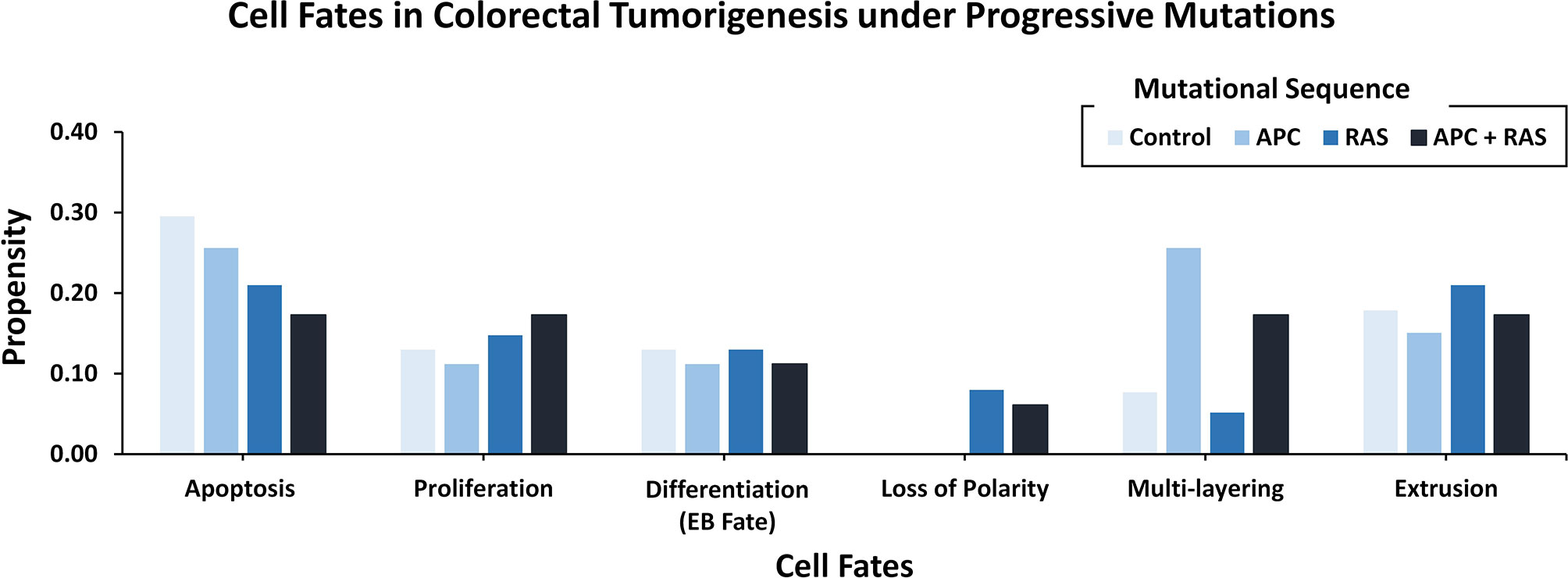
Figure 4 Cell fate outcomes after the introduction of progressive CRC mutations and their validation against Martorell et al.‘s Drosophila CRC model. A high rate of extrusion and loss of polarity was observed in Apc-Ras as well as Ras clones. Alongside, an increased proliferation rate with a decreased apoptosis and differentiation is also highlighted by Martorell et al. in their in vivo model.
Case Study 2 – Therapeutic Evaluation of Raf-Mutation in Drosophila Midgut Using Targets From the Literature
Introduction of gain-of-function Raf-specific driver mutations in our ISC network enabled the replication of Markstein et al.’s (56) therapeutic screen towards a comparative cancer recurrence evaluation of nine FDA-approved drugs. In their gain-of-function Raf tumor model, Markstein and colleagues had classified FDA-approved drugs into class I and II drugs. According to the study class, I drugs induced cancer reversal in mutated cells without affecting the wild-type cells, in contrast, class II drugs induced cancerous phenotype in wild-type cells (Supplementary Table 13). The result of our network analysis of the control case exhibited proliferation and apoptosis with propensities of 0.157 and 0.286, respectively. However, after the induction of Raf mutations, the proliferation (0.162) rate increased along with a decrease in apoptosis (0.175). Treatment of a Raf-mutated network using class I drugs led to a decrease in proliferation (0.089) and an increase in apoptosis (0.263). For the wild type in comparison with the control, almost no effect was observed on apoptosis, which remained steady at 0.283 whereas a slight decrease was observed in proliferation (0.130). This confirmed the action of class I drugs which act to substantially reduce cancerous fates in cancer without having a major impact on wild-type cells.
Alternatively, in the case of class II drugs, the wild type also exhibited hyper-proliferation after therapy with its propensity reaching up to 0.191, and apoptosis increased to 0.336. Importantly, for the mutated network, drug action continued its activities with the propensity of proliferation reaching 0.175 and apoptosis at 0.306. These results suggest that class II drugs are indeed associated with drug cytotoxicity as they induced malignancy in normal cells under therapy. This confirms Markstein et al.’s study which hypothesized that the extracellular environment in animal models is crucial in drug delivery and cytotoxicity (Figure 5 and Supplementary Table 14).
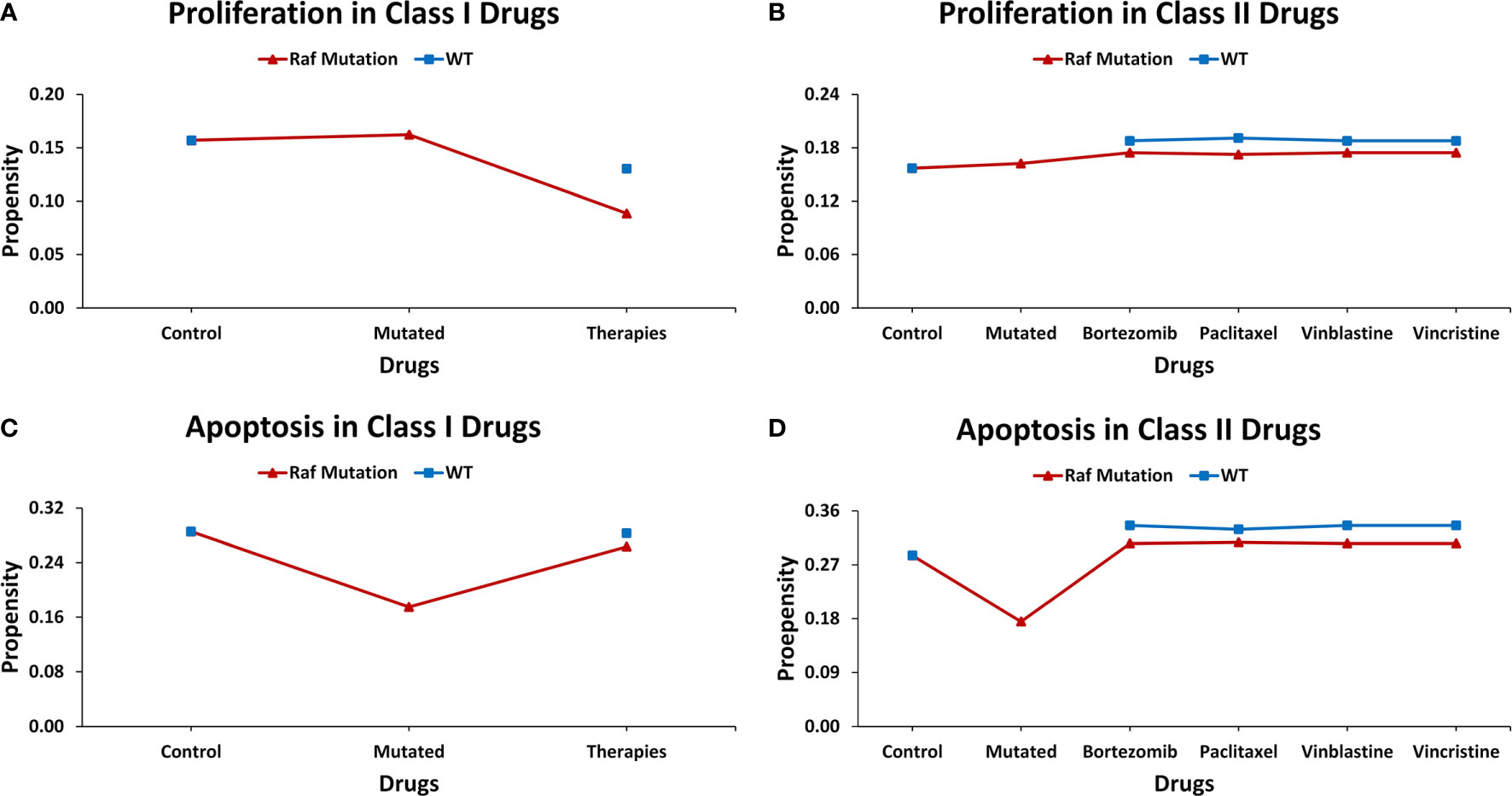
Figure 5 Evaluating cell fates under therapeutic screens taken from Markstein et al.‘s Drosophila model. (A) The effect of class I drugs on cell proliferation in wild type and mutated networks, (B) The effect of class II drugs on cell proliferation in wild type and mutated networks, (C) The effect of class I drugs on apoptosis in wild type and mutated networks, (D) The effect of class II drugs on apoptosis in wild type and mutated network.
Case Study 3 – Employing the In Silico Drosophila Patient Model (DPM) for Personalized Therapeutics
Towards developing a Drosophila-based platform for employment in orchestrating patient-centric cancer therapeutics, we adopted Bangi et al.’s (40) in vivo Drosophila Patient Model (DPM). The in vivo model was first translated into an in silico DPM which incorporated patient-specific mutations from Bangi et al.’s study. These mutations included eight tumor suppressors: Apc, Tp53, Fbxw7, Tgfbr2, Smarca4, Fat4, Mapk14, and Cdh1, along with one oncogenic mutation in Kras (Supplementary Table 15). After inducing these patient-specific mutations into the ISC network (through direct and indirect target identification), we administered trametinib and zoledronate in different combinations to observe the most efficacious therapeutic effect. Our results showed that in control (i.e. healthy cells), the cell fate propensities for proliferation and apoptosis came out to be 0.130 and 0.294, respectively. Upon induction of mutations, proliferation increased to 0.200 and apoptosis decreased to 0.200, respectively. Next, with the administration of trametinib, an inhibitor of MEK kinase (mitogen-activated protein kinase kinase), used to treat patients with Kras mutation, the propensities for proliferation decreased to 0.000, whereas apoptosis increased to 0.386 (Figure 6A). With the administration of zoledronate, the cell fate propensities came out to be 0.130 for proliferation and 0.324 in the case of apoptosis (Figure 6B). Next, with the induction of zoledronate in combination with trametinib, a decrease in proliferation to 0.000 and an increase in apoptosis to 0.386 was observed (Figure 6C). Interestingly, augmentation of therapy with in tandem administration of trametinib, zoledronate, and trametinib with zoledronate showed proliferation to decrease to 0.000 and apoptosis to increase to 0.400 propensities (Figure 6D). These results exhibited cancer reversion on the administration of the drug combination and corroborate with Bangi et al.’s findings.
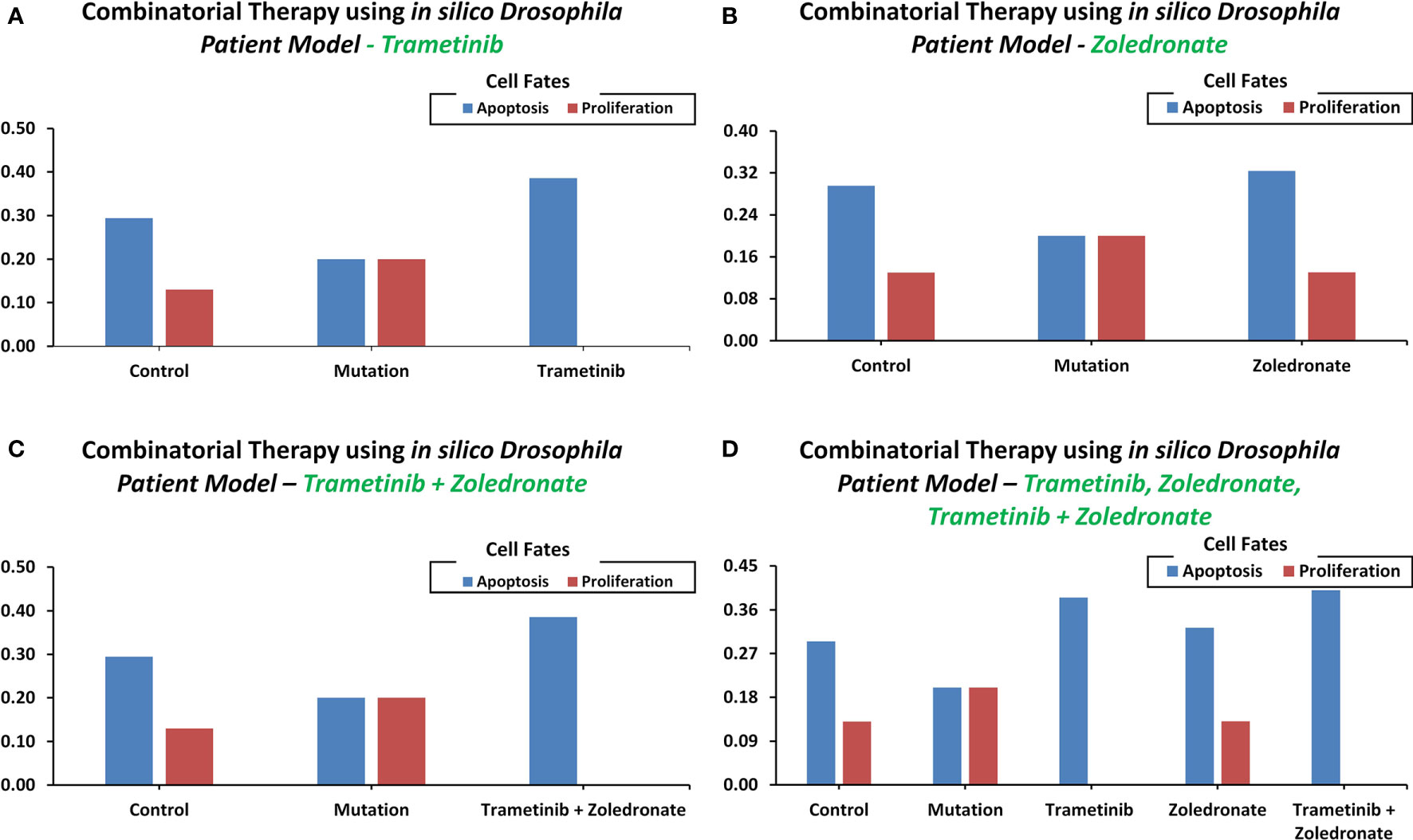
Figure 6 Cell fate propensities were obtained from the in vivo Drosophila Patient Model using Bangi et al.'s study. Cell fate propensities under (A) control, mutated, and therapy (Trametinib), (B) control, mutated, and therapy (Zoledronate), (C) control, mutated, and therapy (Trametinib + Zoledronate), (D) control, mutated, and therapy (Trametinib, Zoledronate, and Trametinib + Zoledronate).
Identification and Evaluation of Personalized Therapeutics for CRC Patients Using In Silico DPM
Towards developing personalized combinatorial therapies for treating colorectal cancer patients, we coupled our in silico DPM with patient-specific gene expression data from cBioPortal (16) (Supplementary Table 16). Patient-specific potential druggable targets were identified (from the 48 nodes in the ISC network) and their oncogenic cell fate (“apoptosis” and “proliferation” rates) propensities were obtained using the DA pipeline (Supplementary Table 17). Next, we employed PanDrugs (77) - an online database that prioritizes direct and indirect targeting of genomic mutations, to search for “druggable genes” in our networks. Each node was then queried in the database to find out the drugs that targeted them directly or indirectly (Supplementary Tables 18, 19). The results from this exercise elicited chemotherapy (paclitaxel/docetaxel) and targeted therapies (regorafenib, bortezomib, imatinib) depending on patient-specific mutations (Supplementary Table 20). Follow up literature review showed that these drugs and their combinations are currently being used in several studies and clinical trials (78–86). Specifically, the combination of the paclitaxel-regorafenib was evaluated for treating advanced esophagogastric cancer (78), and the paclitaxel-bortezomib combination was used in metastatic solid tumors (87). While the docetaxel-bortezomib combination was evaluated for metastatic breast cancer (79), Non-Small Cell Lung Cancer (NSCLC) (80, 81), and prostate cancer (82). Paclitaxel-imatinib combination was tested in metastatic solid tumors (83), NSCLC (84), and ovarian cancer (85).
To test the efficacy of these drug combinations in CRC patients, we administered these therapies using the proposed in silico DPMs to ten patients with colorectal adenocarcinoma obtained from cBioPortal (16). To implement the simultaneous action of chemotherapy wherein the drug introduces widespread inhibition of mitosis by stabilizing polymerized microtubules and not allowing them to function during cell division for that, we surveyed the existing literature on microtubule targeting (Supplementary Table 21, Supplementary Figure 21) and embedded it into ISC network (Supplementary Table 22) to study the behavior of microtubule stabilization-induced cell fates in chemotherapy. The resultant network consists of 54 nodes and 83 edges (Supplementary Figure 22). Our results from combinatorial chemo- and targeted therapy using an extended network showed up to a 100% increase in apoptosis cell fate and a 100% decrease in proliferation rate (Figure 7 and Supplementary Table 23).
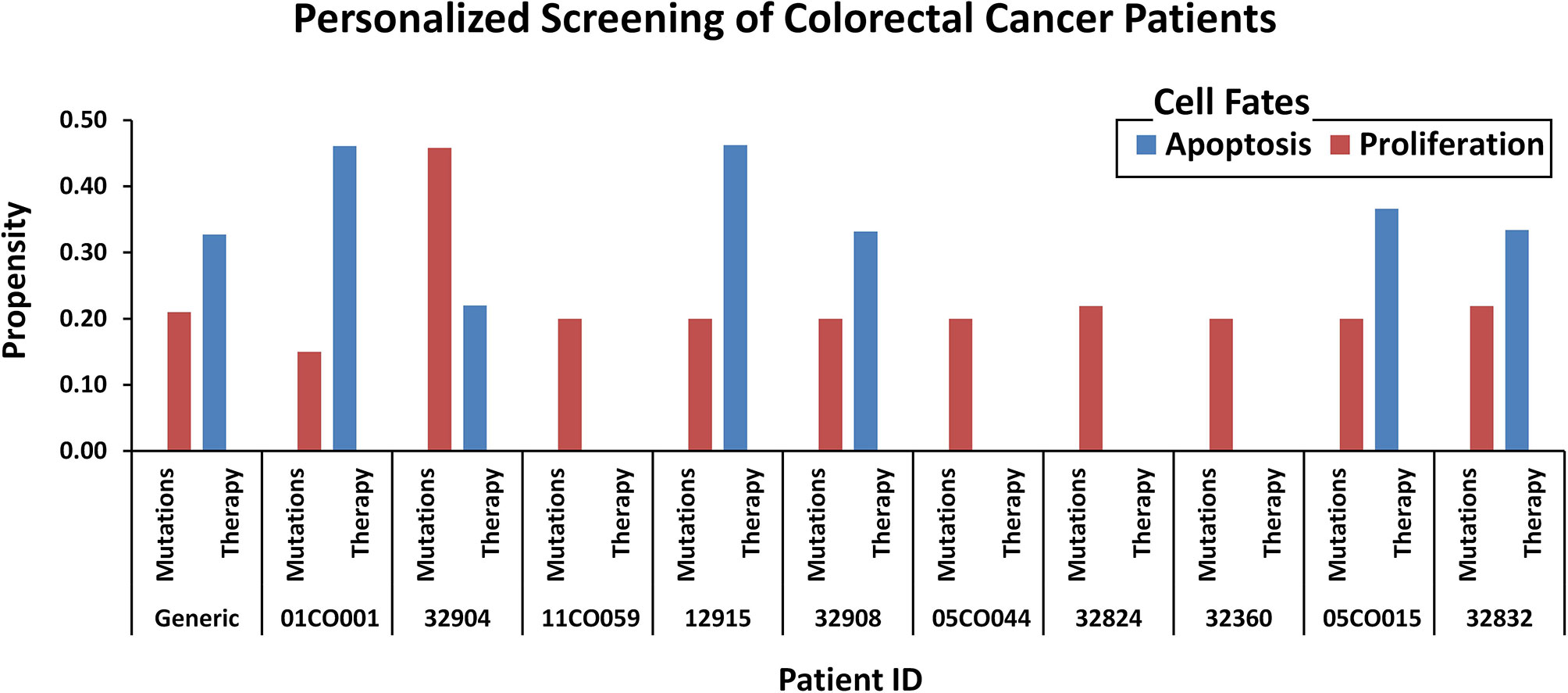
Figure 7 Comparison of oncogenic cell fate propensities obtained from personalized screening. Personalized screening of ten colorectal cancer patients. Patient ID and mutation data were extracted from cBioPortal and cell fates for apoptosis and proliferation were plotted to observe before and after therapy results.
Materials and Methods
The following sub-sections provide details of the methodology employed at each step of the study. The overall workflow of the study is outlined in Supplementary Figure 23.
Data Collection and Boolean Modeling of Five Cell-Type-Specific Networks in Drosophila Midgut
To construct the biomolecular network models involved in the cellular regulation of Drosophila midgut, a comprehensive review of the existing literature and databases was undertaken. The databases employed included the Kyoto Encyclopedia of Genes and Genomes (KEGG) (88), Drosophila Interactions Database (DroID) (89), and data repositories such as FlyGut-seq (28). Alongside, network models of Drosophila by Giot et al. (90), Formstecher et al. (91), and Toku et al. were used to construct five rule-based Boolean biomolecular networks of the conserved signaling pathways in intestinal stem cells (ISCs) (43–47), enteroblasts (EBs) (48), enterocytes (ECs), enteroendocrine cells (EEs) (49–53), and visceral muscle (VM) cells (54). Nine major pathways involved in maintaining the overall homeostatic nature of the fly midgut were selected from the available literature. These included Notch (92), BMP (92), EGFR (93), WNT (94), JAK-STAT (94, 95), JNK (96), HIPPO (97), Insulin (63), and/or Robo (98) pathways for each cell type lining the midgut. The network steady states were used to program cell fate outcomes such as cellular differentiation, proliferation, apoptosis, EC fate determination, etc. Boolean equations (59) were used to model the regulation of each node in the biomolecular network. TISON (99), an in-house theatre for in silico systems oncology was used to translate Boolean rules into network models (see Supplementary Data, Supplementary Table 24 for video tutorial).
Robustness Analysis
To validate the biological plausibility of the proposed networks, a robustness analysis was performed (see Supplementary Table 24 for video tutorial). Physiological conditions were maintained during this process and the input node values were taken from the FlyGut-seq database (28). The normal node states for ISC, EB, EC, and VM were perturbed by ±10%. Bootstrapping was employed on 10,000 network states. The means and standard deviations of the emergent cell fates were then calculated and the standard error of means (SEM) was plotted for each cell fate to determine the biological plausibility of the scale-free networks (100) (see Supplementary Data 1).
Deterministic Analysis
The Boolean networks have been analyzed using the Deterministic Analysis (DA) (59) pipeline reported in ATLANTIS (101) and TISON (99) (see Supplementary Table 24 for video tutorial). The results from DA were used to program “cell fate attractors” which are biological states that a cell can take, along with computation of their propensities (probability of their occurrence). Three different input files are used in this process which includes (i) network file, (ii) fixed node states file, and (iii) cell fate classification file. The network file contains the Boolean rules defining the biomolecular networks. The fixed node states file contained fixed values for generating environmental conditions such as normal, stress, or cancer conditions. The cell fate classification file is used to map network states onto the biological cell fates in the light of particular cell fate markers (101) (Supplementary Table 25). For network analysis, the DA pipeline starts with a set of initial network states. To achieve a steady state, logical rules, and state transition functions are employed. Upon reaching a steady-state a cell fate attractor is formed. This attractor can represent a specific cell fate with a cell fate propensity or basin size ratio. Bootstrapping was employed on 10,000 network states. TISON’s Therapeutics Editor (TE) was used to undertake therapeutic evaluation on the network using the DA pipeline, with mutation and drug data integrated (see Supplementary Table 24 for video tutorial). Fixed node states for normal conditions were obtained from the FlyGut-seq database while for cancer conditions, literature was surveyed to find out if the pathway is up or downregulated. For stress, abnormal values were abstracted by perturbing the stimuli from normal conditions (see Supplementary Data 2).
Network Annotation Using Flygut-seq Database
Towards annotating networks with experimental values, the FlyGut-seq database was employed. For that, an RNA-seq dataset consisting of rpkm values was exported from the database. Data were extracted for the relevant genes present in our networks (ISC, EB, and EC) using their biological names (Supplementary Table 10). Expression data across the five regions of the midgut (i.e. R1, R2, R3, R4, and R5) (102) was normalized for each gene in specific cells. The normalized values were taken as normal input fixed node states for onward analyses. The normalized values were also used to compare the output node propensities from DA that was performed under normal input conditions (Supplementary Table 9, for details, see Supplementary Data 2).
Cell Fate Data Collection for Case Studies and Their Validation
To validate and exemplify our network models, we used three literature-based case studies on colorectal tumorigenesis in Drosophila melanogaster. For case study 1, data including cell fates under Apc and Ras single and simultaneous mutations were obtained from Martorell et al.’s model (55). The differential gene expression screens and data were also obtained from Martorell et al. (see Supplementary Data 3). TISON’s TE was used to implement the mutations in our network using TE’s horizontal therapy pipeline. For case study 2, therapeutic screens including the existing list of FDA-approved drugs for targeting ISC in Drosophila were adapted from Markstein et al.’s (56) study. Existing databases on drugs and drug-gene interactions such as PharmacoDB (103), PanDrugs (77), OncoKB (104), and DGIdb (105), etc (106, 107) were then used to identify target nodes in our ISC network, which were also mentioned in Markstein et al.’s study. TE was employed to deliver drug data into the CRC mutated network using TE’s vertical therapy pipeline (see Supplementary Data 4). For case study 3, patient-specific mutations, along with combinatorial therapy drug candidates were taken from Bangi et al.’s (40) study. Drug databases were used to identify target nodes in the ISC network mentioned in Bangi et al.’s study. Drugs that did not have direct targets in the network were implemented indirectly using literature-based mechanisms (see Supplementary Data 5).
Development of an In Silico Drosophila Patient Model (DPM) and Its Validation
Towards devising a novel drug combination for the treatment of colorectal tumorigenesis, we performed an exhaustive evaluation of each node in our ISC network using TISON’s TE. For that, we started with the sensitivity analysis of both tumor suppressor genes and oncogenes involved in CRC using data from existing databases and literature (55, 103, 106, 107) against patient-specific mutations taken from cBioPortal (16). The therapeutic screening was performed by upregulating the tumor suppressors and downregulating the oncogenes (Supplementary Table 26), to evaluate potential drug combination targets using the PanDrugs (77) database, a platform that prioritizes direct and indirect targeting of genomic mutations (see Supplementary Data 6).
Combination of Chemotherapy and Targeted Therapy to Treat CRC Patients
To induce the effect of chemotherapy we carried an extensive survey of the existing literature and constructed a microtubule network. The microtubule network was incorporated by integrating 6 nodes and 13 interactions to the ISC network. The resultant network contained 54 nodes and 83 interactions. This integrated network was then utilized for chemotherapeutic screening. The combinatorial personalized therapy was used to treat the CRC patients, in a vertical therapy scheme through targeting specific nodes in our ISC network in light of patient-specific mutations. DA pipeline was used to carry out the therapeutic evaluation (see Supplementary Data 6).
Discussion
Combinatorial therapies have created avenues for enhanced treatment of colorectal cancer (CRC) through drug synergy (108). Translational studies using omics-based data can help develop efficacious drug combinations for individualized CRC treatment. In particular, in silico Boolean models that utilize omics datasets can facilitate the process of developing and evaluating different drug combination therapies for the treatment of CRC (109–111). In this work, we propose a novel in silico Drosophila Patient Model (DPM), a computational framework for devising personalized therapeutic combinations for CRC patients. For that, we have constructed Boolean network models of five cell types present in Drosophila midgut: (i) intestinal stem cell, (ii) enteroblast, (iii) enterocyte, (iv) enteroendocrine, and (iv) visceral muscles (Figures 1A–D). We have used these networks to systematically induct tumorigenesis in Drosophila midgut tissue followed by therapeutic interventions for tumor reversion and restoration of physiological homeostasis (Figure 2). We then employed the ISC networks to create an in silico DPM for identifying optimal combinatorial therapeutics to treat CRC in humans. Our modeling pipeline provides a novel roadmap to annotate Boolean network models with patient data towards developing personalized medicine for CRC patients.
Several network models of biomolecular regulation in Drosophila have been reported for investigating the regulatory dynamics in cancer (90, 91, 112–114). Specifically, such applications of adult Drosophila midgut models are particularly useful in investigating CRC due to cellular and organizational similarities between Drosophila midgut and the human colon. More so, the biomolecular signaling pathways involved in maintaining homeostasis and differentiation are also conserved in both. This has given impetus to the development and utilization of Drosophila midgut models for investigating human colorectal cancer (115, 116). As a result, fly-based midgut models have been employed to investigate tissue homeostasis (117), multi-step tumorigenesis (55), epithelium renewal and regeneration upon bacterial infection or tissue damage (118), and its effect on mature and undifferentiated epithelial cells during intestinal cancer initiation (119). However, the employment of Drosophila midgut networks has hitherto remained unannotated with patient-specific mutation to study tumorigenesis in CRC thus limiting their translational potential. In this study, we have employed three literature-based case studies on in vivo Drosophila model to investigate CRC, employing in silico approaches. In our first case study, we used a fly-based network model to help investigate colorectal tumorigenesis under progressive mutations; the results from our analysis were validated against Martorell’s CRC model (55) (Figure 4). The results from our second case study helped elucidate cytotoxicity in nine FDA-approved drugs (Figure 5) and conformed with Markstein et al.’s (56) hypothesis that the extracellular environment plays a crucial role in animal models for evaluating drug delivery and cytotoxicity. Next, for the third case study, we used Bangi et al.’s in vivo DPM to perform personalized therapy for KRAS-mutant metastatic colorectal cancer patient (40) (Figure 6), which re-confirmed the potential of combinatorial treatment; trametinib, zoledronate followed by trametinib in combination with zoledronate.
Onwards, we have performed personalized therapeutics by incorporating patient-specific mutation data into our model towards devising novel combinatorial treatments. For that, we took patient-specific data on ten patients with colorectal adenocarcinoma obtained from cBioPortal (16) to annotate our network model (Supplementary Table 16). We then undertook an exhaustive screening towards identifying efficacious target nodes for each patient which was based on the node’s pro-apoptotic and anti-proliferation cell fate propensities after therapy (Supplementary Table 17). We used the PanDrugs database (77) to identify these target nodes in existing drugs. In light of our personalized screening step, we discovered that four patients can respond well to targeted therapy (imatinib, regorafenib, and everolimus), whereas for the rest a synergistic combination of chemotherapy (paclitaxel/docetaxel) and targeted therapy (imatinib, regorafenib, and bortezomib) was a more efficacious treatment (Supplementary Table 20). Literature also supports our finding that CRC treatment using a combination of chemo- and targeted therapy can provide efficacious results compared to conventional chemotherapy alone (119, 120). Specifically, the combination of the paclitaxel-regorafenib was evaluated for treating advanced esophagogastric cancer (78), and the paclitaxel-bortezomib combination was used in metastatic solid tumors (87). While the docetaxel-bortezomib combination was evaluated for metastatic breast cancer (79), Non-Small Cell Lung Cancer (NSCLC) (80, 81), and prostate cancer (82). Paclitaxel-imatinib combination was tested in metastatic solid tumor (83), NSCLC (84), and ovarian cancer (85, 86). However, further validation of these prognostic drug combinations in large-scale clinical cohorts will be required to test these drug combinations suggested by our study. In unison, our findings suggest that the proposed translational approach is effective in optimizing existing therapies.
Limitations of this study include utilizing abstracted in silico Boolean models (59) which are only qualitative. Moreover, analysis of EE and VM networks remained limited due to a lack of substantive literature. In this work, to overcome exponential computational complexity due to network size, we pruned each network to a minimum while maintaining biological cell fate outcomes. Additionally, ISC-EB-EC interplay is pivotal in determining cell fates, especially for intestinal stem cells in Drosophila midgut, however, due to network-level analysis strategy employed in the study, we are currently unable to investigate cellular interplays as well as continuous lineage tracking for various cell types. Since our networks are independent of each other we can only elucidate individual cell fates programmed by each network at a time.
Several assumptions have been made for constructing this model. Firstly, since Drosophila midgut comprises of several regions with differential niches and context specific cellular processes (42), for the sake of computational scalability, we have not incorporated Drosophila midgut compartmentalization in our model. In view of the exponential relation between computational complexity and network size, we have kept the network size to a minimum by reducing path lengths between critical nodes through removing intermediary nodes. Integrated multi-omics information e.g., from genomics, transcriptomics, and proteomics level was assumed to act on the same time-scale, towards undertaking network analysis.
With regards to drugs, we search the nodes (genes) in our network in PanDrugs database for selecting and prioritizing potential drugs that can efficaciously target the selected nodes. The assumptions made by PanDrugs for declaring a gene-drug relationship, include: for targeted therapies, the genes-drug relationship that PanDrugs provides is a direct relationship, and that the targeted drugs acting directly on the nodes in the network are without any off target pleotropic effects. PanDrugs’s drug prioritization scheme can improve if it also takes into account protein interaction networks, pathway activity, multi-omics information, however, its search is limited to genome-level information only. Moreover, each drug is able to act on all the possible transcriptomic isoforms of a gene, where necessary.
Additionally, during the personalized screening of patients, non-druggable nodes could not be evaluated further due to unsubstantive literature on their employment as drugs. Moreover, some of the genes present in the human genome do not have exact homologs in Drosophila’s genes list, which can limit the study’s translational capabilities.
Onwards, the proposed in silico DPM can be extended to perform probabilistic analysis by converting rules to the weights-based network which can also cater to external perturbations and noise into the system. Further investigations need to be carried out to predict novel druggable genes (direct targets, biomarkers, and pathway members not available in PanDrugs database) for employment in developing new drug combinations. The network models developed can also be extended to multi-scale models towards incorporating spatiotemporal regulations of colorectal cancer. Further verifications with a greater patient sample size can help achieve a better understanding of the relationship between patient-specific data in connection to therapeutic combinations. Moreover, result verification can be enhanced with wet lab validation of the proposed synergistic drug combinations outlined by our computational framework.
Taken together, our preclinical in silico DPM not only captures the regulatory homeostasis of fly midgut but also presents a novel framework to personalize Boolean network models towards their employment in personalized cancer therapeutic interventions.
Data Availability Statement
The original contributions presented in the study are included in the article/Supplementary Material. Further inquiries can be directed to the corresponding author.
Author Contributions
SC designed and supervised the study. MG carried out the literature review, construction of the model, and undertook the analyses. SC, MG, and RN designed the personalized treatment pipeline, SC, MG, RN, ZN, and HK drafted the manuscript. OS helped construct Boolean networks. RH critically reviewed the model development and performed validations, RQ, MT, and AF assisted in the study design and manuscript development. All authors contributed to the article and approved the submitted version.
Funding
This work was supported by the National ICT-R&D Fund (SRG-209), RF-NCBC-015, NGIRI-2020-4771, HEC (21-30SRGP/R&D/HEC/2014, 20-2269/NRPU/R&D/HEC/12/4792 and 20-3629/NRPU/R&D/HEC/14/585), TWAS (RG 14-319 RG/ITC/AS_C) and LUMS (STG-BIO-1008, FIF-BIO-2052, FIF-BIO-0255, SRP-185-BIO, SRP-058-BIO and FIF-477-1819-BIO) grants.
Conflict of Interest
The authors declare that the research was conducted in the absence of any commercial or financial relationships that could be construed as a potential conflict of interest.
Supplementary Material
The Supplementary Material for this article can be found online at: https://www.frontiersin.org/articles/10.3389/fonc.2021.692592/full#supplementary-material
References
1. Hanahan D, Weinberg RA. Hallmarks of Cancer: The Next Generation. Cell (2011) 144(5):646–74. doi: 10.1016/j.cell.2011.02.013
2. Baylin SB, Jones PA. A Decade of Exploring the Cancer Epigenome — Biological and Translational Implications. Nat Rev Cancer (2011) 11(10):726–34. doi: 10.1038/nrc3130
3. Bild AH, Yao G, Chang JT, Wang Q, Potti A, Chasse D, et al. Oncogenic Pathway Signatures in Human Cancers as a Guide to Targeted Therapies. Nature (2006) 439(7074):353–7. doi: 10.1038/nature04296
4. Harris CC, Hollstein M. Clinical Implications of the p53 Tumor-Suppressor Gene. N Engl J Med (1993) 329(18):1318–27. doi: 10.1056/NEJM199310283291807
5. Haggitt RC, Glotzbach RE, Soffer EE, Wruble LD. Prognostic Factors in Colorectal Carcinomas Arising in Adenomas: Implications for Lesions Removed by Endoscopic Polypectomy. Gastroenterology (1985) 89(2):328–36. doi: 10.1016/0016-5085(85)90333-6
6. Hanahan D, Weinberg RA. The Hallmarks of Cancer. Cell (2000) 100(1):57–70. doi: 10.1016/s0092-8674(00)81683-9
7. Meacham CE, Morrison SJ. Tumour Heterogeneity and Cancer Cell Plasticity. Nature (2013) 501(7467):328–37. doi: 10.1038/nature12624
8. Dagogo-Jack I, Shaw AT. Tumour Heterogeneity and Resistance to Cancer Therapies. Nat Rev Clin Oncol (2018) 15(2):81–94. doi: 10.1038/nrclinonc.2017.166
9. Szakács G, Paterson JK, Ludwig JA, Booth-Genthe C, Gottesman MM. Targeting Multidrug Resistance in Cancer. Nat Rev Drug Discov (2006) 5(3):219–34. doi: 10.1038/nrd1984
10. Ocana A, Pandiella A, Siu LL, Tannock IF. Preclinical Development of Molecular-Targeted Agents for Cancer. Nat Rev Clin Oncol (2011) 8(4):200–9. doi: 10.1038/nrclinonc.2010.194
11. DiMasi JA, Reichert JM, Feldman L, Malins A. Clinical Approval Success Rates for Investigational Cancer Drugs. Clin Pharmacol Ther (2013) 94(3):329–35. doi: 10.1038/clpt.2013.117
12. Zamboni WC, Torchilin V, Patri AK, Hrkach J, Stern S, Lee R, et al. Best Practices in Cancer Nanotechnology: Perspective From NCI Nanotechnology Alliance. Clin Cancer Res (2012) 18(12):3229–41. doi: 10.1158/1078-0432.CCR-11-2938
13. Gottesman MM, Fojo T, Bates SE. Multidrug Resistance in Cancer: Role of ATP–dependent Transporters. Nat Rev Cancer (2002) 2(1):48–58. doi: 10.1038/nrc706
14. Kasai Y, Cagan R. Drosophila as a Tool for Personalized Medicine: A Primer. Per Med (2010) 7(6):621–32. doi: 10.2217/pme.10.65
15. Grandori C, Kemp CJ. Personalized Cancer Models for Target Discovery and Precision Medicine. Trends Cancer (2018) 4(9):634–42. doi: 10.1016/j.trecan.2018.07.005
16. Gao J, Aksoy BA, Dogrusoz U, Dresdner G, Gross B, Sumer SO, et al. Integrative Analysis of Complex Cancer Genomics and Clinical Profiles Using the Cbioportal. Sci Signal (2013) 6(269):pl1. doi: 10.1126/scisignal.2004088
17. Lee HJ, Palm J, Grimes SM, Ji HP. The Cancer Genome Atlas Clinical Explorer: A Web and Mobile Interface for Identifying Clinical-Genomic Driver Associations. Genome Med (2015) 7(1):1–14. doi: 10.1186/s13073-015-0226-3
18. Zhang J, Baran J, Cros A, Guberman JM, Haider S, Hsu J, et al. International Cancer Genome Consortium Data Portal—A One-Stop Shop for Cancer Genomics Data. Database (2011) 2011:bar026. doi: 10.1093/database/bar026
19. Papatheodorou I, Fonseca NA, Keays M, Tang YA, Barrera E, Bazant W, et al. Expression Atlas: Gene and Protein Expression Across Multiple Studies and Organisms. Nucleic Acids Res (2018) 46(D1):D246–51. doi: 10.1093/nar/gkx1158
20. Benson DA, Cavanaugh M, Clark K, Karsch-Mizrachi I, Lipman DJ, Ostell J, et al. Genbank. Nucleic Acids Res (2013) 41(D1):D36–42. doi: 10.1093/nar/gks1195
21. Morgan MM, Johnson BP, Livingston MK, Schuler LA, Alarid ET, Sung KE, et al. Personalized In Vitro Cancer Models to Predict Therapeutic Response: Challenges and a Framework for Improvement. Pharmacol Ther (2016) 165:79–92. doi: 10.1016/j.pharmthera.2016.05.007
22. Lieschke GJ, Currie PD. Animal Models of Human Disease: Zebrafish Swim Into View. Nat Rev Genet (2007) 8(5):353–67. doi: 10.1038/nrg2091
23. Olive KP, Tuveson DA. The Use of Targeted Mouse Models for Preclinical Testing of Novel Cancer Therapeutics. Clin Cancer Res (2006) 12(18):5277–87. doi: 10.1158/1078-0432.CCR-06-0436
24. Pandey UB, Nichols CD. Human Disease Models in Drosophila Melanogaster and the Role of the Fly in Therapeutic Drug Discovery. Pharmacol Rev (2011) 63(2):411–36. doi: 10.1124/pr.110.003293
25. Adams MD, Celniker SE, Holt RA, Evans CA, Gocayne JD, Amanatides PG, et al. The Genome Sequence of Drosophila Melanogaster. Science (2000) 287(5461):2185–95. doi: 10.1126/science.287.5461.2185
26. Reiter LT. A Systematic Analysis of Human Disease-Associated Gene Sequences in Drosophila Melanogaster. Genome Res (2001) 11(6):1114–25. doi: 10.1101/gr.169101
27. Mirzoyan Z, Sollazzo M, Allocca M, Valenza AM, Grifoni D, Bellosta P. Drosophila Melanogaster: A Model Organism to Study Cancer. Front Genet (2019) 10:51. doi: 10.3389/fgene.2019.00051
28. Dutta D, Dobson AJ, Houtz PL, Gläßer C, Revah J, Korzelius J, et al. Regional Cell-Specific Transcriptome Mapping Reveals Regulatory Complexity in the Adult Drosophila Midgut. Cell Rep (2015) 12(2):346–58. doi: 10.1016/j.celrep.2015.06.009
29. Chintapalli VR, Wang J, Dow JAT. Using FlyAtlas to Identify Better Drosophila Melanogaster Models of Human Disease. Nat Genet (2007) 39(6):715–20. doi: 10.1038/ng2049
30. Vidal M, Wells S, Ryan A, Cagan R. ZD6474 Suppresses Oncogenic RET Isoforms in a Drosophila Model for Type 2 Multiple Endocrine Neoplasia Syndromes and Papillary Thyroid Carcinoma. Cancer Res (2005) 65(9):3538–41. doi: 10.1158/0008-5472.CAN-04-4561
31. Bangi E, Garza D, Hild M. In Vivo Analysis of Compound Activity and Mechanism of Action Using Epistasis in Drosophila. J Chem Biol (2011) 4(2):55–68. doi: 10.1007/s12154-010-0051-5
32. Levine BD, Cagan RL. Drosophila Lung Cancer Models Identify Trametinib Plus Statin as Candidate Therapeutic. Cell Rep (2016) 14(6):1477–87. doi: 10.1016/j.celrep.2015.12.105
33. Bossen J, Uliczka K, Steen L, Pfefferkorn R, Mai MM-Q, Burkhardt L, et al. An EGFR-Induced Drosophila Lung Tumor Model Identifies Alternative Combination Treatments. Mol Cancer Ther (2019) 18(9):1659–68. doi: 10.1158/1535-7163.MCT-19-0168
34. Bhandari P, Shashidhara LS. Studies on Human Colon Cancer Gene APC by Targeted Expression in Drosophila. Oncogene (2001) 20(47):6871–80. doi: 10.1038/sj.onc.1204849
35. Palmer AC, Sorger PK. Combination Cancer Therapy Can Confer Benefit Via Patient-to-Patient Variability Without Drug Additivity or Synergy. Cell (2017) 171(7):1678–91. doi: 10.1016/j.cell.2017.11.009
36. Chen S-H, Lahav G. Two Is Better Than One; Toward a Rational Design of Combinatorial Therapy. Curr Opin Struct Biol (2016) 41:145–50. doi: 10.1016/j.sbi.2016.07.020
37. Yardley DA. Drug Resistance and the Role of Combination Chemotherapy in Improving Patient Outcomes. Int J Breast Cancer (2013) 2013:137414. doi: 10.1155/2013/137414
38. Aird RE, Cummings J, Ritchie AA, Muir M, Morris RE, Chen H, et al. In Vitro and In Vivo Activity and Cross Resistance Profiles of Novel Ruthenium (II) Organometallic Arene Complexes in Human Ovarian Cancer. Br J Cancer (2002) 86(10):1652–7. doi: 10.1038/sj.bjc.6600290
39. Murayama T, Gotoh N. Patient-Derived Xenograft Models of Breast Cancer and Their Application. Cells (2019) 8(6):621. doi: 10.3390/cells8060621
40. Bangi E, Ang C, Smibert P, Uzilov AV, Teague AG, Antipin Y, et al. A Personalized Platform Identifies Trametinib Plus Zoledronate for a Patient With KRAS-mutant Metastatic Colorectal Cancer. Sci Adv (2019) 5(5):eaav6528. doi: 10.1126/sciadv.aav6528
41. Passini E, Britton OJ, Lu HR, Rohrbacher J, Hermans AN, Gallacher DJ, et al. Human in Silico Drug Trials Demonstrate Higher Accuracy Than Animal Models in Predicting Clinical Pro-Arrhythmic Cardiotoxicity. Front Physiol (2017) 8:668. doi: 10.3389/fphys.2017.00668
42. Richardson HE, Willoughby L, Humbert PO. Screening for Anti-Cancer Drugs in Drosophila. eLS (2015) 1–14. doi: 10.1002/9780470015902.a0022535
43. Micchelli CA, Perrimon N. Evidence That Stem Cells Reside in the Adult Drosophila Midgut Epithelium. Nature (2006) 439(7075):475–79. doi: 10.1038/nature04371
44. Ohlstein B, Spradling A. The Adult Drosophila Posterior Midgut Is Maintained by Pluripotent Stem Cells. Nature (2006) 439(7075):470–4. doi: 10.1038/nature04333
45. Takashima S, Adams KL, Ortiz PA, Ying CT, Moridzadeh R, Younossi-Hartenstein A, et al. Development of the Drosophila Entero-Endocrine Lineage and Its Specification by the Notch Signaling Pathway. Dev Biol (2011) 353(2):161–72. doi: 10.1016/j.ydbio.2011.01.039
46. Ohlstein B, Spradling A. Multipotent Drosophila Intestinal Stem Cells Specify Daughter Cell Fates by Differential Notch Signaling. Science (2007) 315(5814):988–92. doi: 10.1126/science.1136606
47. Lee M, Vasioukhin V. Cell Polarity and Cancer – Cell and Tissue Polarity as a non-Canonical Tumor Suppressor. J Cell Sci (2008) 121(8):1141–50. doi: 10.1242/jcs.016634
48. Pasco MY, Loudhaief R, Gallet A. The Cellular Homeostasis of the Gut: What the Drosophila Model Points Out. Histol Histopathol (2015) 30(3):277–92. doi: 10.14670/HH-30.277
49. Loza-coll MA, Southall TD, Sandall SL, Brand AH, Jones DL. Regulation of Drosophila Intestinal Stem Cell Maintenance and Differentiation by the Transcription Factor Escargot. EMBO J (2014) 33(24):2983–96. doi: 10.15252/embj.201489050
50. Zeng X, Hou SX. Enteroendocrine Cells are Generated From Stem Cells Through a Distinct Progenitor in the Adult Drosophila Posterior Midgut. Development (2015) 142(4):644–53. doi: 10.1242/dev.113357
51. Macara IG, McCaffrey L. Cell Polarity in Morphogenesis and Metastasis. Philos Trans R Soc Lond B Biol Sci (2013) 368(1629):20130012. doi: 10.1098/rstb.2013.0012
52. Guo Z, Ohlstein B. Bidirectional Notch Signaling Regulates Drosophila Intestinal Stem Cell Multipotency. Science (2015) 350(6263):927. doi: 10.1126/science.aab0988
53. Snoeck V, Goddeeris B, Cox E. The Role of Enterocytes in the Intestinal Barrier Function and Antigen Uptake. Microbes Infect (2005) 7(7–8):997–1004. doi: 10.1016/j.micinf.2005.04.003
54. Wolfstetter G, Shirinian M, Stute C, Grabbe C, Hummel T, Baumgartner S, et al. Fusion of Circular and Longitudinal Muscles in Drosophila is Independent of the Endoderm But Further Visceral Muscle Differentiation Requires a Close Contact Between Mesoderm and Endoderm. Mech Dev (2009) 126(8–9):721–36. doi: 10.1016/j.mod.2009.05.001
55. Martorell Ò, Merlos-Suárez A, Campbell K, Barriga FM, Christov CP, Miguel-Aliaga I, et al. Conserved Mechanisms of Tumorigenesis in the Drosophila Adult Midgut. PloS One (2014) 9(2):e88413. doi: 10.1371/journal.pone.0088413
56. Markstein M, Dettorre S, Cho J, Neumuller RA, Craig-Muller S, Perrimon N. Systematic Screen of Chemotherapeutics in Drosophila Stem Cell Tumors. Proc Natl Acad Sci (2014) 111(12):4530–5. doi: 10.1073/pnas.1401160111
57. Stone L. The Feasibility and Stability of Large Complex Biological Networks: A Random Matrix Approach. Sci Rep (2018) 8(1):1–12. doi: 10.1038/s41598-018-26486-2
58. Kwon Y-K, Cho K-H. Quantitative Analysis of Robustness and Fragility in Biological Networks Based on Feedback Dynamics. Bioinformatics (2008) 24(7):987–94. doi: 10.1093/bioinformatics/btn060
59. Glass L, Kauffman SA. The Logical Analysis of Continuous, Non-Linear Biochemical Control Networks. J Theor Biol (1973) 39(1):103–29. doi: 10.1016/0022-5193(73)90208-7
60. Chatterjee M, Ip YT. Pathogenic Stimulation of Intestinal Stem Cell Response in Drosophila. J Cell Physiol (2009) 220(3):664–71. doi: 10.1002/jcp.21808
61. Wang L, Ryoo HD, Qi Y, Jasper H. PERK Limits Drosophila Lifespan by Promoting Intestinal Stem Cell Proliferation in Response to ER Stress. PloS Genet (2015) 11(5):e1005220. doi: 10.1371/journal.pgen.1005220
62. Rodriguez-Fernandez IA, Qi Y, Jasper H. Loss of a Proteostatic Checkpoint in Intestinal Stem Cells Contributes to Age-Related Epithelial Dysfunction. Nat Commun (2019) 10(1):1–15. doi: 10.1038/s41467-019-08982-9
63. Amcheslavsky A, Jiang J, Ip YT. Tissue Damage-Induced Intestinal Stem Cell Division in Drosophila. Cell Stem Cell (2009) 4(1):49–61. doi: 10.1016/j.stem.2008.10.016
64. Jiang H, Patel PH, Kohlmaier A, Grenley MO, McEwen DG, Edgar BA. Cytokine/Jak/Stat Signaling Mediates Regeneration and Homeostasis in the Drosophila Midgut. Cell (2009) 137(7):1343–55. doi: 10.1016/j.cell.2009.05.014
65. Cordero JB, Stefanatos RK, Myant K, Vidal M, Sansom OJ. Non-Autonomous Crosstalk Between the Jak/Stat and Egfr Pathways Mediates Apc1-Driven Intestinal Stem Cell Hyperplasia in the Drosophila Adult Midgut. Development (2012) 139(24):4524–35. doi: 10.1242/dev.078261
66. McLeod CJ, Wang L, Wong C, Jones DL. Stem Cell Dynamics in Response to Nutrient Availability. Curr Biol (2010) 20(23):2100–5. doi: 10.1016/j.cub.2010.10.038
67. Goodlad RA, Wright NA. The Effects of Starvation and Refeeding on Intestinal Cell Proliferation in the Mouse. Virchows Arch B (1984) 45(1):63–73. doi: 10.1007/BF02889852
68. Schell JC, Wisidagama DR, Bensard C, Zhao H, Wei P, Tanner J, et al. Control of Intestinal Stem Cell Function and Proliferation by Mitochondrial Pyruvate Metabolism. Nat Cell Biol (2017) 19(9):1027–36. doi: 10.1038/ncb3593
69. Rojas Villa SE, Meng FW, Biteau B. Zfh2 Controls Progenitor Cell Activation and Differentiation in the Adult Drosophila Intestinal Absorptive Lineage. PloS Genet (2019) 15(12):e1008553. doi: 10.1371/journal.pgen.1008553
70. Koren E, Yosefzon Y, Ankawa R, Soteriou D, Jacob A, Nevelsky A, et al. ARTS Mediates Apoptosis and Regeneration of the Intestinal Stem Cell Niche. Nat Commun (2018) 9(1):1–17. doi: 10.1038/s41467-018-06941-4
71. Tower J. Stress and Stem Cells. Wiley Interdiscip Rev Dev Biol (2012) 1(6):789–802. doi: 10.1002/wdev.56
72. Bach SP, Renehan AG, Potten CS. Stem Cells: The Intestinal Stem Cell as a Paradigm. Carcinogenesis (2000) 21(3):469–76. doi: 10.1093/carcin/21.3.469
73. Pérez-Garijo A, Martín FA, Struhl G, Morata G. Dpp Signaling and the Induction of Neoplastic Tumors by Caspase-Inhibited Apoptotic Cells in Drosophila. Proc Natl Acad Sci USA (2005) 102(49):17664–9. doi: 10.1073/pnas.0508966102
74. Sancho E, Batlle E, Clevers H. Signaling Pathways in Intestinal Development and Cancer. Annu Rev Cell Dev Biol (2004) 20:695–723. doi: 10.1146/annurev.cellbio.20.010403.092805
75. Gou H-F, Li X, Qiu M, Cheng K, Li L-H, Dong H, et al. Epidermal Growth Factor Receptor (EGFR)-RAS Signaling Pathway in Penile Squamous Cell Carcinoma. PloS One (2013) 8(4):e62175. doi: 10.1371/journal.pone.0062175
76. Oberley LW, Oberley TD, Buettner GR. Cell Differentation, Aging and Cancer: The Possible Roles of Superoxide and Superoxide Dismutases. Med Hypotheses (1980) 6(3):249–68. doi: 10.1016/0306-9877(80)90123-1
77. Piñeiro-Yáñez E, Reboiro-Jato M, Gómez-López G, Perales-Patón J, Troulé K, Rodríguez JM, et al. PanDrugs: A Novel Method to Prioritize Anticancer Drug Treatments According to Individual Genomic Data. Genome Med (2018) 10(1):1–11. doi: 10.1186/s13073-018-0546-1
78. Khurshed M, Stroes CI, Schokker S, van der Woude S, Mathôt R, Slingerland M, et al. Regorafenib in Combination With Paclitaxel for Beyond First-Line Treatment of Advanced Esophagogastric Cancer (REPEAT): A Phase Ib Trial With Expansion Cohort. Ann Oncol (2019) 30(October):v307. doi: 10.1093/annonc/mdz247.124
79. Awada A, Albanell J, Canney PA, Dirix LY, Gil T, Cardoso F, et al. Bortezomib/Docetaxel Combination Therapy in Patients With Anthracycline-Pretreated Advanced/Metastatic Breast Cancer: A Phase I/II Dose-Escalation Study. Br J Cancer (2008) 98(9):1500–7. doi: 10.1038/sj.bjc.6604347
80. Lara PN, Koczywas M, Quinn DI, Lenz HJ, Davies AM, Lau DHM, et al. Bortezomib Plus Docetaxel in Advanced Non-Small Cell Lung Cancer and Other Solid Tumors: A Phase I California Cancer Consortium Trial. J Thorac Oncol (2006) 1(2):126–34. doi: 10.1016/s1556-0864(15)31527-6
81. Fanucchi MP, Fossella FV, Belt R, Natale R, Fidias P, Carbone DP, et al. Randomized Phase II Study of Bortezomib Alone and Bortezomib in Combination With Docetaxel in Previously Treated Advanced non-Small-Cell Lung Cancer. J Clin Oncol Off J Am Soc Clin Oncol (2006) 24(31):5025–33. doi: 10.1200/JCO.2006.06.1853
82. Dreicer R, Petrylak D, Agus D, Webb I, Roth B. Phase I/II Study of Bortezomib Plus Docetaxel in Patients With Advanced Androgen-Independent Prostate Cancer. Clin Cancer Res An Off J Am Assoc Cancer Res (2007) 13(4):1208–15. doi: 10.1158/1078-0432.CCR-06-2046
83. Pishvaian MJ, Slack R, Koh EY, Beumer JH, Hartley ML, Cotarla I, et al. A Phase I Clinical Trial of the Combination of Imatinib and Paclitaxel in Patients With Advanced or Metastatic Solid Tumors Refractory to Standard Therapy. Cancer Chemother Pharmacol (2012) 70(6):843–53. doi: 10.1007/s00280-012-1969-9
84. Bauman JE, Eaton KD, Wallace SG, Carr LL, Lee S-J, Jones DV, et al. A Phase II Study of Pulse Dose Imatinib Mesylate and Weekly Paclitaxel in Patients Aged 70 and Over With Advanced Non-Small Cell Lung Cancer. BMC Cancer (2012) 12(1):1–8. doi: 10.1186/1471-2407-12-449
85. Safra T, Andreopoulou E, Levinson B, Borgato L, Pothuri B, Blank S, et al. Weekly Paclitaxel With Intermittent Imatinib Mesylate (Gleevec): Tolerance and Activity in Recurrent Epithelial Ovarian Cancer. Anticancer Res (2010) 30(9):3243–7.
86. Iqbal N, Iqbal N. Imatinib: A Breakthrough of Targeted Therapy in Cancer. Chemother Res Pract (2014) 2014:357027. doi: 10.1155/2014/357027
87. Ramaswamy B, Bekaii-Saab T, Schaaf LJ, Lesinski GB, Lucas DM, Young DC, et al. A Dose-Finding and Pharmacodynamic Study of Bortezomib in Combination With Weekly Paclitaxel in Patients With Advanced Solid Tumors. Cancer Chemother Pharmacol (2010) 66(1):151–8. doi: 10.1007/s00280-009-1145-z
88. Kanehisa M. Kegg: Kyoto Encyclopedia of Genes and Genomes. Nucleic Acids Res (2000) 28(1):27–30. doi: 10.1093/nar/28.1.27
89. Yu J, Pacifico S, Liu G, Finley RL. DroID: The Drosophila Interactions Database, a Comprehensive Resource for Annotated Gene and Protein Interactions. BMC Genomics (2008) 9(1):1–9. doi: 10.1186/1471-2164-9-461
90. Giot L, Bader JS, Brouwer C, Chaudhuri A, Kuang B, Li Y, et al. A Protein Interaction Map of Drosophila Melanogaster. Science (2003) 302(5651):1727–36. doi: 10.1126/science.1090289
91. Formstecher E, Aresta S, Collura V, Hamburger A, Meil A, Trehin A, et al. Protein Interaction Mapping: A Drosophila Case Study. Genome Res (2005) 15(3):376–84. doi: 10.1101/gr.2659105
92. Vinson KE, George DC, Fender AW, Bertrand FE, Sigounas G. The Notch Pathway in Colorectal Cancer. Int J Cancer (2016) 138(8):1835–42. doi: 10.1002/ijc.29800
93. Buchon N, Broderick NA, Kuraishi T, Lemaitre B. Drosophila EGFR Pathway Coordinates Stem Cell Proliferation and Gut Remodeling Following Infection. BMC Biol (2010) 8(1):1–19. doi: 10.1186/1741-7007-8-152
94. Xu N, Wang SQ, Tan D, Gao Y, Lin G, Xi R. Egfr, Wingless and JAK/STAT Signaling Cooperatively Maintain Drosophila Intestinal Stem Cells. Dev Biol (2011) 354(1):31–43. doi: 10.1016/j.ydbio.2011.03.018
95. Slattery ML, Lundgreen A, Kadlubar SA, Bondurant KL, Wolff RK. JAK/STAT/SOCS-Signaling Pathway and Colon and Rectal Cancer. Mol Carcinog (2013) 52(2):155–66. doi: 10.1002/mc.21841
96. Tamamouna V, Panagi M, Theophanous A, Demosthenous M, Michail M, Papadopoulou M, et al. Evidence of Two Types of Balance Between Stem Cell Mitosis and Enterocyte Nucleus Growth in the Drosophila Midgut. Development (2020) 147(11):dev189472. doi: 10.1242/dev.189472
97. Karpowicz P, Perez J, Perrimon N. The Hippo Tumor Suppressor Pathway Regulates Intestinal Stem Cell Regeneration. Development (2010) 137(24):4135–45. doi: 10.1242/dev.060483
98. Huang T, Kang W, Cheng ASL, Yu J, To KF. The Emerging Role of Slit-Robo Pathway in Gastric and Other Gastro Intestinal Cancers. BMC Cancer (2015) 15(1):1–9. doi: 10.1186/s12885-015-1984-4
99. Gondal MN, Sultan MU, Arif A, Rehman A, Awan HA, Arshad Z, et al. TISON: A Next-Generation Multi-Scale Modeling Theatre for in Silico Systems Oncology. BioRxiv (2021). doi: 10.1101/2021.05.04.442539
100. Darabos C, Cunto F, Tomassini M, Moore JH, Provero P. Additive Functions in Boolean Models of Gene Regulatory Network Modules. PloS One (2011) 6(11):e25110. doi: 10.1371/journal.pone.0025110
101. Shah OS, Chaudhary MFA, Awan HA, Fatima F, Arshad Z, Amina B, et al. Atlantis - Attractor Landscape Analysis Toolbox for Cell Fate Discovery and Reprogramming. Sci Rep (2018) 8(1):1–11. doi: 10.1038/s41598-018-22031-3
102. Buchon N, Osman D, David FPA, Yu Fang H, Boquete J-P, Deplancke B, et al. Morphological and Molecular Characterization of Adult Midgut Compartmentalization in Drosophila. Cell Rep (2013) 3(5):1725–38. doi: 10.1016/j.celrep.2013.04.001
103. Smirnov P, Kofia V, Maru A, Freeman M, Ho C, El-Hachem N, et al. PharmacoDB: An Integrative Database for Mining In Vitro Anticancer Drug Screening Studies. Nucleic Acids Res (2018) 46(D1):D994–1002. doi: 10.1093/nar/gkx911
104. Chakravarty D, Gao J, Phillips SM, Kundra R, Zhang H, Wang J, et al. Oncokb: A Precision Oncology Knowledge Base. JCO Precis Oncol (2017) 1:1–16. doi: 10.1200/PO.17.00011
105. Griffith M, Griffith OL, Coffman AC, Weible JV, McMichael JF, Spies NC, et al. Dgidb: Mining the Druggable Genome. Nat Methods (2013) 10(12):1209–10. doi: 10.1038/nmeth.2689
106. Yang W, Soares J, Greninger P, Edelman EJ, Lightfoot H, Forbes S, et al. Genomics of Drug Sensitivity in Cancer (Gdsc): A Resource for Therapeutic Biomarker Discovery in Cancer Cells. Nucleic Acids Res (2013) 41(D1):955–61. doi: 10.1093/nar/gks1111
107. Wishart DS, Feunang YD, Guo AC, Lo EJ, Marcu A, Grant JR, et al. DrugBank 5.0: A Major Update to the DrugBank Database for 2018. Nucleic Acids Res (2018) 46(D1):D1074–82. doi: 10.1093/nar/gkx1037
108. Wang Z, Wei Y, Fang G, Hong D, An L, Jiao T, et al. Colorectal Cancer Combination Therapy Using Drug and Gene Co-Delivered, Targeted Poly(Ethylene Glycol)-ϵ-Poly(Caprolactone) Nanocarriers. Drug Des Devel Ther (2018) 12:3171–80. doi: 10.2147/DDDT.S175614
109. Arshad OA, Datta A. Towards Targeted Combinatorial Therapy Design for the Treatment of Castration-Resistant Prostate Cancer. BMC Bioinf (2017) 18(4):5–15. doi: 10.1186/s12859-017-1522-2
110. Steinway SN, Zañudo JGT, Michel PJ, Feith DJ, Loughran TP, Albert R. Combinatorial Interventions Inhibit Tgfβ-Driven Epithelial-to-Mesenchymal Transition and Support Hybrid Cellular Phenotypes. NPJ Syst Biol Appl (2015) 1(1):1–12. doi: 10.1038/npjsba.2015.14
111. Cho S-H, Park S-M, Lee H-S, Lee H-Y, Cho K-H. Attractor Landscape Analysis of Colorectal Tumorigenesis and Its Reversion. BMC Syst Biol (2016) 10(1):96. doi: 10.1186/s12918-016-0341-9
112. Liu J, Ghanim M, Xue L, Brown CD, Iossifov I, Angeletti C, et al. Analysis of Drosophila Segmentation Network Identifies a JNK Pathway Factor Overexpressed in Kidney Cancer. Science (2009) 323(5918):1218–22. doi: 10.1126/science.1157669
113. Toku AE, Tekir SD, Özbayraktar FBK, Ülgen Ö. Reconstruction and Crosstalk of Protein-Protein Interaction Networks of Wnt and Hedgehog Signaling in Drosophila Melanogaster. Comput Biol Chem (2011) 35(5):282–92. doi: 10.1016/j.compbiolchem.2011.07.002
114. Schönborn JW, Jehrke L, Mettler-Altmann T, Beller M. Flysilico: Flux Balance Modeling of Drosophila Larval Growth and Resource Allocation. Sci Rep (2019) 9(1):1–16. doi: 10.1038/s41598-019-53532-4
115. Jiang H, Grenley MO, Bravo M-J, Blumhagen RZ, Edgar BA. EGFR/Ras/MAPK Signaling Mediates Adult Midgut Epithelial Homeostasis and Regeneration in Drosophila. Cell Stem Cell (2011) 8(1):84–95. doi: 10.1016/j.stem.2010.11.026
116. Casali A, Batlle E. Intestinal Stem Cells in Mammals and Drosophila. Cell Stem Cell (2009) 4(2):124–7. doi: 10.1016/j.stem.2009.01.009
117. Lucchetta EM, Ohlstein B. The Drosophila Midgut: A Model for Stem Cell Driven Tissue Regeneration. Wiley Interdiscip Rev Dev Biol (2012) 1(5):781–8. doi: 10.1002/wdev.51
118. Buchon N, Broderick NA, Chakrabarti S, Lemaitre B. Invasive and Indigenous Microbiota Impact Intestinal Stem Cell Activity Through Multiple Pathways in Drosophila. Genes Dev (2009) 23(19):2333–44. doi: 10.1101/gad.1827009
119. Apidianakis Y, Pitsouli C, Perrimon N, Rahme L. Synergy Between Bacterial Infection and Genetic Predisposition in Intestinal Dysplasia. Proc Natl Acad Sci USA (2009) 106(49):20883–8. doi: 10.1073/pnas.0911797106
Keywords: personalized in silico cancer models, Boolean network models, cancer systems biology, preclinical in silico drug screening, combinatorial therapeutics
Citation: Gondal MN, Butt RN, Shah OS, Sultan MU, Mustafa G, Nasir Z, Hussain R, Khawar H, Qazi R, Tariq M, Faisal A and Chaudhary SU (2021) A Personalized Therapeutics Approach Using an In Silico Drosophila Patient Model Reveals Optimal Chemo- and Targeted Therapy Combinations for Colorectal Cancer. Front. Oncol. 11:692592. doi: 10.3389/fonc.2021.692592
Received: 08 April 2021; Accepted: 30 June 2021;
Published: 16 July 2021.
Edited by:
Aniello Cerrato, Istituto per l’Endocrinologia e l’oncologia “Gaetano Salvatore, Consiglio Nazionale Delle Ricerche (CNR), ItalyReviewed by:
Yiorgos Apidianakis, University of Cyprus, CyprusDakang Xu, Shanghai Jiao Tong University, China
Copyright © 2021 Gondal, Butt, Shah, Sultan, Mustafa, Nasir, Hussain, Khawar, Qazi, Tariq, Faisal and Chaudhary. This is an open-access article distributed under the terms of the Creative Commons Attribution License (CC BY). The use, distribution or reproduction in other forums is permitted, provided the original author(s) and the copyright owner(s) are credited and that the original publication in this journal is cited, in accordance with accepted academic practice. No use, distribution or reproduction is permitted which does not comply with these terms.
*Correspondence: Safee Ullah Chaudhary, c2FmZWUudWxsYWguY2hhdWRoYXJ5QGdtYWlsLmNvbQ==