- 1State Key Laboratory of Genetic Engineering, Institute of Biostatistics, School of Life Sciences, Fudan University, Shanghai, China
- 2Division of Hepatobiliary and Pancreatic Surgery, Department of Surgery, The First Affiliated Hospital, Zhejiang University School of Medicine, Hangzhou, China
- 3Department of Surgery, Shanghai Jiaotong University School of Medicine, Shanghai, China
- 4Department of Hepatobiliary and Hydatid Surgery, The First Affiliated Hospital of Xinjiang Medical University, Urumqi, China
- 5Clinical Laboratory Diagnostics, School of Public Health, Gansu University of Chinese Medicine, Lanzhou, China
- 6Department of Clinical Laboratory, The 940th Hospital of Joint Logistics Support Force of Chinese People’s Liberation Army, Lanzhou, China
- 7Shanghai Center for Mathematical Sciences, Fudan University, Shanghai, China
Background: Surgical treatment remains the best option for patients with hepatocellular carcinoma (HCC) caused by chronic hepatitis B virus (HBV) infection. However, there is no optimal tool based on readily accessible clinical parameters to predict postoperative complications. Herein, our study aimed to develop models that permitted risk of severe complications to be assessed before and after liver resection based on conventional variables.
Methods: A total of 1,047 patients treated by hepatectomy for HCC with HBV infection at three different centers were recruited retrospectively between July 1, 2014, and July 1, 2018. All surgical complications were recorded and scored by the Comprehensive Complication Index (CCI). A CCI ≥26.2 was used as a threshold to define patients with severe complications. We built two models for the CCI, one using preoperative and one using preoperative and postoperative data. Besides, CCI and other potentially relevant factors were evaluated for their ability to predict early recurrence and metastasis. All the findings were internally validated in the Hangzhou cohort and then externally validated in the Lanzhou and Urumqi cohorts.
Results: Multivariable analysis identified National Nosocomial Infections Surveillance (NNIS) index, tumor number, gamma-glutamyltransferase (GGT), total cholesterol (TC), potassium, and thrombin time as the key preoperative parameters related to perioperative complications. The nomogram based on the preoperative model [preoperative CCI After Surgery for Liver tumor (CCIASL-pre)] showed good discriminatory performance internally and externally. A more accurate model [postoperative CCI After Surgery for Liver tumor (CCIASL-post)] was established, combined with the other four postoperative predictors including leukocyte count, basophil count, erythrocyte count, and total bilirubin level. No significant association was observed between CCI and long-term complications.
Conclusion: Based on the widely available clinical data, statistical models were established to predict the complications after hepatectomy in patients with HBV infection. All the findings were extensively validated and shown to be applicable nationwide. Such models could be used as guidelines for surveillance follow-up and the design of post-resection adjuvant therapy.
Introduction
Hepatocellular carcinoma (HCC) is the most common primary liver tumor and the third leading cause of cancer-related deaths around the world. China alone accounts for more than half of the global HCC cases (1, 2). Hepatitis B virus (HBV) infection is one of the main etiologies of HCC around the world, especially in hepatitis B-prevalent regions such as sub-Saharan Africa and East Asia (3, 4). For patients suffering from chronic hepatitis B infection, the treatment of HCC is more difficult, the prognosis is worse, and the recurrence is earlier. Nevertheless, surgical treatment still remains the best option for patients with preserved liver function among a variety of treatments (5, 6).
Currently, there is no consensus regarding the optimal tool for risk stratification derived from surgically managed patients with HBV infection. Suboptimal management of perioperative period may partially lead to severe postoperative complications such as post-hepatectomy liver failure (PHLF) (7), post-hepatectomy hemorrhage (PHH), and postoperative death. For a long time, Child–Pugh scoring system, albumin–bilirubin (ALBI) grading system, and end-stage liver disease (MELD) score were the traditional basic indices of preoperative liver functions (8–10). In recent years, many novel approaches to assessing total liver function and functional remnant liver have emerged, such as indocyanine green (ICG) clearance test (10–13), liver scintigraphy, and liver stiffness measurement (LSM) by transient elastography (TE) (14–18). However, few people have access to these latest liver-specific evaluations in developing countries due to the lack of detection equipment. Hence, a prediction model that only consists of readily accessible clinical and pathological parameters is more practical to help surgeons to identify patients at risk of severe complications.
Clavien–Dindo classification was a traditional widely used grading system of surgical complications (19). On this basis, the Comprehensive Complication Index (CCI) has been proposed recently. The index integrates all recorded complications after surgery in a single formula weighted by severity and shows more sensitivity than the traditional one (20, 21).
CCI is a comprehensive measurement of short-term complications during perioperative period. In addition, tumor recurrence, a major long-term complication, deserves attention as well. Two-year duration is generally used as the cutoff to determine early or late recurrence (22, 23). Early recurrence (i.e., within 2 years after surgery) accounts for more than 70% of tumor recurrence and is considered a “real recurrence” (24). Furthermore, metastasis is also a long-term complication and an important manifestation of recurrence. Different metastatic targets represent different tumor development trends (25). However, no clear relationship has been found between short- and long-term complications after operation. In particular, prediction of tumor metastasis and correlations of metastatic sites were rarely taken into account regarding HBV-related HCC in the previous analysis.
In this study, we recruited a large number of surgically treated patients for HCC with HBV infection from different provinces in China. Comprehensive common clinical, imaging, and pathological parameters were retrospectively reviewed in order to develop and validate models of complication prediction. Two models were first developed: one included parameters available before surgery for prediction of perioperative complications measured by CCI preoperatively, and the other included all accessible variables to enhance the accuracy of prediction. Moreover, risk factors for early recurrence and metastasis were identified from all variables as well as CCI.
Methods
Patient Recruitment
In this national retrospective cohort study, patients were recruited from three centers in different provinces. These centers comprise Hangzhou (the First Affiliated Hospital of Zhejiang University School of Medicine, Hangzhou), Lanzhou (the 940th Hospital of Joint Logistics Support Force of Chinese People’s Liberation Army, Lanzhou), and Urumqi (the First Affiliated Hospital of Xinjiang Medical University, Urumqi).
The inclusion criteria were as follows: 1) patients undergoing liver resection for HCC diagnosed pathologically; 2) HBV surface antigen (HBsAg)- and/or HBV core antibody (HBcAb)-positive patients; 3) Child–Pugh class A or B (score ≤7) patients; 4) patients performing preoperative abdominal contrast-enhanced magnetic resonance imaging (MRI) and/or contrast-enhanced computed tomography (CT) scan; 5) anatomical and non-anatomical hepatectomies before July 1, 2018. The following exclusion criteria were also met: 1) patients undergoing more than one additional procedure in the liver; 2) patients with a history of tumors; 3) HCV-positive patients; 4) patients with incomplete clinical data; 5) patients receiving antitumor therapy before operation [i.e., transarterial chemoembolization (TACE), radiotherapy, or chemotherapy].
Through strict selection and careful data record, 1,047 eligible patients were included in total, 675 of whom were from Hangzhou (415 available survival data), 252 from Lanzhou, and 120 from Urumqi. The study obeyed the ethical guidelines of the 1975 Declaration of Helsinki and was approved by the institutional ethics committee. Informed consent was obtained from each patient before surgery.
Data Collection
The information of patients was prospectively collected into electronic spreadsheets by each center and then retrospectively reviewed. Demographics and comorbidities of patients were obtained from detailed consultation records. Preoperative and postoperative blood was taken from the patients for laboratory tests, including marks related to HBV, a complete blood count, blood chemical analysis, and coagulation testing; all the postoperative laboratory examinations were performed 3–5 days after surgery. Serum tumor markers were also investigated, such as α-fetoprotein (AFP), carbohydrate antigen 199 (CA199), and carcinoembryonic antigen (CEA). Tumor classification and characteristics relied on imaging examination reports and pathologic results of the resected specimens. The imaging data included tumor number, diameter, capsule status, location, lymphadenectasis, esophageal varices, ascites, and cirrhosis based on preoperative contrast-enhanced MRI and/or CT. The presence of arterial enhancement and washout of lesions on each of the dynamic imaging phases were also recorded. Arterial enhancement was defined as lesions exhibiting higher signal intensity on arterial phase images (26–28). Washout was defined as lesions with higher intensity compared with the surrounding liver on any late dynamic images except the arterial phase images (26–28). The pathologic results included the microvascular invasion, tumor giant cell, status of surrounding liver tissue, and differentiation and encapsulation of tumor. In addition, pathological immunohistochemistry tests for cytokeratin 19 (CK19), cytokeratin 7 (CK7), glypican 3, and CD34 were carried out. For both imaging and pathological data, two experienced specialists independently evaluated all results respectively. Any controversies in findings between the specialists were settled by discussion and generated a unified answer. Perioperative data were derived from operative recordings. Patients were followed up in the first 2 years after surgery to observe postoperative complications and early oncological outcomes. Information collection ended on July 1, 2020.
Measurement of Postoperative Complications and Clinical Outcomes
All surgical complications, defined as any deviation from the normal postoperative course that occurred before discharge, were recorded and scored using the CCI, ranging from 0 (uneventful course) to 100 (death). The threshold for defining patients with at least one grade III (major) complication was CCI ≥26.2 according to Clavien–Dindo classification (19, 29). This cutoff also takes into account the weight of multiple low-grade complications (e.g., grades I–II). Although these complications are not usually regarded as endpoints, they are considered to increase the postoperative experience of patients longer than a grade III complication in the CCI model. The CCI was used as the primary endpoint to assess the postoperative situation of patients.
Early recurrence of HCC was defined as the appearance of a newly detected tumor confirmed on two radiologic images within 2 years (30, 31). Relapse-free survival (RFS), the interval between liver resection and recurrence, was measured as the secondary endpoint. Among all kinds of recurrence, new tumor not located as the same as the primary one was diagnosed as metastasis. The metastasis was further divided into six categories depending on the location where tumor was newly discovered (lung, liver, abdomen, bone, lymph nodes, and brain).
Statistical Analyses
Continuous variables were described as medians with interquartile range (IQR), and nonparametric Mann–Whitney U tests were applied for statistical significance between high CCI (≥26.2) group and low CCI (<26.2) group. For categorical variables, we expressed the numbers and percentages of patients in each category. Proportions were compared using the χ2 test, with Yates’ correction or Fisher’s exact test. Serum HBV DNA, AFP, CA199, CA125, and ferritin were natural log transformed due to high skewness to the right.
The preoperative and postoperative CCI After Surgery for Liver tumor (CCIASL) models were built to predict the risk of developing high CCI on the Hangzhou dataset and then internally validated through bootstrap resampling. Subsequently, external validation of the two models was conducted on datasets from Lanzhou and Urumqi. One of the models, CCIASL-pre model, was based on preoperative predictors available before surgery; the other model, CCIASL-post model, was constructed on all available parameters.
Univariable logistic regression analyses were performed to screen potentially relevant variables. Those that reached p < 0.05 at univariable analysis were included in the multivariable logistic regression, and two-way stepwise strategy was applied for predictor selection. A number of potentially clinically plausible interactions were also taken into account during this process. The candidate multivariable regression model was built when Akaike information criterion (AIC) reached the minimum. We retained the statistically significant predictors in the final model. The risk score for prediction of high CCI was defined as the weight sum of the value of those significant parameters, and the weights were model β-estimates. The samples from each cohort were cut to high-risk and low-risk groups based on the median of risk score. Boxplots were drawn to compare risk differences between the two groups. The discrimination performance of the two models in the derivation and validation sets was then measured by the Harrell’s concordance index (C-index) and the receiver operating characteristic (ROC) curves. A relatively corrected C-index was also calculated by 1,000 bootstrap resampling for internal validation. Moreover, we made comparisons between two models. Net reclassification improvement (NRI) and integrated discrimination improvement (IDI) were computed using the category-free approach with 1,000 bootstrap replications to estimate the 95% confidence interval (CI) (32). The significant factors related to high CCI in the two models were used to construct nomograms. Calibration curves were subsequently drawn to assess the Hosmer–Lemeshow goodness of fit (33). Bootstrapping was used for each model to get bias-corrected (overfitting-corrected) estimates of predicted vs. observed values. As a reference line, the diagonal represents the best prediction. We also performed a decision curve analysis to determine whether our established nomogram was suitable for clinical utility by estimating the net benefits at different threshold probabilities.
Moreover, univariable Cox regression was applied to identify the association between CCI and early recurrence of HCC. The samples were stratified into various subgroups based on a number of important HCC-related markers, surgical factors, and tumor characteristics. Subgroup analysis was then conducted to explore possible correlations in each specific group of HCC patients. To identify more predictive factors for RFS, significant variables at univariable analysis were included to build a multivariable Cox regression model by both-direction stepwise selection. The proportional hazards assumption of the models was tested by examining the plots of scaled Schoenfeld residuals against time for each variable in the models. The patients were grouped into high risk and low risk according to median of the risk score, which was computed similar to the logistic regression described above. Kaplan–Meier (KM) survival analysis was performed to estimate the prediction value of the model. Survival nomogram was then constructed based on the significant factors in the multivariable Cox regression model. The predictive accuracy of the models was quantified through the concordance index (C-index) and time-dependent ROC curves with area under the curve (AUC). The level of agreement between the predicted probabilities and the actual possibility of early recurrence was measured by calibration plots. Model discrimination was performed both in the derivation cohort and in the validation cohorts.
To further explore the association between CCI and metastasis of HCC, univariable generalized linear model (GLM, here was logistic regression) was used. To identify more factors related to metastasis, significant variables at univariable analysis were included to build a multivariable GLM by both forward and backward stepwise selection. Given that tumor metastasis involved multiple locations, generalized estimation equation (GEE) was established to obtain more robust coefficients of the variables chosen by GLM selection and explore the correlations between different metastasis sites. The unstructured working correlation matrix was adopted due to the uncertainty of associations between various metastasis sites. The external validation set in this part was composed of Lanzhou and Urumqi cohorts.
All statistical tests were two-tailed, and differences were considered significant at a p-value <0.05. All statistical analyses were performed using R 3.6.1 software (R Foundation for Statistical Computing, Vienna, Austria) and SPSS 24.0 (SPSS, Chicago, USA).
Results
Patient Characteristics
Baseline features and postoperative complications were collected from 675 patients who were infected with HBV and received liver section for HCC in the Hangzhou cohort (Table 1). The median age was 56 years (IQR: 48–63), and the majority of patients were male (81.19%). Nearly all of the patients were Child–Pugh A (98.8%) and had positive HBsAg and HBcAb (99.4%). A total of 92 patients was considered high CCI (≥26.2), accounting for 13.6% of the whole population. Perioperative complications of patients with high CCI in the Hangzhou cohort were shown in detail in Table 2. This proportion was 13.3% in the Urumqi cohort and 7.9% in the Lanzhou cohort. Compared with patients with low CCI, those with high CCI were more likely from the worse physical condition and higher surgery risk according to the National Nosocomial Infections Surveillance (NNIS) index (p < 0.001), American Society of Anesthesiologists (ASA) score (p = 0.009), and Barcelona Clinic Liver Cancer (BCLC) stage (p < 0.001). Patients with high CCI had a longer hospital stay and a larger amount of ascites than those with low CCI. The median HBV-DNA level of all patients was 11.13 IU/ml (after natural log transformation), and insignificantly level increase was observed in high CCI group. High CCI patients also showed numerous differences in preoperative laboratory results, such as proportion of various blood cells, levels of bilirubin and enzymes, and coagulation time. Moreover, these differences became more significant after surgery. Based on imaging examination and pathological analysis, we discovered that tumor number, maximum tumor size, and conditions of hepatic capsule and surrounding satellite nodules were significantly different between the two groups. Of 415 patients with survival data, nearly half relapsed within 2 years and 65 metastasized. The average RFS of all was 16.29 months. No significant differences in clinical outcomes between the two groups of patients were observed. More detailed information of patients was shown in Table S1.
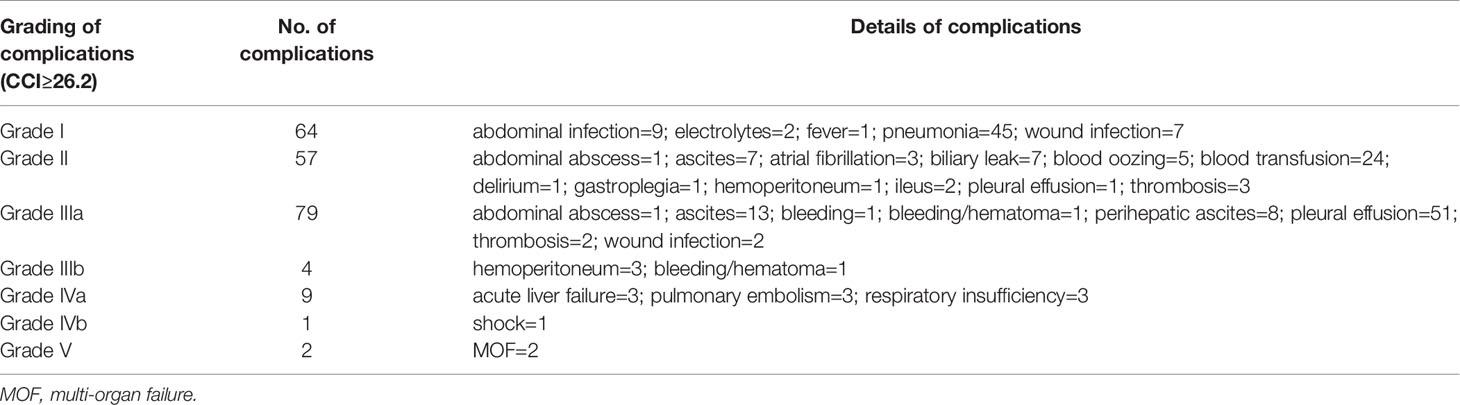
Table 2 Postoperative complications of patients with comprehensive complication index (CCI) ≥26.2 and their grade of severity.
Construction of the Model Predicting Severe Perioperative Complications
The result of univariable logistic regression analysis revealed that NNIS index, ASA score (III vs. I), BCLC stage (A4 vs. 0 and A4 vs. 0), tumor number, hepatic capsule (invaded vs. normal), surrounding satellite nodules, surgical approach, postoperative day (POD) 1 ascites volume, and intraoperative blood loss were significantly relevant with high CCI (Table 3). From the results of laboratory tests, erythrocyte count, total protein (TP), alkaline phosphatase (ALP), gamma-glutamyltransferase (GGT), and total cholesterol (TC) were discovered to be significantly associated with high CCI both before and after operation. Notably, the correlation between proportion of basophils in leukocytes and high CCI became significant in those experiencing surgery. The change of significance was observed in the relation of total bilirubin and high CCI as well.
After a multistep selection of significant variables at univariable analysis available before surgery, six of them were employed for building CCIASL-pre model, including NNIS index, tumor number, GGT, TC, potassium, and thrombin time (TT) (Figure 1A). The risk score formula was shown at the bottom of Table 3. As shown in the boxplot, patients with high CCI were scored significantly higher (Figure 1B). Based on the independent predictors in the final multivariable model, a nomogram was constructed to visualize the relationship with probability of high CCI (Figure 1C). In addition, another multivariable logistic regression model was developed based on all variables significant at univariable analysis to construct CCIASL-post. Five parameters of the CCIASL-pre model, namely, NNIS index, tumor number, GGT, TC, and potassium, maintained independence. On this basis, another four postoperative laboratory findings were identified, including leukocyte count, basophil, erythrocyte count, and total bilirubin (Figure 2A). Similar to the CCIASL-pre model, postoperative model formula of risk score was shown at the bottom of Table 3, and boxplot was drawn to display risk differences between two groups classified by the median risk score (Figure 2B). Nine independent predictors of CCIASL-post model were integrated in the nomogram (Figure 2C).
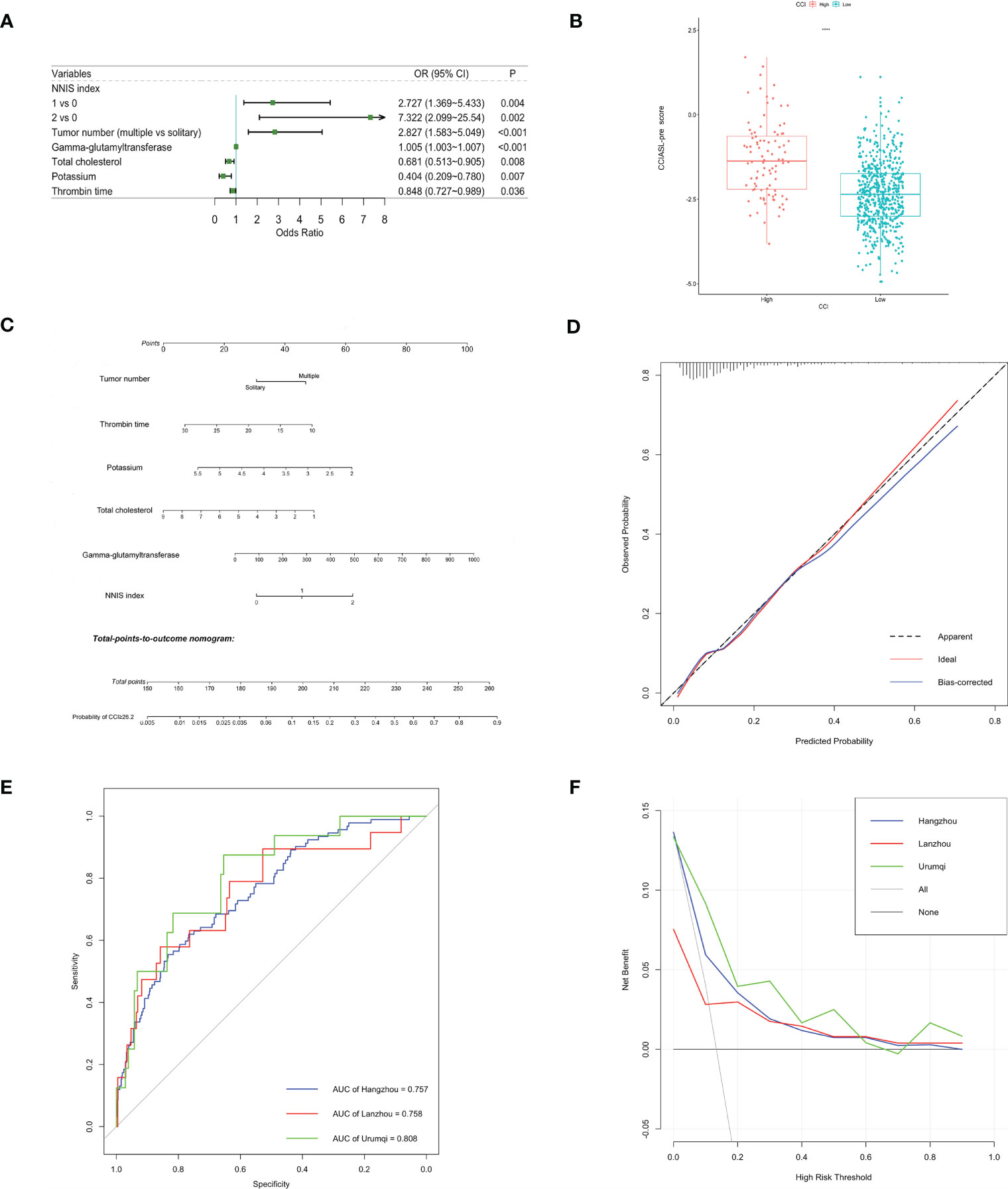
Figure 1 Construction and validation of the CCIASL-pre model. (A) Forest plot of predictors for CCI≥26.2 based on the result of multivariate analysis. (B) Boxplot of the CCIASL-pre risk score between high CCI group and low CCI group. Significant difference was observed. ****: P<0.0001. (C) Nomogram to predict probability of CCI≥26.2 in HCC patients. (D) Calibration curve for the nomogram to predict probability of CCI≥26.2 in the derivation cohort. The x-axis represents the predicted CCI≥26.2 probability and the y-axis denotes the actual proportion of CCI≥26.2. The black diagonal line indicates the best prediction. The red ideal line represents the uncorrected performance of the nomogram while the blue line shows the bias-corrected performance. (E) Receiver operating characteristic (ROC) curves in the derivation and validation cohorts. Corresponding area under curves (AUC) in the Hangzhou, Lanzhou and Urumqi were 0.757, 0.758 and 0.808, respectively. (F) Decision curve for the predictive nomogram. The net benefits were measured at different threshold probabilities. The blue, red and green lines represent the predictive ability of nomogram in the Hangzhou, Lanzhou and Urumqi cohorts, respectively. The gray line represents the assumption that all patients have severe complications. The black line represents the assumption that no patients have severe complications.
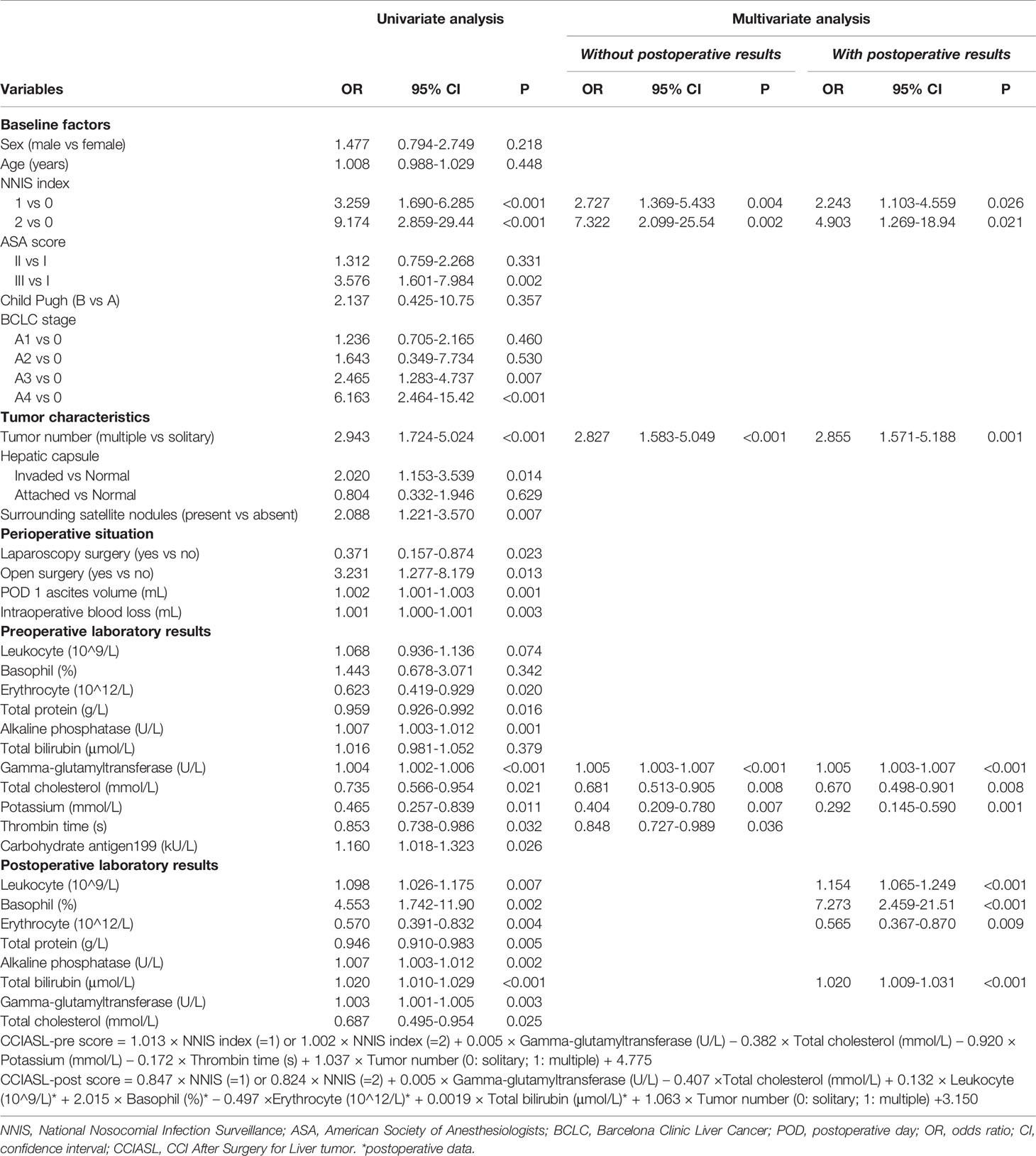
Table 3 Uni- and multivariate logistics regression analysis of predictors for high Comprehensive Complication Index (CCI≥26.2).
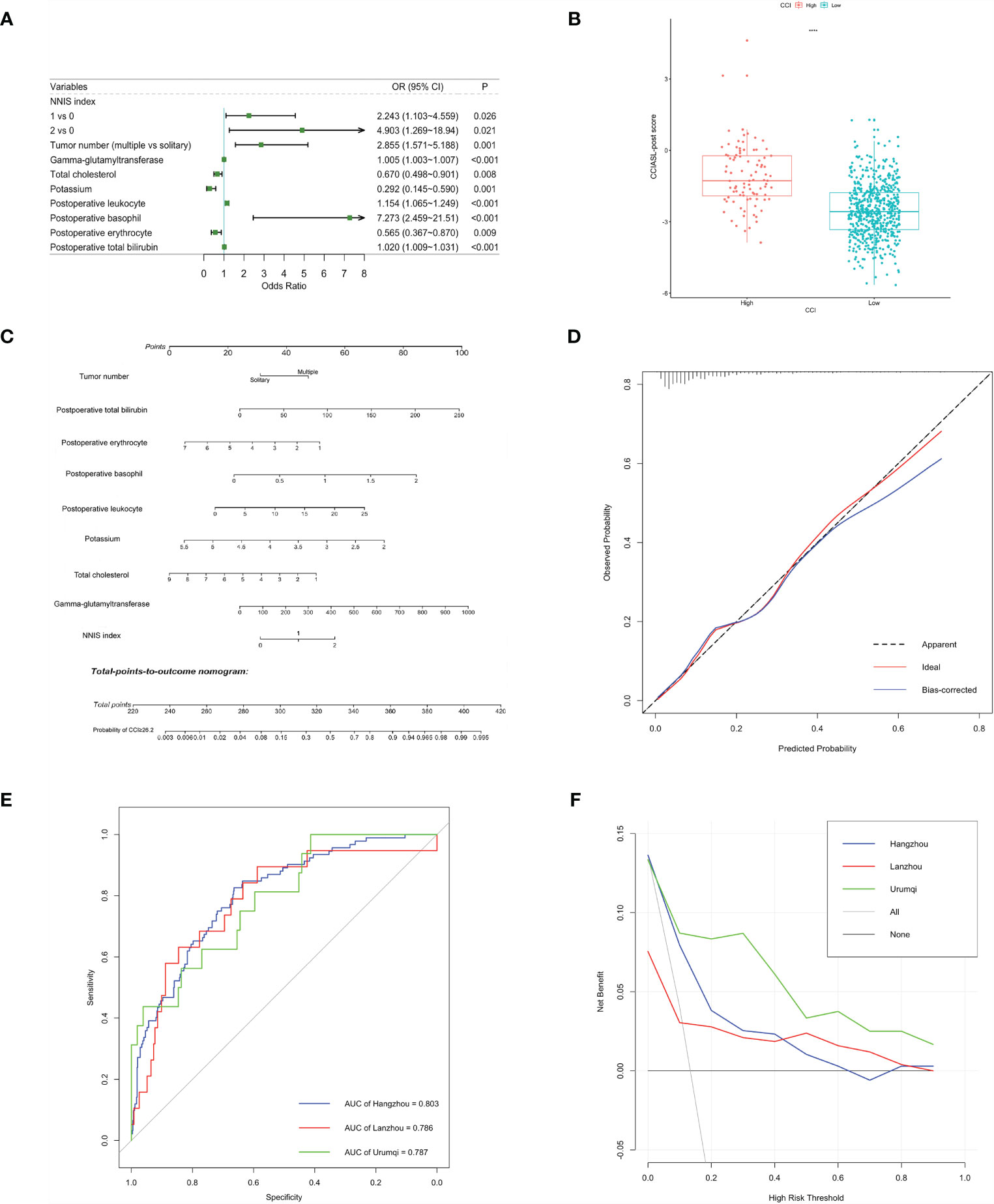
Figure 2 Construction and validation of the CCIASL-post model. (A) Forest plot of predictors for CCI≥26.2 based on the result of multivariate analysis. (B) Boxplot of the CCIASL-post risk score between high CCI group and low CCI group. Significant difference was observed. ****: P<0.0001. (C) Nomogram to predict probability of CCI≥26.2 in HCC patients. (D) Calibration curve for the nomogram to predict probability of CCI≥26.2 in the derivation cohort. The x-axis represents the predicted CCI≥26.2 probability and the y-axis denotes the actual proportion of CCI≥26.2. The black diagonal line indicates the best prediction. The red ideal line represents the uncorrected performance of the nomogram while the blue line shows the bias-corrected performance. (E) Receiver operating characteristic (ROC) curves for severe complications in the derivation and validation cohorts. Corresponding area under curves (AUC) in the Hangzhou, Lanzhou and Urumqi were 0.803, 0.786 and 0.787, respectively. (F) Decision curve for the predictive nomogram. The net benefits were measured at different threshold probabilities. The blue, red and green lines represent the predictive ability of nomogram in the Hangzhou, Lanzhou and Urumqi cohorts, respectively. The gray line represents the assumption that all patients have severe complications. The black line represents the assumption that no patients have severe complications.
Internal and External Assessment of the CCIASL Model
Both CCIASL models were first assessed internally in the Hangzhou cohort. As to CCIASL-pre model, the Hosmer–Lemeshow test did not indicate evidence of poor fit (p = 0.769), and the calibration plot also showed a good prediction capability (Figure 1D). The Harrell’s C-index, equivalent to AUC on ROC curves, was 0.757 (95% CI: 0.704–0.810) and corrected to be 0.767 (95% CI: 0.715–0.817) through bootstrapping validation, which indicated good discriminatory performance of the model (Figure 1E and Table 4). Additionally, the decision curve showed that making use of this model for predicting the probability of high CCI would gain more net benefits than an all-or-none patient intervention scheme if the threshold probability was less than 88%, which suggested a high potential for clinical application (Figure 1F). In the external validation cohorts, good discrimination and prediction ability were also observed, although calibration plots showed a slight deterioration, which was most pronounced in the Urumqi cohort (Figure S1). In terms of clinical usefulness, the Lanzhou cohort displayed better if threshold probability was more than 60%, while the Urumqi cohort performed better if threshold probability was less than 60%.
A better result was obtained regarding discriminatory performance of CCIASL-post model (Figures 2D, E). The C-indices reached around 0.8 in both the derivation cohort and validation cohorts. As shown in Figure 2F, the CCIASL-post model performed better in the validation cohorts, suggesting that its clinical practice was wider than that of the CCIASL-pre model, although the postoperative model would only gain more benefits if the threshold probability was less than 70% in the Hangzhou cohort. However, the results of reclassification analyses detected no significant differences between performance of the two models in all cohorts.
Prediction for Early Recurrence and Metastasis
Among all variables analyzed, CCI (high vs. low) was found not to be potentially relevant with early recurrence in the univariable analysis (hazard ratio = 0.862, 95% CI: 0.563–1.321, p = 0.496) (Table S2). The result was consistent in subgroup analysis, which demonstrated that perioperative complications after liver resection in patients with HBV infection had little association with the prediction for early relapse (Table S3). Nevertheless, by stepwise analysis, independent parameters were identified to be correlated with early recurrence, including HBV DNA, arterial phase, surrounding satellite nodules, microvascular invasion, preoperative TP, preoperative direct bilirubin, postoperative platelets, and postoperative ALP (Figure 3A). We did not detect any significant violation of the proportional hazard assumption, assessed by scaled Schoenfeld residuals on functions of time. The prognostic nomogram for early recurrence was shown in Figure 3B, which had a good prediction capability with a C-index of 0.701 (95% CI: 0.672–0.740) and the bootstrap estimate of C-index was 0.710 (95% CI: 0.649–0.776). The estimator in the validation cohort reached around 0.7, indicating that the prediction performance of the model was stable. The calibration plot showed an overall good agreement between the nomogram-predicted RFS and observed outcome (Figure 3C). This was also the case for the Lanzhou validation sets, but the deviation was larger in the Urumqi cohort (Figures S2A, B). Furthermore, time-dependent ROC analysis indicated that the prediction accuracies exceeded 0.7 in all cohorts (Figure 3E). KM survival curves displayed significantly poorer RFS of high-risk group categorized by the median risk score in the derivation and validation sets (p < 0.0001; Figure 3D; Figures S2C, D).
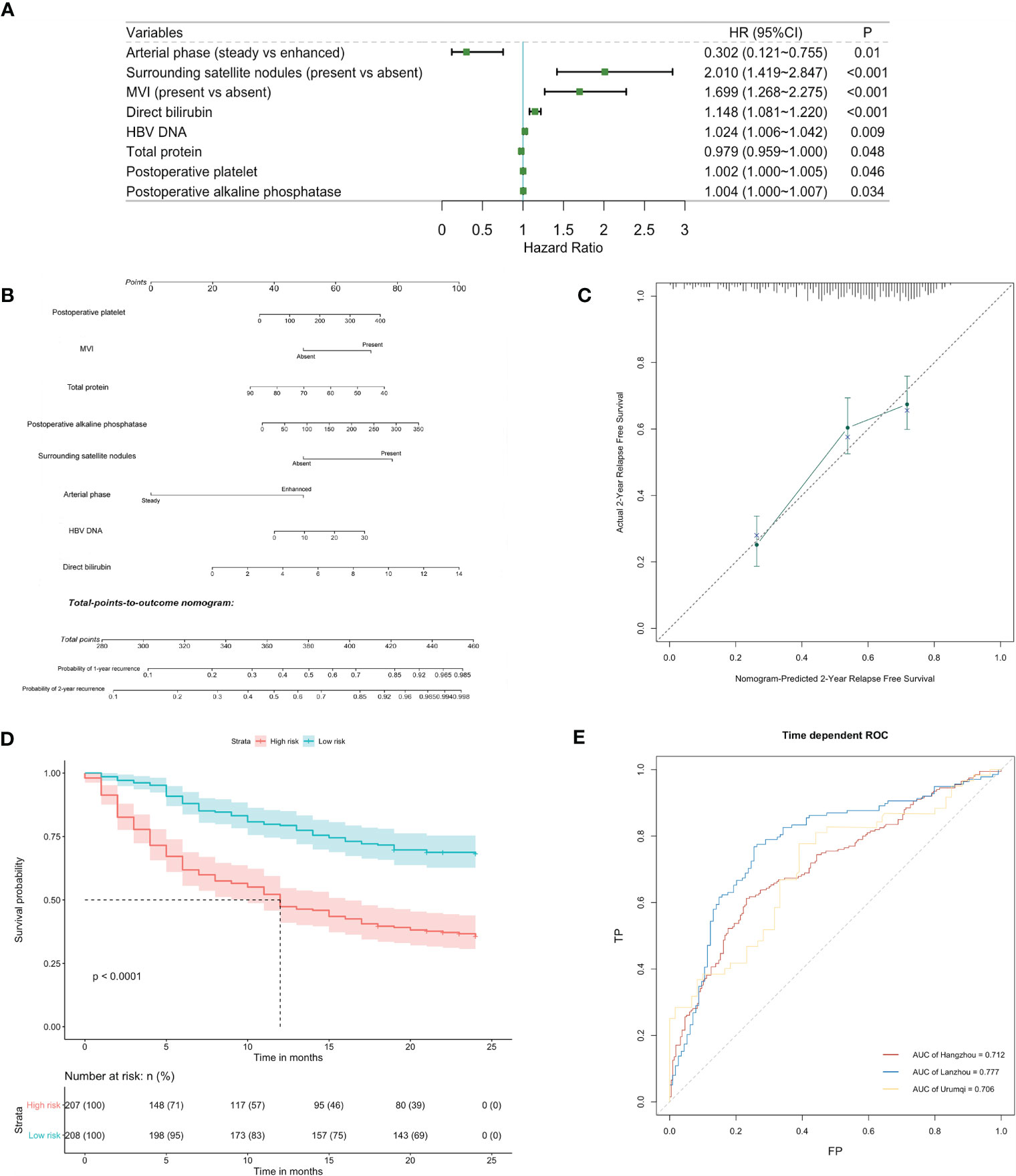
Figure 3 Identification and validation of risk factors for early recurrence. (A) Forest plot of predictors for early recurrence based on the result of multivariate analysis. (B) Nomogram to predict probability of early recurrence. (C) Calibration curve for the nomogram to predict probability of early recurrence in the derivation cohort. (D) Kaplan-Meier survival curve (high-risk vs low-risk patients) for relapse-free survival in the derivation cohort. (E) Time-dependent receiver operating characteristic (ROC) curves for relapse-free survival at 2 years in the derivation and validation cohorts. Corresponding area under curves (AUC) in the Hangzhou, Lanzhou and Urumqi were 0.712, 0.777 and 0.706, respectively.
To analyze impact factors on early relapse in more detail, we identified 65 patients with tumor metastasis from those experiencing recurrence in the internal cohort. The locations of metastasis in more than two cases were lung, liver, abdomen, bone, lymph nodes, and brain. Lung was the most common metastatic site, accounting for 7.23% of the entire population (Table S4). In the univariable analysis, CCI showed no relationship with metastasis (odds ratio = 0.759, 95% CI: 0.327–1.761, p = 0.521), which was consistent with previous findings. However, a total of 20 features were identified to be found potentially associated with metastasis (Table S5). These variables were then included in the multivariable model to select independent factors. As a result, fasting blood glucose (FBG) and TT decreased the odds of metastasis, while postoperative TP, intraoperative blood loss, maximum tumor size, surrounding satellite nodules, and tumor encapsulation increased the odds. In the external set, GEE analysis revealed that TT was a protective factor for metastasis, and absence of tumor encapsulation increased the risk of metastasis (Table 5). The correlations between the locations of tumor metastasis were uncertain according to working correlation matrix (Table S6), but whether negative or positive, the correlation was weak.
Discussion
Two models (CCIASL-pre and CCIASL-post) that enable risk assessment of perioperative complications before and after surgery have been derived and validated in a large national multicenter study of patients who underwent liver resection for HCC. Considering China is a hepatitis B-prevalent region, the models were constructed based on patients infected with HBV. Correspondingly, two predictive nomograms made assessments of complications more accurate by quantitatively establishing the relationship between values of predictors and probability of high CCI in a personalized way. This is a user-friendly tool for surgeons in clinical decision-making. The models are also suitable for simple application by stratifying surgical candidates into different risk levels based on risk scores.
Attentive postoperative management forms a core element in speeding recovery and reducing the chance of complications after hepatectomy, especially in cirrhotic livers with HBV infection. Postoperative bile leakage, ascites, hemorrhage, liver failure, and intra-abdominal abscesses were major short-term complications after liver resection, and the incidence of hepatectomy-related PHLF ranged from 0% to 43.1% that was frequently associated with mortality (34). In recent studies, LSM by emerging imaging examination, especially transient elastography and magnetic resonance elastography (35, 36), has been demonstrated to quantify the status of fibrosis and reflect the liver function in patients with HCC in order to promote perioperative management. Serenari et al. (18) reported a preoperative prediction of high CCI in patients with resectable HCC mainly based on LSM values. However, the accuracy of this transient elastography (FibroScan®) has been challenged by the risk of overestimating LSM. Actually, LSM is influenced by several other confounding factors such as age, obesity or high body mass index (BMI) and serum alanine aminotransferase (ALT), and total bilirubin levels (18, 37). Furthermore, recent studies showed that both acute hepatitis B (38) and chronic HBV infection (39) could increase the LSM value without significant fibrosis, indicating its less diagnostic accuracy in patients with HBV infection. Moreover, these measurements have not been easily accessible in developed countries, suggesting restricted practice in the clinics. Notably, our models are the first to be built solely on simple and readily available clinicopathological parameters. NNIS index, GGT, TC, potassium, and tumor number were identified to be significantly associated with severe complications in both models, indicating that these five preoperative variables possessed strong predictive capabilities for severe complications.
The NNIS system was first proposed by the Centers for Disease Control and Prevention (CDC), Atlanta, in the 1970s to predict surgical site infections (SSIs), which were considered to be the third most frequent nosocomial infection, occurring in 14%–16% of hospital inpatients (40, 41). A total of three risk factors have been evaluated in the NNIS system containing the status of surgical wound, the anesthesia score, and the procedure duration. As a nosocomial infection surveillance system, NNIS index is also an essential component in the Study of the Efficacy of Nosocomial Infection Control (SENIC) index (41) and can be used in different predictive models for postsurgical complications (42, 43). Additionally, coagulation parameters in cancer patients that represent hemostatic and fibrinolytic systems have been proven to have association with tumor progression and dissemination (44). It has been reported that the decreased pretreatment TT was associated with the shorter esophageal squamous cell carcinoma (ESCC) and HCC survival (45, 46). Meanwhile, conventional coagulation tests after liver surgery are frequently prolonged by postoperative hypercoagulability (47), and prolonged TT before surgery indicated hyper-fibrinogenolysis, suggested as a factor that prevents the occurrence of thromboembolic complications. Other parameters that might influence perioperative complications have also been added to our models including tumor number, potassium, GGT, and TC. Although the CCIASL-pre model is applicable solely on the basis of preoperative parameters, it still appears comparable to the existing model. Despite a minor degree of discrepancy in the validation cohorts, good discriminatory performance was maintained in general. Furthermore, CCIASL-post model provides a more accurate prediction for clinicians when all postoperative indicators are available several days after operation. The CCIASL models are quite reliable because of external validation in different geographic regions.
Although the prediction models for early recurrence after liver resection of HCC had been built a lot (48–50), they are seldom derived from comprehensive candidate variables potentially relevant to HBV-related HCC. In our study, the third model for prediction of early recurrence of HCC was constructed by incorporating eight parameters from HBV-related marks, surgical conditions, pathological examination, preoperative and postoperative laboratory results. A high HBV-DNA load was identified to be an independent hepatitis-related risk for early recurrence (51, 52). In addition to HBV-related marks, the capability of laboratory findings and tumor characteristics was also evaluated in predicting early recurrence. An approach to risk stratification for early recurrence of HBV-related HCC was developed by incorporating serum AFP, tumor number, and largest tumor diameter based on the Chinese population (53). From a broader perspective of HCC studies, there is a consensus that microvascular invasion is a well-known independent prognostic factor associated with more advanced tumor stage, tumor progression, and poorer clinical outcome (54). Microvascular invasion is an essential component in the majority of current prediction models (55, 56). However, associations between these predictors were detected as well. A high HBV DNA level was an independent risk factor of microvascular invasion, and HBcAb-positive HCC was much bigger, more often involved with vascular invasion and elevated AFP (50, 56). In this case, if all possible clinical variables are not included in the candidates, it is impossible to determine the truly independent predictors. The predictive factors included in the model we built on the basis of comprehensive candidate variables are more likely to avoid this problem.
Another highlight of this article is that we discovered two significant risk factors for early metastasis, namely, short TT and absence of tumor encapsulation. Thrombin is a serine protease that performs a multifaceted role in coagulation. Previous analysis has revealed that the coagulation system has an effect on solid tumors, such as ESCC and HCC (45, 46). Zhu et al. (57) also reported that the abnormal coagulation system was an independent prognostic factor for brain metastases of non-small cell lung cancer, which is consistent with our findings in HCC. The potential mechanism may refer to the damage of liver cells, the secretion of tumor cells, and the involvement of inflammation (58, 59). Notably, capsulation of tumor was first discovered to be relevant with tumor migration.
There are still three points worth further discussion. First of all, no significant association was observed between CCI and early recurrence. Some variables included in the CCIASL models have been identified in the prediction for long-term complications but never short-term ones. In this setting, the effect of short-term complications on long-term complications still remains inconclusive. Our results provide an exploration for this topic, and no certain relationship is a preliminary conclusion. Secondly, the difference of discrimination performance between preoperative and postoperative model was insignificant according to reclassification analyses. The improvement of prediction accuracy based on postoperative data did not live up to the expectation. However, the C-index of the CCIASL-post model is much higher than that of the pre-model. Better performance is likely to be found in larger samples. Thirdly, antiviral treatment was excluded at univariable analysis of predicting high CCI, suggesting that reduction of HBV load seemed not to be helpful for prevention of severe complications. Despite a lot of evidence that long-term survival is correlated with antiviral therapy (51, 60), the association of short-term complications has never been discovered. Hence, antiviral treatment is likely to be difficult to improve clinical perioperative complications.
In summary, CCI is a well-performed measurement of postoperative complications. Our CCIASL models are clinically relevant, externally validated, and powerful tools to predict severe perioperative complications in patients with HBV-related HCC. Further prospective studies are required to explore the clinical applicability of CCIASL models in patient allocation for more frequent follow-up and clinical trials for adjuvant therapy.
Data Availability Statement
The raw data supporting the conclusions of this article will be made available by the authors, upon reasonable request.
Ethics Statement
This study was approved by the Ethics Committee of the First Affiliated Hospital, College of Medicine, Zhejiang University (IIT2021A0143), the First Affiliated Hospital of Xinjiang Medical University (20200116-04) and the 940th Hospital of Joint Logistics Support force of Chinese People’s Liberation Army (2021KYLL023). The patients/participants provided their written informed consent to participate in this study.
Author Contributions
MB and QZ contributed to the conceptualization, data curation and software, formal analysis, visualization, investigation, and writing–original draft. YH contributed to funding acquisition and project administration. XyH, TA, and SW contributed to the methodology. TT, XqH, and AT contributed to the resources and validation. MB contributed to the supervision. MB and YH contributed to the writing–review and editing. All authors contributed to the article and approved the submitted version.
Funding
This study was supported by grants from the National Natural Science Foundation of China (Grants 11971117 and 11571082) and Scientific Research Foundation of Fudan University.
Conflict of Interest
The authors declare that the research was conducted in the absence of any commercial or financial relationships that could be construed as a potential conflict of interest.
Publisher’s Note
All claims expressed in this article are solely those of the authors and do not necessarily represent those of their affiliated organizations, or those of the publisher, the editors and the reviewers. Any product that may be evaluated in this article, or claim that may be made by its manufacturer, is not guaranteed or endorsed by the publisher.
Supplementary Material
The Supplementary Material for this article can be found online at: https://www.frontiersin.org/articles/10.3389/fonc.2021.717826/full#supplementary-material
References
1. Sung H, Ferlay J, Siegel RL, Laversanne M, Soerjomataram I, Jemal A, et al. Global Cancer Statistics 2020: GLOBOCAN Estimates of Incidence and Mortality Worldwide for 36 Cancers in 185 Countries. CA Cancer J Clin (2021) 71(3):209–249. doi: 10.3322/caac.21660
2. Chen W, Zheng R, Baade PD, Zhang S, Zeng H, Bray F, et al. Cancer Statistics in China, 2015. CA Cancer J Clin (2016) 66(2):115–32. doi: 10.3322/caac.21338
3. Li M, Li J, Li P, Li H, Su T, Zhu R, et al. Hepatitis B Virus Infection Increases the Risk of Cholangiocarcinoma: A Meta-Analysis and Systematic Review. J Gastroenterol Hepatol (2012) 27(10):1561–8. doi: 10.1111/j.1440-1746.2012.07207.x
4. Zhou Y, Zhao Y, Li B, Huang J, Wu L, Xu D, et al. Hepatitis Viruses Infection and Risk of Intrahepatic Cholangiocarcinoma: Evidence From a Meta-Analysis. BMC Cancer (2012) 12:289. doi: 10.1186/1471-2407-12-289
5. Bruix J, Sherman M. Management of Hepatocellular Carcinoma: An Update. Hepatology (2011) 53(3):1020–2. doi: 10.1002/hep.24199
6. Bellissimo F, Pinzone MR, Cacopardo B, Nunnari G. Diagnostic and Therapeutic Management of Hepatocellular Carcinoma. World J Gastroenterol (2015) 21(42):12003–21. doi: 10.3748/wjg.v21.i42.12003
7. Rahbari NN, Garden OJ, Padbury R, Brooke-Smith M, Crawford M, Adam R, et al. Posthepatectomy Liver Failure: A Definition and Grading by the International Study Group of Liver Surgery (ISGLS). Surgery (2011) 149(5):713–24. doi: 10.1016/j.surg.2010.10.001
9. Vauthey JN, Chaoui A, Do KA, Bilimoria MM, Fenstermacher MJ, Charnsangavej C, et al. Standardized Measurement of the Future Liver Remnant Prior to Extended Liver Resection: Methodology and Clinical Associations. Surgery (2000) 127(5):512–9. doi: 10.1067/msy.2000.105294
10. Cholongitas E, Marelli L, Shusang V, Senzolo M, Rolles K, Patch D, et al. A Systematic Review of the Performance of the Model for End-Stage Liver Disease (MELD) in the Setting of Liver Transplantation. Liver Transpl (2006) 12(7):1049–61. doi: 10.1002/lt.20824
11. Hiraoka A, Kumada T, Michitaka K, Kudo M. Newly Proposed ALBI Grade and ALBI-T Score as Tools for Assessment of Hepatic Function and Prognosis in Hepatocellular Carcinoma Patients. Liver Cancer (2019) 8(5):312–25. doi: 10.1159/000494844
12. Johnson PJ, Berhane S, Kagebayashi C, Satomura S, Teng M, Reeves HL, et al. Assessment of Liver Function in Patients With Hepatocellular Carcinoma: A New Evidence-Based Approach-the ALBI Grade. J Clin Oncol (2015) 33(6):550–8. doi: 10.1200/JCO.2014.57.9151
13. Huo TI, Lin HC, Huo SC, Lee PC, Wu JC, Lee FY, et al. Comparison of Four Model for End-Stage Liver Disease-Based Prognostic Systems for Cirrhosis. Liver Transpl (2008) 14(6):837–44. doi: 10.1002/lt.21439
14. Dinant S, de Graaf W, Verwer BJ, Bennink RJ, van Lienden KP, Gouma DJ, et al. Risk Assessment of Posthepatectomy Liver Failure Using Hepatobiliary Scintigraphy and CT Volumetry. J Nucl Med (2007) 48(5):685–92. doi: 10.2967/jnumed.106.038430
15. Hirai I, Kimura W, Fuse A, Suto K, Urayama M. Evaluation of Preoperative Portal Embolization for Safe Hepatectomy, With Special Reference to Assessment of Nonembolized Lobe Function With 99mtc-GSA SPECT Scintigraphy. Surgery (2003) 133(5):495–506. doi: 10.1067/msy.2003.138
16. Marasco G, Colecchia A, Colli A, Ravaioli F, Casazza G, Bacchi Reggiani ML, et al. Role of Liver and Spleen Stiffness in Predicting the Recurrence of Hepatocellular Carcinoma After Resection. J Hepatol (2019) 70(3):440–8. doi: 10.1016/j.jhep.2018.10.022
17. Rajakannu M, Cherqui D, Ciacio O, Golse N, Pittau G, Allard MA, et al. Liver Stiffness Measurement by Transient Elastography Predicts Late Posthepatectomy Outcomes in Patients Undergoing Resection for Hepatocellular Carcinoma. Surgery (2017) 162(4):766–74. doi: 10.1016/j.surg.2017.06.006
18. Serenari M, Han KH, Ravaioli F, Kim SU, Cucchetti A, Han DH, et al. A Nomogram Based on Liver Stiffness Predicts Postoperative Complications in Patients With Hepatocellular Carcinoma. J Hepatol (2020) 73(4):855–62. doi: 10.1016/j.jhep.2020.04.032
19. Clavien PA, Barkun J, de Oliveira ML, Vauthey JN, Dindo D, Schulick RD, et al. The Clavien-Dindo Classification of Surgical Complications: Five-Year Experience. Ann Surg (2009) 250(2):187–96. doi: 10.1097/SLA.0b013e3181b13ca2
20. Slankamenac K, Graf R, Barkun J, Puhan MA, Clavien PA. The Comprehensive Complication Index: A Novel Continuous Scale to Measure Surgical Morbidity. Ann Surg (2013) 258(1):1–7. doi: 10.1097/SLA.0b013e318296c732
21. Clavien PA, Vetter D, Staiger RD, Slankamenac K, Mehra T, Graf R, et al. The Comprehensive Complication Index (CCI®): Added Value and Clinical Perspectives 3 Years "Down the Line". Ann Surg (2017) 265(6):1045–50. doi: 10.1097/SLA.0000000000002132
22. Cucchetti A, Piscaglia F, Caturelli E, Benvegnù L, Vivarelli M, Ercolani G, et al. Comparison of Recurrence of Hepatocellular Carcinoma After Resection in Patients With Cirrhosis to Its Occurrence in a Surveilled Cirrhotic Population. Ann Surg Oncol (2009) 16(2):413–22. doi: 10.1245/s10434-008-0232-4
23. European Association For The Study Of The Liver, European Organisation For Research And Treatment Of Cancer. EASL-EORTC Clinical Practice Guidelines: Management of Hepatocellular Carcinoma. J Hepatol (2012) 56(4):908–43. doi: 10.1016/j.jhep.2011.12.001
24. Chan AW, Chan SL, Wong GL, Wong VW, Chong CC, Lai PB, et al. Prognostic Nutritional Index (PNI) Predicts Tumor Recurrence of Very Early/Early Stage Hepatocellular Carcinoma After Surgical Resection. Ann Surg Oncol (2015) 22(13):4138–48. doi: 10.1245/s10434-015-4516-1
25. Gupta GP, Massagué J. Cancer Metastasis: Building a Framework. Cell (2006) 127(4):679–95. doi: 10.1016/j.cell.2006.11.001
26. Witjes CD, Willemssen FE, Verheij J, van der Veer SJ, Hansen BE, Verhoef C, et al. Histological Differentiation Grade and Microvascular Invasion of Hepatocellular Carcinoma Predicted by Dynamic Contrast-Enhanced MRI. J Magn Reson Imaging (2012) 36(3):641–7. doi: 10.1002/jmri.23681
27. Kim MJ, Lee M, Choi JY, Park YN. Imaging Features of Small Hepatocellular Carcinomas With Microvascular Invasion on Gadoxetic Acid-Enhanced MR Imaging. Eur J Radiol (2012) 81(10):2507–12. doi: 10.1016/j.ejrad.2011.11.014
28. Choi YS, Rhee H, Choi JY, Chung YE, Park YN, Kim KW, et al. Histological Characteristics of Small Hepatocellular Carcinomas Showing Atypical Enhancement Patterns on Gadoxetic Acid-Enhanced MR Imaging. J Magn Reson Imaging (2013) 37(6):1384–91. doi: 10.1002/jmri.23940
29. Yamashita S, Sheth RA, Niekamp AS, Aloia TA, Chun YS, Lee JE, et al. Comprehensive Complication Index Predicts Cancer-Specific Survival After Resection of Colorectal Metastases Independent of RAS Mutational Status. Ann Surg (2017) 266(6):1045–54. doi: 10.1097/SLA.0000000000002018
30. Chan AWH, Zhong J, Berhane S, Toyoda H, Cucchetti A, Shi K, et al. Development of Pre and Post-Operative Models to Predict Early Recurrence of Hepatocellular Carcinoma After Surgical Resection. J Hepatol (2018) 69(6):1284–93. doi: 10.1016/j.jhep.2018.08.027
31. European Association for the Study of the Liver. EASL Clinical Practice Guidelines: Management of Hepatocellular Carcinoma. J Hepatol (2018) 69(1):182–236. doi: 10.1016/j.jhep.2018.03.019
32. Pencina MJ, D'Agostino RBR, Steyerberg EW. Extensions of Net Reclassification Improvement Calculations to Measure Usefulness of New Biomarkers. Stat Med (2011) 30(1):11–21. doi: 10.1002/sim.4085
33. Hosmer DW, Hosmer T, Le Cessie S, Lemeshow S. A Comparison of Goodness-of-Fit Tests for the Logistic Regression Model. Stat Med (1997) 16(9):965–80. doi: 10.1002/(SICI)1097-0258(19970515)16:9<965::AID-SIM509>3.0.CO;2-O
34. Mehrabi A, Golriz M, Khajeh E, Ghamarnejad O, Probst P, Fonouni H, et al. Meta-Analysis of the Prognostic Role of Perioperative Platelet Count in Posthepatectomy Liver Failure and Mortality. Br J Surg (2018) 105(10):1254–61. doi: 10.1002/bjs.10906
35. Singh S, Muir AJ, Dieterich DT, Falck-Ytter YT. American Gastroenterological Association Institute Technical Review on the Role of Elastography in Chronic Liver Diseases. Gastroenterology (2017) 152(6):1544–77. doi: 10.1053/j.gastro.2017.03.016
36. Bae JS, Lee DH, Yi NJ, Lee KW, Suh KS, Kim H, et al. Magnetic Resonance Elastography Versus Transient Elastography in the Prediction of Complications After Resection for Hepatocellular Carcinoma. Ann Surg (2020). doi: 10.1097/SLA.0000000000004576
37. European Association for Study of Liver, Asociacion Latinoamericana para el Estudio del Higado. EASL-ALEH Clinical Practice Guidelines: Non-Invasive Tests for Evaluation of Liver Disease Severity and Prognosis. J Hepatol (2015) 63(1):237–64. doi: 10.1016/j.jhep.2015.04.006
38. Wong GL, Chan HL, Choi PC, Chan AW, Lo AO, Chim AM, et al. Association Between Anthropometric Parameters and Measurements of Liver Stiffness by Transient Elastography. Clin Gastroenterol Hepatol (2013) 11(3):295–302.e1-3. doi: 10.1016/j.cgh.2012.09.025
39. Viganò M, Massironi S, Lampertico P, Iavarone M, Paggi S, Pozzi R, et al. Transient Elastography Assessment of the Liver Stiffness Dynamics During Acute Hepatitis B. Eur J Gastroenterol Hepatol (2010) 22(2):180–4. doi: 10.1097/MEG.0b013e328332d2fa
40. Horan TC, Gaynes RP, Martone WJ, Jarvis WR, Emori TG. CDC Definitions of Nosocomial Surgical Site Infections, 1992: A Modification of CDC Definitions of Surgical Wound Infections. Am J Infect Control (1992) 20(5):271–4. doi: 10.1016/S0196-6553(05)80201-9
41. Emori TG, Culver DH, Horan TC, Jarvis WR, White JW, Olson DR, et al. National Nosocomial Infections Surveillance System (NNIS): Description of Surveillance Methods. Am J Infect Control (1991) 19(1):19–35. doi: 10.1016/0196-6553(91)90157-8
42. Del Toro MD, Peñas C, Conde-Albarracín A, Palomino J, Brun F, Sánchez S, et al. Development and Validation of Baseline, Perioperative and at-Discharge Predictive Models for Postsurgical Prosthetic Joint Infection. Clin Microbiol Infect (2019) 25(2):196–202. doi: 10.1016/j.cmi.2018.04.023
43. Shen Z, Lin Y, Ye Y, Jiang K, Xie Q, Gao Z, et al. The Development and Validation of a Novel Model for Predicting Surgical Complications in Colorectal Cancer of Elderly Patients: Results From 1008 Cases. Eur J Surg Oncol (2018) 44(4):490–5. doi: 10.1016/j.ejso.2018.01.007
44. McAlister RK, Ito S. Minimal Prolongation of Prothrombin Time With Extended Exposure to Argatroban. Pharmacotherapy (2015) 35(7):e122–6. doi: 10.1002/phar.1613
45. Li XH, Wang XP, Gu WS, Lin JH, Huang H, Kang T, et al. Clinical Significance of Preoperative Thrombin Time in Patients With Esophageal Squamous Cell Carcinoma Following Surgical Resection. PLoS One (2015) 10(10):e0140323. doi: 10.1371/journal.pone.0140323
46. Wang XP, Mao MJ, He ZL, Zhang L, Chi PD, Su JR, et al. A Retrospective Discussion of the Prognostic Value of Combining Prothrombin Time(PT) and Fibrinogen(Fbg) in Patients With Hepatocellular Carcinoma. J Cancer (2017) 8(11):2079–87. doi: 10.7150/jca.19181
47. Mahla E, Lang T, Vicenzi MN, Werkgartner G, Maier R, Probst C, et al. Thromboelastography for Monitoring Prolonged Hypercoagulability After Major Abdominal Surgery. Anesth Analg (2001) 92(3):572–7. doi: 10.1213/00000539-200103000-00004
48. Zhang Z, Jiang H, Chen J, Wei Y, Cao L, Ye Z, et al. Hepatocellular Carcinoma: Radiomics Nomogram on Gadoxetic Acid-Enhanced MR Imaging for Early Postoperative Recurrence Prediction. Cancer Imaging (2019) 19(1):22. doi: 10.1186/s40644-019-0209-5
49. Xing H, Zhang WG, Cescon M, Liang L, Li C, Wang MD, et al. Defining and Predicting Early Recurrence After Liver Resection of Hepatocellular Carcinoma: A Multi-Institutional Study. HPB (Oxford) (2020) 22(5):677–89. doi: 10.1016/j.hpb.2019.09.006
50. Saito A, Toyoda H, Kobayashi M, Koiwa Y, Fujii H, Fujita K, et al. Prediction of Early Recurrence of Hepatocellular Carcinoma After Resection Using Digital Pathology Images Assessed by Machine Learning. Mod Pathol (2021) 34(2):417–25. doi: 10.1038/s41379-020-00671-z
51. Li Z, Lei Z, Xia Y, Li J, Wang K, Zhang H, et al. Association of Preoperative Antiviral Treatment With Incidences of Microvascular Invasion and Early Tumor Recurrence in Hepatitis B Virus-Related Hepatocellular Carcinoma. JAMA Surg (2018) 153(10):e182721. doi: 10.1001/jamasurg.2018.2721
52. Sohn W, Paik YH, Kim JM, Kwon CH, Joh JW, Cho JY, et al. HBV DNA and HBsAg Levels as Risk Predictors of Early and Late Recurrence After Curative Resection of HBV-Related Hepatocellular Carcinoma. Ann Surg Oncol (2014) 21(7):2429–35. doi: 10.1245/s10434-014-3621-x
53. Al-Ameri AAM, Wei X, Lin L, Shao Z, Guo H, Xie H, et al. Preoperative Risk Stratification for Early Recurrence of HBV-Related Hepatocellular Carcinoma After Deceased Donor Liver Transplantation: A Five-Eight Model Development and Validation. BMC Cancer (2019) 19(1):1136. doi: 10.1186/s12885-019-6343-4
54. Zhang X, Li J, Shen F, Lau WY. Significance of Presence of Microvascular Invasion in Specimens Obtained After Surgical Treatment of Hepatocellular Carcinoma. J Gastroenterol Hepatol (2018) 33(2):347–54. doi: 10.1111/jgh.13843
55. Lei Z, Li J, Wu D, Xia Y, Wang Q, Si A, et al. Nomogram for Preoperative Estimation of Microvascular Invasion Risk in Hepatitis B Virus-Related Hepatocellular Carcinoma Within the Milan Criteria. JAMA Surg (2016) 151(4):356–63. doi: 10.1001/jamasurg.2015.4257
56. Shim JH, Jun MJ, Han S, Lee YJ, Lee SG, Kim KM, et al. Prognostic Nomograms for Prediction of Recurrence and Survival After Curative Liver Resection for Hepatocellular Carcinoma. Ann Surg (2015) 261(5):939–46. doi: 10.1097/SLA.0000000000000747
57. Zhu JF, Cai L, Zhang XW, Wen YS, Su XD, Rong TH, et al. High Plasma Fibrinogen Concentration and Platelet Count Unfavorably Impact Survival in Non-Small Cell Lung Cancer Patients With Brain Metastases. Chin J Cancer (2014) 33(2):96–104. doi: 10.5732/cjc.012.10307
58. Aytac S, Turkay C, Bavbek N, Kosar A. Hemostasis and Global Fibrinolytic Capacity in Chronic Liver Disease. Blood Coagul Fibrinolysis (2007) 18(7):623–6. doi: 10.1097/MBC.0b013e328285d80e
59. Rak J, Milsom C, May L, Klement P, Yu J. Tissue Factor in Cancer and Angiogenesis: The Molecular Link Between Genetic Tumor Progression, Tumor Neovascularization, and Cancer Coagulopathy. Semin Thromb Hemost (2006) 32(1):54–70. doi: 10.1055/s-2006-933341
Keywords: modeling, hepatocellular carcinoma, complications, liver resection, comprehensive complication index
Citation: Bao M, Zhu Q, Aji T, Wei S, Tuergan T, Ha X, Tulahong A, Hu X and Hu Y (2021) Development of Models to Predict Postoperative Complications for Hepatitis B Virus-Related Hepatocellular Carcinoma. Front. Oncol. 11:717826. doi: 10.3389/fonc.2021.717826
Received: 31 May 2021; Accepted: 13 September 2021;
Published: 05 October 2021.
Edited by:
Francesco Giovinazzo, Catholic University of the Sacred Heart, ItalyReviewed by:
Lujun Shen, Sun Yat-sen University Cancer Center (SYSUCC), ChinaHao Ha, Seventh People’s Hospital of Shanghai, China
Copyright © 2021 Bao, Zhu, Aji, Wei, Tuergan, Ha, Tulahong, Hu and Hu. This is an open-access article distributed under the terms of the Creative Commons Attribution License (CC BY). The use, distribution or reproduction in other forums is permitted, provided the original author(s) and the copyright owner(s) are credited and that the original publication in this journal is cited, in accordance with accepted academic practice. No use, distribution or reproduction is permitted which does not comply with these terms.
*Correspondence: Xiaoyi Hu, MjE4MTgxNDBAemp1LmVkdS5jbg==; Yueqing Hu, eXVlaHVAZnVkYW4uZWR1LmNu
†These authors have contributed equally to this work