- 1Department of Gastrointestinal Surgery, Union Hospital, Tongji Medical College, Huazhong University of Science and Technology, Wuhan, China
- 2Wuhan Blood Center, Wuhan, China
- 3School of Optical and Electronic Information, Huazhong University of Science and Technology, Wuhan, China
- 4College of Life Science and Technology, Huazhong University of Science and Technology, Wuhan, China
- 5Cancer Center, Union Hospital, Tongji Medical College, Huazhong University of Science and Technology, Wuhan, China
- 6Rizhao City Hospital of Traditional Chinese Medicine (TCM), Rizhao City, China
- 7Department of Medical Oncology, Nanyang Central Hospital, Nanyang, China
- 8Department of Urology, Third Affiliated Hospital of Sun Yat-sen University, Guangzhou, China
Liver metastasis in colorectal cancer (CRC) is common and has an unfavorable prognosis. This study aimed to establish a functional nomogram model to predict overall survival (OS) and cancer-specific survival (CSS) in patients with colorectal cancer liver metastasis (CRCLM). A total of 9,736 patients with CRCLM from 2010 to 2016 were randomly assigned to training, internal validation, and external validation cohorts. Univariate and multivariate Cox analyses were performed to identify independent clinicopathologic predictive factors, and a nomogram was constructed to predict CSS and OS. Multivariate analysis demonstrated age, tumor location, differentiation, gender, TNM stage, chemotherapy, number of sampled lymph nodes, number of positive lymph nodes, tumor size, and metastatic surgery as independent predictors for CRCLM. A nomogram incorporating the 10 predictors was constructed. The nomogram showed favorable sensitivity at predicting 1-, 3-, and 5-year OS, with area under the receiver operating characteristic curve (AUROC) values of 0.816, 0.782, and 0.787 in the training cohort; 0.827, 0.769, and 0.774 in the internal validation cohort; and 0.819, 0.745, and 0.767 in the external validation cohort, respectively. For CSS, the values were 0.825, 0.771, and 0.772 in the training cohort; 0.828, 0.753, and 0.758 in the internal validation cohort; and 0.828, 0.737, and 0.772 in the external validation cohort, respectively. Calibration curves and ROC curves revealed that using our models to predict the OS and CSS would add more benefit than other single methods. In summary, the novel nomogram based on significant clinicopathological characteristics can be conveniently used to facilitate the postoperative individualized prediction of OS and CSS in CRCLM patients.
Introduction
Colorectal cancer (CRC) is the third most common cause of cancer-related death worldwide, with an estimated 145,600 new cases diagnosed in 2019; population-based studies have shown that about 30%–55% of CRC patients develop liver metastasis during their course of the disease (1, 2). In recent years, indications for the treatment of colorectal cancer liver metastases (CRCLM) have expanded, and surgical resection is the only chance of long-term survival. The goal of surgery should be to remove all metastases with negative histological margins while preserving sufficient functional liver parenchyma. Despite advances in oncology and surgery, treatment options vary among individuals, with only about 25% of patients benefiting from them (3, 4).
Predicting long-term survival in patients with CRCLM is a challenge due to genetic, ethnic, dietary, and geographical differences. However, accurate prediction of prognosis is critical for treatment selection and communication between doctors and patients. Previous studies have reported that important predictors of survival in CRCLM patients include multiple liver metastases, positive primary nodules, degree of primary tumor differentiation, extrahepatic spread, tumor size, CEA, positive surgical margin, venous infiltration, and tumor emergence (5, 6). At present, some studies have also found that the survival prognosis of CRCLM patients is closely related to the location of the primary tumor, lymphatic invasion, size and number of hepatic metastases, and the location of the metastatic tumors (7–10). In patients with CRCLM, right lobe metastasis is associated with poorer overall survival (OS) compared with the left lobe; central liver metastasis is a poor prognostic factor after hepatectomy and is associated with early recurrence. Intrahepatic lymphatic invasion, especially combined with vascular invasion, is also an important adverse prognostic factor for OS in patients with single CRCLM after hepatectomy. However, these previous studies did not further evaluate these prognostic risk factors in a comprehensive and systematic manner and study their combinations with the aim of establishing a corresponding prediction model that could accurately predict the prognosis of patients with colorectal cancer.
In our study, information on CRCLM patients was gathered from the SEER database and Wuhan Union Hospital Cancer Centre (WUHCC) cohorts. First, we divided the cases from the SEER database into a training set and an internal validation set. Then, we established an accurate and effective prediction nomogram for OS and cancer-specific survival (CSS) of CRC patients with liver metastasis based on common clinicopathological features and to further develop a histogram to evaluate the predictive value of the prediction nomogram.
Materials and Methods
Patients and Study Design
In this study, a total of 9,332 eligible patients from the SEER dataset and 404 patients from the WUHCC cohort with CRCLM were acquired. Selection criteria included the following: CRC patients diagnosed with liver metastasis from 2010 to 2016 of all ages. To identify patients with metastatic CRC cancer to the liver, we selected cases with CRCLM at first diagnosis for further research. Patients who were diagnosed via autopsy or death certificate or whose detailed information was unknown or blank were excluded. The detailed flow diagram of the patient selection process is shown in Figure 1. Analysis of the data from the SEER program was exempt from medical ethics review, and no informed consent was required. The Ethical Committee and Institutional Review Board of the Wuhan Union Medical College Hospital reviewed and approved this study protocol. All patients provided written informed consent, and all procedures performed in studies involving human participants were in accordance with the Helsinki Declaration.
Data Collection
Fourteen variables were extracted in this study, including age, gender, tumor location, differentiation, histological type, tumor size, TNM stage, T stage, N stage, M stage, radiotherapy, chemotherapy, number of sample lymph nodes, number of positive lymph nodes, primary surgery, and metastatic surgery. All clinicopathological factors were classified according to the seventh edition of the American Joint Committee on Cancer staging system. The histological type of CRC patients was identified by the International Classification of Diseases for Oncology, 3rd edition (ICD-O-3). According to ICD-O-3 oncology codes, three histological subtypes of CRCs were classified as follows: adenocarcinoma (8010, 8020, 8140–8144, 8210, 8211, 8255, 8260–8263, 8310, 8440, 8460, 8550, 8560), mucinous adenocarcinoma (8470–8472, 8480, 8481), and signet ring cell adenocarcinoma (8490). Patient survival information was measured by CSS and OS. Finally, patients from the SEER database were divided into training and internal validation sets for model building and evaluation. Eligible patients from the WUHCC cohort were used as the external validation cohort for model validation.
Construction and Validation of the Nomogram
Univariable and multivariable Cox regression analyses were used to calculate the effect of variables on CSS and OS in the training, internal validation, and external validation cohorts. The measure of the effect of each variable on CSS and OS is presented as the hazard ratio (HR) and was used to identify independent risk factors. The variables with P <0.05 in the univariate model were used in the multivariate logistic regression analysis. Based on the multivariable Cox regression analysis results, a nomogram integrating clinicopathological parameters was formulated. The overall points for each patient in the training, internal validation, and external validation cohorts were calculated using the established nomogram, after which a Cox regression analysis of the entire cohort was carried out using the overall points as a parameter.
The Calibration Curve and Area Under the Receiver Operating Characteristic Curve
The calibration of the nomogram was evaluated by the Hosmer–Lemeshow test and displayed in the form of the calibration curve. The accuracy of the predictive ability of the nomogram over time is displayed in the form of 1-, 3-, and 5-year ROC curve, and the discriminative ability of the nomogram to predict OS and CSS in CRCLM is quantitatively expressed by the AUROC.
The Concordance Index and Decision Curve Analysis
The concordance index (C-index) was defined as the ratio of all patient pairs predicted to be consistent with the results. Decision curve analysis (DCA) was recently proposed as a fresh method to visualize the potential clinical value of a risk prediction model. Thus, the DCA method was carried out to compare the clinical consequences of the predictive nomogram in the current research.
Statistical Analysis
The categorical variables were expressed as number and percentage, and the chi-square test or Fisher’s exact test was used for comparison where appropriate. Univariable and multivariable Cox regression analyses were used to calculate the effect of variables on CSS and OS. The effect of each variable was evaluated by HR. Stepwise selection was applied to filter variables in the multivariable Cox regression model. The selection criteria were as follows: significant level for entry is 0.05 and the significant level for stay is 0.05. Statistical analysis was conducted with SAS 9.4 (SAS Institute Inc., Cary, NC, USA) and R3.6.2 (R Foundation for Statistical Computing, Vienna, Austria). The R statistical packages “rms”, “survival”, “Hmisc”, “MASS”, and “timeROC” were used to build a nomogram, plot calibration, and time-dependent ROC curves, while “rmda” was used to draw the DCA curves. All statistical tests were two-sided, with statistical significance set at 0.05.
Results
Clinicopathological Characteristics and Prognosis of Patients
A total of 9,332 patients from the SEER dataset and 404 patients from the WUHCC cohort with CRCLM between 2010 and 2016 were included in our study. In the SEER database, 60% (n = 5,597) of the patients were randomly assigned to the training cohorts, while the remaining patients (n = 3,735) were included in the internal validation cohorts. Patients (n = 404) from the WUHCC cohort were included in the external validation cohort, and the detailed flow diagram of the patient inclusion and exclusion criteria process is shown in Figure 1.
The mortality was 69.9% (41.8% for TVA stage and 26.3% for TVB stage) and 61.6% (38.4% for TVA stage and 21.0% for TVB stage) for all CRCLM patients in the SEER and WUHCC cohorts, respectively. Most patients were older than 60 years (5,313; 56.9%) and male (5,123; 54.9%) in the SEER cohorts and (222; 54.9%; 219; 54.2%) in the WUHCC cohorts, respectively. In both the SEER and WUHCC cohorts, compared with alive patients, dead patients were more likely to have poor tumor differentiation, larger tumor diameter, poor tumor stage, higher rates of lymph node metastasis, tumors localized more commonly in the colon, and metastatic surgery. Moreover, a total of 8.5% and 11.6% of patients underwent adjuvant radiotherapy in the SEER and WUHCC cohorts, respectively. A total of 72.2% and 74.25% of patients underwent adjuvant chemotherapy in the SEER and WUHCC cohorts, respectively (Table 1).
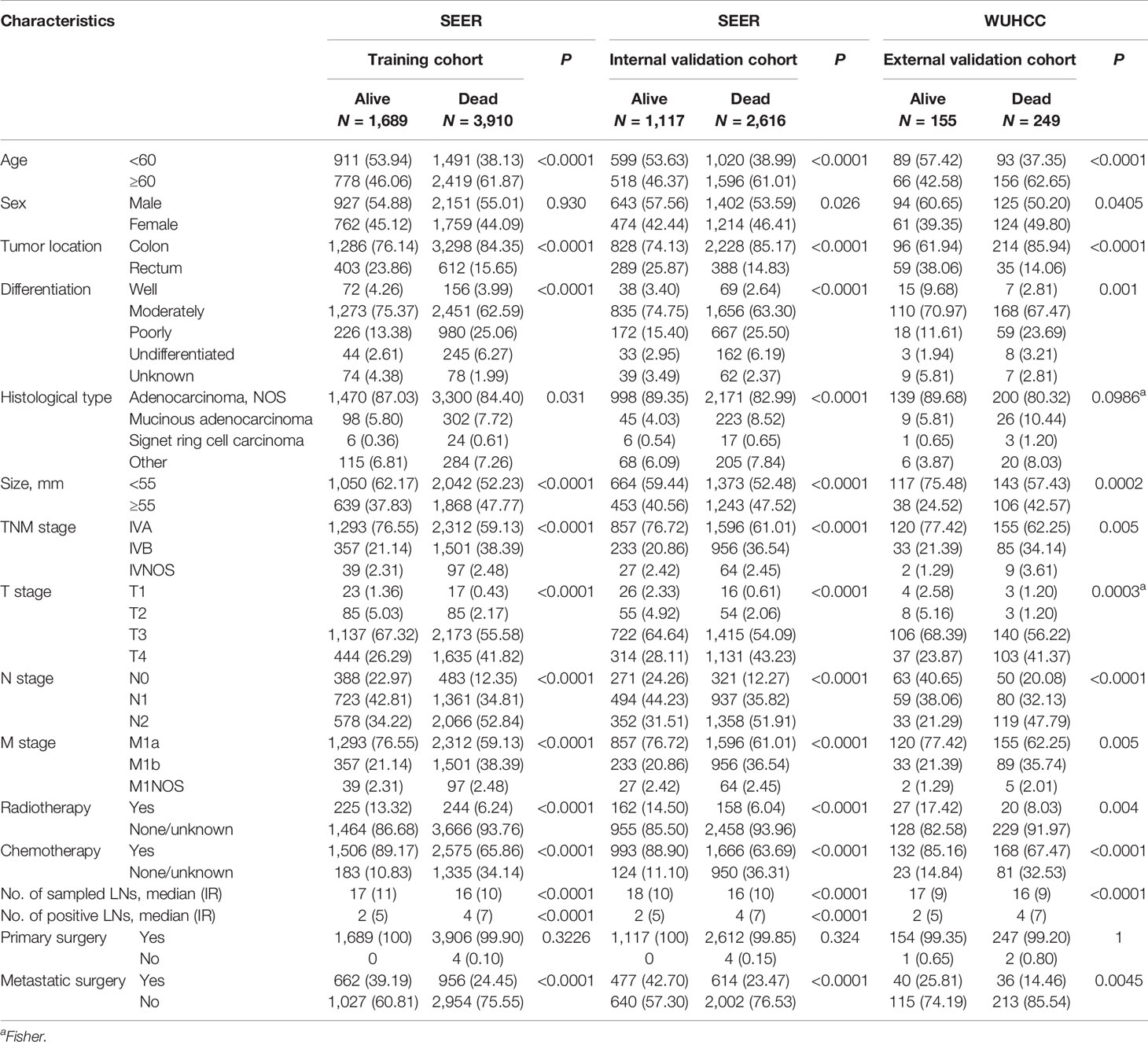
Table 1 Clinicopathological characteristics of patients in the training, internal validation, and external validation cohorts [N (%)].
Independent Predictive Features in Patients With Colorectal Liver Metastasis
According to the results based on the univariate Cox regression analysis in the training cohort, 13 factors, namely, age, tumor location, differentiation, histological type, tumor size, TNM stage, T stage, N stage, M stage, radiotherapy, chemotherapy, number of sample lymph nodes, and number of positive lymph nodes, were associated with CSS and OS (Tables 2, 3). In the multivariate Cox regression analysis, 10 parameters, including age, tumor location, differentiation, histological type, tumor size, TNM stage, chemotherapy, number of sample lymph nodes, number of positive lymph nodes, tumor size, and metastatic surgery, were independent predictors predicting the OS and CSS for patients with CRCLM (Tables 4, 5).
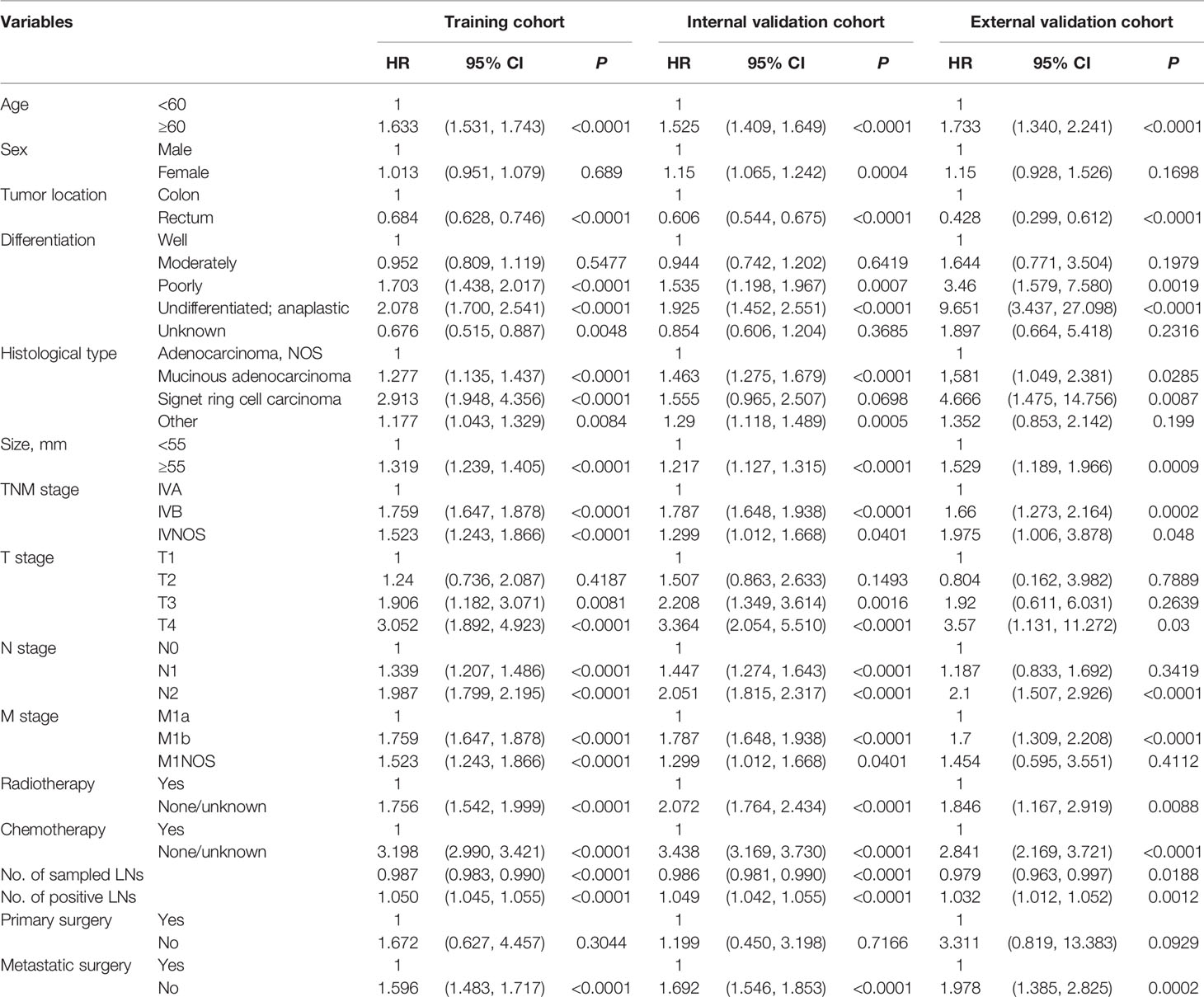
Table 2 Univariate Cox regression model in the training, internal validation, and external validation cohorts of OS.
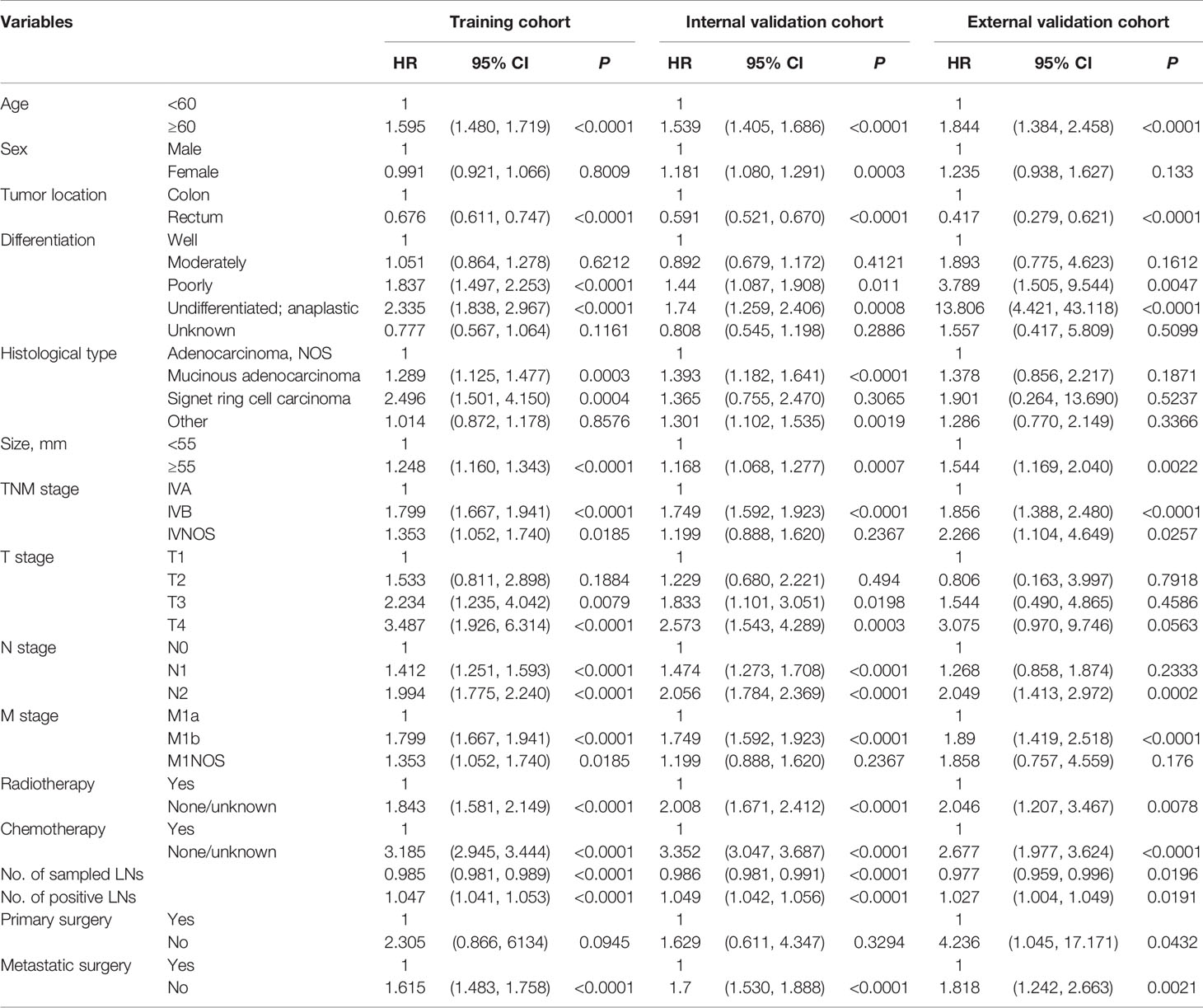
Table 3 Univariate Cox regression model in the training, internal validation, and external validation cohorts of CSS.
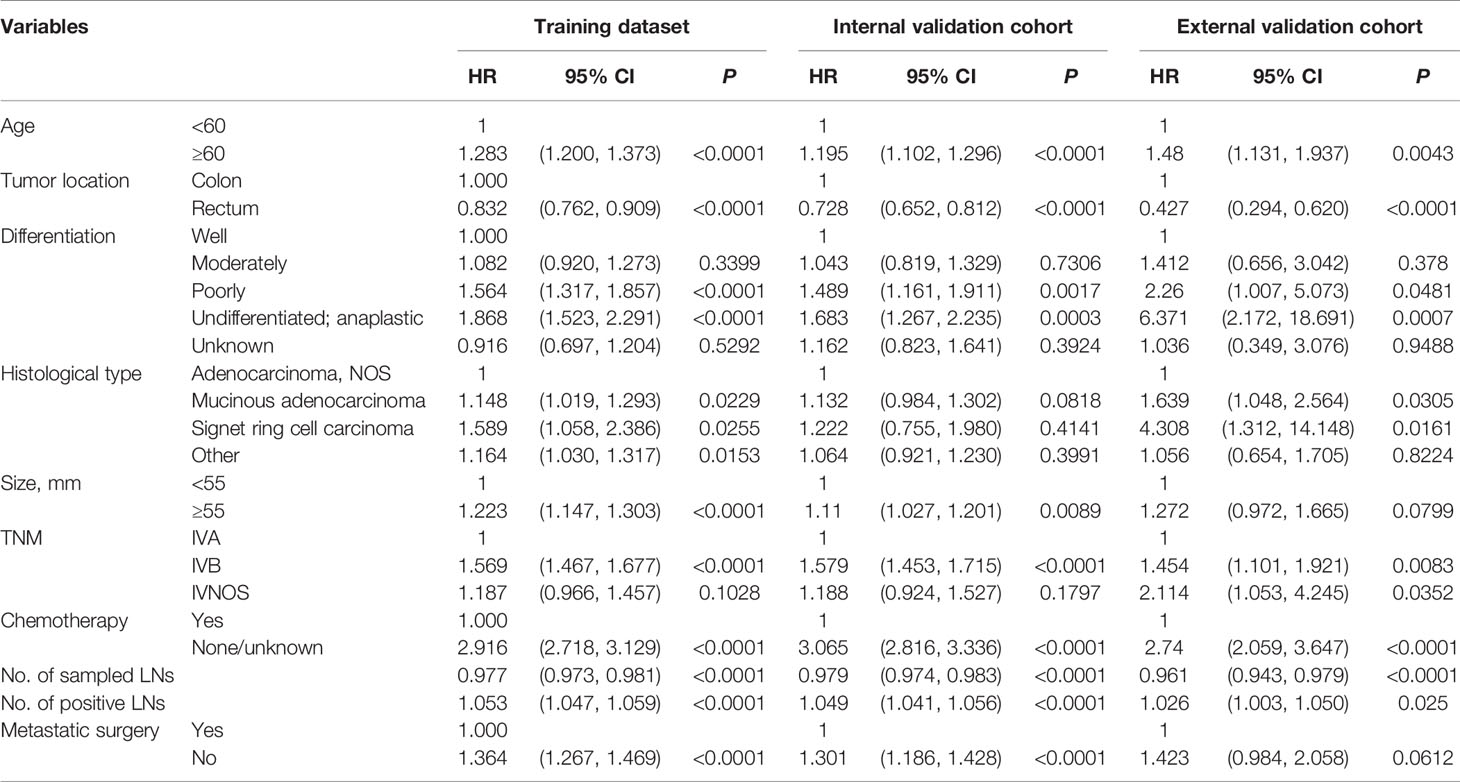
Table 4 Multivariable cox regression model in the training, internal validation, and external validation cohorts of OS.
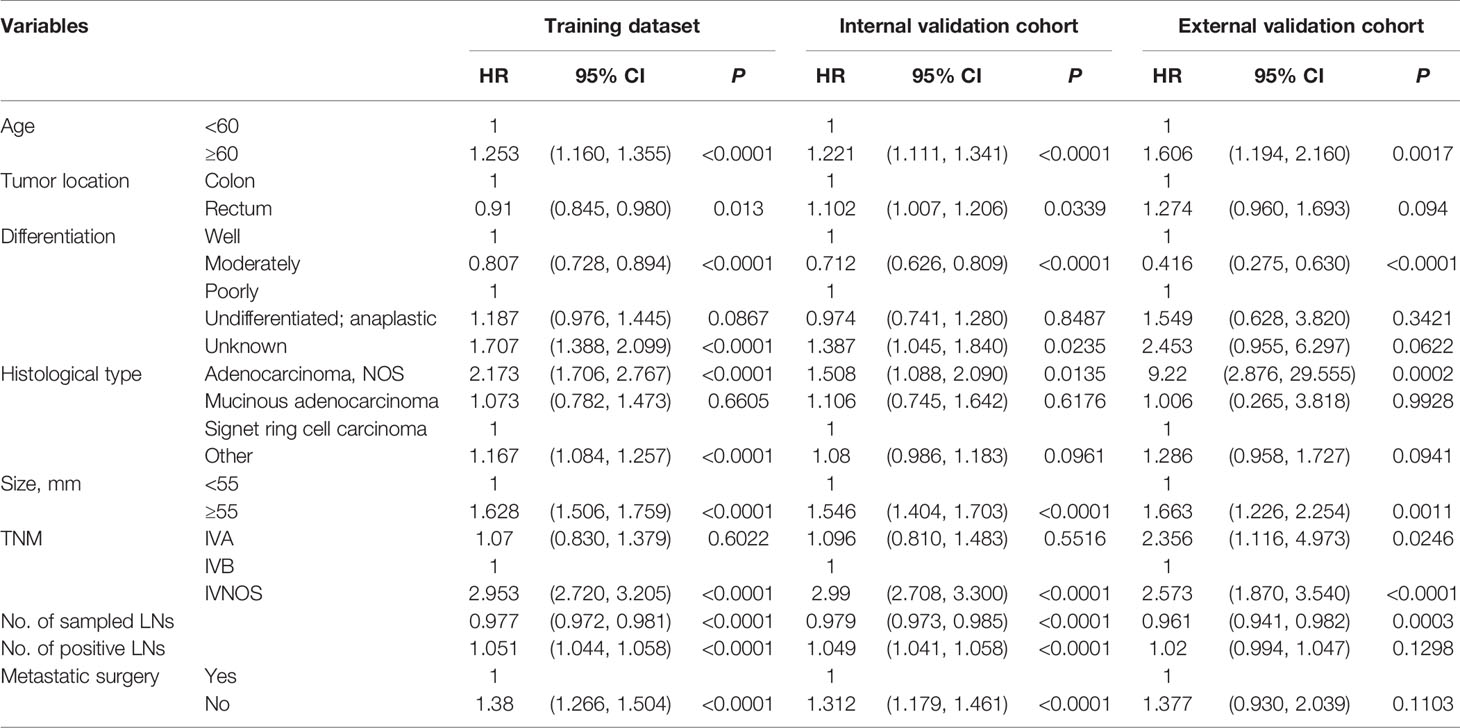
Table 5 Multivariable Cox regression model in the training, internal validation, and external validation cohorts of CSS.
Construction of the Nomogram
Multivariable Cox regression analysis was used to identify the significant features associated with survival status, based on which the nomogram for OS and CSS was developed. The risk score associated with each variable and projection can be obtained by the top ruler, and the probabilities for 1-, 3-, and 5-year CSS and OS can be estimated by superposing the risk score of each variable to the bottom ruler (Figures 2A, B).
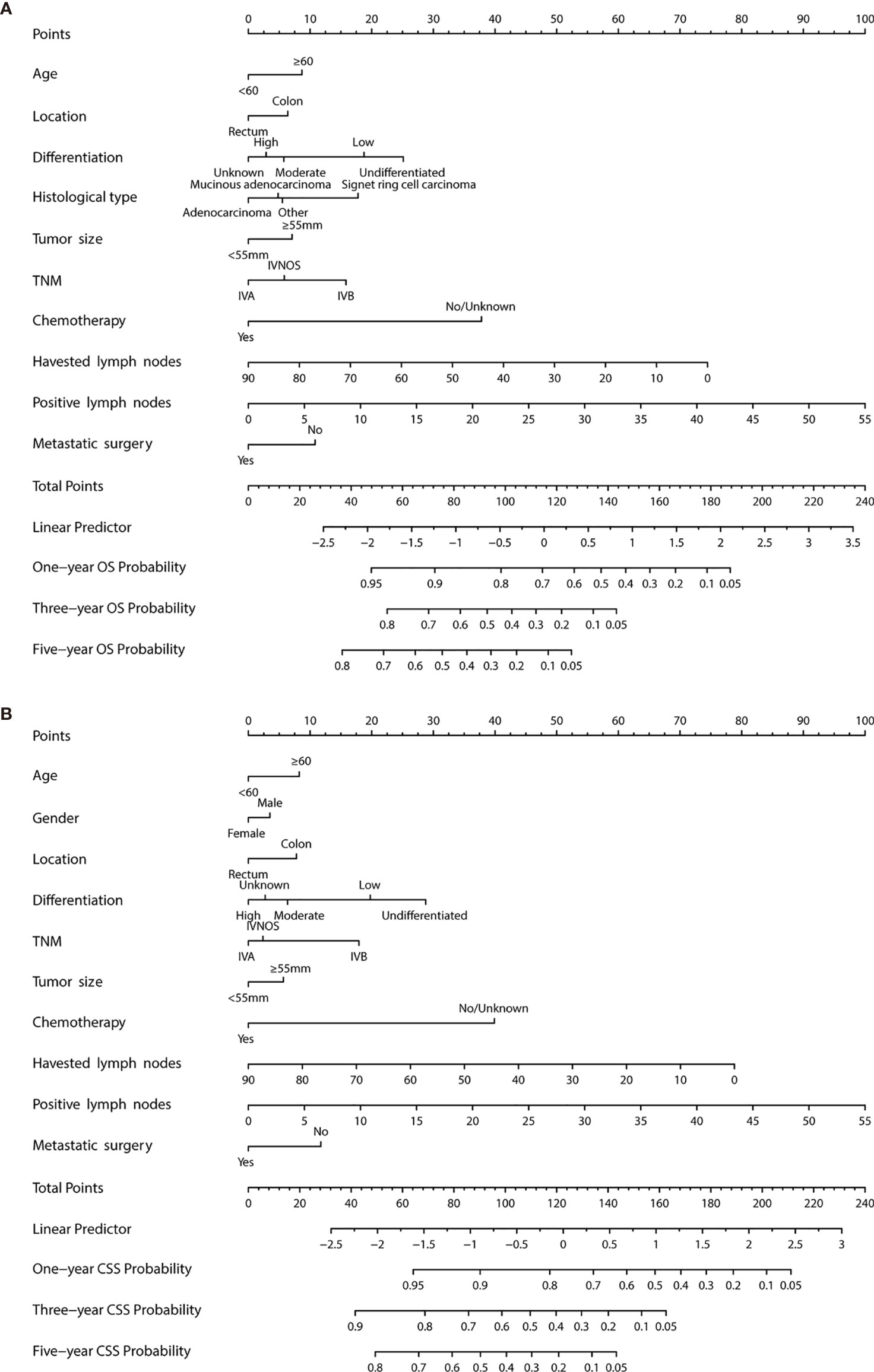
Figure 2 Evaluation of overall survival (OS) and cancer-specific survival (CSS)-associated nomograms for patients with colorectal liver metastasis (CRCLM). (A) OS nomogram integrating age, tumor location, differentiation, gender, TNM stage, chemotherapy, no. of sample LNs, no. of positive LNs, tumor size, and metastatic surgery for predicting 1-, 3-, and 5-year OS rates. (B) CSS nomogram integrating age, tumor location, differentiation, gender, TNM stage, chemotherapy, no. of sample LNs, no. of positive LNs, tumor size, and metastatic surgery for predicting 1-, 3-, and 5-year CSS rates.
Comparisons of the Nomogram and TNM Stage
To confirm that the nomogram had higher efficacy in predicting the prognosis of patients with CRCLM than TNM stage, time-dependent ROC analyses at 1, 3, and 5 years were conducted. The 1-, 3-, and 5-year AUC values of the nomogram for the prediction of CSS were 0.825, 0.771, and 0.772 in the training cohorts, respectively, compared with 0.584, 0.604, and 0.622, respectively, for the AUC values of TNM stage. The 1-, 3-, and 5-year AUC values of the nomogram for the prediction of CSS were 0.828, 0.753, and 0.758 in the internal validation cohorts, compared with 0.580, 0.590, and 0.611, respectively, for the AUC values of TNM stage. The 1-, 3-, and 5-year AUC values of the nomogram for the prediction of CSS were 0.828, 0.737, and 0.772 in the external validation cohorts, compared with 0.615, 0.595, and 0.594, respectively, for the AUC values of TNM stage (Figures 3A–I).
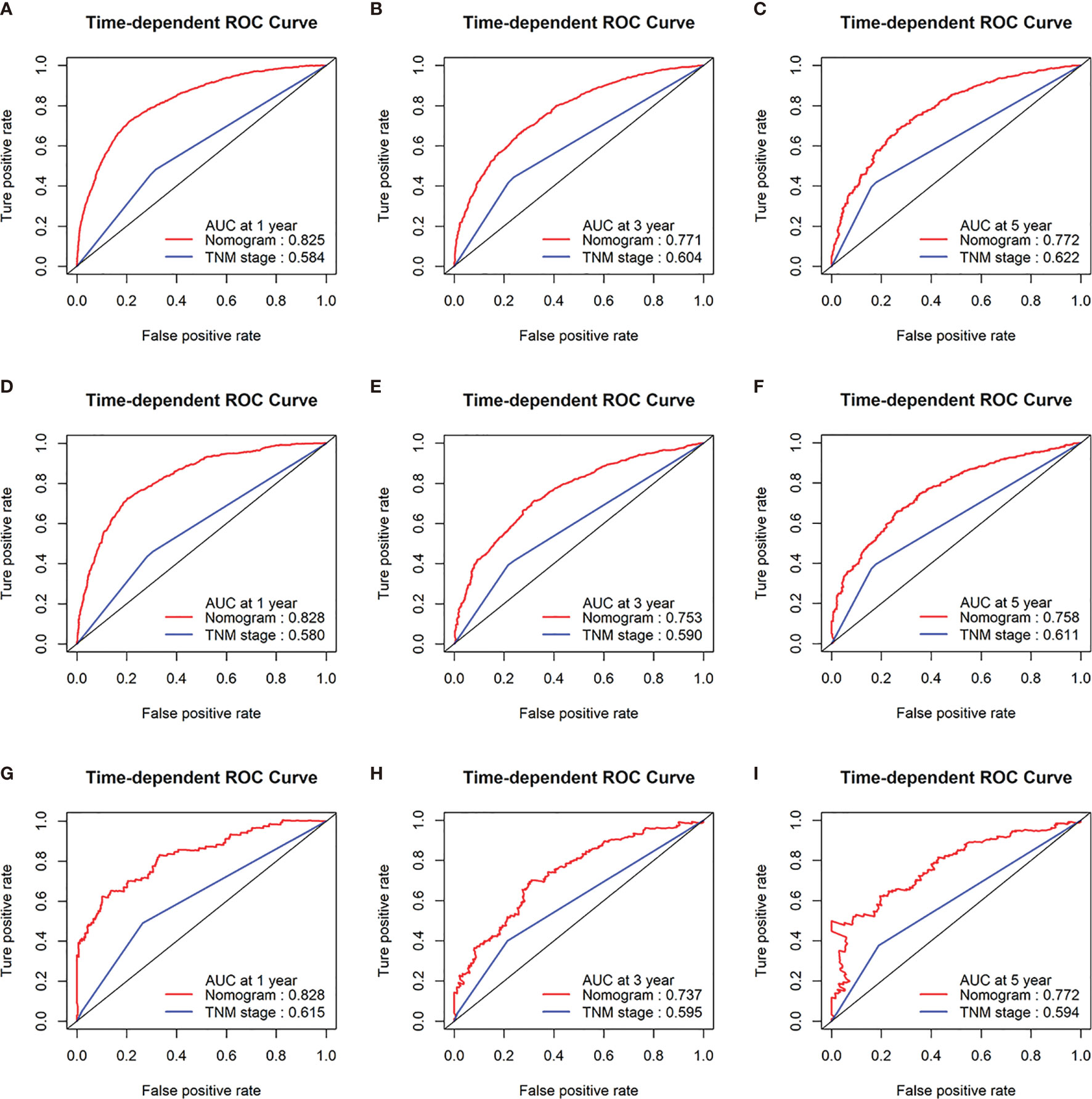
Figure 3 Receiver operating characteristic (ROC) curves of TNM stage and the nomogram of the CSS. AUC values of ROC predicted 1-, 3-, and 5-year CSS rates of the nomogram and TNM stage in the training cohorts (A–C); AUC values of ROC predicted 1-, 3-, and 5-year CSS rates of the nomogram and TNM stage in the internal validation cohorts (D–F); AUC values of ROC predicted 1-, 3-, and 5-year CSS rates of the nomogram and TNM stage in the external validation cohorts (G–I).
In addition, the 1-, 3-, and 5-year AUC values of the nomogram for the prediction of OS were 0.816, 0.782, and 0.787 in the training cohorts, respectively, compared with 0.584, 0.627, and 0.608, respectively, for the AUC values of TNM stage. The 1-, 3-, and 5-year AUC values of the nomogram for the prediction of OS were 0.827, 0.769, and 0.774 in the internal validation cohorts, compared with 0.594, 0.597, and 0.621, respectively, for the AUC values of TNM stage. The 1-, 3-, and 5-year AUC values of the nomogram for the prediction of OS were 0.819, 0.745, and 0.767 in the external validation cohorts, compared with 0.598, 0.576, and 0.574, respectively, for the AUC values of TNM stage (Figures 4A–I).
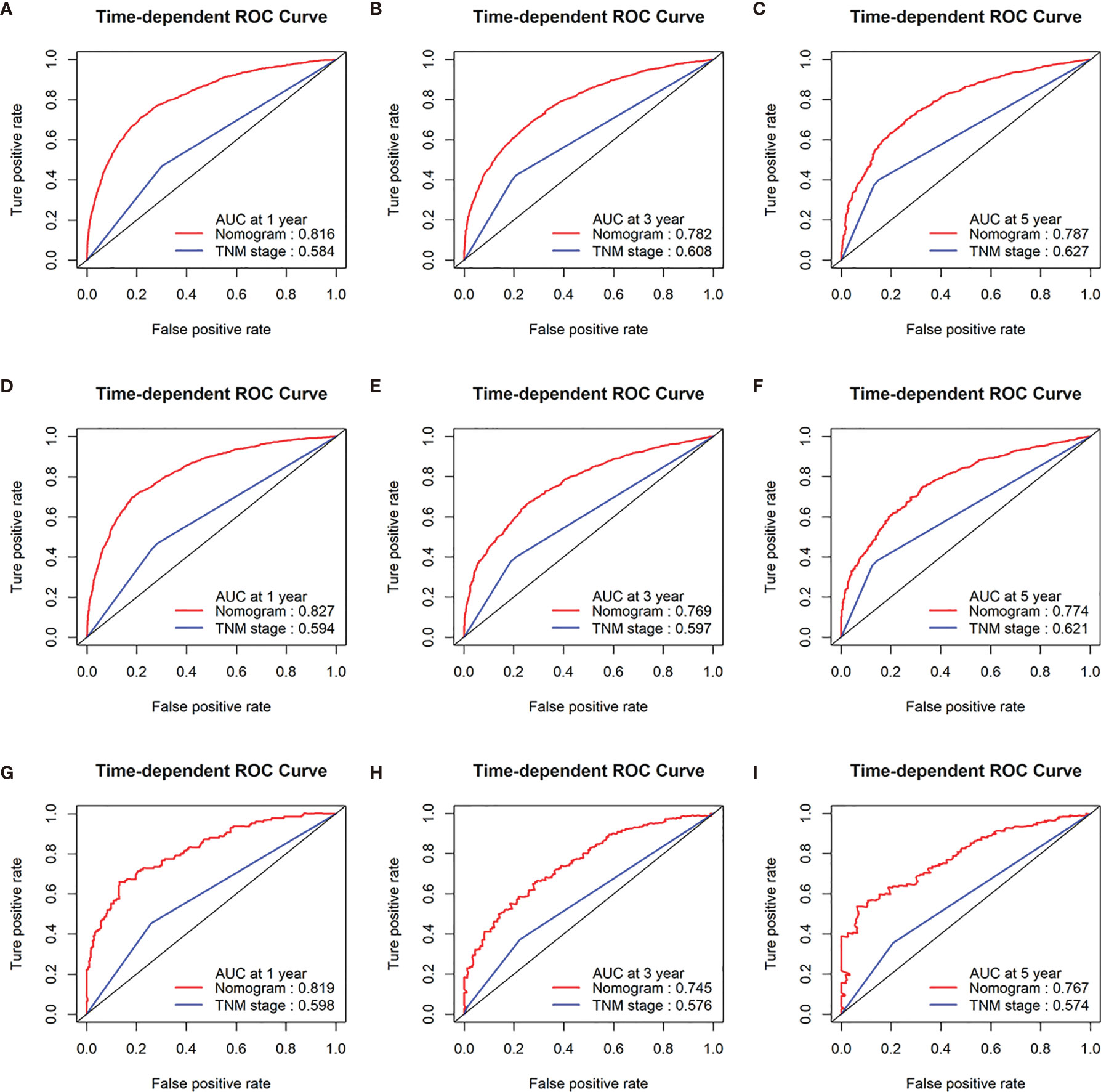
Figure 4 Receiver operating characteristic (ROC) curves of TNM stage and the nomogram of the OS. AUC values of ROC predicted 1-, 3-, and 5-year OS rates of the nomogram and TNM stage in the training cohorts (A–C); AUC values of ROC predicted 1-, 3-, and 5-year OS rates of the nomogram and TNM stage in the internal validation cohorts (D–F); AUC values of ROC predicted 1-, 3-, and 5-year OS rates of the nomogram and TNM stage in the external validation cohorts (G–I).
Evaluation and External Validation of the OS and CSS Prediction Nomogram
The discrimination ability of the nomogram was represented by the ROC curve. The nomograms showed favorable sensitivity at predicting 1-, 3-, and 5-year OS, with the AUROC values of 0.816, 0.782, and 0.787 in the training cohort, respectively; 0.827, 0.769, and 0.774 in the internal validation cohort, respectively; and 0.819, 0.745, and 0.767 in the external validation cohort, respectively. For CSS, the 1-, 3-, and 5-year predictive power of survival nomograms measured by AUC in the training cohort was 0.825, 0.771, and 0.772, respectively; in the internal validation cohort, it was 0.828, 0.753, and 0.758, respectively; and in the external validation cohort, it was 0.828, 0.737, and 0.772 (Figure 5).
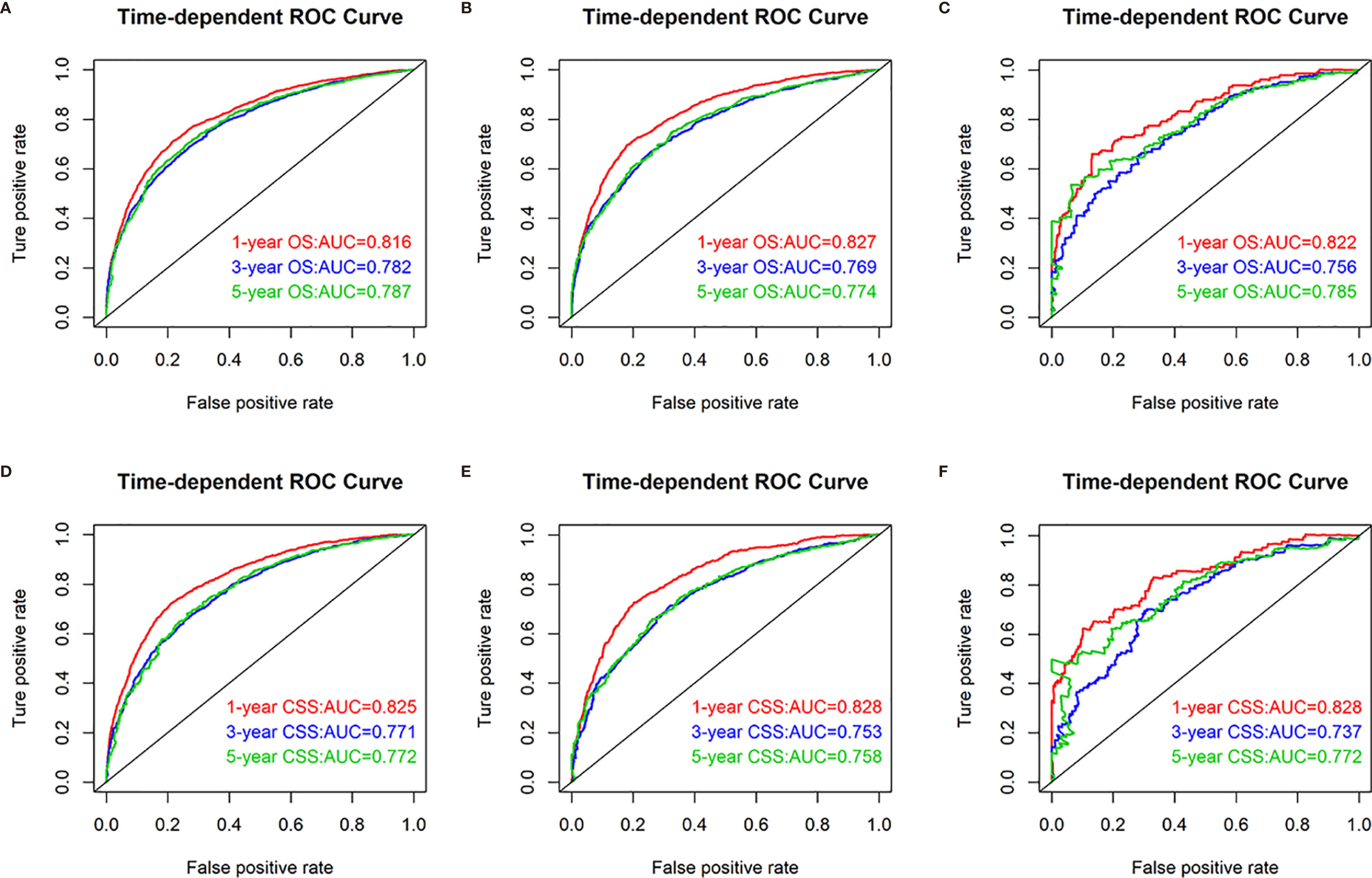
Figure 5 Nomograms of time-dependent receiver operating characteristic (ROC) curves associated with overall survival (OS) and cancer-specific survival (CSS). (A–C) Represents AUC values of ROC predicted 1-, 3-, and 5-year OS rates of the nomogram for the training, internal validation, and external validation cohorts; (D–F) represents AUC values of ROC predicted 1-, 3-, and 5-year CSS rates of the nomogram for the training, internal validation, and external validation cohorts.
C-index values and ROC curves are ordinarily used to evaluate the discriminatory power of a nomogram. The C-indexes for the prediction of CSS were 0.74 (95% CI 0.730, 0.750), 0.746 (95% CI 0.734, 0.758), and 0.755 (95% CI 0.718, 0.792) in the training, internal validation, and external validation cohorts, respectively. In addition, the C-indexes for the prediction of OS were 0.743 (95% CI 0.735, 0.751), 0.748 (95% CI 0.738, 0.758), and 0.756 (95% CI 0.723, 0.789) in the training, internal validation, and external validation cohorts, indicating that the nomogram had favorable discrimination in patients with CRCLM.
In addition, calibration curves for the nomogram showed no deviations from the reference line, indicating a high degree of credibility (Figures 6, 7).
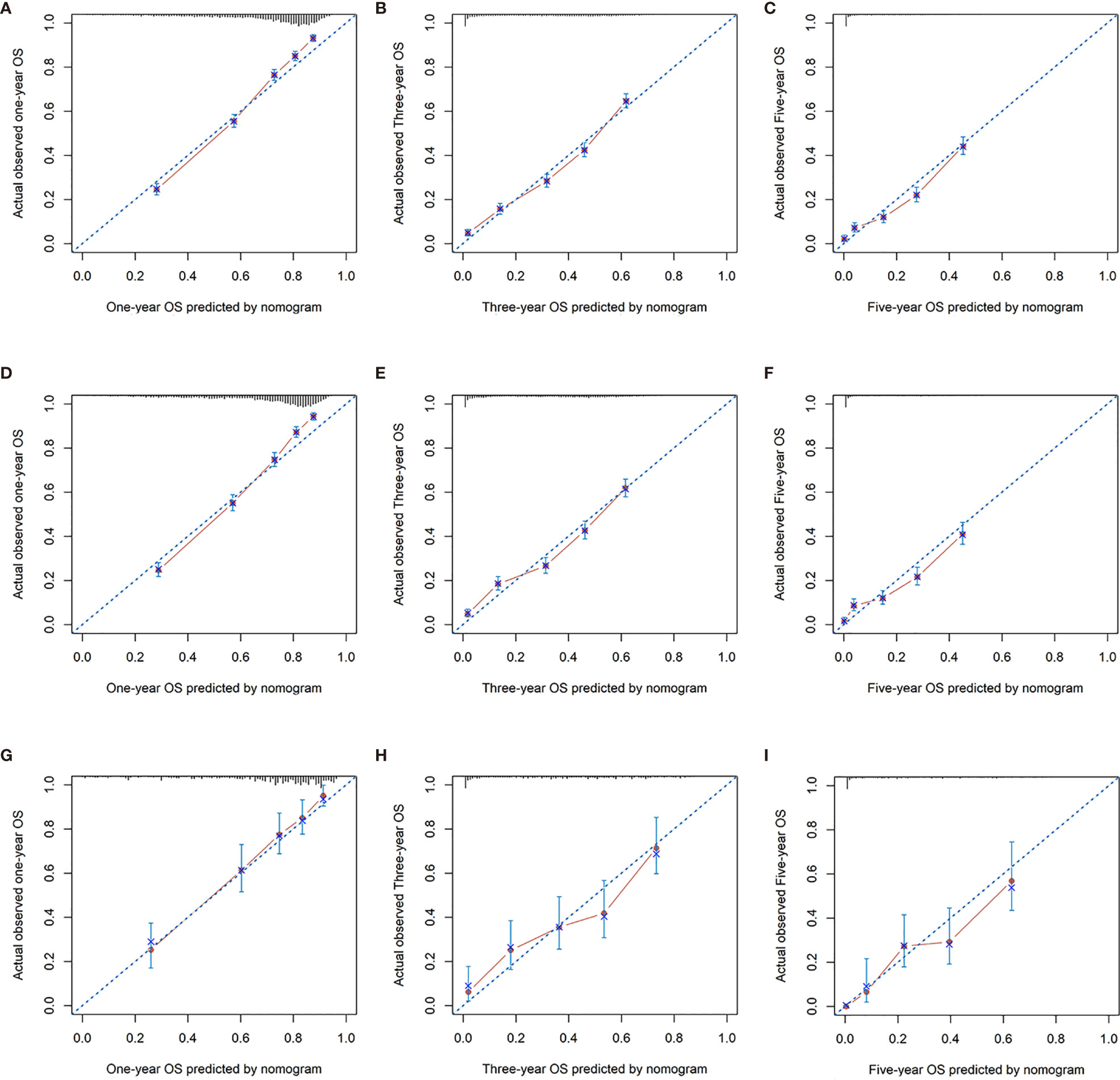
Figure 6 Calibration curves for 1-, 3-, and 5-year OS rate of nomogram predictions. (A–C) Represents the calibration curve for predicting the OS of patients at 1, 3, and 5 years in the training cohorts; (D–F) represents the calibration curve for predicting the OS of patients at 1, 3, and 5 years in the internal validation cohorts; (G–I) represents the calibration curve for predicting the OS of patients at 1, 3, and 5 years in the external validation cohorts.
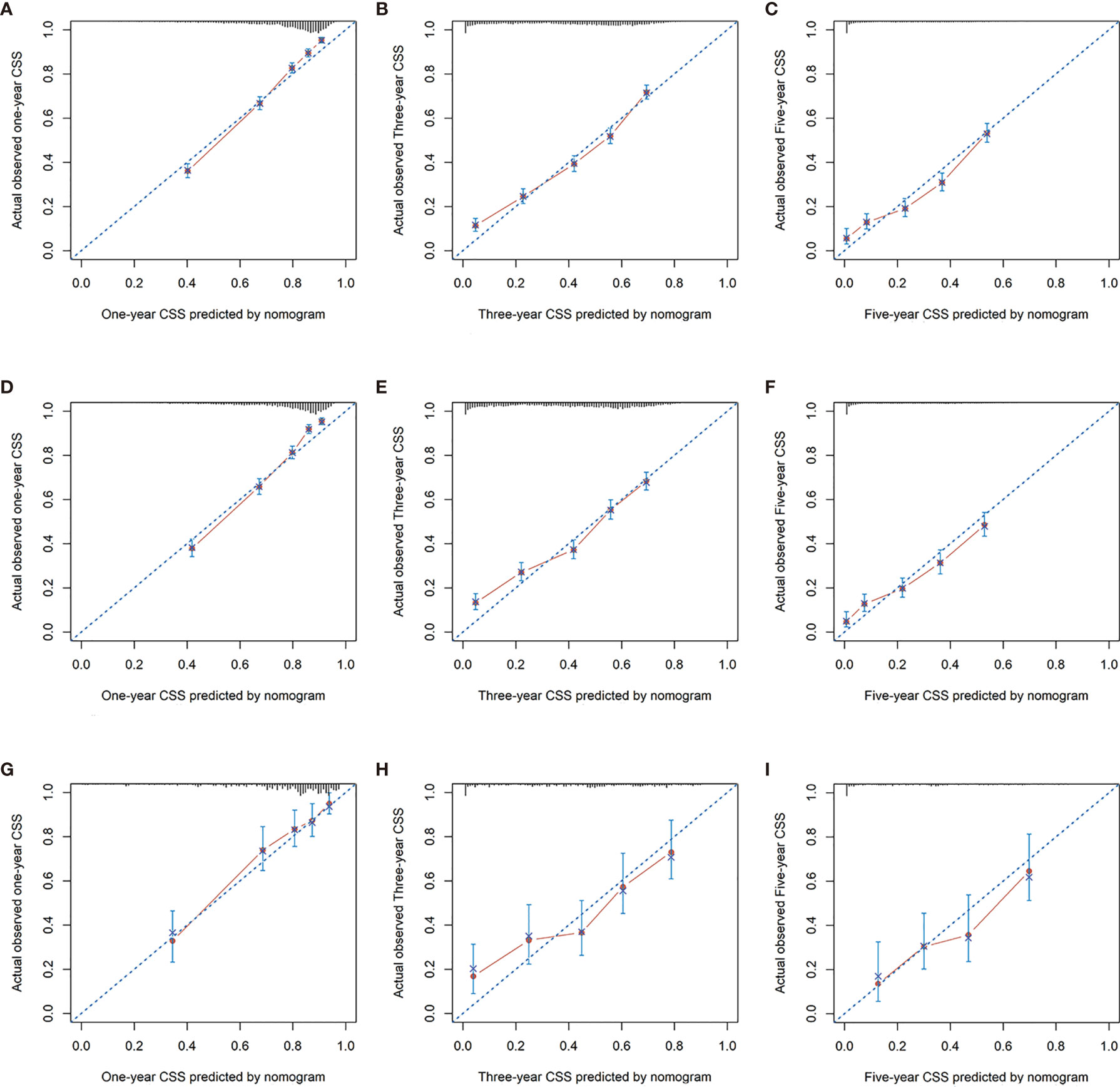
Figure 7 Calibration curves for 1-, 3-, and 5-year CSS rate of nomogram predictions. (A–C) Represents the calibration curve for predicting the CSS of patients at 1, 3, and 5 years in the training cohorts; (D–F) represents the calibration curve for predicting the CSS of patients at 1, 3, and 5 yeara in the internal validation cohorts; (G–I) Represents the calibration curve for predicting the CSS of patients at 1, 3, and 5 years in the external validation cohorts.
Clinical Value of the Nomogram
DCA is a novel strategy for evaluating alternative predictive treatment methods and has advantages over AUROC in clinical value evaluation. The DCA curves for the developed nomogram and TNM stage in the training, internal validation, and external validation cohorts are presented in Figure 8. Compared with the TNM staging system, the DCA of the nomogram has higher net benefits, indicating that it had better clinical outcome values than TNM stage.
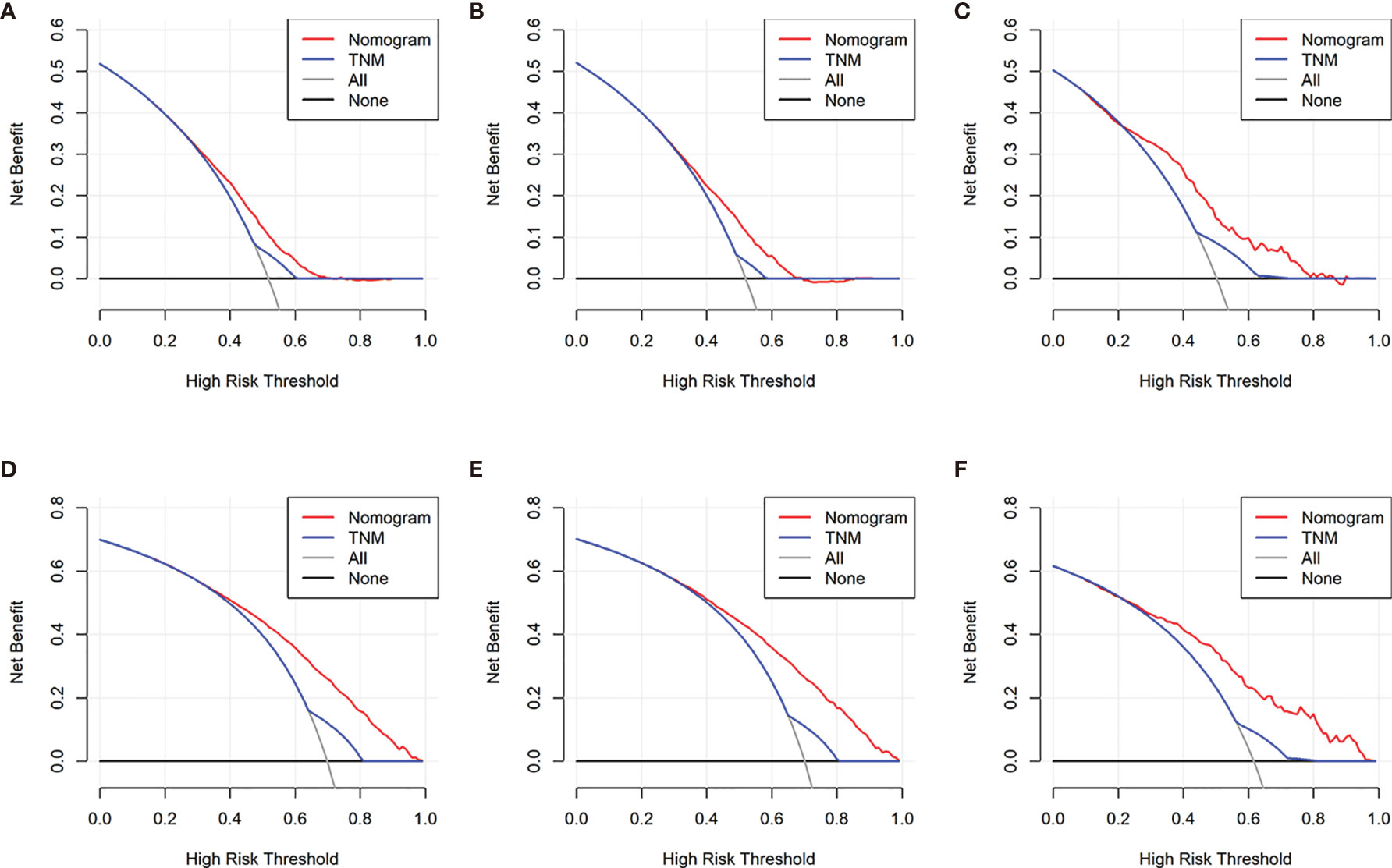
Figure 8 Decision curve analysis of the nomogram and TNM stage for the cancer-specific survival (CSS) and overall survival (OS) prediction of patients with colorectal liver metastasis (CRCLM). (A) Training, (B) internal validation, and (C) external validation cohorts for the CSS; (D) training, (E) internal validation, and (F) external validation cohorts for the OS.
Discussion
Comprehensive evaluation of the clinicopathological characteristics of patients with CRCLM for developing a corresponding prognosis model is becoming more and more important in terms of prognosis prediction for patients. In this study, a nomogram merging the clinicopathological parameters with the TNM staging system was built to assess the definite 1-, 3-, and 5-year CSS and OS probabilities of CRCLM patients. The characteristics of the nomogram were confirmed by identifying the parameters and it was calibrated, which can contribute to a wide range of applications. From the perspective of ROC curve analysis and detrended correspondence analysis (DCA), the nomogram showed better predictive accuracy and prognostic value compared with the current TNM staging system.
The effect of primary site of CRC on prognosis after CLCLM is still largely unknown. In this study, we found that primary tumor site is closely related to prognosis of patients with CRCLM. In both the training cohort and the internal and external validation cohorts, patients with colorectal primaries had poorer outcomes in OS and CSS than patients with rectal primaries (P < 0.0001). The location of primary lesions in the colon was also an independent prognostic factor for patients with CRCLM, similar to previous studies (10). In a retrospective study, it was found that the recurrence-free survival of patients with left-sided primary tumors was shorter than that of patients with right-sided tumors, and the OS of patients with right colorectal cancer after recurrence was shorter (P = 0.01) (10). A study determining the influence on CRCLM after portal vein embolization and right hepatic resection and evaluating progression-free survival and prognostic factors for OS found that the location of the primary colorectal cancer was a statistically significant predictor, and right-sided colorectal cancers had a significantly shorter progression-free survival than left-sided colorectal cancers. The presence of extrahepatic disease was associated with poor OS (11). However, a meta-analysis found that the 5-year OS rate of patients with right-sided primary colorectal cancer with liver metastasis after hepatectomy was worse than that of the left-sided primaries (12). Further studies are needed to determine the prognostic impact of the location of the primary tumor in patients with CRCLM.
Lymph node metastasis, total number of lymph nodes obtained, and the number of positive lymph nodes are of prognostic significance in gastrointestinal malignancies. In gastric cancer, the number of acquired lymph nodes less than 16 is an independent adverse prognostic factor (P < 0.0001, HR 2.48; 95% CI 1.60–3.70), and the number of acquired lymph nodes may affect the prognosis and staging migration of stage II and stage III gastric cancer patients (13). In a prognostic study of patients with colorectal cancer, the greater the ratio of positive lymph nodes to examined lymph nodes (LNR), the worse the 5-year OS of the patients was (P < 0.001), and LNR was shown to be a powerful prognostic factor in colorectal cancer (14). TNM stage, differentiation degree, and lymph node status of the primary tumor are closely related to the prognosis of patients with CRCLM. Studies have shown that patients with resected liver metastasis from colorectal cancer with local lymph node metastasis have a poor survival compared with patients with negative lymph nodes, but a small number of patients with affected lymph nodes can achieve long-term survival (15). The presence of primary lymph node metastasis is associated with prognosis, possibly because the presence of primary lymph node metastasis is a significant risk factor for perihepatic lymph node metastases, while perihepatic lymph node metastases are a negative prognostic factor (16). Zong et al. found that Musashi 2 overexpression, lymph node metastasis, distant metastasis, and TNM stage were associated with liver metastasis of colorectal cancer. Musashi 2 overexpression is associated with poor prognosis and may be a potential biomarker for liver metastasis of colorectal cancer patients (17). In this study, we obtained similar results. Lymph node metastasis and the number of lymph node metastasis have important prognostic significance in patients with CRCLM. In addition, our study also found that several clinicopathological features were closely related to the prognosis of patients with CRCLM, and established the corresponding prognosis model, which could accurately predict their prognosis.
At present, the prognosis prediction models of CRCLM are mainly studied with respect to histopathology and clinicopathology. Liang et al. calculated the immune score based on immunostaining CD3+ and CD8+ cell density and established a predictive model combined with histopathological growth pattern (HGPS) and clinical risk score (CRS), which could be used to stratify the survival of CRCLM patients, but this study lacked internal and external validation (18). Wang et al. established an immune score by detecting the number and density of CD3+ and CD8+ T cells in the tumor center and peritumoral area and predicted the prognosis of recurrence-free survival and OS of CRCLM patients, which was confirmed by internal validation. The results of this study showed that liver metastasis immune score can be used to predict the prognosis of CRCLM patients after hepatectomy (19). Studies have used neutrophil–lymphocyte ratio (NLR), derived NLR, platelet–lymphocyte ratio (PLR), and lymphocyte–monocyte ratio (LMR) inflammation score in resectable CRCLM as prognostic tools. The study found that patients with high preoperative NLR and PLR were independent prognostic factors for CRCLM patients and its prognostic value was superior to other systemic inflammation scores based on cells, but there was no further validation (20, 21). Previous studies used the clinical risk score (CRSS) of Fong and Nordlinger to predict the prognosis of patients with CRCLM (22, 23), but some studies found that CRSS was not a reliable prognostic tool for patients who received neoadjuvant chemotherapy before hepatectomy. Current prognostic models for CRCLM have some deficiencies, mainly due to the limitation of sample size and secondly due to the fact that the indicators are not good enough for accurate prediction. In this study, we analyzed common clinicopathological characteristics, individually and in combination, to establish a convenient, fast, and accurate prediction model. The calculation chart of the calibration curve shows a high degree of credibility.
Our research has some limitations: First, treatment information except for surgery was not available in the SEER database and could not be incorporated into the analysis. Second, in addition to the primary tumor characteristics, tumor location was also related to the location and number of metastatic liver tumors. Because such data were missing in the validation cohort, we could not further analyze the location and number of metastatic tumors in the liver. Third, this was a retrospective study based on limited clinical records, and further prospective multicenter clinical studies are needed to demonstrate the clinical validity of the model. However, this study also has significant advantages. We used routinely available clinicopathological data to establish a convenient, rapid, and accurate prediction model; secondly, this study is a retrospective analysis of a large sample, and internal and external validation queues were used to verify the prediction model to ensure reliability. A relatively large retrospective cohort analysis (large sample size) and a broad meta-analysis of various factors that may be associated with colorectal cancer OS and CSS should provide an important reference point for clinicians.
In conclusion, we established and validated a nomogram based on significant clinicopathological characteristics for predicting CSS and OS in CRCLM patients. The novel nomogram has sufficient discriminatory and calibration capability in addition to exceptional clinical effectiveness and could be an easy-to-use tool for clinicians to promote a personalized postoperative prognostic assessment and further identify treatment strategies for CRCLM patients.
Data Availability Statement
The original contributions presented in the study are included in the article/Supplementary Material. Further inquiries can be directed to the corresponding authors.
Ethics Statement
The studies involving human participants were reviewed and approved by the Ethical Committee and Institutional Review Board of Wuhan Union Medical College. The patients/participants provided their written informed consent to participate in this study. Written informed consent was obtained from the individual(s) for the publication of any potentially identifiable images or data included in this article.
Author Contributions
KW, NZ, and KC conceived and designed the study. YC, SK, SD, LY, JG, FM, YX, CZ, WC, HLL, HL, FS, and ZS collected and analyzed the data. YC, SK, and SD wrote the paper. KW, NZ, and KC reviewed and edited the manuscript. All authors contributed to the article and approved the submitted version.
Funding
This study was supported by grants from the Wuhan High Magnetic Field Interdisciplinary Fund (WHMFC202113) and Free Innovation Pre-Research Fund and Platform Scientific Research Fund in 2019 (02.03.2019-111) and 2020 (F016.02004.20001.023).
Conflict of Interest
The authors declare that the research was conducted in the absence of any commercial or financial relationships that could be construed as a potential conflict of interest.
Publisher’s Note
All claims expressed in this article are solely those of the authors and do not necessarily represent those of their affiliated organizations, or those of the publisher, the editors and the reviewers. Any product that may be evaluated in this article, or claim that may be made by its manufacturer, is not guaranteed or endorsed by the publisher.
Supplementary Material
The Supplementary Material for this article can be found online at: https://www.frontiersin.org/articles/10.3389/fonc.2021.719638/full#supplementary-material
Supplementary Figure 1 | The metastatic surgery indicators were statistically associated with OS and CSS in CRCLM. (A, B) The CSS in SEER data and in external validation cohorts; (C, D) The OS in SEER data and in external validation cohorts.
References
1. Miller KD, Nogueira L, Mariotto AB, Rowland JH, Yabroff KR, Alfano CM, et al. Cancer Treatment and Survivorship Statistics, 2019. CA Cancer J Clin (2019) 69(5):363–85. doi: 10.3322/caac.21565
2. Hackl C, Neumann P, Gerken M, Loss M, Klinkhammer-Schalke M, Schlitt HJ. Treatment of Colorectal Liver Metastases in Germany: A Ten-Year Population-Based Analysis of 5772 Cases of Primary Colorectal Adenocarcinoma. BMC Cancer (2014) 14:810. doi: 10.1186/1471-2407-14-810
3. Akgül Ö, Çetinkaya E, Ersöz Ş, Tez M. Role of Surgery in Colorectal Cancer Liver Metastases. World J Gastroenterol (2014) 20(20):6113–22. doi: 10.3748/wjg.v20.i20.6113
4. Engstrand J, Nilsson H, Strömberg C, Jonas E, Freedman J. Colorectal Cancer Liver Metastases - a Population-Based Study on Incidence, Management and Survival. BMC Cancer (2018) 18(1):78. doi: 10.1186/s12885-017-3925-x
5. Rees M, Tekkis PP, Welsh FK, O’Rourke T, John TG. Evaluation of Long-Term Survival After Hepatic Resection for Metastatic Colorectal Cancer: A Multifactorial Model of 929 Patients. Ann Surg (2008) 247:125–35. doi: 10.1097/SLA.0b013e31815aa2c2
6. Ueno H, Mochizuki H, Hashiguchi Y, Hatsuse K, Fujimoto H, Hase K. Predictors of Extrahepatic Recurrence After Resection of Colorectal Liver Metastases. Br J Surg (2004) 91:327–33. doi: 10.1002/bjs.4429
7. Wang XY, Zhang R, Wang Z, Geng Y, Lin J, Ma K, et al. Meta-Analysis of the Association Between Primary Tumour Location and Prognosis After Surgical Resection of Colorectal Liver Metastases. Br J Surg (2019) 106(13):1747–60. doi: 10.1002/bjs.11289
8. Sasaki K, Morioka D, Conci S, Margonis GA, Sawada Y, Ruzzenente A, et al. The Tumor Burden Score: A New “Metro-Ticket” Prognostic Tool For Colorectal Liver Metastases Based on Tumor Size and Number of Tumors. Ann Surg (2018) 267(1):132–41. doi: 10.1097/SLA.0000000000002064
9. Kuo IM, Huang SF, Chiang JM, Yeh CY, Chan KM, Chen JS, et al. Clinical Features and Prognosis in Hepatectomy for Colorectal Cancer With Centrally Located Liver Metastasis. World J Surg Oncol (2015) 13:92. doi: 10.1186/s12957-015-0497-6
10. Sasaki K, Andreatos N, Margonis GA, He J, Weiss M, Johnston F, et al. The Prognostic Implications of Primary Colorectal Tumor Location on Recurrence and Overall Survival in Patients Undergoing Resection for Colorectal Liver Metastasis. J Surg Oncol (2016) 114(7):803–9. doi: 10.1002/jso.24425
11. Hitpass L, Heise D, Schulze-Hagen M, Pedersoli F, Ulmer F, Amygdalos I, et al. Primary Tumor Location Is a Prognostic Factor for Intrahepatic Progression-Free Survival in Patients With Colorectal Liver Metastases Undergoing Portal Vein Embolization as Preparation for Major Hepatic Surgery. Cancers (Basel) (2020) 12(6):1638. doi: 10.3390/cancers12061638
12. Liu W, Wang HW, Wang K, Xing BC. The Primary Tumor Location Impacts Survival Outcome of Colorectal Liver Metastases After Hepatic Resection: A Systematic Review and Meta-Analysis. Eur J Surg Oncol (2019) 45(8):1349–56. doi: 10.1016/j.ejso.2019.04.017
13. Okajima W, Komatsu S, Ichikawa D, Kosuga T, Kubota T, Okamoto K, et al. Prognostic Impact of the Number of Retrieved Lymph Nodes in Patients With Gastric Cancer. J Gastroenterol Hepatol (2016) 31(9):1566–71. doi: 10.1111/jgh.13306
14. Zhang CH, Li YY, Zhang QW, Biondi A, Fico V, Persiani R, et al. The Prognostic Impact of the Metastatic Lymph Nodes Ratio in Colorectal Cancer. Front Oncol (2018) 8:628. doi: 10.3389/fonc.2018.00628
15. Nanji S, Tsang ME, Wei X, Booth CM. Regional Lymph Node Involvement in Patients Undergoing Liver Resection for Colorectal Cancer Metastases. Eur J Surg Oncol (2017) 43(2):322–9. doi: 10.1016/j.ejso.2016.10.033
16. Okuno M, Goumard C, Mizuno T, Kopetz S, Omichi K, Tzeng CD, et al. Prognostic Impact of Perihepatic Lymph Node Metastases in Patients With Resectable Colorectal Liver Metastases. Br J Surg (2018) 105(9):1200–9. doi: 10.1002/bjs.10822
17. Zong Z, Zhou T, Rao L, Jiang Z, Li Y, Hou Z, et al. Musashi2 as a Novel Predictive Biomarker for Liver Metastasis and Poor Prognosis in Colorectal Cancer. Cancer Med (2016) 5(4):623–30. doi: 10.1002/cam4.624
18. Liang JY, Xi SY, Shao Q, Yuan YF, Li BK, Zheng Y, et al. Histopathological Growth Patterns Correlate With the Immunoscore in Colorectal Cancer Liver Metastasis Patients After Hepatectomy. Cancer Immunol Immunother (2020) 69(12):2623–34. doi: 10.1007/s00262-020-02632-6
19. Wang Y, Lin HC, Huang MY, Shao Q, Wang ZQ, Wang FH, et al. The Immunoscore System Predicts Prognosis After Liver Metastasectomy in Colorectal Cancer Liver Metastases. Cancer Immunol Immunother (2018) 67(3):435–44. doi: 10.1007/s00262-017-2094-8
20. Neal CP, Cairns V, Jones MJ, Masood MM, Nana GR, Mann CD, et al. Prognostic Performance of Inflammation-Based Prognostic Indices in Patients With Resectable Colorectal Liver Metastases. Med Oncol (2015) 32(5):144. doi: 10.1007/s12032-015-0590-2
21. Wu Y, Li C, Zhao J, Yang L, Liu F, Zheng H, et al. Neutrophil-To-Lymphocyte and Platelet-to-Lymphocyte Ratios Predict Chemotherapy Outcomes and Prognosis in Patients With Colorectal Cancer and Synchronous Liver Metastasis. World J Surg Oncol (2016) 14(1):289. doi: 10.1186/s12957-016-1044-9
22. Fong Y, Fortner J, Sun RL, Brennan MF, Blumgart LH. Clinical Score for Predicting Recurrence After Hepatic Resection for Metastatic Colorectal Cancer: Analysis of 1001 Consecutive Cases. Ann Surg (1999) 230:309–18. doi: 10.1097/00000658-199909000-00004
23. Nordlinger B, Guiguet M, Vaillant JC, Balladur P, Boudjema K, Bachellier P, et al. Surgical Resection of Colorectal Carcinoma Metastases to the Liver. A Prognostic Scoring System to Improve Case Selection, Based on 1568 Patients. Association Française De Chirurgie. Cancer (1996) 1(77):1254–62. doi: 10.1002/(SICI)1097-0142(19960401)77:7<1254::AID-CNCR5>3.0.CO;2-I
Keywords: colorectal cancer, liver metastasis, primary tumpur site, nomogram, overall survival, cancer-specific survival
Citation: Cao Y, Ke S, Deng S, Yan L, Gu J, Mao F, Xue Y, Zheng C, Cai W, Liu H, Li H, Shang F, Sun Z, Wu K, Zhao N and Cai K (2021) Development and Validation of a Predictive Scoring System for Colorectal Cancer Patients With Liver Metastasis: A Population-Based Study. Front. Oncol. 11:719638. doi: 10.3389/fonc.2021.719638
Received: 02 June 2021; Accepted: 10 November 2021;
Published: 01 December 2021.
Edited by:
Michele Caraglia, University of Campania Luigi Vanvitelli, ItalyReviewed by:
Xueliang Wu, First Affiliated Hospital of Hebei North University, ChinaMichele Ghidini, IRCCS Foundation Ca ‘Granda Ospedale Maggiore Policlinico, Italy
Copyright © 2021 Cao, Ke, Deng, Yan, Gu, Mao, Xue, Zheng, Cai, Liu, Li, Shang, Sun, Wu, Zhao and Cai. This is an open-access article distributed under the terms of the Creative Commons Attribution License (CC BY). The use, distribution or reproduction in other forums is permitted, provided the original author(s) and the copyright owner(s) are credited and that the original publication in this journal is cited, in accordance with accepted academic practice. No use, distribution or reproduction is permitted which does not comply with these terms.
*Correspondence: Ke Wu, wuke201288@126.com; Ning Zhao, zhaoning_hust_whuh@126.com; Kailin Cai, caikailin@hust.edu.cn
†These authors have contributed equally to this work