- 1InORP ONCOCLÍNICAS Group, Oncology Institute of Ribeirão Preto, Ribeirão Preto, Brazil
- 2Molecular Oncology Research Center, Barretos Cancer Hospital, Barretos, Brazil
- 3Epidemiology and Biostatistics Nucleus, Barretos Cancer Hospital, Barretos, Brazil
- 4Departament of Pathology, Barretos Cancer Hospital, Barretos, Brazil
- 5Discipline of Gynecology, Instituto do Cancer do Estado de São Paulo (ICESP), Faculdade de Medicina da Universidade de São Paulo, São Paulo, Brazil
- 6Life and Health Sciences Research Institute (ICVS), School of Medicine, University of Minho, Braga, Portugal
- 7ICVS/3B’s - PT Government Associate Laboratory, Braga/Guimarães, Portugal
- 8Department of Gynecologic Oncology, Barretos Cancer Hospital, Barretos, Brazil
Background: The molecular profile of endometrial cancer has become an important tool in determining patient prognosis and their optimal adjuvant treatment. In addition to The Cancer Genome Atlas (TCGA), simpler tools have been developed, such as the Proactive Molecular Risk Classifier for Endometrial Cancer (ProMisE). We attempted to determine a genetic signature to build a recurrence risk score in patients diagnosed with low- and intermediate-risk endometrial cancer.
Methods: A case-control study was conducted. The eligible patients were women diagnosed with recurrence low- and intermediate-risk endometrial cancer between January 2009 and December 2014 at a single institution; the recurrence patients were matched to two nonrecurrence patients with the same diagnosis by age and surgical staging. Following RNA isolation of 51 cases, 17 recurrence and 34 nonrecurrence patients, the expression profile was determined using the nCounter® PanCancer Pathways Panel, which contains 770 genes.
Results: The expression profile was successfully characterized in 49/51 (96.1%) cases. We identified 12 genes differentially expressed between the recurrence and nonrecurrence groups. The ROC curve for each gene was generated, and all had AUCs higher than 0.7. After backward stepwise logistic regression, four genes were highlighted: FN1, DUSP4, LEF1, and SMAD9. The recurrence risk score was calculated, leading to a ROC curve of the 4-gene model with an AUC of 0.93, sensitivity of 100%, and specificity of 72.7%.
Conclusion: We identified a four-gene signature that may be associated with recurrence in patients with low- and intermediate-risk endometrial cancer. This finding suggests a new prognostic factor in this poorly explored group of patients with endometrial cancer.
Introduction
Endometrial cancer is the most prevalent gynecological tumor in developed countries, such as the USA and members of the European Union (1). The number of cases in the last decade have increased, possibly due to the increase in obesity in these countries (2). In Brazil, endometrial cancer is the eighth most commonly diagnosed cancer in women, with 6,540 new cases in 2020 (3). When diagnosed at an early stage, these patients have an excellent prognosis. Countless risk stratifications associate staging with other variables, such as tumor grade, lymphovascular space invasion (LVSI) and histology, to define sequential adjuvant treatments (4).
Traditionally, it has been considered two distinct diseases since Bokhman’s publication in the early 1980s (low-grade endometrioid adenocarcinomas (type I, “well-differentiated”) and nonendometrioid carcinomas (type II, “poorly differentiated”) (5). Recently, The Cancer Genome Atlas (TCGA) project changed the understanding of the carcinogenesis of this tumor, leading to four molecular subgroups with different prognoses (DNA polymerase epsilon (POLE) ultramutated, microsatellite instability (MSI) hypermutated, copy number (CN) low, and CN high) (6). Due to the complexity (whole genome sequencing, exome sequencing, microsatellite assays, and CN aberration analysis), costs, and need for ideally frozen tissue for reproducibility of this classification in clinical practice, new methodologies have been developed. The two most currently used are the Proactive Molecular Risk Classifier for Endometrial Cancer (ProMisE) and Leiden/TransPORTEC classification, in which the four groups with different prognoses are also described (7, 8). Immunohistochemistry was used to detect the presence/absence of mismatch repair (MMR) proteins and to evaluate TP53 mutations, and only one step used genetic sequencing (next-generation or Sanger sequencing) to identify POLE hotspot exonuclease domain mutations (7, 8).
Using the TCGA consortium database, some studies have built prognostic models of endometrial cancer recurrence according to genetic signatures or evaluated RNA expression (9, 10). Furthermore, other studies correlate potential genetic signatures with histopathological markers such as tumor-infiltrating immune cells (11, 12).
The aim of this study was to determine a genetic signature of recurrence risk in patients diagnosed with low- and intermediate-risk endometrial cancer in routine formalin-fixed paraffin-embedded (FFPE) tissue using a large panel of 770 genes covering 13 key cancer-related pathways by NanoString, a highly sensitive and robust methodology for RNA expression of FFPE samples.
Material and Methods
Patients and Specimens
From a retrospective cohort of 195 patients diagnosed with low- and intermediate-risk endometrial cancer between January 2009 and December 2014 at Barretos Cancer Hospital (BCH), two pathologists with oncogynecologic expertise reviewed the initial report of all patients to confirm their diagnosis. Clinical and pathological features were obtained from the medical records. Of the 22 patients who initially presented recurrence, the diagnosis remained low- and intermediate-risk endometrial cancer for 17 patients. We define low- and intermediate-risk endometrial cancer according to the European Society of Medical Oncology (ESMO)-modified criteria (4). Based on these results, a case-control study was carried out. Nonrecurrence patients with the same histopathological diagnosis were matched to recurrence cases in a 2:1 ratio by age and FIGO (International Federation of Gyneocologic and Obstetrics) staging (IA and IB). Overall, 51 patients (17 recurrence and 34 non-recurrence) were analyzed.
This study was conducted following the ethical principles of the Declaration of Helsinki, and the BCH Ethical Review Board approved it in March 2017 (Reference number 1.942.488).
DNA and RNA Isolation
DNA and RNA were isolated from 10 µm-thick formalin-fixed paraffin-embedded (FFPE) tumor samples sectioned on slides, as previously reported (13). One slide was stained with hematoxylin and eosin (H&E) and evaluated by experienced pathologists for identification, sample adequacy assessment, and selection of the tumor tissue area (minimum of 60% tumor area). DNA and RNA were isolated using the QiaAmp DNA Micro kit (Qiagen, Hilden, Germany) and the RecoverAll™ Total Nucleic Acid Isolation kit (Ambion by Life Technologies, Austin, TX, USA), respectively. The quality and concentration of DNA and RNA were measured by both a NanoDrop ND-200 spectrophotometer (NanoDrop Products, Wilmington, DE, USA) and Qubit Fluorometric Quantitation (Thermo Fisher Scientific, USA). The samples were stored at -80°C until molecular analysis.
ProMisE Evaluation
The ProMisE assessment was performed using molecular methodologies, namely, molecular evaluation of MSI, TP53 mutation analyses by next-generation sequencing, and POLE hotspot mutations by Sanger sequencing.
To define MSI, we performed hexaplex PCR with six monomorphic mononucleotide markers (NR21, NR24, NR27, BAT25, BAT26, and HSP110), followed by fragment analysis in a 3500XL Genetic Analyzer sequencer (Applied Biosystems, Foster City, CA, USA), as previously described by our group (14). The presence of two or more markers with instability classified the cases as high microsatellite instability (MSI-H), the presence of one marker with instability was classified as low MSI (MSI-L), and the absence of any marker with instability as microsatellite stable (MSS). Presence of MSI was determined only for MSI-H cases.
To evaluate POLE mutations, we used direct Sanger sequencing, as described by Britton et al. PCR was performed using targeted primers for the exonuclease domain (exons 9-14) of POLE (15). The purified samples were subjected to capillary electrophoresis in a 3500XL Genetic Analyzer sequencer (Applied Biosystems, Foster City, CA, USA), and the results were analyzed with SegScape v2.7 software (Applied Biosystems, Foster City, CA, USA).
TP53 mutations were detected with an NGS-based assay using the Illumina TruSight Tumor 15 (TST15) on the MiSeq instrument (Illumina, San Diego, CA, USA) according to the manufacturer’s instructions, as previously reported (16). The TST15 panel assesses all coding sequences of the TP53 gene. Read alignment and variant calling were performed with BaseSpace BWA Enrichment version 2.1 (Illumina, San Diego, CA, USA) and Sophia DDM® software version 5.7.3 (Sophia Genetics SA, Switzerland). The identification of pathogenic variants occurred after the application of filters to remove low-quality variants. Variants with < 500X read depth, VAF <10%, and intronic, intergenic, 3’ UTR, and synonymous variants were excluded. Thereafter, the variants that presented as polymorphisms, within a frequency >1% in the GnomAD database, were removed (those that had no population frequency information followed in the analyses). Finally, the pathogenicity of variants was checked in the databases ClinVar, IARC TP53, COSMIC, and CGI.
NanoString nCounter Analysis
Samples were processed for analysis on the NanoString nCounter Flex system using the 770 gene nCounter® PanCancer Pathways Panel (NanoString Technologies, Inc., Seattle, WA, USA), as previously reported (17). This panel assesses 13 cancer-associated canonical pathways related to basic cancer biology (Notch, Wnt, Hedgehog, Chromatin modification, Transcriptional regulation, DNA damage control, TGF-β, MAPK, STAT, PI3K, RAS, Cell cycle, Apoptosis). Briefly, 100 ng of total RNA, quantified by a Qubit Fluorometric System (Thermo Fisher Scientific, USA), from each sample was hybridized for 21 hours at 65°C, followed by purification and RNA/probe complex immobilization in nCounter PrepStation (NanoString Technologies, Inc., Seattle, WA, USA) and cartridge scanning in a digital analyzer (NanoString Technologies, Inc., Seattle, WA, USA), according to the manufacturer’s protocol. Reading with 280 field-of-views (FOVs) was used in the study samples.
Bioinformatics and Statistical Analysis
We used nSolver™ Analysis Software, version 4.0 (NanoString Technologies, Inc., Seattle, WA, USA) to assess the quality control parameters of all samples. Further analyses were performed using the R language and environment for statistical computing (R-project (v3.6.3); The R Foundation, Vienna, Austria) (18). The quantro package (v1.18.0) was applied for cartridge evaluation and to assist in choosing the normalization method. The gene expression data were normalized by the quantile method implemented in the NanoStringNorm package and transformed into a log2 scale. RNA differential expression was evaluated in the NanoStringNorm package considering two different groups (recurrent vs. nonrecurrent low- and intermediate-risk endometrial cancer) with a significance level of p ≤ 0.01 and fold change of 2.0 (19). Heatmaps and hierarchical clustering of differentially expressed genes were built with the ComplexHeatmap package (v2.0.0) (20). The STRING database was applied to predict interaction networks from differentially expressed genes (21).
Through the ROC curves, we evaluated the sensitivity and specificity of differential RNA expression by comparing patients with recurrence with those who did not have recurrence using the pROC package (22). An area under the curve (AUC) above 0.7 was considered acceptable for further gene evaluation. We used the backward stepwise logistic regression technique within the MASS package (version 7.3.53) to build a recurrence risk model according to the RNA expression of the samples.
Data analysis was performed using IBM Statistical Package for the Social Sciences (SPSS) database version 27.0 (SPSS, Chicago, IL, USA). Descriptive statistical analysis for quantitative variables used mean, maximum, and minimum and for qualitative variables used percentage. Once the variables were defined, univariate analysis was performed using the chi-square test and Mann-Whitney’s U-test. Variables with a p value < 0.2 in univariate analyses were entered into the logistic regression analysis. The threshold for statistical significance was 5%. Study data were collected and managed using REDCap (Research Electronic Data Capture) electronic data capture tools hosted at BCH (23).
Results
Patient Features
The clinical and pathological information of the two groups is summarized in Table 1. More than 96% of patients are ECOG 0-1. In the recurrence group, we had four patients diagnosed with endometrial adenocarcinoma with squamous differentiation, whereas in the nonrecurrence group, we did not have any diagnosis of this histological subtype (p = 0.01). There was a higher prevalence of white patients in the recurrence group (94.1%) than in the nonrecurrence group (70.6%) (p = 0.075). Other clinical and pathological features were well balanced between the two groups.
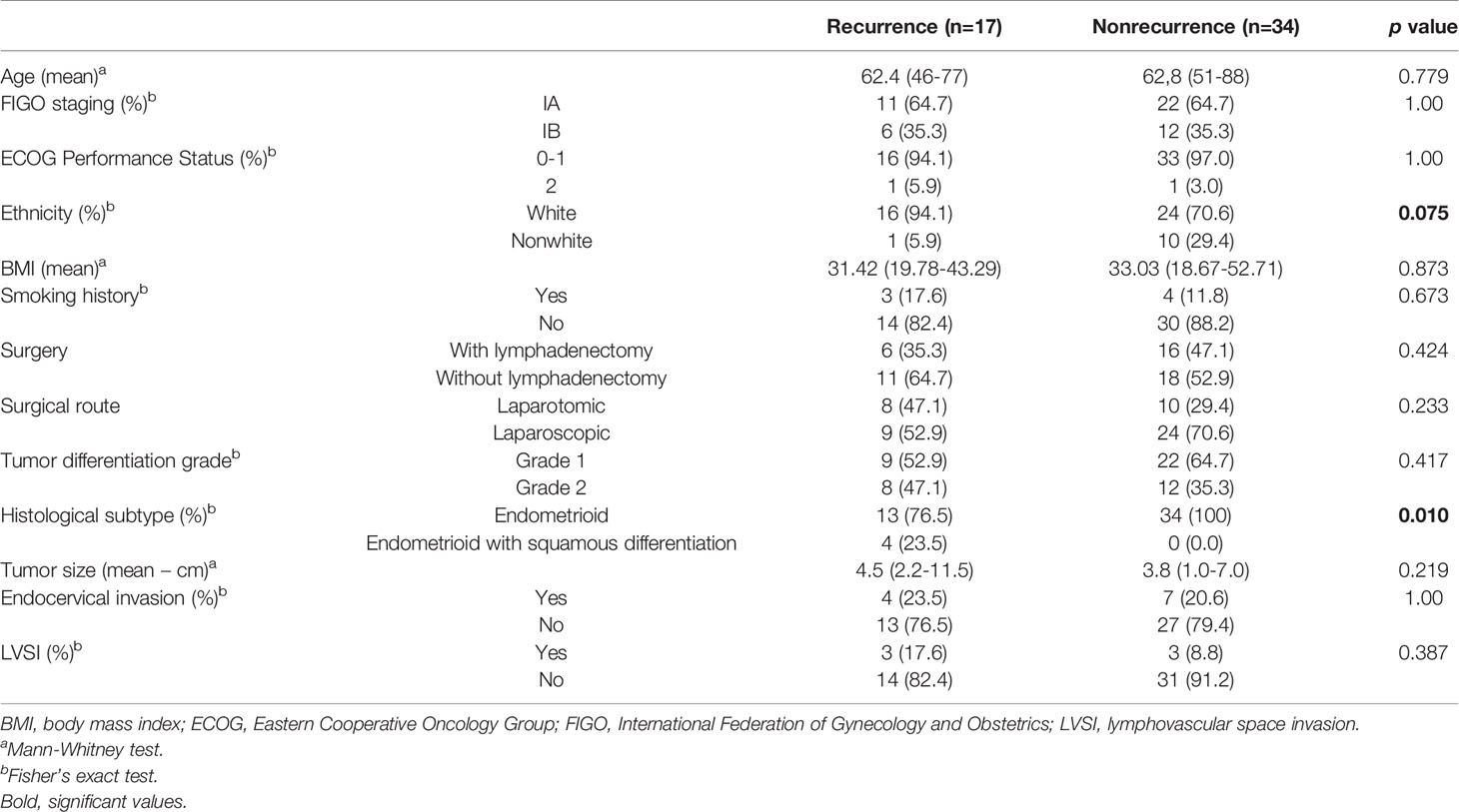
Table 1 Clinical and pathological characteristics of patients with low- and intermediate-risk endometrial cancer.
Of the 51 samples that were sequenced for ProMisE, we observed a high frequency of inconclusive results due to the poor quality of the DNA obtained, hampering meaningful analysis. The assessment of MSI was inconclusive in one case, and among the other 50 cases, 12 (24%) were MSI-H. Of the 39 remaining samples for POLE sequencing, 18 were inconclusive, and only one (4.8%) was mutated [exon 9 – c.857C>G; p. (Pro286Arg)]. Concerning the 38 samples for TP53 mutation analysis, 10 cases (66.7%) were wild-type, and five (33.3%) were mutated (Supplementary Table 1). There was no difference between the four groups of the ProMisE methodology and the increased chance of recurrence (p = 0.823).
Gene Expression
Concerning the gene expression profile, 49 of the 51 cases (96%) were conclusive, leading to 16 recurrence and 33 nonrecurrence samples for further analysis. Two samples were excluded due to low-quality RNA. The expression profile based on unsupervised clustering showed 12 genes with differential RNA expression between the two groups studied (Figure 1). The LEF1, PLA2G4A, DKK1, BMP4, FGF19, FN1, SMAD9, and DUSP4 genes showed increased RNA expression in the recurrence group, while HIST1H3G, SIX1, TNF, and IL8 were downregulated compared to nonrecurrence group.
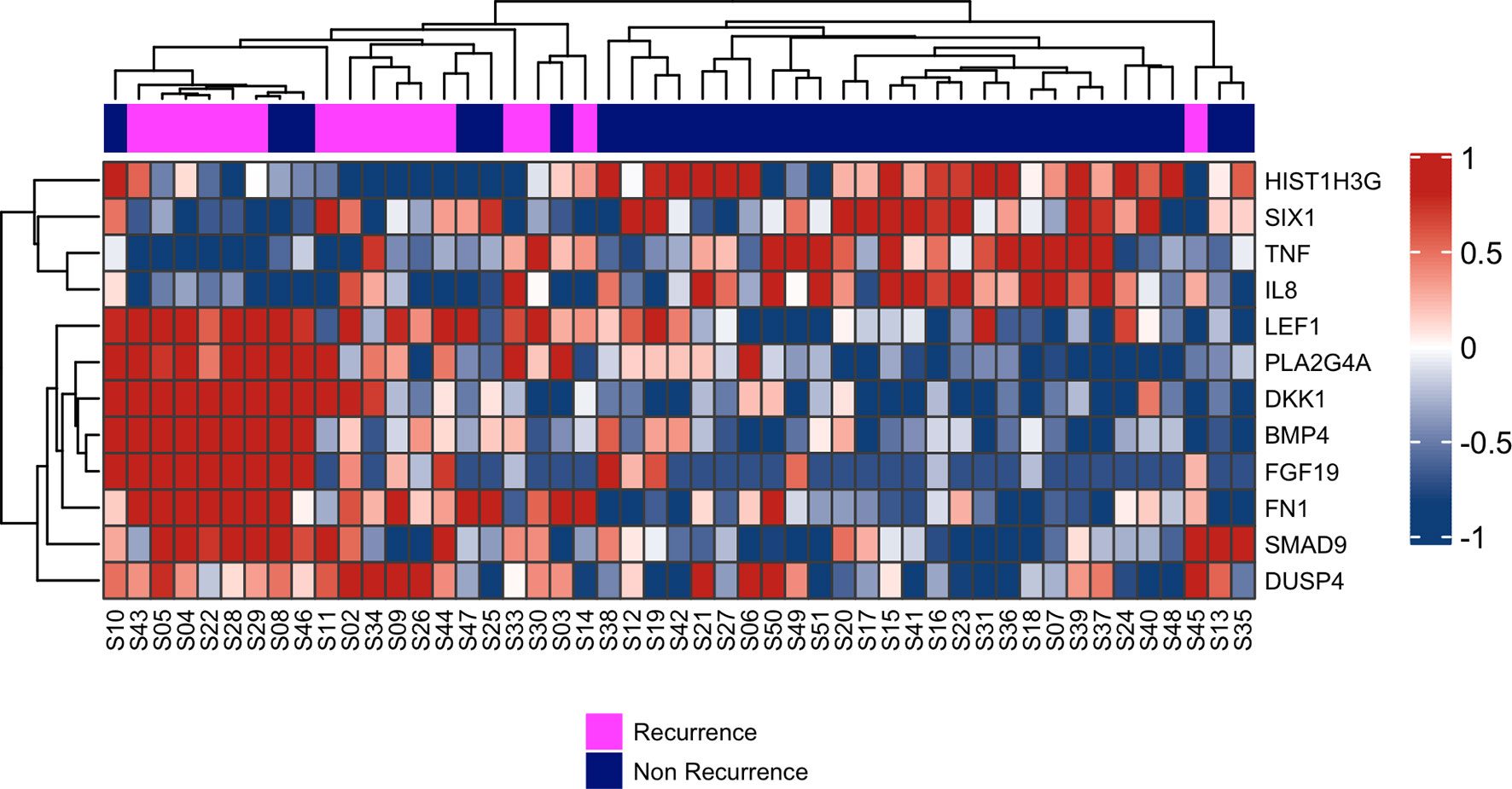
Figure 1 Hierarchical clustering of the 12 RNAs differentially expressed between patients who presented recurrence (pink) compared to those who did not relapse (purple). On the right side: gene expression scaling from dark blue (downregulated) to dark red (upregulated).
We next generated a ROC curve for each of the 12 genes described above to assess the performance of each gene to discriminate between the recurrence and nonrecurrence groups (Table 2). All 12 genes presented an AUC higher than 0.7.
To understand the crosstalk among the 12 genes, an interaction network was constructed and is depicted in Figure 2. Except for the DUSP4 and HIST1H3G genes, interactions are known among the differentially expressed genes.
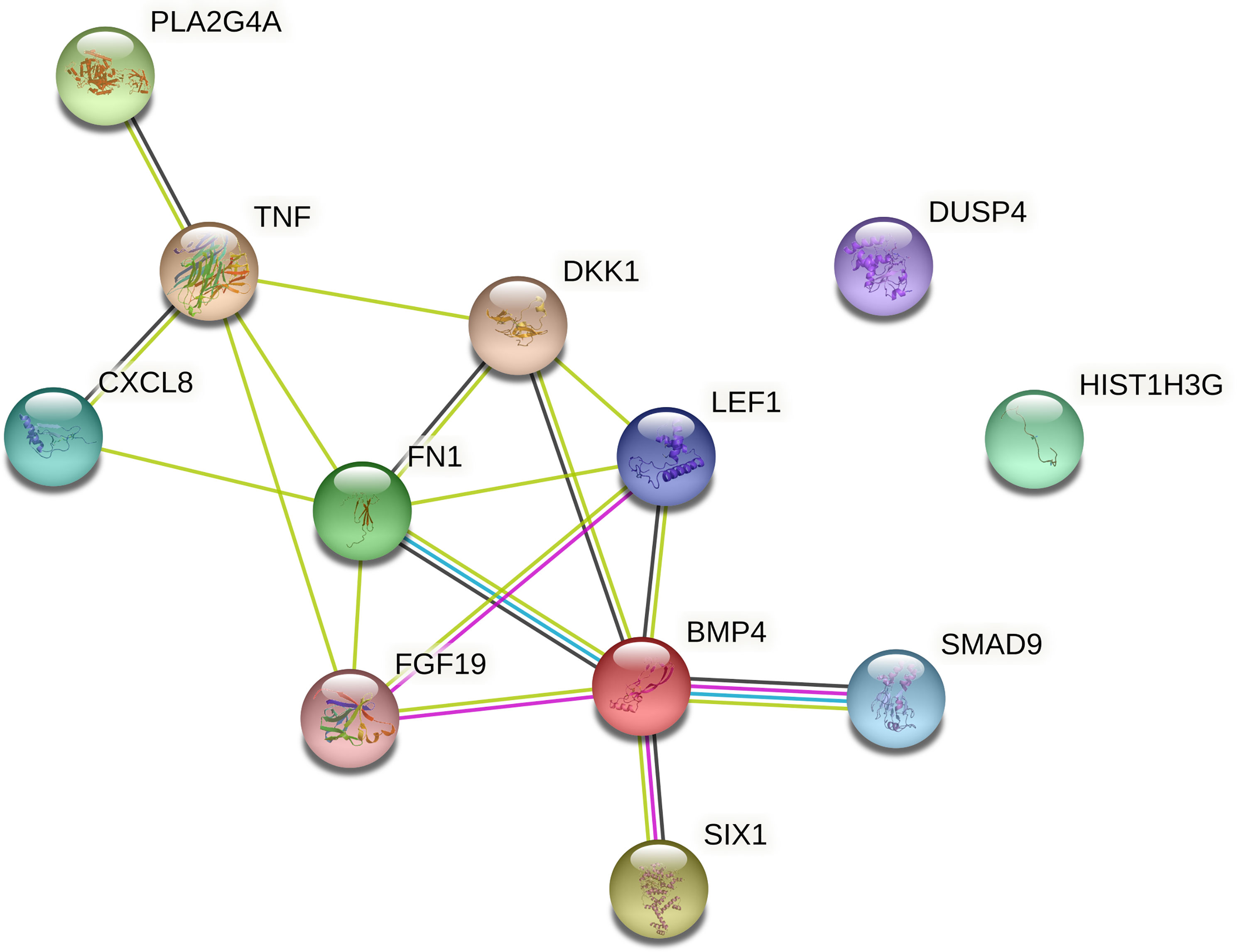
Figure 2 STRING interaction network of the 12 genes differentially expressed in the recurrence and nonrecurrence groups of patients [known interactions (light blue – from curated databases; purple – experimentally determined); predicted interactions (green, gene neighborhood; red, gene fusions; dark blue, gene cooccurrence); others (yellow, text mining; black, coexpression; gray, protein homology)].
Recurrence Risk Score
Based on the 12 differentially expressed genes, we applied logistic regression to build a recurrence risk score (RRS) and improve predictive performance. Through the backward stepwise logistic regression model, four genes with the best performance were selected: FN1, DUSP4, LEF1, and SMAD9 (increased RNA expression in the recurrence cases). The RRS was calculated as the logit from the logistic regression as follows: RRS = -21.14 + 1.02*FN1 + 1.07*DUSP4 + 0.6211*LEF1 + 0.8832*SMAD9 (Supplementary Table 2).
Univariate analysis was performed to calculate the odds ratio (OR) for each gene and for the final score (Table 3). Cases with overexpression of the FN1 gene had an OR of 3.3 for recurrence compared to cases without overexpression. In addition, the final gene score showed an OR of 2.7 for recurrence.
Moreover, the combination of the expression of the four genes showed an AUC of 0.93, a sensitivity of 100%, and a specificity of 72.7% to identify low- and intermediate-risk endometrial cancer with recurrence trough the RNA expression (Figure 3).
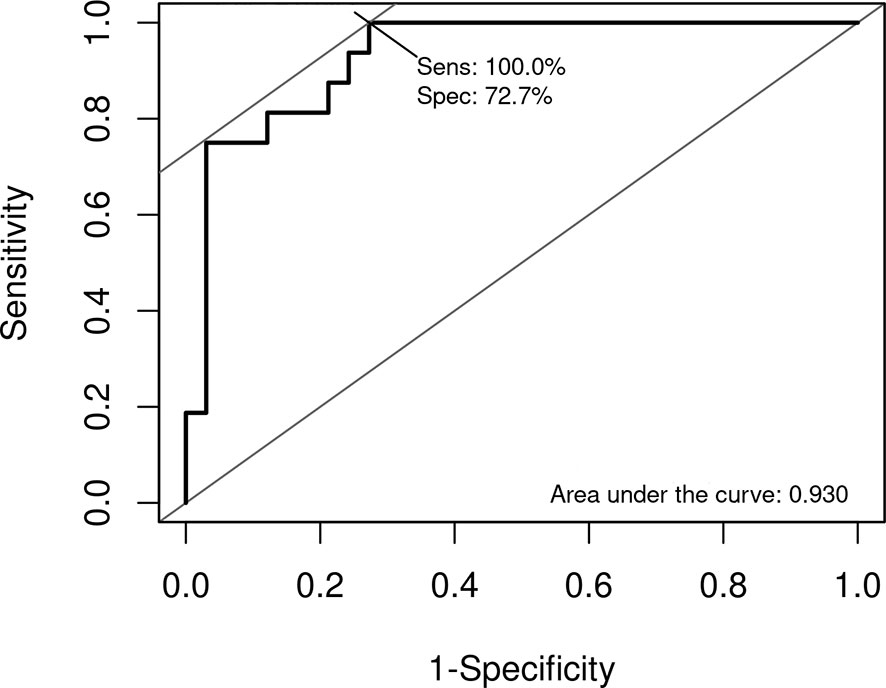
Figure 3 Receiver operating characteristic (ROC) curve of the recurrence risk score (RRS). Sensitivity and specificity were determined by the Youden index.
We performed a logistic regression analysis with the four differentially expressed genes score and two significant clinicopathological variables (ethnicity and histological subtype). The histological subtype variable was withdrawn from this model since one of its categories did not contain subjects (no endometrioid with squamous differentiation in the nonrecurrence group), resulting in no data conversion to the odds ratio value. Using a backward stepwise logistic regression technique, a new model was constructed with two parameters: four differentially expressed genes score (OR: 2.616; p = 0.001) and white ethnicity (OR: 0.299; p = 0.342).
Discussion
In this study, we characterized the expression profile of two distinct groups (recurrence and nonrecurrence) of low- and intermediate-risk endometrial cancer. Twelve genes were differentially expressed. After performed a logistic regression, four genes remained to define a possible RRS model, exhibiting an impressive AUC of 0.93, with a sensitivity of 100% and a specificity of 73%. To the best of our knowledge, this is the first study to identify a gene signature associated with recurrence in low- and intermediate-risk endometrial cancer.
The four genes are associated with important cancer pathways, namely, the MAPK/PI3K pathways (FN1 and DUSP4), the Wnt pathway (LEF1), and the TGF pathway (SMAD9).
The Wnt/beta-catenin signaling pathway plays an essential role in tumorigenesis and recurrence in endometrial cancer. Two studies demonstrated the role of the beta-catenin/CTNNB1 gene as a poor prognostic factor in low-risk endometrial cancer (24, 25). First, in a large study with 342 patients with low-grade and early-stage endometrial cancer through next-generation sequencing, the worst recurrence-free and overall survival was demonstrated in patients with CTNNB1 and TP53 mutations (24). In a case-control study similar to ours with recurrent stage I and grade 1 endometrioid endometrial cancers, Moroney et al. showed that CTNNB1 mutations are present at higher rates in recurrent patients (25).
LEF1 (lymphoid enhancer factor) is a nuclear transcription factor that interacts with beta-catenin to activate the Wnt pathway (26). The role of LEF1 protein overexpression in the carcinogenesis of endometrial cancer may be related to the modulation of cell surface adhesion proteins, influencing the prognosis of this tumor (27). A study with LEF1 knockout mice demonstrated its importance in endometrial cancer carcinogenesis. The LEF1 protein is essential in uterine glandular formation, and its overexpression possibly influences the disordered growth of glandular cells and the development of cancer (28).
The MAPK/PI3K pathway is a central pathway in the tumorigenesis of several tumors, and it is even a target in breast cancer treatment (29). The role of FN1, which encodes fibronectin, and DUSP4, which encodes dual-specificity protein phosphatase 4, in endometrial cancer is not well understood. A recent study by Raglan et al. evaluated the TCGA proteomic data of 560 endometrial cancers and demonstrated that obese patients without cancer had upregulation of several proteins, including DUSP4 (30). Another recent study evaluated the predictive model of lymph node involvement in endometrial cancer using a combined proteomic and transcriptomic approach. The authors reported that high protein expression of fibronectin, cyclin D1, and tumor grade were associated with lymph node involvement. Moreover, overexpression of both FN1 and CCND1 (cyclin D1 encoded gene) genes correlated with greater potential for mesenchymal invasion (31).
The third pathway identified was TGF-β through the overexpression of the SMAD9 gene. This gene belongs to the SMAD superfamily (Drosophila mothers against decapentaplegic protein) made up of important cytokines in the TGF-β family (32). SMAD9 overexpression is associated with the prevalence of hamartomatous polyposis and is a prognostic factor for lung cancer (33, 34). So far, no studies have addressed its impact in endometrial cancer.
Analyzing the clinical and pathological features of this case-control study, having squamous differentiation in the histopathological diagnosis could be a risk factor for recurrence in this population, according to previously published studies (35, 36). Related to ethnicity, some studies have already shown less medical access in the nonwhite population in the USA impacting oncologic outcomes (37); however, demonstrated a risk due to ethnicity itself. In this study, there was a higher prevalence of white patients in the recurrence group.
Despite these notable findings, our study has some limitations, such as having a small sample size and a retrospective nature. The small number of cases can be explained by the excellent prognosis and low risk of recurrence in low- and intermediate-risk endometrial cancer patients. Therefore, validation of this 4-gene signature in a larger cohort is needed to confirm its predictive value. Moreover, it would be interesting to validate these 4 biomarkers by other methodologies, such as immunohistochemistry. On the other hand, our study has several strengths. First, the robustness of the NanoString methodology proved to be effective for gene expression evaluation in routine samples, even after many years of storage, up to 10 years in our study (38). Second, we evaluated a restricted subpopulation of endometrial cancer to detect risk factors for recurrence in this population. As this is a retrospective study, all patients who relapsed and their matched controls had their pathological reports reviewed by expert gynecologic oncology pathologists to minimize selection bias. Some studies have shown that gynecologic oncology has one of the highest rates of disagreement in the expert pathologist’s report compared to the nonspecialized report (39, 40). In addition, this case-control study represents the experience of a reference cancer center hospital where well-defined treatment protocols minimize possible sample heterogeneity.
Conclusion
For the first time, we identified a four-gene signature associated with recurrence in low and intermediate endometrial cancer. Additionally, the four genes (FN1, DUSP4, LEF1, and SMAD9) identified can shed light on the mechanisms of recurrence in endometrial cancer. This study can pave the way for personalized approaches of low- and intermediate-risk endometrial cancer.
Data Availability Statement
The datasets presented in this study can be found in online repositories. The names of the repository/repositories and accession number(s) can be found below: https://www.ncbi.nlm.nih.gov/geo/, GSE178671.
Ethics Statement
The studies involving human participants were reviewed and approved by BCH Ethical Review Board in March 2017. Written informed consent for participation was not required for this study in accordance with the national legislation and the institutional requirements.
Author Contributions
Conception and design: DA, RMR, and RdR. Development of methodology: DA, RMR, and RdR. Acquisition of data: DA. Analysis and interpretation of data: DA, LS, AL, ML, GB, VS, GM, MB, AS, RMR, and RdR. Writing, review, and/or revision of the manuscript: DA, LS, AL, ML, GB, VS, GM, MB, AS, AE, JC, RMR, and RdR. Study supervision: RMR and RdR. All authors contributed to the article and approved the submitted version.
Conflict of Interest
The authors declare that the research was conducted in the absence of any commercial or financial relationships that could be construed as a potential conflict of interest.
Publisher’s Note
All claims expressed in this article are solely those of the authors and do not necessarily represent those of their affiliated organizations, or those of the publisher, the editors and the reviewers. Any product that may be evaluated in this article, or claim that may be made by its manufacturer, is not guaranteed or endorsed by the publisher.
Acknowledgments
We thank Barretos Cancer Hospital and the Public Ministry of Labor Campinas (Research, Prevention, and Education of Occupational Cancer Project), Campinas, Brazil, for partially funding the present study. RMR is recipient of a CNPq productivity fellowship.
Supplementary Material
The Supplementary Material for this article can be found online at: https://www.frontiersin.org/articles/10.3389/fonc.2021.729219/full#supplementary-material
References
1. Siegel RL, Miller KD, Fuchs HE, Jemal A. Cancer Statistics, 2021. CA: Cancer J Clin (2021) 71(1):7–33. doi: 10.3322/caac.21654
2. Smrz SA, Calo C, Fisher JL, Salani R. An Ecological Evaluation of the Increasing Incidence of Endometrial Cancer and the Obesity Epidemic. Am J Obstet Gynecol (2021) 224(5):506.e1–.e8. doi: 10.1016/j.ajog.2020.10.042
3. Estimativa 2020: Incidência De Câncer No Brasil Rio De Janeiro: INCA - Instituto Nacional De Câncer José Alencar Gomes Da Silva (2020). Available at: www.inca.gov.br/estimativa/2020/.
4. Bendifallah S, Canlorbe G, Raimond E, Hudry D, Coutant C, Graesslin O, et al. A Clue Towards Improving the European Society of Medical Oncology Risk Group Classification in Apparent Early Stage Endometrial Cancer? Impact of Lymphovascular Space Invasion. Br J Cancer (2014) 110(11):2640–6. doi: 10.1038/bjc.2014.237
5. Bokhman JV. Two Pathogenetic Types of Endometrial Carcinoma. Gynecol Oncol (1983) 15(1):10–7. doi: 10.1016/0090-8258(83)90111-7
6. Kandoth C, Schultz N, Cherniack AD, Akbani R, Liu Y, Shen H, et al. Integrated Genomic Characterization of Endometrial Carcinoma. Nature (2013) 497(7447):67–73. doi: 10.1038/nature12113
7. Talhouk A, McConechy MK, Leung S, Li-Chang HH, Kwon JS, Melnyk N, et al. A Clinically Applicable Molecular-Based Classification for Endometrial Cancers. Br J Cancer (2015) 113(2):299–310. doi: 10.1038/bjc.2015.190
8. Stelloo E, Nout RA, Osse EM, Jürgenliemk-Schulz IJ, Jobsen JJ, Lutgens LC, et al. Improved Risk Assessment by Integrating Molecular and Clinicopathological Factors in Early-Stage Endometrial Cancer-Combined Analysis of the PORTEC Cohorts. Clin Cancer Res (2016) 22(16):4215–24. doi: 10.1158/1078-0432.CCR-15-2878
9. Deng F, Mu J, Qu C, Yang F, Liu X, Zeng X, et al. A Novel Prognostic Model of Endometrial Carcinoma Based on Clinical Variables and Oncogenomic Gene Signature. Front Mol Biosci (2020) 7:587822. doi: 10.3389/fmolb.2020.587822
10. Wang Z, Zhang J, Liu Y, Zhao R, Zhou X, Wang H. An Integrated Autophagy-Related Long Noncoding RNA Signature as a Prognostic Biomarker for Human Endometrial Cancer: A Bioinformatics-Based Approach. BioMed Res Int (2020) 2020:5717498. doi: 10.1155/2020/5717498
11. Chen B, Wang D, Li J, Hou Y, Qiao C. Screening and Identification of Prognostic Tumor-Infiltrating Immune Cells and Genes of Endometrioid Endometrial Adenocarcinoma: Based on the Cancer Genome Atlas Database and Bioinformatics. Front Oncol (2020) 10:554214. doi: 10.3389/fonc.2020.554214
12. Horeweg N, de Bruyn M, Nout RA. Prognostic Integrated Image-Based Immune and Molecular Profiling in Early-Stage Endometrial Cancer. Cancer Immunol Res (2020) 8(12):1508–19. doi: 10.1158/2326-6066.CIR-20-0149
13. Gomes I, Moreno DA, Dos Reis MB, da Silva LS, Leal LF, Gonçalves GM, et al. Low MGMT Digital Expression Is Associated With a Better Outcome of IDH1 Wildtype Glioblastomas Treated With Temozolomide. J Neuro-Oncol (2021) 151(2):135–44. doi: 10.1007/s11060-020-03675-6
14. Berardinelli GN, Scapulatempo-Neto C, Durães R, Antônio de Oliveira M, Guimarães D, Reis RM. Advantage of HSP110 (T17) Marker Inclusion for Microsatellite Instability (MSI) Detection in Colorectal Cancer Patients. Oncotarget (2018) 9(47):28691–701. doi: 10.18632/oncotarget.25611
15. Britton H, Huang L, Lum A, Leung S, Shum K, Kale M, et al. Molecular Classification Defines Outcomes and Opportunities in Young Women With Endometrial Carcinoma. Gynecol Oncol (2019) 153(3):487–95. doi: 10.1016/j.ygyno.2019.03.098
16. Campanella NC, Silva EC, Dix G, de Lima Vazquez F, Escremim de Paula F, Berardinelli GN, et al. Mutational Profiling of Driver Tumor Suppressor and Oncogenic Genes in Brazilian Malignant Pleural Mesotheliomas. Pathobiology (2020) 87(3):208–16. doi: 10.1159/000507373
17. Rosa MN, Evangelista AF, Leal LF, De Oliveira CM, Silva VAO, Munari CC, et al. Establishment, Molecular and Biological Characterization of HCB-514: A Novel Human Cervical Cancer Cell Line. Sci Rep (2019) 9(1):1913. doi: 10.1038/s41598-018-38315-7
18. Team RC. R: A Language and Environment for Statistical Computing (2019). Available at: https://wwwR-projectorg/ (Accessed 06 February 2021).
19. Waggott D, Chu K, Yin S, Wouters BG, Liu FF, Boutros PC. Nanostringnorm: An Extensible R Package for the Pre-Processing of Nanostring mRNA and MiRNA Data. Bioinf (Oxf Engl) (2012) 28(11):1546–8. doi: 10.1093/bioinformatics/bts188
20. Gu Z, Eils R, Schlesner M. Complex Heatmaps Reveal Patterns and Correlations in Multidimensional Genomic Data. Bioinf (Oxf Engl) (2016) 32(18):2847–9. doi: 10.1093/bioinformatics/btw313
21. Szklarczyk D, Gable AL, Lyon D, Junge A, Wyder S, Huerta-Cepas J, et al. STRING V11: Protein-Protein Association Networks With Increased Coverage, Supporting Functional Discovery in Genome-Wide Experimental Datasets. Nucleic Acids Res (2019) 47(D1):D607–d13. doi: 10.1093/nar/gky1131
22. Robin X, Turck N, Hainard A, Tiberti N, Lisacek F, Sanchez JC, et al. Proc: An Open-Source Package for R and s+ to Analyze and Compare ROC Curves. BMC Bioinf (2011) 12:77. doi: 10.1186/1471-2105-12-77
23. Harris PA, Taylor R, Thielke R, Payne J, Gonzalez N, Conde JG. Research Electronic Data Capture (Redcap)–a Metadata-Driven Methodology and Workflow Process for Providing Translational Research Informatics Support. J Biomed Inf (2009) 42(2):377–81. doi: 10.1016/j.jbi.2008.08.010
24. Kurnit KC, Kim GN, Fellman BM, Urbauer DL, Mills GB, Zhang W, et al. CTNNB1 (Beta-Catenin) Mutation Identifies Low Grade, Early Stage Endometrial Cancer Patients at Increased Risk of Recurrence. Modern Pathol (2017) 30(7):1032–41. doi: 10.1038/modpathol.2017.15
25. Moroney MR, Davies KD, Wilberger AC, Sheeder J, Post MD, Berning AA, et al. Molecular Markers in Recurrent Stage I, Grade 1 Endometrioid Endometrial Cancers. Gynecol Oncol (2019) 153(3):517–20. doi: 10.1016/j.ygyno.2019.03.100
26. McMellen A, Woodruff ER, Corr BR, Bitler BG, Moroney MR. Wnt Signaling in Gynecologic Malignancies. Int J Mol Sci (2020) 21(12):4272. doi: 10.3390/ijms21124272
27. Hsu YT, Osmulski P, Wang Y, Huang YW, Liu L, Ruan J, et al. Epcam-Regulated Transcription Exerts Influences on Nanomechanical Properties of Endometrial Cancer Cells That Promote Epithelial-to-Mesenchymal Transition. Cancer Res (2016) 76(21):6171–82. doi: 10.1158/0008-5472.CAN-16-0752
28. Shelton DN, Fornalik H, Neff T, Park SY, Bender D, DeGeest K, et al. The Role of LEF1 in Endometrial Gland Formation and Carcinogenesis. PloS One (2012) 7(7):e40312. doi: 10.1371/journal.pone.0040312
29. André F, Ciruelos EM, Juric D, Loibl S, Campone M, Mayer IA, et al. Alpelisib Plus Fulvestrant for PIK3CA-Mutated, Hormone Receptor-Positive, Human Epidermal Growth Factor Receptor-2-Negative Advanced Breast Cancer: Final Overall Survival Results From SOLAR-1. Ann Oncol (2021) 32(2):208–17. doi: 10.1016/j.annonc.2020.11.011
30. Raglan O, Assi N, Nautiyal J, Lu H, Gabra H, Gunter MJ, et al. Proteomic Analysis of Malignant and Benign Endometrium According to Obesity and Insulin-Resistance Status Using Reverse Phase Protein Array. Trans Res (2020) 218:57–72. doi: 10.1016/j.trsl.2019.12.003
31. Berg HF, Ju Z, Myrvold M, Fasmer KE, Halle MK, Hoivik EA, et al. Development of Prediction Models for Lymph Node Metastasis in Endometrioid Endometrial Carcinoma. Br J Cancer (2020) 122(7):1014–22. doi: 10.1038/s41416-020-0745-6
32. Dai ZT, Wang J, Zhao K, Xiang Y, Li JP, Zhang HM, et al. Integrated TCGA and GEO Analysis Showed That SMAD7 Is an Independent Prognostic Factor for Lung Adenocarcinoma. Medicine (2020) 99(44):e22861. doi: 10.1097/MD.0000000000022861
33. Ngeow J, Yu W, Yehia L, Niazi F, Chen J, Tang X, et al. Exome Sequencing Reveals Germline SMAD9 Mutation That Reduces Phosphatase and Tensin Homolog Expression and is Associated With Hamartomatous Polyposis and Gastrointestinal Ganglioneuromas. Gastroenterology (2015) 149(4):886–9.e5. doi: 10.1053/j.gastro.2015.06.027
34. Gao L, Tian Q, Wu T, Shi S, Yin X, Liu L, et al. Reduction of MiR-744 Delivered by NSCLC Cell-Derived Extracellular Vesicles Upregulates SUV39H1 to Promote NSCLC Progression via Activation of the Smad9/BMP9 Axis. J Trans Med (2021) 19(1):37. doi: 10.1186/s12967-020-02654-9
35. Andrade DAP, da Silva VD, Matsushita GM, de Lima MA, Vieira MA, Andrade C, et al. Squamous Differentiation Portends Poor Prognosis in Low and Intermediate-Risk Endometrioid Endometrial Cancer. PloS One (2019) 14(10):e0220086. doi: 10.1371/journal.pone.0220086
36. Misirlioglu S, Guzel AB, Gulec UK, Gumurdulu D, Vardar MA. Prognostic Factors Determining Recurrence in Early-Stage Endometrial Cancer. Eur J Gynaecol Oncol (2012) 33(6):610–4.
37. Kaspers M, Llamocca E, Quick A, Dholakia J, Salani R, Felix AS. Black and Hispanic Women are Less Likely Than White Women to Receive Guideline-Concordant Endometrial Cancer Treatment. Am J Obstetrics Gynecol (2020) 223(3):398.e1–.e18. doi: 10.1016/j.ajog.2020.02.041
38. Leal LF, Evangelista AF, de Paula FE, Caravina Almeida G, Carloni AC, Saggioro F, et al. Reproducibility of the Nanostring 22-Gene Molecular Subgroup Assay for Improved Prognostic Prediction of Medulloblastoma. Neuropathol: Off J Jpn Soc Neuropathol (2018) 38(5):475–83. doi: 10.1111/neup.12508
39. Manion E, Cohen MB, Weydert J. Mandatory Second Opinion in Surgical Pathology Referral Material: Clinical Consequences of Major Disagreements. Am J Surg Pathol (2008) 32(5):732–7. doi: 10.1097/PAS.0b013e31815a04f5
Keywords: low- and intermediate-risk endometrioid endometrial carcinoma, genetic signature, recurrence risk score, biomarkers, Brazil
Citation: de Andrade DAP, da Silva LS, Laus AC, de Lima MA, Berardinelli GN, da Silva VD, Matsushita GdM, Bonatelli M, da Silva ALV, Evangelista AF, Carvalho JP, Reis RM and dos Reis R (2021) A 4-Gene Signature Associated With Recurrence in Low- and Intermediate-Risk Endometrial Cancer. Front. Oncol. 11:729219. doi: 10.3389/fonc.2021.729219
Received: 22 June 2021; Accepted: 30 July 2021;
Published: 17 August 2021.
Edited by:
Jian-Jun Wei, Northwestern University, United StatesReviewed by:
Enes Taylan, Mount Sinai Hospital, United StatesJulio de la Torre-Montero, Comillas Pontifical University, Spain
Copyright © 2021 de Andrade, da Silva, Laus, de Lima, Berardinelli, da Silva, Matsushita, Bonatelli, da Silva, Evangelista, Carvalho, Reis and dos Reis. This is an open-access article distributed under the terms of the Creative Commons Attribution License (CC BY). The use, distribution or reproduction in other forums is permitted, provided the original author(s) and the copyright owner(s) are credited and that the original publication in this journal is cited, in accordance with accepted academic practice. No use, distribution or reproduction is permitted which does not comply with these terms.
*Correspondence: Diocésio Alves Pinto de Andrade, ZGlvY2VzaW9AeWFob28uY29t